- 1Peoples’ Friendship University of Russia, Moscow, Russia
- 2Volgograd State Technical University, Volgograd, Russia
- 3Volgograd State Agricultural University, Volgograd, Russia
Introduction
The economy of artificial intelligence (AI) is a technocratic model of the organization of an economic system in which automation is carried out on the basis of “smart” technologies controlled by AI. This new model emerged as a result of the worldwide technology race and embodies the heyday of scientific and technological progress (Ruffolo, 2022).
Globalization dictates the need for countries of the world to transition to an AI-enabled economy, which is reflected in digital competitiveness, the presence (for example, inclusion in the relevant international rankings) and the level of which determines the ability of countries to overcome barriers to entry and expand their presence in global high-tech markets (Feijóo and Kwon, 2020).
The problem is that the transition to an AI-enabled economy is being forced by the pressure of the market mechanism, and the countries of the world cannot fully adapt it to their priorities, among the most important of which are decarbonization and waste reduction. The reason for the problem is that each new technological way first complements and then displaces the previous one. In this regard, the countries of the world cannot simply overlook the Fourth Industrial Revolution, and they must participate in it in order to be able to demonstrate the growth, development and efficiency of the economy, maintain and improve the standard of living of the population.
The consequence of this problem is the environmental risks of the formation of the AI-enabled economy. This is because AI development pursues economic goals that are often accompanied by environmental costs (Arganda et al., 2022; de Chevalier et al., 2022).
Along with this, it is necessary to take into account that environmental protection is an independent direction of the economy, which is increasingly integrated into economic activity. The Sustainable Development Goals (SDGs) cover both the economic and environmental priorities of humanity, which require full and simultaneous consideration.
The AI economy should participate in achieving the SDGs, comply with them and support them, although modern science does not answer questions about to what extent and by what means this could be achieved in practice (Som, 2021; Wilson et al., 2022).
This article aims to explore the essence of the environmental AI economy and its contribution to decarbonization and waste reduction. The originality and uniqueness of the conducted research is ensured through the use of a systemic approach (based on the provisions of evolutionary economics), which allows more in-depth studies of the logic of formation, cause-and-effect relationships of the functioning of the economy of artificial intelligence and thereby form a holistic view of the economic and environmental aspects of the AI economy.
The Evolution of the Artificial Intelligence Economy
To study the prospects for the development of AI-economy, this article is based on the Evolutionary Theory of Economic Change (evolutionary economics) and the Theory of Bifurcations of dynamic systems (in accordance with the systemic approach to conducting scientific research). Based on these theories and the existing literature, the process of AI-economy evolution is modeled.
Initially (on the first tap), the AI economy emerged as a result of spontaneous technological progress. At that time, economic interests prevailed, according to which, with the help of artificial intelligence, developed digital economies tried to take leading positions in developing high-tech markets.
Subsequently (at the second stage), the AI economy began to serve the commercial interests of business, since the spread of “smart” technologies was financed by venture investments. AI was becoming increasingly widespread in the business environment and no longer had an elitist character. Intense competition had formed in the global high-tech markets.
The third stage is associated with a technological shift, in which the technologies of the previous technological order are replaced by digital technologies. To achieve this, there must be massive public support for the economy of artificial intelligence, which is possible only if “smart” technologies begin to serve the interests of society. Scientists have not yet reached agreement on the characteristics of this stage, since it is currently taking place and is determined by the interests of society.
In the works of Venkatesh et al. (2022), Wise (2022), it is noted that the deep and prolonged global economic depression in the context of the COVID-19 pandemic caused the priority attention of society to the restoration of pre-crisis living standards and the acceleration of global economic growth. This implies a secondary priority to environmental protection issues.
In contrast, in their works Donoso et al. (2022), Gwenzi (2022), Shao et al. (2022) indicate that environmental interests are critically important and come to the fore. This is due, firstly, to the great attention to environmental protection in the SDGs, most of which have an environmental focus.
The popularity of the SDGs around the world is very high, and it continues to grow within the framework of the “decade of action”. Secondly, It is also a widely held view (Argentiero et al. (2022), Felix et al. (2022), Sarmento et al. (2022) that the pandemic and crisis caused by COVID-19 are of an ecological nature, and therefore green growth is urgently needed to overcome highly probable future environmental crises.
We adhere to the second point of view and, based on it, we hypothesize that at the upcoming, but already outlined, third stage, there is a transition to the environmental economy of artificial intelligence, which contributes to decarbonization and waste reduction.
The Formation of the Environmental Artificial Intelligence Economy at the Present Stage of its Evolution and its Contribution to Decarbonization and Waste Reduction.
To determine whether the modern artificial intelligence economy is aimed at environmental sustainability, its impact on decarbonization (CO₂ emissions from fossil fuel combustion and cement production) and waste reduction (municipal solid waste, production-based SO₂ emissions) is assessed. The most accurate indicators for measuring the AI economy are three elements of digital competitiveness: knowledge, technology and future readiness.
In accordance with the generally accepted separation (differentiation) of the elements of digital competitiveness, the available literature suggests different approaches to managing the development of the environmental AI economy for decarbonization and waste reduction:
—In the works of Fytili and Zabaniotou (2022) the basis for the development of the environmental AI economy is knowledge—personnel for the digital economy, highly qualified specialists and a high level of public consciousness. This approach can be called social; —
The publications of Ahmad et al. (2022) indicate the paramount importance of the institutional, financial and telecommunication infrastructure of digital technologies for their use with the purpose of decarbonization and waste reduction. This approach can be called technological;
Khan et al. (2022) in their works note the key role of flexibility and environmental consciousness of society and progressiveness of economy (future readiness), in the presence of which AI is used for decarbonization and waste reduction. This approach can be called adaptive.
To determine the approach that most reliably describes the source of achieving environmental benefits in the development of the AI economy, we will conduct an econometric analysis of available statistical data on digital competitiveness (IMD, 2022), as well as on decarbonization, production and consumption waste (UN, 2022) in 2021. The top 15 countries/territories in artificial intelligence were selected as objects for study according to Nature (2022)—the data are given in Table 1.
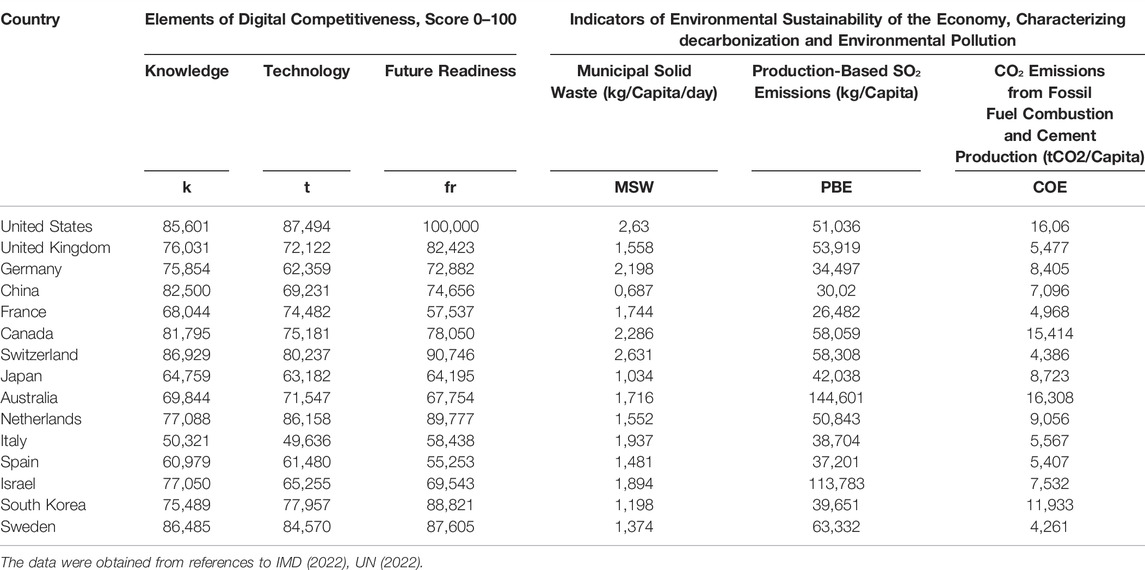
TABLE 1. Elements of digital competitiveness, greenhouse gas emissions and production and consumption waste in top15 countries/territories in artificial intelligence in 2021.
The analysis of the data from Table 1 allowed to obtain the following results. Firstly, it has been established that the technological approach makes it possible to reduce consumption waste due to the development of infrastructure. The dependence was obtained: MSW = 1.04 + 0.002k-0.009t+0.02fr, according to which an increase in the level of technology by 1 point contributes to a reduction of municipal solid waste by 0.009 kg/capita/day. The correlation is moderate: 29.54%.
Secondly, it was revealed that the adaptive approach allows to reduce production waste by increasing the flexibility and environmental consciousness of society and the economy. The dependence was obtained: PBE = 122.84 + 0.83k+0.25t-0.61fr, according to which an increase in the level of future readiness by 1 point contributes to a reduction in production-based so₂ emissions by 0.61 kg/capita. The correlation is moderate: 18.01%.
Thirdly, it has been established that the social approach makes it possible to reduce carbon emissions through the development of knowledge. The dependence was obtained: COE = 2.40–0.09k+0.08t+0.09fr, according to which an increase in the knowledge level by 1 point contributes to a reduction in CO₂ emissions from fossil fuel combustion and cement production by 0.009 tCO2/capita. The correlation is moderate: 32.08%.
Consequently, each of the elements of digital competitiveness is important and makes its own specific contribution for decarbonization and waste reduction, but none of the existing approaches is exhaustive to explain and support the development of the environmental AI economy. Therefore, a new approach is needed that embodies the features of all existing ones.
Systemic Approach to Management of the Development of the Environmental Artificial Intelligence Economy to Maximize Its Contribution to Decarbonization and Waste Reduction.
This paper proposes a systemic approach to management of the development of the environmental AI economy. The new approach is designed to maximize its contribution to decarbonization and waste reduction through targeted and more effective practical application of each of the three elements of digital competitiveness.
In accordance with the authors’ approach, it is recommended to develop the infrastructure of the environmental AI economy for reducing consumption waste. The normative-legal system should strictly regulate consumption waste. Financial incentives for environmental responsibility of the population should be provided, for example, tax incentives and, on the contrary, special environmental penalties. The telecommunications infrastructure should offer a large number of massively available free applications with social advertising for environmental protection, as well as clear instructions on everyone’s contribution to decarbonization and waste reduction (for example, explanation of the principles of safe waste disposal and sorting for subsequent recycling).
It is proposed to increase the flexibility and environmental consciousness of society and progressiveness of economy to reduce production waste. This radically changes the current practice, which involves direct stimulation of business to reduce production waste. In contrast, indirect stimulation through the development of demand for environmentally responsible products is advisable Regulatory control should be replaced by public control, and government fines should be replaced by consumers’ refusal to purchase products from such enterprises that have an insufficiently high level of environmental responsibility (with large volumes of production waste). The AI economy can help with this through automated environmental monitoring of business.
It is recommended to develop knowledge to reduce carbon emissions. Decarbonization should be based on social norms. The danger and harm of greenhouse gases should be explained and clearly understood by every member of society, by every social category. Thanks to this, low-carbon economic practice will become regular, normal. The contribution of the AI economy should be to inform the population about the current carbon emissions and the success achieved in reducing them, as well as to measure the individual contribution of each household and business to the overall result.
Discussion
The conducted research provides new insights into the essence of the environmental AI economy and its contribution to decarbonization and waste reduction. In contrast to Venkatesh et al. (2022), Wise (2022), it is shown that the pandemic and the COVID-19 crisis did not distract, but rather riveted the attention of the world community on environmental protection issues. The AI economy serves these interests by supporting decarbonization and reduction of production and consumption waste.
In contrast to Ahmad et al. (2022), Fytili and Zabaniotou (2022), Khan et al. (2022), it is proved that the development of elements of digital competitiveness separately provides scattered and insignificant results. The existing narrowly focused approaches should be replaced by a systemic approach to management of the development of the environmental AI economy to maximize its contribution to decarbonization and waste reduction, recommendations for practical application of which are proposed in the article.
The contribution of the article to the literature consists in clarifying the cause-and-effect relationships of the development of the environmental AI economy: the contribution of each element of digital competitiveness to achieving the corresponding advantages of decarbonization, reduction of production or consumption waste is determined. The theoretical significance of the article consists in creating a model of the evolution of the artificial intelligence economy and defining the environmental economy of artificial intelligence as its current (ongoing) stage.
The practical significance of the article is ensured by the development of framework applied recommendations in public administration aimed at maximizing the benefits of the current stage of the evolution of the environmental AI economy for decarbonization and waste reduction.
Author Contributions
All authors listed have made a substantial, direct, and intellectual contribution to the work and approved it for publication.
Conflict of Interest
The authors declare that the research was conducted in the absence of any commercial or financial relationships that could be construed as a potential conflict of interest.
Publisher’s Note
All claims expressed in this article are solely those of the authors and do not necessarily represent those of their affiliated organizations, or those of the publisher, the editors and the reviewers. Any product that may be evaluated in this article, or claim that may be made by its manufacturer, is not guaranteed or endorsed by the publisher.
References
Ahmad, M. R., Lao, J., Dai, J.-G., Xuan, D., and Poon, C. S. (2022). Upcycling of Air Pollution Control Residue Waste into Cementitious Product through Geopolymerization Technology. Resour. Conservation Recycl. 181, 106231. doi:10.1016/j.resconrec.2022.106231
Arganda, S., Arganda-Carreras, I., Gordon, D. G., Hoadley, A. P., Pérez-Escudero, A., Giurfa, M., et al. (2022). Statistical Atlases and Automatic Labeling Strategies to Accelerate the Analysis of Social Insect Brain Evolution. Front. Ecol. Evol. 9, 745707. doi:10.3389/fevo.2021.745707
Argentiero, A., D’Amato, A., and Zoli, M. (2022). Waste Recycling Policies and Covid-19 Pandemic in an E-DSGE Model. Waste Manag. 141, 290–299. doi:10.1016/j.wasman.2021.12.036
de Chevalier, G., Bouret, S., Bardo, A., Simmen, B., Garcia, C., and Prat, S. (2022). Cost-Benefit Trade-Offs of Aquatic Resource Exploitation in the Context of Hominin Evolution. Front. Ecol. Evol. 10, 812804. doi:10.3389/fevo.2022.812804
Donoso, I., Fricke, E. C., Hervías-Parejo, S., Rogers, H. S., and Traveset, A. (2022). Drivers of Ecological and Evolutionary Disruptions in the Seed Dispersal Process: Research Trends and Biases. Front. Ecol. Evol. 10, 794481. doi:10.3389/fevo.2022.794481
Feijóo, C., and Kwon, Y. (2020). AI Impacts on Economy and Society: Latest Developments, Open Issues and New Policy Measures. Telecommun. Policy 44 (6), 101987. doi:10.1016/j.telpol.2020.101987
Felix, C. B., Ubando, A. T., Chen, W.-H., Goodarzi, V., and Ashokkumar, V. (2022). COVID-19 and Industrial Waste Mitigation via Thermochemical Technologies towards a Circular Economy: A State-Of-The-Art Review. J. Hazard. Mater. 423, 127215. doi:10.1016/j.jhazmat.2021.127215
Fytili, D., and Zabaniotou, A. (2022). Organizational, Societal, Knowledge and Skills Capacity for a Low Carbon Energy Transition in a Circular Waste Bioeconomy (CWBE): Observational Evidence of the Thessaly Region in Greece. Sci. Total Environ. 813, 151870. doi:10.1016/j.scitotenv.2021.151870
Gurnani, B., Venkatesh, R., and Kaur, K. (2022). Impact of COVID-19 Pandemic on Carbon Footprint and Strategies to Mitigate Waste Generation. Indian J. Ophthalmol. 70 (2), 690–691. doi:10.4103/ijo.IJO_2945_21
Gwenzi, W. (2022). Wastewater, Waste, and Water-Based Epidemiology (WWW-BE): A Novel Hypothesis and Decision-Support Tool to Unravel COVID-19 in Low-Income Settings? Sci. Total Environ. 806, 150680. doi:10.1016/j.scitotenv.2021.150680
IMD (2022). World Digital Competitiveness Ranking – 2021. Available at: https://www.imd.org/centers/world-competitiveness-center/rankings/world-digital-competitiveness/.
Khan, S., Anjum, R., Raza, S. T., Ahmed Bazai, N., and Ihtisham, M. (2022). Technologies for Municipal Solid Waste Management: Current Status, Challenges, and Future Perspectives. Chemosphere 288, 132403. doi:10.1016/j.chemosphere.2021.132403
Nature (2022). Top 25 Countries/territories in Artificial Intelligence. Available at: https://www.natureindex.com/supplements/nature-index-2020-ai/tables/countries.
Ruffolo, M. (2022). The Role of Ethical AI in Fostering Harmonic Innovations that Support a Human-Centric Digital Transformation of Economy and Society. Lect. Notes Netw. Syst. 282, 139–143. doi:10.1007/978-3-030-81190-7_15
Sarmento, P., Motta, M., Scott, I. J., Pinheiro, F. L., and de Castro Neto, M. (2022). Impact of COVID-19 Lockdown Measures on Waste Production Behavior in Lisbon. Waste Manag. 138, 189–198. doi:10.1016/j.wasman.2021.12.002
Shao, P., Han, H., Yang, H., Li, T., Zhang, D., Ma, J., et al. (2022). Responses of above- and Belowground Carbon Stocks to Degraded and Recovering Wetlands in the Yellow River Delta. Front. Ecol. Evol. 10, 856479. doi:10.3389/fevo.2022.856479
Som, T. (2021). Sustainability in Energy Economy and Environment: Role of AI Based Techniques. Model. Optim. Sci. Technol. 18, 647–682. doi:10.1007/978-3-030-72929-5_31
UN (2022). Sustainable Development Report 2021. Available at: https://dashboards.sdgindex.org/rankings.
Wilson, M., Paschen, J., and Pitt, L. (2022). The Circular Economy Meets Artificial Intelligence (AI): Understanding the Opportunities of AI for Reverse Logistics. Meq 33 (1), 9–25. doi:10.1108/MEQ-10-2020-0222
Keywords: artificial intelligence, the environmental AI economy, decarbonization, waste reduction, the sustainable development goals
Citation: Ragulina YV, Dubova YI, Litvinova TN and Balashova NN (2022) The Environmental AI Economy and its Contribution to Decarbonization and Waste Reduction. Front. Environ. Sci. 10:914003. doi: 10.3389/fenvs.2022.914003
Received: 06 April 2022; Accepted: 14 April 2022;
Published: 15 June 2022.
Edited by:
Elena G. Popkova, Moscow State Institute of International Relations, RussiaReviewed by:
Yelena Petrenko, Plekhanov Russian University of Economics, RussiaSadriddin Khudaykulov, Tashkent State Economic University, Uzbekistan
Copyright © 2022 Ragulina, Dubova, Litvinova and Balashova. This is an open-access article distributed under the terms of the Creative Commons Attribution License (CC BY). The use, distribution or reproduction in other forums is permitted, provided the original author(s) and the copyright owner(s) are credited and that the original publication in this journal is cited, in accordance with accepted academic practice. No use, distribution or reproduction is permitted which does not comply with these terms.
*Correspondence: Tatiana Nikolaevna Litvinova, bGl0dmlub3ZhX3RfbkBtYWlsLnJ1