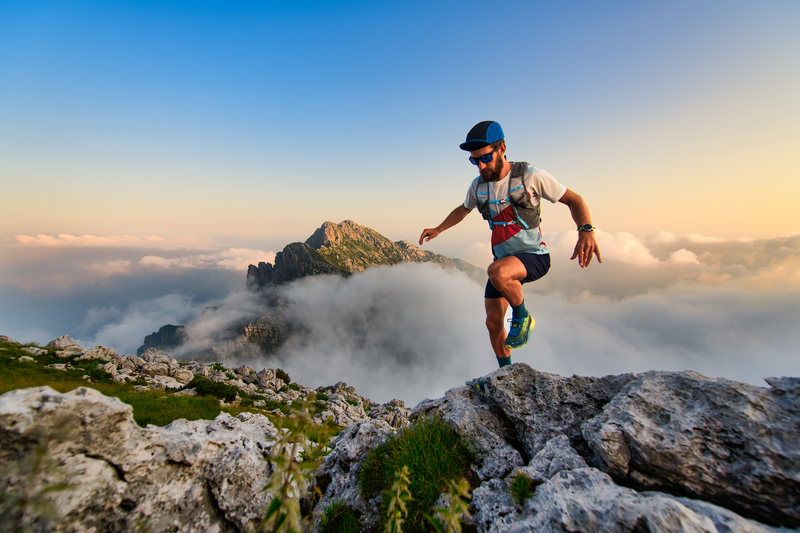
95% of researchers rate our articles as excellent or good
Learn more about the work of our research integrity team to safeguard the quality of each article we publish.
Find out more
ORIGINAL RESEARCH article
Front. Environ. Sci. , 06 June 2022
Sec. Environmental Economics and Management
Volume 10 - 2022 | https://doi.org/10.3389/fenvs.2022.921374
This article is part of the Research Topic Export Product Quality, Renewable Energy, and Sustainable Production View all 48 articles
The complex and severe problem of energy poverty seriously affects the health and quality of life of residents and poses a significant challenge to the country’s macroeconomic development. The purpose of this paper is to analyze the spatial distribution of energy poverty and the characteristics of regional differences in 30 provinces in mainland China from 2008 to 2019 by constructing an energy poverty evaluation index system and explore the main reasons for the gap between energy poverty regions in China from the perspective of “relational data.” The results show that the spatial distribution of energy poverty in China is “high in the west and central area and low in the east,” and the spatial pattern is relatively stable. From the perspective of the time dimension, the problem of energy poverty in China has been alleviated during the sample period, and the overall gap in energy poverty has shown a decreasing trend. The difference in energy poverty between regions is the primary source of the overall gap, but its contribution is gradually decreasing. The empirical results suggest that reducing the imbalance of industrial structure, energy price, and energy investment among regions can effectively narrow the inter-regional energy poverty gap. Moreover, there are differences in the dominant factors that determine the difference in energy poverty within the eastern, central, and western regions. In this regard, it is recommended to further improve the relevant policies in developing new energy sources according to local conditions, reasonably increasing energy construction investment, strengthening technological innovation, establishing financial guarantee mechanisms, and guiding residents to consume modern, clean energy.
Energy poverty is widespread in all countries of the world and is a major challenge to the energy system of the international community. Energy poverty seriously impacts the health and quality of life of residents and poses a considerable challenge to the macroeconomic development of countries (Che et al., 2021). According to the International Energy Agency International Energy Agency (IEA), 2017], it is estimated that despite rapid advances in globalization and information technology, more than 2.1 billion people worldwide still rely on traditional biomass for cooking, 82% of which are concentrated in Asia and Africa, and 900 million people still lack access to electricity. As the world’s largest developing country, China has become a major energy producer and consumer, but there is still a significant gap between China’s energy development quality and that of developed countries. In particular, China has a vast territory and a large population, and energy poverty ranges widely and varies in severity. The severe and complex energy poverty phenomenon deserves our attention. Therefore, it is urgent to objectively and truly analyze the current situation and regional differences in energy poverty in China and explore the key factors affecting regional differences in energy poverty, which is not only conducive to seeking effective strategies to alleviate energy poverty in China but also can provide a meaningful reference for other developing countries in the world.
International research on energy poverty began in the 1980’s, when Lewis (1982) initially proposed the concept of energy poverty from the perspective of energy affordability, pointing out that insufficient energy use would affect people’s living standards. Since then, Boardman (1991) proposed that people who spend more than 10% of their income on energy use could be considered energy poor. However, this criterion ignores the difficulty of access to adequate energy in some developing countries (Papada and Kaliampakos, 2018). In fact, energy poverty is mainly reflected in: first, the low level of energy use, with the current level of per capita domestic energy use in developing countries being much lower than that in developed countries; second, the poor structure of energy use, which is reflected in the lack of access to modern and clean energy represented by electricity and the still more frequent use of solid fuels such as coal, firewood, and straw; third, the weak capacity to use electricity, which makes it difficult to pay for relatively expensive clean energy (Bazilian et al., 2010). For this reason, the scholars have subsequently focused more on modern energy access when studying energy poverty in developing countries (Tang and Liao, 2014; Sadath and Acharya, 2017) and have gradually adopted multidimensional indicators to quantify energy poverty (Ogwumike and Ozughalu, 2016; Berry, 2018; Zhang et al., 2019). In addition, academic studies on energy poverty have widely focused on the impact of energy poverty on the health of residents and the environment, the causes of energy poverty, energy poverty policies, and energy poverty prediction (Duflo et al., 2008; Adkins et al., 2010; Lim et al., 2012; Lacey et al., 2017; Mohr, 2018; Khanna et al., 2019; Primc and Slabe-Erker, 2020; Leitão, 2021). In contrast, research on energy poverty in China is relatively recent, and the literature is mostly focused on the adverse effects of energy poverty, especially the negative impact of solid fuel use on the health of the population (Sinton et al., 2004; Chapman et al., 2005; Baumgartner et al., 2011; Kim et al., 2016; Liu et al., 2018; Yun et al., 2020). Moreover, some literature has analyzed the energy poverty situation in China at the micro-level with the help of questionnaires and other methods (Sun et al., 2014; Zheng et al., 2014; Liu et al., 2020). For example, Tang and Liao (2014) analyzed the cooking energy use of rural households in China using Chinese census data; Yang and Mukhopadhaya (2017) and Zhang et al. (2019) studied the multidimensional energy poverty in China using the data of China Family Panel Studies (CFPS). Wu et al. (2021) studied the impact of energy poverty on rural labor wages using data from the Chinese General Social Survey (CGSS) in 2015.
In general, there is a lack of research on the spatial characteristics and influencing factors of energy poverty in China from the macro level. Li et al. (2014) designed a multidimensional energy poverty assessment index with Chinese characteristics, but no relevant empirical analysis was conducted due to data limitations. Zhao et al. (2018) and Li and Li (2020) analyzed the regional differences and influencing factors of energy poverty mainly for rural areas in China and concluded that energy poverty in rural China has significant regional differences. Furthermore, Lu et al. (2022) used the data envelopment method to evaluate and cluster the performance of energy poverty in 30 provinces for 11 years and explored the spatial and temporal variation characteristics of energy poverty in China but did not conduct empirical tests on its influencing factors.
Consequently, we take 30 provinces in mainland China as the research object and construct the evaluation index system of energy poverty. Based on the analysis of the overall spatial distribution characteristics of energy poverty in China, we focus on the spatial differences and influencing factors of energy poverty in China from 2008 to 2019 through the Dagum Gini coefficient and the Quadratic Assignment Procedure (QAP) method. This paper aims to provide a scientific basis for the government to narrow the regional energy poverty gap and formulate policies to alleviate energy poverty. Compared with the existing literature, the innovation of this paper is mainly reflected in two aspects: on the one hand, this paper adopts multidimensional indicators to measure the energy poverty in 30 provinces in China and then conducts a comparative analysis of spatial differences; on the other hand, the influencing factors of regional differences in energy poverty in China is empirically analyzed based on the perspective of relational data.
The rest of the paper is organized as follows. Section 2 constructs the evaluation system of energy poverty indicators in China and introduces the data resources and research methods. Section 3 mainly analyzes the spatial characteristics and center of gravity distribution of energy poverty in China. Section 4 discusses the spatial differences in energy poverty in China. Section 5 empirically examines the main factors affecting the regional disparity of energy poverty based on the relational data. Section 6 concludes the paper and discusses the policy implications.
Energy poverty is a complex and constantly improving concept with regional solid and contemporary characteristics. The design and construction of the energy poverty comprehensive index is the basis for analyzing the spatial characteristics of energy poverty. The problem of energy poverty in China is unique, and there is no energy poverty assessment index system that is widely recognized by academia and universally applicable. Therefore, based on the EDI framework designed by IEA and existing studies, we construct a comprehensive evaluation index system of energy poverty in China from two dimensions of energy supply and energy consumption according to the socio-economic development characteristics and the availability of data in China, which is shown in Figure 1.
Energy supply capacity ensures the availability of energy for residents. Compared with modern energy sources, traditional biomass energy such as fuelwood and coal are not only inefficient but also prone to environmental damage and health impairment. Thus the extensive use of fuelwood and coal is regarded as a typical sign of energy poverty. The international community also generally considers energy poverty to be the absence of access to modern energy services such as electricity and clean fuels. Therefore, energy supply is central to measuring energy poverty. It is worth noting that since the full coverage of domestic electricity has been basically achieved in China’s urban and rural areas, “access to electricity,” which is commonly used in the international assessments of energy poverty, is no longer a key indicator for assessing energy poverty in China. Specifically, the following four indicators are selected to measure energy supply capacity.
Natural gas plays a vital role in the energy transition as a clean energy source that contributes to green and low-carbon development, a directional energy source that promotes energy restructuring, and an alternative energy source that improves residents’ quality of life. As a residential energy resource that can be used for household cooking and heating, natural gas not only emits less CO2 than coal, oil, and other fossil energy but also has a more stable and mature supply model compared with other renewable energy sources (Abánades, 2018). At present, the natural gas industry has entered into a medium-high speed development stage in China. We use the ratio of natural gas supply to the number of people supplied to measure natural gas supply per capita in cities (m3/person), and all data is from the China Energy Statistical Yearbook.
The thermal efficiency of burning coal is only 15%–18%, but if coal is converted into gas fuel, the thermal energy efficiency can be increased by more than 30% according to the conversion efficiency of gas production technology. Therefore, gas is an important part of the energy supply, which provides high-quality gas fuel for industry, commerce, and residential life in cities. Improving the gas penetration rate in cities is not only a need to save energy consumption and improve energy efficiency but also an important measure to protect the urban environment and improve people’s living standards. The gas penetration rate in cities is characterized by the ratio of the urban population using gas to the total urban population, and the data is obtained from the China Statistical Yearbook.
Biogas is a combustible gas produced by microbial fermentation of various organic substances under certain conditions and is important modern biomass energy in rural areas. As a renewable energy source, biogas is important for supplementing domestic energy in rural areas, adjusting the energy structure of rural households, and solving the problem of energy scarcity in China. We use the indicator of rural biogas production per capita (m3/person) to measure the supply of modern biomass energy in the rural domestic energy mix. The data of rural biogas production are obtained from the China Agricultural Statistics, and the rural population data are obtained from the China Statistical Yearbook.
Solar water heaters convert sunlight energy into heat energy to meet residents’ living needs and are pollution-free renewable energy. However, solar water heaters are affected by unfavorable factors such as seasons and weather. With the continuous improvement of photoelectric complementary technology and control technology, as well as solar vacuum tube and pipe insulation technology, the advantages of solar water heaters in terms of safety and energy efficiency have come to the fore, playing an important role in using renewable energy in rural areas. Hence, we take the area covered by solar water heaters per capita in rural areas as an important indicator to measure renewable energy supply with the data from the China Agricultural Statistics.
Household energy consumption is the second largest area of energy consumption besides the industrial sector in China. Energy consumption is the purpose of energy production and supply, which satisfies people’s energy needs, such as power and heat in their daily life, and is an important link in the energy system. The way of energy consumption directly determines the efficiency of energy use and also puts forward the requirements for energy supply. To eliminate energy poverty and accelerate the green and low-carbon transformation of energy, we not only need to achieve energy conservation and control total energy consumption but also actively promote the transformation of energy consumption mode, focus on the modernization and low-carbon energy use structure, and build a new energy consumption system. Consequently, energy poverty is assessed in terms of both energy consumption and energy consumption structure, considering the following indicators:
The representative household energy sources include biomass energy, coal, oil, and natural gas, and electricity, and electrification is the key to alleviating energy poverty (Kooijman-van Dijk, 2012). At this stage, although residential coal consumption is decreasing year by year in China, there is still a big gap compared with the developed countries in the world. Therefore, the domestic electricity and natural gas consumption per capita are selected to measure the living energy consumption of residents measured by the ratio of domestic electricity consumption and natural gas consumption of residential departments to the total population of the province respectively, including urban and rural areas. The data of energy consumption of each province comes from the energy balance sheet in the China Energy Statistical Yearbook.
The proportion of household energy consumption to household income is an important indicator of energy poverty. Energy poverty is not only reflected in residents’ choice of non-clean and non-modern energy but also in their high living energy costs. Considering the availability of data, we use the proportion of residents’ living expenses to total consumption to measure this index, in which the living expense cover the expenditure of rent, water, electricity, fuel, property management, etc. The data are obtained from the China Statistical Yearbook.
Solid commodity energy refers to the solid combustible material that generates heat or power and mainly refers to coal and coal products such as raw coal and briquette in living energy. Compared with non-solid commodity energy, such as electricity and natural gas, the direct combustion of solid commercial energy releases a lot of pollutants. Therefore, the proportion of solid energy in the energy consumption structure should be balanced, and residents should be encouraged to consume non-solid commodity energy, which is beneficial to improving the living environment and atmospheric environment. Solid commodity energy is calculated by coal and coal product consumption and standard coal coefficient in the energy balance sheet of each province in the China Energy Statistical Yearbook. Commodity energy is calculated from the energy consumption and standard coal coefficient in the provincial energy balance sheet. The above data include both urban and rural areas.
Thermal power generation mainly uses the heat energy generated by combustible materials, which is converted into electricity by the power generating devices. At present, thermal power generation is still the primary source of electricity in China, while other forms of energy generation such as hydro, wind, and nuclear power are relatively small in scale. However, as the environmental pollution problem becomes more prominent and people’s living standards continue to improve, the need to use clean energy to replace coal for power generation becomes more urgent (Jiang et al., 2020). Since the breakdown of domestic electricity consumption by different generation methods is not available, we choose the proportion of non-thermal power generation in the whole industry to characterize the sources of domestic electricity consumption. Data on thermal power generation and total power generation are obtained from the China Energy Statistical Yearbook.
The energy consumption structure is the amount of each type of energy consumed by each sector of the national economy and its share in the total energy consumption in a certain period. Improving the energy consumption structure is an inevitable requirement to improve energy use efficiency and optimize the habitat after economic development reaches a certain level (Zhang et al., 2021). We measure the energy consumption structure by calculating the ratio of coal consumption to total energy consumption. The coal consumption ratio can be obtained after converting various energy consumption into standard coal and excluding the interference of intermediate products. The coal consumption is the sum of seven energy end-use consumption, and the total energy consumption is the sum of twenty relevant energy end-use consumption and all data are obtained from the China Energy Statistical Yearbook.
To assess the degree of energy poverty in different regions, we standardize the above indicators and then use the entropy value method to determine the weights. On this basis, the energy poverty index of different provinces per year can be calculated using the weighted summation method.
Where yit is the energy poverty index of province i in year t, a is the number of specific indicators of energy poverty, wia is the weight of the indicator a of province i, and miat is the standardized value of the indicator a of province i in year t. The larger the value of yit, the larger the energy poverty index, and the more serious the energy poverty problem is.
Based on the calculation of the energy poverty index obtained for each province, we further measure the regional differences in energy poverty. The Dagum Gini coefficient and the decomposition method by subgroups effectively solve the problem of the source of regional disparities and the problem of cross-over between subsamples.
The Gini coefficient is defined as shown in Eq. 2.
Where G is the overall Gini coefficient, which is the average of the national energy poverty index, n is the number of provinces, k is the number of regional divisions, yji (yhr) is the energy poverty index for any province in region j(h), nj (nh) is the number of provinces in region j(h), j and h are the number of regional divisions, and i and r are the numbers of provinces in the region.
The Gini coefficient can be decomposed into three components: the contribution of intra-regional variation Gw, the contribution of inter-regional disparity Gnb, and the contribution of hypervariable density Gt, which satisfy the relationship G = Gw + Gnb + Gt. Eqs. 3, 4 represent the Gini coefficiSent Gjj and the contribution of intra-regional variation Gw for region j, respectively. Eqs. 5, 6 represent the inter-regional Gini coefficient Gjh and the contribution of inter-regional net hypervariable disparity Gnb for regions j and h, respectively. Moreover, Eq. 7 represents the contribution of hypervariance density Gt.
Where
Essentially, the energy poverty gap between regions can be defined as a gap between two regions. Therefore, in order to specifically measure the energy poverty gap between different regions, we assume that there are n regions, and the energy poverty level of each region is expressed by yi (i = 1, 2, ..., n), where i denotes the i region. The energy poverty gap between different regions can be expressed in the form shown in Table 1.
There is also a difference between regions for a factor that affects the energy poverty gap. Similarly, in this paper, a factor affecting the energy poverty gap is denoted as xi (i = 1, 2, ..., n), where i denotes the i region so that the regional gap of a factor between two regions can be expressed in the following form, as shown in Table 2.
For this purpose, the following relational data econometric model is set:
In Eq. 11, β0, β1, and β2 are the parameters to be estimated; X and Y are the explanatory and explained variables, respectively; Z is the control variable, and U is the residual term. Different from the attribute data model, in the relational data model, all variables are n-order square matrices, and the specific matrix form is shown in Eq. 12. The observations yi,j, xi,j, and zi,j in the matrix represent the differences between two regions for the explained variables, explanatory variables, and control variables, respectively. The specific values can be obtained by calculating yi − yj, xi − xj, and zi − zj. Since the observed values are the subtraction of indicators between two regions, the main diagonal elements yi,j, xi,j, and zi,j are all 0 when i = j.
For the relational data model, the correlation coefficients between the row and column elements in the residual matrix U are non-zero, meaning that the row and column elements are not independent, leading to the autocorrelation problems in the econometric model. In addition to the autocorrelation problem, there is often serious multicollinearity between variables in the form of relational data. If traditional statistical tests are used, the variance and standard deviation of the parameter estimates will increase, and the significance test of the variables will lose its meaning (Tsai, 2002; Stanton and Mann, 2014; Zagenczyk et al., 2015). To address the autocorrelation as well as multicollinearity problems of the relational data model, the quadratic assignment procedure (QAP), which is a nonparametric test based on random permutation, is developed (Barnett, 2011; Scott, 2017).
QAP is performed by converting the relationship matrix into a “long” vector, calculating the correlation coefficients (regression coefficients), and performing a random permutation to determine the significance of the parameter estimates. This step is repeated several times, and the regression coefficients and the goodness-of-fit R2 are saved for each random permutation to obtain the set of regression coefficients Γ [Y, π (
Using the above comprehensive evaluation index system of energy poverty, we assessed the energy poverty status of 30 provinces, cities, and autonomous regions (Tibet, Hong Kong, Macao, and Taiwan are not covered) in China from 2008 to 2019. Figure 2 shows the average values of the energy poverty index for each province from 2008 to 2019. In terms of ranking, Zhejiang, Hainan, Guangdong, Shanghai, and Jiangsu, and other eastern coastal regions have the lowest energy poverty index, ranging from 0.24 to 0.26, with relatively less energy poverty phenomenon. Guizhou, Yunnan, Gansu, and Henan provinces have the highest energy poverty index, above 0.52, which means the energy poverty problem is most serious in these regions. The maximum value of the energy poverty index value in Guizhou province reaches 0.64, while the minimum value of the energy poverty index in Zhejiang province is only 0.24, which means that there is a large gap between the maximum and minimum value of the indicator. Furthermore, the average value of the overall energy poverty index in China is 0.38, and the energy poverty index of 17 provinces is concentrated in the range of [0.3, 0.5], indicating that the distribution of the middle range value is relatively concentrated. By region, the average energy poverty index in the eastern region is only 0.29, which is the lowest level, while the energy poverty index in the central, western, and northeastern regions is similar, all of which are around 0.4. Specifically, among the top 10 provinces with the highest energy poverty index, only one province is located in the eastern region, one in the northeastern region, three in the central region, and five in the western region.
To reflect the spatial distribution characteristics of energy poverty in China more intuitively, we used the natural breakpoint classification method of ArcGIS software to classify the 30 provinces into energy poverty high value area, medium high value area, medium equivalent value area, medium low value area and low value area. The results are shown in Figure 3. It can be found that there is a significant difference in the spatial distribution of energy poverty among provinces, which is generally characterized as “high in the western and central regions and low in the eastern regions.” Specifically, in 2008, high value areas of energy poverty formed contiguous areas represented by Gansu, Inner Mongolia, Henan, and Shanxi, while the low value areas were concentrated in Shanghai, Zhejiang, Jiangsu, Guangdong, and eastern coastal areas of Fujian, as well as Beijing and Tianjin. By 2019, the spatial distribution of energy poverty in China has changed significantly, and the problem of energy poverty has been alleviated overall. The number of high value areas of energy poverty has been significantly reduced, and the spatial contiguous distribution characteristics have been broken, with only Yunnan being classified as a high value area of energy poverty.
From the perspective of time evolution, from 2008 to 2019, the degree of energy poverty in eastern China is dominated by low, medium-low, and medium values, and the energy poverty gap between internal provinces shows a decreasing trend. The central region changed from being dominated by high value and medium-high value provinces to being dominated by medium-high value and medium value provinces. In the western region, the predominance of high value and medium-high value provinces changes to a pattern where high value, medium-high value, medium value, and low value provinces coexist. In addition, it is worth noting that the northeast region has changed from being dominated by medium value provinces to being dominated by medium-high value provinces, and the problem of energy poverty has become increasingly serious. The reason is that as the old industrial base of China, solid commodity energy is relatively abundant in the northeast region. The rural energy consumption in these areas is still mainly coal and firewood, with a lower degree of energy cleanliness and thus a more serious degree of energy poverty. In contrast, as the eastern region is an economically developed region, and the West-East Gas Transmission Project has been completed, which has greatly enhanced the security of the modern energy supply, the degree of energy cleanliness is higher, so the degree of energy poverty is lighter. Moreover, because the central and western regions, especially Guangxi, Inner Mongolia, and Sichuan, have vigorously promoted the development of hydropower, wind power, and photovoltaic in recent years, the level of renewable energy development and utilization efficiency has been improved, and the problem of energy poverty has been significantly improved.
It is worth emphasizing that the level of energy poverty and economic development in each province do not actually remain completely consistent with the above analysis. Thus, we cannot rely solely on economic development to evaluate the level of energy poverty. Some of the regions in China where energy poverty is more significant are not the most economically backward regions, while some of the economically backward regions do not have serious energy poverty problems. For example, Henan is always in the middle and lower reaches of the energy poverty index in ascending order, with prominent energy poverty problems, while its economic development level is at the top of the country. On the contrary, Qinghai is always at the top of the ascending ranking of the energy poverty index, maintaining its ranking around the tenth in the country, and is one of the provinces with fewer energy poverty problems, while its GDP in 2021 is only higher than that of Tibet, which is one of the poorest provinces in the economy.
The standard deviation ellipse is used to explore the spatial distribution characteristics and evolutionary trend of the energy poverty center of gravity in China in this part. According to Figure 4, we can find that the standard deviation ellipse shows a spatial distribution pattern of “northeast-southwest” in general, and the ellipse area accounts for about 1/3 of the national territory. Compared with the eastern region, energy poverty in central and western provinces such as Guizhou and Gansu is more serious. Since the initial year 2008, the ellipse area decreased and then slightly increased in subsequent years, and the spatial pattern of energy poverty in China is relatively stable. During the sample examination period, the center of gravity of the ellipse is mainly distributed in Luoyang City, Henan Province. However, the center of gravity of the ellipse follows a northeast-southeast-northeast-southeast trajectory, moving 84 km to the northeast overall, from Luochuan County, Luoyang City (111.31°E, 33.84°N) to Song County, Luoyang City (112.16°E, 33.97°N).
According to the standard deviation ellipse related parameters shown in Table 3, from the change of the short semi-axis length, it shortens from 913.91 to 887.64 km from 2008 to 2014 and extends from 887.64 to 911.32 km from 2014 to 2019, showing a relatively stable trend overall, indicating that the distribution of energy poverty in China is relatively stable in the north-south direction. From the change of the length of the long semi-axis, it is extended from 1,275.37 km in 2008 to 1,318.71 km in 2019 during the examination period, showing a lengthening trend overall, indicating that energy poverty in China shows a spreading trend in the east-west direction, and energy poverty is more severe in the western and northeastern regions. From the angle of rotation, the rotation angle decreased from 69.06° to 68.28° from 2008 to 2014, and the rotation angle increased again to 70.30°from 2014 to 2019. The magnitude of the change in the rotation angle is always within 2°, indicating that the offsetting trend of the spatial distribution pattern of energy poverty in China is not apparent, and the overall trend is still northeast-southwest.
The Gini coefficient of energy poverty in China by province is shown in Figure 5. During the sample examination period, the overall gap of energy poverty in China shows a decreasing trend, with the Gini coefficient decreasing from 0.193 in 2008 to 0.111 in 2019, with an annual average value of 0.161 and an annual average decrease of 0.007. This indicates that the overall gap of energy poverty in China is narrowing, and energy poverty in the central and western regions has been alleviated. From the perspective of evolution, the overall gap of energy poverty in China has roughly experienced three stages. In the first stage, from 2008 to 2010, the overall Gini coefficient showed a slow decline trend from 0.193 to 0.189, a decrease of 1.93%. In the second stage, from 2010 to 2012, the overall Gini coefficient showed a slight upward trend, reaching the maximum value of 0.21 during the sample study period. Finally, after 2012, the overall Gini coefficient declined, gradually dropping to 0.111, with an overall decrease of 47.04% and an average annual decrease of 0.014. This finding indicates that the energy development in China has entered a transition period in line with the trend due to increasingly severe environmental risk challenges and energy resource constraints. By sticking to the path of high-quality development, the capacity to develop energy resources in rural and poor areas continues to improve, and the construction of major power and livelihood projects continues to be carried out, thus making up for weak links in energy infrastructure.
The intra-regional disparity of energy poverty in the four major regions of China is shown in Figure 6. During the sample examination period, the intra-regional Gini coefficient is the highest in the western region and the second highest in the eastern region, related to more provinces in the eastern and western regions and greater differences in internal energy development. From 2008 to 2013, the intra-regional Gini coefficient in the central region is higher than that in the northeastern region. However, after 2013, the intra-regional Gini coefficient in the central region further decreased, gradually lower than the northeast region, and became the lowest among the four regions. Specifically, from the perspective of the evolution of the regional Gini coefficient in the four regions, the Gini coefficient in the western region shows three stages of “slow rise-rapid decline-gradual stabilization,” with the Gini coefficient decreasing from 0.151 to 0.138, a decline of 8.71%. From 2009 to 2012, the Gini coefficient increased from 0.145 to 0.173, with an average annual decrease of 0.009 and an average annual decrease rate of 6.14%. During the sample investigation period, the Gini coefficient reached its maximum value in 2012. From 2012 to 2014, the Gini coefficient declined rapidly to 0.14, with an average annual decline of 0.016. After 2014, the Gini coefficient declined slowly, with an average annual decline of 0.001 and an average annual decline rate of only 0.38%. Moreover, the regional Gini coefficient in the eastern region shows a trend of “first increasing and then decreasing,” with the gini coefficient decreasing from 0.137 to 0.088, a decrease of 35.86%. From 2009 to 2013, the Gini coefficient rose from 0.119 to 0.142, with an average annual increase of 0.005 and an average annual increase of 4.54%. After 2013, the Gini coefficient gradually declined to 0.088, with an average annual decline of 0.009 and an average annual decline rate of 7.39%. The Gini coefficient in the central region shows a “rapid decline,” with the Gini coefficient falling from 0.113 to 0.051, a decline of 55.22%. Among them, the Gini coefficient reached the highest value of 0.104 in 2012 and reached the highest annual decline rate of 27.65% from 2012 to 2014. The Gini coefficient in the northeast region shows a more dynamic trend and is the only region among the four regions where the Gini coefficient has increased, showing a trend of “up-down-up again-slow down,” with the Gini coefficient rising from 0.034 to 0.047, an increase of 38.84%. The Gini coefficient reached the highest value of 0.072 in 2012. Among the three regions, the Gini coefficient decreased in the order of central, eastern, and western regions, indicating that the inter-provincial energy poverty difference in the central region has the highest reduction rate.
This section examines the inter-regional disparities in energy poverty in China based on the extended Gini coefficient, and the results are shown in Figure 7. We divided the four regions into six pairs for comparative analysis. The differences among different regions are heterogeneous, among which the level of difference in the degree of energy poverty between the east and the west is the highest, with a mean Gini coefficient between regions of 0.228. In contrast, the difference in energy poverty between the central and northeastern regions is the lowest, with an average inter-regional Gini coefficient of 0.084. In terms of temporal evolution, the Gini coefficients of four regions show a decreasing trend during the sample period, but there are differences in the specific evolutionary patterns.
The Gini coefficients between the east and west and the east and northeast regions show a trend of “rising-rapidly declining-steadily declining,” with the Gini coefficient between the east and west reaching a peak of 0.322 in 2012 and declining at a rate of 8.63% after 2012, and the Gini coefficient between the east and northeast regions reaching a peak of 0.269 in 2013 and declining at a rate of 11.79% after 2013. The Gini coefficients between the eastern and central and central and northeastern regions show a decreasing trend yearly. Among them, the greatest decreases are observed from 2013 to 2014, with 36.81% and 27.84%, respectively, indicating the highest variation in energy poverty between regions. Furthermore, the Gini coefficients between the central and western regions and between the western and northeastern regions show a trend of “slowly increasing and slowly decreasing.” Before 2012, the Gini coefficients increased at an average annual rate of 2.81% and 4.41%, respectively, and reached the highest values of 14.58 and 17.44 in 2012. After 2012, the Gini coefficient decreased at an average annual rate of 4.98% and 4.99%, respectively. In 2019, the Gini coefficient was the lowest at 0.105 and 0.111, respectively. Among the six regional pairs, the Gini coefficient between the east and the center has the most remarkable average annual change, decreasing by 0.017 at the rate of 8.31%, indicating the difference between the east and the center energy poverty shows a more rapid trend of narrowing.
To investigate the sources of overall differences in energy poverty in China, the total Dagum Gini coefficient is decomposed into intra-regional Gini coefficient, inter-regional Gini coefficient, and super-variable density, and its contribution rate to the overall coefficient is calculated. The results are shown in Table 4. During the examination period, the annual average contribution rates of intra-regional Gini coefficient, inter-regional Gini coefficient, and super-variable density are 22.98%, 53.51%, and 23.51%, respectively. In the order of contribution rate, the sources of spatial differences in energy poverty in China are inter-regional disparity, super-variable density, and intra-regional disparity, respectively. Therefore, the inter-regional gap is the main source of the difference in energy poverty in China.
From the time evolution trend, the intra-regional Gini coefficient shows a decreasing trend year by year, from 0.004 in 2008 to 0.035 in 2019, while the contribution rate increases from 20.68% to 27.52%. The inter-regional Gini coefficient and contribution rate both show a decreasing trend, with the Gini coefficient decreasing from 0.125 to 0.041 and the contribution rate decreasing from 64.963% to 37.051%. Moreover, the inter-regional Gini coefficient decreases more rapidly compared to the intra-regional Gini coefficient, with an average annual rate of change of 8.39%. In addition, both the super-variable density Gini coefficient and the contribution rate show an increasing trend, with the Gini coefficient increasing from 0.027 to 0.039 and the contribution rate increasing from 14.35% to 35.43%. The above analysis shows that although inter-regional disparities are the main source of energy poverty disparities in China, their contribution rate gradually decreases. With the introduction of measures to alleviate energy poverty, the inter-provincial and inter-regional differences in energy poverty are gradually reduced.
The causes of energy poverty are intricate and complex, which are not only economic problems but also social problems, and even historical and cultural problems.
Firstly, one of the most important factors affecting energy poverty is economic factors. With the increasing level of regional economic development, residents’ ability to pay for clean and green energy has increased. Domestic energy use has begun to show a trend toward cleanliness, efficiency, and modernization, thus helping to improve the energy poverty situation. According to the energy ladder theory, rising income increases household modern energy consumption (Hosier and Dowd, 1987; Leach, 1992; Davis, 1998). In general, high-income households are more likely to make the energy transition than low-income households, and thus, increasing income is one of the most critical factors in significantly reducing energy poverty (Zhang and Hassen, 2017; Muller and Yan, 2018; Lin and Zhao, 2021). However, since data on per capita disposable income of urban and rural residents in China are more severely missing in some years, we use the indicator of urbanization rate measured by the proportion of the urban population in total population as a proxy. The significant impact of the urbanization rate on the increase in income levels, especially for rural residents, and the reduction of the urban-rural income gap has been well documented. Moreover, economic factors are reflected in the price of energy in addition to income levels (Wang et al., 2017). Raising the energy price is not conducive to improving energy poverty because the increase in the price of energy increases the cost of energy use and suppresses the demand for some normal energy use. In addition, residential sector energy consumption may be affected by energy prices because different energy species can serve both residential cooking and heating needs and are strongly substitutable for each other. We use the residential consumer price index for utilities and fuels to characterize residential energy prices for domestic use in each province.
Secondly, the development and utilization of energy, the promotion, and application of energy-saving technologies, the normal operation of energy management and promotion agencies and other aspects of energy development are inseparable from the financial investment. The construction of energy infrastructure, even more, determines energy transmission and utilization. Data released by the National Energy Administration of China show that in the past eight years of 2020, China has invested more than 2.7 trillion yuan in major energy projects in poor areas, which has played an important role in effectively increasing local energy supply and promoting economic development (Wu et al., 2021). Therefore, we characterize the level of energy investment in terms of fixed asset investment in the energy industry of the state-owned economy and examine its impact on energy poverty.
At last, alleviating energy poverty and improving energy use efficiency also depend on two factors. On the one hand, technological progress can improve energy use efficiency and thus reduce energy consumption intensity. On the other hand, industrial restructuring can also play a role in the flow of energy from low-productivity industries to high-productivity ones, such as agriculture to industry and industry to services. To this end, we use the number of patent applications granted in each province as a proxy variable for the level of technology and measure its impact on energy poverty. The adjustment of industrial structure is measured by the proportion of industrial value added to GDP and the proportion of service value added to GDP. Specifically, the descriptive statistics of each influencing factor are shown in Table 5.
The following paper will reveal the main factors affecting the energy poverty gap in China through QAP regression analysis at three levels: full sample, period, and year by year, and then find the determining forces affecting energy poverty.
Table 6 reports the QAP regression results for the entire sample period. It can be observed that, unlike the traditional OLS regression, the QAP regression results report two types of regression coefficients: the un-standardized regression coefficients and the standardized regression coefficients. The standardized regression coefficients are estimated after standardizing all matrices, while the un-standardized regression coefficients are estimated directly on the original matrix (Borgatti et al., 2014). The standardized regression coefficients have two distinct advantages. On the one hand, the standardized regression coefficients are independent of the observed magnitude, whereas the unstandardized regression coefficients are closely related to the observed magnitude. On the other hand, the standardized regression coefficients can provide more useful information. Although the values of the standardized and unstandardized regression coefficients are different, the signs are the same, meaning that the standardization has not changed the direction of the variable effects. In the regression results of the same model, it is meaningless to compare the unstandardized regression coefficients of different variables because of the constraint of the magnitude of the observations. However, since standardization eliminates the effect of the observed magnitude, the standardized regression coefficients of different variables can not only be directly compared, but also the size of standardized regression coefficients can be compared (Christakis and Fowler, 2009), which can help us analyze the differences in the strength of the effects of different variables on the explanatory variables.
According to the regression results in Table 6, from the model fit, the adjusted R2 reaches 0.691, which means that the above six variables can explain 69.1% of the inter-regional disparity in energy poverty in China. All the standardized regression coefficients of the six variables passed the significance test from the standardized regression coefficients.
By comparing the absolute values of the standardized regression coefficients of all influencing factors, it can be found that the influence intensity of the six factors on the regional disparity of energy poverty in China from high to low is ser (0.9989), urb (−0.9870), ind (0.5164), pat (−0.3257), pri (0.2197), and inv (0.1826). This indicates that reducing the imbalance of industrial structure among regions can effectively reduce the regional energy poverty gap. Industrial structure adjustment is important for residents to improve the energy use structure of household life and induce them to use relatively modern and clean electricity instead of inefficient and highly polluting solid energy. The impact of urbanization rate and technology development on the energy poverty gap between regions is significantly negative, probably because energy poverty areas in China are not economically backward regions, and not all economically poor regions face the problem of energy poverty, which is consistent with the findings of the previous analysis. Furthermore, the intensity of the impact of energy prices on the inter-regional disparity of energy poverty ranked second from the bottom, slightly higher than the intensity of energy investment. This result suggests that the energy consumption of Chinese residents is still in the current unmet demand; reducing the inter-regional energy price gap is beneficial to encourage residents to use modern energy and reduce the energy poverty gap. Similarly, increasing the level of energy investment can significantly reduce energy poverty, which indicates that increasing government investment in the energy industry and gradually improving energy infrastructure and energy management institutions are important for the development of the energy industry.
To illustrate the robustness of the above regression results, we conduct further tests, and the regression results are shown in Table 7. Given the focus of the QAP regression analysis is on standardized regression coefficients, unstandardized regression coefficients will not be reported in this section and thereafter. The valid diagonals are selected in the model (1) during regression analysis. Different from basic regression, the regression results also included the value of the diagonal of the matrix for displacement. Model (2) and model (3) change the number of permutations, and the more the number of model permutations, the more stable the simulation results. In the basic regression, we set the number of permutations to 2000, while model (2) and model (3) are replaced with 5,000 and 10,000 permutations, respectively. From the regression results, we can find that the basic results of this paper remain robust after making the above series of changes.
According to the above complete sample regression, we further consider regional heterogeneity and divide the sample into eastern, central, and western regions for sub-sample regression. Among them, the regression analysis is not conducted because the northeast region contains only three provinces, and the sample size is too small. The specific results are shown in Table 8, and models (1), (2), and (3) correspond to the eastern, central, and western regions, respectively. At the regional level, the energy price and energy investment are the key factors affecting the regional gap in energy poverty in eastern China. In contrast, the effects of scientific and technological development and the share of the service industry are no longer significant. Urbanization is the critical factor affecting the regional gap in energy poverty in the central region. The effects of energy price, energy investment, and science and technology development are not significant. The influence of energy price and energy investment on energy poverty regional disparity in the western region is greater, while all other variables are insignificant. Although the western region has the advantage of unique resources, clean energy development is still limited due to the geographical environment and topography, and the cost of energy infrastructure construction is higher than that of the eastern coastal region. Therefore, the level of energy investment determines the construction of energy infrastructure in the western region. In addition, most of the energy developed in the western region is imported to the eastern coastal region, resulting in a limited and expensive energy supply in the west, but the level of energy prices directly affects the ability of residents to consume commodity energy.
In the same model, comparing the standardized regression coefficients in different periods helps to dynamically examine the changes in the influencing factors of the inter-regional gap in energy poverty in China. Therefore, based on the above regional heterogeneity, we further consider temporal heterogeneity and conduct QAP regression analysis by dividing the sample period into three periods: 2008–2011, 2012–2015, and 2016–2019. Table 9 reports the QAP regression results by period. It can be observed that with the continuous improvement of regional economic development, the living and development needs of residents are gradually met, and energy has gradually become middle and low-end consumer goods in the whole residential consumption system. Consequently, the impact of energy prices and energy investment on the regional gap in energy poverty becomes insignificant. Besides, the level of urbanization, technological development, and industrial structure still play an important role in the regional disparity of energy poverty. Therefore, with the continuous optimization of the energy consumption structure of residents in China, improving technological innovation, promoting the revolution of energy production and consumption, and developing renewable energy with high quality are the necessary ways to solve the problem of energy poverty nowadays.
Energy poverty manifests in unbalanced energy development, inequitable distribution of resources, and unsustainable energy use structure in the residential sector. The existence of energy poverty will hinder the continuous improvement of people’s livelihood and is not conducive to the sustainable development of the country. The current energy poverty situation assessment is a prerequisite for the scientific formulation of energy poverty mitigation policies and scientific support for determining the targets of relevant policy implementation. Therefore, we take 30 provinces in China as the research objects, construct the evaluation index system of energy poverty, analyze the spatial distribution characteristics of energy poverty in China from 2008 to 2019 as well as the inter-regional variability and further explore the main reasons affecting the inter-regional disparity of energy poverty through empirical analysis. The study finds that the spatial distribution of energy poverty in each province of China shows significant differences, with the overall characteristics of “high in the west and central part and low in the east.” The spatial pattern is relatively stable, and the center of gravity of the standard deviation ellipse is mainly distributed in Luoyang City, Henan Province. Regarding the time dimension, between 2008 and 2019, the problem of energy poverty in China has been alleviated, and the overall gap in energy poverty between regions has shown a decreasing trend. Furthermore, the difference in energy poverty between regions is the main source of the gap, but the contribution is decreasing. Except for the northeast, where intra-regional disparities have become more prominent, both intra-regional and inter-regional disparities in other regions show a decreasing trend, but exhibit divergent trends. The results of the empirical analysis show that the regional differences in energy poverty are the result of a combination of factors, among which reducing the imbalance in industrial structure, energy prices, and energy investment between regions can effectively reduce the energy poverty gap, which is important for solving the problem of energy poverty and improving energy use efficiency as a whole. In addition, the impact of the urbanization rate and technology development on the inter-regional gap of energy poverty is significantly negative, which may be because the degree of energy poverty and the level of economic development of provinces and cities in China are not completely consistent. The level of energy poverty cannot be evaluated solely by the level of economic development, which is also consistent with the conclusion obtained from the previous spatial distribution analysis. Furthermore, the results of regional heterogeneity analysis show that there are also differences in the dominant factors determining the differences in energy poverty within the eastern and western regions.
The results of the time dynamics test show that with the development of the regional economy, the living needs of residents are gradually met, and energy gradually becomes a low-end consumer good in the consumption system, so the impact of energy prices and energy investment on regional disparities in energy poverty becomes insignificant. However, the level of urbanization, technological development, and industrial structure still play an essential role in regional energy poverty disparity. It indicates that the continuous optimization of the energy consumption structure of residents in China, improving scientific and technological innovation, promoting the energy production and consumption revolution, and developing renewable energy are the necessary ways to solve the energy poverty problem nowadays.
The above findings have some policy implications. To be sure, the significant reduction and complete elimination of energy poverty still need the attention of the government and the introduction and implementation of specific policies.
First, according to the findings of the article, there is significant spatial variability in the extent of energy poverty across provinces, and there are differences in the dominant causes of energy poverty between regions. Therefore, when formulating energy poverty mitigation policies, regional differences should be fully considered to grasp the leading causes and main contradictions of energy poverty in each province and city. For example, the western region can vigorously promote the use of photovoltaic and wind power equipment. In contrast, the central region should highlight the rational transformation of straw resources, promote the diversified use of rural biomass energy, and accelerate the construction of a renewable energy-based rural clean energy use system.
Second, we should pay attention to the inconsistency between energy poverty and economic poverty, as this paper emphasizes. When choosing the target of energy poverty alleviation policy and deciding the proportion of inputs, it should be distinguished from other related policies that promote economic and social development. The implementation of energy poverty alleviation policies and investment targets should not focus on economically poor areas, but should fully consider the current situation of energy poverty in each province, which also reflects the significance of energy poverty assessment.
Third, we should vigorously promote the technical cooperation between enterprises, universities, research departments, and government departments to strengthen the research of new technologies and products of low-carbon and energy-saving. In particular, developed regions should encourage enterprises to develop core technologies, low-energy high-end industries and form an optimized sustainable development model.
Fourth, a financial guarantee mechanism should be established to give certain tax incentives to clean energy industries and products. Especially in less developed areas, appropriate policy support can be provided to promote the cultivation and development of clean energy industries. Specifically, the government can organize the construction of rural clean energy demonstration projects and conditionally develop clean energy-related industries, which can not only facilitate the transformation of energy use structure but also provide more local employment opportunities in the clean energy industry. Finally, rural residents should be reasonably guided to consume modern living energy and encouraged to develop low-carbon and green consumption habits. At present, rural areas in China have basically achieved full coverage of electricity, but some residents still consume relatively little electricity due to factors such as income and living habits. It is an important means to alleviate energy poverty in China by guiding the rural residents to use electricity to meet their living needs and promoting clean energy alternatives at the household level, thus reducing traditional solid energy consumption. In the future, this paper can expand the direction. First, expand the data from the provincial level to the city level, and examine the energy poverty problem at the urban level in China. Second, after examining the regional disparity of energy poverty, we further analyze the distribution dynamics and convergence of energy poverty.
The original contributions presented in the study are included in the article/supplementary material, further inquiries can be directed to the corresponding author.
WJ: Conceptualization, Data curation, Methodology SW: Software, Writing—Reviewing and Editing.
This work is financially supported by the Shanghai University of Finance and Economics Innovation Team Project (Grant No. 2016110393); the Fundamental Research Funds for the Central Universities (Grant No. CXJJ-2020-307). Shanghai University of Finance and Economics 2021 Graduate Innovation Fund Project (Grant No.2019310074).
The authors declare that the research was conducted in the absence of any commercial or financial relationships that could be construed as a potential conflict of interest.
All claims expressed in this article are solely those of the authors and do not necessarily represent those of their affiliated organizations, or those of the publisher, the editors and the reviewers. Any product that may be evaluated in this article, or claim that may be made by its manufacturer, is not guaranteed or endorsed by the publisher.
Abánades, A. (2018). Natural Gas Decarbonization as Tool for Greenhouse Gases Emission Control. Front. Energy Res. 6, 47. doi:10.3389/fenrg.2018.00047
Adkins, E., Eapen, S., Kaluwile, F., Nair, G., and Modi, V. (2010). Off-Grid Energy Services for the Poor: Introducing Led Lighting in the Millennium Villages Project in Malawi. Energy Policy 38 (2), 1087–1097. doi:10.1016/j.enpol.2009.10.061
Barnett, G. A. (2011). “Encyclopedia of Social Networks,” in Encyclopedia of Social Networks (Thousand Oaks, CA: Sage Publications). doi:10.4135/9781412994170
Baumgartner, J., Schauer, J. J., Ezzati, M., Lu, L., Cheng, C., Patz, J. A., et al. (2011). Indoor Air Pollution and Blood Pressure in Adult Women Living in Rural China. Environ. Health Perspect. 119 (10), 1390–1395. doi:10.1289/ehp.1003371
Bazilian, M., Sagar, A., Detchon, R., and Yumkella, K. (2010). More Heat and Light. Energy Policy 38 (10), 5409–5412. doi:10.1016/j.enpol.2010.06.007
Berry, A. (2018). “Measuring Energy Poverty: Uncovering the Multiple Dimensions of Energy Poverty. Working Paper, HAL archives-ouvertes.
Borgatti, S. P., Everett, M. G., and Freeman, L. C. (2014). UCINET for Windows: Software for Social Network Analysis. Harvard: Analytic Technologies.
Chapman, R. S., He, X., Blair, A. E., and Lan, Q. (2005). Improvement in Household Stoves and Risk of Chronic Obstructive Pulmonary Disease in Xuanwei, China: Retrospective Cohort Study. BMJ-British Med. J. 331 (7524), 1050–1052A. doi:10.1136/bmj.38628.676088.55
Che, X., Zhu, B., and Wang, P. (2021). Assessing Global Energy Poverty: An Integrated Approach. Energy Policy 149, 112099. doi:10.1016/j.enpol.2020.112099
Christakis, N. A., and Fowler, J. H. (2009). Connected: The Surprising Power of Our Social Networks and How They Shape Our Lives. New York, NY: Little, Brown Spark.
Davis, M. (1998). Rural Household Energy Consumption: The Effects of Access to Electricity—Evidence from South Africa. Energy Policy 26 (3), 207–217. doi:10.1016/S0301-4215(97)00100-6
Duflo, E., Greenstone, M., and Hanna, R. (2008). Indoor Air Pollution, Health and Economic Well-Being. Surv. Perspect. Integrating Environ. Soc. 1 (1), 1–9. doi:10.5194/sapiens-1-1-2008
Hosier, R. H., and Dowd, J. (1987). Household Fuel Choice in Zimbabwe: An Empirical Test of the Energy Ladder Hypothesis. Resour. Energy 9 (4), 347–361. doi:10.1016/0165-0572(87)90003-X
Jiang, L., Yu, L., Xue, B., Chen, X., and Mi, Z. (2020). Who is Energy Poor? Evidence from the Least Developed Regions in China. Energy Policy 137, 111122. doi:10.1016/j.enpol.2019.111122
Khanna, R. A., Li, Y., Mhaisalkar, S., Kumar, M., and Liang, L. J. (2019). Comprehensive Energy Poverty Index: Measuring Energy Poverty and Identifying Micro-Level Solutions in South and Southeast Asia. Energy Policy 132, 379–391. doi:10.1016/j.enpol.2019.05.034
Kim, C., Seow, W. J., Shu, X.-O., Bassig, B. A., Rothman, N., Chen, B. E., et al. (2016). Cooking Coal Use and All-Cause and Cause-Specific Mortality in a Prospective Cohort Study of Women in Shanghai, China. Environ. Health Perspect. 124 (9), 1384–1389. doi:10.1289/ehp236
Kooijman-van Dijk, A. L. (2012). The Role of Energy in Creating Opportunities for Income Generation in the Indian Himalayas. Energy Policy 41, 529–536. doi:10.1016/j.enpol.2011.11.013
Lacey, F. G., Henze, D. K., Lee, C. J., van Donkelaar, A., and Martin, R. V. (2017). Transient Climate and Ambient Health Impacts Due to National Solid Fuel Cookstove Emissions. Proc. Natl. Acad. Sci. U.S.A. 114 (6), 1269–1274. doi:10.1073/pnas.1612430114
Leach, G. (1992). The Energy Transition. Energy Policy 20 (2), 116–123. doi:10.1016/0301-4215(92)90105-B
Leitão, N. C. (2021). The Effects of Corruption, Renewable Energy, Trade and Co2 Emissions. Economies 9 (2), 62. doi:10.3390/economies9020062
Li, K., Wang, K., and Wang, Y. X. (2014). Comprehensive Evaluation of Regional Energy Poverty in China. J. Beijing Inst. Tech. Soc. Sci. Ed. 16, 1–12. (in Chinese). doi:10.15918/j.jbitss1009-3370.2014.02.015
Li, S. X., and Li, L. J. (2020). Regional Differences and Influencing Factors of Rural Energy Poverty in China. J. Agric. For. Econ. Manag. 19, 210–217. (in Chinese). doi:10.16195/j.cnki.cn36-1328/f.2020.02.22
Lim, S. S., Vos, T., Flaxman, A. D., Danaei, G., Shibuya, K., Adair-Rohani, H., et al. (2012). A Comparative Risk Assessment of Burden of Disease and Injury Attributable to 67 Risk Factors and Risk Factor Clusters in 21 Regions, 1990-2010: A Systematic Analysis for the Global Burden of Disease Study 2010. Lancet 380 (9859), 2224–2260. doi:10.1016/s0140-6736(12)61766-8
Lin, B., and Zhao, H. (2021). Does Off-Farm Work Reduce Energy Poverty? Evidence from Rural China. Sustain. Prod. Consum. 27, 1822–1829. doi:10.1016/j.spc.2021.04.023
Liu, J., Hou, B., Ma, X.-W., and Liao, H. (2018). Solid Fuel Use for Cooking and its Health Effects on the Elderly in Rural China. Environ. Sci. Pollut. Res. 25 (4), 3669–3680. doi:10.1007/s11356-017-0720-9
Liu, Z. M., Deng, M. Y., Cui, Z. W., and Cao, H. (2020). Impact of Energy Poverty on the Welfare of Residents and its Mechanism: An Analysis Based on CGSS Data. China Soft Sci. 356 (08), 143–163. (in Chinese). doi:10.3969/j.issn.1002-9753.2020.08.014
Lu, S., Ren, J., Lee, C. K. M., and Zhang, L. (2022). Spatial-Temporal Energy Poverty Analysis of China from Subnational Perspective. J. Clean. Prod. 341, 130907. doi:10.1016/j.jclepro.2022.130907
Mohr, T. M. (2018). Fuel Poverty in the Us: Evidence Using the 2009 Residential Energy Consumption Survey. Energy Econ. 74, 360–369. doi:10.1016/j.eneco.2018.06.007
Muller, C., and Yan, H. (2018). Household Fuel Use in Developing Countries: Review of Theory and Evidence. Energy Econ. 70, 429–439. doi:10.1016/j.eneco.2018.01.024
Nagpaul, P. S. (2003). Exploring a Pseudo-Regression Model of Transnational Cooperation in Science. Scientometrics 56 (3), 403–416. doi:10.1023/A:1022335021834
Ogwumike, F. O., and Ozughalu, U. M. (2016). Analysis of Energy Poverty and its Implications for Sustainable Development in Nigeria. Envir. Dev. Econ. 21 (3), 273–290. doi:10.1017/s1355770x15000236
Papada, L., and Kaliampakos, D. (2018). A Stochastic Model for Energy Poverty Analysis. Energy Policy 116, 153–164. doi:10.1016/j.enpol.2018.02.004
Primc, K., and Slabe-Erker, R. (2020). Social Policy or Energy Policy? Time to Reconsider Energy Poverty Policies. Energy Sustain. Dev. 55, 32–36. doi:10.1016/j.esd.2020.01.001
Sadath, A. C., and Acharya, R. H. (2017). Assessing the Extent and Intensity of Energy Poverty Using Multidimensional Energy Poverty Index: Empirical Evidence from Households in India. Energy Policy 102, 540–550. doi:10.1016/j.enpol.2016.12.056
Sinton, J. E., Smith, K. R., Peabody, J. W., Yaping, L., Xiliang, Z., Edwards, R., et al. (2004). An Assessment of Programs to Promote Improved Household Stoves in China. Energy Sustain. Dev. 8 (3), 33–52. doi:10.1016/S0973-0826(08)60465-2
Stanton, M. A., and Mann, J. (2014). “Social Network Analysis: Applications to Primate and Cetacean Societies,” in Primates and Cetaceans. Editors J. Yamagiwa, and L. Karczmarski (Tokyo: Springer). doi:10.1007/978-4-431-54523-1_18
Sun, W., Han, X. X., and Liang, Y. T. (2014). The Identification Method of Energy Poverty and Analysis of its Application: A Case Study of Nujiang Prefecture in Yunan Province. J. Nat. Resour. 29 (04), 575–586. (in Chinese). doi:10.11849/zrzyxb.2014.04.003
Tang, X., and Liao, H. (2014). Energy Poverty and Solid Fuels Use in Rural China: Analysis Based on National Population Census. Energy Sustain. Dev. 23, 122–129. doi:10.1016/j.esd.2014.08.006
Tsai, W. (2002). Social Structure of “Coopetition” within a Multiunit Organization: Coordination, Competition, and Intraorganizational Knowledge Sharing. Organ. Sci. 13 (2), 179–190. doi:10.1287/orsc.13.2.179.536
Wang, B., Li, H.-N., Yuan, X.-C., and Sun, Z.-M. (2017). Energy Poverty in China: A Dynamic Analysis Based on a Hybrid Panel Data Decision Model. Energies 10 (12), 1942. doi:10.3390/en10121942
Wu, W.-P., Zeng, W.-K., Gong, S.-W., and Chen, Z.-G. (2021). Does Energy Poverty Reduce Rural Labor Wages? Evidence from China's Rural Household Survey. Front. Energy Res. 9, 8. doi:10.3389/fenrg.2021.670026
Yang, J., and Mukhopadhaya, P. (2017). Disparities in the Level of Poverty in China: Evidence from China Family Panel Studies 2010. Soc. Indic. Res. 132 (1), 411–450. doi:10.1007/s11205-016-1228-2
Yun, X., Shen, G., Shen, H., Meng, W., Chen, Y., Xu, H., et al. (2020). Residential Solid Fuel Emissions Contribute Significantly to Air Pollution and Associated Health Impacts in China. Sci. Adv. 6 (44), 7. doi:10.1126/sciadv.aba7621
Zagenczyk, T. J., Purvis, R. L., Shoss, M. K., Scott, K. L., and Cruz, K. S. (2015). Social Influence and Leader Perceptions: Multiplex Social Network Ties and Similarity in Leader-Member Exchange. J. Bus. Psychol. 30 (1), 105–117. doi:10.1007/s10869-013-9332-7
Zhang, D., Li, J., and Han, P. (2019). A Multidimensional Measure of Energy Poverty in China and its Impacts on Health: An Empirical Study Based on the China Family Panel Studies. Energy Policy 131, 72–81. doi:10.1016/j.enpol.2019.04.037
Zhang, J., Liu, Y., Zhang, W., and Lin, X. (2021). Study on the Impact of Energy Poverty on Energy Efficiency of Construction Industry: Mediating Role of Energy Consumption Structure. Front. Energy Res. 9, 12. doi:10.3389/fenrg.2021.760895
Zhang, X.-B., and Hassen, S. (2017). Household Fuel Choice in Urban China: Evidence from Panel Data. Envir. Dev. Econ. 22 (4), 392–413. doi:10.1017/s1355770x17000092
Zhao, X. Y., Chen, H. H., Ma, Y. Y., Gao, Z. Y., and Xue, B. (2018). Spatio-Temporal Variation and its Influencing Factors of Rural Energy Poverty in China from 2000 to 2015. Geogr. Res. 37 (06), 1115–1126. (in Chinese). doi:10.11821/dlyj201806005
Keywords: energy poverty, regional difference, influencing factors, relational data, solid fuel, energy consumption
Citation: Jia W and Wu S (2022) Spatial Differences and Influencing Factors of Energy Poverty: Evidence From Provinces in China. Front. Environ. Sci. 10:921374. doi: 10.3389/fenvs.2022.921374
Received: 15 April 2022; Accepted: 16 May 2022;
Published: 06 June 2022.
Edited by:
Gagan Deep Sharma, Guru Gobind Singh Indraprastha University, IndiaCopyright © 2022 Jia and Wu. This is an open-access article distributed under the terms of the Creative Commons Attribution License (CC BY). The use, distribution or reproduction in other forums is permitted, provided the original author(s) and the copyright owner(s) are credited and that the original publication in this journal is cited, in accordance with accepted academic practice. No use, distribution or reproduction is permitted which does not comply with these terms.
*Correspondence: Shengnan Wu, c2hhbm5hbjA1MDFAMTYzLmNvbQ==
Disclaimer: All claims expressed in this article are solely those of the authors and do not necessarily represent those of their affiliated organizations, or those of the publisher, the editors and the reviewers. Any product that may be evaluated in this article or claim that may be made by its manufacturer is not guaranteed or endorsed by the publisher.
Research integrity at Frontiers
Learn more about the work of our research integrity team to safeguard the quality of each article we publish.