- Romanian Academy, Institute of National Economy, Bucharest, Romania
In our days, a growing attention is paid to explain the influence of innovation on recycling. While many studies on this subject have been carried out, it's still needed for more investigations on measuring the effect of innovation on recycling. This paper is dedicated to measuring the intensity of the innovation influence on the recycling within EU member states. The methodology follows the next steps: visualization of data used, determining the stationarity of the time series analyzed, developing a panel model for 28 countries, applying specific statistical tests in case of the two indicators selected. After analyzing the models that resulted and applying Hausman test, the authors concluded that the regression panel with fixed effects is appropriate for our research. Thus, it is possible to show that the influence of the innovation on recycling is moderate and not instantaneous because there is manifested a lag of 2 years. In addition, the Fixed Effects model allows highlighting the heterogeneity that is present among member states. In addition, the authors concluded that the membership of the Euro Area has a positive influence on recycling and on circular economy as well. The article has several originality aspects: it took into account criteria that are not discussed very often as membership of Euro area; it has developed a model that brings quantitative aspects to describe the influence of innovation on recycling, it highlighted the heterogeneity existing among EU member states. Future research direction would be to consider including in the model some other variables as eco-investment.
1 Introduction
The latest objectives and endeavors towards a sustainable development have led to the conceptual and political promotion of new concepts such as the green growth and circular economy, as a reaction to the global recession and climate change.
The model of green economic growth presents an alternative to the conventional economic paradigm of resource exploitation, and this theory of growth involves concepts such as the sustainable use of natural resources, including greater energy and resource efficiency and improved natural capital as drivers of growth. The circular economy (CE) means the recycling of resources used in products whose life-cycle has come to an end or which have lost their usefulness to construct new objects of the same quality or even better. It is obvious that the shift towards Circular Economy involves a systemic and radical change. The historic tradition perceived socio-economic paradigm of production and consumption as a linear process, based on “extracting, processing, manufacturing and disposing.” In today circumstances this paradigm needs to be radically transformed in order to fulfil the objectives of the Circular Economy, namely, eliminating or reducing disposable waste as much as possible. In these circumstances, recycling is one of the main drivers of Circular Economy (EC COM., 2015).
On the other hand, innovation will play a key part in the systemic change for the economy. In order to rethink our ways of producing and consuming, and transforming waste into high value-added products as well recovering the energy incorporated into various materials (aluminum, glass etc.) there are needed new technologies, processes, services and business models to shape the future of the economy.
The relationship innovation–circular economy was the subject of many papers and models. For instance, Horbach and Rammer (2020) focused their paper on modeling how companies react within the perspective of a circular economy. The two authors considered that companies are important players for the realization of a Circular Economy (CE). The paper investigated the link between CE and firm growth and whether higher sales and employment growth emerges at the cost of worsened productivity or financial performance. The authors have used a panel model at firm-level with data of 2 years (2014 and 2016) for German companies. It resulted that all firms in Germany reported CE innovations during 2012–2014. Due to high price of energy in Germany caused by extensive use of regenerable energies, the single most important innovation activity was the reduction of energy use per unit of output. In this context process-related innovations are more frequent than product-related ones. So, out of the three main types of recycling: mechanical, energy and chemical only reducing energy consumption was the main objective for innovation.
Pieroni et al. (2019) analyzed in their paper the innovation business models for circular economy and sustainability. They systematically analyzed literature and identified 94 publications and 92 approaches (including conceptual models, methods or tools). Main findings show that business model innovation for sustainability and circularity is still fragmented. Within the models, mentioned-above we face a lack of holistic approaches covering multiple stages of innovation. As well a stronger integration between circularity and sustainability is required. The authors have proposed a unifying agenda for future research.
The topics related to reasons for non-acceptance of circular business models is also present in some research papers. Patrik Planing (2015) explored the topic of innovation acceptance/non-acceptance with the aim to develop a new conceptual framework for business to model innovation in a circular economy. As well, the author explored the reasons for consumer non-adoption of circular business models. In this context, learning about consumer motives leading to non-adoption of eco-innovation is important for removing barriers that still are in place. The paper mentioned provides support for designing better and more successful circular business models.
Other researchers have studied the way in which the innovation in the production chain influences the advances towards CE (Potting et al., 2017). The study took into account product chains from the extraction of natural resources to waste elimination. Recovering materials from discarded waste or used products often requires large amounts of energy and associated costs in additional processing. In many cases mixing of materials when discarded reduces their quality which means that very often recycled (secondary) materials do not have the same qualities as virgin raw materials so cannot be used again for the same type of product. Frequently, these recycled materials do find an application in other product chains with lower quality requirements. In a circular economy, the recovery/reuse chains are dissimilar. It is assumed that, the materials recycled from a discarded product ideally retain their original quality so that they can be used again and again in a similar product without any addition in terms of material and energy. As a result of such a logic, in the recycling process no extra natural resources are needed to manufacture the same product. In this way, discarded products no longer become waste but a raw material for a new production cycle. This ultimate circularity, in which a product chain is closed because used materials can be used over and over again is aspirational and is probably not at all feasible in practice. In most cases it is needed a supplementary input of energy or other materials.
The transition from linear model of production to a circular model is a topic of peculiar importance. In his paper, Mentink (2014) analyzed the role of innovation in transition towards a Circular Business Model. The author affirmed that: “both 100% linear and 100% circular economies or business models do not exist due to practical limitations (friction, leakages, growth, energy losses etc.).” This affirmation is related to the fact that all production cycles do need an input of materials, work and energy. The author mentioned the risks or barriers to implement a circular business model. It is important to know that the complexity of organization and management often increases the need for new information related to recycled materials, components and products. As it is the reality for now, the current system of legislation, consumer behavior, financing, etc. is still to the advantage of the current dominant linear models so a transition is needed.
In general, recycling serves two important purposes: 1) avoiding landfilling and incinerating so helping to reduce soil, air and water pollution and 2) valuable materials like aluminum, metal, plastic and glass are reused in other forms and not wasted. In the case of several energy-intensive materials as aluminum and glass, significant energy saving is made.
As well, it should be mentioned that in our days, it is important to minimize the waste generated and reclaim residues, as much as possible, through effective recycling. Improving the effectiveness of recycling is important and could be carried out via measures taken in various stages of recycling: collection, sorting, storage, transport, and manufacturing etc.
In all stages, one major factor that helps improving recycling is innovation. It is quite obvious that there are some conditions to be fulfilled in order to persuade consumers to change their buying behavior in favor of recycled materials. The main conditions are: the recycled product should have comparable characteristics as the product made of virgin materials and, very important, should not cost more. These conditions are not easy fulfilled; however, innovation may be a key tool to achieve them (Frone, 2017).
Therefore, considering innovation would play a major role in the new development of the circular economy, the general objective of this research is to analyze the influence of innovation on recycling. The paper has three parts, as follows: the first part is dedicated to the review of the relevant literature regarding the relationship between recycling and innovation and the influence of some other variables such as membership of Euro area. In the second part we present the research methodology, including the data sources used, indicators and research hypotheses. The third part proposes an econometric model and analyses the outcomes obtained. Finally, the authors conclude regarding the findings resulted from the econometric analysis and present some policy recommendations.
Thus, the paper could fill the gap in modelling the relationship innovation-recycling by better understanding the quantitative aspects as well as highlighting the existing heterogeneity within EU.
The main aim of this paper is to bring a contribution to the discussions concerning the influence of innovation on the recycling process, measuring this influence by statistical evidence analysis.
The research questions of the paper are the following:
1) How important is the influence of innovation on recycling within EU?
2) What is the influence of Euro membership on recycling?
3) How important is heterogeneity among EU member states?
2 Materials and Methods
2.1 Literature Review
An important topic for science community was that of similarities and relationship between the notions sustainable development—circular economy. (Geissdoerfer et al., 2017) explained that the similarities and differences between both concepts are not so clear. So, the authors, after a special incursion in the existing literature, define the Circular Economy as: “a regenerative system in which resource input and waste, emission, and energy leakage are minimized by slowing, closing, and narrowing material and energy loops.” On the other hand, Sustainable Development is: “balanced integration of economic performance, social inclusiveness, and environmental resilience, to the benefit of current and future generations.” This is indeed a useful differentiation that can help understanding how is the Circular Economy conceptually related to sustainability. The authors found that Circular Economy is considered in many papers and research works as a condition for sustainability. As well, the beneficial relation between the two concepts can be structured into eight different relationships.
In this context, the relationship innovation—recycling was the subject of several papers and articles. For instance, a recent study (Sumrin et al., 2021) has explored major drivers. In many cases, new requirements of legislation for environmental protection and waste management have determined companies to adopt innovation as an instrument for achieving a competitive advantage. Packaging is one important area of waste management, in which firms are willing to adopt innovation. The article mentioned that irrespective of innovative technological advancement, expanding the number of global supply chains for various products has encouraged measures at the source as the utilization of smart packaging and related waste minimization solutions all along the supply process. It is well known that packaging recycling is complicated due to the multitude of packages involved but offers significant opportunities for innovation. The authors stated that innovation in packaging to facilitate recycling was not the main goal until recently. This is a new way to look at recycling innovation: taking measures at the source and not at the end of pipe. The reason for this is that recycling itself cannot cope with the entire amount of waste generated so it is important to take into account measures that could reduce at source the flow of waste. Thus, the authors consider important to better examine the innovation of packaging recycling from various perspectives such as technological capabilities, human and organizational capabilities, eco-design and innovation in packaging in order to increase its impact on waste recycling and prevention (Vence et al., 2019; Sumrin et al., 2021; Pieroni et al., 2019). The reality shows that innovations are needed more in order to minimize waste generation and to enhance the recycling process.
Other researchers have studied the way innovation influences advances in recycling (Potting et al., 2017) considering the product chains from extraction of natural resources to waste elimination for two product groups: plastic packaging and electrical and electronic equipment. Recovering materials from discarded waste or used products often requires large amounts of energy and labor so associated costs in additional processing could be significant. The conclusion of the paper highlighted a partial role allocated to technological innovation. The data shows that industrial innovation plays a role in economies that are in transitions and adopt recycling as a circularity strategy. The majority of innovations are found in a simpler form as modifications and adaptations of existing processing methods and technologies in order to meet some peculiar requirements. This procedure is known as incremental innovation (can have relatively low intensity changes in the economic system) as compared with radical innovation (can determine ample changes in the economic system by favoring the occurrence of new economic and social activities). Radical innovation is based on fundamentally new scientific knowledge. The study reveals that this form of disruptive innovation is scarcely found in the case studied.
From the research papers analyzed and other policy documents, we may report the importance of innovation in enhancing recycling. By developing innovation abilities and practices, it is possible to augment the commercial potential across all economic sectors. The second aspect worth mentioning is the fact that innovation contributes to reduce uncertainty about future market developments in EU (Pieroni et al., 2019). This will help boost investment and accelerate introduction of environmentally friendly technologies, products and services (Frone and Constantinescu, 2018).
In a real economy, the recovery/reuse chains are dissimilar. It is assumed ideally that materials recycled from a discarded product would retain their original quality so they may follow the same cycle repeatedly in a similar product without any addition in terms of materials and energy. Theoretically, in order to manufacture the same product, the recycling process would use no extra (or only a few) natural resources. This way, the discarded products no longer become waste but raw material for a new production cycle (Repp et al., 2021).
The idea regarding limits of recycling and indirect limits of circular economy is discussed by Korhonen J., et al. (Korhonen et al., 2018) in the paper Circular Economy: The Concept and its Limitations. The authors analyzed the concept of circular economy and discuss it from the perspective of environmental sustainability. In this framework, the authors identified six limits and barriers for the circular economy as thermodynamic limits, system boundary limits, rebound effect (Jevon’s paradox, boomerang effect), limits of governance and management, etc. The thermodynamic limit for CE is derived from the writings of Nicolae Georgescu-Roegen. The authors pointed out that, according to N. Georgescu-Roegen, recycling will always need external energy. In the process of recycling will be losses of energy and materials that dissipate into the environment and cannot be recovered or the effort to recover all these will be enormous, consuming more resources that can be recovered. The conclusion of the Entropy Law is that complete recycling is not possible so a limit for recycling would occur. The paper is important for drawing attention on challenges related to CE. Authors see these six limitations and challenges as research themes and project proposals for scientists.
Another interesting viewpoint of analysis is the regional feature of innovation in the EU. The evaluation of the regional innovation degree has assembled a series of indicators used for the analysis of the macroeconomic innovation level and statistical analysis techniques and econometric classical or modern methods. Innovation represented a priority of the various EU Strategies, while the regions strengthen their position of key-actors in the process of re-ascertaining economic and social cohesion at Community level. Therefore, the authors concluded that synergy is necessary between the support instruments corresponding to innovation and social cohesion at community and regional level (Antonescu, 2015).
In order to better gather the knowledge and dissipate it, the Eco-Innovation Observatory was created as a platform for the structured collection and analysis of an extensive range of eco-innovation information, collected from across the European Union. Doranova et al. (2016) analyzed the eco-innovation performance in EU country and discussed the progress towards circular economy of EU member states.
For our article, it is of interest the way in which innovation was scrutinized. The main indicator used was the Eco-Innovation Scoreboard (Eco-IS). This indicator explains eco-innovation performance across the EU Member States using scores and indicators. The scoreboard has a complex aim of apprehending the different characteristics of eco-innovation by using 16 sub-indicators grouped into five thematic groups:
• Inputs containing investments, financial or other resources, which aim at triggering eco-innovation activities;
• Direct activities, illustrating to what extent companies, in a specific country, are active involved in eco-innovation;
• Outputs, quantifying the outputs of eco-innovation activities in terms of patents, academic literature and media contributions;
• Resource efficiency results, interpreting eco-innovation performance in the context of a country’s resource efficiency and GHG emission intensity;
• Socio-economic outcomes, showing to what extent eco-innovation performance generates positive outcomes for social aspects (employment) and other economic aspects (turnover, exports).
The recent relevant interactive tool shows the results from the aggregated scoreboard for EU member states in 2019. In the current figured report, countries are clustered into three groups:
• Eco-innovation leaders, scoring Eco-IS significantly higher than the EU average; this group includes seven countries as Luxembourg (has the highest aggregate score—165), followed by Denmark, Finland (aggregate score 146 and 145), Sweden, Austria, Germany, and United Kingdom;
• Average eco-innovation performers are ten countries with scores around the EU average (aggregate score 100), ranging from 85 (Belgium) to 112 (Italy), also including: Netherlands, Spain, France, Portugal, etc.;
• Countries catching up in eco-innovation (Romania, Estonia, Poland, Greece, Malta etc.). There are eleven countries recording an aggregate score less than EU average (between 34 Bulgaria and 82 Lithuania).
In the paper Eco-Innovation: opportunities for advancing waste prevention, Rene van Berkel (Van Berkel, 2007) has discussed opportunities that can deliver waste prevention outcomes, broadly categorized as efficiency practices, design strategies and creativity templates. For instance, the efficiency practices could be expressed in several ways: cleaner production, waste minimization, eco-efficiency and pollution prevention. The author showed that in the industry case, efficiency improvements are related to minimizing inputs, reducing energy consumption, introducing new technologies. These actions have frequently demonstrated multiple environmental benefits coupled with commercial benefits. In this area there could be noted several positive aspects, such as: cost savings, enhanced process operability and/or better product quality (Van Berkel, 2007).
The importance of recycling in advancing circular economy is well known. A remarkable paper (Di Maio and Rem, 2015) has assigned a more robust indicator for promoting circular economy through recycling. The authors considered there was still lack of an effective key performance indicator for motivating the recycling industry. In order to solve this, the paper has proposed a new indicator named Circular Economy Index (CEI). The indicator would be calculated as the ratio of the material value produced by the recycler (market value) divided by the material value entering the recycling facility. Such an indicator could be useful but it is not clear how big would be the effort to alter the existing reporting system at the level or Eurostat and what would be the benefits of replacing the classic indicators of recycling.
Hysa E. et al. (Hysa et al., 2020) have examined the influence of several indicators on economic growth, measured by GDP. The goal was to identify the main factors, which are supportive of both sustainability and development. The authors have used a semi-log model including a panel data with fixed effects for 28 EU countries. As well, the authors included a dynamic panel of data computed using Arellano–Bond method. It is interesting to mention that the model used took into account five independent variables, such as a tax rate related to environment resources used, the recycling rate of waste, private investment and jobs, patents related to recycling and trade of recyclable materials. The results of both econometric models showed a positive correlation between selected indicators and GDP. That means all indicators have a positive influence on GDP. There could be mentioned one issue that was not solved yet by this model: the fact that some independent variables as taxes, trade, private investment and jobs are, by definition, constitutive parts of the GDP so the linkage should be positive anyhow. Another unsolved aspect of models with many independent variables is the occurrence of multicollinearity among respective variables, which implies some negative effects, such as the oversizing and/or wrong signs of estimated parameters, low Student test statistics, increasing confidence intervals etc.
The linkage among recycling and other economic indicators was analyzed also by Banacu et al. (2019). This paper discussed the implications of entrepreneurial innovation for recycling municipal waste and scrutinizes the main factors of recycling municipal waste at the European Union level. The model used was that of a linear regression to explore the influence of business expenditure on research and development (R&D), private investments, resource productivity, and environmental taxes on the recycling rate of municipal waste. The model included 27 European Union countries and a period of 8 years. In this case, the model has indicated that the business expenditure on R&D, private investments, R&D expenditures as a share of GDP and resource productivity have a direct and moderate impact on the municipal waste recycling. These four variables showed a positive and moderate effect mirrored by positive coefficients smaller than the unit. The variable Environmental Taxes showed a statistically significant effect but that value is negative meaning the impact on the waste recycling is adverse (the coefficient of that variable has a value of −0.187). This particular finding is opposed to general theory according to which environmental taxes may improve the recycling behavior of the population. In practice it is well known that the principle pays as you throw is the main instrument to reduce waste at the source so many countries have developed and implemented tariffs proportional with the quantity of waste generated.
It is important to note that some researchers (Camilleri, 2020) showed the role and status of policies and plans elaborated at EU level. These plans and policies bring an important help in diminishing the uncertainty faced by companies and population in promoting circular economy. On the opposite side, the author mentioned some of the possible challenges that could have a negative influence on the businesses’ push towards a more circular activity. The reality is that advancement toward the circular economic practices is not guarantee. In many cases circularity still prove to be difficult and challenging for some industrial branches. It is well known that there are many companies maintaining the existing status quo as they still rely on linear models (Camilleri, 2019; Camilleri, 2021).
In the next period, the recycling activity will become more important in EU. One reason is the new and very ambitious Circular Economy Package promoted by EU, which includes revised legislative proposals on waste. Some of the most important targets for recycling are as follows: a common EU target for recycling 65% of municipal waste by 2035 and a common EU target for recycling 70% of packaging waste by 2030. There could be mentioned also the ambitious recycling targets for specific packaging materials: paper and cardboard: 85%; glass: 75%; plastic: 55%; wood: 30%. Also, the European Green Deal aims at revising some of these targets, in order to facilitate circularity.
2.2 Methodology and Data Sources; Theoretical Background
2.2.1 Theoretical Background
In order to detect the influence of innovation on recycling in EU member states, we used a panel data analysis. We considered two series: recycling rate (expressed by the indicator Circular material use rate) as dependent variable and innovation as independent variable.
At first, the series were tested whether they are stationary or not. Stationarity was determined using the Unit Root Test. Not having a unit root means that series are stationaries in levels (I (0)). If such a condition is present, there is no need to differentiate. Hence, there is no need to perform the co-integration tests. Consequently, the model will be of the OLS (Ordinary Least Squares) type.
In our case, we are going to use a panel data model in order to describe the evolution in time and across individuals (countries). E-View 11 package was the software used for all estimations. We have estimated three types of panel data models: a pooled model, a fixed effect model and a random effect model and selected the most appropriate.
In the Pooled model there are specified constant coefficients as the usual hypothesis for cross-sectional analysis; this model is the most restrictive and has a limited use:
Where:
- Yit is the dependent variable and the matrix Xit includes the explanatory variables;
- i represents the cross-section dimension (countries);
- t represents the time dimension;
- uit is the error term.
Individual-specific effects models take into account unobserved heterogeneity along cross-sections and include it in the term αi. If αi correlates with the regressors Xit, we deal with a fixed effects model. If there is no correlation detected, we are in case of a random effect model.
A Fixed effect (FE) model allows the individual specific effects (αi) to correlate with the regressors; this term is included in the model as intercept. The FE model is as follows:
After estimating the regression outcomes, it is possible to determine the cross-sectional fixed effects for all countries included in the panel data. Therefore, each country will have a specific intercept (αi) but the slope (β) will be the same.
The Random effect (RE) model is based on the assumption that the individual specific effects (αi) are distributed autonomously of the regressors and is included in the error term. The RE model is as follows:
This model considers that random effects do not correlate with the explanatory variables. A method for testing this assumption is to use the Hausman test in order to compare the fixed and random effects estimates of coefficients. If the Housman test is statistically significant (probability lower than 5%), we should use FE model. If the test is not statistically significant, we will use the RE model (Wooldridge, 2002; Baltagi, 2005).
2.2.2 Data Sources
Eurostat provides sets of data related to recycling and innovation within the Circular Economy set of indicators. Out of the 15 indicators structured in four sections we have chosen two indicators: Circular material use rate (CMUR) to stand (as proxy variable) for recycling and Patents related to recycling and secondary raw materials (called simply Patents) to stand (as proxy variable) for innovation1.
2.2.3 Circular material Use Rate
In the last years, Eurostat developed one new indicator (circular material use rate) in order to compensate for the absence of a single summary indicator about the circularity at macro economical level. The circular material use rate (CMUR) measures the contribution of recycled materials to overall materials use. The indicator includes flows of solid materials but it does not include flows of liquids (used water).
The CMUR is defined as the ratio of the circular use of materials (U) to an indicator of the overall material use (M): CMUR = U/M and is measured in percentages.
This indicator is approximated by the amount of waste recycled excluding imported waste destined for recovery and adding exported waste destined for recovery abroad. Waste recycled in domestic recovery plants comprises the recovery operations R2 to R11—as defined in the Waste Framework Directive 75/442/EEC. The imports and exports of waste intended for recycling—i.e., the amount of imported and exported waste bound for recovery—are approximated from the European statistics on international trade in goods.
A higher value for CMUR means that more recycling is taking place and secondary materials replace primary raw materials in the economy. This way the environmental impact of extracting primary material is well diminished.
Circular Material Use Rate data are available for the period 2010–2017. For the 28 EU countries taken into account there are 224 observations with a maximum value of 29.9%, a minimum value of 1.2% and a mean of 8.6%. More details are in the Annex 1.
Figure 1 displays the average value of the indicator Circular Material Use Rate across 28 EU member states, in the period 2010–2017. Netherlands has the highest CMUR (26.9%); a group of leader countries (Luxemburg, Italy, United Kingdom, Belgium and France) follows, with registered average values of this indicator between 14.4 and 17.9%. Another group of countries, called average performers (Poland, Germany and Estonia), recorded average values between 10.8 and 12.4%. There are 10 catching up countries, which recorded CMUR lower than 5%. The data shows that the spread of CMUR is large; indicating that recycling is not at all uniform across EU member states.
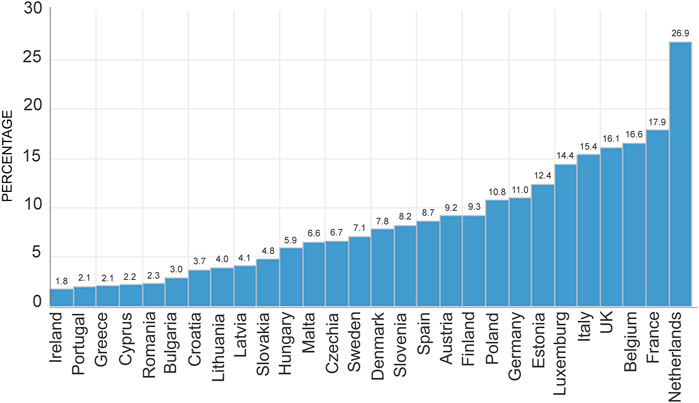
FIGURE 1. Mean of circular material use in EU, percentage (2010–2017)/Source: own calculation with Eurostat data.
Another perspective of the CMUR could be presented by considering the inclusion or not of a member state into the Euro area. In 2018, nine countries were not included in the Euro Area i.e.: Bulgaria, Denmark, Croatia, Czech Republic, Hungary, Poland, Romania, Sweden and United Kingdom.
States that are part of the Euro area have an average of the CMUR of 9.3%, compared with 7.0% recorded in states that are not part of Euro area (Table 1). The absolute difference is 2.3%, which represents 24.7% of the value recorded by states belonging to the Euro area. It is clear that member states included in the Euro area perform better in recycling.
2.2.4 Patents related to Recycling and Secondary raw Materials
Innovation will be will be analyzed through the indicator Patents related to recycling and secondary raw materials. The respective indicator will be called simply Patents in this article. By definition, the indicator Patents measures the number of patents2 related to recycling and secondary raw materials. The term “patents” refers to patent families, which include all documents relevant to a distinct invention (e.g., applications to multiple authorities), thus preventing multiple counting. A fraction of the patent family is allocated to each applicant and relevant technology.
Eurostat provides this indicator and data are available for the period 2000–2015, from 28 EU countries. There are available 360 entries, with a maximum of 141.66 patents/year and a minimum of zero. The average value is 13.7 patents/country/year and the total number of patents registered by the 28 countries analyzed is 4,930 (for more details see Annex 1).
During the period 2000–2015, most patents were registered by far in Germany (1,688 patents), followed by France (612 patents), Poland (395 patents), United Kingdom (332 patents) and Spain (308 patents). Other countries had not such a significant contribution to innovation, having registered a low number of patents (Figure 2).
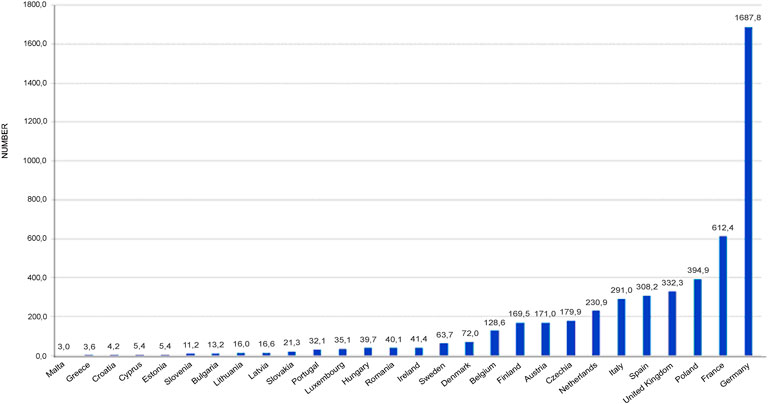
FIGURE 2. Innovation in EU by total patents registered (2000–2015) (no.)/Source: own calculation with Eurostat data.
When analyzing innovation in EU member states (2010–2015), we could deepen the perspective by aggregating countries that belong to Euro Area and others that are not yet part of it. Countries members of Euro area have recorded an average of 16.3 patents/country/year while countries which are not part have recorded 9.0 pa-tents/country/year (Table 2). We may notice the advance of Euro Area countries with an average of 7.3 patents/country/year.

TABLE 2. Mean of Patents related to recycling and secondary raw materials, by Euro area during the 2000–2015 period (no./year/country).
3 Results—Model Used
3.1 Visualization of Data Used in the Model
Data visualization is the first stage in our modelling approach by observing the graphical representation of information and data available. By using visual elements, we may have an accessible and easy way to see and understand trends, outliers and patterns in the data analyzed.
All calculations have been made on a panel data of 28 countries and a period of 2010–2017. Main statistics of the two indicators are in Annex 1. We have used the notation presented in Table 3.
Figure 3 presents the scatterplot of the two main variables Circular material used rate and Patents. In the model, for the variable Log(Patents_recycl (-2)) we have used a lag 2, assuming that the Patents variables would demonstrate their effect on CMUR at least 2 years later.
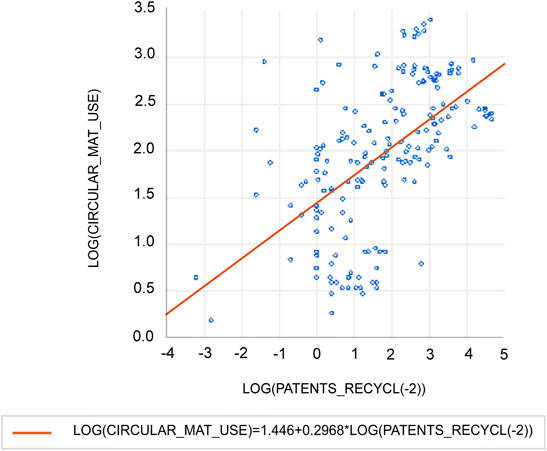
FIGURE 3. Scatterplot of the two variables considered/Source: own calculation with Eurostat 2020 data.
As observed from Figure 3, there is a relation between the two variables, represented by a regression line with a positive slope. In other words, the Circular material use rate is positively correlated with the Patents indicator. The regression line in Figure 3 was estimated using panel OLS method. Therefore, we are going to explore this relationship.
As stated earlier, dummy variable EURO is a binary variable (1 for countries members of the Euro Area and 0 for the opposite case); Figure 4 shows its influence on the scatterplot of the two main variables. We notice that the regression line corresponding to EURO countries is above the regression line corresponding to non-Euro countries for the entire interval considered. The slope of the regression line corresponding to the EURO area countries is 0.301 compared with the regression line on the non-EURO countries which has a smaller slope (0.2775).
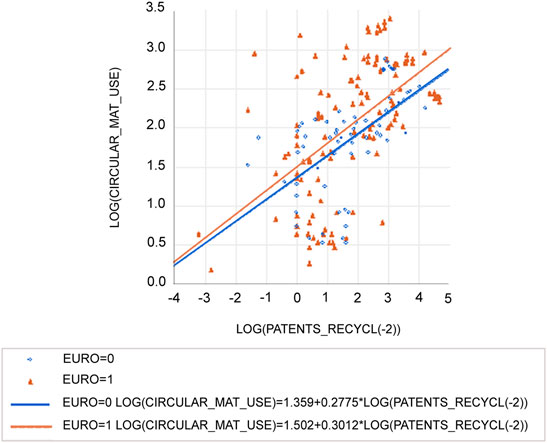
FIGURE 4. Scatterplot of the two main variables considered and the influence of dummy variable EURO/Source: own calculation with Eurostat 2020 data.
3.2 Main Steps and Results
Step 1: Verifying stationarity of the panel data series
The stationarity was determined using Unit Root Test. The hypothesis H0 is: series have a unit root. We accept it if the probability is higher than 5%. If not, we reject H0. The detailed results of the Unit Root Test for both series are in Annex 2. The main results of the test are.
1) Series CIRCULAR_MAT_USE: the Unit Root Test using Levin-Lin-Chu (assuming common unit root process) has the probability <0.0000. As well, the test Im, Pesaran and Shin W-stat and the test ADF—Fisher Chi-square, both show a probability much lower that 5%, therefore we reject H0. We conclude the series CIRCULAR_MAT_USE has no unit root, therefore is stationary in levels.
2) Series PATENTS_RECYCL: in the case of series PATENTS_RECYCL, the Unit Root test shows that the series has no unit root, therefore it is stationary in levels. All tests performed have probabilities much lower that the threshold of 5%, therefore we reject the H0 hypothesis that the series has a unit root. We conclude that the series PATENTS_RECYCL has no unit root, therefore is stationary in levels.
Hence, both series analyzed are stationary implying that their order of integration is I (0) (stationarity in level), therefore we can find a long-term relationship between them. Consequently, the model would be estimated using OLS.
Step 2: Building the econometric model
Estimating model of panel data was carried out based on the variables:
Yit = log(CIRCULAR_MAT_USE) is the dependent variable and
Xit = log(PATENTS_RECYCL(-2)) is the independent variable.
The model was built having natural logarithm of the raw data. This way, we are able to estimate directly the elasticity of the CMUR related to the independent variable (Patents).
We are going to estimate three models for panel regression, i.e.: Pooled OLS, Fixed effects and Random effects. Table 4 presents the estimations of the regression coefficients and the related statistical tests.
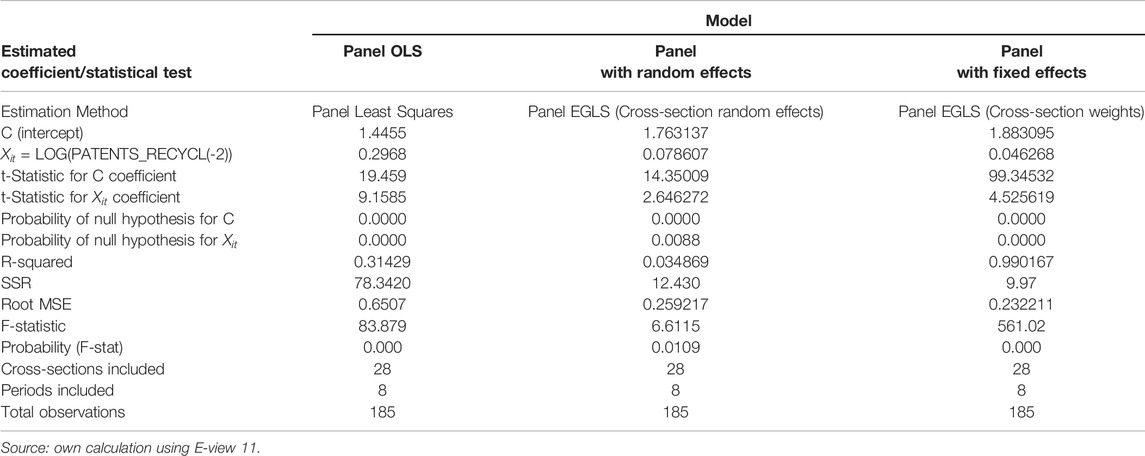
TABLE 4. The estimated coefficients and statistical tests of the econometric models taken into consideration.
Pooled LS Model
In case of the Pooled LS model, we have obtained the results presented in Table 4. The estimated coefficients of the regression are statistically significant from the Student Test point of view.
The coefficient of determination is equal to 0.31429. That means only 31.42% of the variation of the dependent variable is explained by the independent variable. We may conclude that the quality of the regression could be improved. Hence, we try to obtain an improvement of the estimation by using the method Panel EGLS (Cross-section weights) with the variant of random and fixed effects.
Random Effects Model
In case of the use of panel EGLS with random effects, we obtained parameters which are significant from the Student Test point of view (Table 4). We note that the quality of the regression remains quite poor. The coefficient of determination is very low (0.0348). Therefore, we consider that this model could be improved.
Fixed Effects Model
When we used the method of panel EGLS with fixed effects, we obtained significant estimated parameters from the Student Test point of view (Table 4). Comparing with the previous estimations, the elasticity of the Yi related to Xi is positive, respectively 0.0462. These results reveal that an intensification of the innovative activities, quantified by the number of patents related to the analyzed domain contributes to an increase of the rate of circular material use, but in a moderate way.
The quality of the regression is sensibly higher in comparison to the previous ones (Table 4). The coefficient of determination is higher than 0.99. In addition, the F-statistic is 561.02 and statistically significant. As well, FEM has better indicators as SSR and Root MSE both have the lowest values (9.87 and 0.2322) comparing with the previous models. The F-statistic is statistically significant and is highest among the models considered. Therefore, we may conclude that the FEM improved in a significant manner all good-ness-of-fit measures like F-test, SSE, root MSE and (adjusted) R2.
As previously stated, these models showed the strength (coefficients) and the direction of influence (positive/negative) of the independent variable (patents) on the dependent variable (recycling).
Step 3. Performing Hausman test and selecting the model
We use the Hausman test to verify if the panel model with random effects is appropriate or not. Null Hypothesis (H0) is: a panel model with random effects is appropriate. As the probability given by Hausman test (p = 0.001) is much lower than 5%, we reject the H0 hypothesis (Table 5). We may conclude that a model with fixed effects is appropriate in our case.
The Hausman Test confirms that the Panel EGLS model with fixed effects is an appropriate form for the relationship of the rate of circular material use and the innovative activity, quantified by the number of the patents related to the recycling and secondary raw materials use.
Therefore, after comparing all the three models, we selected the model with fixed effects, i.e.:
The coefficient of the Xit (Patents) has the value 4.626%, being sensibly lower than the unit (it signifies a slope of 2.65°). This means that a modification of the independent variable (Xit) by one unit has an influence on the dependent variable (Yit) of 4.63%, holding all other variables constant (p < 0.0000). We consider Patents a significant predictor.
As well, we noticed a lag between the two variables; the Innovation (Patents) practically influences the dependent variable (Circular_Mat_Use) 2 years after a patent is registered. This lag accounts for all administrative and technical procedures that have to be in place before a patent effectively applies in economy. The practice confirms that a patent once registered has to proceed through various stages until it is finally applied.
The model shows us that, if the independent variable is zero (Xit = 0) then the dependent variable (Yit) is still positive. That means, in this case, we still expect to have a positive CMUR (exp (1.8831) = 6.573%) (p < 0.0000).
While this model fits the data well, from the analysis exposed earlier regarding FE model, we may expect that the regression line for each country has different initial starting point (intercept). That is, each country may have its own initial value for CMUR, its Y-intercept, which is significantly different from those of other country but share the same slope. Therefore, next, we get country specific intercepts while the slope is the same.
As we mentioned earlier, each country will have a specific intercept (αi) called cross-section fixed effect. The values for these intercepts are in Table 6.
The highest value for cross section effect is that of the Netherlands (1.2816), followed by France, Belgium, Estonia, United Kingdom etc. (highlighted in green). By adding the value of the coefficient C (1.8831) we obtain the intercepts for all countries. Negative intercept means that, if the independent regressor is zero (no innovation) then the recycling rate will diminish so innovation is critical to have a positive recycling rate. The intercept calculated for the 28 countries has positive values: a minimum value of 0.5172 (Ireland) and a maximum value of 3.1647 (the Netherlands). The heterogeneity of the intercept values is significant. If we calculate the coefficient of variation for the intercept values, as a measure for heterogeneity, we end up with a value of 40%.
Having cross-section effects, we could calculate and draw the regression line for each country. Because there are 28 country and it is difficult to visualize so many regression lines, we chose four countries to be represented. As well, we are going to plot the regression line resulted from the OLS panel and from the fixed effect model with cross-section weights (Eq. 4). Table 7 presents the respective equations.

TABLE 7. Equations of the FEM model and for some countries (Figure 5).
As can be acknowledged from Figure 5 and how was previously stated, the FEM regression line (the green line) has a smaller slope than the OLS model (the blue line) but it has better indicators and has improved all goodness-of-fit statistics. For each individual country could be derived a regression line.
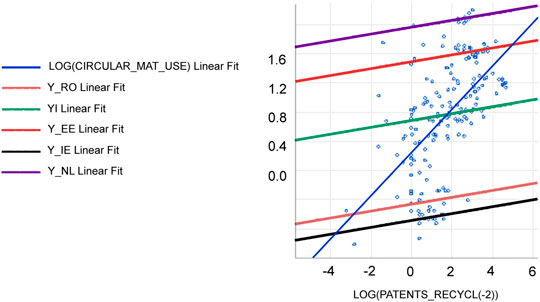
FIGURE 5. Comparative regression lines for OLS model and Fixed Effect Model (FEM)/Source: own calculation.
As remarked earlier, countries with negative cross section effects will have regression lines below the FEM regression line, as it is the case of the two countries (Romania and Ireland) selected as an example.
Countries with positive cross section effects will have regression lines above of the FEM regression line. It is not feasible to draw all regression line for the 28 countries analyzed.
Step 4. Introducing Dummy variable
The model described so far allows us to expand the discussion introducing the dummy variable EURO. The results are in Table 8.

TABLE 8. The estimated coefficients and statistical tests of Eq. 5.
After including the dummy variable EURO, the model will look as described in Eq. 5. Table 8 presents the estimated coefficients and corresponding statistical tests.
Eq. 5 acknowledges that all coefficients are positive, meaning that all of them have a productive contribution to the dependent variable. The estimated parameter of the dummy variable EURO is statistically significant for a probability of 10% but not for 5%. The estimated parameter for the independent variable and for the intercept (C) are statistically significant for 5% probability. So, from statistically point of view the membership of Euro area has a positive influence.
4 Discussion and Conclusion
In the endeavors to develop a low carbon, resource efficient and competitive economy and ultimately to support the sustainable development in the European Union, recycling is an important practical approach with essential contribution.
In this respect, the main objective of the paper is a theoretical and methodological grounding and analysis of the correlation of innovation and recycling required for the implementation of the circular economy in the European Union. There are also some original or less discussed aspects regarding the influence of the adoption of Euro as national currency on the performance in recycling.
The article brings new understandings regarding the relationship between innovation and recycling within EU. The 2016 report of the Eco-Innovation Observatory mentioned that innovation and CE is not homogeneous across member states. There are groups of member states: a group of leaders that have a scoring significantly higher than the EU average; a group of average performers that are countries with scores around the EU average and a catching up—group of countries—countries that recorded aggregate scores less than EU average.
Analyzing more thoroughly the two indicators selected (Circular material use rate and Patents), we found a similar pattern: uneven distribution among member states and three groups of states: leaders, average performers and catching up countries.
The step ahead made within this research is that of showing the influence of EURO membership. In case of the indicator CMUR, considered dependent variable, we found that Euro membership has a positive influence, on average, of +2.3% compared with countries that are not using Euro as a currency. Examination of the main regressor considered in the article (Patents) showed a similar pattern. There is an uneven distribution and the Euro Area membership has a positive influence on the indicator. In Euro Area, the average of the number of patents/country/years was 16.3 compared with 9.0 in case of the group of countries which are not yet members of Euro Area.
Therefore, we conclude that indicators considered have an asymmetric distribution across member states identified as leaders, average performers and catching up countries.
Furthermore, the paper provides evidence of the relationship between innovation and recycling. The model that resulted, carrying out a regression on panel data, showed the relationship between Circular Material Use Rate and Patents. The linkage is statistically significant (p < 0.0000) and by analyzing three similar models, the Panel Data model with fixed effects was selected as a plausible one. After comparison of the statistical values, we concluded that Fixed Effects Model (FEM) improved all goodness-of-fit measures like F-test, SSE, root MSE, and (adjusted) R2 in a significant manner.
This paper demonstrates that the influence of the eco-innovation on recycling is moderate but it matters more when the initial status of recycling is low. If the initial status is negative then innovation is very important to bring recycling to positive values. As well, another aspect highlighted is that the influence of innovation is not instantaneous and there is a 2 years lag between the innovation inception and its implementation in practical activities, when its effects are measured by recycling rates.
From this paper could be derived some practical implication. One direction is to make more efforts to comply with the requirements of the Euro Area in order to speed up the admission of countries that still use their own currency. Of course, membership of Euro Area is not a miraculous solution but it will bring discipline, stability and financial rigor that, in turn, could add benefits in development of recycling activities.
The answer to the questions raised in the Introduction are as follows:
Answer 1: the influence of innovation on recycling is moderate with a gap of 2 years; Answer 2: the influence of the Euro area is important on recycling (+24,7%); Answer 3: the heterogeneity among member state is significant (40%)
As with the majority of studies, the design of the current article is subject to boundaries and limitations. Some of the limitations are related to available resources, since there are few prior research studies that are relevant to the topic of the article and also the statistical data are not completely available. In these circumstances, we consider as sufficient the sample of 28 countries.
For future studies it will be useful that some other explanatory variables would be used to have a better model to determine more drivers and barriers of the Circular Economy. One aspect that could be further explored is the determination of the relationship between recycling and the scoreboard defining eco-innovation as well as to explore the influence of eco-investment on the Circular Economy.
The paper has several originality aspects: it has taken into account criteria that are not so often discussed (membership of Euro Area), it has developed a model that brings quantitative values of the link between recycling and innovation. Until now, this link was analyzed so far, mainly from a qualitative point of view and less from the quantitative perspective.
Data Availability Statement
Publicly available datasets were analyzed in this study. This data can be found here: https://ec.europa.eu/eurostat/data/database.
Author Contributions
VP and FMP conceived the study and were responsible for the design and development of the data analysis. VP and FMP were responsible for data interpretation. VP wrote the first draft of the article. DA and SF were responsible for data collection, analysis and for reviewing first draft of the article. AC and FP were responsible for general assembly of the article, English translation, template compliance and other proofreading corrections and syntax modifications.
Conflict of Interest
The authors declare that the research was conducted in the absence of any commercial or financial relationships that could be construed as a potential conflict of interest.
Publisher’s Note
All claims expressed in this article are solely those of the authors and do not necessarily represent those of their affiliated organizations, or those of the publisher, the editors and the reviewers. Any product that may be evaluated in this article, or claim that may be made by its manufacturer, is not guaranteed or endorsed by the publisher.
Footnotes
1https://ec.europa.eu/eurostat/data/database
2According to Eurostat, the attribution to recycling and secondary raw materials was done using the relevant codes in the Cooperative Patent Classification (CPC) (list of CPC codes selected) (online data code: CEI_CIE020 last update: 01/02/2020).
References
Antonescu, D. (2015). Theoretical and Practical Approaches of Innovation at Regional Level”. Munich: GRIN Verlag. Available at: https://mpra.ub.uni-muenchen.de/68178/.
Baltagi, B. H. (2005). Econometric Analysis of Panel Data. Third Edition. West Sussex, England: John Wiley & Sons.
Banacu, C. S., Busu, M., Ignat, R., and Trica, C. L. (2019). Entrepreneurial Innovation Impact on Recycling Municipal Waste. A Panel Data Analysis at the EU Level. Sustainability 11, 5125. doi:10.3390/su11185125
Camilleri, M. A. (2020). European Environment Policy for the Circular Economy: Implications for Business and Industry Stakeholders. Sustain. Dev. 28 (6), 1804–1812. doi:10.1002/sd.2113
Camilleri, M. A. (2021). Sustainable Production and Consumption of Food. Mise-En-Place Circular Economy Policies and Waste Management Practices in Tourism Cities. Sustainability 13 (17), 9986. doi:10.3390/su13179986
Camilleri, M. A. (2019). The Circular Economy's Closed Loop and Product Service Systems for Sustainable Development: A Review and Appraisal. Sustain. Dev. 27 (3), 530–536. doi:10.1002/sd.1909
Di Maio, F., and Rem, P. C. (2015). A Robust Indicator for Promoting Circular Economy through Recycling. Jep 06, 1095–1104. doi:10.4236/jep.2015.610096
Doranova, A., Roman, L., Bahn-Walkowiak, B., Wilts, H., O’Brien, M., Giljum, S., et al. (2016). Policies and Practices for Eco-Innovation Uptake and Circular Economy Transition. Eco-Innovation Observatory, bi-annual report. Available at: https://www.eco-innovation.eu/index.php/reports/annual-reports.
EC COM (2015). Final: Communication from the Commission to the European Parliament, the Council, the European Economic and Social Committee and the Committee of the Regions Closing the Loop - an EU Action Plan for the Circular Economy. Brussels: European Commission, 614. Available at: http://eur-lex.europa.eu/legal-content/en/TXT/?uri=CELEX%3A52015DC0614.
Frone, S., and Constantinescu, A. (2018). Issues and Trends of Eco-Innovation for Resource Efficiency Improvements in Romanian SMEs. Annals of the „Constantin Brâncuşi” University of Târgu Jiu, Economy Series, Issue 3/2018. Available at: https://www.utgjiu.ro/revista/ec/pdf/2018-03/28_Frone.pdf.
Frone, S. (2017). Eco-innovation Promoting the Circular Economy in Romania. Romanian J. Econ. 44 (1), 155–184.
Geissdoerfer, M., Savaget, P., Bocken, N. M. P., and Hultink, E. J. (2017). The Circular Economy - A New Sustainability Paradigm? J. Clean. Prod. 143, 757–768. doi:10.1016/j.jclepro.2016.12.048
Horbach, J., and Rammer, C. (2020). Circular Economy Innovations, Growth and Employment at the Firm Level: Empirical Evidence from Germany. J. Industrial Ecol. 24 (3), 615–625. doi:10.1111/jiec.12977
Hysa, E., Kruja, A., Rehman, N. U., and Laurenti, R. (2020). Circular Economy Innovation and Environmental Sustainability Impact on Economic Growth: An Integrated Model for Sustainable Development. Sustainability 12 (4831), 4831. doi:10.3390/su12124831
Korhonen, J., Honkasalo, A., and Seppälä, J. (2018). Circular Economy: the Concept and its Limitations. Ecol. Econ. 143, 37–46. doi:10.1016/j.ecolecon.2017.06.041
Mentink, B., (2014). ”Circular Business Model Innovation, A Process Framework and a Tool for Business Model Innovation in a Circular Economy”, master thesis.
Pieroni, P. P., Marina, T., McAloone, C., and Pigosso, C. A. (2019). Business Model Innovation for Circular Economy and Sustainability: A Review of Approaches. J. Clean. Prod. 215, 198–216. doi:10.1016/j.jclepro.2019.01.036
Planing, P. (2015). Business Model Innovation in a Circular Economy Reasons for Non-acceptance of Circular Business Models. Berlin: Open Journal of Business Model Innovation. Available at: https://www,researchgate,net/profile/Patrick_Planing/publication/273630392_Business_Model.
Potting, J., Hekkert, M. P., Worrell, E., and Hanemaaijer, A. (2017). Circular Economy: Measuring Innovation in the Product Chain. Utrecht: Planbureau voor de Leefomgeving. Available at: https://dspace.library.uu.nl/handle/1874/358310.2544.
Repp, L., Hekkert, M., and Kirchherr, J. (2021). Circular Economy-Induced Global Employment Shifts in Apparel Value Chains: Job Reduction in Apparel Production Activities, Job Growth in Reuse and Recycling Activities. Resour. Conservation Recycl. 171, 105621. doi:10.1016/j.resconrec.2021.105621
Sumrin, S., Gupta, S., Asaad, Y., Wang, Y., Bhattacharya, S., and Foroudi, P. (2021). Eco-Innovation for Environment and Waste Prevention. J. Bus. Res. 122, 627–639. doi:10.1016/j.jbusres.2020.08.001
Van Berkel, R. (2007). Eco-Innovation: Opportunities for Advancing Waste Prevention. Int. J. Environ. Technol. Manag. 7, 1. doi:10.1504/ijetm.2007.015629
Vence, X., and Pereira, Á. (2019). Eco-innovation and Circular Business Models as Drivers for a Circular Economy. CyA 64, 64–19. doi:10.22201/fca.24488410e.2019.1806
Appendix
Keywords: recycling, patent, fixed effects, random effects, material use rate
Citation: Platon V, Pavelescu FM, Antonescu D, Frone S, Constantinescu A and Popa F (2022) Innovation and Recycling—Drivers of Circular Economy in EU. Front. Environ. Sci. 10:902651. doi: 10.3389/fenvs.2022.902651
Received: 23 March 2022; Accepted: 01 June 2022;
Published: 05 July 2022.
Edited by:
Jan Brusselaers, VU Amsterdam, NetherlandsReviewed by:
Larisa Ivascu, Politehnica University of Timișoara, RomaniaMark Anthony Camilleri, University of Malta, Malta
Copyright © 2022 Platon, Pavelescu, Antonescu, Frone, Constantinescu and Popa. This is an open-access article distributed under the terms of the Creative Commons Attribution License (CC BY). The use, distribution or reproduction in other forums is permitted, provided the original author(s) and the copyright owner(s) are credited and that the original publication in this journal is cited, in accordance with accepted academic practice. No use, distribution or reproduction is permitted which does not comply with these terms.
*Correspondence: Victor Platon, dmljdG9yLnBsYXRvbjU0QGdtYWlsLmNvbQ==