- College of Geological Engineering and Geomatics, Chang’an University, Xi’an, China
The increasing trend in drought events under the background of global warming makes it more important to understand the drought effect on vegetation photosynthesis. While diverse global gross primary production (GPP) datasets were adopted to investigate the drought impact on photosynthesis, few studies focused on the discrepancies of drought response among different GPP datasets, especially for the cumulative drought impact. Therefore, a total of twenty-six global GPP datasets based on process, machine learning (ML), and light-use efficiency (LUE) model schemes were obtained to appraise the cumulative impact of drought stress on photosynthesis from 2001 to 2010. Moreover, a relatively reliable global pattern of drought’s cumulative effect on vegetation photosynthesis was acquired from these global GPP products through probability analysis. The results illustrated that the cumulative impact of drought existed in 52.11% of vegetation cover land with the cumulative time scales dominantly at a short term (1–4 months, 31.81%). Obvious heterogeneity of the drought cumulative effect in space and different vegetation functional types was observed, as the reliability of the drought effect decreased with latitude decreasing and a higher sensitivity to drought in herbaceous vegetation than woody plants. Our findings highlighted the importance of ways in characterizing moisture conditions across vegetation types among various GPP models and the necessity of GPP dataset selection in investigating drought effect on photosynthesis.
1 Introduction
Global climate change will cause significant effects on plant photosynthesis (Cramer et al., 2001; Richardson et al., 2013; Chen et al., 2014), especially with an increasing trend of infrequently severe drought events under the background of global warming (AghaKouchak et al., 2014; Naumann et al., 2018). In this way, it is essential to understand the various effects of drought on vegetation status and further investigate the terrestrial ecosystem carbon cycle (van der Molen et al., 2011; Barman et al., 2014; Anderegg et al., 2015).
Drought, as a complex and intermittent disturbance among climatic phenomena, can influence the traits of the terrestrial ecosystem and vegetation status (van der Molen et al., 2011; Reichstein et al., 2013; Anderegg et al., 2015), such as reducing the expansion of foliage and stomatal conductance (Passioura, 1991), inducing plant mortality (Huang et al., 2010; Rao et al., 2019), or even causing biotic disturbance and wildfire (Wendler et al., 2011; Huang et al., 2017). As the observable changes in plant status induced by drought are hard to be timely detected, it is difficult to understand the reactions of the plant to drought (Zhao et al., 2020). Moreover, except for the current moisture conditions, early drought events may also control vegetation growth (Peng et al., 2019; Yuan et al., 2020). In this way, knowing the various time-scales of plant reactions to the drought effect is critical for understanding the interactions between climate and plants (Zhao et al., 2018; Peng et al., 2019; Wen et al., 2019). The drought effect on plants can be summarized in the cumulative and time-lag responses primarily (Zhao et al., 2020). Time-lag impacts illustrate the effect of early drought events at a specific time on the current plant status, and cumulative impacts are associated with lagged effects and climatic dynamics during a given period (Zhao et al., 2020). While there were an increasing number of studies that surveyed the time-lag impact of drought on the plant, it was found that it varies across species and ranges (Braswell et al., 1997; Huang et al., 2018; Peng et al., 2019). Some cumulative events (e.g., cumulative water deficits) could intensify drought effects on terrestrial ecosystems (Ivits et al., 2016). Therefore, it is necessary to assess the cumulative drought impact on global plant photosynthesis more robustly.
In general, the response sensitivity of vegetation photosynthesis (i.e., GPP) to dry conditions at the ecosystem scale was used to characterize drought effect on vegetation (Ciais et al., 2005; Sun et al., 2021). With the development of satellite remote sensing technology (Xiao et al., 2019; Chu et al., 2021; Pan et al., 2021; Guo et al., 2022), massive remotely sensed images provided unique opportunities for researching the ecosystem carbon cycle at multiple scales (e.g., regional, continental, or global). On this basis, many researchers have established a variety of models driven by remote sensing data to simulate GPP over the past few decades (Tan et al., 2012; Anav et al., 2015; Lees et al., 2018; Xie and Li, 2020b; a). Generally, all these remotely sensed data-driven GPP models could be divided into three parts based on different schemes, namely, process, machine learning (ML), and light-use efficiency (LUE) models. The process-oriented models always comprehensively considered the effects of several main biophysical and chemical processes on vegetation photosynthesis over the terrestrial ecosystem (Ito, 2010; Hayes et al., 2011; Tian et al., 2011; Zhu et al., 2014; Jiang and Ryu, 2016). In this way, GPP estimations obtained from them were more mechanistic and rigorous, while the ML models established complex nonlinear statistical relations between physiologically relevant input data and GPP, thus needing huge amounts of data for model training (Tramontana et al., 2016; Zheng et al., 2020). For LUE models, which simulated GPP through the theory of radiation conversion efficiency (Monteith, 1972), it is assumed that GPP had a direct relationship with the combination of absorbed photosynthetically active radiation and factual LUE (Xiao et al., 2004; Yuan et al., 2010; Guan et al., 2022) as the factual LUE was linked with the potential ceiling amount of LUE and various regulations of environmental factors on it (Hilker et al., 2008; Guan et al., 2021). Based on these various models and large amounts of remotely sensed and auxiliary data (e.g., meteorological reanalysis product), diverse GPP datasets have been generated and used to investigate the global climate change and carbon cycle (Campbell et al., 2017; Curasi et al., 2019). However, previous studies indicated that the model structures, model parameters, and input data among different models could bring uncertainties to final GPP simulations (Zhao et al., 2006; Xiao et al., 2011; Sanchez et al., 2015; Zhou et al., 2016; Zheng et al., 2018). In this way, the global pattern of drought effect on photosynthesis acquired from different GPP products might exist in unavoidable discrepancies.
As there were more and more global GPP datasets that could be accessed directly online (Zhang et al., 2017; Huntzinger et al., 2018; Zheng et al., 2020), they provided a feasible opportunity to derive a more reliable and robust global pattern of drought impact on vegetation photosynthesis. Considering these findings mentioned above, this study aimed to evaluate the cumulative impact of drought on plant photosynthesis quantitatively based on multiple global GPP products and a multiscale time-series global Standardized Precipitation Evapotranspiration Index (SPEI) dataset. Here, the major objectives of this work were as follows: 1) to explore the spatial pattern of cumulative drought impact on global photosynthesis, 2) to analyze the discrepancies of drought cumulative effect on photosynthesis across different vegetation types, and 3) to derive a global reliability pattern of drought cumulative effect on photosynthesis using the probability statistic based on numerous global GPP products.
2 Data and Methods
2.1 Datasets
2.1.1 Global GPP Products
In this study, a total of twenty-six global GPP products were acquired to evaluate the cumulative drought impact on photosynthesis. These GPP products contain seventeen process-oriented models (i.e., BESS, BIOME-BGC, CLASS-CTEM-N+, CLM4, CLM4VIC, DLEM, GTEC, ISAM, JULES, LPJ-wsl, ORCHIDEE-LSCE, SIB3, SIBCASA, TEM6, TRIPLEX-GHG, VEGAS2.1, and VISIT), five LUE models (i.e., EC-LUE, MOD17, OPT-LUE, RC-LUE, and VPM), and four ML models (i.e., ANN, MARS, MTE, and RF). While these GPP datasets had different spatial and temporal resolutions, all GPP datasets were processed into a uniform format (i.e., 0.5° and monthly) based on the spatial average resampling and accumulated GPP within a month. In addition, the overlapped years of these GPP datasets (i.e., 2001–2010) were used as the study period in this work. More detailed information about these GPP datasets can be found in Supplementary Material.
2.1.2 Multiscale Global SPEI Data
In this work, monthly SPEI data at multiple time scales (1–12 months) from 2001 to 2010 were acquired from the SPEIbase v.2.5 datasets (Beguería et al., 2017) to identify the duration and intensity of drought. This product contained monthly SPEI data at 1–48 months’ time-scales from 1901–2015 with a spatial resolution of 0.5°, while SPEI at i time-scale (i.e., i month SPEI) represented the cumulative climatic moisture cycling process for the earlier i months. In this way, SPEI data can be used to characterize different types of drought (e.g., short, middle, and long period drought) and various effects on vegetation status (Begueria et al., 2010; Vicente-Serrano et al., 2010; Peng et al., 2019). As for this product, the SPEI value at each grid was computed through the discrepancy among precipitation and reference evapotranspiration first, and then the final SPEI value was a standardized parameter related to the climatic moisture cycling process that obeys a log-logistic distribution (Vicente-Serrano et al., 2010). In addition, a more large positive SPEI value indicated surplus water supply after meeting the coincident moisture requirement, and a smaller negative value represented a more exigent water deficit (Begueria et al., 2014). In this way, SPEI was a reasonable parameter to characterize the degree of drought. Here, the 1–12 month SPEI data for 2001–2010 were adopted to explore the drought’s cumulative impact on global photosynthesis according to previous studies (Kang et al., 2018; Peng et al., 2019).
2.1.3 Global Land Cover Data
In this work, moderate-resolution imaging spectroradiometer yearly global land cover types dataset Version 6 (MCD12C1 v006) was adopted to compare the cumulative effect on photosynthesis across vegetation types. The MCD12C1 dataset (Friedl and Sulla-Menashe., 2015) provided three land cover classification scheme layers at 0.05° spatial resolution yearly, and the International Geosphere–Biosphere Program (IGBP) layer was used in this work. Moreover, the 0.05° resolution land cover maps were resampled to the 0.5° resolution ones based on the assumption that the land cover of every 0.5- grid was the major stamp (i.e., with the maximal area percentage) among all the 0.05° subpixels. As for demonstrating the cumulative drought impact on global photosynthesis and reducing the uncertainties attracted by land cover alterations, merely the unchanged vegetation cover pixels (i.e., these pixels that remained the same vegetation type during 2001–2010) were selected as the study area. In this way, the unchanged vegetation cover map is based on the IGBP scheme as shown in Figure 1. A total of eleven vegetation types were selected from the unchanged vegetation cover map (the total amount of pixels was 77,930, accounting for 93.04% of all the land surface). All the used vegetation types, their total numbers of pixels, and area proportions to the land surface are shown below: deciduous broadleaf forests (DBFs, 941, 1.12%), deciduous needleleaf forests (DNFs, 120, 0.14%), evergreen broadleaf forests (EBFs, 4008, 4.79%), evergreen needleleaf forests (ENFs, 1144, 1.37%), mixed forests (MFs, 2204, 2.63%), closed shrublands (CSHs, 116, 0.14%), open shrublands (OSHs, 6638, 7.93%), savannas (SAVs, 6217, 7.42%), woody savannas (WSAs, 4412, 5.27%), croplands (CROs, 4717, 5.63%), and grasslands (GRAs, 12592, 15.03%).
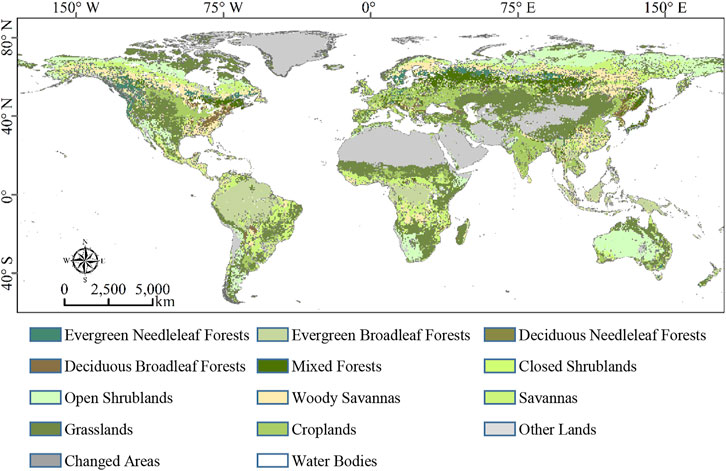
FIGURE 1. Spatial distribution of unchanged IGBP land cover types obtained from MCD12C1 Version six during the study period.
2.2 Determining the Cumulative Drought Impact on Plant Photosynthesis
The cumulative drought effect on photosynthesis was concluded through correlation analysis in which time scale SPEI (i.e., any month in 1–12 months) had the maximum significant correlation with monthly GPP. For example, assuming that the i month SPEI data (i could be anyone in the range of 1–12) showed the largest correlation with monthly GPP at a pixel, the cumulative impact of drought on photosynthesis would be set as i months for this pixel, which indicated that the earlier i month climatic water balance was important to affect vegetation photosynthesis. The specific processes to determine the cumulative effect of drought for each GPP dataset could be summarized into three steps. At first, time series monthly GPP product and 1–12 months SPEI in the timeperiod of 2001–2010 were extracted. Second, the reaction of monthly GPP to 1–12 months SPEI was characterized through Pearson’s correlation coefficient, while the significance level (i.e., p) was set to 0.05 for each pixel. Third, the accumulated months and intensity of drought cumulative impact on photosynthesis was determined as i month and Rmax-cum (Rmax-cum with a range of −1 to 1), while the absolute largest significant Pearson’s correlation coefficient (i.e., |Rmax-cum| when p < 0.05) happened between monthly GPP and i month SPEI.
2.3 Statistical Analyses
Based on Pearson’s correlation coefficients between monthly GPP data and multiscale monthly SPEI, the Rmax-cum and accumulated mouth maps were obtained for each GPP dataset according to the method mentioned in Section 2.2. Furthermore, in order to compare the cumulative drought impacts on global photosynthesis which were acquired through different GPP datasets, the Rmax-cum were divided into three types, including a significant positive correlation (i.e., Rmax-cum > 0 when p < 0.05), significant negative correlation (i.e., Rmax-cum < 0 when p < 0.05), and no significant correlation (i.e., p ≥ 0.05). Moreover, the accumulated months could be ranged likewise into three terms, namely, short term (1 ≤ months ≤4), medium term (5 ≤ months ≤8), and long term (9 ≤ months ≤12). In this way, there were both three possible results of Rmax-cum (i.e., positive, negative, and no significant correlation) and accumulated months (i.e., short, medium, and long term) for different GPP datasets at each pixel. Based on these results of Rmax-cum acquired from multiple GPP datasets, the final Rmax-cum outcome for each pixel was determined through probability statistics wherein one result had the highest proportion within all these GPP datasets. Moreover, the reliability (Re) of the final Rmax-cum for each pixel was determined as follows:
where NRmax-cum represented the total number of final Rmax-cum from all GPP datasets and NGPP was the total amount of GPP products. At the same time, the final accumulated month outcome and its reliability for each pixel were also obtained using the same processes as the final Rmax-cum.
As for comparing the cumulative drought impact on photosynthesis across vegetation types, the percentage (Pe) of different types of Rmax-cum for each vegetation cover was obtained as follows:
where j was the types of Rmax-cum (i.e., positive, negative, and no significant correlation), M was the number of pixels that showed Rmax-cum, and Mall was the total pixels for each vegetation type. Similar to the Rmax-cum, the percentage of different types of accumulated months for each vegetation cover land was also acquired as follows:
where i was the types of accumulated months (i.e., short, medium, and long term), S was the number of pixels showing accumulated months, and Sall was all the significant pixels for one vegetation type. In addition, the variation of mean reliability with latitudes can be obtained as follows:
where ReMean was the averaged reliability for each latitude, P was the reliability of pixels at the same latitude, and i was the number of pixels with reliability for the same latitude.
3 Results
3.1 Spatial Pattern of Drought Cumulative Effect on Photosynthesis
The spatial distribution of Rmax-cum which is based on probability statistics is shown in Figure 2. The results indicated that 52.11% of vegetation cover lands have shown significant Rmax-cum, and the positive correlations had a higher proportion (38.30%) than negative correlations (13.81%). Meanwhile, the positive Rmax-cum was primarily observed in North America, South America, South-central Africa, Central Asia, South Asia, Oceania, and some parts of East Asia. The negative Rmax-cum was mostly discovered at the medium and high latitudes of the Northern Hemisphere, such as eastern North America, Northern Asia, and some parts of Eastern Europe. As shown in Figure 3, the spatial distribution of accumulated months was significantly different at the global scale, while the accumulated months were mainly concentrated in the short term (31.81%), followed by medium term (16.32%) and long term (3.98%). The accumulated months in the short term were mostly found in North America, South America, Central Africa, Northern Asia, and Oceania. The medium-term accumulated months were mainly observed among Central Asia, Southern Africa, and some parts of East Asia. Moreover, the long term accumulation mainly occurred in East and South Asia.
3.2 Cumulative Drought Impact on Photosynthesis Across Plant Types
As shown in Table 1, the area percentages among Rmax-cum and accumulated months changed obviously in different plant cover types. The area percentages of the vegetation cover land revealed positive Rmax-cum following the order: CSHs (69.17%) > EBFs (58.28%) > GRAs (43.11%) > OSHs (42.54%) > SAVs (37.30%) > CROs (36.36%) > DBFs (28.80%) > WSAs (24.48%) > ENFs (21.85%) > MFs (8.89%) > DNFs (7.76%), as the negative Rmax-cum with the order: MFs (28.95%) > OSHs (19.77%) > ENFs (19.58%) > DNFs (18.97%) > DBFs (17.64%) > WSAs (17.48%) > SAVs (13.64) > CSHs (13.33%) > GRAs (11.26%) > CROs (7.89%) > EBFs (4.17%). In addition, the accumulated months were primarily at the short term in most vegetation types, while DBFs was at the medium term (50.11%).
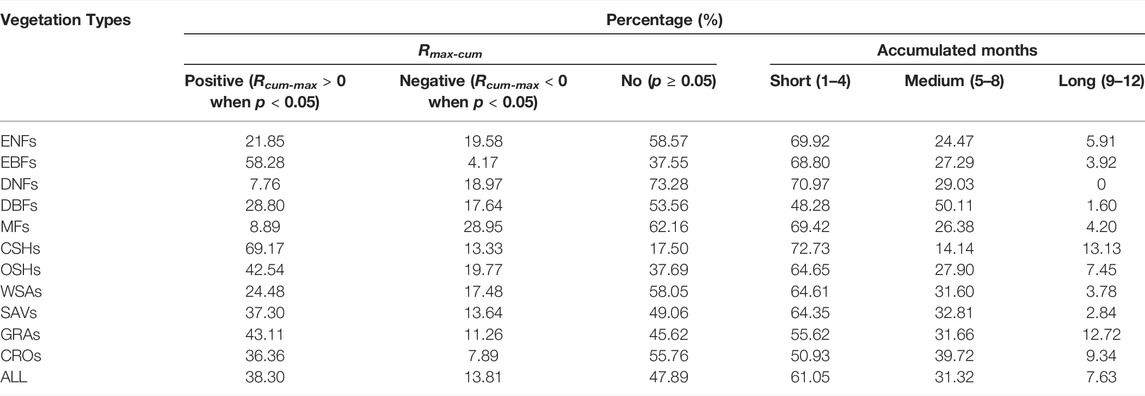
TABLE 1. Percentages of different Rmax-cum (i.e., positive, negative, and no significant correlation) and accumulated months (i.e., short, medium, and long term) across vegetation types.
3.3 Global Reliability Pattern of Drought Cumulative Effect on Photosynthesis
The global reliability pattern of Rmax-cum thought probability analysis is shown in Figure 4. The Rmax-cum had relatively high reliability at the global scale (characterized by the averaged value of 80.37 ± 17.33%). Moreover, the Rmax-cum showed high reliability in high latitudes and low reliability around the equatorial regions. The reliability of accumulated months displayed a similar global pattern with Rmax-cum (Figure 5), characterized by the mean value of 74.68 ± 20.79%. Notably, there were obvious discrepancies in the reliability of Rmax-cum and accumulated months around Oceania, South Asia, and South Africa, while the Rmax-cum presented high reliability and the accumulated months showed low reliability in these areas.
Figure 6 showed the variability trend of reliability in Rmax-cum and accumulated months across latitudes. The reliability of Rmax-cum was decreased with the decreasing of latitude, while the reliability of accumulated months was undulated in the Southern Hemisphere. As for the reliabilities of both Rmax-cum and accumulated months, they were various across vegetation types (Table 2). The averaged reliabilities of Rmax-cum in diverse plant types followed the order: DNFs (90.02 ± 14.44%) > ENFs (89.88 ± 14.53%) > MFs (86.22 ± 15.97%) > OSHs (85.41 ± 14.63%) > WSAs (84.17 ± 17.17%) > DBFs (84.01 ± 15.68%) > SAVs (83.52 ± 16.57%) > GRAs (79.41 ± 16.60%) > CROs (77.27 ± 16.38%) > CSHs (74.58 ± 17.15%) > EBFs (62.74 ± 14.87%), while the averaged reliability of accumulated months showed a lower value than Rmax-cum among different species, characterized by the following order: DNFs (89.36 ± 15.24%) > ENFs (88.26 ± 16.12%) > MFs (85.28 ± 16.67%) > WSAs (81.88 ± 18.98%) > DBFs (81.39 ± 17.14%) > SAVs (79.34 ± 19.23%) > GRAs (73.93 ± 19.38%) > OSHs (72.74 ± 24.11%) > CROs (72.26 ± 18.68%) > EBFs (56.83 ± 15.81%) > CSHs (55.71 ± 16.61%).
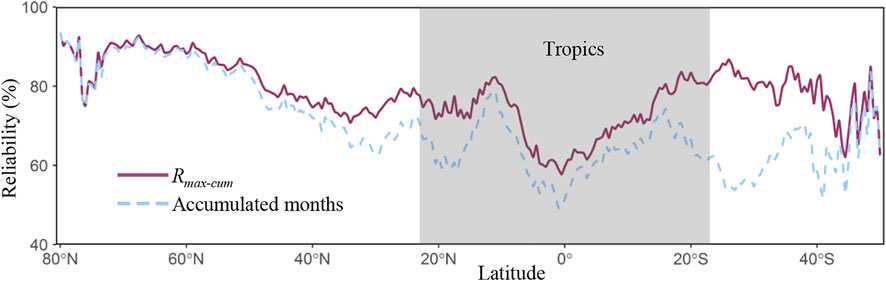
FIGURE 6. Variability trend of reliability in Rmax-cum and accumulated months acquired from probability statistics across latitudes.

TABLE 2. Averaged reliability and standard deviation of Rmax-cum and accumulated months across vegetation types.
4 Discussion
This study evaluated the cumulative drought impact on global photosynthesis across vegetation types grounded on numerous GPP products and derived a global reliability pattern of drought cumulative effect through probability analysis. The results illustrated that the cumulative effects of drought occurred in 52.11% of the vegetation cover areas (Figure 2) and the dominant accumulated months were short term (1–4 months, 31.81%). Our study indicated the high spatial heterogeneity of drought effect at the global scale and the importance of the GPP dataset in evaluating the cumulative drought impact on photosynthesis.
In this study, the global reliability pattern of drought cumulative effect on photosynthesis was acquired through numerous GPP products, and both Rmax-cum and accumulated months exhibited spatially heterogenous distributions (Figure 4 and Figure 5). Moreover, the reliability of cumulative effect in time scales was more undulated, especially in the southern hemisphere (Figure 6). These findings revealed that the water supply and availability could play a key role in GPP simulation. Generally, a total of twenty-six global GPP datasets generated from different model schemes (i.e., based on LUE, ML, and process) were used as indicators to represent the photosynthesis of vegetation. Among various models, these process-oriented models always considered many physiological or biogeochemical processes of photosynthesis mechanistically (Jiang and Ryu, 2016; Wagle et al., 2016; Xie et al., 2021), thus making the GPP estimation more rigorous. While these LUE models were developed through the theory of radiation conversion efficiency (Monteith, 1972; Yuan et al., 2014), the ML models estimated GPP based on the statistical relationship established between input data and outcome (Jiang and Ryu, 2016; Wolanin et al., 2019; Xie et al., 2022). In this way, different theoretical foundations among diverse models would bring unavoidable uncertainties in characterizing global photosynthesis (Zheng et al., 2020; Wang S. et al., 2021; Wang Z. et al., 2021). In addition, multiple GPP models have used various strategies to describe the water stress of vegetation. For example, the EC-LUE model used the rate between evapotranspiration and net radiation to consider the moisture regulations on LUE (Yuan et al., 2010), while the VPM model adopted a scaled Land Surface Water Index to characterize the moisture limitation for photosynthesis (Zhang et al., 2017). Moreover, the water limiting processes could be more complex in process-oriented models (Jiang and Ryu, 2016; Slevin et al., 2017). These different ways of regulating the moisture status of plants might also lead to a diverse response of photosynthesis to drought among different GPP datasets.
The cumulative drought impact on photosynthesis and its reliability showed obvious discrepancies among vegetation types (Table 1 and Table 2). The results showed that there were higher proportions of significant Rmax-cum pixels in CSHs, OSHs, and GRAs than those in DNFs, MFs, ENFs, and WSAs, confirming the importance of water supply and availability for grasslands and shrubs (Wu et al., 2015; Peng et al., 2019; Fan et al., 2020), while this might be attributed to the different structural and functional characteristics of rhizomes between herbs and woody plants (Chimento and Amaducci, 2015; Hudek et al., 2017; Lobmann et al., 2020), such as root diameter (Hudek et al., 2017), root depth (Seghieri, 1995), and stem specific gravity (McCoy-Sulentic et al., 2017). Herbaceous vegetations usually have abundant fine and shallow roots in the topsoil layer, making them respond more sensitively to drought due to the limitation in water availability through more deep layers of soil (Dodd et al., 1998; Wu et al., 2018; Lobmann et al., 2020). However, woody plants always have taproot structures with thick roots, and deeper roots facilitate the absorption of groundwater which causes them to be more resistant to drought (Dodd et al., 1998; Midwood et al., 1998; Bleby et al., 2010; Wang et al., 2020). Moreover, the roots of woody plants are further in evolution than herbaceous plants and are characterized by secondary growth (Ma et al., 2018; Wang et al., 2020), helping them withstand stress caused by drought. Different water storage modes may also result in diverse responses to drought across vegetations (Tian et al., 2018) because woody vegetations hold most plant water in their woody tissues and have more complex hydraulic strategies, while the moisture storage of herbs primarily depends on the amount of foliage (Sternberg and Shoshany, 2001; Morris et al., 2016).
5 Conclusion
In this work, a total of twenty-six global GPP products based on process, LUE, and ML models were obtained to evaluate and derive a global reliability pattern of drought’s cumulative effect on photosynthesis. The results illustrated that the cumulative drought impact was observed in 52.11% of vegetation cover land primarily in the short term (31.81%). Obvious discrepancies of drought cumulative effect were observed in different plant functional types, while herbaceous vegetations were more sensitive to drought than woody plants. This could be attributed to the difference in root functional traits among vegetation functional types. Because the reliability pattern of drought depended on multiple GPP datasets, the results also showed marked heterogeneity in terms of space and vegetation type, while lower reliability was found in the tropics. Our findings highlighted the importance of water characterization strategies under different vegetation functional types in GPP models and the necessity of GPP dataset selection in assessing drought effect on vegetation.
Data Availability Statement
The raw data supporting the conclusions of this article will be made available by the authors, without undue reservation.
Author Contributions
CW: conceptualization, software, investigation, writing—original draft preparation, and writing—review and editing. TW: supervision, data curation, and funding acquisition.
Conflict of Interest
The authors declare that the research was conducted in the absence of any commercial or financial relationships that could be construed as a potential conflict of interest.
Publisher’s Note
All claims expressed in this article are solely those of the authors and do not necessarily represent those of their affiliated organizations, or those of the publisher, the editors, and the reviewers. Any product that may be evaluated in this article, or claim that may be made by its manufacturer, is not guaranteed or endorsed by the publisher.
Acknowledgments
All global GPP datasets used in this work are shown in Supplementary Material, and we sincerely thank all data providers. The fifteen process-oriented GPP products (BIOME-BGC, CLASS-CTEM-N+, CLM4, CLM4VIC, DLEM, GTEC, ISAM, LPJ-wsl, ORCHIDEE-LSCE, SiB3, SiBCASA, TEM6, TRIPLEX-GHG, VEGAS2.1, and VISIT) were acquired through “The North American Carbon Program (NACP) Multiscale Synthesis and Terrestrial Model Intercomparison Project (MsTMIP). (https://doi.org/10.3334/ORNLDAAC/1225).” The JULES GPP dataset was downloaded at “The University of Edinburgh. School of GeoSciences. (https://doi.org/10.7488/ds/1461),” while BESS data could be obtained at “Ecological Sensing AI Lab. Seoul National University. (https://www.environment.snu.ac.kr/).” EC-LUE data supported from “National Earth System Science Data Center. National Science & Technology Infrastructure of China. (http://www.geodata.cn),” and VPM data could be found at “(https://doi.org/10.6084/m9.figshare.c.3789814.v1)”. Moreover, multiscale SPEI data could be accessed at “(https://spei.csic.es/database.html).” We would also like to thank the anonymous reviewers for their constructive comments.
Supplementary Material
The Supplementary Material for this article can be found online at: https://www.frontiersin.org/articles/10.3389/fenvs.2022.908875/full#supplementary-material
References
AghaKouchak, A., Cheng, L., Mazdiyasni, O., and Farahmand, A. (2014). Global Warming and Changes in Risk of Concurrent Climate Extremes: Insights from the 2014 California Drought. Geophys. Res. Lett. 41 (24), 8847–8852. doi:10.1002/2014gl062308
Anav, A., Friedlingstein, P., Beer, C., Ciais, P., Harper, A., Jones, C., et al. (2015). Spatiotemporal Patterns of Terrestrial Gross Primary Production: A Review. Rev. Geophys. 53 (3), 785–818. doi:10.1002/2015rg000483
Anderegg, W. R. L., Schwalm, C., Biondi, F., Camarero, J. J., Koch, G., Litvak, M., et al. (2015). Pervasive Drought Legacies in forest Ecosystems and Their Implications for Carbon Cycle Models. Science 349 (6247), 528–532. doi:10.1126/science.aab1833
Barman, R., Jain, A. K., and Liang, M. (2014). Climate-driven Uncertainties in Modeling Terrestrial Gross Primary Production: a Site Level to Global-Scale Analysis. Glob. Change Biol. 20 (5), 1394–1411. doi:10.1111/gcb.12474
Beguería, S., Vicente-Serrano, S. M., and Angulo-Martínez, M. (2010). A Multiscalar Global Drought Dataset: The SPEIbase: A New Gridded Product for the Analysis of Drought Variability and Impacts. Bull. Amer. Meteorol. Soc. 91 (10), 1351–1356. doi:10.1175/2010bams2988.1
Beguería, S., Vicente-Serrano, S. M., Reig, F., and Latorre, B. (2014). Standardized Precipitation Evapotranspiration index (SPEI) Revisited: Parameter Fitting, Evapotranspiration Models, Tools, Datasets and Drought Monitoring. Int. J. Climatol. 34 (10), 3001–3023. doi:10.1002/joc.3887
Bleby, T. M., McElrone, A. J., and Jackson, R. B. (2010). Water Uptake and Hydraulic Redistribution across Large Woody Root Systems to 20 M Depth. Plant Cel Environ. 33 (12), 2132–2148. doi:10.1111/j.1365-3040.2010.02212.x
Braswell, B. H., Schimel, D. S., Linder, E., and Moore, B. (1997). The Response of Global Terrestrial Ecosystems to Interannual Temperature Variability. Science 278 (5339), 870–873. doi:10.1126/science.278.5339.870
Campbell, J. E., Berry, J. A., Seibt, U., Smith, S. J., Montzka, S. A., Launois, T., et al. (2017). Large Historical Growth in Global Terrestrial Gross Primary Production. Nature 544 (7648), 84–87. doi:10.1038/nature22030
Chen, B., Xu, G., Coops, N. C., Ciais, P., Innes, J. L., Wang, G., et al. (2014). Changes in Vegetation Photosynthetic Activity Trends across the Asia-Pacific Region over the Last Three Decades. Remote Sensing Environ. 144, 28–41. doi:10.1016/j.rse.2013.12.018
Chimento, C., and Amaducci, S. (2015). Characterization of fine Root System and Potential Contribution to Soil Organic Carbon of Six Perennial Bioenergy Crops. Biomass and Bioenergy 83, 116–122. doi:10.1016/j.biombioe.2015.09.008
Chu, D., Shen, H., Guan, X., Chen, J. M., Li, X., Li, J., et al. (2021). Long Time-Series NDVI Reconstruction in Cloud-Prone Regions via Spatio-Temporal Tensor Completion. Remote Sensing Environ. 264, 112632. doi:10.1016/j.rse.2021.112632
Ciais, P., Reichstein, M., Viovy, N., Granier, A., Ogée, J., Allard, V., et al. (2005). Europe-wide Reduction in Primary Productivity Caused by the Heat and Drought in 2003. Nature 437 (7058), 529–533. doi:10.1038/nature03972
Cramer, W., Bondeau, A., Woodward, F. I., Prentice, I. C., Betts, R. A., Brovkin, V., et al. (2001). Global Response of Terrestrial Ecosystem Structure and Function to CO2 and Climate Change: Results from Six Dynamic Global Vegetation Models. Glob. Change Biol. 7 (4), 357–373. doi:10.1046/j.1365-2486.2001.00383.x
Curasi, S. R., Parker, T. C., Rocha, A. V., Moody, M. L., Tang, J., and Fetcher, N. (2019). Differential Responses of Ecotypes to Climate in a Ubiquitous Arctic Sedge: Implications for Future Ecosystem C Cycling. New Phytol. 223 (1), 180–192. doi:10.1111/nph.15790
Dodd, M. B., Lauenroth, W. K., and Welker, J. M. (1998). Differential Water Resource Use by Herbaceous and Woody Plant Life-Forms in a Shortgrass Steppe Community. Oecologia 117 (4), 504–512. doi:10.1007/s004420050686
Fan, J., Xu, Y., Ge, H., and Yang, W. (2020). Vegetation Growth Variation in Relation to Topography in Horqin Sandy Land. Ecol. Indicators 113, 106215. doi:10.1016/j.ecolind.2020.106215
Friedl, M., and Sulla-Menashe, D. (2015). MCD12C1 MODIS/Terra+Aqua Land Cover Type Yearly L3 Global 0.05Deg CMG V006. NASA EOSDIS Land Process. DAAC. Accessed May 04, 2022. doi:10.5067/MODIS/MCD12C1.006
Guan, X., Chen, J. M., Shen, H., and Xie, X. (2021). A Modified Two-Leaf Light Use Efficiency Model for Improving the Simulation of GPP Using a Radiation Scalar. Agric. For. Meteorology 307, 108546. doi:10.1016/j.agrformet.2021.108546
Guan, X., Chen, J. M., Shen, H., Xie, X., and Tan, J. (2022). Comparison of Big-Leaf and Two-Leaf Light Use Efficiency Models for GPP Simulation after Considering a Radiation Scalar. Agric. For. Meteorology 313, 108761. doi:10.1016/j.agrformet.2021.108761
Guo, Y., Xia, H., Pan, L., Zhao, X., and Li, R. (2022). Mapping the Northern Limit of Double Cropping Using a Phenology-Based Algorithm and Google Earth Engine. Remote Sensing 14 (4), 1004. doi:10.3390/rs14041004
Hayes, D. J., McGuire, A. D., Kicklighter, D. W., Gurney, K. R., Burnside, T. J., and Melillo, J. M. (2011). Is the Northern High-Latitude Land-Based CO2sink Weakening? Glob. Biogeochem. Cycles 25, 1. doi:10.1029/2010gb003813
Hilker, T., Coops, N. C., Wulder, M. A., Black, T. A., and Guy, R. D. (2008). The Use of Remote Sensing in Light Use Efficiency Based Models of Gross Primary Production: A Review of Current Status and Future Requirements. Sci. Total Environ. 404 (2-3), 411–423. doi:10.1016/j.scitotenv.2007.11.007
Huang, C.-y., Asner, G. P., Barger, N. N., Neff, J. C., and Floyd, M. L. (2010). Regional Aboveground Live Carbon Losses Due to Drought-Induced Tree Dieback in Piñon-Juniper Ecosystems. Remote Sensing Environ. 114 (7), 1471–1479. doi:10.1016/j.rse.2010.02.003
Huang, L., He, B., Han, L., Liu, J., Wang, H., and Chen, Z. (2017). A Global Examination of the Response of Ecosystem Water-Use Efficiency to Drought Based on MODIS Data. Sci. Total Environ. 601-602, 1097–1107. doi:10.1016/j.scitotenv.2017.05.084
Huang, M., Wang, X., Keenan, T. F., and Piao, S. (2018). Drought Timing Influences the Legacy of Tree Growth Recovery. Glob. Change Biol. 24 (8), 3546–3559. doi:10.1111/gcb.14294
Hudek, C., Sturrock, C. J., Atkinson, B. S., Stanchi, S., and Freppaz, M. (2017). Root Morphology and Biomechanical Characteristics of High Altitude alpine Plant Species and Their Potential Application in Soil Stabilization. Ecol. Eng. 109, 228–239 . doi:10.1016/j.ecoleng.2017.05.048
Huntzinger, D. N., Schwalm, C. R., Wei, Y., Cook, R. B., Michalak, A. M., Schaefer, K., et al. (2018). NACP MsTMIP: Global 0.5-degree Model Outputs in Standard Format, Version 1.0. Oak Ridge, TN: ORNL Distributed Active Archive Center.
Ito, A. (2010). Changing Ecophysiological Processes and Carbon Budget in East Asian Ecosystems under Near-Future Changes in Climate: Implications for Long-Term Monitoring from a Process-Based Model. J. Plant Res. 123 (4), 577–588. doi:10.1007/s10265-009-0305-x
Ivits, E., Horion, S., Erhard, M., and Fensholt, R. (2016). Assessing European Ecosystem Stability to Drought in the Vegetation Growing Season. Glob. Ecol. Biogeogr. 25 (9), 1131–1143. doi:10.1111/geb.12472
Jiang, C., and Ryu, Y. (2016). Multi-scale Evaluation of Global Gross Primary Productivity and Evapotranspiration Products Derived from Breathing Earth System Simulator (BESS). Remote Sensing Environ. 186, 528–547. doi:10.1016/j.rse.2016.08.030
Kang, W., Wang, T., and Liu, S. (2018). The Response of Vegetation Phenology and Productivity to Drought in Semi-arid Regions of Northern China. Remote Sensing 10 (5), 727. doi:10.3390/rs10050727
Lees, K. J., Quaife, T., Artz, R. R. E., Khomik, M., and Clark, J. M. (2018). Potential for Using Remote Sensing to Estimate Carbon Fluxes across Northern Peatlands - A Review. Sci. Total Environ. 615, 857–874. doi:10.1016/j.scitotenv.2017.09.103
Löbmann, M. T., Geitner, C., Wellstein, C., and Zerbe, S. (2020). The Influence of Herbaceous Vegetation on Slope Stability - A Review. Earth-Science Rev. 209, 103328. doi:10.1016/j.earscirev.2020.103328
Ma, Z., Guo, D., Xu, X., Lu, M., Bardgett, R. D., Eissenstat, D. M., et al. (2018). Evolutionary History Resolves Global Organization of Root Functional Traits. Nature 555 (7694), 94–97. doi:10.1038/nature25783
McCoy‐Sulentic, M. E., Kolb, T. E., Merritt, D. M., Palmquist, E. C., Ralston, B. E., and Sarr, D. A. (2017). Variation in Species‐level Plant Functional Traits over Wetland Indicator Status Categories. Ecol. Evol. 7 (11), 3732–3744. doi:10.1002/ece3.2975
Midwood, A. J., Boutton, T. W., Archer, S. R., and Watts, S. E. (1998). Water Use by Woody Plants on Contrasting Soils in a savanna Parkland: Assessment with delta H-2 and delta O-18. Plant and Soil 205 (1), 13–24. doi:10.1023/a:1004355423241
Monteith, J. L. (1972). Solar Radiation and Productivity in Tropical Ecosystems. J. Appl. Ecol. 9 (3), 747–766. doi:10.2307/2401901
Morris, H., Plavcová, L., Cvecko, P., Fichtler, E., Gillingham, M. A. F., Martínez‐Cabrera, H. I., et al. (2016). A Global Analysis of Parenchyma Tissue Fractions in Secondary Xylem of Seed Plants. New Phytol. 209 (4), 1553–1565. doi:10.1111/nph.13737
Naumann, G., Alfieri, L., Wyser, K., Mentaschi, L., Betts, R. A., Carrao, H., et al. (2018). Global Changes in Drought Conditions under Different Levels of Warming. Geophys. Res. Lett. 45 (7), 3285–3296. doi:10.1002/2017gl076521
Pan, L., Xia, H., Zhao, X., Guo, Y., and Qin, Y. (2021). Mapping Winter Crops Using a Phenology Algorithm, Time-Series Sentinel-2 and Landsat-7/8 Images, and Google Earth Engine. Remote Sensing 13 (13), 2510. doi:10.3390/rs13132510
Passioura, J. B. (1991). “The Yield of Crops in Relation to Drought,” in International Symposium on Physiology and Determination of Crop Yield. Editors K. J. Boote, J. M. Bennett, T. R. Sinclair, and G. M. Paulsen (MADISON: Soil Science Soc Amer), 343–359.
Peng, J., Wu, C., Zhang, X., Wang, X., and Gonsamo, A. (2019). Satellite Detection of Cumulative and Lagged Effects of Drought on Autumn Leaf Senescence over the Northern Hemisphere. Glob. Change Biol. 25 (6), 2174–2188. doi:10.1111/gcb.14627
Rao, K., Anderegg, W. R. L., Sala, A., Martínez-Vilalta, J., and Konings, A. G. (2019). Satellite-based Vegetation Optical Depth as an Indicator of Drought-Driven Tree Mortality. Remote Sensing Environ. 227, 125–136. doi:10.1016/j.rse.2019.03.026
Reichstein, M., Bahn, M., Ciais, P., Frank, D., Mahecha, M. D., Seneviratne, S. I., et al. (2013). Climate Extremes and the Carbon Cycle. Nature 500 (7462), 287–295. doi:10.1038/nature12350
Richardson, A. D., Keenan, T. F., Migliavacca, M., Ryu, Y., Sonnentag, O., and Toomey, M. (2013). Climate Change, Phenology, and Phenological Control of Vegetation Feedbacks to the Climate System. Agric. For. Meteorology 169, 156–173. doi:10.1016/j.agrformet.2012.09.012
Sánchez, M. L., Pardo, N., Pérez, I. A., and García, M. A. (2015). GPP and Maximum Light Use Efficiency Estimates Using Different Approaches over a Rotating Biodiesel Crop. Agric. For. Meteorology 214-215, 444–455. doi:10.1016/j.agrformet.2015.09.012
Seghieri, J. (1995). The Rooting Patterns of Woody and Herbaceous Plants in a savanna; Are They Complementary or in Competition? Afr. J Ecol 33 (4), 358–365. doi:10.1111/j.1365-2028.1995.tb01045.x
Slevin, D., Tett, S. F. B., Exbrayat, J.-F., Bloom, A. A., and Williams, M. (2017). Global Evaluation of Gross Primary Productivity in the JULES Land Surface Model v3.4.1. Geosci. Model. Dev. 10 (7), 2651–2670. doi:10.5194/gmd-10-2651-2017
Sternberg, M., and Shoshany, M. (2001). Aboveground Biomass Allocation and Water Content Relationships in Mediterranean Trees and Shrubs in Two Climatological Regions in Israel. Plant Ecol. 157 (2), 173–181. doi:10.1023/a:1013916422201
Sun, S., Du, W., Song, Z., Zhang, D., Wu, X., Chen, B., et al. (2021). Response of Gross Primary Productivity to Drought Time‐Scales across China. J. Geophys. Res. Biogeosci 126 (4), 19. doi:10.1029/2020jg005953
Tan, K. P., Kanniah, K. D., and Cracknell, A. P. (2012). A Review of Remote Sensing Based Productivity Models and Their Suitability for Studying Oil palm Productivity in Tropical Regions. Prog. Phys. Geogr. Earth Environ. 36 (5), 655–679. doi:10.1177/0309133312452187
Tian, F., Wigneron, J.-P., Ciais, P., Chave, J., Ogée, J., Peñuelas, J., et al. (2018). Coupling of Ecosystem-Scale Plant Water Storage and Leaf Phenology Observed by Satellite. Nat. Ecol. Evol. 2 (9), 1428–1435. doi:10.1038/s41559-018-0630-3
Tian, H., Xu, X., Lu, C., Liu, M., Ren, W., Chen, G., et al. (2011). Net Exchanges of CO2, CH4, and N2O between China's Terrestrial Ecosystems and the Atmosphere and Their Contributions to Global Climate Warming. J. Geophys. Res. 116, 13. doi:10.1029/2010jg001393
Tramontana, G., Jung, M., Schwalm, C. R., Ichii, K., Camps-Valls, G., Ráduly, B., et al. (2016). Predicting Carbon Dioxide and Energy Fluxes across Global FLUXNET Sites with Regression Algorithms. Biogeosciences 13 (14), 4291–4313. doi:10.5194/bg-13-4291-2016
van der Molen, M. K., Dolman, A. J., Ciais, P., Eglin, T., Gobron, N., Law, B. E., et al. (2011). Drought and Ecosystem Carbon Cycling. Agric. For. Meteorology 151 (7), 765–773. doi:10.1016/j.agrformet.2011.01.018
Vicente-Serrano, S. M., Beguería, S., and López-Moreno, J. I. (2010). A Multiscalar Drought Index Sensitive to Global Warming: The Standardized Precipitation Evapotranspiration Index. J. Clim. 23 (7), 1696–1718. doi:10.1175/2009jcli2909.1
Wagle, P., Zhang, Y., Jin, C., and Xiao, X. (2016). Comparison of Solar‐induced Chlorophyll Fluorescence, Light‐use Efficiency, and Process‐based GPP Models in maize. Ecol. Appl. 26 (4), 1211–1222. doi:10.1890/15-1434
Wang, P., Huang, K., and Hu, S. (2020). Distinct fine‐root Responses to Precipitation Changes in Herbaceous and Woody Plants: a Meta‐analysis. New Phytol. 225 (4), 1491–1499. doi:10.1111/nph.16266
Wang, S., Zhang, Y., Ju, W., Qiu, B., and Zhang, Z. (2021a). Tracking the Seasonal and Inter-annual Variations of Global Gross Primary Production during Last Four Decades Using Satellite Near-Infrared Reflectance Data. Sci. Total Environ. 755, 142569. doi:10.1016/j.scitotenv.2020.142569
Wang, Z., Liu, S., Wang, Y.-P., Valbuena, R., Wu, Y., Kutia, M., et al. (2021b). Tighten the Bolts and Nuts on GPP Estimations from Sites to the Globe: An Assessment of Remote Sensing Based LUE Models and Supporting Data Fields. Remote Sensing 13 (2), 168. doi:10.3390/rs13020168
Wen, Y., Liu, X., Xin, Q., Wu, J., Xu, X., Pei, F., et al. (2019). Cumulative Effects of Climatic Factors on Terrestrial Vegetation Growth. J. Geophys. Res. Biogeosci. 124 (4), 789–806. doi:10.1029/2018jg004751
Wendler, G., Conner, J., Moore, B., Shulski, M., and Stuefer, M. (2011). Climatology of Alaskan Wildfires with Special Emphasis on the Extreme Year of 2004. Theor. Appl. Climatol 104 (3-4), 459–472. doi:10.1007/s00704-010-0357-9
Wolanin, A., Camps-Valls, G., Gómez-Chova, L., Mateo-García, G., van der Tol, C., Zhang, Y., et al. (2019). Estimating Crop Primary Productivity with Sentinel-2 and Landsat 8 Using Machine Learning Methods Trained with Radiative Transfer Simulations. Remote Sensing Environ. 225, 441–457. doi:10.1016/j.rse.2019.03.002
Wu, D., Zhao, X., Liang, S., Zhou, T., Huang, K., Tang, B., et al. (2015). Time-lag Effects of Global Vegetation Responses to Climate Change. Glob. Change Biol. 21 (9), 3520–3531. doi:10.1111/gcb.12945
Wu, X., Liu, H., Li, X., Ciais, P., Babst, F., Guo, W., et al. (2018). Differentiating Drought Legacy Effects on Vegetation Growth over the Temperate Northern Hemisphere. Glob. Change Biol. 24 (1), 504–516. doi:10.1111/gcb.13920
Xiao, J., Chevallier, F., Gomez, C., Guanter, L., Hicke, J. A., Huete, A. R., et al. (2019). Remote Sensing of the Terrestrial Carbon Cycle: A Review of Advances over 50 Years. Remote Sensing Environ. 233, 111383. doi:10.1016/j.rse.2019.111383
Xiao, J., Davis, K. J., Urban, N. M., Keller, K., and Saliendra, N. Z. (2011). Upscaling Carbon Fluxes from Towers to the Regional Scale: Influence of Parameter Variability and Land Cover Representation on Regional Flux Estimates. J. Geophys. Res. 116, 15. doi:10.1029/2010jg001568
Xiao, X., Zhang, Q. Y., Braswell, B., Urbanski, S., Boles, S., Wofsy, S., et al. (2004). Modeling Gross Primary Production of Temperate Deciduous Broadleaf forest Using Satellite Images and Climate Data. Remote Sensing Environ. 91 (2), 256–270. doi:10.1016/j.rse.2004.03.010
Xie, X., Chen, J. M., Gong, P., and Li, A. (2021). Spatial Scaling of Gross Primary Productivity over Sixteen Mountainous Watersheds Using Vegetation Heterogeneity and Surface Topography. J. Geophys. Res. Biogeosci 126 (5), 21. doi:10.1029/2020jg005848
Xie, X., and Li, A. (2020a). An Adjusted Two‐Leaf Light Use Efficiency Model for Improving GPP Simulations over Mountainous Areas. J. Geophys. Res. Atmos. 125 (13), 19. doi:10.1029/2019jd031702
Xie, X., and Li, A. (2020b). Development of a Topographic-Corrected Temperature and Greenness Model (TG) for Improving GPP Estimation over Mountainous Areas. Agric. For. Meteorology 295, 108193. doi:10.1016/j.agrformet.2020.108193
Xie, X., Tian, J., Wu, C., Li, A., Jin, H., Bian, J., et al. (2022). Long-term Topographic Effect on Remotely Sensed Vegetation index-based Gross Primary Productivity (GPP) Estimation at the Watershed Scale. Int. J. Appl. Earth Observation Geoinformation 108, 102755. doi:10.1016/j.jag.2022.102755
Yuan, M., Zhao, L., Lin, A., Wang, L., Li, Q., She, D., et al. (2020). Impacts of Preseason Drought on Vegetation spring Phenology across the Northeast China Transect. Sci. Total Environ. 738, 140297. doi:10.1016/j.scitotenv.2020.140297
Yuan, W., Cai, W., Xia, J., Chen, J., Liu, S., Dong, W., et al. (2014). Global Comparison of Light Use Efficiency Models for Simulating Terrestrial Vegetation Gross Primary Production Based on the LaThuile Database. Agric. For. Meteorology 192-193, 108–120. doi:10.1016/j.agrformet.2014.03.007
Yuan, W., Liu, S., Yu, G., Bonnefond, J.-M., Chen, J., Davis, K., et al. (2010). Global Estimates of Evapotranspiration and Gross Primary Production Based on MODIS and Global Meteorology Data. Remote Sensing Environ. 114 (7), 1416–1431. doi:10.1016/j.rse.2010.01.022
Zhang, Y., Xiao, X., Wu, X., Zhou, S., Zhang, G., Qin, Y., et al. (2017). A Global Moderate Resolution Dataset of Gross Primary Production of Vegetation for 2000-2016. Sci. Data 4, 13. doi:10.1038/sdata.2017.165
Zhao, A., Yu, Q., Feng, L., Zhang, A., and Pei, T. (2020). Evaluating the Cumulative and Time-Lag Effects of Drought on Grassland Vegetation: A Case Study in the Chinese Loess Plateau. J. Environ. Manage. 261, 110214. doi:10.1016/j.jenvman.2020.110214
Zhao, A., Zhang, A., Cao, S., Liu, X., Liu, J., and Cheng, D. (2018). Responses of Vegetation Productivity to Multi-Scale Drought in Loess Plateau, China. Catena 163, 165–171. doi:10.1016/j.catena.2017.12.016
Zhao, M., Running, S. W., and Nemani, R. R. (2006). Sensitivity of Moderate Resolution Imaging Spectroradiometer (MODIS) Terrestrial Primary Production to the Accuracy of Meteorological Reanalyses. J. Geophys. Res. 111 (G1), 13. doi:10.1029/2004jg000004
Zheng, Y., Shen, R., Wang, Y., Li, X., Liu, S., Liang, S., et al. (2020). Improved Estimate of Global Gross Primary Production for Reproducing its Long-Term Variation, 1982-2017. Earth Syst. Sci. Data 12 (4), 2725–2746. doi:10.5194/essd-12-2725-2020
Zheng, Y., Zhang, L., Xiao, J., Yuan, W., Yan, M., Li, T., et al. (2018). Sources of Uncertainty in Gross Primary Productivity Simulated by Light Use Efficiency Models: Model Structure, Parameters, Input Data, and Spatial Resolution. Agric. For. Meteorology 263, 242–257. doi:10.1016/j.agrformet.2018.08.003
Zhou, Y., Wu, X., Ju, W., Chen, J. M., Wang, S., Wang, H., et al. (2016). Global Parameterization and Validation of a Two‐leaf Light Use Efficiency Model for Predicting Gross Primary Production across FLUXNET Sites. J. Geophys. Res. Biogeosci. 121 (4), 1045–1072. doi:10.1002/2014jg002876
Keywords: cumulative effect, drought, GPP dataset, photosynthesis, SPEI, reliability
Citation: Wu C and Wang T (2022) Evaluating Cumulative Drought Effect on Global Vegetation Photosynthesis Using Numerous GPP Products. Front. Environ. Sci. 10:908875. doi: 10.3389/fenvs.2022.908875
Received: 31 March 2022; Accepted: 12 April 2022;
Published: 12 May 2022.
Edited by:
Honglei Wang, Nanjing University of Information Science and Technology, ChinaReviewed by:
Shenxin Li, Central South University, ChinaLchihiro An, Shandong Agricultural University, China
Copyright © 2022 Wu and Wang. This is an open-access article distributed under the terms of the Creative Commons Attribution License (CC BY). The use, distribution or reproduction in other forums is permitted, provided the original author(s) and the copyright owner(s) are credited and that the original publication in this journal is cited, in accordance with accepted academic practice. No use, distribution or reproduction is permitted which does not comply with these terms.
*Correspondence: Tengjun Wang, d2FuZ3RqQGNoZC5lZHUuY24=