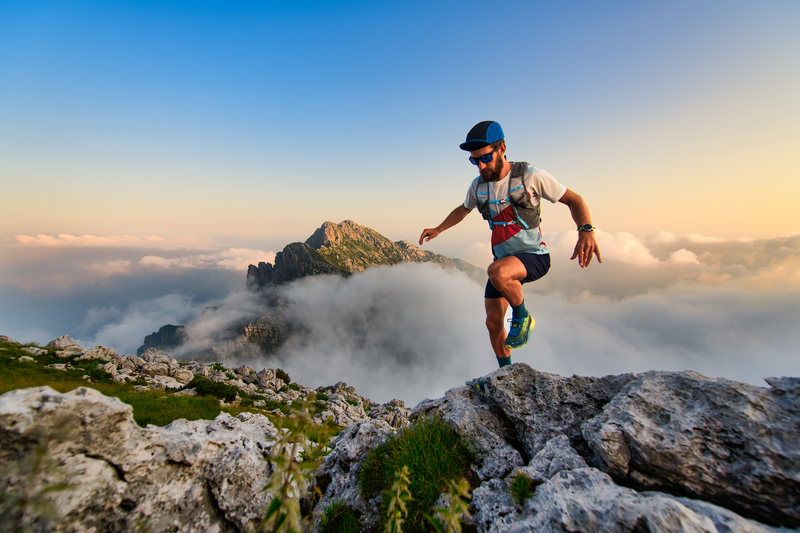
95% of researchers rate our articles as excellent or good
Learn more about the work of our research integrity team to safeguard the quality of each article we publish.
Find out more
ORIGINAL RESEARCH article
Front. Environ. Sci. , 29 June 2022
Sec. Conservation and Restoration Ecology
Volume 10 - 2022 | https://doi.org/10.3389/fenvs.2022.917597
This article is part of the Research Topic Effects of Different Forms of Management or Patterns of Climate Change on the Grassland Ecosystem View all 7 articles
Shrub expansion has been reported mostly in dry and semi-arid ecosystems worldwide. Shrub expansion is also a serious ecological issue in alpine and cold regions. Multiple drivers, including anthropogenic and environmental factors, contribute to this phenomenon. However, the relative effects of climate and soil factors on shrub expansion are incompletely quantified or understood in the alpine meadow, and quantifying how these factors result in variations in functional traits associated with shrub expansion is crucial considering that functional traits are tightly related to ecosystem processes. In this study, we investigated the vegetation of an alpine shrub meadow composed of Potentilla fruticosa L. In the Zoige Plateau, along an elevation gradient. We assessed the direct and indirect effects of climate and soil factors on functional traits, shrub expansion strength (measured as the total biomass), and relative abundance (via functional traits). We found that climate factors, namely, the mean temperature of the warmest quarter (bio10), mostly affected specific leaf areas and specific lobe volume, which were the most important traits related to shrub expansion. Soil factors, except soil water content (SWC), had weak effects on functional traits closely related to shrub expansion. Our partial least square path modeling model explained 99% of the variation in shrub biomass. Results suggest that climate change not only affected functional traits but also influenced shrub expansion in the Zoige Plateau. Thus, management measures to control expansion should consider these drivers for more accurate forecasting and cost effectiveness. Understanding the mechanism of alpine shrub expansion contributes to the delaying of the expansion process and ensures steady pastoral production.
Shrub expansion is defined as the increase in density, cover, and biomass of native woody species (Van Auken, 2009). It has been reported mostly in dry and semi-arid ecosystems (Eldridge et al., 2011; Zhao et al., 2021). Shrub expansion is also a serious ecological issue in alpine and cold regions (Brandt et al., 2013; Gao et al., 2016). Shrub expansion has been constantly regarded as an ecological expression of degradation or desertification according to considerable literature (MEa, 2005), which is mostly from the viewpoint of pastoral production. Shrublands are often regarded to have less pastoral values than grasslands because they pose substantial management challenges to pastoralists and livestock managers, such as greater competition for forage (Morgan et al., 2007) and difficulty mustering livestock (Eldridge et al., 2011). However, they have been reported to increase carbon sequestration (Li et al., 2016; Gobelle and Gure, 2018), net ecosystem productivity (Knapp et al., 2008), soil fertility, and nitrogen mineralization rate (Maestre et al., 2009; Gómez-Rey et al., 2013). Although the impact of expansion on ecosystems is controversial, a consensus revealed that expansion negatively affects livestock production. Shrub expansion resulted from several factors that were difficult to entangle, including human-induced factors (Coetzee et al., 2008) and environmental factors (Eldridge et al., 2011) such as overgrazing and recovery from human disturbance, fire frequency, availability of water, spatial distribution of soil resources, climate change, CO2, and nitrogen deposition. Recent studies have suggested that functional traits of woody species, which impact the fitness of individual species via their effects on growth, reproduction, and survival (Bu et al., 2019), play an important role in shrub expansion (Collins et al., 2020; Eldridge and Ding, 2021), such as life cycle, plant height, specific leaf area, and root depth.
Previous studies have focused on the effect of a single factor on shrub expansion. Some of them suggested that rising annual temperature resulted in greater shrub productivity (Gao et al., 2016); others found that shrub expansion was mostly associated with changes in soil conditions (Liu et al., 2022). By comparing dry and semi-arid ecosystems, the mechanisms of shrub expansion in alpine areas have received less attention. The few studies that have been published mostly focused on the effects of climate factors (Gao et al., 2016) and grazing (Komac et al., 2013) on shrub expansion, while functional traits were rarely considered. These conditions highlight the need to further explore the influencing factors of shrub expansion in alpine grasslands, especially the role played by functional traits. To the best of our knowledge, no one has quantified jointly the relative effects of space, climate, and soil factors on shrub expansion and associated changes in functional traits in the Qinghai–Tibetan plateau.
The alpine shrub meadow is one of the typical grassland ecosystems on the Qinghai–Tibetan plateau (Fu et al., 2018). The major alpine shrub on the plateau is Potentilla fruticosa L. (P. fruticosa), which is distributed widely from 3,000 m to 4,500 m (Li et al., 2010). The alpine shrub is sensitive to climate change. Considering global warming, the biomass and productivity of P. fruticosa will increase in the Qinghai–Tibetan Plateau (Yashiro et al., 2010). Our previous study found that functional traits of P. fruticosa, such as plant height, leaf area, abundance, and biomass, varied greatly at different altitudes and habitats (Su et al., 2020).
Differences in climate and soil conditions make the Qinghai–Tibetan Plateau an ideal place to study the effects of various factors on shrub expansion. The mechanism of alpine shrub expansion is critical for delaying the expansion process and ensuring steady pastoral production. Thus, we assessed i) the influence of climate and soil factors on functional traits and ii) the direct and indirect effect of these factors on expansion strength (via functional traits). The expansion strength of the shrub was measured as the total biomass. Notably, the shrub expansion is stronger when the total biomass is larger (Wolf et al., 2021). We also studied the leaf, stem, root, and reproductive traits of shrub plants related to plant establishment, persistence, regeneration, and dispersal, which can provide clues about shrub expansion strength. Distinguishing which functional traits are primarily influenced by local environmental factors is also critical. Our first hypothesis is that local soil factors, as well as climate factors, are drivers of shrub expansion. Our second hypothesis is that shrub relative abundance will change in response to environmental changes via affecting functional traits, namely, asexual reproduction traits.
The study was conducted in the alpine meadow area of the Zoige Plateau (33°10′ to 34°06′N, 101°36′ to 103°25′E) in the northeastern corner of the Qinghai–Tibetan Plateau (Figure 1). This region has a typical humid and semi-humid continental monsoon climate of the plateau cold temperate zone (Bai et al., 2013). More rain and heat are observed in the same season, and the air temperature swings greatly from day to night. The annual mean air temperature ranges between 0.6 and 1.2°C, with a long frost season. The annual mean precipitation is 600–800 mm, while the annual evaporation is higher than the annual mean precipitation (Ding et al., 2021). The representative soil types of the Zoige wetland include meadow soil, marsh soil, peat soil, and sandy soil (Xiang et al., 2009; Zhang and Liu, 2009). The predominant vegetation type is an alpine shrub-meadow having a two-layer structure, with P. fruticosa as the dominating species in the upper layer and Korbresia humilis as the prominent species in the lower layer. The grassland vegetation mainly includes P. fruticose, Kobresia tibetica, Muli sedge, Carex lasiocarpa, and Ligularia virgaurea.
In August 2018, we selected three permanent meadows dominated by P. fruticosa in the Zoige Plateau, with an elevation range from 3,000 m asl to 4,000 m asl (Figure 1), and these areas are the important pastures for summer grazing of Tibetan sheep from June to October (Zhou et al., 2005). For each meadow, three plots with the size of 10 × 10 m2 were randomly selected to measure the functional traits of P. fruticosa and soil data, and 10 m was considered between adjacent plots. Three quadrats (1 × 1 m2) were set up along the diagonal (two ends and one midpoint) line of each plot. In July 2020, we recorded the relative abundance and plant height of P. fruticosa at the center and four corners of each quadrat. Furthermore, five leaves of P. fruticosa were collected from healthy plants with a similar degree of development within each plot. A total of 135 intact and clean leaves (3 meadows × 3 plots × 3 quadrats × 5 leaves) were collected. These leaves were then used to measure the leaf water content, the specific leaf area (SLA), and the specific lobe volume (SLV). After that measurement, the leaves were crushed to determine the concentration of C, N, and P elements. The total biomass, root biomass, and plant bud characteristics were measured by digging up the 135 plants (3 meadows × 3 plots × 3 quadrats × 5 plants) of P. fruticosa. Five soil cores were collected at 30 cm radial distances from the ground at the center and four corners of each quadrat. A total of 135 soil samples (3 meadows × 3 plots × 3 quadrats × 5 soil cores) were collected. Soil samples were then divided into two parts. One part was used to measure soil water content (SWC), while the other one was used to determine the physical and chemical characteristics.
We used a scanner (Canon Scan Lide 110, Canon, Japan) to scan plant leaves. Plant leaf area (S, cm2) was then determined by Image J (version 1.47 v, Wayne Rasband National Institutes of Health, United States) software (Abràmoff et al., 2004). Leaf volume (V, cm3) was determined by the drainage method.
The fresh weight of leaves (m1, g) was weighed on an electronic balance with an accuracy of 0.1 g. These leaves were then completely soaked in deionized water for 24 h in the dark until saturation. After the soak, the water on the surface of the leaves was quickly absorbed with bibulous paper, and the saturated fresh weight of the leaves (m2, g) was weighed. Finally, these leaves were placed in a weighing bottle, dried at 105°C for 30 min, and then dried at 70°C for 24 h to a constant weight, and the dry weight (m3, g) of the leaves was determined.
Soil samples were dried at room temperature in a darkened environment, the impurities were removed, and the soil was ground and filtered through a 2 mm-mesh sieve. Physical and chemical characteristics were then measured using these samples. Soil organic matter was quantified using the potassium dichromate volumetric method (Nelson and Sommers, 1983); total organic carbon (TOC) and leaf carbon concentration (C P. fruticosa) were measured using a high-temperature combustion method; soil total nitrogen and leaf nitrogen concentration (N P. fruticosa) were analyzed by the semi-micro-Kjeldahl method (Bao, 2000); alkali-hydrolyzable nitrogen was determined using alkaline hydrolysis process; nitrate-nitrogen (NO3+-N) was determined by Nessler’s reagent spectrophotometry; flame photometry was used to measure available potassium in 1 M ammonium acetate extracts (FP640, INASA); soil total phosphorus (TP), available phosphorus (AP), and leaf phosphorus concentration (PP. fruticosa) were determined by the molybdenum blue method (Crouch and Malmstadt, 1967). The core cutter method was used to determine SWC. Soil pH was assessed using a pH meter in a 1:2.5 (mass: volume) soil–water suspension.
Climate data for the period of 1950–2000 was calculated from the WorldClim database (http://www.worldclim.org/) using geographic coordinates with a spatial resolution of 1 km × 1 km to explore the effect of climate factors on shrub expansion strength.
The SLA and SLV were calculated according to the following formulas (Marenco et al., 2009):
The leaf moisture trait index was calculated according to the following formulas (Marenco et al., 2009):
The environmental factors include soil and climate factors. The Pearson correlation coefficient was used to select variables with a correlation coefficient of less than 0.8 for multiple linear regression model analysis for reducing the overfitting issue caused by the collinearity between soil variables. The soil variables of the deciduous shrubs including TOC, SWC, TP, pH, AK, AP, and NO3+-N were selected. Then, we selected climate factors that affected the growth and development of P. fruticosa, including temperature variables: bio1, annual mean temperature; bio4, temperature seasonality; bio5, the max temperature of the warmest month; bio10, mean temperature of the warmest quarter; and precipitation variables: bio13, precipitation of the wettest month; bio15, precipitation seasonality; bio16, precipitation of the wettest quarter. Plant functional traits included leaf traits (TWC, DWWC, RWC, SLA, SLV, C P. fruticosa, PP. fruticosa, and N P. fruticosa), stem traits (plant height), root traits (root–top ratio), and reproductive traits (tillering bud and long rhizome bud).
Considering that the environmental indicators include soil and climate factors, two new indicators, namely, EVPC1 and EVPC2, were generated by principal component analysis (PCA) to simplify the analysis. Plant functional traits were simplified to LFTVPC1 and LFTVPC2. Two linear mixed models (LMMs) were run with environmental variables EVPC1 and EVPC2 as the fixed main effects to holistically assess the impact of environmental factors on functional traits. The response variable was either PTVPC1 or PTVPC2, and the plot was included as a random effect. We ran a very similar mixed-effect model to examine the impact of functional traits on shrub expansion strength. The explained variables in the model were PTVPC1 and PTVPC2, and the plot was included as the random effect. Meanwhile, the total biomass and relative abundance were the response variables.
After the abovementioned analysis, correlation analysis (Spearman correlation) and a multiple linear regression model combined with variation partitioning analysis were used to evaluate the contribution of various environmental variables to each functional trait of P. fruticosa, and the main environmental variables were identified.
Plspm is an R package for performing partial least square path modeling (PLS-PM) analysis, which especially suits situations when data are not normally distributed. Plspm package can be used for placing in relation a model of the latent variable and the observed variable modeling. In our study, PLS-PM was used to estimate direct and indirect pathways that affect the biomass and relative abundance of P. fruticosa. We found that numerous soil variables insignificantly affected functional traits (details were shown in the LMM, and multiple linear regression models). As a result, the PLS-PM model did not contain soil factors. PLS-PM is divided into two sub-models termed the inner and outer models (Senner and Coddington, 2011). The outer model describes the relationships between a group of observed variables and a synthetic latent variable, and the inner model describes the relationships of latent variables. Our PLS-PM model contained six latent variables: 1) space factor, including elevation; 2) climate factors, including bio1, bio4, bio5, and bio10; 3) leaf traits, including SLA and SLV; 4) root trait, covering the root–top ratio; 5) shrub abundance, including relative abundance; and 6) shrub expansion strength, revealed by the total biomass. The coefficient of determination (R2) and goodness of fit (GoF) were used to assess the overall robustness of the models (Akter et al., 2011).
All analyses were performed in R 3.6.0 (R Core Team, 2013) with an alpha value of p < 0.05 for all significance tests. Mixed-effect models were run using the lme4 package (Liu et al., 2018), and slopes were extracted using the reghelper package (Hughes and Beiner, 2021). The PCA was completed with the princomp function (Pratt et al., 2021). The Pearson correlation analysis was performed by the (cor. test) function in the stats package (Field et al., 2012). The multiple regression model (lm) function in the stats package (Field et al., 2012) with variation decomposition analysis (calc.relimp) function in the relaimpo package (Grömping, 2015) was applied to identify the main environmental variables. Structural equation modeling was run by the plspm package (Sanchez et al., 2015).
This study found significant differences in bio1, bio5, bio10, bio13, bio16, TWC, DWWC, RWC, DMC, SLA, and SLV, CP. fruticose, plant height, root–top ratio, long rhizome bud, tillering bud, relative abundance, and total biomass between sampling sites (Table 1). S1 had the largest values in bio1, bio5, bio10, bio13, TWC, DWWC, RWC, SLA, SLV, C P. fruticose, plant height, tillering bud, and total biomass; however, it had the smallest values in bio16, DMC, root–top ratio, long rhizome bud, and relative abundance (Table 1).
PCA of environmental factors decomposed numerous variables into two composite variables. The principal component explained 77.68% of the variation after dimensionality reduction (Figure 2).
FIGURE 2. Results of the principal components analysis (PCA) examining the distribution of environment variables across the experimental site. Arrows indicate directions of loading for each variable. NO3+-N, soil nitrate-nitrogen; AP, soil available phosphorus; AK, soil available potassium; pH, pH of the soil in 1:2.5 (mass:volume), TP, soil total phosphorus, SWC, soil water content, TOC, soil total organic carbon. bio1, annual mean temperature; bio4, temperature seasonality; bio5, the max temperature of the warmest month; bio10, mean temperature of the warmest quarter; bio13, precipitation of the wettest month; bio15, precipitation seasonality; bio16, precipitation of wettest quarter.
The first axis of the PCA (EVPC1) was mostly aligned with climate variables. Positive scores on EVPC1 reflected cool and wet environments, and they had less seasonal variation in temperature (particularly in the temperature seasonality, bio4) than negative scores; meanwhile, negative values were associated with warm and dry environments, and they had less seasonal variation in precipitation (particularly in the precipitation seasonality, bio15) than positive scores (Figure 2). The second axis of the PCA (EVPC2) was mostly associated with soil variables. Positive values of EVPC2 were associated with AK and SWC, whereas negative values were associated with NO3+-N, TOC, AP, and TP (Figure 2). The respective differences in explained variation of EVPC1 (56.54%) and EVPC2 (21.14%) indicated that the variation explained by the climate variables was nearly three times that of the soil variables (Figure 2). The differences between sampling points were mainly in the climate variables.
Many functional traits were decomposed into two composite variables using PCA. The first two axes of the principal component explained 87.33% of the variation (Figure 3). The first axis of the PCA (PTVPC1) was largely aligned with morphological traits (Figure 3). Positive scores on the first axis of the principal component analysis (PTVPC1) were associated with the root–top ratio, DMC, and long rhizome bud. Negative scores of PTVPC1 were related to high leaf water content, plant height, SLA and SLV (Figure 3). The second axis of the PCA (PTVPC2) was linked with leaf nutrient traits (Figure 3). The difference in explained variation between PTVPC1 (78.27%) and PTVPC2 (9.56%) indicates that plant morphological traits explain more than nine times the variation explained by leaf nutrient traits (Figure 3). The functional trait differences between plants collected from various sampling points were mainly in PTVPC1.
FIGURE 3. Results of the PCA examining the distribution of plant trait variables across the experimental site. Arrows indicate directions of loading for each variable. TWC, total water content; DWWC, dry weight water content; RWC, relative water content; DMC, dry matter content; SLA, the specific leaf area; SLV, specific lobe volume; CP. fruticosa, leaf carbon concentration; P。 fruticosa, leaf phosphorus concentration; N P. fruticosa, leaf nitrogen concentration; Plant height, plant height of P. fruticosa; Root–top ratio, root to aboveground biomass ratio; Long rhizome bud, long rhizome bud of P. fruticosa; Tillering bud, tillering bud of P. fruticosa.
PTVPC1 was influenced by EVPC1 significantly and positively (F = 354.20, slope = 0.116, p < 0.001), and the proportion of variation explained was 99.62%; meanwhile, PTVPC1 was not significantly influenced by EVPC2 (p > 0.05) (Table 2). PTVPC2 was insignificantly affected by EVPC1 or EVPC2 (p-values were 0.656 and 0.774, respectively), as observed in Table 2.
TABLE 2. Linear mixed model results of the effects of environmental factors on functional traits of P. fruticosa. p-values less than 0.05 were marked in bold. EVPC1, the first axis of the PCA of environmental factors; EVPC2, the second axis of the PCA of environmental factors; PTVPC1, the first axis of the PCA of functional traits; PTVPC2, the second axis of the PCA of functional traits.
Environmental variables significantly explained all plant traits except PP. fruticosa. Leaf traits were mostly influenced by AP, SWC, bio13, and bio1 (Figure 4). Leaf water traits and DMC were significantly predicted by SWC and bio13; leaf size and thickness (SLA and SLV) were significantly affected by bio10; N P. fruticosa was significantly explained by TP and bio15; stem trait (plant height) was significantly explained by SWC, TOC, bio1, and bio10; root trait (root–top ratio) was significantly influenced by bio16; and reproductive trait (tillering bud) was significantly explained by bio13 (Figure 4).
FIGURE 4. Contribution of environmental variables to each functional trait of P. fruticosa. Spearman correlations between environmental variables and functional traits are shown by colors. The size of the circle reflected variation explained the rate of the selected variable. The significance of each functional trait explained by environmental variables is indicated by the asterisks above the bar (*p<0.05, **p<0.01, ***p<0.001). NO3+-N, soil nitrate-nitrogen; AP, soil available phosphorus; AK, soil available potassium; pH, pH of the soil in 1:2.5 (mass:volume), TP, soil total phosphorus, SWC, soil water content, TOC, soil total organic carbon. bio1, annual mean temperature; bio4, temperature seasonality; bio5, the max temperature of the warmest month; bio10, mean temperature of the warmest quarter; bio13, precipitation of wettest month; bio15, precipitation seasonality; bio16, precipitation of wettest quarter. TWC, total water content; DWWC, dry weight water content; RWC, relative water content; DMC, dry matter content; SLA, the specific leaf area; SLV, specific lobe volume; C P. fruticosa., leaf carbon concentration; P。fruticosa, leaf phosphorus concentration; N P. fruticosa, leaf nitrogen concentration; Plant height, plant height of P. fruticosa; Root–top ratio, the ratio of root to aboveground biomass; Long rhizome bud, long rhizome bud number of P. fruticosa; Tillering bud, tillering bud number of P. fruticosa.
Biomass was influenced by PTVPC1 significantly and negatively (F = 655.68, slope = −0.103, p = 0.001), and the proportion of variation explained was 99.84% (Table 3). Conversely, PTVPC2 significantly positively affected the biomass (F = 28.37, slope = 0.009, p = 0.038), despite the fact that the proportion of variation explained was only 0.16% (Table 3). PTVPC1 significantly positively affected the relative abundance (F = 1,126.43, slope = 0.421, p < 0.001), which accounted for 94.33% of the variation; meanwhile, PTVPC2 significantly negatively affected the relative abundance (F = 655.68, slope = −0.373, p = 0.043), which explained 5.67% of the variation (Table 3).
TABLE 3. Linear mixed model results of the effects of functional traits on total biomass and relative abundance of P. fruticosa. The p-values less than 0.05 are in bold. PTVPC1, the first axis of the PCA of functional traits; PTVPC2, the second axis of the PCA of functional traits.
The PLS-PM analysis showed that the space factor (increased altitude) had significantly negative total effects on the climate factors (temperature) and the total biomass, while they significantly positively affected the root–top ratio (Figure 5; Table 4). Climate factors significantly positively affected SLA and SLV, whereas they had significantly negative total effects on the relative abundance (Figure 5; Table 4). Leaf traits (SLA and SLV) significantly reduced the root–top ratio, whereas they significantly increased the total biomass (Figure 5; Table 4). The root–top ratio significantly positively affected the relative abundance (Figure 5; Table 4).
FIGURE 5. PLS-PM analysis showing the direct and indirect effects of biomass. The values at the ends of the arrows indicate path coefficients, and the arrow width represents the strength of the path coefficients. The significance of each path coefficient is indicated by the asterisks beside the numbers (*p<0.05, **p<0.01, ***p<0.001). R2, the coefficient of determination; GoF, the goodness of fit. space factor, including elevation; climate factors, including bio1, bio4, bio5, and bio10; leaf traits, including SLA and SLV; root trait, including root–top ratio; relative abundance, the relative abundance of P. fruticosa; total biomass, the total biomass of P. fruticosa.
TABLE 4. The path coefficients in the partial least squares path modeling (PLS-PM) model. Only paths among latent variables connected significantly were shown.
A better understanding of how environmental factors shape plant traits is critical for assessing the impacts of environmental change on ecosystem processes and function. Ecosystems in high-altitude regions are suggested to be more sensitive to climate change (Izrael et al., 2007), and plant functional traits can be used as predictors of vegetation response to climate warming (Soudzilovskaia et al., 2013). In this study, shrub morphological traits (PTVPC1) were found to be significantly affected by simplified climate factors (Figure 3; Table 2), and elevation significantly negatively affected biomass (Figure 5). Meanwhile, simplified functional traits were insignificantly affected by simplified soil factors. Our results were similar to the finding that space and climate factors were more important than soil indicators in predicting functional characteristic patterns in communities (Pakeman et al., 2009; Dubuis et al., 2013). However, they were inconsistent with the finding that climate and topography mainly influenced tree trait distribution (Blundo et al., 2015; Zarzosa et al., 2021). Shrub functional traits were mostly affected by topographic and edaphic factors in the dry land, namely, slope variations and ratio of soil carbon to nitrogen (Nunes et al., 2019). This finding was inconsistent with our result that simplified functional traits were insignificantly affected by simplified soil factors. A previous study found that temperature was generally related to altitude while soil factors were not (Körner, 2007; Liu et al., 2011), and this finding supported our result that the variation in soil factors was minor and inconsistent across the elevation gradient (Table 1; Figure 2). This condition may result in simplified functional traits that were insignificantly affected by simplified soil factors in this study.
Simplified environmental factors cause difficulty in identifying the impact of each factor on plant functional traits. The correlation and best multiple regression model helped us identify the contribution of each selected factor to the traits of P. fruticosa. bio13 significantly increased across the elevation gradient (Table 1; Figure 4). The result was similar to a clear increase of 42% in rainfall from 3,500 m to 4,050 m elevation (Rodríguez-Morales et al., 2019). Leaf moisture content significantly decreased across the elevation gradient, and this result was inconsistent with the finding that leaf water potential of Achnatherum splendens and Allium tanguticum significantly responded to changes in shallow and middle SWC in alpine grassland (Wu et al., 2018). The reason could be that SWC was not the primary limiting factor in humid and semi-humid plateau areas. SLA, SLV, and plant height were found to be mainly affected by bio10 (Figure 4). This result was similar to the finding that temperature affected SLAs significantly (Rosbakh et al., 2015). Plant phosphorus uptake was mainly affected by pH, temperature, and SWC (Figure 4). This result was similar to the finding that phosphorus absorption by plants was affected by pH, moisture, and temperature (Dotaniya and Meena, 2015). Abiotic factors affected plant root traits (Callis-Duehl et al., 2017), which supported our finding that the underground parts (i.e., root–top ratio and tillering bud) of P. fruticosa were significantly affected by moisture and temperature (Figure 4).
Plants have evolved diverse functional strategies to perform optimally in a given ecosystem (Díaz et al., 2016). Plant functional traits have been mainly associated with abiotic tolerance and competition in the alpine areas (Callis-Duehl et al., 2017); for example, alpine plants might have multiple modes of reproduction forms, such as with a rhizome. Furthermore, traits associated with competition can show significant variation (e.g., SLA). The temperature variables significantly decreased across altitude gradient in this study (Figure 2; Table 1), which created a more stressful abiotic environment for P. fruticosa. In our study, plant competitive traits (i.e., SLA, SLV, and plant height) were positively associated with temperature (Figure 2, Figure 3, Table 1), and trait shifts (e.g., higher root–top ratio, lower SLA) were found toward the stress-tolerant end of the leaf economics spectrum. Previous studies reported that air temperature significantly positively affected SLA (Long et al., 2011; Rosbakh et al., 2015). Specifically, the leaf mass per area increased dramatically with the rise in altitude for alpine shrubs (Zhang et al., 2020), and SLA was significantly positively correlated with SLV in alpine areas (Su et al., 2020). This finding supported our result that leaf traits shifted toward the stress-tolerant end. Climate change has driven alpine plants to shift to a more conservative leaf water economy (higher leaf mass per area, thicker leaves) and massive investment in belowground reserves (Soudzilovskaia et al., 2013), which has contributed to the persistence of species. This previous finding was similar to our result that plants growing in higher elevations shifted toward a persistence strategy with a higher root–top ratio (Figure 3). Long rhizome buds (a conservative reproductive strategy) allow plants to disperse over greater distances, which reduces local intraspecific competition. Meanwhile, tillering buds are an effective means for interspecific competition. A previous study found that intraspecific competition declined in unfavorable environments (Hart and Marshall, 2013), which supported our result that species adjusted reproductive strategy as altitude increased (Figure 3).
Numerous soil indicators had no significant effect on functional trait variations and shrub expansion (Figure 4; Table 3), while space (increased altitude), climate (temperature) factors, and functional traits (SLA and SLV) explained 99% of the variation in the shrub biomass (Figure 5). Shrub expansion strength increased in areas with lower elevations, where plants had higher SLA and SLV. As environmental abiotic stress increased (mainly in temperature reduction) with the rise in altitude, plants of P. fruticosa reduced growth inputs and invested massively in belowground reserves, thereby shifting to a persistence strategy. Low-temperature limitation reduced shrub expansion strength to some extent (Figure 5). The result was similar to the finding that climate warming persistence triggered tree ingression after shrub expansion in a high alpine area (Malfasi and Cannone, 2020). Numerous studies found that most encroaching shrub species were limited by low temperatures, which indicated that warming temperatures could induce shrub expansion. For example, Larrea tridentate is vulnerable to death from freezing-induced cavitation (Martínez-Vilalta and Pockman, 2002). Our findings matched our second hypothesis, that is, the relative abundance of P. fruticosa increased in response to low-temperature environmental via affecting asexual reproduction traits. The ability of low temperatures to limit shrub expansion weakened as the asexual reproduction strategy changed; specifically, plants shifted to the reproduction mode of long rhizome bud in the low-temperature environment, which reduced local intraspecific competition (Figure 5).
Shrub removal, along with the use of prescribed burns, is a common method to deal with expansion (Archer, 2010). However, previous techniques frequently fail to achieve their objectives (Archer and Predick, 2014). Many shrub-removal strategies fail or gain temporary success, which implies that shrub expansion is often irreversible. The essence of shrub expansion is the transition of competitive advantage from grasses to shrubs. Therefore, all strategies to control shrub expansion should ensure the competitive advantage of grasses.
The Qinghai–Tibetan Plateau showed trends toward warmer and wetter conditions. The modified Jena dynamic global vegetation model predicted that the annual temperature would increase by 1.3–4.2°C, and the annual precipitation would increase by 2%–5% (Gao et al., 2016). Climate factors mainly affected shrub expansion strength in the Zoige Plateau. However, large-scale temperature control faces huge challenges. First, we suggest developing a classification of shrub expansion levels. Carbon starvation could lead to the mortality of shrubs (Liberati et al., 2018), and the main means of carbon starvation was insufficient photosynthesis. Second, combining the spraying of organic light-shading on a shrub with prescribed burns is an efficient method of controlling expansion. Finally, management measures that stimulate grass growth, such as grazing reduction and seeding grass, might result in lower shrub cover.
However, we cannot confirm or count out the possible impact of grazing on shrub expansion because we did not analyze its effect in our observed field research. Furthermore, our research was conducted in a regional climate gradient with a humid and semi-humid continental monsoon climate. Thus, further research along broader climate gradients with more aridity levels would be required to fully assess the potential impact of climate change on shrub expansion.
Shrub expansion has been reported mostly in dry and semi-arid ecosystems worldwide. Alpine and cold regions also regard shrub expansion as a serious ecological issue. Multiple drivers, including anthropogenic and environmental factors, are contributors to this phenomenon. However, the relative effects of climate and soil factors on shrub expansion are incompletely quantified or understood in the alpine meadow, and quantifying how these factors result in variations in functional traits associated with shrub expansion is crucial given that the functional traits are tightly related to ecosystem processes.
In this work, we studied the vegetation of an alpine shrub meadow composed of P. fruticosa in the Zoige Plateau, along an elevation gradient. The direct and indirect effects of climate and soil factors on functional traits, the shrub expansion strength (measured as the total biomass), and the relative abundance (via functional traits) were assessed. We found that climate factors, namely, the mean temperature of the warmest quarter (bio10), mostly affected SLA and specific lobe volume (SLV), which were the most important traits related to shrub expansion. Soil factors, except SWC, had weak effects on functional traits closely related to shrub expansion. Our proposed PLS-PM model explained 99% of the variation in the shrub biomass. Our results suggest that climate change not only affected functional traits but also influenced shrub expansion in the Zoige Plateau. Therefore, more accurate forecast and cost effectiveness could be obtained when these drivers are considered in management measures to control expansion. The expansion process could be delayed and a steady pastoral production could be guaranteed by understanding the mechanism of the alpine shrub expansion.
The raw data supporting the conclusion of this article will be made available by the authors, without undue reservation.
JY and PS conceived and designed the experiments; JY performed the experiments with the help of ZZ, YQ, and RS; JY and PS analyzed the data and wrote the paper. All authors confirm their approval for final publication of the manuscript.
This research was funded by the Strategic Priority Research Program of the China Academy of Sciences (XDA20050102), the National Science Foundation of China (41871043, 41701106), and the Youth Science and Technology Fund of Gansu Province (21JR7RA064).
The authors declare that the research was conducted in the absence of any commercial or financial relationships that could be construed as a potential conflict of interest.
All claims expressed in this article are solely those of the authors and do not necessarily represent those of their affiliated organizations, or those of the publisher, the editors and the reviewers. Any product that may be evaluated in this article, or claim that may be made by its manufacturer, is not guaranteed or endorsed by the publisher.
Abràmoff, M. D., Magalhães, P. J., and Ram, S. J. (2004). Image Processing with ImageJ. Biophot. Int. 11 (7), 36–42. doi:10.1201/9781420005615.ax4
Akter, S., D'ambra, J., and Ray, P. (2011)“An Evaluation of PLS Based Complex Models: The Roles of Power Analysis, Predictive Relevance and GoF Index,” in Proceedings of the 17th Americas Conference on Information Systems (AMCIS2011) (Detroit: Association for Information Systems), 1–7.
Archer, S. R., and Predick, K. I. (2014). An Ecosystem Services Perspective on Brush Management: Research Priorities for Competing Land-Use Objectives. J. Ecol. 102 (6), 1394–1407. doi:10.1111/1365-2745.12314
Archer, S. R. (2010). “Rangeland Conservation and Shrub Encroachment: New Perspectives on an Old Problem,” in Wild Rangelands: Conserving Wildlife While Maintaining Livestock in Semi-Arid Ecosystems. Editors J. du Toit, R. Kock, and J. Deutsch (Oxford, England: Wiley-Blackwell Publishing).
Bai, J.-h., Lu, Q.-q., Wang, J.-j., Zhao, Q.-q., Ouyang, H., Deng, W., et al. (2013). Landscape Pattern Evolution Processes of Alpine Wetlands and Their Driving Factors in the Zoige Plateau of China. J. Mt. Sci. 10 (1), 54–67. doi:10.1007/s11629-013-2572-1
Blundo, C., Malizia, L. R., and González-Espinosa, M. (2015). Distribution of Functional Traits in Subtropical Trees across Environmental and Forest Use Gradients. Acta Oecol. 69, 96–104. doi:10.1016/j.actao.2015.09.008
Brandt, J. S., Haynes, M. A., Kuemmerle, T., Waller, D. M., and Radeloff, V. C. (2013). Regime Shift on the Roof of the World: Alpine Meadows Converting to Shrublands in the Southern Himalayas. Biol. Conserv. 158, 116–127. doi:10.1016/j.biocon.2012.07.026
Bu, W., Huang, J., Xu, H., Zang, R., Ding, Y., Li, Y., et al. (2019). Plant Functional Traits Are the Mediators in Regulating Effects of Abiotic Site Conditions on Aboveground Carbon Stock-Evidence from a 30 Ha Tropical Forest Plot. Front. Plant Sci. 9, 1958. doi:10.3389/fpls.2018.01958
Callis-Duehl, K., Vittoz, P., Defossez, E., and Rasmann, S. (2017). Community-level Relaxation of Plant Defenses against Herbivores at High Elevation. Plant Ecol. 218 (3), 291–304. doi:10.1007/s11258-016-0688-4
Coetzee, B. W., Tincani, L., Wodu, Z., and Mwasi, S. M. (2008). Overgrazing and Bush Encroachment by Tarchonanthus Camphoratus in a Semi-arid Savanna. Afr. J. Ecol. 46 (3), 449–451. doi:10.1111/j.1365-2028.2007.00842.x
Collins, C. G., Spasojevic, M. J., Alados, C. L., Aronson, E. L., Benavides, J. C., Cannone, N., et al. (2020). Belowground Impacts of Alpine Woody Encroachment Are Determined by Plant Traits, Local Climate, and Soil Conditions. Glob. Change Biol. 26 (12), 7112–7127. doi:10.1111/gcb.15340
Crouch, S. R., and Malmstadt, H. V. (1967). Mechanistic Investigation of Molybdenum Blue Method for Determination of Phosphate. Anal. Chem. 39 (10), 1084–1089. doi:10.1021/ac60254a027
Díaz, S., Kattge, J., Cornelissen, J. H., Wright, I. J., Lavorel, S., Dray, S., et al. (2016). The Global Spectrum of Plant Form and Function. Nature 529 (7585), 167–171. doi:10.1038/nature16489
Ding, X., Su, P., Zhou, Z., Shi, R., and Yang, J. (2021). Responses of Plant Bud Bank Characteristics to the Enclosure in Different Desertified Grasslands on the Tibetan Plateau. Plants 10 (1), 141. doi:10.3390/plants10010141
Dotaniya, M. L., and Meena, V. D. (2015). Rhizosphere Effect on Nutrient Availability in Soil and its Uptake by Plants: a Review. Proc. Natl. Acad. Sci. India, Sect. B Biol. Sci. 85 (1), 1–12. doi:10.1007/s40011-013-0297-0
Dubuis, A., Rossier, L., Pottier, J., Pellissier, L., Vittoz, P., and Guisan, A. (2013). Predicting Current and Future Spatial Community Patterns of Plant Functional Traits. Ecography 36 (11), 1158–1168. doi:10.1111/j.1600-0587.2013.00237.x
Eldridge, D. J., Bowker, M. A., Maestre, F. T., Roger, E., Reynolds, J. F., and Whitford, W. G. (2011). Impacts of Shrub Encroachment on Ecosystem Structure and Functioning: towards a Global Synthesis. Ecol. Lett. 14 (7), 709–722. doi:10.1111/j.1461-0248.2011.01630.x
Eldridge, D. J., and Ding, J. (2021). Remove or Retain: Ecosystem Effects of Woody Encroachment and Removal Are Linked to Plant Structural and Functional Traits. New Phytol. 229 (5), 2637–2646. doi:10.1111/nph.17045
Field, A., Miles, J., and Field, Z. (2012). Discovering Statistics Using R. California: Sage publications.
Fu, Y., Liu, C., Lin, F., Hu, X., Zheng, X., Zhang, W., et al. (2018). Quantification of Year-Round Methane and Nitrous Oxide Fluxes in a Typical Alpine Shrub Meadow on the Qinghai-Tibetan Plateau. Agric. Ecosyst. Environ. 255, 27–36. doi:10.1016/j.agee.2017.12.003
Gao, Q., Guo, Y., Xu, H., Ganjurjav, H., Li, Y., Wan, Y., et al. (2016). Climate Change and its Impacts on Vegetation Distribution and Net Primary Productivity of the Alpine Ecosystem in the Qinghai-Tibetan Plateau. Sci. total Environ. 554-555, 34–41. doi:10.1016/j.scitotenv.2016.02.131
Gómez-Rey, M. X., Madeira, M., Gonzalez-Prieto, S. J., and Coutinho, J. (2013). Soil C and N Dynamics in a Mediterranean Oak Woodland with Shrub Expansion. Plant soil 371 (1), 339–354. doi:10.1007/s11104-013-1695-z
Gobelle, S. K., and Gure, A. (2018). Effects of Bush Encroachment on Plant Composition, Diversity and Carbon Stock in Borana Rangelands, Southern Ethiopia. Int. J. Biodivers. Conserv. 10 (5), 230–245. doi:10.5897/IJBC2017.1143
Grömping, U. (2015). Variable Importance in Regression Models. Wiley Interdiscip. Rev. Comput. Stat. 7 (2), 137–152. doi:10.1002/wics.1346
Hart, S. P., and Marshall, D. J. (2013). Environmental Stress, Facilitation, Competition, and Coexistence. Ecology 94 (12), 2719–2731. doi:10.1890/12-0804.1
Hughes, J., and Beiner, D. J. (2021). Reghelper: Helper Functions for Regression Analysis. R package version 1.1.0. Available at: https://CRAN.R-project.org/package=reghelper.
Izrael, Y. A., Semenov, S. M., Anisimov, O. A., Anokhin, Y. A., Velichko, A. A., Revich, B. A., et al. (2007). The Fourth Assessment Report of the Intergovernmental Panel on Climate Change: Working Group II Contribution. Russ. Meteorol. Hydrol. 32 (9), 551–556. doi:10.3103/s1068373907090014
Knapp, A. K., Briggs, J. M., Collins, S. L., Archer, S. R., Bret-Harte, M. S., Ewers, B. E., et al. (2008). Shrub Encroachment in North American Grasslands: Shifts in Growth Form Dominance Rapidly Alters Control of Ecosystem Carbon Inputs. Glob. change Biol. 14 (3), 615–623. doi:10.1111/j.1365-2486.2007.01512.x
Komac, B., Kefi, S., Nuche, P., Escós, J., and Alados, C. L. (2013). Modeling Shrub Encroachment in Subalpine Grasslands under Different Environmental and Management Scenarios. J. Environ. Manag. 121, 160–169. doi:10.1016/j.jenvman.2013.01.038
Körner, C. (2007). The Use of ‘altitude’in Ecological Research. Trends Ecol. Evol. 22 (11), 569–574. doi:10.1016/j.tree.2007.09.006
Li, C., Shimono, A., Shen, H., and Tang, Y. (2010). Phylogeography of Potentilla Fruticosa, an Alpine Shrub on the Qinghai-Tibetan Plateau. J. Plant Ecol. 3 (1), 9–15. doi:10.1093/jpe/rtp022
Li, H., Shen, H., Chen, L., Liu, T., Hu, H., Zhao, X., et al. (2016). Effects of Shrub Encroachment on Soil Organic Carbon in Global Grasslands. Sci. Rep. 6 (1), 1–9. doi:10.1038/srep28974
Liberati, D., de Dato, G., Guidolotti, G., and De Angelis, P. (2018). Linking Photosynthetic Performances with the Changes in Cover Degree of Three Mediterranean Shrubs under Climate Manipulation. Oikos 127 (11), 1633–1645. doi:10.1111/oik.05263
Liu, Y.-F., Zhang, Z., Liu, Y., Cui, Z., Leite, P. A. M., Shi, J., et al. (2022). Shrub Encroachment Enhances the Infiltration Capacity of Alpine Meadows by Changing the Community Composition and Soil Conditions. CATENA 213, 106222. doi:10.1016/j.catena.2022.106222
Liu, Y., Ding, Z., Bachofen, C., Lou, Y., Jiang, M., Tang, X., et al. (2018). The Effect of Saline-Alkaline and Water Stresses on Water Use Efficiency and Standing Biomass of Phragmites Australis and Bolboschoenus Planiculmis. Sci. total Environ. 644, 207–216. doi:10.1016/j.scitotenv.2018.05.321
Liu, Y., He, J., Shi, G., An, L., Öpik, M., and Feng, H. (2011). Diverse Communities of Arbuscular Mycorrhizal Fungi Inhabit Sites with Very High Altitude in Tibet Plateau. FEMS Microbiol. Ecol. 78 (2), 355–365. doi:10.1111/j.1574-6941.2011.01163.x
Long, W., Zang, R., Schamp, B. S., and Ding, Y. (2011). Within- and Among-Species Variation in Specific Leaf Area Drive Community Assembly in a Tropical Cloud Forest. Oecologia 167 (4), 1103–1113. doi:10.1007/s00442-011-2050-9
Maestre, F. T., Bowker, M. A., Puche, M. D., Belén Hinojosa, M., Martínez, I., García-Palacios, P., et al. (2009). Shrub Encroachment Can Reverse Desertification in Semi-arid Mediterranean Grasslands. Ecol. Lett. 12 (9), 930–941. doi:10.1111/j.1461-0248.2009.01352.x
Malfasi, F., and Cannone, N. (2020). Climate Warming Persistence Triggered Tree Ingression After Shrub Encroachment in a High Alpine Tundra. Ecosystems 23 (8), 1657–1675. doi:10.1007/s10021-020-00495-7
Marenco, R. A., Antezana-Vera, S. A., and Nascimento, H. C. S. (2009). Relationship between Specific Leaf Area, Leaf Thickness, Leaf Water Content and SPAD-502 Readings in Six Amazonian Tree Species. Photosynt. 47 (2), 184–190. doi:10.1007/s11099-009-0031-6
Martínez-Vilalta, J., and Pockman, W. T. (2002). The Vulnerability to Freezing‐induced Xylem Cavitation of Larrea Tridentata (Zygophyllaceae) in the Chihuahuan Desert. Am. J. Bot. 89 (12), 1916–1924. doi:10.3732/ajb.89.12.1916
Morgan, J. A., Milchunas, D. G., LeCain, D. R., West, M., and Mosier, A. R. (2007). Carbon Dioxide Enrichment Alters Plant Community Structure and Accelerates Shrub Growth in the Shortgrass Steppe. Proc. Natl. Acad. Sci. U.S.A. 104 (37), 14724–14729. doi:10.1073/pnas.0703427104
Nelson, D. a., and Sommers, L. E. (1983). Total Carbon, Organic Carbon, and Organic Matter. Methods soil analysis Part 2 Chem. Microbiol. Prop. 9, 539–579.
Nunes, A., Köbel, M., Pinho, P., Matos, P., Costantini, E. A. C., Soares, C., et al. (2019). Local Topographic and Edaphic Factors Largely Predict Shrub Encroachment in Mediterranean Drylands. Sci. Total Environ. 657, 310–318. doi:10.1016/j.scitotenv.2018.11.475
Pakeman, R. J., Lepš, J., Kleyer, M., Lavorel, S., Garnier, E., and Consortium, V. (2009). Relative Climatic, Edaphic and Management Controls of Plant Functional Trait Signatures. J. Veg. Sci. 20 (1), 148–159. doi:10.1111/j.1654-1103.2009.05548.x
Pratt, R., Jacobsen, A., Percolla, M., De Guzman, M., Traugh, C., and Tobin, M. (2021). Trade-offs Among Transport, Support, and Storage in Xylem from Shrubs in a Semiarid Chaparral Environment Tested with Structural Equation Modeling. Proc. Natl. Acad. Sci. 118 (33). doi:10.1073/pnas.2104336118
R Core Team (2013). R: A Language and Environment for Statistical Computing. Vienna, Austria: R Foundation for Statistical Computing.
Rodríguez-Morales, M., Acevedo-Novoa, D., Machado, D., Ablan, M., Dugarte, W., and Dávila, F. (2019). Ecohydrology of the Venezuelan Páramo: Water Balance of a High Andean Watershed. Plant Ecol. Divers. 12 (6), 573–591. doi:10.1080/17550874.2019.1673494
Rosbakh, S., Römermann, C., and Poschlod, P. (2015). Specific Leaf Area Correlates with Temperature: New Evidence of Trait Variation at the Population, Species and Community Levels. Alp. Bot. 125 (2), 79–86. doi:10.1007/s00035-015-0150-6
Salazar Zarzosa, P., Diaz Herraiz, A., Olmo, M., Ruiz-Benito, P., Barrón, V., Bastias, C. C., et al. (2021). Linking Functional Traits with Tree Growth and Forest Productivity in Quercus ilex Forests along a Climatic Gradient. Sci. Total Environ. 786, 147468. doi:10.1016/j.scitotenv.2021.147468
Sanchez, G., Trinchera, L., and Russolillo, G. (2015). Plspm: Tools for Partial Least Squares Path Modeling (PLS-PM). R package version 0.4.9. Available at: https://github.com/gastonstat/plspm.
Senner, N. R., and Coddington, K. S. (2011). Habitat Use and Foraging Ecology of Hudsonian Godwits Limosa haemastica in Southern South America. Wader Study Group Bull. 118, 105–108.
Soudzilovskaia, N. A., Elumeeva, T. G., Onipchenko, V. G., Shidakov, I. I., Salpagarova, F. S., Khubiev, A. B., et al. (2013). Functional Traits Predict Relationship between Plant Abundance Dynamic and Long-Term Climate Warming. Proc. Natl. Acad. Sci. U.S.A. 110 (45), 18180–18184. doi:10.1073/pnas.1310700110
Su, P-X., Ding, X-J., Chen, H-S., Zhou, Z-J., Shi, R., and Ding, X-J. 2020. Alpine Shrub Potentilla Fruticosa and the Galsang Flower. Plant Sci. J. 38 (6), 844–852. (in chinese).
Van Auken, O. W. (2009). Causes and Consequences of Woody Plant Encroachment into Western North American Grasslands. J. Environ. Manag. 90 (10), 2931–2942. doi:10.1016/j.jenvman.2009.04.023
Wolf, A. A., Funk, J. L., Selmants, P. C., Morozumi, C. N., Hernández, D. L., Pasari, J. R., et al. (2021). Trait-based Filtering Mediates the Effects of Realistic Biodiversity Losses on Ecosystem Functioning. Proc. Natl. Acad. Sci. 118 (26), e2022757118. doi:10.1073/pnas.2022757118
Wu, H., Li, J., Li, X.-Y., He, B., Liu, J., Jiang, Z., et al. (2018). Contrasting Response of Coexisting Plant's Water-Use Patterns to Experimental Precipitation Manipulation in an Alpine Grassland Community of Qinghai Lake Watershed, China. PloS one 13 (4), e0194242. doi:10.1371/journal.pone.0194242
Xiang, S., Guo, R., Wu, N., and Sun, S. (2009). Current Status and Future Prospects of Zoige Marsh in Eastern Qinghai-Tibet Plateau. Ecol. Eng. 35 (4), 553–562. doi:10.1016/j.ecoleng.2008.02.016
Yashiro, Y., Shizu, Y., Hirota, M., Shimono, A., and Ohtsuka, T. (2010). The Role of Shrub (Potentilla Fruticosa) on Ecosystem CO2 Fluxes in an Alpine Shrub Meadow. J. Plant Ecol. 3 (2), 89–97. doi:10.1093/jpe/rtq011
Zhang, L., Yang, L., and Shen, W. (2020). Dramatic Altitudinal Variations in Leaf Mass Per Area of Two Plant Growth Forms at Extreme Heights. Ecol. Indic. 110, 105890. doi:10.1016/j.ecolind.2019.105890
Zhang, X., and Liu, H. (2009). Degradation Features and Ecological Restoration Approaches of Peatlands in Ruoergai Plateau. Wetl. Sci. 7, 243–249. (in chinese)
Zhao, Y., Liu, X., Wang, Y., Zheng, Z., Zheng, S., Zhao, D., et al. (2021). UAV-based Individual Shrub Aboveground Biomass Estimation Calibrated against Terrestrial LiDAR in a Shrub-Encroached Grassland. Int. J. Appl. Earth Observation Geoinformation 101, 102358. doi:10.1016/j.jag.2021.102358
Keywords: shrub expansion, climate factor, soil factor, functional traits, sustainable land management
Citation: Yang J, Su P, Zhou Z, Shi R and Qin Y (2022) Shrub Expansion is Mainly Affected by Climate-Dominated Functional Traits in Alpine Meadow. Front. Environ. Sci. 10:917597. doi: 10.3389/fenvs.2022.917597
Received: 11 April 2022; Accepted: 07 June 2022;
Published: 29 June 2022.
Edited by:
Zhanhuan Shang, Lanzhou University, ChinaReviewed by:
Xiangtai Wang, Lanzhou University, ChinaCopyright © 2022 Yang, Su, Zhou, Shi and Qin. This is an open-access article distributed under the terms of the Creative Commons Attribution License (CC BY). The use, distribution or reproduction in other forums is permitted, provided the original author(s) and the copyright owner(s) are credited and that the original publication in this journal is cited, in accordance with accepted academic practice. No use, distribution or reproduction is permitted which does not comply with these terms.
*Correspondence: Peixi Su, c3VweEBsemIuYWMuY24=
Disclaimer: All claims expressed in this article are solely those of the authors and do not necessarily represent those of their affiliated organizations, or those of the publisher, the editors and the reviewers. Any product that may be evaluated in this article or claim that may be made by its manufacturer is not guaranteed or endorsed by the publisher.
Research integrity at Frontiers
Learn more about the work of our research integrity team to safeguard the quality of each article we publish.