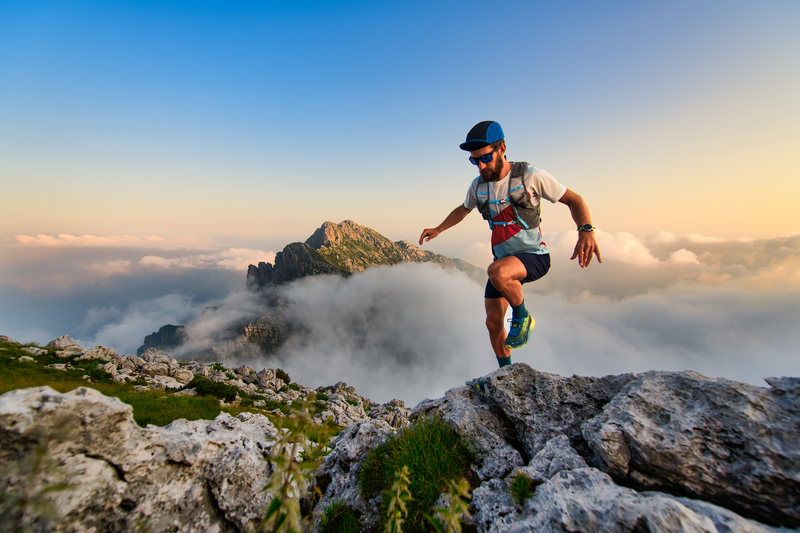
95% of researchers rate our articles as excellent or good
Learn more about the work of our research integrity team to safeguard the quality of each article we publish.
Find out more
MINI REVIEW article
Front. Environ. Sci. , 15 September 2022
Sec. Environmental Systems Engineering
Volume 10 - 2022 | https://doi.org/10.3389/fenvs.2022.924842
Microorganisms have evolved to inhabit virtually all environments on the planet, from oceanic hot-seeps to pipelines transporting crude and refined hydrocarbons. Often microbial colonization of man-made structures results in the reduction of their service life requiring preemptive or corrective human intervention. Microbiologically Influenced Corrosion (MIC) is caused by a set of intricate bioelectrochemical interactions between a diverse group of microorganisms and metallic surfaces. The complexity of MIC microbiomes and their mechanisms as well as the logistics constraints of industrial facilities are factors to consider when choosing suitable analytical methods for MIC monitoring. These generally reflect only a partial view of the phenomenon and in consequence, might lead to ineffective mitigation measures. This paper acknowledges the discrepancies between the fieldwork for MIC monitoring and the currently available technological advancements. It also highlights the most pressing issues that operators have in the field in light of the diversity of the microbial key players present in corrosive microbiomes. Finally, it compiles and outlines a strategy for the integration of novel molecular approaches aiming for a practical and accurate assessment of the microbial threat.
The wide metabolic potential and adaptation capabilities of microorganisms enable them to colonize virtually any man-made habitat at times with detrimental consequences. As particular microbes interact with building materials and other constituents, structural, mechanical and even aesthetic properties are often compromised. Microbiologically Influenced Corrosion (MIC) is considered to be a significant threat to mechanical integrity in industries such as water distribution, oil and gas production and processing, and many others (Guo et al., 2018; Jia et al., 2019; Stamps et al., 2020; Lavanya, 2021; Lou et al., 2021; Zaidi et al., 2021). The global cost of corrosion in 2015 was estimated to be ∼2.5 trillion dollars and has been continuously increasing (Koch et al., 2016; Ma et al., 2020). It has been calculated that approximately 20% of the cost of all failures caused by corrosion are consequence of microbial activity (Maxwell et al., 2004; Fatah et al., 2013; Sachan and Singh, 2020; Lavanya, 2021). Still, analysts argue that many MIC-related failures remain unreported due to limitations in field diagnostics and thus the cost of their environmental impact might also be underestimated (Brauer et al., 2015; Guo et al., 2018; Inaba et al., 2019; Jia et al., 2019; Liu et al., 2019; Little et al., 2020; Shi et al., 2020; Stamps et al., 2020; Chatterjee et al., 2021; Kleinbub et al., 2021; Lou et al., 2021; Omar et al., 2021; Zaidi et al., 2021).
MIC was first described over 100 years ago (Gaines, 1910). Since then, numerous studies have been dedicated to unraveling its microbial, biochemical and electrochemical mechanisms (Skovhus et al., 2017; Emerson, 2018; Jia et al., 2019; Lekbach et al., 2021). Although there is still plenty to be discovered, it is clear that microbes are most deleterious to a system or a process when attached to metallic surfaces while associated into biofilms. These are dense aggregations of microorganisms embedded in a self-produced matrix known as Extracellular Polymeric Substance (EPS) (Gordon et al., 2017; Procópio, 2019; Omar et al., 2021). As it is the case with sediments, in multispecies biofilms groups of physiologically diverse microbes tend to arrange in a stratified manner according to their redox preferences (Figure 1) (Beech and Sunner, 2004; Tripathi et al., 2021). In oxygenated environments, aerobic and facultative microorganisms tend to be found in the outer layers of the biofilm, whereas strict anaerobes are preferentially located in the inner layers where oxygen is absent for the most part. The rate of exchange of gasses and solutes between the biofilm and its surroundings is, in part, determined by the composition of the EPS, but mostly by diffusion or advection, depending on the dynamics of the system (Brauer et al., 2015; Rossy et al., 2019; Procópio, 2019; Van den Berg et al., 2021). While growing on metallic surfaces, biofilms can actively produce corrosive metabolites, consume directly or indirectly reducing equivalents (e.g., cathodic electrons), and/or passively generate concentration cells, thereby inducing or accelerating corrosion processes (Franklin et al., 2015; Dou et al., 2020; Muhammad et al., 2020; Tripathi et al., 2021).
FIGURE 1. Representation of a stratified multispecies biofilm at different stages of development on a corroding metal surface. Most relevant microbial groups are depicted in an idealized redox zonation from the oxidized outer layers to the more anaerobic inner core. Only the most representative half reactions are shown.
To reduce the impact of ubiquitous yet aggressive microorganisms and thereby preserve the mechanical integrity of their assets, field operators must implement MIC management strategies. The backbone of such strategies relies on the constant assessment of the microbial threat and thus reliable monitoring tools are required. Although, there is a wide variety of methods for microbiome characterization available to laboratory scientists, the conditions in often remote and complex industrial facilities limit their transfer to the industry. At the same time, over the years, several statistical models for predicting MIC have been developed, although with little integration into industrial practices (Sooknah et al., 2008; Taleb-Berrouane et al., 2018; Dawuda et al., 2021). This short review aims to highlight the ever present gap between research and industry, while providing field researchers and infrastructure operators with a short summary of available wet-lab techniques, and their pros and cons. Finally, it delivers a vision of the importance of performing field studies using multiple data collection techniques and compiles a roadmap for an applicable, but comprehensive and adjustable MIC monitoring strategy.
A wide group of microorganisms can initiate and/or accelerate corrosion processes. The list includes bacteria, archaea and, occasionally, fungi, and microalgae. While in the laboratory these organisms can be monitored using a myriad of specialized methods, in the industry field operators must find middle ground between costs, reliability and field applicability (Pannekens et al., 2019; Shi et al., 2020; Stamps et al., 2020; Lou et al., 2021; Omar et al., 2021). As a reference, a selection of methods that have been historically used and others that are receiving growing attention in the field are summarized in Figure 2.
FIGURE 2. Overview of alternatives for monitoring microbial corrosion. (A) Methods based on testing physiological features of potentially corrosive microbes (B); microscopy methods for evaluating biofilm formation and structure, determination of localized metal loss, metallography, etc., and (C) novel methods based on molecular biology for qualitative and quantitative analysis of corrosive microbiomes.
Traditionally, the assessment of the microbial threat has been limited to a handful of methodologies mostly dictated by international standards (NACE-International, 2014, 2016, 2018). These literature prompts the (semi-)quantification of a selection of microbial groups using principally synthetic culture media, which detect only a minor portion of the populations introducing biases to the assessment and the management programs. Typical industrial standards usually categorize parameters and integrity threats into severity levels aiming to preserve infrastructure integrity (Ebrahimi, 2015). These indicators, known as Key Performance Indicators (KPI), are not existent in MIC technical standards. That is to say, they do not suggest maximum tolerable concentrations for potentially corrosive microorganisms in industrial systems. This lack of KPIs in the field is not incidental, but rather a consequence of the fact that thus far it has not been possible to establish a direct correlation between the type and concentration of microorganisms neither planktonic nor sessile and MIC kinetics (Seyeux et al., 2014; Rapp, 2018; Telegdi et al., 2018; Koerdt, 2021). The former is true for any culture- or molecular-based method used in the field (Eckert and Skovhus, 2018). Hence, the detection of potentially aggressive microbes in a system must be regarded as an indication that the probability of failure is higher than when microbes are absent. Still, given the need for microbial KPIs in the praxis, companies internally define their own, most if not all using culture-based methods. Once defined by a major company, KPI values often replicate across the industry and are used to assess the efficacy of mitigation programs (El-Sherik, 2017). Typical empirical KPIs used by field operators in the Oil and Gas sector can range between 1 and 10,000 bacteria per milliliter or cm2, being in most cases 100 bacteria per ml or cm2 the maximum tolerable concentration for cultivable sulfate reducers (Al-Shamari et al., 2013). Yet, ideally, the definition of microbial KPIs should be made in a case-by-case basis, taking into account trends of the microbiological, physicochemical and operational context, rather than adopting a generic figure (NACE-International, 2018). Understanding this is pivotal since although in the laboratory multiple reports show microorganisms and even single strains as direct causes of corrosion, in the field MIC is a complex process and usually acting along with other (sometimes pre-existing) corrosion mechanisms such as dissolved gasses, under-deposit corrosion, crevice corrosion, etc. (Li et al., 2018; Ishii and Wakai, 2020).
The broad microbial diversity associated to MIC represents a challenge for an accurate field diagnosis. Due to the highly specialized life style of most such microbes, they are either poorly detected using conventional detection methods or are out of scope of routine monitoring altogether. In fact, MIC monitoring has been simplified in such a way that the phylogeny and taxonomy of corrosion-associated microorganisms have been set aside and replaced by grouping them on the basis of physiological traits (Okoro and Amund, 2015; Loto, 2017; Permeh et al., 2017; Skovhus et al., 2017; Lekbach et al., 2021; Bennet, 2022). Among these are the Sulfate-Reducing Bacteria (SRB), the most reported corrosion-related microbes (Supplementary Table S1), known for the production of the highly toxic and corrosive hydrogen sulfide. SRB are part of a wider group known as Sulfate-Reducing Prokaryotes (SRP), a polyphyletic group that encompasses microorganisms from the domains of Bacteria and Archaea, and possesses a relatively conserved set of genes that enable them to perform the dissimilatory reduction of sulfate (Tian et al., 2017; Enzien et al., 2021). Despite their commonalities, only a few representatives from bacterial genera such as Desulfovibrio and Desulfotomaculum are recovered and semi-quantified in culture media used in the field, compromising the accuracy of the assessment (Zhang et al., 2016; Li et al., 2017; Tian et al., 2017; Al-Sultani et al., 2021; Chatterjee et al., 2021). The second most reported physiological category in corrosion monitoring are the Acid Producing Bacteria (APB) (Supplementary Table S1) within what can be regarded as Acid-Producing Prokaryotes (APP). In general terms, APP includes microorganisms that have the potential to excrete organic and inorganic acids using heterotrophic or lithoautotrophic metabolic pathways (Telegdi et al., 2018). This grouping is much fuzzier than SRP, since there is not a straightforward match between physiology and a unique genetic arsenal to produce acids, rendering molecular biology methods such as qPCR or sequencing approaches of little value for their detection. Hence, what in the praxis can be classified as APP or APB is reduced to the microbes detected using complex growth media supplemented with fermentable substrates (mostly sugars), as indicators for the potential of the microbiome to acidify its environment. Most of these substrates are not common in such habitats, skewing the APP assessment to a portion of fermenters, not necessarily to the most abundant, nor the most active (Williamson et al., 2015; Okoro et al., 2016; Salgar-Chaparro et al., 2020). The use of transcriptomics or metabolomics approaches for resolving some physiological features of the microbiomes have shown promising results in the laboratory; however, their use in routine monitoring is still challenging (Beech et al., 2014; Bonifay et al., 2017; Wang et al., 2021).
Beyond SRP and APP, other populations known to play important roles in metal corrosion in the field include Sulfur-Oxidizing Prokaryotes (SOP), Iron-Reducing Prokaryotes (IRP), Iron-Oxidizing Prokaryotes (IOP), Non-SRP Sulfide-Producing Prokaryotes (SPP) and Methanogenic Archaea (MA), among others (Figure 1) (Liu et al., 2019; Kleinbub et al., 2021; Zaidi et al., 2021). In the praxis, monitoring many microbial groups is unfeasible and therefore it is important to establish a handful of microbes that can be routinely monitored, in a sense, acting as proxy for the overall microbial threat. Although one can argue that in the last decades such a role has been fulfilled by cultivable SRB and APB (Sowards et al., 2014; Okoro and Amund, 2015; Permeh et al., 2017; Senthilmurugan et al., 2021), the recent technological advances have brought to light the need to rethink both the microorganisms being monitored and the way they are monitored (Mukherjee et al., 2017; Koerdt, 2021; McDaniel et al., 2021; Pilloni et al., 2022).
Biochemical methods for MIC monitoring including culture-based techniques have been widespread in the industry for decades; while, culture-independent methods have emerged just recently as feasible alternatives (Figure 2, Supplementary Table S2). Electrochemistry has also been suggested to monitor MIC in the field (Pavanello et al., 2011; Cristiani and Perboni, 2014). However, the existing technical possibilities are typically limited to relatively clean water systems which is barely the case in most situations particularly in oil and gas. Electrochemical techniques can detect corrosion reactions and might in a general way provide an indication of the onset of a corrosion process. However, to date electrochemistry cannot distinguish MIC from abiotic corrosion (Little et al., 2020). In consequence, they need to be accompanied by biological data to distinguish whether the process has a biological origin (Little and Wagner, 2002), making them not informative enough for most field industrial conditions.
A literature search from the last 12 years found 52 manuscripts which focused exclusively on the characterization of the MIC microbiome in industrial samples (Supplementary Table S1). In 39 of the manuscripts (75%) molecular biology approaches were used often in combination with other techniques, whereas in only 5 papers culture media were used as the sole detection method. This points out the current level of penetration of state-of-the-art technology in published cases. In addition, in 96% of the surveyed cases, SRP were the main focus of analysis (Supplementary Table S1), being culture media the most prevalent analytical tool for their detection (29 reports), followed by molecular biology methods (22). On the other hand, despite being considered highly significant in MIC, acid producers and methanogens are mentioned only in 38% of the manuscripts, showing that even among scientists, there is a tendency to overlook microbes other than SRP while studying corrosion. Other groups such as General Heterotrophic Bacteria (GHB) are covered in 15% of the publications; while others with highly corrosive metabolic potential, i.e., SOP, IRP, IOP, Thiosulfate-Reducing Prokaryotes (TRP), and Manganese-Oxidizing Bacteria (MOB) are analyzed in less than 10% of the reviewed papers. In terms of sample type, strikingly over 67% of the manuscripts used the aqueous phase over solids or surface swabs, exhibiting a bias towards the planktonic component of the microbiomes (Supplementary Table S1). The remaining 33% of the reports did evaluate solid samples, though with a scarce 11% of them concentrated explicitly on the characterization of biofilms. Unfortunately, this reflects the practices in the field where most efforts lean towards the analysis of free-living bacteria (Stevenson et al., 2011; Enzien et al., 2021), even though it is well known that MIC is mostly a consequence of biofilm formation (Figure 1) (Loto, 2017; Verderosa et al., 2019; Lekbach et al., 2021).
Monitoring variables that require specific technology and training is challenging in most industrial settings (Skovhus et al., 2017). This is particularly true for infrastructure prone to MIC such as hydrocarbon-producing and -processing facilities, which are commonly located in remote regions. Adding another layer of complexity are essential aspects such as the representativeness of the sample, the collection and preservation methods, the techniques used for microbiome characterization, and the correlation with other relevant variables (e.g., history of MIC failures and the physicochemistry of the work matrix) (Albahri et al., 2021; Chatterjee et al., 2021; Jack, 2021). The introduction of biases at any stage of the process can lead to the over- or underestimation of MIC (Papavinasam, 2014; Rodrigues and Akid, 2014; Liduino et al., 2019; Salgar-Chaparro et al., 2020). To avoid this, a strategy for MIC management containing the three major elements can be implemented: 1) the construction of an informative baseline of microbiological information at several stages of the production process, 2) the design and implementation of MIC monitoring routines and 3) eventually the application of mitigation plans. Figure 3 summarizes some key aspects that in this regard are commonly suggested by both industry standards and scientific literature.
As technology becomes ever more available, an ideal baseline characterization of the biological threat should contain a qualitative and a quantitative assessment of the planktonic and sessile populations using New Generation Sequencing techniques and quantitative PCR, respectively. The former refers to the use of tools such as shotgun metagenomics, metatranscriptomics or 16S rRNA-based metagenomics, also referred to as metataxonomics, to qualitatively analyze microbial community compositions (Marchesi and Ravel, 2015; Pal et al., 2019; Krohn et al., 2021; McDaniel et al., 2021). Since unlike microbial cultures such molecular biology methods do not rely on microbial growth but rather on the detection of microbial components or products, they can reflect more accurately the composition of field microbiomes (Larsen et al., 2013; Eckert and Skovhus, 2018). In the praxis, metataxonomics is most convenient to industrial applications, due to its lower complexity and costs. However, in order to reduce biases while using it the PCR primers for 16S rRNA amplification must be carefully picked. A widely accepted and cost-efficient option for surveying total prokaryotes (Bacteria and Archaea) in a single sequencing run implies the use of universal PCR primers such as those recommended by the Earth Microbiome Project (Gittel et al., 2014; Apprill et al., 2015; Parada et al., 2016; Caporaso et al., 2018; Wasimuddin et al., 2020; Skipper et al., 2022). The steady reduction in sequencing costs and constant improvement in sequencing chemistries facilitate the outsourcing of the technology, being the data processing the highest constrain for their implementation (Supplementary Table S2). Recently, the portability and evolving read accuracy of nanopore technology presents itself as a compelling alternative for industrial applications (Feng et al., 2015; Grottoli et al., 2020). To facilitate data interpretation and integration with historical and monitoring records, the relative abundances of bacterial and archaeal taxa obtained after sequencing can be organized into clusters based on their predicted metabolic activity relevant to corrosion e.g., sulfate reducers, methanogens, iron reducers/oxidizers, etc. In terms of absolute quantification by qPCR, the use of the same nucleic acids extraction protocols and 16S primer pairs used for sequencing is recommended for consistency and bias reduction. A more robust characterization can include the use of functional marker genes for additional quantification of discrete physiological groups such as sulfate reducers (apsA, dsrA genes) and methanogenic archaea (mcrA) (Ben-Dov et al., 2007; Schippers and Blazejak, 2011; McKay et al., 2017; Senthilmurugan et al., 2021). The integration of relative and absolute abundances of the different taxonomic levels and physiological groups allows to identify and pinpoint the most relevant populations targeted for mitigation plans as well as for routine monitoring. The use of RNA-based methods such as metatranscriptomics and RT-qPCR have also shown to be useful for discriminating the active portions of the microbiomes during MIC monitoring, although implying more meticulous sample handling and processing (Carvalhais et al., 2012; Thomsen and Oehler, 2018; Shakya et al., 2019). These are particularly advantageous where population dynamics are being examined, for example measuring the efficacy of biocide treatment. Additional omics technologies, such as metabolomics or metaproteomics, might also be used to complement baseline generation or when a deeper understanding is desired or needed, e.g., during failure analysis, clogging, or product contamination. (Brauer et al., 2015; Beale et al., 2016; Bonifay et al., 2017; Gutleben et al., 2018; Little et al., 2020; Procopio, 2020; Kumar et al., 2021; McDaniel et al., 2021; Harik et al., 2022; Malik et al., 2022). This baseline generation can be implemented as a cycle, where the metagenomic characterization is iterated for instance every 6 months to 2 years, according to the dynamics of each system. The latter in order to monitor whether major changes in the microbiomes have emerged. If so, adjustments in the monitoring and mitigation plans can be implemented accordingly.
Once the microbiomes have been profiled, a less generic MIC monitoring strategy can be tailored for each particular system (Figure 2, Supplementary Table S3). For instance, the detection of microbial agents in sampling sites showing hardly to non-cultivable microorganisms such as sulfate reducing or methanogenic archaea should involve the use of universal and locus-specific qPCR or RT-qPCR (Figure 3). However, whenever inconvenient due to logistics, budget, location, etc., optimized conventional methods such as modified culture media can be an alternative. The modifications may include the use of (biocide-free) produced water for media preparation, the replacement of complex organic nitrogen sources for ammonium salts and the addition of alternative or a mixture of carbon sources, among others (Ghazy et al., 2011; Zamora, 2012). Although still introducing biases, the use of rationally modified culture media can to some extent widen the spectrum of detected microbes. On the other hand, although the baseline characterization may reveal that a considerable portion of the microbiomes cannot be considered corrosive, these can include organisms with biofilm formation capability (Dou et al., 2021). Hence a sporadic screening for total prokaryotes or general heterotrophs is recommended, particularly when biocide treatments are being applied (Figure 3). As mentioned above, it is essential to monitor both the planktonic and the sessile portions of the microbiomes. Although less accessible, the latter can be obtained from deposits, solids from internal cleaning or surface swabs from corrosion coupons or probes when handled properly and aseptically (Franklin et al., 2015; Jia et al., 2019; Marciales et al., 2019).
The generation and fine-tuning of MIC management plans the results of any MIC monitoring must be confronted with additional evidence such as the chemistry of the matrix, the operational conditions of each sampling point, history of bacterial contamination, the presence of solids, tubercles and/or pits on metallic surfaces and corrosion coupons, etc., (Figure 3). Unluckily, MIC modelling algorithms combining operational and environmental variables with microbial data are still to be developed. Until then, the full potential of novel technologies won’t be exploited in full in the field and operators will have to continue making decisions based on empirical concepts (Eckert and Skovhus, 2018; Little et al., 2020).
GC-V and EP-C participated in the outline planning of the manuscript. VT-P, DE-V, GC-V, MR-R, DQ-S, PS-G, HS, and SV-D participated in data collection. DE-V, EP-C, MR, and DQ-S participated in figure design. DE-V, VT-P, MR-R, and PS-G contributed with tables writing and editing. EP-C, GC-V, VT-P, DE-V, and RM contributed with writing and editing. GC-V, EP-C, DQ-S, and RS-R contributed with project planning and execution. RS-R, SV-D, HS, and DQ-S participated in the final editing. All authors contributed to the article and approved the submitted version.
This work was funded by the MINCIENCIAS-COLOMBIA-BIO grant number (80740-532-2020) and for the initiative productividad para las empresas, in the frame of the joint call SENA—Colombia Productiva, 2020.
The authors would like to thank Luz Dary Galindo for all her help in the preparation of the figures. Special thanks also to the students Luis Moliner and Hugo Colmenares for their assistance in the project activities. Finally, the authors would like to thank engineers Pilar Pinto and Yohan Prada for their help in the administrative tasks associated with the preparation of this document.
The authors declare that the research was conducted in the absence of any commercial or financial relationships that could be construed as a potential conflict of interest.
All claims expressed in this article are solely those of the authors and do not necessarily represent those of their affiliated organizations, or those of the publisher, the editors and the reviewers. Any product that may be evaluated in this article, or claim that may be made by its manufacturer, is not guaranteed or endorsed by the publisher.
The Supplementary Material for this article can be found online at: https://www.frontiersin.org/articles/10.3389/fenvs.2022.924842/full#supplementary-material
Albahri, M. B., Barifcani, A., Iglauer, S., Lebedev, M., O’Neil, C., Salgar-Chaparro, S. J., et al. (2021). Investigating the mechanism of microbiologically influenced corrosion of carbon steel using X-ray micro-computed tomography. J. Mater. Sci. 56, 13337–13371. doi:10.1007/s10853-021-06112-9 |
Al-Shamari, A. R., Al-Mithin, A. W., Olabisi, O., and Mathew, A. (2013). “Developing a metric for microbilogically influenced corrosion (MIC) in oilfield water handling systems,” in NACE International Conference, Corrosion 2013, Orlando, Florida. NACE-2013-2299. doi:10.13140/2.1.3234.1446 |
Al-Sultani, K. F., Khulief, Z. T., and Hasan, A. A. (2021). Characterization of microbiological influence corrosion for API 5L X46 pipeline by sulphate-reducing bacteria (SRB). Mater. Today Proc. 42, 2169–2176. doi:10.1016/j.matpr.2020.12.301 |
Apprill, A., McNally, S., Parsons, R., and Weber, L. (2015). Minor revision to V4 region SSU rRNA 806R gene primer greatly increases detection of SAR11 bacterioplankton. Aquat. Microb. Ecol. 75, 129–137. Available at: https://www.int-res.com/abstracts/ame/v75/n2/p129-137/. doi:10.3354/ame01753 |
Beale, D. J., Karpe, A. V., Jadhav, S., Muster, T. H., and Palombo, E. A. (2016). Omics-based approaches and their use in the assessment of microbial-influenced corrosion of metals. Corros. Rev. 34, 1–15. doi:10.1515/corrrev-2015-0046 |
Beech, I. B., and Sunner, J. (2004). Biocorrosion: towards understanding interactions between biofilms and metals. Curr. Opin. Biotechnol. 15, 181–186. doi:10.1016/j.copbio.2004.05.001 | |
Beech, I. B., Sztyler, M., Gaylarde, C. C., Smith, W. L., and Sunner, J. (2014). “Biofilms and biocorrosion,” in Understanding biocorrosion: Fundamentals and applications, 33–50. |
Ben-Dov, E., Brenner, A., and Kushmaro, A. (2007). Quantification of sulfate-reducing bacteria in industrial wastewater, by real-time polymerase chain reaction (PCR) using dsrA and apsA genes. Microb. Ecol. 54, 439–451. doi:10.1007/s00248-007-9233-2 | |
Bennet, D. G. (2022). “Oilfield microbiology: Case study of molecular techniques for determining the risk of microbiologically influenced corrosion MIC,” in Paper presented at the Offshore Technology Conference Asia, D031S030R004. doi:10.4043/31498-MS |
Bonifay, V., Wawrik, B., Sunner, J., Snodgrass, E. C., Aydin, E., Duncan, K. E., et al. (2017). Metabolomic and metagenomic analysis of two crude oil production pipelines experiencing differential rates of corrosion. Front. Microbiol. 8, 99. doi:10.3389/fmicb.2017.00099 | |
Brauer, J., Makama, Z., Bonifay, V., Aydin, E., Kaufman, E., Beech, I., et al. (2015). Mass spectrometric metabolomic imaging of biofilms on corroding steel surfaces using laser ablation and solvent capture by aspiration. Biointerphases 10, 019003. doi:10.1116/1.4906744 | |
Caporaso, J. G., Ackermann, G., Apprill, A., Bauer, M., Berg-Lyons, D., Betley, J., et al. (2018). EMP 16S Illumina Amplicon Protocol. Protocols.io. Available at: https://www.protocols.io/view/emp-16s-illumina-amplicon-protocol-kqdg3dzzl25z/v1.
Carvalhais, L., Dennis, P., Tyson, G., and Schenk, P. (2012). Application of metatranscriptomics to soil environments. J. Microbiol. Methods 91, 246–251. doi:10.1016/j.mimet.2012.08.011 | |
Chatterjee, M., Fan, Y., Cao, F., Jones, A. A., Pilloni, G., Zhang, X., et al. (2021). Proteomic study of Desulfovibrio ferrophilus IS5 reveals overexpressed extracellular multi-heme cytochrome associated with severe microbiologically influenced corrosion. Sci. Rep. 11, 15458. doi:10.1038/s41598-021-95060-0 | |
Cristiani, P., and Perboni, G. (2014). Antifouling strategies and corrosion control in cooling circuits. Bioelectrochemistry 97, 120–126. doi:10.1016/j.bioelechem.2014.01.002 | |
Dawuda, A.-W., Taleb-berrouane, M., and Khan, F. (2021). A probabilistic model to estimate microbiologically influenced corrosion rate. Process Saf. Environ. Prot. 148, 908–926. doi:10.1016/j.psep.2021.02.006 |
Dou, W., Xu, D., and Gu, T. (2020). Biocorrosion caused by microbial biofilms is ubiquitous around us. Microb. Biotechnol. 14, 803–805. doi:10.1111/1751-7915.13690 | |
Dou, W., Xu, D., and Gu, T. (2021). Biocorrosion caused by microbial biofilms is ubiquitous around us. Microb. Biotechnol. 14, 803–805. doi:10.1111/1751-7915.13690 | |
Ebrahimi, N. (2015). Implementation of 50 Key Performance Indicators (KPIs) in Corrosion Control of an Oil Transmission Pipeline. STEM-Corrosion, 1–6. Available at: https://corrmagnet.com/journal/papers/.
Eckert, R. B., and Skovhus, T. L. (2018). Advances in the application of molecular microbiological methods in the oil and gas industry and links to microbiologically influenced corrosion. Int. Biodeterior. Biodegrad. 126, 169–176. doi:10.1016/j.ibiod.2016.11.019 |
El-Sherik, A. M. (2017). “Corrosion in oil and gas production,” in Trends in oil and gas corrosion research and technologies: Production and transmission. Editor A. M. El-Sherik, 548.
Emerson, D. (2018). The role of iron-oxidizing bacteria in biocorrosion: a review. Biofouling 34, 989–1000. doi:10.1080/08927014.2018.1526281 | |
Enzien, M., Starustka, S., Gurecki, M., Fincher-Miller, T., Kuhn, B., Sowecke, C., et al. (2021). “Metagenomics microbial characterization of production and process fluids in the powder river basin: Identification and sources of problematic microorganisms associated with SWD facilities,” in Paper presented at the SPE International Conference on Oilfield Chemistry. doi:10.2118/204335-MS |
Fatah, M. C., Ismail, M. C., and Wahjoedi, B. A. (2013). Effects of sulphide ion on corrosion behaviour of X52 steel in simulated solution containing metabolic products species: a study pertaining to microbiologically influenced corrosion (MIC). Corros. Eng. Sci. Technol. 48, 211–220. doi:10.1179/1743278212Y.0000000065 |
Feng, Y., Zhang, Y., Ying, C., Wang, D., and Du, C. (2015). Nanopore-based fourth-generation DNA sequencing technology. Genomics Proteomics Bioinforma. 13, 4–16. doi:10.1016/j.gpb.2015.01.009 | |
Franklin, M. J., Chang, C., Akiyama, T., and Bothner, B. (2015). New technologies for studying biofilms. Microbiol. Spectr. 3, 1–23. doi:10.1128/microbiolspec.MB-0016-2014 |
Gaines, R. H. (1910). Bacterial activity as a corrosive influence in the soil. J. Ind. Eng. Chem. 2, 128–130. doi:10.1021/ie50016a003 |
Ghazy, E., Mahmoud, M., Asker, M., Mahmoud, M. N., Abo Elsoud, M., and Sami, M. E. (2011). Cultivation and detection of sulfate reducing bacteria (SRB) in sea water. J. Am. Sci. 7, 604–608.
Gittel, A., Bárta, J., Kohoutová, I., Mikutta, R., Owens, S., Gilbert, J., et al. (2014). Distinct microbial communities associated with buried soils in the Siberian tundra. ISME J. 8, 841–853. doi:10.1038/ismej.2013.219 | |
Gordon, V. D., Davis-Fields, M., Kovach, K., and Rodesney, C. A. (2017). Biofilms and mechanics: a review of experimental techniques and findings. J. Phys. D. Appl. Phys. 50, 223002. doi:10.1088/1361-6463/aa6b83 |
Grottoli, A., Beccaccioli, M., Zoppis, E., Fratini, R. S., Schifano, E., Santarelli, M. L., et al. (2020). Nanopore sequencing and bioinformatics for rapidly identifying cultural heritage spoilage microorganisms. Front. Mater. 7, 1–11. doi:10.3389/fmats.2020.00014 |
Guo, J., Yuan, S., Jiang, W., Lv, L., Liang, B., Pehkonen, S. O., et al. (2018). Polymers for combating biocorrosion. Front. Mater. 5. doi:10.3389/fmats.2018.00010 |
Gutleben, J., Chaib De Mares, M., van Elsas, J. D., Smidt, H., Overmann, J., Sipkema, D., et al. (2018). The multi-omics promise in context: from sequence to microbial isolate. Crit. Rev. Microbiol. 44, 212–229. doi:10.1080/1040841X.2017.1332003 | |
Harik, A.-M. G., Griffiths, Z., and Hazen, T. C. (2022). Omics of oil biodegradation. Curr. Opin. Chem. Eng. 36, 100800. doi:10.1016/j.coche.2022.100800 |
Inaba, Y., Xu, S., Vardner, J. T., West, A. C., Banta, S., and Robert, K. M. (2019). Microbially influenced corrosion of stainless steel by Acidithiobacillus ferrooxidans supplemented with pyrite: Importance of thiosulfate. Appl. Environ. Microbiol. 85, e01381-19. doi:10.1128/AEM.01381-19 | |
Ishii, M., and Wakai, S. (2020). Electron-based bioscience and biotechnology. 1st ed. Singapore: Springer Nature Singapore. doi:10.1007/978-981-15-4763-8 |
Jack, T. R. (2021). Biological corrosion failures. Fail. Anal. Prev. 11, 615–636. doi:10.31399/asm.hb.v11.a0006788 |
Jia, R., Unsal, T., Xu, D., Lekbach, Y., and Gu, T. (2019). Microbiologically influenced corrosion and current mitigation strategies: A state of the art review. Int. Biodeterior. Biodegrad. 137, 42–58. doi:10.1016/j.ibiod.2018.11.007 |
Kleinbub, S., An-Stepec, A. B., and Koerdt, A. (2021). “Iron to gas: The mechanisms behind methanogen-induced microbiologically influenced corrosion (Mi-MIC) and their importance for the industry and infrastructure,” in Failure analysis of microbiologically influenced corrosion, 1–18. Available at: https://www.taylorfrancis.com/chapters/edit/10.1201/9780429355479-7/iron-gas-mechanisms-behind-methanogen-induced-microbiologically-influenced-corrosion-mi-mic-importance-industry-infrastructure-sherin-kleinbub-annie-biwen-stepec-andrea-koerdt. |
Koch, G., Varney, J., Thompson, N., Moghissi, O., Gould, M., and Payer, J. (2016). International measures of prevention , application , and economics of corrosion technologies study. Houston. Available at: http://impact.nace.org/economic-impact.aspx.
Koerdt, A. (2021). Mikrobiell beeinflusste korrosion — die testungsart entscheidet. BIOspektrum 27, 100. doi:10.1007/s12268-021-1507-7 |
Krohn, I., Bergmann, L., Qi, M., Indenbirken, D., Han, Y., Perez-Garcia, P., et al. (2021). Deep (Meta)genomics and (Meta)transcriptome analyses of fungal and bacteria consortia from aircraft tanks and kerosene identify key genes in fuel and tank corrosion. Front. Microbiol. 12, 722259. doi:10.3389/fmicb.2021.722259 | |
Kumar, V., Singh, K., Shah, M. P., Singh, A. K., Kumar, A., and Kumar, Y. (2021). “Chapter 1 - application of omics technologies for microbial community structure and function analysis in contaminated environment,” in Wastewater treatment. Editors M. P. Shah, and A. Sarkar (Elsevier), 1–40. doi:10.1016/B978-0-12-821881-5.00001-5 |
Larsen, J., Sørense, K. B., Juhler, S., and Pedersen, D. S. (2013). “The application of molecular microbiological methods for early warning of MIC in pipelines,” in Paper presented at the CORROSION 2013. NACE-2013-2029.
Lavanya, M. (2021). A brief insight into microbial corrosion and its mitigation with eco-friendly inhibitors. J. Bio. Tribocorros. 7, 125. doi:10.1007/s40735-021-00563-y |
Lekbach, Y., Liu, T., Li, Y., Moradi, M., Dou, W., Xu, D., et al. (2021). “Chapter Five - microbial corrosion of metals: The corrosion microbiome,” in Advances in microbial physiology. Editors R. K. Poole, and M. P. Kelly (Academic Press), 317–390. doi:10.1016/bs.ampbs.2021.01.002 | |
Li, X., Duan, J., Xiao, H., Li, Y., Liu, H., Guan, F., et al. (2017). Analysis of bacterial community composition of corroded steel immersed in sanya and xiamen seawaters in China via method of illumina MiSeq sequencing. Front. Microbiol. 8, 1737. doi:10.3389/fmicb.2017.01737 | |
Li, Y., Xu, D., Chen, C., Li, X., Jia, R., Zhang, D., et al. (2018). Anaerobic microbiologically influenced corrosion mechanisms interpreted using bioenergetics and bioelectrochemistry: A review. J. Mater. Sci. Technol. 34, 1713–1718. doi:10.1016/j.jmst.2018.02.023 |
Liduino, V. S., Cravo-Laureau, C., Noel, C., Carbon, A., Duran, R., Lutterbach, M. T., et al. (2019). Comparison of flow regimes on biocorrosion of steel pipe weldments: Community composition and diversity of biofilms. Int. Biodeterior. Biodegrad. 143, 104717. doi:10.1016/j.ibiod.2019.104717 |
Little, B. J., and Wagner, P. A. (2002). “Application of electrochemical techniques to the study of microbiologically influenced corrosion BT,” in modern aspects of electrochemistry. Editors J. O. Bockris, B. E. Conway, and R. E. White (Boston, MA: Springer US), 205–246. doi:10.1007/0-306-46923-5_5 |
Little, B. J., Blackwood, D. J., Hinks, J., Lauro, F. M., Marsili, E., Okamoto, A., et al. (2020). Microbially influenced corrosion—any progress? Corros. Sci. 170, 108641. doi:10.1016/j.corsci.2020.108641 |
Liu, H., Meng, G., Li, W., Gu, T., and Liu, H. (2019). Microbiologically influenced corrosion of carbon steel beneath a deposit in CO2-saturated formation water containing Desulfotomaculum nigrificans. Front. Microbiol. 10, 1298. doi:10.3389/fmicb.2019.01298 | |
Loto, C. A. (2017). Microbiological corrosion: mechanism, control and impact—a review. Int. J. Adv. Manuf. Technol. 92, 4241–4252. doi:10.1007/s00170-017-0494-8 |
Lou, Y., Chang, W., Cui, T., Qian, H., Huang, L., Ma, L., et al. (2021). Microbiologically influenced corrosion inhibition of carbon steel via biomineralization induced by Shewanella putrefaciens. npj Mater. Degrad. 5, 59. doi:10.1038/s41529-021-00206-0 |
Ma, L., Ren, C., Wang, J., Liu, T., Yang, H., Wang, Y., et al. (2020). Self-reporting coatings for autonomous detection of coating damage and metal corrosion : A review. Chem. Eng. J. 421, 127854. doi:10.1016/j.cej.2020.127854 |
Malik, G., Arora, R., Chaturvedi, R., and Paul, M. S. (2022). Implementation of genetic engineering and novel omics approaches to enhance bioremediation: A focused review. Bull. Environ. Contam. Toxicol. 108, 443–450. doi:10.1007/s00128-021-03218-3 | |
Marchesi, J. R., and Ravel, J. (2015). The vocabulary of microbiome research: a proposal. Microbiome 3, 31. doi:10.1186/s40168-015-0094-5 | |
Marciales, A., Peralta, Y., Haile, T., Crosby, T., and Wolodko, J. (2019). Mechanistic microbiologically influenced corrosion modeling—A review. Corros. Sci. 146, 99–111. doi:10.1016/j.corsci.2018.10.004 |
Maxwell, S., Devine, C., and Rooney, F. (2004). “Monitoring and control of bacterial biofilms in oilfield water handling systems,” in Paper presented at the CORROSION 2004. NACE-04752.
McDaniel, E. A., Wahl, S. A., Ishii, S., Pinto, A., Ziels, R., Nielsen, P. H., et al. (2021). Prospects for multi-omics in the microbial ecology of water engineering. Water Res. 205, 117608. doi:10.1016/j.watres.2021.117608 | |
McKay, L. J., Hatzenpichler, R., Inskeep, W. P., and Fields, M. W. (2017). Occurrence and expression of novel methyl-coenzyme M reductase gene (mcrA) variants in hot spring sediments. Sci. Rep. 7, 7252. doi:10.1038/s41598-017-07354-x | |
Muhammad, M. H., Idris, A. L., Fan, X., Guo, Y., Yu, Y., Jin, X., et al. (2020). Beyond risk: Bacterial biofilms and their regulating approaches. Front. Microbiol. 11, 928. doi:10.3389/fmicb.2020.00928 | |
Mukherjee, A., Chettri, B., Langpoklakpam, J. S., Basak, P., Prasad, A., Mukherjee, A. K., et al. (2017). Bioinformatic approaches including predictive metagenomic profiling reveal characteristics of bacterial response to petroleum hydrocarbon contamination in diverse environments. Sci. Rep. 7, 1108. doi:10.1038/s41598-017-01126-3 | |
NACE-International (2014). TM0194—Field Monitoring of Bacterial Growth in Oil and Gas Systems. Houston TX: NACE International, 1–32.
NACE-International (2016). TM0106—Detection, Testing, and Evaluation of Microbiologically Influenced Corrosion (MIC) on External Surfaces of Buried Pipelines. Houston TX: NACE International, 1–36.
NACE-International (2018). TM0212—Detection, Testing, and Evaluation of Microbiologically Influenced Corrosion on Internal Surfaces of Pipelines. Houston TX: NACE International, 1–52.
Okoro, C. C., and Amund, O. (2015). Souring and corrosion potentials of onshore and offshore oil-producing facilities in the Nigerian oil-rich Niger delta. Pet. Sci. Technol. 33, 1563–1570. doi:10.1080/10916466.2015.1072559 |
Okoro, C., Ekun, O. A., Nwume, M. I., and Lin, J. (2016). Molecular analysis of microbial community structures in Nigerian oil production and processing facilities in order to access souring corrosion and methanogenesis. Corros. Sci. 103, 242–254. doi:10.1016/j.corsci.2015.11.024 |
Omar, S. H., Khalil, N. E. S., El-Ahwany, A. M. D., El-Sayed, H. A. E. F., and Arafat, S. O. Y. (2021). Mitigation of microbiologically induced corrosion (MIC) and preventive strategies. Res. Trends Microbiol. MedDocs Publ. 3, 1–11.
Pal, S., Roy, A., and Kazy, S. K. (2019). “Chapter 11 - exploring microbial diversity and function in petroleum hydrocarbon associated environments through omics approaches,” in Microbial Diversity in the Genomic Era. Editors S. Das, and H. Dash (Academic Press), 171–194. doi:10.1016/B978-0-12-814849-5.00011-3 |
Pannekens, M., Kroll, L., Müller, H., Mbow, F. T., and Meckenstock, R. U. (2019). Oil reservoirs, an exceptional habitat for microorganisms. N. Biotechnol. 49, 1–9. doi:10.1016/j.nbt.2018.11.006 | |
Papavinasam, S. (2014). Chapter 14 - management. Editors G. I. Papavinasam (Boston: Gulf Professional Publishing), 841–918. doi:10.1016/B978-0-12-397022-0.00014-5 |
Parada, A. E., Needham, D. M., and Fuhrman, J. A. (2016). Every base matters: assessing small subunit rRNA primers for marine microbiomes with mock communities, time series and global field samples. Environ. Microbiol. 18, 1403–1414. doi:10.1111/1462-2920.13023 | |
Pavanello, G., Faimali, M., Pittore, M., Mollica, A., Mollica, A., Mollica, A., et al. (2011). Exploiting a new electrochemical sensor for biofilm monitoring and water treatment optimization. Water Res. 45, 1651–1658. doi:10.1016/j.watres.2010.12.003 | |
Permeh, S., Reid, C., Boan, M., Lau, K., Tansel, B., Duncan, M., et al. (2017). “Microbiological influenced corrosion (MIC) in Florida marine environment: A case study,” in Corros 2017.
Pilloni, G., Cao, F., Ruhmel, M., and Mishra, P. (2022). Proteins identified through predictive metagenomics as potential biomarkers for the detection of microbiologically influenced corrosion. J. Ind. Microbiol. Biotechnol. 49, kuab068. doi:10.1093/jimb/kuab068 | |
Procópio, L. (2019). The role of biofilms in the corrosion of steel in marine environments. World J. Microbiol. Biotechnol. 35, 73. doi:10.1007/s11274-019-2647-4 | |
Procopio, L. (2020). The era of ‘omics’ technologies in the study of microbiologically influenced corrosion. Biotechnol. Lett. 42, 341–356. doi:10.1007/s10529-019-02789-w | |
Rapp, B. E. (2018). Electrochemical methods for biomass and biocorrosion monitoring. Editor K. Wandelt (Oxford: Elsevier), 166–172. doi:10.1016/B978-0-12-409547-2.13507-3 |
Rodrigues, E., and Akid, R. (2014). “Internal corrosion assessment of the otter oil production spool,” in Paper presented at the SPE International Oilfield Corrosion Conference and Exhibition. doi:10.2118/169603-MS |
Rossy, T., Nadell, C. D., and Persat, A. (2019). Cellular advective-diffusion drives the emergence of bacterial surface colonization patterns and heterogeneity. Nat. Commun. 10, 2471. doi:10.1038/s41467-019-10469-6 | |
Sachan, R., and Singh, A. K. (2020). Comparison of microbial influenced corrosion in presence of iron oxidizing bacteria (strains DASEWM1 and DASEWM2). Constr. Build. Mater. 256, 119438. doi:10.1016/j.conbuildmat.2020.119438 |
Salgar-Chaparro, S. J., Darwin, A., Kaksonen, A. H., and Machuca, L. L. (2020). Carbon steel corrosion by bacteria from failed seal rings at an offshore facility. Sci. Rep. 10, 12287. doi:10.1038/s41598-020-69292-5 | |
Schippers, A., and Blazejak, A. (2011). Real-time PCR quantification and diversity analysis of the functional genes aprA and dsrA of sulfate-reducing prokaryotes in marine sediments of the Peru continental margin and the black sea. Front. Microbiol. 2, 253. doi:10.3389/fmicb.2011.00253 | |
Senthilmurugan, B., Radhakrishnan, J. S., Poulsen, M., Tang, L., and AlSaber, S. (2021). Assessment of microbiologically influenced corrosion in oilfield water handling systems using molecular microbiology methods. Upstream Oil Gas Technol. 7, 100041. doi:10.1016/j.upstre.2021.100041 |
Seyeux, A., Zanna, S., and Marcus, P. (2014). “Surface analysis techniques for investigating biocorrosion,” in Understanding biocorrosion: Fundamentals and applications. Editors T. Liengen, D. Féron, R. Basséguy, and I. B. B. T.-U. B. Beech (Oxford: Woodhead Publishing), 197–212. doi:10.1533/9781782421252.2.197 |
Shakya, M., Lo, C.-C., and Chain, P. S. G. (2019). Advances and challenges in metatranscriptomic analysis. Front. Genet. 10, 904. doi:10.3389/fgene.2019.00904 | |
Shi, X., Yang, K., Yan, M., Yan, W., and Shan, Y. (2020). Study on microbiologically influenced corrosion resistance of stainless steels with weld seams. Front. Mater. 7. doi:10.3389/fmats.2020.00083 |
Skipper, P. J. A., Skipper, L. K., and Dixon, R. A. (2022). A metagenomic analysis of the bacterial microbiome of limestone, and the role of associated biofilms in the biodeterioration of heritage stone surfaces. Sci. Rep. 12, 4877. doi:10.1038/s41598-022-08851-4 | |
Skovhus, T., Enning, D., and Lee, J. (2017). Microbiologically influenced corrosion in the upstream oil and gas industry. 1st Edn. Boca Raton FL: CRC Press. doi:10.1201/9781315157818 |
Sooknah, R., Papavinasam, S., and Revie, R. W. (2008). “Validation of A predictive model for microbiologically influenced corrosion,” in Paper presented at the CORROSION 2008. NACE-08503.
Sowards, J. W., Williamson, C. H. D., Weeks, T. S., McColskey, J. D., and Spear, J. R. (2014). The effect of Acetobacter sp. and a sulfate-reducing bacterial consortium from ethanol fuel environments on fatigue crack propagation in pipeline and storage tank steels. Corros. Sci. 79, 128–138. doi:10.1016/j.corsci.2013.10.036 |
Stamps, B. W., Bojanowski, C. L., Drake, C. A., Nunn, H. S., Lloyd, P. F., Floyd, J. G., et al. (2020). In situ linkage of fungal and bacterial proliferation to microbiologically influenced corrosion in B20 biodiesel storage tanks. Front. Microbiol. 11, 167. doi:10.3389/fmicb.2020.00167 | |
Stevenson, B. S., Drilling, H. S., Lawson, P. A., Duncan, K. E., Parisi, V. A., Suflita, J. M., et al. (2011). Microbial communities in bulk fluids and biofilms of an oil facility have similar composition but different structure. Environ. Microbiol. 13, 1078–1090. doi:10.1111/j.1462-2920.2010.02413.x | |
Taleb-Berrouane, M., Khan, F., Hawboldt, K., Eckert, R., and Skovhus, T. (2018). Model for microbiologically influenced corrosion potential assessment for the oil and gas industry. Corros. Eng. Sci. Technol. 53, 378–392. doi:10.1080/1478422X.2018.1483221 |
Telegdi, J., Shaban, A., and Vastag, G. (2018). “Biocorrosion—Steel,” in Encyclopedia of interfacial chemistry: Surface science and electrochemistry. Editor K. Wandelt (Oxford: Elsevier), 28–42. doi:10.1016/B978-0-12-409547-2.13591-7 |
Thomsen, U. S., and Oehler, M. C. (2018). “A combination of qPCR, RT-qPCR and NGS provides a new tool for analyzing MIC risk in pipelines,” in Paper presented at the CORROSION 2008. NACE-2018-11103.
Tian, H., Gao, P., Chen, Z., Li, Y., Li, Y., Wang, Y., et al. (2017). Compositions and abundances of sulfate-reducing and sulfur-oxidizing microorganisms in water-flooded petroleum reservoirs with different temperatures in China. Front. Microbiol. 8, 143. doi:10.3389/fmicb.2017.00143 | |
Tripathi, A. K., Thakur, P., Saxena, P., Rauniyar, S., Gopalakrishnan, V., Singh, R. N., et al. (2021). Gene sets and mechanisms of sulfate-reducing bacteria biofilm formation and quorum sensing with impact on corrosion. Front. Microbiol. 12, 754140. doi:10.3389/fmicb.2021.754140 | |
Van den Berg, L., van Loosdrecht, M. C. M., and de Kreuk, M. K. (2021). How to measure diffusion coefficients in biofilms: A critical analysis. Biotechnol. Bioeng. 118, 1273–1285. doi:10.1002/bit.27650 | |
Verderosa, A. D., Totsika, M., and Fairfull-Smith, K. E. (2019). Bacterial biofilm eradication agents: A current review. Front. Chem. 7, 824. doi:10.3389/fchem.2019.00824 | |
Wang, D., Kijkla, P., Mohamed, M., Saleh, M., Kumseranee, S., Punpruk, S., et al. (2021). Aggressive corrosion of carbon steel by Desulfovibrio ferrophilus IS5 biofilm was further accelerated by riboflavin. Bioelectrochemistry 142, 107920. doi:10.1016/j.bioelechem.2021.107920 | |
Wasimuddin, S. K., Ronchi, F., Leib, S. L., Erb, M., Ramette, A., et al. (2020). Evaluation of primer pairs for microbiome profiling from soils to humans within the One Health framework. Mol. Ecol. Resour. 20, 1558–1571. doi:10.1111/1755-0998.13215 | |
Williamson, C. H. D., Jain, L. A., Mishra, B., Olson, D. L., and Spear, J. R. (2015). Microbially influenced corrosion communities associated with fuel-grade ethanol environments. Appl. Microbiol. Biotechnol. 99, 6945–6957. doi:10.1007/s00253-015-6729-4 | |
Zaidi, M., Masri, M., and Kew, W. (2021). Microbiologically influenced corrosion of iron by nitrate reducing Bacillus sp. J. Phys. Conf. Ser. 2129, 12066. doi:10.1088/1742-6596/2129/1/012066 |
Zamora, A. (2012). Methodological aspects for the culture and quantification of heterotrophic sulfate-reducing bacteria. Rev. Soc. Venez. Microbiol. 32, 121–125.
Keywords: biofilms, microbiologically-influenced-corrosion, biodeterioration, microbiomes, corrosion, omics
Citation: Puentes-Cala E, Tapia-Perdomo V, Espinosa-Valbuena D, Reyes-Reyes M, Quintero-Santander D, Vasquez-Dallos S, Salazar H, Santamaría-Galvis P, Silva-Rodríguez R and Castillo-Villamizar G (2022) Microbiologically influenced corrosion: The gap in the field. Front. Environ. Sci. 10:924842. doi: 10.3389/fenvs.2022.924842
Received: 20 April 2022; Accepted: 13 July 2022;
Published: 15 September 2022.
Edited by:
Antonis A. Zorpas, Open University of Cyprus, CyprusReviewed by:
Satoshi Wakai, Japan Agency for Marine-Earth Science and Technology (JAMSTEC), JapanCopyright © 2022 Puentes-Cala, Tapia-Perdomo, Espinosa-Valbuena, Reyes-Reyes, Quintero-Santander, Vasquez-Dallos, Salazar, Santamaría-Galvis, Silva-Rodríguez and Castillo-Villamizar. This is an open-access article distributed under the terms of the Creative Commons Attribution License (CC BY). The use, distribution or reproduction in other forums is permitted, provided the original author(s) and the copyright owner(s) are credited and that the original publication in this journal is cited, in accordance with accepted academic practice. No use, distribution or reproduction is permitted which does not comply with these terms.
*Correspondence: Genis Castillo-Villamizar, Z2Nhc3RpbGxvQGNvcnJvc2lvbmNpYy5jb20=
†ORCID: Edinson Puentes-Cala, https://orcid.org/0000-0003-0587-2177; Genis Castillo-Villamizar, https://orcid.org/0000-0001-6730-6889
Disclaimer: All claims expressed in this article are solely those of the authors and do not necessarily represent those of their affiliated organizations, or those of the publisher, the editors and the reviewers. Any product that may be evaluated in this article or claim that may be made by its manufacturer is not guaranteed or endorsed by the publisher.
Research integrity at Frontiers
Learn more about the work of our research integrity team to safeguard the quality of each article we publish.