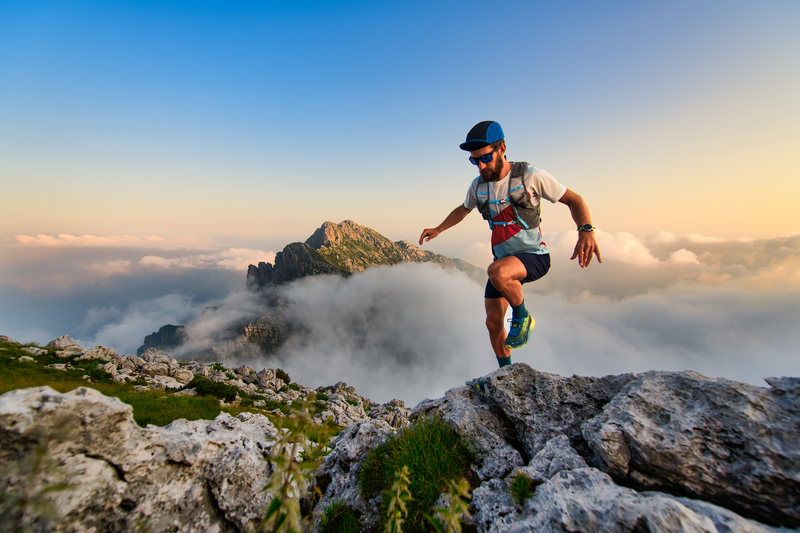
95% of researchers rate our articles as excellent or good
Learn more about the work of our research integrity team to safeguard the quality of each article we publish.
Find out more
ORIGINAL RESEARCH article
Front. Environ. Sci. , 15 August 2022
Sec. Environmental Economics and Management
Volume 10 - 2022 | https://doi.org/10.3389/fenvs.2022.922151
As the world’s largest developing country and the largest carbon emitter, China must consider economic growth and carbon emission reduction in development. Therefore, improving carbon productivity is an important goal of China at present. At the same time, China’s foreign capital inflow has always been at the forefront of the world, and foreign direct investment (FDI) has had various impacts on China’s carbon productivity. Based on the panel data of 25 provinces in China from 2007 to 2019, this paper uses a spatial econometric model to study the difference in the impact of FDI on China’s carbon productivity under different entry modes. The study found that: when FDI enters China in the mode of joint ventures, there is a positive spatial spillover effect, which is conducive to improving China’s carbon productivity; while when FDI enters China in the mode of wholly foreign-owned enterprises, there is a negative spatial spillover effect, which will inhibit the improvement of China’s carbon productivity. Therefore, when introducing foreign capital, the Chinese government should formulate differentiated foreign investment policies according to the different entry modes of FDI, and encourage more FDI to enter China in the mode of joint ventures.
Since China’s reform and opening up in 1978, with the attraction of the low labor cost, good development prospects, and the policy of attracting investment, a large amount of foreign capital has been pouring into China with a yearly increasing trend (As shown in Figure 1). When FDI enters China, it will comprehensively consider its development strategy and China’s policy environment to choose different entry modes. There are two main entry modes: joint venture and wholly foreign-owned enterprises (Wei et al., 2005). When FDI entered China, it has produced a significant positive spillover effect by promoting the transfer of advanced technology and knowledge, which has greatly promoted the improvement of the productivity of local Chinese enterprises (Abraham et al., 2010), thus injecting a strong impetus into China’s economic growth (Zeng and Zhou, 2021). Since the spillover effect of FDI depends to a large extent on the choice of FDI entry mode (Tian et al., 2015), when FDI enters China in different modes, it will have different effects on economic growth (Jin et al., 2016). At the same time, the entry of FDI has also led to significant carbon emissions (Yu and Xu, 2019). China faces great pressure and heavy tasks to reduce carbon emissions as the world’s largest energy consumer and carbon emitter (Li and Zhou, 2019). In October 2021, the State Council of China issued the Action Plan for Carbon Peaking by 2030, which clearly stated that by 2025, carbon dioxide emissions per unit of GDP would drop by 18% compared with 2020, laying a solid foundation for achieving carbon peaking. By 2030, carbon dioxide emissions per unit of GDP will be reduced by more than 65% compared with 2005, and the goal of peaking carbon emissions by 2030 is expected. These goals put forward higher requirements for China’s low-carbon economy. China is still a developing country, and its economic development must move forward steadily. Therefore, the Chinese government must coordinate the relationship between economic development and carbon emission reduction (Bao and Fang, 2013) and strive to achieve energy saving and emission reduction without sacrificing economic development (Gazheli et al., 2016). As carbon productivity is an indicator of coordinating economic development and carbon emission reduction (Li et al., 2018), China needs to improve the level of carbon productivity. Therefore, this paper is devoted to exploring the differences in the impact of FDI on China’s carbon productivity under different entry modes to find the most favourable entry mode for China’s carbon productivity improvement and put forward relevant policy recommendations accordingly. In this way, China can make better use of the advantages of a large amount of FDI and a stable growth trend so that FDI can better serve China’s high-quality economic development.
Carbon productivity represents the gross domestic product ratio to carbon dioxide emissions in a certain period (Kaya and Yokobori, 1997) and it is the core concept for evaluating low-carbon economic development (Shen et al., 2021). The research on carbon productivity in academia mainly focuses on identifying the driving factors of carbon productivity. Lu et al. (2015) used the Logarithmic Mean Divisia Index (LMDI) decomposition model to study and found that economic output, provincial carbon productivity, and energy structure are the most important factors affecting China’s carbon productivity. Liu and Zhang (2021) found that industrial agglomeration and carbon productivity have an inverted “U”-shaped relationship, and technological innovation plays an important role in determining the “inflection point”. Research by Li et al. (2018) found that after 2010, the transformation of economic development mode and the substantial increase in green investment led to a rapid increase in China’s total carbon productivity.
Due to differences in samples and methods, different scholars have come to conflicting conclusions on whether the same factors positively or negatively impact carbon productivity. Li and Wang (2019) found that per capita GDP, technological level, trade openness and foreign direct investment positively impact China’s carbon productivity, while energy consumption structure, industrial structure, and urbanization level negatively impact China’s carbon productivity. Regarding the impact of industrial structure on carbon productivity, Niu et al. (2021) came to the opposite conclusion that industrial structure is the factor that promotes carbon productivity. In addition, Long et al. (2016) found that per capita GDP negatively impacts carbon productivity. This is also contrary to the conclusion of Li and Wang (2019). It can be seen that carbon productivity is affected by many factors, and the specific impact of some factors on carbon productivity is still controversial.
Choosing an entry mode is an important part of a multinational company’s strategy, directly related to the investment risk, control rights, and income distribution of multinational companies. Since the reform and opening up, with the continuous expansion of the openness of China’s market and the constant changes in foreign investment policies, more multinational companies have chosen to enter China through wholly foreign-owned enterprises (Puck et al., 2009). The academic research on FDI entry mode mainly includes two aspects. The first aspect is to study the factors that affect the choice of FDI entry mode. The analysis result of Shen and Puig (2018) shows that the entry mode of foreign investors considers conditions that vary among countries and within the host economy and place. Cui and Jiang (2009) argue that a Chinese firm’s FDI entry mode choice is primarily influenced by the variables related to the firm’s strategic fit in the hosting industry and its strategic intent of conducting FDI. Chung et al. (2016) found that the institutional pressure exerted by the home government has a significant impact on the decision of FDI entry mode. However, for enterprises with less resource dependence and more institutional freedom, institutional government pressure has a weaker impact on the choice of FDI entry mode.
The second aspect is to study the difference in the impact of FDI under different entry modes. Through systematic research, Javorcik and Spatareanu (2008) found that, under different entry modes, there are significant differences between the intra-industry and inter-industry spillover effects of FDI. Konwar et al. (2015) examined the spillover effects of FDI under different entry modes. They found that the spillover effect of majority foreign-owned joint ventures is greater than that of wholly-owned subsidiaries and minority foreign-owned venture FDI. Jin et al. (2016) conducted an empirical study and found that joint ventures mainly promote China’s economic growth through spillover effects. In addition, wholly foreign-owned enterprises mainly promote China’s economic growth through their competitive advantages. The joint venture has a greater impact on China’s economic growth than wholly foreign-owned enterprises. When FDI enters through different modes, its impact on the host country varies greatly. Therefore, it is reasonable to study the impact of FDI on carbon productivity from the perspective of different entry modes.
Studying the relationship between FDI and carbon emissions is the foundation for studying the relationship between FDI and carbon productivity. Academia generally believes that FDI has both positive and negative effects on carbon emissions, corresponding to two hypotheses: the “Pollution Heaven Hypothesis” and the “Pollution Halo Hypothesis”.
The “Pollution Heaven Hypothesis” believes that due to the weak environmental supervision of the host country, some pollution-intensive enterprises will be transferred from other countries to the host country through FDI, resulting in a substantial increase in carbon emissions. Liu et al. (2021) found that FDI increased China’s carbon emissions by examining the role of FDI in China’s carbon neutrality goal. Nasir et al. (2019) study concluded that in the ASEAN-5 countries, FDI would increase carbon emissions, which supports the “Pollution Heaven Hypothesis”. In addition, Shahbaz et al. (2019) examined the role of FDI on carbon emissions in the U.S. The results showed that FDI increases carbon emissions.
The “Pollution Halo Hypothesis” believes that FDI can bring high-standard production models and advanced technologies to the host country, thereby helping to reduce the host country’s carbon emissions. Zhang and Zhou (2016) used China’s provincial panel data as a sample and adopted the Technology (STIRPAT) model in their study. Their results showed that FDI is beneficial to China’s carbon emission reduction. Yu and Xu (2019) investigated the impact of FDI on China’s industrial carbon emission reduction and found that FDI positively impacted industrial CO2 emissions reduction at the national level. In addition, Zhu et al. (2016) analyzed the effects of FDI on carbon emissions in ASEAN-5 and found that FDI can mitigate carbon emissions in high-emissions ASEAN countries, which supports the “Pollution Halo Hypothesis”.
Regarding the research on the impact of foreign direct investment on carbon emissions, academia has formed a systematic research framework. In contrast, the current research on the relationship between FDI and carbon productivity is relatively lacking. Since carbon emission intensity and productivity are reciprocal, the literature on carbon emission intensity and carbon productivity is regarded as the same category. Shao (2018) found through dynamic panel analysis that foreign direct investment had a significant negative impact on the carbon intensity of host countries. Pan et al. (2014) found a significant spatial autocorrelation between China’s FDI and carbon emission intensity. FDI is conducive to reducing carbon emission intensity of the local region whose space spillover effects and significantly reduces that of the surrounding area. Long et al. (2020), based on China’s provincial panel data from 1998 to 2016, combined with the impact mechanisms such as scale effect, structural effect, technical effect, environmental effect, and other influencing mechanisms, found that local FDI positively impacts local carbon productivity, while FDI in surrounding areas harms local carbon productivity. It can be seen that the existing research conclusions on the relationship between FDI and carbon productivity are relatively consistent, and they all believe that FDI will promote the increase of carbon productivity in the host country.
To sum up, the existing research has the following research gaps, which also become the possible marginal contribution of this paper. First of all, most of the current research focuses on the relationship between FDI and carbon emissions. The research on the impact of FDI on carbon productivity is still relatively scarce, and a systematic theoretical system has not yet been formed. This paper can further enrich the research content in this field. Secondly, the existing literature mainly studies the spatial spillover effect of FDI from the perspective of geographical attributes. This paper adds the economic distance matrix to explore the spatial spillover effect of FDI from the perspective of economic attributes. Finally, the existing research mainly starts from the total amount of FDI, ignoring that FDI may have different impacts on the host country under different modes. From the perspective of entry mode, this paper divides the total FDI into joint venture FDI and wholly foreign-owned FDI and studies the difference in the impact of FDI on China’s carbon productivity under different entry modes.
Therefore, based on the integration of existing research, this paper selects the panel data of 25 provinces in China from 2007 to 2019 and uses a spatial econometric model to analyze the difference in the impact of FDI on China’s carbon productivity under different entry modes, to provide certain policy guidance for developing China’s low-carbon economy.
The influencing mechanism of FDI on carbon productivity under different entry modes is shown in Figure 2. FDI affects the host country’s environment (including carbon productivity) mainly through scale, structural and technical effects (Grossman and Krueger, 1995). The scale effect means that the entry of FDI expands the overall production and output scale of the host country through capital accumulation and, at the same time, improves the level of economic development through taxation and employment, which in turn affects carbon productivity. Structural effect means that FDI promotes the transformation of the host country’s industrial structure, thereby affecting carbon productivity. The technical effect means that FDI brings advanced environmental protection, technology production, and management experience to the host country through technology spillover, which promotes the technological progress of the host country and affects carbon productivity.
Firstly, the scale effect of FDI under different entry modes is analyzed. FDI under both entry modes will expand the output scale of the host country, so the scale effect under both entry modes is positive. To prevent the proliferation of advanced technologies in the host country, foreign companies may apply the most advanced production technology and management experience to their wholly-owned subsidiaries and less sophisticated technology to their joint ventures with less control (Jin et al., 2016). Therefore, wholly foreign-owned enterprises are likely to have a larger output scale than a joint venture and contribute more tax revenue and employment opportunities to the host country. That is, wholly foreign-owned enterprises have a greater scale effect.
Secondly, the structural effect of FDI under different entry modes is analyzed. Compared with joint ventures, wholly foreign-owned enterprises may have advantages in technology and management. Therefore, its products will be more competitive, seizing the host country’s market, threatening the survival of the host country’s enterprises, and leading to the decline of the industrial structure. Although joint ventures may lag behind sole proprietorships in technology, they can have technological spillovers to the host country to a certain extent, driving the transformation and upgrading of its industrial structure. To sum up, the structural effect of wholly foreign-owned enterprises is negative, while the structural effect of joint ventures is positive.
Finally, the technical effect of FDI under different entry modes is analyzed. The articles of association or contracts of the joint venture cannot cover all aspects of the use of the intangible assets of the enterprise, which provides the possibility of technology spillover. The joint venture party from the host country can apply the new knowledge from the joint venture to the new enterprise. Other enterprises in the host country can also use personnel trained by the joint venture to improve production and operation (Jin et al., 2016). All of these can produce technology spillover effects. And these conditions are difficult to establish for wholly foreign-owned enterprises. Therefore, the technical effect is positive in both entry modes, and the technical effect of joint ventures is greater than that of wholly foreign-owned enterprises.
Before spatial econometric analysis, variables must be analyzed for spatial autocorrelation. Spatial autocorrelation refers to the potential interdependence between the observed data of variables in the same distribution area. When the variables have spatial autocorrelation, the spatial econometric model is necessary for analysis. Moran’s I is a common method for spatial autocorrelation analysis, and the calculation formula is:
In the formula,
The setting of the spatial weight matrix is a key step in spatial econometric analysis. The calculation of the Moran’s I depend on the spatial weight matrix, and the estimation results of the spatial econometric model are also affected by the spatial weight matrix. Based on the research of other scholars, this paper sets three spatial weights for research: geographic adjacency matrix, geographic distance matrix, and economic distance matrix.
The implicit assumption of the geographic adjacency matrix is that adjacent areas are more correlated than non-adjacent areas. This paper sets the geographic adjacency matrix based on the binary algorithm:
Where
The implicit assumption of the geographic distance matrix is that there is a stronger correlation between regions with closer distances than regions with farther distances. In this paper, the inverse of the distance between provinces is used to set the geographic distance matrix:
The implicit assumption of the economic distance matrix is that there is a stronger correlation between regions with similar economic attributes than regions with large differences in economic attributes. In this paper, the difference between the annual averages of GDP per capita in the two provinces is used to represent the difference in economic attributes of the two provinces, and the economic distance matrix is set as:
The spatial econometric model addresses the correlation between samples from different regions and fully accounts for the spatial factors’ influence. Currently, the more commonly used spatial measurement models include the spatial error model (SEM), the spatial lag model (SLM), and the spatial Durbin model (SDM). The spatial error model assumes that inter-regional interactions are realized through the error term and that random shocks cause spatial spillover effects. The spatial lag model contains a spatial lag operator for the dependent variable. All independent variables will affect other regions through a spatial conduction mechanism. The Spatial Durbin Model contains both the dependent variable and the independent variable spatial lag operator, which can simultaneously analyze the spatial spillover effects of the dependent variable and the independent variable in a region.
The general form of the spatial Durbin model is as follows. This paper uses it as the basis for empirical analysis and then judges whether it is the optimal model through the spatial applicability test.
In the formula,
The assumptions of SDM are as follows: first,
Carbon Productivity (cp). Carbon productivity is the ratio of gross domestic product to carbon dioxide emissions. This paper, in eliminating the impact of price changes, uses the 2006 price level as the base price and adjusts the nominal GDP of each region using the exponential smoothing method to obtain the real GDP of each region, and then use the real GDP to calculate carbon productivity.
Different entry modes of foreign direct investment: joint venture FDI (jv), wholly foreign-owned FDI (wfo). When FDI enters China, it will choose the appropriate entry mode based on its situation and China’s foreign investment policy in different periods. By consulting the China Statistical Yearbook, it can be known that the entry modes of FDI include the following: wholly foreign-owned enterprises, joint ventures, cooperative enterprises, foreign share-holding corporations Ltd. and cooperative development. Cooperative enterprises, foreign share-holding corporations Ltd. and cooperative development are essentially special forms of joint ventures resulting from changes in China’s foreign policy at different stages. Referring to Jin et al. (2016), this paper uses the sum of the capital of FDI under the four entry modes of the joint venture, cooperative enterprises, foreign share-holding corporations Ltd. and cooperative development as the joint venture capital. The joint venture FDI is expressed as the share of joint venture capital in regional GDP in each region. In contrast, the wholly foreign-owned FDI is expressed as the share of wholly foreign-owned capital in regional GDP.
From the literature review part, in addition to FDI, there are many factors affecting China’s carbon productivity. Based on the research on the driving factors of carbon productivity by Lu et al. (2015) and Li and Wang (2019), and drawing on the STIRPAT model (York et al., 2003), this paper selects the level of economic development, industrial structure, energy structure, and population size as control variables from the perspectives of economy, society, resources and environment (Ke et al., 2021).
The level of economic development (edl) is measured by the real GDP per capita of each province, and the calculation formula is as follows:
In the formula, GDP is the actual gross regional product, P is the number of permanent residents at the end of the year, i is the province, and t is the time.
In addition, the industrial structure (is) is represented by the proportion of the output value of the secondary industry in the regional GDP, representing the proportion of traditional industries in the industrial structure; the energy structure (es) is expressed as the proportion of coal consumption in the total regional energy consumption, representing the proportion of traditional energy in the energy consumption structure; the population size (ps) is the number of permanent residents in each region at the end of the year.
The above data are sourced from the “China Statistical Yearbook”, “China Energy Statistical Yearbook”, China Emission Accounts and Datasets (CEADs) (Guan et al., 2021; Shan et al., 2020; Shan et al., 2018; Shan et al., 2016), Provincial Statistical Yearbook and China Stock Market and Accounting Research (CSMAR) Database over the years, etc. The Tibet Autonomous Region was deleted due to a serious lack of data. The Hong Kong, Macao, and Taiwan regions were not within the scope of this paper due to the difficulty in obtaining data. In addition, Jilin, Liaoning, Ningxia, Qinghai, and Sichuan are also excluded from the sample because the amount of FDI under different entry modes is not listed in the statistical yearbook. Therefore, the research sample in this paper includes data from 25 provinces. The descriptive statistics of each variable are shown in Table 1. The maximum value of the energy structure is greater than one because some regions consume a large amount of coal for power generation, and the resulting power is exported to other regions. However, the energy consumption statistics for the region do not include electricity exported to other provinces, resulting in the province’s coal consumption being greater than energy consumption. Economic development and population size are logarithmically treated in the following analysis to reduce the effect of the magnitude. Detailed data are listed in Supplementary Table 1.
Multicollinearity refers to the distortion of model estimates due to high correlations between explanatory variables. We use the Pearson correlation matrix and the Variance Inflation Factor (VIF) to perform the multicollinearity test. Their results are shown in Table 2. The absolute values of the correlation coefficients between the explanatory variables are all less than 0.5, and the VIF of each explanatory variable is less than 2. It can be seen that there is no multicollinearity between the explanatory variables.
First, the Moran’s I was used to measure the spatial correlation of carbon productivity in China. The results are shown in Figure 3 and Figure 4. As far as the geographic adjacency matrix W1 is concerned, the Moran’s I of carbon productivity between 2007 and 2019 is always positive, has a downward trend, and is significant at the 5% level. This shows that China’s carbon productivity has a very significant positive spatial correlation, but the spatial agglomeration of carbon productivity between adjacent regions has weakened over time. As far as the geographic distance matrix W2 is concerned, the Moran’s I of carbon productivity between 2007 and 2018 was positive but small and changed from positive to negative in 2019. Combined with the p-value, it can be seen that China’s carbon productivity has a positive spatial correlation at the 10% significance level from 2007 to 2017, while it failed the 10% significance test from 2018 to 2019. As far as the economic distance matrix W3 is concerned, the Moran’s I of carbon productivity between 2007 and 2019 is positive. Its value is between W1 and W2, and it passes the 10% significance test except for 2007 and 2008. This shows a significant positive spatial correlation between China’s carbon productivity in general and regions with smaller economic gaps with similar carbon productivity levels. To sum up, it can be concluded that there is a positive spatial correlation between carbon productivity among all provinces in China under the three spatial weight matrices, and it is reasonable to use the analysis method of spatial measurement.
FIGURE 3. Moran’s I of carbon productivity in China under three spatial weight matrices from 2007 to 2019.
FIGURE 4. p-value of Moran’s I of carbon productivity in China under three spatial weight matrices from 2007 to 2019.
Firstly, the applicability of the spatial Durbin model is tested, and the test results are shown in Table 3. While the Robust LM test for the spatial error model under the economic distance matrix was not statistically significant, the other Robust LM tests and all LM tests passed the 1% significance level. Thus, significantly rejecting the null hypothesis of no spatial autocorrelation. It shows that compared with the spatial lag and error models, the spatial Durbin model is more suitable for the analysis in this paper. And because all LR test results have passed the significance test at the 1% level, the spatial Durbin model cannot degenerate into a spatial error model and a spatial lag model. Although one of the Wald tests failed the significance test, overall, it was determined that the spatial Durbin model was appropriate.
Through the Hausman test, the spatial Durbin model under the three spatial weight matrix assumptions rejected the null hypothesis of random effects; that is, the fixed-effects model was adopted (Abonazel and Shalaby, 2020). In this paper, using stata16 as a measurement tool, the regression of the time fixed-effect model, the individual fixed-effect model, and the two-way fixed effect model under the three spatial weight matrices were carried out, respectively. The R-squared of these three models is shown in Table 4. It can be seen that the R-squared of the time fixed effect model is the largest under the three spatial weight matrices, indicating that the time fixed effect model has better goodness of fit than other models. The formula of the spatial Durbin model is as follows:
In the formula, i is the province, t is the year,
The regression results of the time fixed effects model are analyzed below, and the results are shown in Table 5. The spatial autocorrelation coefficients of carbon productivity under the three spatial weight matrices are all less than zero. They passed the significance test at the 1 and 10% levels under the geographic and economic distance matrices. These indicate that China’s carbon productivity has an obvious siphon effect. That is, the carbon productivity of a province will be negatively affected by the carbon productivity of adjacent provinces and provinces with similar economic attributes. The possible explanation is that provinces with higher carbon productivity usually have corresponding national policy support and occupy more high-quality development resources. This limits the improvement of carbon productivity in neighbouring provinces and provinces with similar economic attributes.
Since the spatial Durbin model contains the spatial lag term of the dependent variable, its regression coefficient cannot directly reflect the influence of the independent variable on the dependent variable. Therefore, the effects of the spatial Durbin model are decomposed, and the decomposition results of direct, indirect, and total effects are obtained, as shown in Table 6. Firstly, the direct effect is analyzed. The joint venture FDI’s direct effect is insignificant under the three spatial weight matrices. The direct effect of the wholly foreign-owned FDI is not significant under the geographic and economic distance matrices. Still, it is significantly positive under the geographic adjacency matrix. Since wholly foreign-owned enterprises have strong protection for their technology, the parent company is more willing to apply the latest technology to its wholly-owned subsidiaries so that wholly foreign-owned enterprises have more advanced technology than joint ventures. With the support of advanced technology, the carbon productivity of wholly foreign-owned enterprises is likely to be much higher than the local carbon productivity prior to its entry, making the local carbon productivity rise. Joint ventures have no obvious impact on the local carbon productivity due to their low carbon productivity. Secondly, the indirect effect is analyzed. Under the three spatial weight matrices, the indirect effect of the joint venture FDI is significantly positive, while the indirect effect of the wholly foreign-owned FDI is significantly negative. Through technology spillovers, joint ventures drive industrial transformation and upgrading in adjacent areas and areas with similar economic attributes and then promote the improvement of carbon productivity in adjacent areas and areas with similar economic attributes through structural and technical effects. Wholly foreign-owned enterprises will have a crowding-out effect on local enterprises, which is not conducive to upgrading the industrial structure. This leads to a negative structural effect which is not conducive toimproving carbon productivity in adjacent areas and areas with similar economic attributes. Finally, the total effect is analyzed. Under the three spatial weight matrices, the total effect of the joint venture FDI is significantly positive, and the total effect of the wholly foreign-owned FDI is significantly negative. This shows that, on the whole, joint venture FDI is beneficial to the improvement of China’s carbon productivity. At the same time, wholly foreign-owned FDI is not conducive to improving China’s carbon productivity.
From the decomposition results of the control variables, it can be seen that the direct effect of the industrial structure is significantly negative under the three spatial weight matrices. The indirect effect is significantly positive under the geographic adjacency and geographic distance matrices, but significantly negative under the economic distance matrix. Traditional industries are often characterized by high energy consumption and low production efficiency, adversely affecting local carbon productivity. The concentration of traditional industries in a certain area will help improve the carbon productivity of adjacent areas but will hurt the carbon productivity of areas with similar economic attributes. Secondly, under the three spatial weight matrices, the direct effect of population size is significantly positive, and the indirect effect is significantly negative. This shows that the concentration of human resources in a certain area will make local carbon productivity develop well. In contrast, it will make the human resources in adjacent areas and areas with similar economic attributes deficient, thus inhibiting their carbon productivity. Then, the direct effect of the economic development level is significantly positive under both the geographic adjacency and geographic distance matrices, but not significant under the economic distance matrix. Its indirect effect is significantly negative under the geographic adjacency matrix, not significant under the geographic distance matrix, and significantly positive under the economic distance matrix. Economically developed regions have advantages in both resources and technology, which facilitates the improvement of local carbon productivity and drives the improvement of carbon productivity in regions with similar economic attributes. However, the development of carbon productivity in adjacent regions is inhibited due to lack of resources. Finally, under the three spatial weight matrices, the energy structure’s direct and indirect effects are significantly negative. Compared with new clean energy, traditional energy will produce more carbon emissions with the same production capacity. Excessive use of traditional energy will adversely affect the carbon productivity in the region and adjacent regions and regions with similar economic attributes.
To avoid endogeneity, the first-order lag term of carbon productivity is used as the explanatory variable, and a robustness test is carried out. The results are shown in Table 7. It can be seen from Table 7 that there is no fundamental change in the significance of the coefficients of each variable, indicating that the empirical conclusions are robust.
This paper measures the carbon productivity of each province in China, selecting panel data from 25 provinces in China from 2007 to 2019. Three spatial weight matrices were established: geographic adjacency matrix, geographic distance matrix, economic distance matrix, and Moran index for spatial autocorrelation analysis. The spatial Durbin model is used to study the impact of different FDI entry modes on China’s carbon productivity. The following conclusions are drawn: 1) There is a high positive spatial correlation of carbon productivity in all provinces in China. Not only do areas with high carbon productivity agglomerate geographically, but the carbon productivity of areas with similar economic attributes is also relatively close. 2) When FDI enters China in the mode of joint ventures, the impact on local carbon productivity is insignificant. Still, there is a significant positive spatial spillover to carbon productivity in adjacent regions and regions with similar economic attributes. Overall, the joint ventures FDI has contributed to improving China’s carbon productivity. When FDI enters China in the mode of wholly foreign-owned enterprises, it positively impacts the local carbon productivity. Still, there is a significant negative spatial spillover to carbon productivity in adjacent regions and regions with similar economic attributes. Overall, the wholly foreign-owned FDI restrains China’s carbon productivity growth. 3) The industrial structure will promote local carbon productivity. It has positive spatial spillovers to the improvement of carbon productivity in adjacent regions and negative spatial spillovers to carbon productivity in regions with similar economic attributes. The population size can promote local carbon productivity. It has negative spatial spillovers on carbon productivity in adjacent regions and regions with similar economic attributes. The level of economic development can promote the improvement of local carbon productivity. It has negative spatial spillovers on carbon productivity in adjacent regions and positive in regions with similar economic attributes. The energy structure is not conducive to the improvement of local carbon productivity. It has negative spatial spillovers on the carbon productivity of adjacent regions and regions with similar economic attributes.
This paper puts forward the following suggestions:
1) The state should better play the role of macro-control and allocate resources more efficiently and reasonably at the national level. So that areas with development potential can be supported by corresponding resources and policies. Provinces and cities should fully consider the interests of surrounding areas in policy formulation, establish a good policy coordination system, and form a regional policy linkage mechanism. Eliminate regional barriers and promote the market-oriented flow of production factors such as technology and manpower, thereby optimizing resource allocation. Guide the synchronous optimization of industrial structure and energy structure among regions, promote the common development of economy among regions, and form a high-quality development model in which carbon productivity in each region promotes each other.
2) At present, more and more foreign capital chooses to enter China in the mode of wholly foreign-owned enterprise, which is not conducive to improving China’s carbon productivity. First, when developing the economy, local governments should formulate investment promotion policies compatible with local resource endowments and provide corresponding incentives or preferential treatment to joint ventures based on local specific conditions. Second, China must speed up the process of opening up the capital market. And actively promote joint ventures such as foreign share-holding corporations Ltd. and cooperative development, and innovate in the form of joint ventures under the current institutional framework, thereby attracting more FDI to enter China in the form of joint ventures. Finally, pay attention to the digestion and absorption of core technological achievements and advanced management concepts in the process of introducing foreign capital, and increase the technological spillover effect, thereby promoting the improvement of China’s carbon productivity and the process of China’s low-carbon economy.
3) In economic construction, all regions should accelerate transforming and upgrading traditional high-polluting and low-efficiency industries. Introduce advanced technology and equipment to improve the energy utilization efficiency of the industry, promote the use of clean energy to replace traditional high-polluting energy gradually, and promote the development of Chinese industries in the direction of high-end, intelligent, and green development (Youssef et al., 2020).
The original contributions presented in the study are included in the article/Supplementary Material, further inquiries can be directed to the corresponding authors.
All authors listed have made a substantial, direct, and intellectual contribution to the work and approved it for publication.
The authors declare that the research was conducted without any commercial or financial relationships that could be construed as a potential conflict of interest.
All claims expressed in this article are solely those of the authors and do not necessarily represent those of their affiliated organizations, or those of the publisher, the editors and the reviewers. Any product that may be evaluated in this article, or claim that may be made by its manufacturer, is not guaranteed or endorsed by the publisher.
The Supplementary Material for this article can be found online at: https://www.frontiersin.org/articles/10.3389/fenvs.2022.922151/full#supplementary-material
Abonazel, M. R., and Shalaby, O. A. (2020). Using dynamic panel data modeling to study net FDI inflows in MENA countries. Stud. Econ. Econ. 44 (2), 1–28. doi:10.1080/10800379.2020.12097360
Abraham, F., Konings, J., and Slootmaekers, V. (2010). FDI spillovers in the Chinese manufacturing sector: Evidence of firm heterogeneity. Econ. Transition 18 (1), 143–182. doi:10.1111/j.1468-0351.2009.00370.x
Bao, C., and Fang, C. (2013). Geographical and environmental perspectives for the sustainable development of renewable energy in urbanizing China. Renew. Sustain. Energy Rev. 27, 464–474. doi:10.1016/j.rser.2013.07.008
Chung, C. C., Xiao, S. S., Lee, J. Y., and Kang, J. (2016). The interplay of top-down institutional pressures and bottom-up responses of transition economy firms on FDI entry mode choices. Manag. Int. Rev. 56 (5), 699–732. doi:10.1007/s11575-015-0256-5
Cui, L., and Jiang, F. (2009). FDI entry mode choice of Chinese firms: A strategic behavior perspective. J. World Bus. 44 (4), 434–444. doi:10.1016/j.jwb.2008.11.004
Gazheli, A., Van Den Bergh, J., and Antal, M. (2016). How realistic is green growth? Sectoral-level carbon intensity versus productivity. J. Clean. Prod. 129, 449–467. doi:10.1016/j.jclepro.2016.04.032
Grossman, G. M., and Krueger, A. B. (1995). Economic growth and the environment. Q. J. Econ. 110 (2), 353–377. doi:10.2307/2118443
Guan, Y., Shan, Y., Huang, Q., Chen, H., Wang, D., and Hubacek, K. (2021). Assessment to China's recent emission pattern shifts. Earth's. Future 9 (11), e2021EF002241. doi:10.1029/2021EF002241
Javorcik, B. S., and Spatareanu, M. (2008). To share or not to share: Does local participation matter for spillovers from foreign direct investment? J. Dev. Econ. 85 (1-2), 194–217. doi:10.1016/j.jdeveco.2006.08.005
Jin, H., Zhou, X., and Zhang, Q. J. V. F. D. I. (2016). Wholly funded FDI and Chinese economic growth — the empirical analysis based on provincial panel data. Mod. Econ. Sci. 38 (3), 44–52. (in Chinese).
Kaya, Y., and Yokobori, K. (1997). Environment, energy, and economy: Strategies for sustainability. Tokyo: United Nations University Press.
Ke, H., Dai, S., and Yu, H. (2021). Spatial effect of innovation efficiency on ecological footprint: City-level empirical evidence from China. Environ. Technol. Innovation 22, 101536. doi:10.1016/j.eti.2021.101536
Konwar, Z., Macdonald, F., Wang, C., and Wei, Y. (2015). The rise of multinationals from emerging economies——achiving a new balance[M]. UK: Palgrave Macmillam, 243–261.
Li, S., and Wang, S. (2019). Examining the effects of socioeconomic development on China's carbon productivity: A panel data analysis. Sci. Total Environ. 659, 681–690. doi:10.1016/j.scitotenv.2018.12.409
Li, S., and Zhou, C. (2019). What are the impacts of demographic structure on CO2 emissions? A regional analysis in China via heterogeneous panel estimates. Sci. Total Environ. 650, 2021–2031. doi:10.1016/j.scitotenv.2018.09.304
Li, W., Wang, W., Wang, Y., and Ali, M. (2018). Historical growth in total factor carbon productivity of the Chinese industry–a comprehensive analysis. J. Clean. Prod. 170, 471–485. doi:10.1016/j.jclepro.2017.09.145
Liu, X., Wahab, S., Hussain, M., Sun, Y., and Kirikkaleli, D. (2021). China carbon neutrality target: Revisiting FDI-trade-innovation nexus with carbon emissions. J. Environ. Manag. 294, 113043. doi:10.1016/j.jenvman.2021.113043
Liu, X., and Zhang, X. (2021). Industrial agglomeration, technological innovation and carbon productivity: Evidence from China. Resour. Conservation Recycl. 166, 105330. doi:10.1016/j.resconrec.2020.105330
Long, R., Shao, T., and Chen, H. (2016). Spatial econometric analysis of China’s province-level industrial carbon productivity and its influencing factors. Appl. Energy 166, 210–219. doi:10.1016/j.apenergy.2015.09.100
Long, R., Gan, X., Chen, H., Wang, J., and Li, Q. (2020). Spatial econometric analysis of foreign direct investment and carbon productivity in China: Two-tier moderating roles of industrialization development. Resour. Conservation Recycl. 155, 104677. doi:10.1016/j.resconrec.2019.104677
Lu, J., Fan, W., and Meng, M. (2015). Empirical research on China’s carbon productivity decomposition model based on multi-dimensional factors. Energies 8 (4), 3093–3117. doi:10.3390/en8043093
Nasir, M. A., Huynh, T. L. D., and Tram, H. T. X. (2019). Role of financial development, economic growth & foreign direct investment in driving climate change: A case of emerging ASEAN. J. Environ. Manag. 242, 131–141. doi:10.1016/j.jenvman.2019.03.112
Niu, M., Tan, X., Guo, J., Li, G., and Huang, C. (2021). Driving factors and growth potential of provincial carbon productivity in China. Sustainability 13 (17), 9759. doi:10.3390/su13179759
Pan, X., Yang, Y., and Zhang, W. (2014). Spatial effect analysis of foreign direct investment (FDI) on carbon intensity in China. Environ. Eng. Manag. J. 13 (5), 1251–1255. doi:10.30638/eemj.2014.131
Puck, J. F., Holtbrügge, D., and Mohr, A. T. (2009). Beyond entry mode choice: Explaining the conversion of joint ventures into wholly owned subsidiaries in the People's Republic of China. J. Int. Bus. Stud. 40 (3), 388–404. doi:10.1057/jibs.2008.56
Shahbaz, M., Gozgor, G., Adom, P. K., and Hammoudeh, S. (2019). The technical decomposition of carbon emissions and the concerns about FDI and trade openness effects in the United States. Int. Econ. 159, 56–73. doi:10.1016/j.inteco.2019.05.001
Shan, Y., Liu, J., Liu, Z., Xu, X., Shao, S., Wang, P., et al. (2016). New provincial CO2 emission inventories in China based on apparent energy consumption data and updated emission factors. Appl. Energy 184, 742–750. doi:10.1016/j.apenergy.2016.03.073
Shan, Y., Guan, D., Zheng, H., Ou, J., Li, Y., Meng, J., et al. (2018). China CO2 emission accounts 1997–2015. Sci. Data 5 (1), 170201–170214. doi:10.1038/sdata.2017.201
Shan, Y., Huang, Q., Guan, D., and Hubacek, K. (2020). China CO2 emission accounts 2016–2017. Sci. Data 7 (1), 54–59. doi:10.1038/s41597-020-0393-y
Shao, Y. (2018). Does FDI affect carbon intensity? New evidence from dynamic panel analysis. Int. J. Clim. Chang. Strateg. Manag. 10, 27–42. doi:10.1108/IJCCSM-03-2017-0062
Shen, N., Peng, H., and Wang, Q. (2021). Spatial dependence, agglomeration externalities and the convergence of carbon productivity. Socio-Economic Plan. Sci. 78, 101060. doi:10.1016/j.seps.2021.101060
Shen, Z., and Puig, F. (2018). Spatial dependence of the FDI entry mode decision: Empirical evidence from emerging market enterprises. Manag. Int. Rev. 58 (1), 171–193. doi:10.1007/s11575-017-0332-0
Tian, X., Lo, V. I., and Song, M. (2015). FDI technology spillovers in China: Implications for developing countries. J. Dev. Areas 49 (6), 37–48. doi:10.1353/jda.2015.0082
Wei, Y., Liu, B., and Liu, X. (2005). Entry modes of foreign direct investment in China: A multinomial logit approach. J. Bus. Res. 58 (11), 1495–1505. doi:10.1016/j.jbusres.2004.10.002
York, R., Rosa, E. A., and Dietz, T. (2003). STIRPAT, IPAT and ImPACT: Analytic tools for unpacking the driving forces of environmental impacts. Ecol. Econ. 46 (3), 351–365. doi:10.1016/S0921-8009(03)00188-5
Youssef, A. H., Abonazel, M. R., and Shalaby, O. A. (2020). Determinants of per capita personal income in the US: Spatial fixed effects panel data modeling. JoARAMS. 5, 1–13. doi:10.24321/2455.7021.202001
Youssef, A. H., Abonazel, M. R., and Shalaby, O. A. (2022). Spatial and non-spatial panel data estimators: Simulation study and application to personal income in US states. WSEAS Trans. Math. 21, 487–514. doi:10.37394/23206.2022.21.56
Yu, Y., and Xu, W. (2019). Impact of FDI and R&D on China's industrial CO2 emissions reduction and trend prediction. Atmos. Pollut. Res. 10 (5), 1627–1635. doi:10.1016/j.apr.2019.06.003
Zeng, S., and Zhou, Y. (2021). Foreign direct investment’s impact on China’s economic growth, technological innovation and pollution. Int. J. Environ. Res. Public Health 18 (6), 2839. doi:10.3390/ijerph18062839
Zhang, C., and Zhou, X. (2016). Does foreign direct investment lead to lower CO2 emissions? Evidence from a regional analysis in China. Renew. Sustain. Energy Rev. 58, 943–951. doi:10.1016/j.rser.2015.12.226
Keywords: carbon productivity, foreign direct investment, entry mode, joint venture, wholly foreign owned enterprise, spatial spillover effect
Citation: Tang D, Yi R, Kong H, Da D and Boamah V (2022) Foreign direct investment entry mode and China’s carbon productivity based on spatial econometric model. Front. Environ. Sci. 10:922151. doi: 10.3389/fenvs.2022.922151
Received: 17 April 2022; Accepted: 25 July 2022;
Published: 15 August 2022.
Edited by:
Elena Moltchanova, University of Canterbury, New ZealandReviewed by:
Fei Fan, Wuhan University, ChinaCopyright © 2022 Tang, Yi, Kong, Da and Boamah. This is an open-access article distributed under the terms of the Creative Commons Attribution License (CC BY). The use, distribution or reproduction in other forums is permitted, provided the original author(s) and the copyright owner(s) are credited and that the original publication in this journal is cited, in accordance with accepted academic practice. No use, distribution or reproduction is permitted which does not comply with these terms.
*Correspondence: Decai Tang, dGFuZ2RlY2FpQG51aXN0LmVkdS5jbg==; Haojia Kong, YmVzdGtvbmdAbmp1c3QuZWR1LmNu
Disclaimer: All claims expressed in this article are solely those of the authors and do not necessarily represent those of their affiliated organizations, or those of the publisher, the editors and the reviewers. Any product that may be evaluated in this article or claim that may be made by its manufacturer is not guaranteed or endorsed by the publisher.
Research integrity at Frontiers
Learn more about the work of our research integrity team to safeguard the quality of each article we publish.