- 1Laboratory of Plankton Ecology, Department of Zoology, Institute of Biology, Federal University of Juiz de Fora, Juiz de Fora, Brazil
- 2Laboratory of Ficology, National Museum, Federal University of Rio de Janeiro, Rio de Janeiro, Brazil
- 3Laboratory of Limnology, Department of Biology, Institute of Biology, Federal University of Rio de Janeiro, Rio de Janeiro, Brazil
- 4Laboratory of Aquatic Ecology, Department of Biology, Institute of Biology, Federal University of Juiz de Fora, Juiz de Fora, Brazil
Small Hydroelectric Power Plants (SHPs) are considered hydroelectric plants of reduced size and power that modify the landscape in a lower degree. As they are generally built-in sequence along the course of a river, these reservoirs are subject to the Cascading Reservoir Continuum Concept (CRCC), which provides a gradient effect mainly observed on suspended particles and nutrients. These effects can reflect in the phytoplankton community, which respond in terms of changes in their structure and function to environmental changes. Here, we aimed to investigate whether spatial variation would be more explanatory than temporal variation for phytoplankton structure and composition in cascading SHPs reservoirs. Furthermore, we sought to understand the effect of the hydrodynamics of run-of-river and storage reservoirs for phytoplankton community composition. We assessed the predictive power of physical and chemical conditions of the water, and zooplankton density as explanatory variables for phytoplankton taxonomic and functional diversity. The study was carried out over 6 years in three SHPs located on the Paraibuna River, Brazil, totaling 24 campaigns. After counting and identification, the phytoplankton species were classified according to qualitative functional traits related to morphology, physiology, and behavior. The reservoirs had significant differences in nutrients and suspended solids, corroborating with the CRCC. Nonetheless, these variables alone were not able to explain the distribution of phytoplanktonic species. However, as significant coupling between phytoplankton and zooplankton was found, and zooplankton might have influenced the phytoplankton distribution along the longitudinal gradient of the river. Furthermore, changes in taxonomic and functional composition of phytoplankton were mainly related to reservoir hydrodynamics and temporal variation. The last reservoir in the cascade was the one with the greatest phytoplankton species and functional diversity. Taxonomic and functional diversity indices were positively correlated, but at a certain point, functional richness reached a plateau. Here we highlight the complexity of understanding the role of cascading reservoirs in the structure and function of phytoplanktonic communities, which are subject to large spatial and temporal variations, even within the same stretch of a river.
Introduction
Reservoirs are artificial aquatic ecosystems widely distributed in the landscape due to their multiple uses (Berga et al., 2006; Lehner et al., 2011). Among the reservoirs destined to produce electric energy, there are the Small Hydroelectric Power Plants (SHPs), considered hydroelectric plants of reduced size and power. In Brazil, SHPs have a power of 5–30 MW and less than 13 km2 of reservoir area (ANEEL—Agência Nacional de Energia Elétrica, 2020). The SHPs have been built as an alternative to large dams, once they interfere less with the dynamics of drainage basins (Couto and Olden, 2018). Since they use the natural course of the river for energy generation, SHPs that are run-of-river type have been preferred over other types (e.g., storage) as they maintain more natural water flow conditions, reducing environmental impacts (Egré and Milewski, 2002; Csiki and Rhoads 2010; Almeida et al., 2019).
Although SHPs have less impact in the landscape when compared to large hydroelectric reservoirs, the construction of their dams also causes a river discontinuity, generating a series of changes in hydrodynamics, river flow regulation and habitat fragmentation (Ward and Stanford, 1983). Moreover, in order to support the increase in demand for electricity generated by demographic and economic growth, many reservoirs are built in sequence, known as cascade reservoirs. The Cascading Reservoir Continuum Concept (CRCC) predicts that reservoirs in series are subject to a potential dilution effect, mainly nutrients and suspended particles (Barbosa et al., 1999). However, even under similar external conditions (e.g., climate, altitude), reservoirs might have particular features and biological communities. For instance, system may vary locally in hydrological conditions which change the inflow, outflow, water level and residence time in each of the reservoirs belonging to the cascade. Consequently, limnological conditions of the system such as turbidity, dissolved oxygen, total, suspended and dissolved solids are directly affected according to the type of reservoir (Barbosa et al.,1999; Nogueira et al., 2012; Ferrareze et al., 2014; Smith et al., 2014).
The phytoplankton constitutes the basis of the aquatic food chain and has been widely used as a biological indicator in the monitoring of aquatic environments (Reynolds, 2006). Phytoplankton can be regulated both by the bottom-up effects of nutrients, and the top-down effects of zooplankton. Through grazing, for example, zooplankton controls primary productivity and connects primary producers with other trophic levels (Quintana et al., 2015; Sailley et al., 2015). These organisms can rapidly signal changes in ecosystems through shifts in their structure, composition, and metabolism (Padisák et al., 2006; Crossetti and Bicudo, 2008). Thus, when a dam is built, the phytoplankton responds quickly to environmental changes resulting from the discontinuity of the river (Li et al., 2013). Several studies demonstrate that the dams disrupted the longitudinal connectivity in the river and altered the dynamics of water flow, nutrients, light availability, and biogeochemical processes present there. As a consequence, the structure and composition of biological communities may change, including phytoplanktonic communities (Nogueira et al., 2010; Li et al., 2013; Dos Santos et al., 2018). In other hand, some studies did not find a direct relationship between the phytoplankton community and the CRCC, associating the phytoplankton changes to the intrinsic features of the system (e.g., hydrodynamics, morphometry) (Bonilla, 1997; Silva et al., 2005). Even though investigations on the effects of river damming are abundant, there are still few studies that address the impact of river damming on the distribution of functional traits of phytoplankton upstream and downstream of dams in continuous systems (Da Silva et al., 2020; Graco-Roza et al., 2021; Pineda et al., 2022).
Traditionally, changes in the phytoplankton community have been investigated through community dynamics at the taxonomic level (e.g., species richness and abundance) (Nogueira et al., 2010; Perbiche-Neves et al., 2011). Nonetheless, alternative tools of measuring these responses, like those linked to the functional aspects of communities, are becoming increasingly used. This approach, often based on the use of functional traits of species as descriptors, more comprehensively indicates changes in ecosystem functions (Tilman, 2001; Petchey and Gaston, 2006; Violle et al., 2007; Cardinale et al., 2012; Hébert et al., 2017).
It is well known that phytoplankton is essential for some aquatic ecosystem functions like carbon uptake and primary production (Lewis, 2011; Naselli-Flores and Padisák, 2022). Functional traits namely size, cell arrangement, mixotrophy, presence of silica provide answers about the individual performance of species, likewise it provides information about population dynamics, community response mechanisms to changes in environmental conditions and trophic interactions. In this sense, traits can indicate fundamental properties of ecosystems, including primary production and energy transfer to other trophic levels (Tilman, 2001; Violle et al., 2007; Edwards et al., 2013; Hébert et al., 2017). However, the understanding on how environmental factors drive phytoplankton communities and traits is still a great challenge in aquatic ecology.
Considering that upstream and downstream inputs and outputs interconnect cascade reservoirs, here we aim to investigate whether the spatial variation was more representative than the temporal variation for the phytoplankton community for reservoirs inserted in the same environmental context (i.e., in the same stretch of a river). We hypothesize that: 1) Spatial variation is more explanatory than temporal variation for changes in phytoplankton structure and composition due to the cascading dilution effect. 2) The run-of-river SHPs and storage SHP reservoir have different taxonomic and functional diversity, since the damming of the river creates systems with different features that may directly influence phytoplankton.
Material and Methods
Study Area
The Paraibuna River is located across the states of Minas Gerais and Rio de Janeiro. It is one of the main tributaries of the Paraíba do Sul River, which plays a central role in the energy generation in Southeast Brazil (Da Silva and Zaidan, 2010). The Paraíba do Sul basin comprise one of the most developed industrial areas in the country, corresponding to around 10% of the national Gross Domestic Production (GDP). In addition, it supplies around 15 million people, where 87% of them live in metropolitan areas (Marengo and Alves, 2005).
The reservoirs of Monte Serrat (22° 1′ 11.207″ S - 43° 18′ 15.356″ W) and Bonfante (22° 0′ 44.716″ S - 43° 16′ 14.782″ W) were built in sequence on the final extension of the Paraibuna River, next to the cities of Comendador Levy Gasparian—RJ and Simão Pereira—MG, Brazil, with an approximate area of 0.14 and 0.21 km2, respectively. These two reservoirs are of the run-of-river type, with a small flooding area, low water retention time and use of the natural course of the river to generate energy, representing a semi-lotic environment. In the region of Três Rios—RJ, Brazil, the Paraibuna river is diverted to supply the Santa Fé reservoir, the last of the cascade (22° 3′ 32.943″ S - 43° 9′ 32.713″ W). This one has a large flooding area (2.05 km2) and, although its free spillway, it is considered a storage reservoir. Monte Serrat (MS) and Bonfante (BF) are quite similar reservoirs in terms of morphometry, with homogeneous flow dynamics (further details can be found in Supplementary Table S1, Supplementary Material I). Nonetheless, Santa Fé (SF) exhibits a different hydrodynamic, with greater depth, longer water residence time and thermal stratification throughout the year.
Sampling and Laboratory Analysis
The samplings were carried out quarterly (March/April; June/July; September and December) from 2013 to 2018, totaling 24 campaigns distributed in 4 sampling stations inside reservoirs (Figure 1). Due to the size of the SF reservoir, two sampling stations were established. Abiotic variables were sampled to characterize the reservoirs in time and space. In the field, the values of water temperature (temp, °C), conductivity (cond, µS.cm-1), pH, turbidity (turb, NTU) and dissolved oxygen (DO, mg. L−1) were measured with a multiparameter probe (YSI 556). Aliquots of water were collected at the respective points for further analysis of alkalinity (alk, mg. L−1), true color (TC, mg Pt. L−1), total hardness (TH, CaCO3 mg. L−1), total solids (TS, mg. L−1), dissolved solids (DS, mg. L−1) and suspended solids (SS, mg. L−1) (APHA, 2012). The analyzis of nutrients, such as silicates (Sil, mg. L−1), total phosphorus (TP, mg. L−1), orthophosphate (PO4, mg. L−1), nitrite (NO2, mg. L−1), nitrate (NO3, mg. L−1), ammonium (NH4, mg. L−1), soluble iron (SFe, mg. L−1) and total iron (TFe, mg. L−1) were performed according to the methodology proposed by APHA (2012). The molar ratio of nitrogen: phosphorus (N:P) of water was calculated as the sum of the molar concentrations (mmol. L−1) of the nitrogenous forms divided by the sum of the concentrations of the phosphorous forms. The flow data (m3s−1) were provided by the company Brasil PCH S.A. (Eletroriver S.A., BSB Energética S.A.).
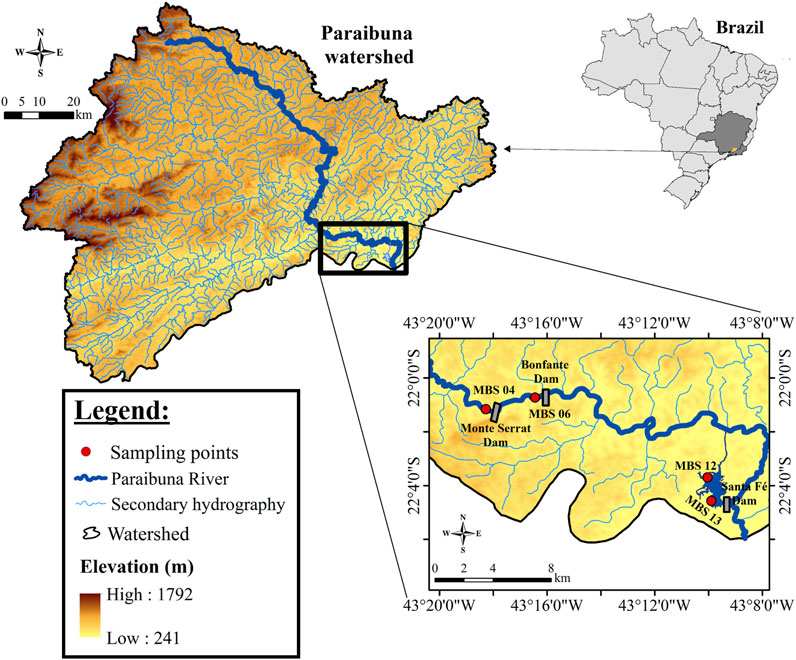
FIGURE 1. Map of the study area displaying the location of the three Small Hydroelectric Power Plant (SHP) reservoirs: Monte Serrat, Bonfante and Santa Fé along the Paraibuna River. The red dots represent the sampling stations: MBS04—Monte Serrat, MBS06—Bonfante, MBS12—Santa Fé I and MBS13—Santa Fé II.
For zooplankton community analysis, the samples were obtained from the filtration of 100 L of water through a plankton net (mesh size 68 μm) with the help of a bucket with 10 L capacity. The filtered material was fixed in 4% formalin solution and the zooplanktonic composition was evaluated under an optical microscope (Olympus BX40). Species richness was defined by the number of species present in each sample and species density was estimated by counting water aliquots in Sedgwick-Rafter chambers. Zooplankton was classified into major groups: Rotifera, Cladocera, Copepoda (adult and immature copepods - nauplii and copepodites).
Qualitative samples of the phytoplankton community were obtained using a plankton net (mesh size 25 µm) and fixed with Transeau’s solution. Quantitative samples were collected with a horizontal haul in the subsurface (∼0.3 m) and were subsequently conditioned and fixed with acetic Lugol’s solution. The counts followed the random field method for enumeration of cells and individuals using the Utermöhl sedimentation technique (Utermöhl, 1958) in an inverted light microscope (Olympus IX71). The phytoplankton community was identified into species level and the units (cells, colonies and filaments) were enumerated considering 100 individuals (Lund et al., 1958). Taxonomic identification was performed according to Hoek et al. (1995), Round et al. (1990), Komárek and Anagnostidis (1999), Komárek (2005) and Bicudo and Menezes (2006), considering the major groups: Bacillariophyceae, Cyanobacteria, Chlorophyceae, Euglenophyceae, Cryptophyceae, Zygnemaphyceae, Dinophyceae, Chrysophyceae, Raphidophyceae e Xanthophyceae.
After counting and identification, the phytoplankton community was classified according to their functional traits. Solely qualitative traits (presence/absence) were considered, being separated into three groups: 1) Morphological: mucilage and biological form (unicellular/colonial/coenobium//filament/chains); 2) Physiological: (a) silica demand, heterocysts, mixotrophy and toxin production; 3) Behavioral: flagella and aerotypes (Weithoff and Beisner, 2019). These traits were selected because they are related to survival strategies and energy efficiency, ecosystem functions that are of interest for this study. It is important to notice that the mixotrophy and toxin production traits were considered at potential levels, since we did not measure if organisms were producing toxins or performing a mixotrophic metabolism. Therefore, data from the literature were used to support this classification.
Data Analysis
To assess the total difference in environmental conditions of reservoirs, as well as the difference between the phytoplankton taxonomic and functional diversity, the nonparametric paired samples Wilcoxon test was performed. Reservoirs were compared pairwise ranking the medians and values of p < 0.05 were considered significant. Considering the great number of variables analyzed in this study, a Principal Component Analysis (PCA) [PCA function, R package FactoMineR (Husson et al., 2020)] was performed to identify the variables that better explained the spatial and temporal variations of the physical and chemical parameters of the system.
Zooplankton abundance was used as an explanatory variable for the phytoplankton community in the cascade reservoirs. Zooplankton and phytoplankton richness were estimated by the number of species per sample. Species diversity was calculated using the Shannon diversity index (H’) (Shannon and Weaver, 1963; Magurran and McGill, 2010). To verify the gradient of taxonomic variation of the phytoplankton community in time and space, a Non-metric Multidimensional Scaling (NMDS) was performed based on the Bray-Curtis similarity [metaMDS function, R package vegan (Oksanen et al., 2020)]. Then, a one-way Similarity Analysis was performed to verify the significant difference in phytoplankton abundance in time and space (ANOSIM) [anosim function, R package vegan (Oksanen et al., 2020)].
To investigate whether environmental factors were determinant for the composition of the major phytoplanktonic groups, a Redundancy Analysis was performed (RDA) [rda function, R package vegan (Oksanen et al., 2020]. The multicollinearity between the explanatory variables was verified through the Variance Inflation Factor (VIF) [vif.cca function, R package vegan (Oksanen et al., 2020]. Finally, the variables that entered the model were: water temperature + pH + turbidity + dissolved oxygen + dissolved solids + alkalinity + total hardness + ammonium (NH4) + nitrite (NO2) + nitrate (NO3) + orthophosphate (PO4) + silicates (SiO) + N:P ratio + flow.
To evaluate the correlation between the major phytoplankton and zooplankton groups, a Procrustes analysis was carried out [procrustes function, R package vegan (Oksanen et al., 2020)] to investigate the degree of agreement between these data sets (Gower, 1971). The degree of agreement between the ordination results was performed pairwise, where the Procrustes sum of squares and the Procrustes root mean squared error were considered. The significance of Procrustes analysis was verified with the PROTEST randomization test (9999 permutations; Jackson, 1995) [protest function, R package vegan (Oksanen et al., 2020)]. This analysis generates a statistic where 1 is a perfect agreement, and 0 is the complete absence of any agreement and an associated p-value (significant when p < 0.05).
The functional richness index (FRic) [dbFD function, R package FD (Laliberté et al., 2014)] was calculated for the phytoplankton community to estimate the amount of niche space occupied by the species within a community (Legras et al., 2018). To assess the phytoplankton functional composition in time and space, the CWM (Community Weighted Mean value; Pla et al., 2011) of the functional traits of the community was calculated and weighted by the relative abundance of the species [functcomp function, R package FD (Laliberté et al., 2014)]. The purpose of the CMW is to assess the contribution of community traits, taking into account characteristics of the entire community, not individual species. The relative importance of the phytoplankton traits was evaluated through the sum of the CWM values (total CWM). Nonparametric Spearman rank correlations were performed to test the correlation across species and functional richness, as well as Shannon diversity index and CWM, in order to compare the responses obtained through measures of taxonomic and functional diversity [cor.test function, R package stats (R Core Team and contributors worldwide, 2016)].
All analyzes were performed using R software version 4.0.5 (R Core Team, 2021) and JMP®Pro 14.0.0 software.
Results
Description of Environmental Variability
The environmental conditions presented a similar dynamic between Monte Serrat (MS) and Bonfante (BF) and between Santa Fé I e II (SF I and II) throughout the monitored period (2013–2018). The water temperature was higher in the downstream reservoir (SF I e II). Few pH peaks in the driest months (September 2014, 2015, and 2017) were observed in the last reservoir (SF I e II). Total and suspended solids, NH4, NO2, PO4, TP, and TFe presented higher values in MS and BF, indicating a potential dilution effect of the cascade. The other variables observed in this study did not reveal a pattern, exhibiting similar or random dynamics among all reservoirs.
Statistical differences were observed on the pH in MS and SF I, MS and SF II and BF and SF I (Table 1), and suspended solids in MS and SF II and BF and SF II (Table 1 and Supplementary Table S1, Supplementary Material II), and suspended solids in MS. Nutrients also exhibited differences among reservoirs, with lower concentrations in downstream reservoir (SF). Differences had been observed for NH4 in MS and SF I, MS and SF II, BF and SF I and BF and SF II (Table 1). For PO4, differences were found in MS and SF I, MS and SF II, BF and SF I and BF and SF II (Table 1), and for TP in MS and SF I, MS and SF II and BF and SF II (Table 1). Lastly, total iron (TFe) also exhibited differences between the reservoirs further upstream and downstream of the system. TFe presented differences in MS and SF II and BF and SF II (Table 1) and flow rate presented difference between MS and SF I and II (Table 1).
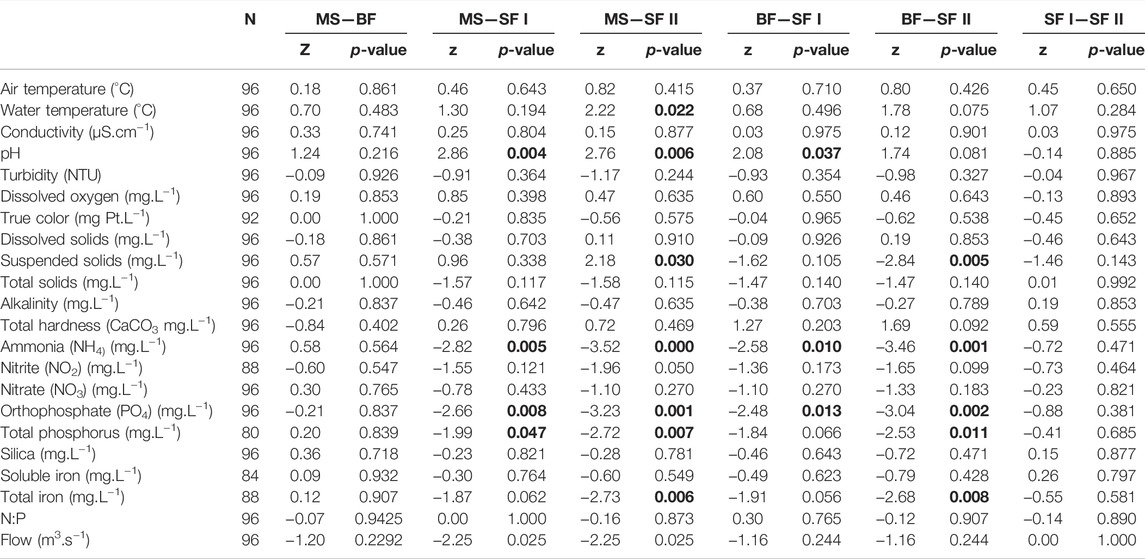
TABLE 1. Difference of physical and chemical characteristics of Monte Serrat (MS), Bonfante (BF) and Santa Fé (SF I and SF II) reservoirs. Wilcoxon signed-rank test was used for variables with non-normal distribution. Significant values (p < 0.05) are highlighted in bold.
The first two axes of Principal Component Analysis (PCA) explained 42% of the data variability (Figure 2). The first axis (28.3%) smooth summarized the spatial gradient, separating the run-of-river and storage reservoirs. It was noticed that MS and BF reservoirs were overlapped, as well as SF I and II, indicating a possible correlation between these sites. The variables that presented the most significant contribution to the distribution of data on the first axis were flow rate, suspended solids, turbidity, and TFe. The second axis (13.7%) also exhibited a spatial variation between run-of-river and storage reservoirs. Nevertheless, a seasonal trend was observed between dry (June/July and September) and rainy (December and March/April) periods. The variables with the most significant partial contribution to axis 2 were total and dissolved solids, NO2 and TP.
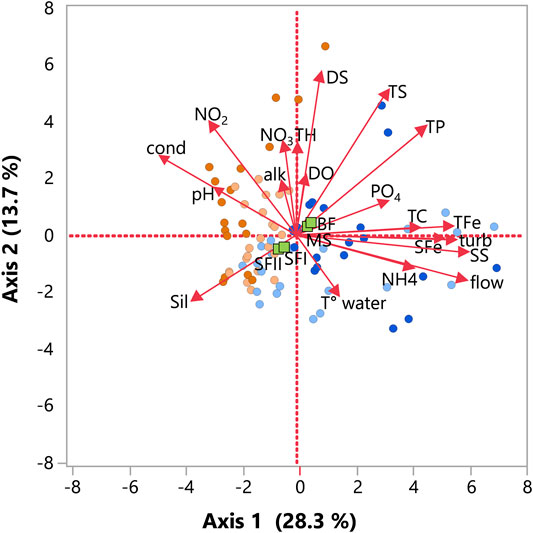
FIGURE 2. Principal component analysis (PCA) applied to investigate the spatial and temporal variation of environmental variables in the three Small Hydroelectric Power Plant (SHP) reservoirs: Monte Serrat (MS), Bonfante (BF) and Santa Fé (SF I e II) along the Paraibuna River (n = 96). The green squares represent the reservoirs and the colored dots represent the samples. The colors of the dots indicate the interannual periods, as follows: light blue = March, rainy season; dark blue = December, rainy season; light orange = June, dry season; dark orange = September, dry season. Variables are: T°_water, water temperature; NH4, ammonium, SS, suspended solids; DS, dissolved solids; TS, total solids; SFe, soluble iron; TFe, total iron; turb, water turbidity; TC, water true color; PO4, orthophosphate; TP, total phosphorus; TS, total solids; DO, dissolved oxygen; TH, total hardness; NO3, nitrate; NO2, nitrite; alk, alkalinity; cond, water conductivity; sil, silicates, pH and flow.
Zooplankton Community
The zooplankton community varied over time among the upstream reservoirs (MS and BF) and the downstream reservoir (SF) (Figure 3). In the upstream reservoirs (MS and BF) the dominant group was Rotifera. The total species richness in MS was 50 species, being 37 rotifers, 11 cladocerans and 2 adult copepods. The total density found throughout the sampled period was 34.6 ind. L−1 (28.5 ind. L−1 for rotifers, 1.99 ind. L−1 for cladocerans, 0.46 ind. L−1 for adult copepods and 3.65 ind. L−1 for immature copepods). In BF, the total richness was 45 species, being 36 rotifers, 7 cladocerans and 2 adult copepods. The total density was 31 ind. L−1 (18.71 ind. L−1 for rotifers, 3.59 ind. L−1 for cladocerans, 1.40 ind. L−1 for adult copepods, 7.30 ind. L−1 for immature copepods). The downstream reservoir (SF I and II) revealed a more diverse composition. SF I had a total richness of 79 species, being 54 rotifers, 20 cladocerans and 5 copepods. This site presented a total density of 3103.34 ind. L−1, evidenced by the dominance of rotifers (1281.99 ind. L−1) and immature copepods (1257.77 ind. L−1) and a meaningful contribution of cladocerans (389.33 ind. L−1) and copepods (174.24 ind. L−1) over the sampled period. In SF II the total richness was 88 species, being 58 rotifers, 24 cladocerans and 6 copepods. Although the higher species richness, the total density of SF II was lower than that of SF I, presenting a total density of 2695.1 ind. L−1, pointed by the dominance of immature copepods (1394.15 ind. L−1) and great contribution of rotifers (713.85 ind. L−1), cladocerans (241.55 ind. L−1) and adult copepods (345.54 ind. L−1) over the sampled period. Despite the difference in richness and density, the three main groups (Rotifera, Cladocera and Copepoda) were present in the three reservoirs throughout the sampled period, except December 2013 and 2017, when no group was found in BF.
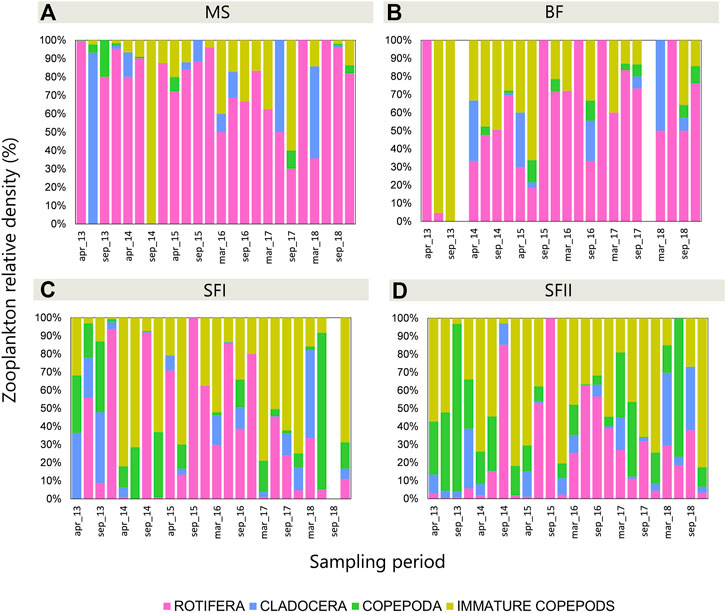
FIGURE 3. Zooplankton relative density (%) in the three Small Hydroelectric Power Plant (SHP) reservoirs: (A) Monte Serrat (MS; n = 24), (B) Bonfante (BF; n = 24), (C) Santa Fé I (SF I; n = 24) and (D) Santa Fé II (SF II; n = 24) sampled from 2013 to 2018.
Phytoplankton Community
The composition of the phytoplankton community also changed over time (Figure 4). In total, 217 taxa were identified. The structural change in the phytoplankton community was highlighted by three distinct periods in MS and BF: 1) dominance of diatoms (2013–2014); 2) dominance shift from diatoms to green algae and a significant increase in phytoplankton density (2015–2017); 3) change of dominance from green algae to diatoms and decrease of phytoplankton density (2018) (Figure 4). In SF (I and II) these periods were not clear, with seasonal density peaks in September (dry season), mainly in Chlorophyceae, Cyanobacteria, Chrysophyceae and Cryptophyceae groups. Non-metric multidimensional scaling (NMDS) analysis demonstrated that the reservoirs were overlapped throughout the sampled period (stress value: 0.1677), implying that the cascade reservoirs shared numerous species throughout the sampled period (Supplementary Figure S1, Supplementary Material I). The similarity analysis (ANOSIM) revealed that the communities were significantly similar, both in space (R = 0.12, p-value = 0.001) and time (R = 0.29, p-value = 0.001) (Supplementary Figures S2, S3, Supplementary Material I), corroborating the results found in the NMDS.
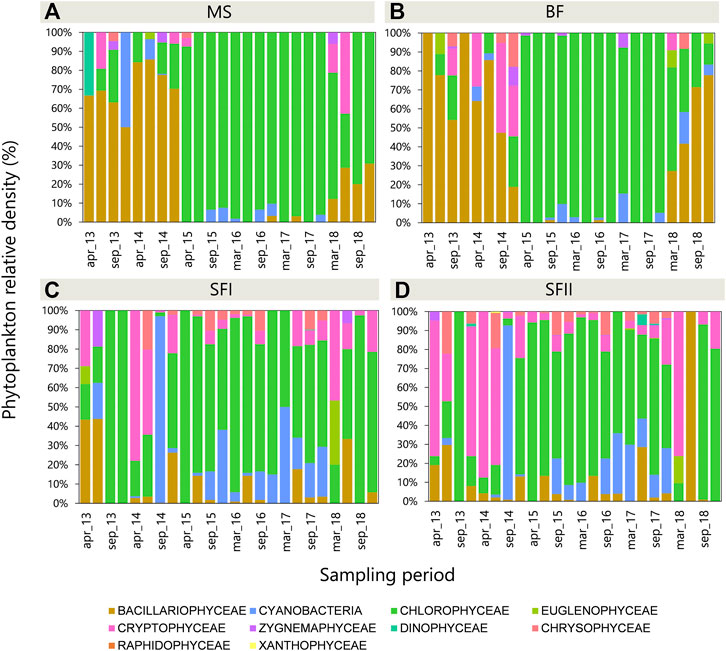
FIGURE 4. Phytoplankton relative density (%) in the three Small Hydroelectric Power Plant (SHP) reservoirs: (A) Monte Serrat (MS; n = 24), (B) Bonfante (BF; n = 24), (C) Santa Fé I (SF I; n = 24) and (D) Santa Fé II (SF II; n = 24) sampled from 2013 to 2018.
The phytoplankton species richness in the three systems ranged from 1 to 31, with a pronounced decrease in MS and BF reservoirs and an increase in SF during 2015–2017 (Figure 5A). In terms of functional richness, the same was not observed. (Figure 5B). Reservoirs maintained their functional richness ranging between 0 and 0.26, representing a small value. A similar trend in species richness was observed through the Shannon diversity index (H′) (Figure 6A). Species diversity (H′) was different among the upstream and downstream reservoirs (MS-BF and SF), mainly between the period from 2015 to 2017 (Figure 6A). Until the end of 2014, the species diversity oscillated between MS-BF and SF. From 2015, species diversity decreased in MS and BF, remaining so until 2017. In 2018, the species diversity of these reservoirs changed with the diversity of SF.
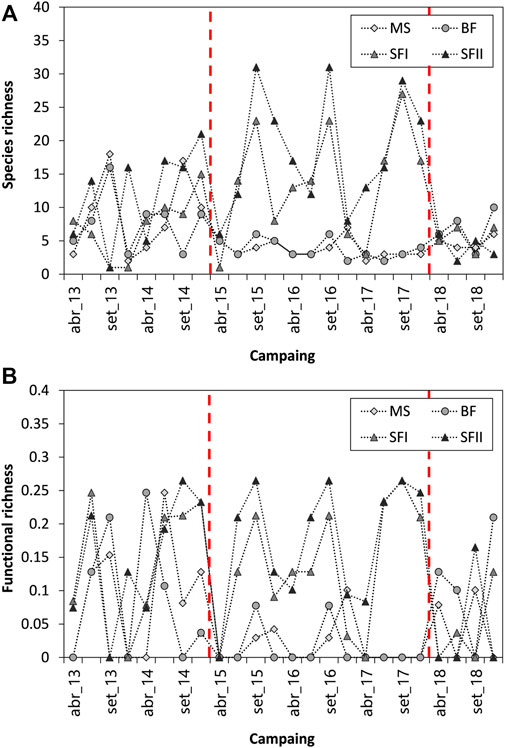
FIGURE 5. Temporal variation of phytoplankton (A) Species richness and (B) Functional richness (FRic) sampled from 2013 to 2018 in the three Small Hydroelectric Power Plant (SHP) reservoirs: Monte Serrat (MS; n = 24), Bonfante (BF; n = 24) and Santa Fé (SF I and II; n = 24). The red dashed lines indicate the periods of significant changes in the dominance of the main phytoplankton groups.
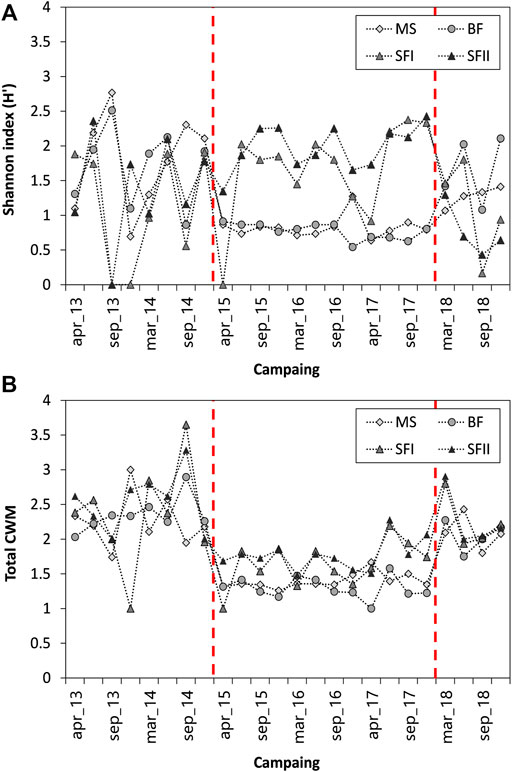
FIGURE 6. Temporal variation of (A) Shannon Diversity Index and (B) Community Weighted Mean (CWM) sampled from 2013 to 2018 in the three Small Hydroelectric Power Plant (SHP) reservoirs: Monte Serrat (MS; n = 24), Bonfante (BF; n = 24) and Santa Fé (SF I and II; n = 24). The red dashed lines indicate the periods of significant changes in the dominance of the main phytoplankton groups.
Redundancy analysis (RDA) explained only 30% of the species distribution related to environmental variables (axis 1: 0.22, F: 28.30, p-value: 0.001; axis 2: 0.08, F: 11.02, p-value: 0.017) and did not evidence spatial clustering related to the different environmental features (further details can be found in Supplementary Figures S4, S5; Supplementary Table S3, Supplementary Material I). Bacillariophyceae group was correlated with the flow. For Chlorophyceae, the most explanatory variable was dissolved oxygen. The other phytoplankton groups remained superimposed in the center of the two axes, indicating that there was no specific variable correlated with their distributions. Ordination patterns using the biological matrices (i.e., phytoplankton and zooplankton) of the systems were compared using Procrustes analysis. The results revealed that there was a significant correlation among the phytoplankton and zooplankton matrices (Procrustes, sum of squares: 0.46, correlation: 0.73, p-value: 9.999e-05). Nevertheless, few significant correlations among main groups were found in the reservoirs, namely: Chlorophyceae and Rotifera (SF I: r = 0.45, p-value = 0.026); Euglenophyceae and Rotifera (BF:, r = 0.47, p-value = 0.017); Euglenophyceae and Cladocera (BF: r = 0.56, p-value = 0.003; SF I: r = 0.84, p-value = <0.001; SF II: r = 0.44, p-value = 0.03); Cryptophyceae and Rotifera (SF I: r = 0.43, p-value = 0.034; SF II: r = 0.5, p-value = 0.011); Chrysophyceae and Rotifera (SF I: r = 0.65, p-value = <0.001; SF II: r = 0.64, p-value = <0.001); Zygnematophyceae and Rotifera (SF II: r = 0.60, p-value = 0.001); Dinophyceae and Copepoda (SF II: r = 0.44, p-value = 0.029).
The total CWM presented a similar trend for all reservoirs, with near values (Figure 6B). During the years 2015–2017, a decrease in the total contribution of traits was observed, which increased again in 2018 (Figure 6B). According to the total CWM (i.e., considering all reservoirs throughout the entire study period), the traits with the most significant contribution in the communities were: unicellular (single-cell arrangement), mixotrophy, presence of silica structure, mucilage and presence of flagellum (Figure 7).
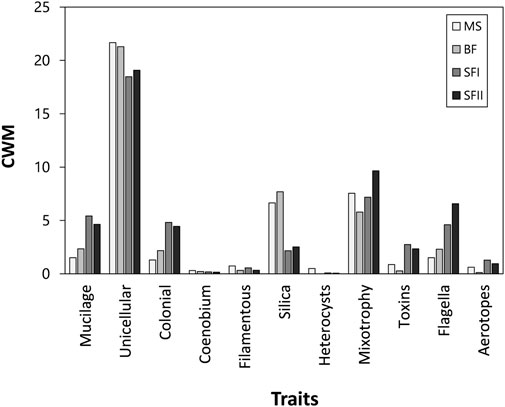
FIGURE 7. Total phytoplankton community trait values weighted by the abundance of species in that community (Community Weighted Mean—CWM), summarizing the most important functional traits in the structure of phytoplankton communities in the three Small Hydroelectric Power Plant (SHP) reservoirs: Monte Serrat (MS; n = 24), Bonfante (BF; n = 24) and Santa Fé (SF I and II; n = 24) sampled from 2013 to 2018.
Assuming the average among all samples per reservoir, statistical differences were observed in species richness in MS and SF I (z = 2.424, p-value: 0.015), MS and SF II (z = 3.405, p-value: 0.0007), BF and SF I (z = 2.282, p-value: 0.022) and BF and SF II (z = 3.369, p-value: 0.0008). The difference in functional richness was also observed in MS and SF I (z = 2.269, p-value: 0.023) and MS and SF II (z = 2.803, p-value: 0.005). Species diversity estimated using the Shannon diversity index revealed a difference in MS and SF II (z = 1.979, p-value: 0.047). Finally, the CWM differed among MS and SF II (z = 2.144, p-value: 0.032) and BF and SF II (z = 2.041, p-value: 0.041).
According to the correlation among species richness and functional richness, it has been found that when the number of species increased in the downstream reservoir (SF I and II), the functional richness also increased (SF I: r = 0.60, p-value = 0.009; SF II: r = 0.68, p-value = 0.001) (Figure 8A). However, functional richness reached a plateau when species richness approached 15 taxa. In the upstream reservoirs (MS and BF), this correlation between species richness and functional richness differed, being significant in the MS reservoir but not significant in the BF reservoir (MS: r = 0.66, p-value = 0.019; BF: r = 0.52, p-value = 0.126) (Figure 8A). In contrast, the correlation between the Shannon diversity index and the CWM was positive for the upstream reservoirs (MS: r = 0.48, p-value = 0.019; BF: r = 0.72, p-value = 0.000) (Figure 8B), indicating a trend between an increase in species diversity and an increase in the contribution of functional traits weighted by abundance. Nonetheless, it was not observed the same trend in the downstream reservoir (SF I: r = 0.08, p-value = 0.722; SF II: r = −0.22, p-value = 0.310) (Figure 8B).
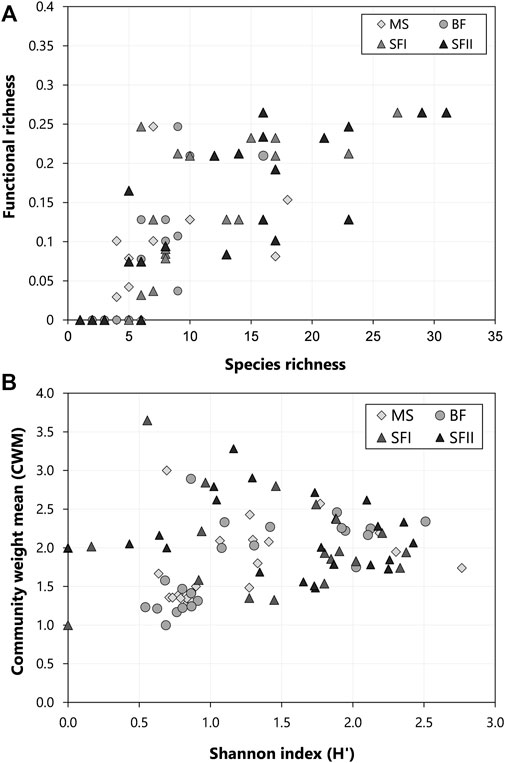
FIGURE 8. Relationship between phytoplankton taxonomic and functional diversity index sampled from 2013 to 2018 in the three Small Hydroelectric Power Plant (SHP) reservoirs: Monte Serrat (MS; n = 24), Bonfante (BF; n = 24) and Santa Fé (SF I and II; n = 24). (A) Species richness versus functional richness and (B) Shannon Index versus Total CWM.
Discussion
Our goal was to investigate whether the spatial variation would be more significant than the temporal variation for the functional and taxonomic diversity of phytoplankton in cascade reservoirs, located in the same stretch of the river. The hypothesis that the taxonomic and functional diversity of the phytoplankton community would be different between the reservoirs was accepted, since the upstream reservoirs (MS and BF) did not present differences. However, they were distinguished from SF, the last of the cascade. Notwithstanding, the hypothesis that spatial variation would be more explanatory than temporal variation for changes in phytoplankton structure and composition was rejected, since strong temporal and seasonal variation was observed in the three reservoirs. Our results revealed changes in the taxonomic and functional composition of phytoplankton over time in the different reservoirs and that the reservoir downstream of the system (SF) was the one with the highest species diversity and functional diversity. The cascade reservoir system also modified the dynamics of nutrients and suspended particles. These changes, however, have not been correlated with the structure and composition of the phytoplankton community, indicating that the chemical features of the system were not the main drivers of this community. Alternatively, phytoplankton was strongly coupled to the zooplankton matrix, indicating a possible agreement in the variation of these communities.
Influence of Reservoir Dynamics on Functional Traits
The first two reservoirs of the cascade (MS and BF) had a similar flow dynamic, mainly because they are run-of-river reservoirs and are next to each other. These systems presented a similar pattern in terms of environmental variables as well as species richness and functional diversity. In contrast, the storage reservoir and the last of the cascade (SF) was distinguished from the others. SF was different in terms of functional diversity and when compared in sequence, had a smooth reduction in the contribution of the traits unicellular (single-cell arrangement) and presence of silica. An increase in the contribution of the traits mixotrophy, flagella, mucilage and colonial arrangement was also observed, indicating a potential gradient of the contribution of functional traits along the cascade. The last reservoir of the cascade (SF) was influenced by the other reservoirs located upstream of the system. The dams of the other reservoirs usually act as physical barriers, retaining substantial amounts of the particulate material carried by the river (Barbosa et al., 1999). Furthermore, SF has a different nature from the others, such as depth and water retention time. These factors may directly interfere in the dynamics of the system (e.g., depth, photic zone, type of predominant metabolism, mineralization rates of organic matter) and consequently in the biological communities (Kimmel et al., 1990; Loken et al., 2018; Naselli-Flores et al., 2021).
In our study systems, the years 2013–2014 exhibited a dominance of diatoms, especially in run-of-river reservoirs. Diatoms are usually found associated with sediment or attached to some substrate (Martin-Jézéquel et al., 2000). Accordingly, the greater the depth of a system, the lower the probability of finding these species in surface waters, since they tend to sediment and to be limited by light availability (Smol and Stoermer, 2010). In this sense, we argue that this may have been the reason why this group was found in a smaller proportion in the storage reservoir. Run-of-river reservoirs are shallower and have a higher flow rate, which generates greater resuspension of the water column, creating a more favorable environment for the establishment of diatoms (Smol and Stoermer, 2010; Zorzal-Almeida et al., 2017a; Kuriqi et al., 2021). Furthermore, it is important to point out that the phytoplankton density, in general, was substantially lower during this time (2013–2014) than in the other years that followed (2015–2018). This result indicates that, in addition to the change in the presence of certain groups, the density of individuals also represented a change in terms of community structure and function (Bell and Kalf, 2001; Callieri, 2008). Unlike biomass, the increase in density only does not necessarily imply a higher rate of primary production or a greater energy flow through the trophic chain (Sterner et al., 2002). Still, the increase in density allows us to infer which species succeed in relation to the others, providing answers about the structure of the community.
The storage reservoir (SF) presented the highest species richness and diversity, and groups other than Chlorophyceae had a considerable contribution. In the run-of-river reservoirs, Chlorophyceae dominated and other phytoplanktonic groups were not able to establish themselves during 2015–2017. This result was also observed in the functional traits, since the functional richness decreased during this time.
Taxonomic Composition and Functional Traits
During 2015–2017, a change in phytoplanktonic composition was observed, with the Bacillariophyceae group being replaced by the Chlorophyceae group. The trait “unicellular” was the trait with the greatest contribution. Nevertheless, its contribution almost doubled compared to period of 2013–2014, mainly because of the dominance of a few unicellular species belonging to picoplankton (0.2–2 μm) and nanoplankton (2–20 μm), which had high abundances (e.g., Chlorella minutíssima, Chlorella vulgaris and Choricystis minor) (Table 2). It is important to notice that, although the unicellular trait has remained dominant, diatoms and green algae have different contributions to ecosystem functions due to their different sizes and shapes. Picoplankton and nanoplankton play an important role in increasing biomass and primary production in aquatic ecosystems (Stockner, 1988). Small cells have a greater surface area/volume ratio, that is, a greater membrane area per cell. Therefore, photosynthesis is enhanced by higher CO2 and nutrient diffusion rates (Raven and Kübler, 2002). Similarly, light absorption per cell volume might also be higher, representing a great advantage in oligotrophic systems, where nutrients limiting primary production, such as phosphorus (P) and nitrogen (N), are scarce (Sandgren, 1988). Although these small algae suffer little loss from sedimentation, as with diatoms, their increase in biomass is restricted by high predation rates (Callieri, 2008).
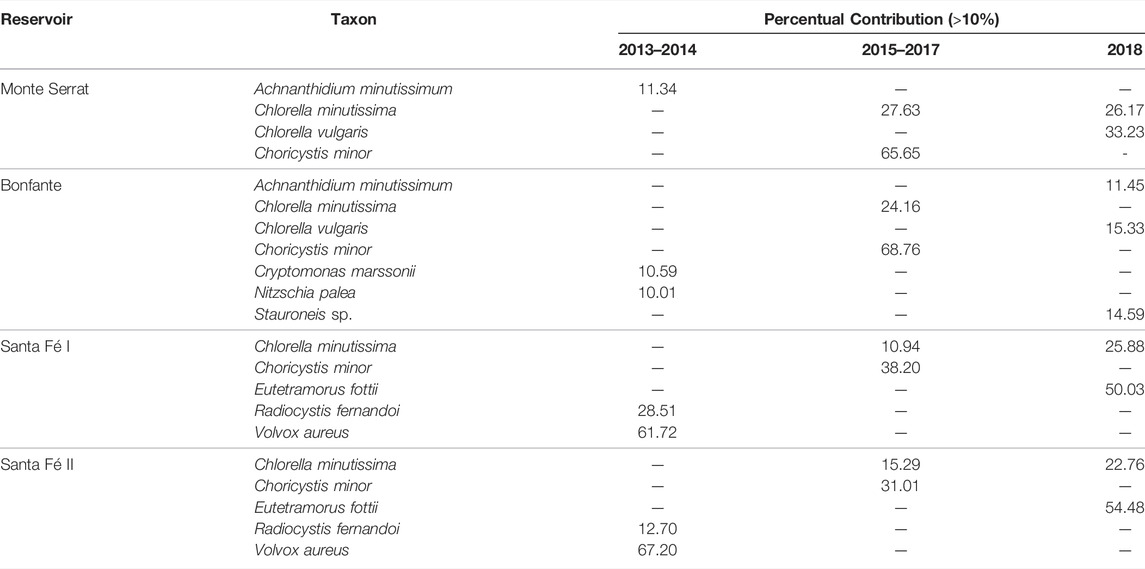
TABLE 2. Density contribution of the main phytoplankton species in the Monte Serrat, Bonfante and Santa Fé reservoirs, considering the periods between 2013 and 2014, 2015–2017 and 2018. Only contributions greater than 10% were considered.
The species found in this study, with small-sized cells, are species recognized as colonizers and are commonly the first to arrive and establish themselves in the environment (Reynolds, 2006). Its success is attributed to its morphological and reproductive characteristics, such as spherical or ellipsoidal floating, isolated or colonial cells involved in mucilage and quickly reproduction by simple cell division, without cell differentiation (Sandgren, 1988).
Furthermore, during this time, the trait “mixotrophy” had a notable contribution. Mixotrophy consists of the combination of different types of metabolism (Naselli-Flores and Barone, 2019). Through photosynthesis, algae incorporate inorganic carbon (CO2) and light energy. Through heterotrophy, some species are able to incorporate organic carbon through dissolved and/or particulate organic substrates (phagotrophy). In this way, the mixotrophic species use pools of organic nutrients, enhancing photosynthetic energy and primary production (Reynolds, 2006). Furthermore, they act at several trophic levels, which gives them a competitive advantage over photosynthetic or strict heterotrophic organisms (Sanders et al., 1990; Burkholder et al., 2008; Litchman and Klausmeier 2008). The factors that trigger mixotrophy as well as which species are potentially mixotrophic are still contradictory (Gerea et al., 2016). It is known, however, that light limitation and predator density are capable of triggering mixotrophic metabolism (Caron et al., 1990; Sanders et al., 1990; Jones et al., 1995). In addition, some species use mixotrophy only for nutritional complementation while others rely on mixotrophy to grow in complete darkness (Granéli et al., 1999).
Link Between Phytoplankton and Zooplankton Communities
Our results demonstrated that there were spatial and temporal changes in the environmental variables observed. However, these variables did not clearly contribute to explaining the structure of the phytoplankton community. We believe the main reason for these results was due to passive dispersion between connected reservoirs, promoting the homogenization of the local community and masking the effect of the environment (Zorzal-Almeida et al., 2017b). These results corroborate the findings of Bortolini et al. (2020) who identified differences between reservoirs and, in contrast, a similar composition of the phytoplankton community.
Since environmental features were not the main drivers of the phytoplankton community, as observed in Gameiro et al. (2007), Edwards et al. (2013) e Cardoso et al. (2017), the trophic relationships between phytoplankton and zooplankton became the alternative explanation to be considered, once it may control population dynamics and selection of phytoplankton species (Lehman and Sandgren, 1985; Sommer and Sommer, 2006). The interactions between phytoplankton and zooplankton play a crucial role in determining the relative contribution of primary production to other trophic levels (Sailley et al., 2015). Typically, zooplankton grazing is defined as being dependent on total prey concentration, with food selectivity expressed only as a function of prey size and abundance (Sailley et al., 2013). This behavior ignores the taxonomic preferences exhibited by predators and the ability of some species to actively select or reject an individual prey. Nevertheless, a study by Sailley et al. (2015), who sought to compare the impacts of zooplankton with passive and active selection, revealed that passive selection of zooplankton resulted in top-down prey control with a rapid exchange of nutrients. On the other hand, an active selection led to bottom-up control, with slower rotation of nutrients restricting primary production, shifting the system towards the export of particulate matter. Despite evidence on the inability of zooplankton to shape and control phytoplankton in the tropics (de Melo and Huszar, 2000; Von Rückert and Giani, 2008), cascade reservoirs compose complex systems (Nogueira et al., 2010). In this sense, phytoplankton might be controlled by physical processes in run-of-river reservoirs (lotic system), while biotic interactions would be more important in systems with high water retention time (lentic system) (Mineeva et al., 2008).
The Procrustes analysis between the phytoplankton and zooplankton communities revealed a strong relationship among these biological matrices. Still, this link between the change in phytoplankton and zooplankton composition was not clear, indicating that taxonomic and functional relationships are complex and often difficult to measure (Petchey and Gaston, 2006; Magurran and McGill, 2010; Inkpen et al., 2017). The upstream reservoirs (MS and BF) had a higher proportion of rotifers while the downstream reservoir (SF) had a higher proportion of copepods (adult and immature). Rotifers are passive filter, omnivores and generalist feeders, therefore have low food dependence on phytoplankton, mainly because they also prey on bacteria, flagellates and organic detritus (Arndt, 1993). Copepods, on the other hand, have greater food selectivity, using different active feeding strategies, with hunting and handling of their food (Lehman and Sandgren, 1985; DeMott, 1986; Sommer and Sommer, 2006; Kiørboe, 2011).
Phytoplankton is considered an extremely important environmental filter for determining the distribution of the zooplankton community (Vogt et al., 2013). Changes in phytoplankton quality and availability might reflect on zooplankton diversity, once it is closely related to resource use and supply (Ptacnik et al., 2008). Different species of phytoplankton have distinct chemical compositions (e.g., carbohydrates, lipids). Thus, the greater the species diversity, the greater the variability in the quality of carbon available for the other trophic levels (Gearing et al., 1984). The last reservoir of the cascade (SF) was the one with the greatest diversity of phytoplankton species. In other words, this reservoir presents a greater availability of food resources, thus justifying the difference in composition of the zooplanktonic community from rotifers to copepods.
Species Diversity Versus Functional Diversity
Here, the species and functional richness index revealed similar patterns over time, with the greatest differences related to the type of reservoir. A strong positive correlation between these two indices was observed (Figure 8A). At one point, although, the functional richness reached a plateau, indicating that the increase in the number of species did not necessarily represent a greater number of functional traits. Therefore, even if an environment has a high species richness, it is not necessarily performing additional ecosystem functions. At the sight of this, different species might express the same functional traits and can perform similar functions, characterizing what is well known as functional redundancy (Rosenfeld, 2002). It is important to emphasize that functional redundancy can generate stability to the ecosystem, once a given ecosystem function would not be restricted to just one or a few species. In this sense, even if determined species were lost, ecosystem processes would not be drastically affected. On the other hand, even if the species share the same functional traits, they can also occupy partially different niches, which would then characterize ecological complementarity and not redundancy (Loreau, 2000; Loreau and Hector 2001).
The correlation between Shannon diversity index and CWM was weak, indicating that species abundances are not closely related to their functional contributions in the studied system. Thus, even if some species showed high densities in a specific period (e.g., bloom), the functional diversity did not change substantially. Nevertheless, despite our results did not find a correlation, Spaak et al. (2017) demonstrated that an ecosystem function might be drastically altered when population density and community composition are pressured by the environment, even though richness remains the same. According to Petchey et al. (2004), communities with high functional diversity showed high rates of primary production and resource use efficiency, attributed to the effects of functional complementarity of species. On the other hand, communities with low functional diversity managed to maintain the ecosystem functions of the communities, which may be attributed to the effects of species selection (Smeti et al., 2019).
Conclusion
Our results highlight the complexity in understanding the role of cascading reservoirs in phytoplankton communities. The temporal variation observed in the three reservoirs proved to be an important factor, indicating that stochastic events over time had a great influence on the results. We point out that seasonal events were also observed, separating dry and wet seasons. Although, it was not possible to say that the spatial variation was the main drive for the change in the structure and composition of the phytoplankton community. The potential dilution effect predicted by the Cascading Reservoir Continuum Concept (CRCC) was observed, mainly in terms of nutrients and suspended particles. However, environmental variables were not able to predict changes in the phytoplankton community. A significant coupling between the phytoplankton and zooplankton communities was found and we believe that this group might have had an influence on the phytoplankton distribution along the longitudinal gradient of the river. Futhermore, changes in taxonomic and functional composition were observed mainly related to the hydrodynamics of the reservoirs. The run-of-river reservoirs presented lower species and functional diversity when compared to the storage reservoir. Ultimately, taxonomic and functional indexes exhibited a positive correlation, but this does not necessarily represent that taxonomic diversity is a good predictor of functional diversity. We emphasize that our results are important to deepen the knowledge about the temporal and spatial variation of phytoplankton in cascade reservoirs. Understanding how these processes act in communities is crucial for the conservation and management of aquatic ecosystems.
Data Availability Statement
The original contributions presented in the study are included in the article/Supplementary Material, further inquiries can be directed to the corresponding authors.
Author Contributions
NR conceived the idea, analyzed the data, and wrote the first draft of the manuscript. JS identified and quantified the phytoplankton species and commented on manuscript drafts. IJ contributed to the data analysis and commented on manuscript drafts. NB conceived the idea, coordinated the sampling, and commented on the manuscript drafts. SC conceived the idea, analyzed the data, and commented on all manuscript drafts.
Funding
The present study was partly funded by Brazil’s National Council for Scientific and Technological Development (CNPq), which provided a postgraduate grant (GM/GD 132507/2019-6) to NR. Further, NB is also partially supported by CNPq (Grant No. 316265/2021-7).
Conflict of Interest
The authors declare that the research was conducted in the absence of any commercial or financial relationships that could be construed as a potential conflict of interest.
Publisher’s Note
All claims expressed in this article are solely those of the authors and do not necessarily represent those of their affiliated organizations, or those of the publisher, the editors and the reviewers. Any product that may be evaluated in this article, or claim that may be made by its manufacturer, is not guaranteed or endorsed by the publisher.
Acknowledgments
The authors would like to thank José Reinaldo Paranaiba for creating the map of studied area and Gladson Marques for the support in field work and laboratory analysis.
Supplementary Material
The Supplementary Material for this article can be found online at: https://www.frontiersin.org/articles/10.3389/fenvs.2022.903180/full#supplementary-material
References
Almeida, R. M., Hamilton, S. K., Rosi, E. J., Arantes, J. D., Barros, N., Boemer, G., et al. (2019). Limnological Effects of a Large Amazonian Run-Of-River Dam on the Main River and Drowned Tributary Valleys. Sci. Rep. 9 (1), 1–11. doi:10.1038/s41598-019-53060-1
ANEEL – Agência Nacional de Energia Elétrica (2020). Resolução normativa N° 875, de 10 de março de 2020. Estabelece os requisitos e procedimentos necessários à aprovação dos Estudos de Inventário Hidrelétrico de bacias hidrográficas. Available at: <http://www2.aneel.gov.br/cedoc/ren2020875.pdf> (Accessed February 13, 2022).
APHA - American Public Health Association (2012). “Water Pollution Control Federation, … Water Environment Federation,” in Standard Methods for the Examination of Water and Wastewater. Editors W. C. Lipps, T. E. Baxter, and E. Braun-Howland (Washington, DC: APHA Press).
Arndt, H. (1993). “Rotifers as Predators on Components of the Microbial Web (Bacteria, Heterotrophic Flagellates, Ciliates) - a Review,” in Rotifer Symposium VI (Dordrecht: Springer), 231–246. doi:10.1007/978-94-011-1606-0_31
Barbosa, F. A. R., Padisák, J., Espíndola, E. L. G., Borics, G., and Rocha, O. (1999). The Cascading Reservoir Continuum Concept (CRCC) and its Application to the River Tietê-Basin, São Paulo State, Brazil. doi:10.1590/S1519-69842013000200009
Bell, T., and Kalff, J. (2001). The Contribution of Picophytoplankton in Marine and Freshwater Systems of Different Trophic Status and Depth. Limnol. Oceanogr. 46 (5), 1243–1248. doi:10.4319/lo.2001.46.5.1243
L. Berga, J. M. Buil, E. Bofill, J. C. De Cea, J. G. Perez, G. Mañuecoet al. (Editors) (2006). Dams and Reservoirs, Societies and Environment in the 21st Century (Taylor & Francis). ISBN: 9780429173523.
Bicudo, C. D. M., and Menezes, M. (2006). Gêneros de algas de águas continentais do Brasil. Rima: São Carlos. ISBN: 857656064X.
Bonilla, S. (1997). Composición y abundancia fitoplanctónica de tres embalses en cadena sobre el Río Negro, Uruguay. Iheringia, Ser. Bot. 49, 47–61. doi:10.23818/limn.22.07
Bortolini, J. C., da Silva, P. R. L., Baumgartner, G., and Bueno, N. C. (2020). Environmental Filters Influencing Phytoplankton Taxonomic Structure in Cascade Reservoirs. Braz. J. Bot. 43 (2), 405–412. doi:10.1007/s40415-020-00604-9
Burkholder, J. M., Glibert, P. M., and Skelton, H. M. (2008). Mixotrophy, a Major Mode of Nutrition for Harmful Algal Species in Eutrophic Waters. Harmful algae. 8 (1), 77–93. doi:10.1016/j.hal.2008.08.010
Callieri, C. (2008). Picophytoplankton in Freshwater Ecosystems: the Importance of Small-Sized Phototrophs. Freshw. Rev. 1 (1), 1–28. doi:10.1608/FRJ-1.1.1
Cardinale, B. J., Duffy, J. E., Gonzalez, A., Hooper, D. U., Perrings, C., Venail, P., et al. (2012). Biodiversity Loss and its Impact on Humanity. Nature 486 (7401), 59–67. doi:10.1038/nature11148
Cardoso, S. J., Nabout, J. C., Farjalla, V. F., Lopes, P. M., Bozelli, R. L., Huszar, V. L. M., et al. (2017). Environmental Factors Driving Phytoplankton Taxonomic and Functional Diversity in Amazonian Floodplain Lakes. Hydrobiologia 802 (1), 115–130. doi:10.1007/s10750-017-3244-x
Caron, D. A., Porter, K. G., and Sanders, R. W. (1990). Carbon, Nitrogen, and Phosphorus Budgets for the Mixotrophic Phytoflagellate Poterioochromonas Malhamensis (Chrysophyceae) during Bacterial Ingestion. Limnol. Oceanogr. 35 (2), 433–443. doi:10.4319/lo.1990.35.2.0433
Couto, T. B., and Olden, J. D. (2018). Global Proliferation of Small Hydropower Plants - Science and Policy. Front. Ecol. Environ. 16 (2), 91–100. doi:10.1002/fee.1746
Crossetti, L. O., and Bicudo, C. E. d. M. (2008). Phytoplankton as a Monitoring Tool in a Tropical Urban Shallow Reservoir (Garças Pond): the Assemblage Index Application. Hydrobiologia 610 (1), 161–173. doi:10.1007/s10750-008-9431-z
Csiki, S., and Rhoads, B. L. (2010). Hydraulic and Geomorphological Effects of Run-Of-River Dams. Prog. Phys. Geogr. Earth Environ. 34 (6), 755–780. doi:10.1177/0309133310369435
Da Silva, A. C., and Zaidan, R. T. (2010). Bacia Hidrográfica Do Rio Paraibuna: O Zoneamento Do Uso e Cobertura da Terra por Imagens de Satélite como Subsídio para o Planejamento e a Gestão de Recursos. Periódico Eletrônico Fórum Ambient. Alta Paul. 6 (2), 124–140. doi:10.17271/1980082762201086
Da Silva, I. G., Pelicice, F. M., and Rodrigues, L. C. (2020). Loss of Phytoplankton Functional and Taxonomic Diversity Induced by River Regulation in a Large Tropical River. Hydrobiologia 847 (16), 3471–3485. doi:10.1007/s10750-020-04355-2
de Melo, S., and Huszar, V. L. M. (2000). Phytoplankton in an Amazonian Flood-Plain Lake (Lago Batata, Brasil): Diel Variation and Species Strategies. J. Plankton Res. 22 (1), 63–76. doi:10.1093/plankt/22.1.63
DeMott, W. R. (1986). The Role of Taste in Food Selection by Freshwater Zooplankton. Oecologia 69 (3), 334–340. doi:10.1007/BF00377053
Dos Santos, N. C. L., García-Berthou, E., Dias, J. D., Lopes, T. M., Affonso, I. D. P., Severi, W., et al. (2018). Cumulative Ecological Effects of a Neotropical Reservoir Cascade across Multiple Assemblages. Hydrobiologia 819 (1), 77–91. doi:10.1007/s10750-018-3630-z
Edwards, K. F., Litchman, E., and Klausmeier, C. A. (2013). Functional Traits Explain Phytoplankton Responses to Environmental Gradients across Lakes of the United States. Ecology 94 (7), 1626–1635. doi:10.1890/12-1459.1
Egré, D., and Milewski, J. C. (2002). The Diversity of Hydropower Projects. Energy Policy 30 (14), 1225–1230. doi:10.1016/S0301-4215(02)00083-6
Ferrareze, M., Casatti, L., and Nogueira, M. G. (2014). Spatial Heterogeneity Affecting Fish Fauna in Cascade Reservoirs of the Upper Paraná Basin, Brazil. Hydrobiologia 738 (1), 97–109. doi:10.1007/s10750-014-1922-5
Gameiro, C., Cartaxana, P., and Brotas, V. (2007). Environmental Drivers of Phytoplankton Distribution and Composition in Tagus Estuary, Portugal. Estuar. Coast. Shelf Sci. 75 (1-2), 21–34. doi:10.1016/j.ecss.2007.05.014
Gearing, J. N., Gearing, P. J., Rudnick, D. T., Requejo, A. G., and Hutchins, M. J. (1984). Isotopic Variability of Organic Carbon in a Phytoplankton-Based, Temperate Estuary. Geochimica Cosmochimica Acta 48 (5), 1089–1098. doi:10.1016/0016-7037(84)90199-6
Gerea, M., Saad, J., Izaguirre, I., Queimaliños, C., Gasol, J., and Unrein, F. (2016). Presence, Abundance and Bacterivory of the Mixotrophic Algae Pseudopedinella (Dictyochophyceae) in Freshwater Environments. Aquat. Microb. Ecol. 76 (3), 219–232. doi:10.3354/ame01780
Gomes, M., Perbiche-Neves, G., and O. Naliato, D. A. (2012). “Limnology of Two Contrasting Hydroelectric Reservoirs (Storage and Run-Of-River) in Southeast Brazil,” in HS BOROUGENI, Org. Hydropower: Practice and Application (Rijeka: INTECH), 167–184. doi:10.5772/31829
Gower, J. C. (1971). Statistical Methods of Comparing Different Multivariate Analyses of the Same Data. Mathematics in the Archaeological and Historical Sciences, 138–149.
Graco-Roza, C., Soininen, J., Corrêa, G., Pacheco, F. S., Miranda, M., Domingos, P., et al. (2021). Functional rather Than Taxonomic Diversity Reveals Changes in the Phytoplankton Community of a Large Dammed River. Ecol. Indic. 121, 107048. doi:10.1016/j.ecolind.2020.107048
Granéli, E., Carlsson, P., and Legrand, C. (1999). The Role of C, N and P in Dissolved and Particulate Organic Matter as a Nutrient Source for Phytoplankton Growth, Including Toxic Species. Aquat. Ecol. 33 (1), 17–27. doi:10.1023/A:1009925515059
Hébert, M.-P., Beisner, B. E., and Maranger, R. (2017). Linking Zooplankton Communities to Ecosystem Functioning: toward an Effect-Trait Framework. J. Plankton Res. 39 (1), 3–12. doi:10.1093/plankt/fbw068
Hoek, C., Mann, D., Jahns, H. M., and Jahns, M. (1995). Algae: An Introduction to Phycology. Cambridge: Cambridge University Press. ISBN: 0 521 30419 9.
Husson, F., Josse, J., Le, S., Mazet, J., and Husson, M. F. (2020). Package ‘FactoMineR’. Multivariate Exploratory Data Analysis and Data Mining. R package version 2.4.
Inkpen, S. A., Douglas, G. M., Brunet, T. D. P., Leuschen, K., Doolittle, W. F., and Langille, M. G. I. (2017). The Coupling of Taxonomy and Function in Microbiomes. Biol. Philos. 32 (6), 1225–1243. doi:10.7554/eLife.4762610.1007/s10539-017-9602-2
Jackson, D. A. (1995). Protest: A PROcrustean Randomization TEST of Community Environment Concordance. Ecoscience 2 (3), 297–303. doi:10.1080/11956860.1995.11682297
Jones, H. L. J., Durjun, P., Leadbeater, B. S. C., and Green, J. C. (1995). The Relationship between Photoacclimation and Phagotrophy with Respect to Chlorophyll a, Carbon and Nitrogen Content, and Cell Size of Chrysochromulina Brevifilum (Prymnesiophyceae). Phycologia 34 (2), 128–134. doi:10.2216/i0031-8884-34-2-128.1
Kimmel, B. L., Lind, O. T., and Paulson, L. J. (1990). “Reservoir Primary Production,” in Reservoir Limnology: Ecological Perspectives (New York: Wiley), 133–193.
Kiørboe, T. (2011). How Zooplankton Feed: Mechanisms, Traits and Trade-Offs. Biol. Rev. 86 (2), 311–339. doi:10.1111/j.1469-185X.2010.00148.x
Komárek, J., and Anagnostidis, K. (1999). Cyanoprokaryota 1. Teil: Chroococcales. Stuttgart: Fisher. Süßwasserflora von Mitteleuropa 19/1.
Komárek, J. (2005). “Cyanoprokaryota 2. Teil/2nd Part: Oscillatoriales,” in Susswasserflora von Mitteleuropa (München: Elsevier GmbH), 19, 1–759.
Kuriqi, A., Pinheiro, A. N., Sordo-Ward, A., Bejarano, M. D., and Garrote, L. (2021). Ecological Impacts of Run-Of-River Hydropower Plants-Current Status and Future Prospects on the Brink of Energy Transition. Renew. Sustain. Energy Rev. 142, 110833. doi:10.1016/j.rser.2021.110833
Laliberté, E., Legendre, P., Shipley, B., and Laliberté, M. E. (2014). Package ‘FD’. Measuring Functional Diversity from Multiple Traits, and Other Tools for Functional Ecology. R package version 1.0-12.
Legras, G., Loiseau, N., and Gaertner, J.-C. (2018). Functional Richness: Overview of Indices and Underlying Concepts. Acta Oecol. 87, 34–44. doi:10.1016/j.actao.2018.02.007
Lehman, J. T., and Sandgren, C. D. (1985). Species-specific Rates of Growth and Grazing Loss Among Freshwater Algae1. Limnol. Oceanogr. 30 (1), 34–46. doi:10.4319/lo.1985.30.1.0034
Lehner, B., Liermann, C. R., Revenga, C., Vörösmarty, C., Fekete, B., Crouzet, P., et al. (2011). High‐resolution Mapping of the World's Reservoirs and Dams for Sustainable River‐flow Management. Front. Ecol. Environ. 9 (9), 494–502. doi:10.1890/100125
Lewis, W. (2011). Global Primary Production of Lakes: 19th Baldi Memorial Lecture. Iw 1 (1), 1–28. doi:10.5268/IW-1.1.384
Li, J., Dong, S., Liu, S., Yang, Z., Peng, M., and Zhao, C. (2013). Effects of Cascading Hydropower Dams on the Composition, Biomass and Biological Integrity of Phytoplankton Assemblages in the Middle Lancang-Mekong River. Ecol. Eng. 60, 316–324. doi:10.1016/j.ecoleng.2013.07.029
Litchman, E., and Klausmeier, C. A. (2008). Trait-based Community Ecology of Phytoplankton. Annu. Rev. Ecol. Evol. Syst. 39, 615–639. doi:10.1146/annurev.ecolsys.39.110707.173549
Loken, L. C., Crawford, J. T., Dornblaser, M. M., Striegl, R. G., Houser, J. N., Turner, P. A., et al. (2018). Limited Nitrate Retention Capacity in the Upper Mississippi River. Environ. Res. Lett. 13 (7), 074030. doi:10.1088/1748-9326/aacd51
Loreau, M. (2000). Biodiversity and Ecosystem Functioning: Recent Theoretical Advances. Oikos 91 (1), 3–17. doi:10.1034/j.1600-0706.2000.910101.x
Loreau, M., and Hector, A. (2001). Partitioning Selection and Complementarity in Biodiversity Experiments. Nature 412 (6842), 72–76. doi:10.1038/35083573
Lund, J. W. G., Kipling, C., and Le Cren, E. D. (1958). The Inverted Microscope Method of Estimating Algal Numbers and the Statistical Basis of Estimations by Counting. Hydrobiologia 11 (2), 143–170. doi:10.1007/BF00007865
A. E. Magurran, and B. J. McGill (Editors) (2010). Biological Diversity: Frontiers in Measurement and Assessment (Oxford, United Kingdom: OUP Oxford). ISBN: 9780199580675.
Marengo, J. A., and Alves, L. M. (2005). Tendências hidrológicas da bacia Do rio Paraíba Do Sul. Rev. Bras. Meteorol. 20 (2), 215–226.
Martin-Jezequel, V., Hildebrand, M., and Brzezinski, M. A. (2000). Silicon Metabolism in Diatoms: Implications for Growth. J. Phycol. 36 (5), 821–840. doi:10.1046/j.1529-8817.2000.00019.x
Mineeva, N. M., Litvinov, A. S., Stepanova, I. E., and Kochetkova, M. Y. (2008). Chlorophyll Content and Factors Affecting its Spatial Distribution in the Middle Volga Reservoirs. Inland Water Biol. 1 (1), 64–72. doi:10.1007/s12212-008-1010-5
Naselli-Flores, L., and Barone, R. (2019). Mixotrophic Phytoplankton Dynamics in a Shallow Mediterranean Water Body: How to Make a Virtue Out of Necessity. Hydrobiologia 831 (1), 33–41. doi:10.1007/s10750-018-3507-1
Naselli-Flores, L., and Padisák, J. (2022). “Ecosystem Services provided by Marine and Freshwater Phytoplankton,” in Hydrobiologia, 1–16. doi:10.1007/s10750-022-04795-y
Naselli-Flores, L., Zohary, T., and Padisák, J. (2021). Life in Suspension and its Impact on Phytoplankton Morphology: an Homage to Colin S. Reynolds. Hydrobiologia 848 (1), 7–30. doi:10.1007/s10750-020-04217-x
Nogueira, M., Ferrareze, M., Moreira, M., and Gouvêa, R. (2010). Phytoplankton Assemblages in a Reservoir Cascade of a Large Tropical - Subtropical River (SE, Brazil). Braz. J. Biol. 70 (3), 781–793. doi:10.1590/S1519-69842010000400009
Oksanen, J., Blanchet, F. G., Kindt, R., Legendre, P., Minchin, P. R., O’hara, R. B., et al. (2020). Package ‘vegan’. Community Ecology Package. R package version 2.5-7.
Padisák, J., Borics, G., Grigorszky, I., and Soróczki-Pintér, É. (2006). Use of Phytoplankton Assemblages for Monitoring Ecological Status of Lakes within the Water Framework Directive: the Assemblage Index. Hydrobiologia 553 (1), 1–14. doi:10.1007/s10750-005-1393-9
Perbiche-Neves, G., Ferreira, R. A. R., and Nogueira, M. G. (2011). Phytoplankton Structure in Two Contrasting Cascade Reservoirs (Paranapanema River, Southeast Brazil). Biologia 66 (6), 967–976. doi:10.2478/s11756-011-0107-1
Petchey, O. L., and Gaston, K. J. (2006). Functional Diversity: Back to Basics and Looking Forward. Ecol. Lett. 9 (6), 741–758. doi:10.1111/j.1461-0248.2006.00924.x
Petchey, O. L., Hector, A., and Gaston, K. J. (2004). How Do Different Measures of Functional Diversity Perform? Ecology 85 (3), 847–857. doi:10.1890/03-0226
Pineda, A., Bortolini, J. C., and Rodrigues, L. C. (2022). Effects of Space and Environment on Phytoplankton Distribution in Subtropical Reservoirs Depend on Functional Features of the Species. Aquat. Sci. 84 (1), 1–13. doi:10.1007/s00027-021-00837-0
Pla, L., Casanoves, F., and Di Rienzo, J. (2011). Quantifying Functional Biodiversity. Dordrecht: Springer Science & Business Media. doi:10.1007/978-94-007-2648-2
Ptacnik, R., Solimini, A. G., Andersen, T., Tamminen, T., Brettum, P., Lepistö, L., et al. (2008). Diversity Predicts Stability and Resource Use Efficiency in Natural Phytoplankton Communities. Proc. Natl. Acad. Sci. U.S.A. 105 (13), 5134–5138. doi:10.1073/pnas.0708328105
Quintana, X. D., Arim, M., Badosa, A., Blanco, J. M., Boix, D., Brucet, S., et al. (2015). Predation and Competition Effects on the Size Diversity of Aquatic Communities. Aquat. Sci. 77 (1), 45–57. doi:10.1007/s00027-014-0368-1
R Core Team and contributors worldwide (2016). Package ‘stats’. Community Ecology Package. R package version 3.3.1.
R Core Team (2021). R: A Language and Environment for Statistical Computing. Vienna: R Foundation for Statistical Computing. R version 4.0.5. Available in: <https://www.R-project.org> (Accessed on March, 2022).
Raven, J. A., and Kubler, J. E. (2002). New Light on the Scaling of Metabolic Rate with the Size of Algae. J. Phycol. 38 (1), 11–16. doi:10.1046/j.1529-8817.2002.01125.x
Reynolds, C. S. (2006). The Ecology of Phytoplankton. Cambridge: Cambridge University Press. doi:10.1017/CBO9780511542145
Rosenfeld, J. S. (2002). Functional Redundancy in Ecology and Conservation. Oikos 98 (1), 156–162. doi:10.1034/j.1600-0706.2002.980116.x
Round, F. E., Crawford, R. M., and Mann, D. G. (1990). F.E. Round, R.M. Crawford & D.G. Mann the Diatoms: Biology and Morphology of the Genera, Ix, 747p. Cambridge University Press, 1990. Price £125.00. J. Mar. Biol. Ass. 70, 924. Diatoms: biology and morphology of the genera. Cambridge university press. doi:10.1017/S0025315400059245
Sailley, S. F., Polimene, L., Mitra, A., Atkinson, A., and Allen, J. I. (2015). Impact of Zooplankton Food Selectivity on Plankton Dynamics and Nutrient Cycling. J. Plankton Res. 37 (3), 519–529. doi:10.1093/plankt/fbv020
Sailley, S. F., Vogt, M., Doney, S. C., Aita, M. N., Bopp, L., Buitenhuis, E. T., et al. (2013). Comparing Food Web Structures and Dynamics across a Suite of Global Marine Ecosystem Models. Ecol. Model. 261-262, 43–57. doi:10.1016/j.ecolmodel.2013.04.006
Sanders, R. W., Porter, K. G., and Caron, D. A. (1990). Relationship between Phototrophy and Phagotrophy in the Mixotrophic chrysophytePoterioochromonas Malhamensis. Microb. Ecol. 19 (1), 97–109. doi:10.1007/BF02015056
Sandgren, C. D. (1988). Growth and Reproductive Strategies of Freshwater Phytoplankton. Cambridge: Cambridge University Press. ISBN: 0521327229.
Shannon, C. E., and Weaver, W. (1963). The Mathematical Theory of Communication. Urbana: Illinois University Press.
Silva, C. A. D., Train, S., and Rodrigues, L. C. (2005). Phytoplankton Assemblages in a Brazilian Subtropical Cascading Reservoir System. Hydrobiologia 537 (1), 99–109. doi:10.1007/s10750-004-2552-0
Smeti, E., von Schiller, D., Karaouzas, I., Laschou, S., Vardakas, L., Sabater, S., et al. (2019). Multiple Stressor Effects on Biodiversity and Ecosystem Functioning in a Mediterranean Temporary River. Sci. Total Environ. 647, 1179–1187. doi:10.1016/j.scitotenv.2018.08.105
Smith, W. S., Espíndola, E. L. G., and Rocha, O. (2014). Environmental Gradient in Reservoirs of the Medium and Low Tietê River: Limnological Differences through the Habitat Sequence. Acta Limnol. Bras. 26, 73–88. doi:10.1590/S2179-975X2014000100009
J. P. Smol, and E. F. Stoermer (Editors) (2010). The Diatoms: Applications for the Environmental and Earth Sciences (Cambridge: Cambridge University Press). ISBN: 9781107564961.
Sommer, U., and Sommer, F. (2006). Cladocerans versus Copepods: the Cause of Contrasting Top-Down Controls on Freshwater and Marine Phytoplankton. Oecologia 147 (2), 183–194. doi:10.1007/s00442-005-0320-0
Spaak, J. W., Baert, J. M., Baird, D. J., Eisenhauer, N., Maltby, L., Pomati, F., et al. (2017). Shifts of Community Composition and Population Density Substantially Affect Ecosystem Function Despite Invariant Richness. Ecol. Lett. 20 (10), 1315–1324. doi:10.1111/ele.12828
Sterner, R. W., Clasen, J., Lampert, W., and Weisse, T. (1998). Carbon:phosphorus Stoichiometry and Food Chain Production. Ecol. Lett. 1 (3), 146–150. doi:10.1046/j.1461-0248.1998.00030.x
Stockner, J. G. (1988). Phototrophic Picoplankton: an Overview from Marine and Freshwater Ecosystems. Limnol. Oceanogr. 33 (4part2), 765–775. doi:10.4319/LO.1988.33.4PART2.0765
Tilman, D. (2001). Functional Diversity. Encycl. Biodivers. 3 (1), 109–120. doi:10.1016/B0-12-226865-2/00132-2
Utermöhl, H. (1958). Zur Vervollkommnung der quantitativen Phytoplankton-Methodik. SIL Commun. 9 (1), 1–38. doi:10.1080/05384680.1958.11904091
Violle, C., Navas, M.-L., Vile, D., Kazakou, E., Fortunel, C., Hummel, I., et al. (2007). Let the Concept of Trait Be Functional!. Oikos 116 (5), 882–892. doi:10.1111/j.0030-1299.2007.15559.x
Vogt, R. J., Peres-Neto, P. R., and Beisner, B. E. (2013). Using Functional Traits to Investigate the Determinants of Crustacean Zooplankton Community Structure. Oikos 122 (12), 1700–1709. doi:10.1111/j.1600-0706.2013.00039.x
von Ruckert, G., and Giani, A. (2008). Biological Interactions in the Plankton Community of a Tropical Eutrophic Reservoir: Is the Phytoplankton Controlled by Zooplankton? J. Plankton Res. 30 (10), 1157–1168. doi:10.1093/plankt/fbn065
Ward, J. V., and Stanford, J. A. (1983). The Serial Discontinuity Concept of Lotic Ecosystems. Ann Arbor: Ann Arbor Science Publishers. ISBN: 978-0250406128.
Weithoff, G., and Beisner, B. E. (2019). Measures and Approaches in Trait-Based Phytoplankton Community Ecology - from Freshwater to Marine Ecosystems. Front. Mar. Sci. 6, 40. doi:10.3389/fmars.2019.00040
Zorzal-Almeida, S., Bini, L. M., and Bicudo, D. C. (2017a). Beta Diversity of Diatoms Is Driven by Environmental Heterogeneity, Spatial Extent and Productivity. Hydrobiologia 800 (1), 7–16. doi:10.1007/s10750-017-3117-3
Keywords: aquatic connectivity, functional richness, microalgae, run-of-river reservoir, small hydroelectric power plants
Citation: Resende NdS, Santos JBOd, Josué IIP, Barros NO and Cardoso SJ (2022) Comparing Spatio-Temporal Dynamics of Functional and Taxonomic Diversity of Phytoplankton Community in Tropical Cascading Reservoirs. Front. Environ. Sci. 10:903180. doi: 10.3389/fenvs.2022.903180
Received: 24 March 2022; Accepted: 13 June 2022;
Published: 13 July 2022.
Edited by:
Vinicius Fortes Farjalla, Federal University of Rio de Janeiro, BrazilReviewed by:
Marcelo Curtarelli, CERTI, BrazilElżbieta Zębek, University of Warmia and Mazury in Olsztyn, Poland
Copyright © 2022 Resende, Santos, Josué, Barros and Cardoso. This is an open-access article distributed under the terms of the Creative Commons Attribution License (CC BY). The use, distribution or reproduction in other forums is permitted, provided the original author(s) and the copyright owner(s) are credited and that the original publication in this journal is cited, in accordance with accepted academic practice. No use, distribution or reproduction is permitted which does not comply with these terms.
*Correspondence: Nathália da Silva Resende, bmF0aGFsaWEucy5yZXNlbmRlQGdtYWlsLmNvbQ==; Simone Jaqueline Cardoso, c2ltb25lLmpjYXJkb3NvQGdtYWlsLmNvbQ==