- 1School of Business and Management, Jilin University, Changchun, China
- 2School of Statistics, Jilin University of Finance and Economics, Changchun, China
Amid rising industrialization and economic progress, China has shown exponential growth in energy and fossil fuel consumption; therefore, it faces great global concern and widespread criticism for energy and fuel conservation to reduce fuel-related emissions. In addition, the recent spread of COVID-19 instigates the impact of environmental pollution, exaggerates the virus intensity, and lowers people’s immunity due to poor air quality. Therefore, this study explored the role of green energy efficiency and climate technologies in achieving carbon neutrality in China using an advanced quantile autoregressive distributed lag (QARDL) framework. The results indicated that green energy efficiency and climate technologies significantly reduce environmental pollution across all quantiles in the long run. In contrast, urbanization enhances environmental degradation at lower and higher emissions quantiles, while trade only promotes environmental pollution at lower quantiles. These findings suggested using alternative energy sources and carbon-reducing technologies to ensure a sustainable environment.
Introduction
Energy is vital for economic stability, and its demand has continuously grown worldwide (Asiedu et al., 2021). However, its ecological implication has started from its exploration and exploitation and finally from its consumption (Osiyevskyy et al., 2020). Moreover, the rapid growth of industrialization caused the excessive use of energy and increased the divestment of fossil fuels (Pata, 2018; Sun et al., 2022a). The world’s major industrial countries are dependent quite heavily on inevitably depleted fossil fuels. Getting control of the climate changes invariably, governments are now seeking ways to reduce the consumption of carbon-laden fuels (Ahmad et al., 2022; Atchike et al., 2022). For instance, these issues can be valuably responded to by reducing energy consumption, developing green energy sources, and improving energy efficiency (Razzaq et al., 2021a; Irfan et al., 2021; Sun et al., 2022b; Elavarasan et al., 2022).
Since 1978 after the reforms and opening up toward the sustainable path, China’s economic growth has mainly depended on the high consumption of nonrenewable energy resources (Lu et al., 2019). The ongoing accelerated pace in China’s total energy consumption accounts for almost 23.6% of the complete energy depletion of the world in 2018 (K. Dong F. et al., 2018; Lei et al., 2022) and contributed more than 34% of the net increase of global energy utilization. Moreover, the energy consumption growth rate reached 4.3% in China, which is relatively higher than the global average growth in energy consumption in 2018 (Liu et al., 2021a). Global energy growth is outpacing decarburization despite the positive progress of many countries whose economies have grown over the last decade, and their emissions have declined due to the replacement of energy-dense nonrenewable energy with green energy sources and the improvement in energy efficiency (reliance on eco-innovation) (Brown, 2021; Song et al., 2021; Fang et al., 2022; Sun and Razzaq, 2022). China is the largest energy consumer, and greenhouse gas emitter faces great global concern and widespread criticism for energy conservation and GHG emanation decline (Song et al., 2021; Yu et al., 2019; Sun et al., 2022b).
Green energy efficiency is necessary to meet the rapidly growing energy demand and the targets against climate change with sustainable development (Wu et al., 2021). In this regard, as per the Paris Agreement, China has set a target of 60–65% reduction in its emission intensity (the emission intensity is measured as the amount of GHGs released against the economic activity of per dollar) over 2005 levels in 2030 (P. Li and Ouyang, 2020; Miao et al., 2021). According to Figure 1, the share of renewable energy to the total energy is 17.11%, whereas the total CO2 emission reached 9,876.5 MT in 2018. Moreover, according to the China Electric Power Yearbook for 2020, the current thermal and hydropower energy generation data state that hydropower contributes up to 68.9%; in contrast, thermal power is accounted for 17.8% of total power generation in China.
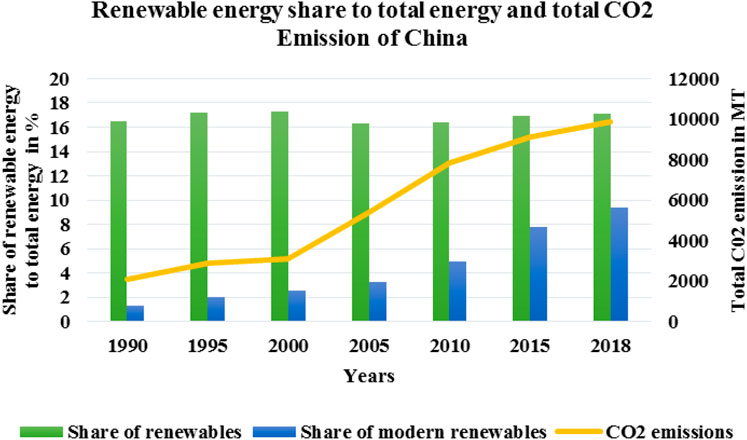
FIGURE 1. Renewable energy share to total energy and total CO2 emission of China. Source: IEA Sustainable Development Goal 7 https://www.iea.org/reports/tracking-sdg7-the-energy-progress-report-2021.
Energy efficiency refers to less consumption of energy resources while ensuring the same production level. It is the elimination of energy waste in production or any other economic activity (Liu et al., 2021b). According to the International Energy Agency (IEA), “Energy efficiency is key to ensuring a safe, reliable, affordable and sustainable energy system for the future. It is the only energy resource that every country possesses in abundance and is the quickest and least costly way of addressing energy security, environmental and economic challenges".
Improvement in green energy efficiency (GEE) ensures improved energy supply security, which is decisive for sustainable development (Murshed et al., 2020; Miao et al., 2022). Moreover, ramping up green energy efficiency by deploying green innovations, smart grids, and new green transport technologies contribute to curtailing greenhouse gas emissions, reducing the imported energy demand, and lowering the energy cost of households and the economy. Moreover, green energy efficiency has vast opportunities for each economic sector, such as manufacturing, transportation, real estate, and energy production, in terms of pollution control. China has significantly improved its industrial energy efficiency in the last couple of years by investing in technology innovations of RE resources (Irfan et al., 2020; Ling et al., 2021). However, there is still a significant demand and supply gap for which the improvement of GEE is the crucial step to reducing carbon intensity and energy protection.
Similarly, various studies have pronounced technology innovation as crucial for economic growth. However, these innovations boost not only financial actions but also spur environmental degradation (Chien et al., 2021a), whereas eco-innovation (as green innovation proxy) is a pivotal means of economic sustainability and an effective means to reduce the emanation of greenhouse gases (Ali et al., 2021). Eco-innovations (ECO) is “the adaptation of new concepts, ideas, and technologies for the procedural development of economic restructuring and optimization”. The enhancement of ECO enables countries to transform the traditional economic structure into a more energy-efficient production structure (Chien et al., 2021b; Ding et al., 2021; Hu et al., 2022).
Most of the existing literature has explored the dynamic relationship between renewable energy and carbon emissions, such as Chen et al. (2019), Chien et al. (2021c), Lin and Zhu (2019), and Wang B. et al. (2018), energy efficiency (Yu et al., 2019; Wang et al., 2021; Chien et al., 2022), and eco-innovation and carbon emission (Afshan and Yaqoob, 2022; Chien et al., 2021a; Hsu et al., 2021; Jin et al., 2021) for China. However, no such study explains the role of GEE and ECO on CO2 in China. As discussed earlier, China is a significant greenhouse gas emitter and nonrenewable and the largest energy consumer; thus, for China, the role of GEE and ECO is crucial to pursuing the environmental sustainability objective. Therefore, to respond to the gap, the current study explores the role of green energy efficiency (GEE) and eco-innovation (ECO) on environmental pollution (CO2) in China along with two control variables such as urbanization (URB) and trade (TRD). Moreover, the study’s outcomes will provide insights for establishing better policies.
Moreover, to examine the relationship between GEE, ECO, URB, and TR and CO2 more precisely, the study employs the QARDL (quantile autoregressive distributed lag) method. This method of estimations is advantageous over the other traditional regression analysis methods in many ways; for instance, this method considers the magnitude, such as quantiles to get parameter estimates and elaborates the association among the study variables in the long run and short run. These estimates provide more reliable, accurate, and detailed relationships. Moreover, the QARDL method incorporates the potential asymmetric and nonlinear relationship between the GEE, ECO, URB, and TR and CO2 (Cho et al., 2015; Hsu et al., 2021; Ji et al., 2021; Jin et al., 2021; Sharif et al., 2020; Song et al., 2021). Thus, based on these motivations, the study incorporated the QARDL as the most effective method for the analysis.
The study’s outcomes illustrate that the GEE and ECO significantly reduce carbon emissions at all pollution levels in the long run. At the same time, URB enhances carbon emission at lower and significant pollution levels in the long run, while TRD only harms the quality of the environment at the low level of pollution in China in the long term. However, for the short-term impact, it has been observed that GEE significantly decreases CO2 at a low pollution level while ECO drops CO2 significantly at a high pollution level. At the same time, URB promotes CO2 at all levels of pollution, while TRD only promotes CO2 at the low level of pollution.
The remaining part of the study is structured as follows: section 4 discusses the relevant studies that already explored relationships among the study variables. Section 3 defines the variables data source and the QARDL model from a theoretical perspective. Moreover, section 4 interprets the outcomes of all incorporated tests. Section 5 summarized the analysis in the form of a conclusion and suggested some policy recommendations based on that conclusion.
Literature Review
Recent studies have a growing concern to explore the ways to reduce environmental pollution and obtain economic and environmental sustainability. As discussed earlier in the introduction section, various recent studies have accounted for different driving factors to control environmental degradation in China, for instance, RE resource consumption (Khattak et al., 2020; Godil et al., 2021), technological innovations (Shahbaz et al., 2020), green innovation (Hu et al., 2022), industrialization (Dong K. et al., 2018; Wang and Su, 2019), globalization (Ling et al., 2021), economic growth (Liu et al., 2019), trade openness (Liu et al., 2021a), environmental regulations (Li et al., 2022), environmental taxes (Hsu et al., 2021), tourism (Razzaq et al., 2021a), FDI inflows (Shahbaz et al., 2020), and financial development (Godil et al., 2020). It has been observed that the existing literature does not provide any single study which has addressed the role of green energy efficiency on environmental degradation in China. Therefore, the current study incorporates the variable of green energy efficiency first time to investigate its impact on environmental pollution in China. Moreover, this section compiles the existing literature on the study variables.
Green Energy Efficiency and Environmental Pollution
Renewable energy implies a decrease in nonrenewable energy consumption and mitigates the detrimental effect of environmental pollution (Razmjoo et al., 2021). Similarly, energy efficiency is also decisive in controlling carbon emissions (Qin et al., 2020; Irfan et al., 2022); therefore, green energy efficiency is considered the foremost tool to mitigate the adverse effects of greenhouse gases (GHGs). There is no such study that accounts for the green or renewable energy efficiency specifically to analyze. However, few studies incorporate renewable energy and energy efficiency separately to examine their diminishing role in carbon emission (Bhadbhade et al., 2019; Chien et al., 2022; Dong F. et al., 2018; Guo and Pachauri, 2017; Huang et al., 2018; Shao et al., 2019; Wang et al., 2021; Zhang et al., 2017).
Gökgöz and Güvercin (2018) have evaluated the role of the GEE on the energy security of EU countries from 2004 to 2014. The study explored that renewable energy efficiency decreases with the increase in energy production; therefore, the emission level of GHGs is enhanced. Murshed (2020) has studied the indirect impact of ICT trade on CO2 emission through energy efficiency in the South Asian economies. The outcomes of the panel estimation of CUP-FM suggested that the ICT trade enhances renewable energy consumption, leading to improved energy efficiency and environmental quality.
Furthermore, Akram et al. (2021) have also examined the impact of RE and energy efficiency on CO2 for 66 underdeveloped countries. The findings of the quantile regression method are based on the panel data from 1990 to 2014 and endorse the negative impact of energy efficiency (EE) on CO2 at high quantiles. In addition, Liu et al. (2021b) have investigated provisional policies’ role in China’s energy efficiency. The findings stated that the EE is the only way through which China can achieve its predefined goal of 2030 to reduce its fossil fuel consumption; therefore, by adopting low carbon technologies, China can rely more on renewable energy resources and improve its energy efficiency (Khan et al., 2021; Khan et al., 2022).
Another study by Li and Ouyang (2020) has explored the factors in the heavy industrial sector of china that can mitigate carbon emissions without compromising economic growth. The result of the study exhibited that clean energy consumption and the technological progress of EE can reduce the carbon intensity in China. Moreover, the studies by Bhadbhade et al. (2019), Chien et al. (2022), and Huang et al. (2018) have also found a negative association between energy efficiency and CO2 (Irfan et al., 2019).
Nexus Between Eco-innovation and Environmental Pollution
The association between ECO and CO2 has been analyzed by a vast number of studies (Khan et al., 2020; Chien et al., 2021b; Ding et al., 2021; Ji et al., 2021; Jin et al., 2021; Tao et al., 2021). ECO motivates the economies to adopt new technologies to reduce environmental pollution. It reduces traditional energy resource consumption by providing alternate solutions and controlling CO2 (Ji et al., 2021; Hu et al., 2022). Ali et al. (2021) have analyzed the role of ECO to mitigate CO2 in the top 10 GHG emitters of the world and found that the ECO negatively influences carbon emanation and enhances economic activities by promoting renewable energy resource consumption. Similarly, the outcomes of the study presented by Shahbaz et al. (2020) support the findings of previous studies and consider the ECO as the primary tool to control CO2 in highly industrial economies.
Moreover, the finding of (Ji et al., 2021) study has redefined the impact of ECO on environmental quality. They explored that the highly fiscal decentralized economies experienced a significant decline in CO2 in the long term due to ECO. Ding et al. (2021) have investigated the influence of ECO on CO2 in G-7 countries from 1990 to 2018. The outcomes of the CS-ARDL estimator also endorse the mitigating impact of ECO on CO2.
Materials and Methods
Data Description
The current study attempted to explore the impact of GEE and ECO to control CO2 in China; therefore, the study incorporates the data from 1995 to 2019 on carbon emission (CO2) as dependent variables, green energy efficiency (GEE) and eco-innovation (ECO) as focus variables while the urbanization (URB) and trade (TRD) are taken as control variables. Table 1 illustrates the details of the explained variables. This has been converted from annual to quarters following the match sum approach (Razzaq et al., 2021b). Table 2 presents the detail descriptive statistics of model variables.
Theoretical Background and Methodology
The study intended to investigate the dynamic long-run correlation between green energy efficiency (GEE), eco-innovation (ECO), urbanization (URB), trade (TRD), and environmental pollution (CO2) for China by employing the QARDL model introduced by Cho et al. (2015) which helps to test the long-run equilibrium effect of all variables on carbon emission across the different grids of quantiles. The relationship between the socioeconomic variables is not necessarily linear, as assumed by the general regression method of ordinary least squares (OLS) (Liu et al., 2021a; Razzaq et al., 2021a). Moreover, another econometric method known as ARDL (autoregressive distribution lag) considers the different order cointegration and determines only the cointegration among variables. Therefore, to alleviate the analysis’s methodological deficiencies, this study incorporates the QARDL method.
QARDL method determines the nonlinear or asymmetric long-run and the short-run association between the variables over the different conditional distributions of the variables (Song et al., 2021). Moreover, for the robustness analysis and the time-varying integration, the study applied the Wald test, which allowed examining the dependency of parameters and steadiness of the integrated coefficient in each quartile (Godil et al., 2021; Song et al., 2021; Razzaq et al., 2022b). The ARDL model for this study is given as follows:
According to Su et al. (2021) and Sharif et al. (2020), technological innovations accelerate fossil fuel consumption, improve economic growth, and enhance environmental degradation. However, eco-innovation reduces the traditional energy resource consumption by providing alternate solutions and controlling CO2 (Ji et al., 2021; Hu et al., 2022); therefore, the ECO is expected to reduce CO2, such as
Results and Discussion
Table 1 illustrates the outcomes of the descriptive analysis of environmental pollution (CO2), green energy efficiency (GEE), eco-innovation (ECO), urbanization (URB), and trade (TRD). It has been observed that GEE has the lowest mean value of 0.498 between the range of 0.428–0.560. In comparison, URB has the highest mean value of 10.958, which lies between 3.00 and 14.00. Similarly, the highest standard deviation value is also demonstrated by URB, which is 3.076, while the least standard deviation of 0.045 is observed for GEE. Moreover, the Jarque–Bera statistic evaluates the normality among the variables. The results illustrated that all the variables meet the significance level of 1% and endorse that the data distribution is not normal. Therefore, this study is allowed to apply the QARDL method to obtain the regression estimates of the selected data (Sharif et al., 2020; Razzaq et al., 2021b; Chien et al., 2021c; Godil et al., 2021; Song et al., 2021).
In the time-series data, it is crucial to ensure the integration order of the series before applying the QARDL model for estimations (Razzaq et al., 2021c; Song et al., 2021). Therefore, this study conducted the ZA (Zivot–Andrew) and ADF (Augmented Dickey–Fuller test) unit root tests to determine whether the time series data are stationary. However, the ZA test has more importance because it considers the structural break. Table 3 presents the estimates of the ADF and ZA test, which affirm the non-stationarity of variables at the level. However, ZA and ADF test results reject the null hypothesis and endorse all variables’ stationarity at the first difference at 1 and 5% significance levels. Based on these results, it has been assumed that the QARDL is the most appropriate method for estimations that account for the structural break, nonlinearity, and dynamic trend in the data (Sharif et al., 2020; Godil et al., 2021).
Table 4 illustrates the estimates of the QARDL methods. The test outcomes revealed the interaction between the GEE, ECO, URB, TRD, and CO2. Moreover, the parameter of ECM or the value of
Similarly, the results from Table 4 revealed that the ECO has a significantly negative association with CO2 at all quantiles in the long run which has proven that ECO is the adaptation of advanced technology to prevent the waste of energy resources, promote a green economy, and ultimately control carbon emission. Eco-innovation is considered the main component of policy formulation related to environmental sustainability. It reduces the pollution of the environment with the mutual influence of the other economic variables, for instance, energy efficiency, renewable energy resources, and the development of infrastructure and production mechanisms to reduce the dependency on traditional energy recourse. The result is aligned with previous study results (Hu et al., 2022; Ji et al., 2021; Khattak et al., 2020; Shao et al., 2021).
Moreover, in the long run, the coefficient of URB shows a positively significant impact on CO2 at the lowest quartile (0.05) at a 10% level of significance. In contrast, at a low quantile (0.2), the effect is positive but significant at 5% significance, while at the medium to high quartile (0.60–0.95), the influence is positive and highly significant, indicating that urbanization puts environmental pressure on the economy at a high pollution level. The increase in urbanization drives industrial production accelerating energy demand and infrastructure development, increasing the consumption of nonrenewable energy resources, and enhancing carbon emissions. The findings are supported by recent studies (Afridi et al., 2019; Huang et al., 2021; Wang and Su, 2019; Wang S. et al., 2018; Yao et al., 2021).
Similarly, the outcome for the nexus between TRD and CO2 revealed that TRD has a positive impact on environmental pollution across all quantiles. However, it is significant only at a lower quantile (0.05–0.30) at the 5% level of significance, which exhibits that the increase in trade activities accelerates the carbon emission at the low pollution level in China. Trade has an essential role in enhancing production activities and economic development, leading to more energy consumption and becoming the reason for more carbon emissions in the long run. This result is in line with the existing studies (Du et al., 2019; Lv and Xu, 2019; Wang and Zhang, 2021), whereas the findings are contrary to the results of Wang and Zhang (2021).
On the other hand, to evaluate the short-run dynamics of analysis (Table 4), the study’s findings revealed that variation in the present CO2 is significantly enhanced by their previous level in China at all quantiles at a 1% level of significance. The accumulated variation of past and present GEEs negatively and greatly influenced the current CO2 level at the lowest to low quantiles (0.05–0.20) at the 1% significance level. In comparison, at the middle quantile (0.30), the impact remains significantly opposite at 5% significance, indicating that green energy efficiency combats the carbon emission at a low pollution level in China in the short run. Similarly, the combined variation of previous and current ECO significantly reduces the current level of CO2. However, this negative impact remains significant only at high to the highest quantile of (0.80) at 10%, (0.90) at 5%, and (0.95) at 1% level of significance, which has exhibited that the ECO can only help reduce the carbon emission at a high pollution level in the short run.
Moreover, in the short run, the past and present changes in URB significantly promote the current changes in CO2 across all quantiles. In contrast, the cumulative variations in the current and previous TRD enhance the current level of CO2 at the lowest to middle quantiles (0.05–0.40), representing that the TRD only increases the carbon emission at a low level of environmental pollution. Hence, the overall outcomes of the QARDL model revealed that for China, the GEE and ECO have a negative influence, whereas URB and TRD have a positive influence on CO2 in the long run and the short run at different pollution levels.
The Wald test results presented in Table 5 confirm the long-run and short-run asymmetric associations of the GEE, ECO, URB, and TRD with CO2. The outcomes to check the consistency or stability of the parameter revealed that the null hypothesis of the linearity of the speed of adjustment parameter has been rejected. Similarly, the hypothesis of long-run parameters consistency has also been rejected by the coefficients of
Conclusion and Policy Recommendations
The current study investigated the relationship between GEE, ECO, URB, TRD, and CO2 in China. The study employed the quarterly time-series data from 1995 to 2019 and applied the most appropriate and advanced quantile autoregressive distributed lag (QARDL) method to get detailed, reliable, and accurate estimates. The study’s empirical finding reveals that green energy efficiency and eco-innovation significantly reduced environmental pollution in the long run. China has heavily invested in and subsidized various green energy projects such as FIT schemes and the subsidies for solar PV installation, which has enhanced the green energy efficiency to work as a catalyst to reduce environmental degradation. The negative association of the GEE with CO2 proves that energy efficiency can be used as the appropriate tool to curb environmental pollution (Chien et al., 2022; Xu et al., 2021; Zhou et al., 2018).
Similarly, the negative correlation between ECO and CO2 also endorses that the ECO is considered the main component of policy formulation related to environmental sustainability. It enhances the efficiency of renewable energy resources, prevents energy waste, and promotes a green economy by reducing carbon emissions (Khattak et al., 2020; Ji et al., 2021; Tao et al., 2021; Hu et al., 2022). At the same time, urbanization enhances environmental degradation at low and high quantiles. The increase in urbanization drives industrial production and accelerates energy demand and infrastructure development, leading to the increased consumption of nonrenewable energy resources and carbon emissions (Afridi et al., 2019; Huang et al., 2021). When trade or trade openness only promotes environmental pollution in low quantiles, an increase in trade activities accelerates and causes environmental degradation (Du et al., 2019; Wang and Zhang, 2021). However, the findings remain the same in the short run except for the green energy efficiency, which decreases CO2 at a low quantile, and eco-innovation, which reduces CO2 at a high quantile. Moreover, the study found bidirectional causality among all variables at all quantiles. Thus, it has been concluded that GEE and ECO are the most reliable ways to control CO2 in China.
Based on the study’s conclusion, the government of China should develop policies to promote green energy efficiency and eco-innovation for each sector of the economy. China has additional green energy efficiency potential, which has not yet been quantified due to the obstacles and barriers hampering the cost-effective technologies to improve green energy efficiency. By providing more incentives, the government of China can attract more private investors to boost the investment in green energy efficiency projects. Similarly, eco-innovation effectively promotes economic and environmental sustainability by breaking down the resources and environmental constraints. Therefore, implementing the environmental regulations enhances green energy efficiency, saves energy, and reduces carbon emission. Government and policymakers need to add green energy efficiency and eco-innovations in the planning of smart cities; they can construct green buildings and infrastructure to promote innovative heating, ventilation, and cooling system in urban areas to make more resilient cities. Similarly, optimal renewable energy resources and integrated energy services will make the production sector more intelligent and competitive.
Moreover, for the production sector, the authorities should also promote the investment in private venture capital in R&D related to improving green energy efficiency and eco-innovation by reducing the corporate taxes on energy-saving and energy management projects. Furthermore, the private venture capital will also support the startups to improve green energy efficiency sector-wise. Despite the investment, there is also a need to create awareness and strong skills by knowledge sharing to obtain the maximum benefit of green energy efficiency in terms of business case investment and green energy efficiency business measures. In addition, the inclusion of green energy efficiency standards in the municipal regulations will help the local government adopt the energy efficiency measures to consider environmental sustainability in the city’s expansion plans and new building construction.
Data Availability Statement
The original contributions presented in the study are included in the article/Supplementary materials; further inquiries can be directed to the corresponding author.
Author Contributions
ZL presented the research’s idea and design, and CQ applied the model and drafted the manuscript.
Conflict of Interest
The authors declare that the research was conducted in the absence of any commercial or financial relationships that could be construed as a potential conflict of interest.
Publisher’s Note
All claims expressed in this article are solely those of the authors and do not necessarily represent those of their affiliated organizations, or those of the publisher, the editors, and the reviewers. Any product that may be evaluated in this article, or claim that may be made by its manufacturer, is not guaranteed or endorsed by the publisher.
References
Afridi, M. A., Kehelwalatenna, S., Naseem, I., and Tahir, M. (2019). Per Capita income, Trade Openness, Urbanization, Energy Consumption, and CO2 Emissions: An Empirical Study on the SAARC Region. Environ. Sci. Pollut. Res. 26 (29), 29978–29990. doi:10.1007/s11356-019-06154-2
Afshan, S., and Yaqoob, T. (2022). The Potency of Eco-Innovation, Natural Resource and Financial Development on Ecological Footprint: A Quantile-ARDL-Based Evidence from China. Environ. Sci. Pollut. Res. doi:10.1007/s11356-022-19471-w
Ahmad, B., Irfan, M., Salem, S., and Asif, M. H. (2022). Energy Efficiency in the Post-COVID-19 Era: Exploring the Determinants of Energy-Saving Intentions and Behaviors. Front. Energy Res. 9, 824318. doi:10.3389/fenrg.2021.824318
Akram, R., Chen, F., Khalid, F., Huang, G., and Irfan, M. (2021). Heterogeneous Effects of Energy Efficiency and Renewable Energy on Economic Growth of BRICS Countries: A Fixed Effect Panel Quantile Regression Analysis. Energy 215, 119019. doi:10.1016/j.energy.2020.119019
Ali, S., Dogan, E., Chen, F., and Khan, Z. (2021). International Trade and Environmental Performance in Top Ten‐Emitters Countries: The Role of Eco‐Innovation and Renewable Energy Consumption. Sustain. Dev. 29 (2), 378–387. doi:10.1002/sd.2153
Asiedu, B. A., Hassan, A. A., and Bein, M. A. (2021). Renewable Energy, Non-Renewable Energy, and Economic Growth: Evidence from 26 European Countries. Environ. Sci. Pollut. Res. 28 (9), 11119–11128. doi:10.1007/S11356-020-11186-0/TABLES/11
Atchike, D. W., Irfan, M., Ahmad, M., and Rehman, M. A. (2022). Waste-to-Renewable Energy Transition: Biogas Generation for Sustainable Development. Front. Environ. Sci. 107, 840588. doi:10.3389/fenvs.2022.840588
Bhadbhade, N., Zuberi, M. J. S., and Patel, M. K. (2019). A Bottom-Up Analysis of Energy Efficiency Improvement and CO2 Emission Reduction Potentials for the Swiss Metals Sector. Energy 181, 173–186. doi:10.1016/j.energy.2019.05.172
Brown, M. A. (2021). Could the US Become a Role Model for Electricity Decarbonization? One Earth 4 (4), 466–469. doi:10.1016/j.oneear.2021.04.004
Chen, Y., Wang, Z., and Zhong, Z. (2019). CO2 Emissions, Economic Growth, Renewable and Non-Renewable Energy Production and Foreign Trade in China. Renew. Energy 131, 208–216. doi:10.1016/j.renene.2018.07.047
Chien, F., Ajaz, T., Andlib, Z., Chau, K. Y., Ahmad, P., and Sharif, A. (2021c). The Role of Technology Innovation, Renewable Energy and Globalization in Reducing Environmental Degradation in Pakistan: A Step Towards Sustainable Environment. Renew. Energy 177, 308–317. doi:10.1016/j.renene.2021.05.101
Chien, F., Hsu, C. C., Andlib, Z., Shah, M. I., Ajaz, T., and Genie, M. G. (2021a). The Role of Solar Energy and Eco‐Innovation in Reducing Environmental Degradation in China: Evidence from QARDL Approach. Integr. Envir. Assess. Manag. 18, 555–571. doi:10.1002/ieam.4500
Chien, F., Sadiq, M., Nawaz, M. A., Hussain, M. S., Tran, T. D., and Le Thanh, T. (2021b). A Step toward Reducing Air Pollution in Top Asian Economies: The Role of Green Energy, Eco-Innovation, and Environmental Taxes. J. Environ. Manag. 297, 113420. doi:10.1016/j.jenvman.2021.113420
Chien, F., Zhang, Y., Sadiq, M., and Hsu, C.-C. (2022). Financing for Energy Efficiency Solutions to Mitigate Opportunity Cost of Coal Consumption: An Empirical Analysis of Chinese Industries. Environ. Sci. Pollut. Res. 29 (2), 2448–2465. doi:10.1007/s11356-021-15701-9
Cho, J. S., Kim, T.-h., and Shin, Y. (2015). Quantile Cointegration in the Autoregressive Distributed-Lag Modeling Framework. J. Econ. 188 (1), 281–300. doi:10.1016/j.jeconom.2015.05.003
Ding, Q., Khattak, S. I., and Ahmad, M. (2021). Towards Sustainable Production and Consumption: Assessing the Impact of Energy Productivity and Eco-Innovation on Consumption-Based Carbon Dioxide Emissions (CCO2) in G-7 Nations. Sustain. Prod. Consum. 27, 254–268. doi:10.1016/j.spc.2020.11.004
Dong, F., Yu, B., Hadachin, T., Dai, Y., Wang, Y., Zhang, S., et al. (2018a). Drivers of Carbon Emission Intensity Change in China. Resour. Conservation Recycl. 129, 187–201. doi:10.1016/j.resconrec.2017.10.035
Dong, K., Sun, R., Jiang, H., and Zeng, X. (2018b). CO2 Emissions, Economic Growth, and the Environmental Kuznets Curve in China: What Roles Can Nuclear Energy and Renewable Energy Play? J. Clean. Prod. 196, 51–63. doi:10.1016/j.jclepro.2018.05.271
Du, K., Li, P., and Yan, Z. (2019). Do green Technology Innovations Contribute to Carbon Dioxide Emission Reduction? Empirical Evidence from Patent Data. Technol. Forecast. Soc. Change 146 (June), 297–303. doi:10.1016/j.techfore.2019.06.010
Fang, Z., Razzaq, A., Mohsin, M., and Irfan, M. (2022). Spatial Spillovers and Threshold Effects of Internet Development and Entrepreneurship on Green Innovation Efficiency in China. Technol. Soc. 68, 101844. doi:10.1016/j.techsoc.2021.101844
Godil, D. I., Sharif, A., Agha, H., and Jermsittiparsert, K. (2020). The Dynamic Nonlinear Influence of ICT, Financial Development, and Institutional Quality on CO2 Emission in Pakistan: New Insights from QARDL Approach. Environ. Sci. Pollut. Res. 27 (19), 24190–24200. doi:10.1007/s11356-020-08619-1
Godil, D. I., Yu, Z., Sharif, A., Usman, R., and Khan, S. A. R. (2021). Investigate the Role of Technology Innovation and Renewable Energy in Reducing Transport Sector CO2 Emission in China: A Path Toward Sustainable Development. Sustain. Dev. 29 (4), 694–707. doi:10.1002/sd.2167
Gökgöz, F., and Güvercin, M. T. (2018). Energy Security and Renewable Energy Efficiency in EU. Renew. Sustain. Energy Rev. 96, 226–239. doi:10.1016/j.rser.2018.07.046
Guo, F., and Pachauri, S. (2017). China's Green Lights Program: A Review and Assessment. Energy Policy 110 (August), 31–39. doi:10.1016/j.enpol.2017.08.002
Hsu, C.-C., Zhang, Y., Ch, P., Aqdas, R., Chupradit, S., and Nawaz, A. (2021). A Step Towards Sustainable Environment in China: The Role of Eco-Innovation Renewable Energy and Environmental Taxes. J. Environ. Manag. 299, 113609. doi:10.1016/j.jenvman.2021.113609
Hu, J., Xu, J., Tong, L., and Razi, U. (2022). The Dynamic Role of Film and Drama Industry, Green Innovation towards the Sustainable Environment in China: Fresh Insight from NARDL Approach. Econ. Research-Ekonomska Istraživanja 1, 1–18. doi:10.1080/1331677X.2022.2026239
Huang, L., Krigsvoll, G., Johansen, F., Liu, Y., and Zhang, X. (2018). Carbon Emission of Global Construction Sector. Renew. Sustain. Energy Rev. 81, 1906–1916. doi:10.1016/j.rser.2017.06.001
Huang, S.-Z., Sadiq, M., and Chien, F. (2021). The Impact of Natural Resource Rent, Financial Development, and Urbanization on Carbon Emission. Environ. Sci. Pollut. Res. 1, 1–13. doi:10.1007/s11356-021-16818-7
Irfan, M., Elavarasan, R. M., Hao, Y., Feng, M., and Sailan, D. (2021). An Assessment of Consumers' Willingness to Utilize Solar Energy in China: End-Users' Perspective. J. Clean. Prod. 292, 126008. doi:10.1016/j.jclepro.2021.126008
Irfan, M., Razzaq, A., Chupradit, S., Javid, M., Rauf, A., and Aini Farooqi, T. J. (2022). Hydrogen Production Potential from Agricultural Biomass in Punjab Province of Pakistan. Int. J. Hydrogen Energy 47 (5), 2846–2861. doi:10.1016/j.ijhydene.2021.10.257
Irfan, M., Zhao, Z.-Y., Ahmad, M., and Mukeshimana, M. (2019). Solar Energy Development in Pakistan: Barriers and Policy Recommendations. Sustainability 11 (4), 1206. doi:10.3390/su11041206
Irfan, M., Zhao, Z.-Y., Panjwani, M. K., Mangi, F. H., Li, H., Jan, A., Ahmad, M., and Rehman, A. (2020). Assessing the Energy Dynamics of Pakistan: Prospects of Biomass Energy. Energy Rep. 6, 80–93. doi:10.1016/j.egyr.2019.11.161
Ji, X., Umar, M., Ali, S., Ali, W., Tang, K., and Khan, Z. (2021). Does Fiscal Decentralization and Eco‐Innovation Promote Sustainable Environment? A Case Study of Selected Fiscally Decentralized Countries. Sustain. Dev. 29 (1), 79–88. doi:10.1002/sd.2132
Jin, C., Razzaq, A., Saleem, F., and Sinha, A. (2021). Asymmetric Effects of Eco-Innovation and Human Capital Development in Realizing Environmental Sustainability in China: Evidence from Quantile ARDL Framework. Econ. Research-Ekonomska Istraživanja 1, 24. doi:10.1080/1331677X.2021.2019598
Khan, I., Hou, F., Irfan, M., Zakari, A., and Le, H. P. (2021). Does Energy Trilemma a Driver of Economic Growth? The Roles of Energy Use, Population Growth, and Financial Development. Renew. Sustain. Energy Rev. 146, 111157. doi:10.1016/j.rser.2021.111157
Khan, I., Zakari, A., Ahmad, M., Irfan, M., and Hou, F. (2022). Linking Energy Transitions, Energy Consumption, and Environmental Sustainability in OECD Countries. Gondwana Res. 103, 445–457. doi:10.1016/j.gr.2021.10.026
Khan, Z., Ali, S., Umar, M., Kirikkaleli, D., and Jiao, Z. (2020). Consumption-Based Carbon Emissions and International Trade in G7 Countries: The Role of Environmental Innovation and Renewable Energy. Sci. Total Environ. 730, 138945. doi:10.1016/j.scitotenv.2020.138945
Khattak, S. I., Ahmad, M., Khan, Z. U., and Khan, A. (2020). Exploring the Impact of Innovation, Renewable Energy Consumption, and Income on CO2 Emissions: New Evidence from the BRICS Economies. Environ. Sci. Pollut. Res. 27 (12), 13866–13881. doi:10.1007/s11356-020-07876-4
Lei, W., Liu, L., Hafeez, M., and Sohail, S. (2022). Do economic Policy Uncertainty and Financial Development Influence the Renewable Energy Consumption Levels in China? Environ. Sci. Pollut. Res. 29 (5), 7907–7916. doi:10.1007/s11356-021-16194-2
Li, G., Gao, D., and Li, Y. (2022). Dynamic Environmental Regulation Threshold Effect of Technical Progress on Green Total Factor Energy Efficiency: Evidence from China. Environ. Sci. Pollut. Res. 29 (6), 8804–8815. doi:10.1007/s11356-021-16292-1
Li, P., and Ouyang, Y. (2020). Quantifying the Role of Technical Progress Towards China's 2030 Carbon Intensity Target. J. Environ. Plan. Manag. 64, 379–398. doi:10.1080/09640568.2020.1764343
Lin, B., and Zhu, J. (2019). The Role of Renewable Energy Technological Innovation on Climate Change: Empirical Evidence from China. Sci. Total Environ. 659, 1505–1512. doi:10.1016/j.scitotenv.2018.12.449
Ling, G., Razzaq, A., Guo, Y., Fatima, T., and Shahzad, F. (2021). Asymmetric and Time-Varying Linkages between Carbon Emissions, Globalization, Natural Resources and Financial Development in China. Environ. Dev. Sustain 24, 6702–6730. doi:10.1007/s10668-021-01724-2
Liu, J., Li, Z., Dong, C., and Sriboonchitta, S. (2019). The Impact of Economic Growth, Energy Consumption and Trade Openness on Carbon Emissions: An Empirical Analysis in China. Lect. Notes Comput. Sci. 11471, 235–244. doi:10.1007/978-3-030-14815-7_20
Liu, J., Nathaniel, S. P., Chupradit, S., Hussain, A., Köksal, C., and Aziz, N. (2021a). Environmental Performance and International Trade in China: The Role of Renewable Energy and Eco‐innovation. Integr Envir Assess Manag 18, 813–823. doi:10.1002/ieam.4530
Liu, J., Wei, D., Tian, Y., and Li, Q. (2021b). Evolution and Policy Effect Assessment for the Spatial Heterogeneity Pattern of Regional Energy Efficiency in China. Energy Effic. 14 (8), 1–16. doi:10.1007/s12053-021-09996-3
Lu, Y., Zhang, Y., Cao, X., Wang, C., Wang, Y., Zhang, M., et al. (2019). Forty Years of Reform and Opening up: China's Progress toward a Sustainable Path. Sci. Adv. 5 (8), 9413. doi:10.1126/sciadv.aau9413
Lv, Z., and Xu, T. (2019). Trade Openness, Urbanization and CO2 Emissions: Dynamic Panel Data Analysis of Middle-Income Countries. J. Int. Trade & Econ. Dev. 28 (3), 317–330. doi:10.1080/09638199.2018.1534878
Madurai Elavarasan, R., Pugazhendhi, R., Irfan, M., Mihet-Popa, L., Khan, I. A., and Campana, P. E. (2022). State-of-the-Art Sustainable Approaches for Deeper Decarbonization in Europe - An Endowment to Climate Neutral Vision. Renew. Sustain. Energy Rev. 159, 112204. doi:10.1016/j.rser.2022.112204
Miao, J., Wang, X., Bai, S., Xiang, Y., and Li, L. (2021). Distance-to-Target Weighting Factor Sets in LCA for China Under 2030 Vision. J. Clean. Prod. 314, 128010. doi:10.1016/j.jclepro.2021.128010
Miao, Y., Razzaq, A., Adebayo, T. S., and Awosusi, A. A. (2022). Do Renewable Energy Consumption and Financial Globalisation Contribute to Ecological Sustainability in Newly Industrialized Countries? Renew. Energy 187, 688–697. doi:10.1016/j.renene.2022.01.073
Murshed, M. (2020). An Empirical Analysis of the Non-Linear Impacts of ICT-Trade Openness on Renewable Energy Transition, Energy Efficiency, Clean Cooking Fuel Access and Environmental Sustainability in South Asia. Environ. Sci. Pollut. Res. 27 (29), 36254–36281. doi:10.1007/s11356-020-09497-3
Murshed, M., Chadni, M. H., and Ferdaus, J. (2020). Does ICT Trade Facilitate Renewable Energy Transition and Environmental Sustainability? Evidence from Bangladesh, India, Pakistan, Sri Lanka, Nepal and Maldives. Energy Ecol. Environ. 5 (6), 470–495. doi:10.1007/s40974-020-00190-2
Osiyevskyy, O., Shirokova, G., and Ritala, P. (2020). Exploration and Exploitation in Crisis Environment: Implications for Level and Variability of Firm Performance. J. Bus. Res. 114, 227–239. doi:10.1016/j.jbusres.2020.04.015
Pata, U. K. (2018). The Influence of Coal and Noncarbohydrate Energy Consumption on CO2 Emissions: Revisiting the Environmental Kuznets Curve Hypothesis for Turkey. Energy 160, 1115–1123. doi:10.1016/j.energy.2018.07.095
Qi, X., Han, Y., and Kou, P. (2020). Population Urbanization, Trade Openness and Carbon Emissions: an Empirical Analysis Based on China. Air Qual. Atmos. Health 13 (5), 519–528. doi:10.1007/s11869-020-00808-8
Qin, Y., Niu, G., Wang, X., Luo, D., and Duan, Y. (2020). Conversion of CO2 in a Low-Powered Atmospheric Microwave Plasma: In-Depth Study on the Trade-Off Between CO2 Conversion and Energy Efficiency. Chem. Phys. 538 (June), 110913. doi:10.1016/j.chemphys.2020.110913
Razmjoo, A., Gakenia Kaigutha, L., Vaziri Rad, M. A., Marzband, M., Davarpanah, A., and Denai, M. (2021). A Technical Analysis Investigating Energy Sustainability Utilizing Reliable Renewable Energy Sources to Reduce CO2 Emissions in a High Potential Area. Renew. Energy 164, 46–57. doi:10.1016/j.renene.2020.09.042
Razzaq, A., Ajaz, T., Li, J. C., Irfan, M., and Suksatan, W. (2021b). Investigating the Asymmetric Linkages Between Infrastructure Development, Green Innovation, and Consumption-Based Material Footprint: Novel Empirical Estimations from Highly Resource-Consuming Economies. Resour. Policy 74, 102302. doi:10.1016/j.resourpol.2021.102302
Razzaq, A., Cui, Y., Irfan, M., Maneengam, A., and Acevedo-Duque, Á. (2022b). Asymmetric Effects of Fine Particulate Matter and Stringency Policy on COVID-19 Intensity. Int. J. Environ. Health Res. 1, 13. doi:10.1080/09603123.2022.2059452
Razzaq, A., Fatima, T., and Murshed, M. (2021c). Asymmetric Effects of Tourism Development and Green Innovation on Economic Growth and Carbon Emissions in Top 10 GDP Countries. J. Environ. Plan. Manag. 1, 30. doi:10.1080/09640568.2021.1990029
Razzaq, A., Sharif, A., Ahmad, P., and Jermsittiparsert, K. (2021a). Asymmetric Role of Tourism Development and Technology Innovation on Carbon Dioxide Emission Reduction in the Chinese Economy: Fresh Insights from QARDL Approach. Sustain. Dev. 29 (1), 176–193. doi:10.1002/sd.2139
Shahbaz, M., Raghutla, C., Song, M., Zameer, H., and Jiao, Z. (2020). Public-Private Partnerships Investment in Energy as New Determinant of CO2 Emissions: The Role of Technological Innovations in China. Energy Econ. 86, 104664. doi:10.1016/j.eneco.2020.104664
Shao, S., Yang, Z., Yang, L., and Ma, S. (2019). Can China's Energy Intensity Constraint Policy Promote Total Factor Energy Efficiency? Evidence from the Industrial Sector. Ej 40 (4), 101–128. doi:10.5547/01956574.40.4.ssha
Shao, X., Zhong, Y., Liu, W., and Li, R. Y. M. (2021). Modeling the Effect of Green Technology Innovation and Renewable Energy on Carbon Neutrality in N-11 Countries? Evidence from Advance Panel Estimations. J. Environ. Manag. 296 (March), 113189. doi:10.1016/j.jenvman.2021.113189
Sharif, A., Baris-Tuzemen, O., Uzuner, G., Ozturk, I., and Sinha, A. (2020). Revisiting the Role of Renewable and Non-Renewable Energy Consumption on Turkey's Ecological Footprint: Evidence from Quantile ARDL Approach. Sustain. Cities Soc. 57, 102138. doi:10.1016/j.scs.2020.102138
Song, Y., Zhao, P., Chang, H.-L., Razi, U., Dinca, M. S., Jiang, C., et al. (2021). Does the COVID-19 Pandemic Affect the Tourism Industry in China? Evidence from Extreme Quantiles Approach. Econ. Research-Ekonomska Istraživanja 0 (0), 1–18. doi:10.1080/1331677X.2021.1941180
Su, C.-W., Song, Y., and Umar, M. (2021). Financial Aspects of Marine Economic Growth: From the Perspective of Coastal Provinces and Regions in China. Ocean Coast. Manag. 204, 105550. doi:10.1016/j.ocecoaman.2021.105550
Sun, Y., Anwar, A., Razzaq, A., Liang, X., and Siddique, M. (2022a). Asymmetric Role of Renewable Energy, Green Innovation, and Globalization in Deriving Environmental Sustainability: Evidence from Top-10 Polluted Countries. Renew. Energy 185, 280–290. doi:10.1016/j.renene.2021.12.038
Sun, Y., Guan, W., Asif, R., Shahzad, M., and An, N. B. (2022b). Transition Towards Ecological Sustainability Through Fiscal Decentralization, Renewable Energy and Green Investment in OECD Countries. Renew. Energy 190, 385–395. doi:10.1016/j.renene.2022.03.099
Sun, Y., and Razzaq, A. (2022). Composite Fiscal Decentralization and Green Innovation: Imperative Strategy for Institutional Reforms and Sustainable Development in OECD Countries. Sustain. Dev. doi:10.1002/sd.2292
Tao, R., Umar, M., Naseer, A., and Razi, U. (2021). The Dynamic Effect of Eco-Innovation and Environmental Taxes on Carbon Neutrality Target in Emerging Seven (E7) Economies. J. Environ. Manag. 299, 113525. doi:10.1016/j.jenvman.2021.113525
Wang, B., Wang, Q., Wei, Y.-M., and Li, Z.-P. (2018a). Role of Renewable Energy in China's Energy Security and Climate Change Mitigation: An Index Decomposition Analysis. Renew. Sustain. Energy Rev. 90, 187–194. doi:10.1016/j.rser.2018.03.012
Wang, Q., and Su, M. (2019). The Effects of Urbanization and Industrialization on Decoupling Economic Growth from Carbon Emission - A Case Study of China. Sustain. Cities Soc. 51, 101758. doi:10.1016/j.scs.2019.101758
Wang, Q., and Zhang, F. (2021). The Effects of Trade Openness on Decoupling Carbon Emissions from Economic Growth - Evidence from 182 Countries. J. Clean. Prod. 279, 123838. doi:10.1016/j.jclepro.2020.123838
Wang, R.-M., Tian, Z., and Ren, F.-r. (2021). Energy Efficiency in China: Optimization and Comparison Between Hydropower and Thermal Power. Energ Sustain Soc. 11 (1), 1–21. doi:10.1186/s13705-021-00311-7
Wang, S., Li, G., and Fang, C. (2018b). Urbanization, Economic Growth, Energy Consumption, and CO2 Emissions: Empirical Evidence from Countries with Different Income Levels. Renew. Sustain. Energy Rev. 81, 2144–2159. doi:10.1016/j.rser.2017.06.025
Wu, H., Hao, Y., Ren, S., Yang, X., and Xie, G. (2021). Does Internet Development Improve Green Total Factor Energy Efficiency? Evidence from China. Energy Policy 153, 112247. doi:10.1016/j.enpol.2021.112247
Xu, Y., Qin, Z., Gui, G., Gacanin, H., Sari, H., and Adachi, F. (2021). Energy Efficiency Maximization in NOMA Enabled Backscatter Communications with QoS Guarantee. IEEE Wirel. Commun. Lett. 10 (2), 353–357. doi:10.1109/LWC.2020.3031042
Yao, F., Zhu, H., and Wang, M. (2021). The Impact of Multiple Dimensions of Urbanization on CO2 Emissions: A Spatial and Threshold Analysis of Panel Data on China's Prefecture-Level Cities. Sustain. Cities Soc. 73, 103113. doi:10.1016/j.scs.2021.103113
Yu, J., Zhou, K., and Yang, S. (2019). Regional Heterogeneity of China's Energy Efficiency in “New Normal”: A Meta-Frontier Super-SBM Analysis. Energy Policy 134, 110941. doi:10.1016/j.enpol.2019.110941
Zhang, Y.-J., Peng, Y.-L., Ma, C.-Q., and Shen, B. (2017). Can Environmental Innovation Facilitate Carbon Emissions Reduction? Evidence from China. Energy Policy 100, 18–28. doi:10.1016/j.enpol.2016.10.005
Keywords: green energy efficiency, renewable energy, climate technologies, environmental pollution, COVID-19
Citation: Liu Z, Qiu C and Zhu L (2022) Dynamic Role of Green Energy Efficiency and Climate Technologies in Realizing Environmental Sustainability: Fresh Insights From China. Front. Environ. Sci. 10:908470. doi: 10.3389/fenvs.2022.908470
Received: 30 March 2022; Accepted: 03 May 2022;
Published: 27 September 2022.
Edited by:
Asif Razzaq, Dalian University of Technology, ChinaReviewed by:
Yunpeng Sun, Tianjin University of Commerce, ChinaHafiz Waqas Kamran, Iqra University, Pakistan
Copyright © 2022 Liu, Qiu and Zhu. This is an open-access article distributed under the terms of the Creative Commons Attribution License (CC BY). The use, distribution or reproduction in other forums is permitted, provided the original author(s) and the copyright owner(s) are credited and that the original publication in this journal is cited, in accordance with accepted academic practice. No use, distribution or reproduction is permitted which does not comply with these terms.
*Correspondence: Changbo Qiu, lzmhero_@126.com