- 1College of Business Administration, Liaoning Technical University, Xingcheng, China
- 2Faculty of Management Sciences, National University of Modern Language, Islamabad, Pakistan
- 3Reading Academy, Nanjing University of Information Science and Technology, Nanjing, China
- 4Department of Management Sciences, Qurtaba University, Peshawar, Pakistan
We have extended the literature on how information communication technology (ICT) and renewable energy relates to environmental quality in South-East Asia. Earlier literature has mostly focused on individual country cases, and regional investigations, especially in South-East Asian, are largely absent from the existing body of knowledge. The use of ICT and renewable energy are among the top priorities of each economy in this region. We pursued this study with the intention of identifying trends in the way these countries use ICT and renewable energy, and how these emerging factors contribute to their environmental performance. We analyzed the annual data of six countries from between 2000 and 2018, using Panel Quantile Regression, and Dynamic Fixed Effect estimation techniques to test both the hypothesized short-run and long-run relationship between ICT and renewable energy use and environmental quality. Our empirical results reveal the non-mitigating effect of ICT on CO2 emission, confirming that ICT use in this region does not improve environmental performance, but rather causes more environmental degradation. Renewable energy, on the other hand, results in a significant contribution to environmental quality in this region. Our results are consistent with multiple studies in the existing body of knowledge. The findings are very meaningful and useful for policymakers in these countries to help them to frame strategies for renewable energy and ICT use practices that favor the environment.
Introduction and Background
Globalization has highlighted the significant contribution of information technology, digitalization, and blockchain technology to economic growth (Saberi et al., 2019; Oliveira et al., 2020; Borowski, 2021). The internet and advanced technologies support the flow of foreign direct investment (FDI) and trade liberalization (Bhujabal and Sethi, 2020; Borowski, 2021). In addition, these technologies also contribute to a country’s infrastructure and overall productivity, which increase prosperity (Bollou, 2010; Borowski, 2021). Digitalization and ICT use increase employment, and play a very important role in reducing poverty (Coleman, 2005; Rot et al., 2020). Economic growth is the reason for greater use of digital technology and innovation in technology (Erumban and Das, 2016). Avgerou (2003) and Jin and Cho (2015) also highlighted the significant contribution of ICT to economic growth. Information communication technology (ICT) and energy consumption have significantly increased in the past decade. ICT has become a vital aspect of enhancing many people’s living standards (Moyer and Hughes, 2012). ICT comprises the internet, mobile phones, and other mediums of communication that have vital acceptability in making standard of life ICT increases the demand for smart technology, i.e., touch screens, monitors, and tablets. The latest developments, e.g., wireless and Bluetooth technologies, enhance the effectiveness of machines and humans and increase efficiency over time. ICT is not only improving people’s lives but is also enhancing the economic development of countries. As a result of ICT, communication methods throughout the world have changed, and the world has become a “global village.”
ICT and digitalization also create new business opportunities and foster environmental sustainability. ICT and digitalization ensure the durability of the energy system by increasing its security and efficiency (García-Quismondo et al., 2013; M.; Rahman and Mezbah-ul-Islam, 2012; K.; Wang et al., 2018). Digital technologies and energy efficiency are vital to countering global warming, and appropriate strategies in this regard can promote effective change to the energy system (Alamoush et al., 2020; Kueppers et al., 2021). The journey toward zero emissions and sustainable development could be achieved through drastic innovation in ICT and technology to create more renewable energy options in countries at the corporate and society level, which will contribute to a sustainable environment (Borowski, 2021). In this regard, energy service companies could play a vital role in removing the barriers to energy efficiency implementation (Recalde, 2021; Smith et al., 2021). The emergence of renewable sources and energy-efficient technologies should be adopted as a trend for climate transformation and sustainable development (Borowski, 2021). Climate and energy solutions are based on reducing the emission of greenhouse gases, and switching to efficient renewable energy options (Panwar et al., 2011; Yadoo and Cruickshank, 2012). Efficient energy use through the application of advanced technology and ICT practices could be effective in reducing its harmful effect on the environment. Hence, the use of sophisticated technologies and the block chain is a step forward towards efficient energy consumption and a sustainable environment (Silvestre and Ţîrcă, 2019; Kueppers et al., 2021; Manfren et al., 2021).
However, it has also been observed that in addition to increasing energy efficiency and environmental sustainability, ICT may cause the deterioration of the environment. Using more sophisticated information and telecommunication equipment requires a significant amount of energy, which can degrade the environment if renewable energy is not provided for its operation. The existing literature on this subject explores two dimensions of ICT and digitalization concerning environmental quality: namely, their positive and negative effects in the environment. Many studies confirm that ICT and digitalization can cause environmental quality to deteriorate (Al-Mulali et al., 2015b; Avom et al., 2020; Moyer and Hughes, 2012; Ozcan and Apergis, 2018; Ozcan et al., 2020; Sokolov-Mladenović et al., 2016). In contrast, many studies also highlight the significant contribution of ICT advancement and digitalization to energy efficiency gains and environmental quality (Sadorsky, 2012; Lee and Brahmasrene, 2016; Belkhir and Elmeligi, 2018).
This study is a novel attempt to explore the nexus between ICT, renewable energy, and environmental quality in a single robust study focusing on the emerging economies of South-East Asia, using Panel Quantile and Dynamic Fixed Effect (DFE) estimation techniques. The study is expected to contribute in many ways. First, this research could raise awareness among policymakers in this belt, and enable them to make their strategic frameworks more robust to encourage ICT and digitalization in for energy-efficient production and a sustainable environment. Moreover, this study could have far-reaching implications at both the regional and country levels. Theoretically and methodologically, this study is likely to add value to the existing body of knowledge by using estimation techniques that cover both the short-run and long-run dynamics of the variables. This study could enhance the understanding of many researchers, with a special focus on exploring similar variables and likely to abreast their knowledge repository and insights.
This study aims to investigate the impact of ICT and renewable energy on the environmental quality in South-East Asia to explore the prevailing mechanisms of ICT and digitalization approaches and renewable energy trends underway in these countries.
Literature Review, Theoretical Background and Hypothesis Development
Information Communication Technology and Environmental Quality
This paper is based on the TAM theory, which states that advanced technology is accepted for technological innovation and for the enhancing the individual capabilities (Davis, 1989). Hence in this region the acceptance of ICT and technological development is purely in the spirit of this theory and the Diffusion of Innovations (DOI) theory by Moore and Benbasat (1991), which explain how the diffuson of ideas is related to the spread of technology. Nowadays, there is a debate among academics about how to explore the impact of ICT on the environment. The increasing use of ICT in emerging economies is highly debatable (Asongu and Nwachukwu, 2016). ICT practices enhance human capacity and capabilities, which directly contribute to the country’s GDP (Pradhan et al., 2016), and help to decrease income disparity (Tchamyou et al., 2019). ICT is a significant factor in bringing about financial growth and innovation (Edo et al., 2019), as well as encouraging education and promoting the Human Development Index (Tchamyou et al., 2019). Whereas the fact is not hidden from anyone about the reality of been the ICT, a contributing factor to environmental sustainability. However, the existing literature is divided on whether ICT is beneficial or detrimental to the environment. Mingay (2007) asserts that ICT contributes almost 2% of worldwide greenhouse gases, which negatively affect environmental quality. Similarly, Alcott (2005) states that although ICT plays a pivotal role in enhancing productivity, it has an adverse effect on environmental quality. Likewise, F. N. Khan et al. (2020) confirm the positive nexus between ICT and CO2 emission in the ASEAN region, analyzing data from 1991 to 2009. Internet use and GDP have both short-run and long-run relationships with CO2 emissions, verifying the adverse impact on the environmental quality of OCED countries (Salahuddin et al., 2016). However, a school of thought arguing that ICT positively contributes to environmental quality can also be found in empirical literature. In this regard, Al-Mulali et al. (2015a) found indications of a negative relationship between ICT and CO2 emissions, confirming that ICT reduces CO2 emissions in emerging economies, but that the effect is insignificant. Similarly, Godil et al. (2020) investigated data from numerous countries for the period 1990 to 2015 and outlined that ICT decreases carbon emissions in high- and middle-income countries, suggesting that ICT in high-income countries may be due to efficient energy usage that accounts for low CO2 emissions. Li et al. (2018) argued that ICT reduces the amount of CO2 emissions in specific European regions. Validating the positive nexus of ICT and environmental quality, Ozcan and Apergis (2018) examined the impact of ICT on CO2 emissions, and confirmed that ICT helps to minimize ecological degradation, thereby concluding that ICT is the mitigating and stimulating factor in CO2 emissions. Based on the above discussion, we have developed the following hypothesis Table 1.
H1: ICT reduces greenhouse gases and improves environmental quality.
Renewable Energy and Environmental Quality
Renewable energy is a central focus of study for energy economics researchers. Riti et al. (2018) investigated the impact of both renewable and non-renewable energy on environmental quality, and found that fossil fuel energy consumption increases CO2 emission both in the short and long run, while renewable energy consumption reduces CO2 emission in the long run. Renewable energy has become vital for every country thanks to its cost-effectiveness and its contributions to reducing CO2 emissions compared to conventional energy consumption (Turkenburg et al., 2012). Panwar et al. (2011) also demonstrate that renewable energy is more cost-effective and could be very significant in lowering the emission of greenhouse gases. Moreover, Xie et al. (2018) conducted a study to investigate the effect of SO2 and renewable energy consumption on air pollution in the Jing-Jin-Jie region, and concluded that renewable energy development is vital for reducing the emission of dangerous gases that create a pollutant environment, and suggested that technological innovation in renewable energy would further reduce air pollution. Similarly, H. Yu et al. (2012) carried out a study on the impact of the growth of industry on the ecosystem and revealed that greater consumption can cause ecological problems. Similarly, environmental degradation and the elimination of resources were mainly caused by the development of industrial units. Furthermore, Tilt (2019) asserted that technological innovation in the course of renewable energy enhances the capacity and supply of renewable energy to better meet energy shortages and to increase the energy portfolio. Similarly, Lewis (2010) explored renewable energy as a future source of energy given its environmentally friendly nature. Likewise, B. Lin and Zhu (2019) argued that improving the technological level of renewable energy would promote renewable energy production.
Extensive empirical studies exist on the pollution–economic growth nexus with inconsistent findings (Ahmad et al., 2017; Al-Mulali et al., 2016; Alam and Paramati, 2016; Anastacio, 2017; Awad and Abugamos, 2017; Jebli, 2016; Özokcu and Özdemir, 2017; Zeeshan et al., 2021; Zeeshan et al., 2021). The second pillar of the empirical studies has focused on the relationship between energy consumption and economic growth. These studies were pioneered by Kraft and Kraft (1978) in their seminal work. Earlier versions of these studies, which were conducted in bivariate models, could have resulted in an omitted variable bias resulting in inconsistent estimates (Akarca and Long, 1980). However, recent studies have used multivariate models and advanced time-series estimation approaches, but their findings have been conflicting (Asafu-Adjaye, 2000; Apergis and Payne, 2010; Dergiades et al., 2013; Mutascu, 2016). From the previous eras, environmental sustainability and energy safety have been the most vital and pertinent economic challenges. CO2 emissions are mainly caused by the consumption of more fossil fuels, which is confirmed by IEA (International Energy Agency). Much previous research documented that more fossil fuel energy usage leads to more CO2 emissions in emerging economies across the globe. That is why numerous policymakers and regimes have documented the significance of renewable energy for meeting energy demand and minimizing CO2 emissions, and research has been conducted to investigate the dynamics of renewable energy usage and CO2 emissions. For example, Jaforullah and King (2015) documented a negative association between renewable energy consumption and CO2 emissions in the United States from 1960 to 2007. Moreover, Rafiq et al. (2014) reported one-directional causality running from CO2 emissions to renewable energy, where they found bi-directional causality between the two variables in both countries, i.e., India and China from 1972 to 2011. A similar study was conducted on data from Kenya from 1980 to 2012 by Al-Mulali et al. (2016), who found that renewable energy usage significantly minimized CO2 emissions. Similarly, Bloch et al. (2015) found that renewable energy usage decreases CO2 emissions. Some of the previous researchers established that improvements in the banking sector led to greater energy use, which led to greater CO2 emissions In cross-country studies, numerous findings confirmed a positive nexus between renewable energy use and environmental degradation. Moreover, Apergis and Payne (2012) asserted that there is a positive and long-term cointegrating nexus between renewable energy consumption per capita and CO2 emissions per capita. Similarly, Boutabba (2014) examined a causal nexus exists between CO2 emissions and fossil fuel consumption, suggesting that greater usage of renewable energy will prove to be a factor in minimizing the degradation of the environment. However, Menyah and Wolde-Rufael (2010) asserted that renewable energy had not reduced carbon emissions in the United States and that there was no causality between renewable energy consumption and CO2 emissions. Salim and Islam (2010) documented that CO2 emissions had a positive effect on renewable energy usage and also noticed a one-directional causality from renewable energy consumption to CO2 emissions in India; however, a bidirectional casualty between renewable energy consumption and CO2 emissions in China, Brazil and Indonesia was observed. Based on the above discussion we have developed the following hypothesis.
H2: Renewable Energy Increases Environmental Quality
Population and Environmental Quality
Currently the impact of population on CO2 emissions is still being debated. In previous studies, population has also been considered one of the key indicators. An and Jeon (2006) used a cross-sectional regression non-parametric kernel technique, which validated an inverted U-shape between population, using OECD panel data for the period from 1960 to 2000. Similarly, Menz and Welsch (2012) asserted that population is the main cause of CO2 emissions in 26 OECD countries and suggested that the birth rate needed to be controlled as it affected CO2 emissions. Yang and Wang (2020) reported a negative effect of population on environmental quality in China in 10, suggesting that population must be controlled to counter the emission of greenhouse gases. Similarly, Cai et al. (2021) and Q. Wang and Wang (2020) argued that CO2 emissions are largely caused by the population. However, Avom et al. (2020), and Zhou et al. (2019) argued that population size and energy positively affect CO2 emissions, documenting that environmental quality has direct link with environmental quality. Furthermore, M. M. Rahman et al. (2020) examined the nexus between CO2 emissions and population in five South-Asian regionsS, applying panel co-integration techniques for the period from 1990 to 2017 and found that population has a direct link with CO2 emissions in this region, with a one-directional causality from population to CO2 emissions. Cole and Neumayer (2004), Li et al. (2018) and C. Zhang and Lin (2012) highlighted that the rising pattern in the size of urban populations has resulted in higher energy usage that can lead to serious ecological problems, particularly multiplying effect on the level of CO2 emissions. Similarly, many other studies also reported a proportionate relationship between population size and CO2 emissiosn in the context of regions (Cole and Neumayer, 2004; Poumanyvong and Kaneko, 2010) and megacities ( M. Lin et al., 2012; Shuai et al., 2018; X. Yu et al., 2020).
H3: Population size positively contributes to CO2 emissions.
Trade and Environmental Quality
Currently, growth in international trade and the environment propose that the present state of the trade, energy uses and forms like non-renewable can be vital to sustainable environment. Hassan et al. (2019) explored the relationship between trade and environmental quality, and argued that trade has several economic and ecological consequences. Moreover, Dogan and Turkekul (2016) highlighted that there is a positive impact of trade on environmental quality, which is supported by Shahbaz et al. (2013), and Kellenberg (2008). Furthermore, Kahouli and Omri (2017) also confirmed that trade has a positive effect on environmental quality, and proposed that the promotion of trade from the local to the international market does not increase the market shares of trade in a region, but improves the effectiveness of the use of limited resources, which enhances environmental quality. This recommend that a region that is open to trade will detect minimum pollution. However, Ruta and Venables (2012), and I. Khan et al. (2021) argued that the increase of trade to global market accounts for a reduction in natural resources, and increases the volume of CO2, which will eventually worsen environmental quality. In addition, Hassan et al. (2020) argued that international trade is unfavorable to the environment since it increases the presence of polluting industries. On the other hand, Iorember et al. (2021), and Güngör et al. (2021) studied the effects of trade on environmental quality in South Africa by applying the structural break Maki cointegration test and ARDL, and suggested that an increase in trade may improve environmental quality. This is why policymakers in South Africa are advised to consider the significance of trade to promote environmental quality.
H4: Trade liberalization adversely affects environmental quality.
Methodology and Estimation Techniques for Hypothesis Testing
Data, Estimation and Econometrics Techniques
The study used panel data from six South-East Asian counties from 2000 to 2018, collected from the WDI database, and analyzed it using panel data techniques, i.e., Panel Quantile and Dynamic Fixed Effect.
Baseline Estimation Techniques
With a view to exploring the effect of ICT and renewable energy on environmental quality in South-East Asian regions. We preferred panel quantile regression (PQR) estimation techniques in the spirit of Bassett and Koenker (1978), and Bassett Jr and Koenker (1978). Koenker and Bassett (1978) proposed this technique in which explanatory variables are conditionally distributed that cause fitting of liner function for independent variables and also extend the traditional variable mean regression which is in the form of OLS regression, while comparing QR with simple OLS regression a researcher can select any quantile to be used as an estimation parameter and an estimator that best suits when the data has normality, hetero and auto correlation issues. With the QR technique, the sensitivity to outliers is very minimal and delivers better and more accurate results (Sherwood and Wang, 2016; S.; Zhang et al., 2019). This particular estimation technique is considered an influencing factor with heterogeneous nature, and it very easily explores the data at different quantiles (Cheng et al., 2021; K.-L.; Wang et al., 2020). Based on these stated advantages we prefer panel quantile estimation techniques to explore the impact of ICT and renewable energy on environmental quality at different quantiles. In the spirit of Koenker (2004), we used the following panel quantile technique using equation as follows.
Where
Where
We also applied the Dynamic Fixed Effect model to validate the relationship of the variables explored through penal quantile regression. Panel DOLS and Panel FMOLS estimation techniques perfectly estimate the short-run relationship, and do not account for the long-run relationship (Murthy and Nath, 2009). In comparison, the Dynamic Fixed Effect Model is a good choice among panel models when different levels of heterogeneity exist across units. It is an appropriate panel model when a researcher analyzes both the short-run and long-run relationships of the variables simultaneously. This estimator permits intercept varying and formally put in position of homogeneity restriction over the coefficients of both short-run and long-run associations. Since countries in emerging markets have both unique and similar practices and operations, Dynamic Fixed Effect is an accurate and justified model for the analysis of this research. Sometimes, owing to the omission of numerous common factors, the contemporaneous correlation occurs in various residuals; to deal with this problem econometrically, time-specific effects are made in regression The Dynamic Fixed Effect model is based on the ARDL correction form that is used to examine the short-run and long-run associations of the variables.
Variables Definitions and Measurement
Empirical Results and Discussion
In Table 2, we tested the existence of cross-sectional dependence in the data. We considered countries in South-East Asia, which represent the dynamics of different countries. Our results validated the existence of cross-sectional dependence in the data generated through numerous tests (Breusch and Pagan, 1980; Frees, 1995; Pesaran et al., 2004). All these tests showed significant values that clearly indicated the rejection of null hypothesis, meaning that the significance level of cross-sectional dependence exists in the data.
Table 3 shows the results of the panel unit root test. We first explored the existence of cross-sectional dependence in the data. We investigated the order of co-integration, unit roots, and stationarity in the data. To identify the basic roots in the panel across various variables, we preferred first- and second-generation tests, i.e., LLC, IPS and ADF-Fisher as a first-generation technique to explore unit root in the data, and Pesaran (2007), and Breitung and Pesaran (2008) as second-generation tools. We employed second-generation techniques, as they are very supportive in cases where the data exhibit serious cross-correlation. Both categories of tests, i.e., first generation and second generation, outline that the variables exhibit the combination of 1(0) and 1(1) order.
Once panel unit root in each variable was verified, the long-run relationship of the variables was assessed through the co-integration test. In this regard, we applied the Westerland (2007) test to analyze the co-integration between variables (see Table 4). Westerland (2007) is an accurate technique, when the data is highly heterogeneous in the short run and long run (Persyn and Westerlund, 2008). We used data from numerous countries in this region that contain cross-sectional dependence; this is why this co-integration technique was an appropriate choice (Zeeshan et al., 2021). ICT and renewable energy show the group means tests, and Pt and pa show the alternative hypothesis for capturing co-integration in the panel (Persyn and Westerlund, 2008). The outcomes of the test validate the existence of co-integration between variables as a result of the significant values of both ICT and Pop, and this clearly outlines the rejection of a null hypothesis for co-integration. Based on the significant test values, long-run co-integration in our data is pervasive.
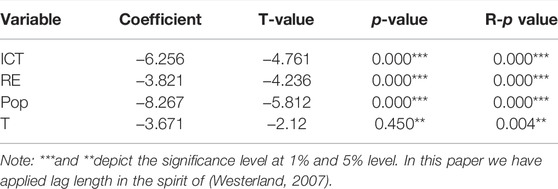
TABLE 4. Co-integration technique (Westerland, 2007).
We also apply Pedroni (1999), and Kao (1999) tests for analyzing co-integration between variables for more validation. Table 5 depicts the Pedroni co-integration tests based on seven diverse statistics. Based on the tabulated values there is a co-integrating relationship in the variables of this paper. Similarly, in Table 6, which shows the results of the Kao co-integration test, the statistics are significant at a 1% probability level, thereby rejecting the null hypothesis of no co-integration. Thus, all three co-integration tests validate the existence of co-integration between variables.
In Table 7, the results of quantile regression are displayed. Our results showed that ICT was significant in the majority of quantiles with positive signs, implying that an increase in ICT will increase the level of CO2 emissions in these countries. In our results, the majority of quantiles showed coefficients significant at a 10% probability level. The results suggested that an increase in ICT and technological advancement in these countries could be effective in production, but showed a marginally significant contributing effect in CO2 emissions. Our results were in line with many previous studies, e.g., Lee and Brahmasrene (2014) for ASEAN, Avom et al. (2020) for sub-Saharan African countries. Our results for renewable energy showed that renewable energy negatively affected CO2 emissions in South-East Asia, demonstrating that the use of renewable energy could be effective in promoting environmental quality and sustainability. Almost seven quantiles out of ten were statistically significant at a 10% probability level. Hence, we concluded that renewable energy was effective in reducing CO2 emissions in South-East Asian countries, and could be a focus point for policymakers in the region as plays a role in environmental sustainability. Many previous studies also argued that renewable energy significantly contributed to environmental sustainability (Ullah et al., 2019; Borowski, 2021; Attia et al., 2022). Both trade and population were shown to have a significant effect on CO2 emissions, confirming that more trade and population increases can badly affect environmental quality.
Table 8 illustrates the results of Dynamic Fixed Effect embodying the short-run and long-run effects of ICT and renewable energy on environmental quality in South-East Asian countries. The results demonstrated that the ICT coefficient was statistically significant in the short run, implying that an increase in ICT platforms and technologies increased the volume of CO2 emission in South-East Asia. However, in the long-run, the coefficient was positive, but insignificant status which determine that due to advancement, mostly energy efficient measures are being underway to cater for environmental sustainability which would definitely contribute to lower down the volume of CO2 emission. Renewable energy showed statistically significant coefficients in both the short run and the long run, with an increase in significance in the long run, suggesting that in the long run more deliberate use of renewable energy in the manufacturing sector and households could be very impactful. Both trade and population had statistically significant relationships with CO2 emissions in both the short and long run. However, in the long run the results are comparatively less impactful on CO2 emissions, which means that due to efficient energy in both trade and households levels in this region would reduce to some extent the level of CO2 emission. Both trade and population showed a significant positive effect on CO2 emissions, suggesting that population and trade liberalization both account for environmental degradation in the short and long run in this region.
Conclusion and Policy Implications
The tremendous growth in ICT, advanced technologies, and renewable energy have significantly contributed to environmental sustainability across the globe. Their significant contributions to environmental sustainability and environmental quality have attracted academics to comprehensively explore ICT, block chain, and renewable energy. This study focused on the South-East Asian region, with a view to ascertaining the impact of ICT and renewable energy on environmental quality. Our empirical results investigated both the short- and long-run relationship between ICT and renewable energy, and environmental quality, and revealed that ICT deliberation in this region contributed positively to the amount of CO2 emissions, and that ICT caused environmental degradation, which may be as a result of the use of fossil fuels in the majority of trade-related technologies and none energy efficient technologies. The empirical results of both estimation techniques, i.e., Penal Quantile regression and Dynamic Fixed Effect, showed positive coefficients for ICT in both the short and long run, hence we noticed that ICT did not improve environmental quality in the region, rather it caused environmental degradation. Some of the previous research also highlighted similar footprints of ICT with environmental quality (Shobande and Ogbeifun, 2022; Zafar et al., 2022).
Our results also highlighted the impact of renewable energy on environmental performance in this part of the world, and showed that renewable energy contributed to environmental quality. Hence, our results are in line with numerous studies that also explored the positive contribution of renewable energy to a country’s environmental performance (Chopra et al., 2022; Suki et al., 2022; Usman and Balsalobre-Lorente, 2022). Both trade and population in this region increased CO2 emissions, which implies that more trade activities and a consistent increase in population size caused environmental degradation.
The study has numerous implications. It could be vital for policymakers in this region as the findings clearly outline the negative effects of ICT on environmental performance, which suggests that think tanks dealing with these areas in this region must use efficient energy in for ICT equipment to bring down greenhouse gas emissions. The strategic formulation should be of such nature that it would encourage the use of green energy and efficient energy in the use of ICT. The national governments in this region should make laws to encourage efficient energy based on ICT equipment at the corporate, government and household level. The findings of the study showed the significant contributions of renewable energy to environmental quality in this region, thereby suggesting that each country must be very prone to efficient energy use at both the corporate and society level. This could be very helpful in developing trends for renewable energy use that will contribute to environmental sustainability of this region. The study could be further extended to compare various developing regions, and a new study comparing two different developed regions to explore the relationship between these variables could also be valuable.
Data Availability Statement
The raw data supporting the conclusions of this article will be made available by the authors, without undue reservation.
Author Contributions
MZ: conceptualization, data curation, writing the original draft. JH: data curation, visualization, writing review, and editing. AR: methodology, visualization, supervision, editing. IU: review and editing. supervision, editing and MM writing review and editing and software.
Funding
Ministry of Education Humanities and Social Science Planning Fund Project. (21YAZH012).
Conflict of Interest
The authors declare that the research was conducted in the absence of any commercial or financial relationships that could be construed as a potential conflict of interest.
Publisher’s Note
All claims expressed in this article are solely those of the authors and do not necessarily represent those of their affiliated organizations, or those of the publisher, the editors and the reviewers. Any product that may be evaluated in this article, or claim that may be made by its manufacturer, is not guaranteed or endorsed by the publisher.
Acknowledgments
I would like to extend my thankfulness to my supervisor JH for his help and guidance in making sure this work has been carried out in a timely and suitable way.
References
Ahmad, N., Du, L., Lu, J., Wang, J., Li, H.-Z., and Hashmi, M. Z. (2017). Modelling the CO 2 Emissions and Economic Growth in Croatia: Is There Any Environmental Kuznets Curve? Energy 123, 164–172. doi:10.1016/j.energy.2016.12.106
Akarca, A., and Long, T. (1980). Dynamic Modeling Using Advanced Time Series Techniques: Energy GNP and Energy-Employment Interactions. Chicago, Illinois, USA: Energy Modeling II, Institute of Gas Technology, 501–516.
Al-Mulali, U., Sheau-Ting, L., and Ozturk, I. (2015a). The Global Move toward Internet Shopping and its Influence on Pollution: an Empirical Analysis. Environ. Sci. Pollut. Res. 22 (13), 9717–9727. doi:10.1007/s11356-015-4142-2
Al-Mulali, U., Solarin, S. A., and Ozturk, I. (2016). Investigating the Presence of the Environmental Kuznets Curve (EKC) Hypothesis in Kenya: an Autoregressive Distributed Lag (ARDL) Approach. Nat. Hazards 80 (3), 1729–1747. doi:10.1007/s11069-015-2050-x
Al-Mulali, U., Weng-Wai, C., Sheau-Ting, L., and Mohammed, A. H. (2015b). Investigating the Environmental Kuznets Curve (EKC) Hypothesis by Utilizing the Ecological Footprint as an Indicator of Environmental Degradation. Ecol. Indic. 48, 315–323. doi:10.1016/j.ecolind.2014.08.029
Alam, M. S., and Paramati, S. R. (2016). The Impact of Tourism on Income Inequality in Developing Economies: Does Kuznets Curve Hypothesis Exist? Ann. Tour. Res. 61, 111–126. doi:10.1016/j.annals.2016.09.008
Alamoush, A. S., Ballini, F., and Ölçer, A. I. (2020). Ports' Technical and Operational Measures to Reduce Greenhouse Gas Emission and Improve Energy Efficiency: A Review. Mar. Pollut. Bull. 160, 111508. doi:10.1016/j.marpolbul.2020.111508
Ali, A., and Haseeb, M. (2019). Radio Frequency Identification (RFID) Technology as a Strategic Tool towards Higher Performance of Supply Chain Operations in Textile and Apparel Industry of Malaysia. Uncertain. Supply Chain Manag. 7 (2), 215–226. doi:10.5267/j.uscm.2018.10.004
An, C.-B., and Jeon, S.-H. (2006). Demographic Change and Economic Growth: An Inverted-U Shape Relationship. Econ. Lett. 92 (3), 447–454. doi:10.1016/j.econlet.2006.03.030
Anastacio, J. A. R. (2017). Economic Growth, CO2 Emissions and Electric Consumption: Is There an Environmental Kuznets Curve? an Empirical Study for North America Countries. Int. J. Energy Econ. Policy 7 (2).
Apergis, N., and Payne, J. E. (2010). Renewable Energy Consumption and Economic Growth: Evidence from a Panel of OECD Countries. Energy policy 38 (1), 656–660. doi:10.1016/j.enpol.2009.09.002
Apergis, N., and Payne, J. E. (2012). Tourism and Growth in the Caribbean - Evidence from a Panel Error Correction Model. Tour. Econ. 18 (2), 449–456. doi:10.5367/te.2012.0119
Asafu-Adjaye, J. (2000). The Relationship between Energy Consumption, Energy Prices and Economic Growth: Time Series Evidence from Asian Developing Countries. Energy Econ. 22 (6), 615–625. doi:10.1016/s0140-9883(00)00050-5
Asongu, S. A., and Nwachukwu, J. C. (2016). The Mobile Phone in the Diffusion of Knowledge for Institutional Quality in Sub-saharan Africa. World Dev. 86, 133–147.
Attia, S., Kosiński, P., Wójcik, R., Węglarz, A., Koc, D., and Laurent, O. (2022). Energy Efficiency in the Polish Residential Building Stock: A Literature Review. J. Build. Eng. 45, 103461. doi:10.1016/j.jobe.2021.103461
Avgerou, C. (2003). “The Link between ICT and Economic Growth in the Discourse of Development,” in Organizational Information Systems in the Context of Globalization (Springer), 373–386. doi:10.1007/978-0-387-35695-2_23
Avom, D., Nkengfack, H., Fotio, H. K., and Totouom, A. (2020). ICT and Environmental Quality in Sub-saharan Africa: Effects and Transmission Channels. Technol. Forecast. Soc. Change 155, 120028. doi:10.1016/j.techfore.2020.120028
Awad, A., and Abugamos, H. (2017). Income-carbon Emissions Nexus for Middle East and North Africa Countries: a Semi-parametric Approach. Int. J. Energy Econ. Policy 7 (2).
Bassett, G., and Koenker, R. (1978). Asymptotic Theory of Least Absolute Error Regression. J. Am. Stat. Assoc. 73 (363), 618–622. doi:10.1080/01621459.1978.10480065
Belkhir, L., and Elmeligi, A. (2018). Assessing ICT Global Emissions Footprint: Trends to 2040 & Recommendations. J. Clean. Prod. 177, 448–463. doi:10.1016/j.jclepro.2017.12.239
Ben Jebli, M. (2016). On the Causal Links between Health Indicator, Output, Combustible Renewables and Waste Consumption, Rail Transport, and CO2 Emissions: the Case of Tunisia. Environ. Sci. Pollut. Res. Int. 23 (16), 16699–16715. doi:10.1007/s11356-016-6850-7
Bhujabal, P., and Sethi, N. (2020). Foreign Direct Investment, Information and Communication Technology, Trade, and Economic Growth in the South Asian Association for Regional Cooperation Countries: An Empirical Insight. J. Public Aff. 20 (1), e2010. doi:10.1002/pa.2010
Bloch, H., Rafiq, S., and Salim, R. (2015). Economic Growth with Coal, Oil and Renewable Energy Consumption in China: Prospects for Fuel Substitution. Econ. Model. 44, 104–115.
Bollou, F. A. (2010). Interrogating the Impact of ICT Infrastructure Expansion in Francophone West Africa 1993-2005: A Critical Theory Study Using Archival Data and Non-parametric Research Methods. University of Cape Town.
Borowski, P. F. (2021). Innovative Processes in Managing an Enterprise from the Energy and Food Sector in the Era of Industry 4.0. Processes 9 (2), 381. doi:10.3390/pr9020381
Boutabba, M. A. (2014). The Impact of Financial Development, Income, Energy and Trade on Carbon Emissions: Evidence from the Indian Economy. Econ. Model. 40, 33–41. doi:10.1016/j.econmod.2014.03.005
Breitung, J., and Pesaran, M. H. (2008). “Unit Roots and Cointegration in Panels,” in The Econometrics of Panel Data (Springer), 279–322.
Breusch, T. S., and Pagan, A. R. (1980). The Lagrange Multiplier Test and its Applications to Model Specification in Econometrics. Rev. Econ. Stud. 47 (1), 239–253. doi:10.2307/2297111
Cai, W., Zhang, C., Suen, H. P., Ai, S., Bai, Y., Bao, J., et al. (2021). The 2020 China Report of the Lancet Countdown on Health and Climate Change. Lancet Public Health 6 (1), e64–e81. doi:10.1016/s2468-2667(20)30256-5
Cheng, C., Ren, X., Dong, K., Dong, X., and Wang, Z. (2021). How Does Technological Innovation Mitigate CO2 Emissions in OECD Countries? Heterogeneous Analysis Using Panel Quantile Regression. J. Environ. Manag. 280, 111818. doi:10.1016/j.jenvman.2020.111818
Chopra, R., Magazzino, C., Shah, M. I., Sharma, G. D., Rao, A., and Shahzad, U. (2022). The Role of Renewable Energy and Natural Resources for Sustainable Agriculture in ASEAN Countries: Do Carbon Emissions and Deforestation Affect Agriculture Productivity? Resour. Policy 76, 102578. doi:10.1016/j.resourpol.2022.102578
Cole, M. A., and Neumayer, E. (2004). Examining the Impact of Demographic Factors on Air Pollution. Popul. Environ. 26 (1), 5–21. doi:10.1023/b:poen.0000039950.85422.eb
Coleman, S. (2005). New Mediation and Direct Representation: Reconceptualizing Representation in the Digital Age. New Media & Soc. 7 (2), 177–198. doi:10.1177/1461444805050745
Davis, F. D. (1989). Perceived Usefulness, Perceived Ease of Use, and User Acceptance of Information Technology. MIS Q. 13, 319–340. doi:10.2307/249008
Dergiades, T., Martinopoulos, G., and Tsoulfidis, L. (2013). Energy Consumption and Economic Growth: Parametric and Non-parametric Causality Testing for the Case of Greece. Energy Econ. 36, 686–697. doi:10.1016/j.eneco.2012.11.017
Dogan, E., and Turkekul, B. (2016). CO2 Emissions, Real Output, Energy Consumption, Trade, Urbanization and Financial Development: Testing the EKC Hypothesis for the USA. Environ. Sci. Pollut. Res. 23 (2), 1203–1213. doi:10.1007/s11356-015-5323-8
Edo, S., Okodua, H., and Odebiyi, J. (2019). Internet Adoption and Financial Development in Sub‐Saharan Africa: Evidence from Nigeria and Kenya. Afr. Dev. Rev. 31 (1), 144–160. doi:10.1111/1467-8268.12370
Ehigiamusoe, K. U., and Lean, H. H. (2019). Effects of Energy Consumption, Economic Growth, and Financial Development on Carbon Emissions: Evidence from Heterogeneous Income Groups. Environ. Sci. Pollut. Res. 26 (22), 22611–22624. doi:10.1007/s11356-019-05309-5
Erumban, A. A., and Das, D. K. (2016). Information and Communication Technology and Economic Growth in India. Telecommun. Policy 40 (5), 412–431. doi:10.1016/j.telpol.2015.08.006
Frees, E. W. (1995). Assessing Cross-Sectional Correlation in Panel Data. J. Econ. 69 (2), 393–414. doi:10.1016/0304-4076(94)01658-m
García-Quismondo, E., Santos, C., Lado, J., Palma, J., and Anderson, M. A. (2013). Optimizing the Energy Efficiency of Capacitive Deionization Reactors Working under Real-World Conditions. Environ. Sci. Technol. 47 (20), 11866–11872. doi:10.1021/es4021603
Godil, D. I., Sharif, A., Afshan, S., Yousuf, A., and Khan, S. A. R. (2020). The Asymmetric Role of Freight and Passenger Transportation in Testing EKC in the US Economy: Evidence From QARDL Approach. Environ. Sci. Pollut. Res. 27 (24), 30108–30117.
Güngör, H., Abu-Goodman, M., Olanipekun, I. O., and Usman, O. (2021). Testing the Environmental Kuznets Curve with Structural Breaks: the Role of Globalization, Energy Use, and Regulatory Quality in South Africa. Environ. Sci. Pollut. Res. Int. 28 (16), 20772–20783. doi:10.1007/s11356-020-11843-4
Han, J., Zeeshan, M., Ullah, F., Rehman, A., and Afridi, F. E. A. (2022). Trade Openness and Urbanization Impact on Renewable and Non-Renewable Energy Consumption in China. Environ. Sci. Pollut. Res. 29 (3), 41653–41668.
Hassan, S. T., Xia, E., Khan, N. H., and Shah, S. M. A. (2019). Economic Growth, Natural Resources, and Ecological Footprints: Evidence From Pakistan. Environ. Sci. Pollut. Res. 26 (3), 2929–2938.
Hassan, S. T., Danish, S. U.-D., Khan, S. U.-D., Xia, E., and Fatima, H. (2020). Role of Institutions in Correcting Environmental Pollution: An Empirical Investigation. Sustain. Cities Soc. 53, 101901. doi:10.1016/j.scs.2019.101901
Iorember, P. T., Jelilov, G., Usman, O., Işık, A., and Celik, B. (2021). The Influence of Renewable Energy Use, Human Capital, and Trade on Environmental Quality in South Africa: Multiple Structural Breaks Cointegration Approach. Environ. Sci. Pollut. Res. 28 (11), 13162–13174. doi:10.1007/s11356-020-11370-2
Jaforullah, M., and King, A. (2015). Does the Use of Renewable Energy Sources Mitigate CO 2 Emissions? A Reassessment of the US Evidence. Energy Econ. 49, 711–717. doi:10.1016/j.eneco.2015.04.006
Jin, S., and Cho, C. M. (2015). Is ICT a New Essential for National Economic Growth in an Information Society? Gov. Inf. Q. 32 (3), 253–260. doi:10.1016/j.giq.2015.04.007
Kahouli, B., and Omri, A. (2017). Foreign Direct Investment, Foreign Trade and Environment: New Evidence from Simultaneous-Equation System of Gravity Models. Res. Int. Bus. Finance 42, 353–364. doi:10.1016/j.ribaf.2017.07.161
Kao, C. (1999). Spurious Regression and Residual-Based Tests for Cointegration in Panel Data. J. Econ. 90 (1), 1–44. doi:10.1016/s0304-4076(98)00023-2
Kellenberg, D. K. (2008). A Reexamination of the Role of Income for the Trade and Environment Debate. Ecol. Econ. 68 (1-2), 106–115. doi:10.1016/j.ecolecon.2008.02.007
Khan, F. N., Sana, A., and Arif, U. (2020). Information and Communication Technology (ICT) and Environmental Sustainability: a Panel Data Analysis. Environ. Sci. Pollut. Res. 27 (29), 36718–36731. doi:10.1007/s11356-020-09704-1
Khan, I., Hou, F., Le, H. P., and Ali, S. A. (2021). Do natural Resources, Urbanization, and Value-Adding Manufacturing Affect Environmental Quality? Evidence from the Top Ten Manufacturing Countries. Resour. Policy 72, 102109. doi:10.1016/j.resourpol.2021.102109
Koenker, R., and Bassett, G. (1978). Regression Quantiles. Econometrica 46, 33–50. doi:10.2307/1913643
Koenker, R. (2004). Quantile Regression for Longitudinal Data. J. Multivar. Analysis 91 (1), 74–89. doi:10.1016/j.jmva.2004.05.006
Kraft, J., and Kraft, A. (1978). On the Relationship between Energy and GNP. J. Energy Dev. 3, 401–403.
Kueppers, M., Paredes Pineda, S. N., Metzger, M., Huber, M., Paulus, S., Heger, H. J., et al. (2021). Decarbonization Pathways of Worldwide Energy Systems - Definition and Modeling of Archetypes. Appl. Energy 285, 116438. doi:10.1016/j.apenergy.2021.116438
Lee, J. W., and Brahmasrene, T. (2014). ICT, CO2 Emissions and Economic Growth: Evidence from a Panel of ASEAN. Glob. Econ. Rev. 43 (2), 93–109.
Lee, J. W., and Brahmasrene, T. (2016). Tourism Effects on the Environment and Economic Sustainability of Sub-saharan Africa. Int. J. Sustain. Dev. World Ecol. 23 (3), 221–232. doi:10.1080/13504509.2015.1114976
Lewis, J. I. (2010). The Evolving Role of Carbon Finance in Promoting Renewable Energy Development in China. Energy policy 38 (6), 2875–2886. doi:10.1016/j.enpol.2010.01.020
Li, S., Zhou, C., Wang, S., and Hu, J. (2018). Dose Urban Landscape Pattern Affect CO2 Emission Efficiency? Empirical Evidence from Megacities in China. J. Clean. Prod. 203, 164–178.
Lin, B., and Zhu, J. (2019). The Role of Renewable Energy Technological Innovation on Climate Change: Empirical Evidence from China. Sci. Total Environ. 659, 1505–1512. doi:10.1016/j.scitotenv.2018.12.449
Lin, M., Tao, J., Chan, C.-Y., Cao, J.-J., Zhang, Z.-S., Zhu, L.-H., et al. (2012). Regression Analyses between Recent Air Quality and Visibility Changes in Megacities at Four Haze Regions in China. Aerosol Air Qual. Res. 12 (6), 1049–1061. doi:10.4209/aaqr.2011.11.0220
Manfren, M., Sibilla, M., and Tronchin, L. (2021). Energy Modelling and Analytics in the Built Environment-A Review of Their Role for Energy Transitions in the Construction Sector. Energies 14 (3), 679. doi:10.3390/en14030679
Menyah, K., and Wolde-Rufael, Y. (2010). Energy Consumption, Pollutant Emissions and Economic Growth in South Africa. Energy Econ. 32 (6), 1374–1382.
Menz, T., and Welsch, H. (2012). Population Aging and Carbon Emissions in OECD Countries: Accounting for Life-Cycle and Cohort Effects. Energy Econ. 34 (3), 842–849. doi:10.1016/j.eneco.2011.07.016
Mingay, S. (2007). “Green ICT: a New Industry Shockwave,” in Paper Presented at the Gartner Symposium-Itxpo Conference (San Francisco: Gartner, Inc.).
Moore, G. C., and Benbasat, I. (1991). Development of an Instrument to Measure the Perceptions of Adopting an Information Technology Innovation. Inf. Syst. Res. 2 (3), 192–222. doi:10.1287/isre.2.3.192
Moyer, J. D., and Hughes, B. B. (2012). ICTs: Do They Contribute to Increased Carbon Emissions? Technol. Forecast. Soc. Change 79 (5), 919–931. doi:10.1016/j.techfore.2011.12.005
Murthy, N. R. V., and Nath, R. (2009). Determinants of Digital Access: a Global Empirical Analysis. Ijss 5 (2), 95–114. doi:10.1504/ijss.2009.027218
Mutascu, M. (2016). A Bootstrap Panel Granger Causality Analysis of Energy Consumption and Economic Growth in the G7 Countries. Renew. Sustain. Energy Rev. 63, 166–171. doi:10.1016/j.rser.2016.05.055
Nath, H. K., and Liu, L. (2017). Information and Communications Technology (ICT) and Services Trade. Inf. Econ. Policy 41, 81–87. doi:10.1016/j.infoecopol.2017.06.003
Oliveira, T. A., Oliver, M., and Ramalhinho, H. (2020). Challenges for Connecting Citizens and Smart Cities: ICT, E-Governance and Blockchain. Sustainability 12 (7), 2926. doi:10.3390/su12072926
Ozcan, B., and Apergis, N. (2018). The Impact of Internet Use on Air Pollution: Evidence from Emerging Countries. Environ. Sci. Pollut. Res. 25 (5), 4174–4189. doi:10.1007/s11356-017-0825-1
Ozcan, B., Tzeremes, P. G., and Tzeremes, N. G. (2020). Energy Consumption, Economic Growth and Environmental Degradation in OECD Countries. Econ. Model. 84, 203–213. doi:10.1016/j.econmod.2019.04.010
Özokcu, S., and Özdemir, Ö. (2017). Economic Growth, Energy, and Environmental Kuznets Curve. Renew. Sustain. Energy Rev. 72, 639–647.
Panwar, N. L., Kaushik, S. C., and Kothari, S. (2011). Role of Renewable Energy Sources in Environmental Protection: A Review. Renew. Sustain. Energy Rev. 15 (3), 1513–1524. doi:10.1016/j.rser.2010.11.037
Park, Y., Meng, F., and Baloch, M. A. (2018). The Effect of ICT, Financial Development, Growth, and Trade Openness on CO2 Emissions: an Empirical Analysis. Environ. Sci. Pollut. Res. 25 (30), 30708–30719. doi:10.1007/s11356-018-3108-6
Pedroni, P. (1999). Critical Values for Cointegration Tests in Heterogeneous Panels with Multiple Regressors. Oxf. Bull. Econ. statistics 61 (S1), 653–670. doi:10.1111/1468-0084.61.s1.14
Persyn, D., and Westerlund, J. (2008). Error-Correction-Based Cointegration Tests for Panel Data. stata J. 8 (2), 232–241. doi:10.1177/1536867x0800800205
Pesaran, M. H. (2007). A Simple Panel Unit Root Test in the Presence of Cross-Section Dependence. J. Appl. Econ. 22 (2), 265–312. doi:10.1002/jae.951
Pesaran, M. H., Schuermann, T., and Weiner, S. M. (2004). Modeling Regional Interdependencies Using a Global Error-Correcting Macroeconometric Model. J. Bus. Econ. Statistics 22 (2), 129–162. doi:10.1198/073500104000000019
Poumanyvong, P., and Kaneko, S. (2010). Does Urbanization Lead to Less Energy Use and Lower CO2 Emissions? A Cross-Country Analysis. Ecol. Econ. 70 (2), 434–444. doi:10.1016/j.ecolecon.2010.09.029
Pradhan, R. P., Arvin, M. B., and Hall, J. H. (2016). Economic Growth, Development of Telecommunications Infrastructure, and Financial Development in Asia, 1991-2012. Q. Rev. Econ. Finance 59, 25–38. doi:10.1016/j.qref.2015.06.008
Rafiq, S., Bloch, H., and Salim, R. (2014). Determinants of Renewable Energy Adoption in China and India: a Comparative Analysis. Appl. Econ. 46 (22), 2700–2710. doi:10.1080/00036846.2014.909577
Rahman, M., and Mezbah-ul-Islam, M. (2012). Issues and Challenges for Sustainable Digital Preservation Practices in Bangladesh. Available at: https://www.researchgate.net/publication/261178681_Issues_and_Challenges_for_Sustainable_Digital_Preservation_Practices_in_Bangladesh.
Rahman, M. M., Saidi, K., and Mbarek, M. B. (2020). Economic Growth in South Asia: the Role of CO2 Emissions, Population Density and Trade Openness. Heliyon 6 (5), e03903. doi:10.1016/j.heliyon.2020.e03903
Recalde, M. Y. (2021). “Which Aspects May Prevent the Development of Energy Service Companies? the Impact of Barriers and Country-specific Conditions in Different Regions,” in Energy Services Fundamentals and Financing (Elsevier), 293–315. doi:10.1016/b978-0-12-820592-1.00013-0
Riti, J. S., Song, D., Shu, Y., Kamah, M., and Atabani, A. A. (2018). Does Renewable Energy Ensure Environmental Quality in Favour of Economic Growth? Empirical Evidence from China's Renewable Development. Qual. Quant. 52 (5), 2007–2030. doi:10.1007/s11135-017-0577-5
Rot, A., Sobińska, M., Hernes, M., and Franczyk, B. (2020). “Digital Transformation of Public Administration through Blockchain Technology,” in Towards Industry 4.0—Current Challenges in Information Systems (Springer), 111–126. doi:10.1007/978-3-030-40417-8_7
Ruta, M., and Venables, A. J. (2012). International Trade in Natural Resources: Practice and Policy. Annu. Rev. Resour. Econ. 4 (1), 331–352. doi:10.1146/annurev-resource-110811-114526
Saberi, S., Kouhizadeh, M., Sarkis, J., and Shen, L. (2019). Blockchain Technology and its Relationships to Sustainable Supply Chain Management. Int. J. Prod. Res. 57 (7), 2117–2135.
Sadorsky, P. (2012). Correlations and Volatility Spillovers between Oil Prices and the Stock Prices of Clean Energy and Technology Companies. Energy Econ. 34 (1), 248–255. doi:10.1016/j.eneco.2011.03.006
Salahuddin, M., Alam, K., and Ozturk, I. (2016). The Effects of Internet Usage and Economic Growth on CO2 Emissions in OECD Countries: A Panel Investigation. Renew. Sustain. Energy Rev. 62, 1226–1235. doi:10.1016/j.rser.2016.04.018
Salim, R. A., and Islam, N. (2010). Exploring the Impact of R&D and Climate Change on Agricultural Productivity Growth: the Case of Western Australia*. Aust. J. Agric. Resour. Econ. 54 (4), 561–582. doi:10.1111/j.1467-8489.2010.00514.x
Shahbaz, M., Hye, Q. M. A., Tiwari, A. K., and Leitão, N. C. (2013). Economic Growth, Energy Consumption, Financial Development, International Trade and CO2 Emissions in Indonesia. Renew. Sustain. Energy Rev. 25, 109–121. doi:10.1016/j.rser.2013.04.009
Sherwood, B., and Wang, L. (2016). Partially Linear Additive Quantile Regression in Ultra-high Dimension. Ann. Statistics 44 (1), 288–317. doi:10.1214/15-aos1367
Shobande, O. A., and Ogbeifun, L. (2022). Has Information and Communication Technology Improved Environmental Quality in the OECD? -a Dynamic Panel Analysis. Int. J. Sustain. Dev. World Ecol. 29 (1), 39–49. doi:10.1080/13504509.2021.1909172
Shuai, C., Chen, X., Wu, Y., Tan, Y., Zhang, Y., and Shen, L. (2018). Identifying the Key Impact Factors of Carbon Emission in China: Results from a Largely Expanded Pool of Potential Impact Factors. J. Clean. Prod. 175, 612–623. doi:10.1016/j.jclepro.2017.12.097
Silvestre, B. S., and Ţîrcă, D. M. (2019). Innovations for Sustainable Development: Moving toward a Sustainable Future. J. Clean. Prod. 208, 325–332. doi:10.1016/j.jclepro.2018.09.244
Smith, K. M., Wilson, S., and Hassall, M. E. (2021). Could Focusing on Barriers to Industrial Energy Efficiency Create a New Barrier to Energy Efficiency? J. Clean. Prod. 310, 127387. doi:10.1016/j.jclepro.2021.127387
Sokolov-Mladenović, S., Milovančević, M., Mladenović, I., and Alizamir, M. (2016). Economic Growth Forecasting by Artificial Neural Network with Extreme Learning Machine Based on Trade, Import and Export Parameters. Comput. Hum. Behav. 65, 43–45.
Suki, N. M., Suki, N. M., Sharif, A., Afshan, S., and Jermsittiparsert, K. (2022). The Role of Technology Innovation and Renewable Energy in Reducing Environmental Degradation in Malaysia: A Step towards Sustainable Environment. Renew. Energy 182, 245–253. doi:10.1016/j.renene.2021.10.007
Tchamyou, V. S., Asongu, S. A., and Odhiambo, N. M. (2019). The Role of ICT in Modulating the Effect of Education and Lifelong Learning on Income Inequality and Economic Growth in Africa. Afr. Dev. Rev. 31 (3), 261–274. doi:10.1111/1467-8268.12388
Tilt, B. (2019). China's Air Pollution Crisis: Science and Policy Perspectives. Environ. Sci. Policy 92, 275–280. doi:10.1016/j.envsci.2018.11.020
Turkenburg, W. C., Arent, D., Bertani, R., Faaij, A., Hand, M., Krewitt, W., et al. (2012). “Renewable Energy,” in Global Energy Assessment-Toward a Sustainable Future (Cambridge: Cambridge University Press), 761–900.
Ullah, I., Ali, S., Shah, M. H., Yasim, F., Rehman, A., and Al-Ghazali, B. M. (2019). Linkages between Trade, CO2 Emissions and Healthcare Spending in China. Ijerph 16 (21), 4298. doi:10.3390/ijerph16214298
Usman, M., and Balsalobre-Lorente, D. (2022). Environmental Concern in the Era of Industrialization: Can Financial Development, Renewable Energy and Natural Resources Alleviate Some Load? Energy policy 162, 112780. doi:10.1016/j.enpol.2022.112780
Wang, K.-L., Ding, L.-L., Wang, J.-M., and Yang, M. (2020). Analysis of Provincial Total-Factor Air Pollution Efficiency in China by Using Context-dependent Slacks-Based Measure Considering Undesirable Outputs. Nat. Hazards 104 (2), 1899–1921.
Wang, K., Yan, X., Yuan, Y., and Tang, D. (2018). Optimizing Ship Energy Efficiency: Application of Particle Swarm Optimization Algorithm. Proc. Institution Mech. Eng. Part M J. Eng. Marit. Environ. 232 (4), 379–391. doi:10.1177/1475090216638879
Wang, Q., and Wang, S. (2020). Preventing Carbon Emission Retaliatory Rebound Post-COVID-19 Requires Expanding Free Trade and Improving Energy Efficiency. Sci. Total Environ. 746, 141158. doi:10.1016/j.scitotenv.2020.141158
Westerland, C. (2007). String Homology of Spheres and Projective Spaces. Algebr. Geom. Topol. 7 (1), 309–325. doi:10.2140/agt.2007.7.309
Xie, Y., Dai, H., and Dong, H. (2018). Impacts of SO2 Taxations and Renewable Energy Development on CO2, NOx and SO2 Emissions in Jing-Jin-Ji Region. J. Clean. Prod. 171, 1386–1395. doi:10.1016/j.jclepro.2017.10.057
Yadoo, A., and Cruickshank, H. (2012). The Role for Low Carbon Electrification Technologies in Poverty Reduction and Climate Change Strategies: A Focus on Renewable Energy Mini-Grids with Case Studies in Nepal, Peru and Kenya. Energy policy 42, 591–602. doi:10.1016/j.enpol.2011.12.029
Yang, T., and Wang, Q. (2020). The Nonlinear Effect of Population Aging on Carbon Emission-Empirical Analysis of Ten Selected Provinces in China. Sci. Total Environ. 740, 140057. doi:10.1016/j.scitotenv.2020.140057
Yu, H., Yu, X., and Chen, K. (2012). Preliminary Ideas to Establish the Framework of Marine Eco-Compensation Management Mechanism Based on Ecosystem Approach [J]. Ecol. Econ. 8.
Yu, X., Wu, Z., Zheng, H., Li, M., and Tan, T. (2020). How Urban Agglomeration Improve the Emission Efficiency? A Spatial Econometric Analysis of the Yangtze River Delta Urban Agglomeration in China. J. Environ. Manag. 260, 110061. doi:10.1016/j.jenvman.2019.110061
Zafar, M. W., Zaidi, S. A. H., Mansoor, S., Sinha, A., and Qin, Q. (2022). ICT and Education as Determinants of Environmental Quality: The Role of Financial Development in Selected Asian Countries. Technol. Forecast. Soc. Change 177, 121547. doi:10.1016/j.techfore.2022.121547
Zeeshan, M., Han, J., Alam Rehman, H. B., Farooq, N., Waseem, M., Hussain, A., et al. (2021a). Nexus between Foreign Direct Investment, Energy Consumption, Natural Resource, and Economic Growth in Latin American Countries. Int. J. Energy Econ. Policy 11 (1), 407–416.
Zeeshan, M., Han, J., Rehman, A., Ullah, I., and Alam Afridi, F. E. (2021b). Exploring Determinants of Financial System and Environmental Quality in High-Income Developed Countries of the World: the Demonstration of Robust Penal Data Estimation Techniques. Environ. Sci. Pollut. Res. 28, 1–16.
Zhang, C., and Lin, Y. (2012). Panel Estimation for Urbanization, Energy Consumption and CO2 Emissions: A Regional Analysis in China. Energy policy 49, 488–498. doi:10.1016/j.enpol.2012.06.048
Zhang, S., Li, Y., Yuan, H., and Sun, D. (2019). An Alternative Benchmarking Tool for Operational Energy Efficiency of Ships and its Policy Implications. J. Clean. Prod. 240, 118223. doi:10.1016/j.jclepro.2019.118223
Keywords: ICT, renewable energy, trade, environmental quality, PQR, DFE
Citation: Zeeshan M, Han J, Rehman A, Ullah I and Mubashir M (2022) Exploring the Role of Information Communication Technology and Renewable Energy in Environmental Quality of South-East Asian Emerging Economies. Front. Environ. Sci. 10:917468. doi: 10.3389/fenvs.2022.917468
Received: 11 April 2022; Accepted: 29 April 2022;
Published: 30 June 2022.
Edited by:
Munir Ahmad, Zhejiang University, ChinaReviewed by:
Arif Hussain, Abdul Wali Khan University Mardan, PakistanElchin Suleymanov, Baku Enginering University, Azerbaijan
Copyright © 2022 Zeeshan, Han, Rehman, Ullah and Mubashir. This is an open-access article distributed under the terms of the Creative Commons Attribution License (CC BY). The use, distribution or reproduction in other forums is permitted, provided the original author(s) and the copyright owner(s) are credited and that the original publication in this journal is cited, in accordance with accepted academic practice. No use, distribution or reproduction is permitted which does not comply with these terms.
*Correspondence: Jiabin Han, MjcxMzM5MDAzQHFxLmNvbQ==; Irfan Ullah, aXJmYW5lY29uQG51aXN0LmVkdS5jbg==
†ORCID: Muhammed Zeeshan, orcid.org/0000-0003-3525-9429; Jiabin Han, orcid.org/0000-0003-3000-4355; Alam Rehman, orcid.org/0000-0001-9490-979X; Irfan Ullah, orcid.org/0000-0003-3961-888X