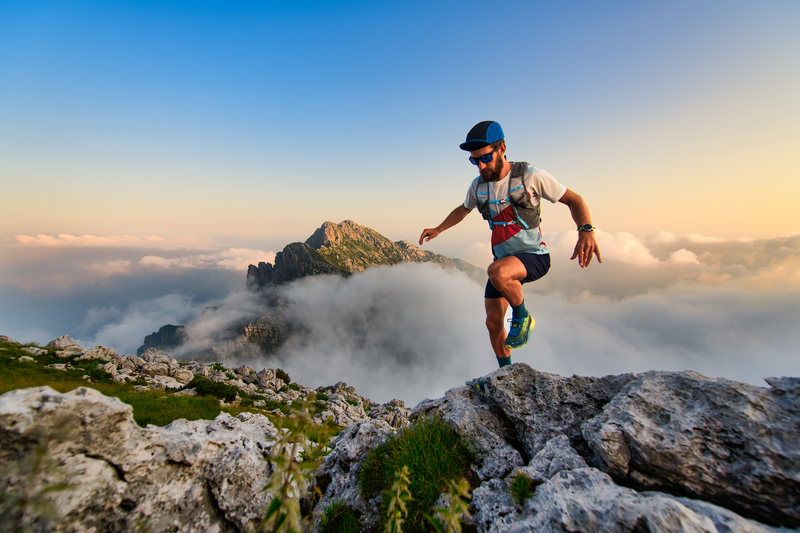
95% of researchers rate our articles as excellent or good
Learn more about the work of our research integrity team to safeguard the quality of each article we publish.
Find out more
ORIGINAL RESEARCH article
Front. Environ. Sci. , 31 August 2022
Sec. Environmental Economics and Management
Volume 10 - 2022 | https://doi.org/10.3389/fenvs.2022.902182
This article is part of the Research Topic Economic Development, Social Consequences, and Technological Innovation Under Climate Change COVID-19 Pandemic Conditions View all 48 articles
Amid rising market competitiveness, Industry Revolution (IR) 4.0 oriented technological integration is considered an imperative driver of sustainable organizational performances and green supply chain management. This study explores the role of IR 4.0 powered process technology innovation in enhancing Leanness, Green Supply Chain Management, and Organizational Performance (including operational, economic, and environmental) during COVID-19. For this purpose, a novel conceptual framework was developed, and Partial Least Square-Structural Equation Modelling (PLSM) was employed on primary data of 314 respondents collected from Chinese manufacturing industries. Moreover, Multi-Group Analysis was also implemented to compare firms’ willingness to implement IR 4.0 technologies powered process innovation. The results exhibit that Green IR 4.0 powered process technology innovation improves firm’s leanness and stimulates environmental, optional, and economic performances. Similar findings are endorsed through the green supply chain management channel. Manifestly, COVID-19 instigated firms to adopt IR 4.0-based technological processes for efficient supply chain management. Based on these results, organizations are recommended to integrate IR 4.0 induced technology innovation to spur manufacturing firms’ eco-economic and operational performance.
In recent times, firms have been facing immense pressure to have a higher level of technology and, accordingly, transform their supply chain to maintain their relevance in the market (Giovanni and Cariola, 2021). In addition to this, with the ongoing debate related to the relevancy and scalability of the Industry Revolution (IR) 4.0, several technological solutions are available. These include the internet of things, big data, Cyber-physical systems, Augmented Reality (AR) and simulation, 3D printing, Automated Guided Vehicles, and so on (Ivanov et al., 2019; Kanapathy et al., 2022). These solutions are designed to bring operational excellence in organizations by deploying green investments and offering a solution through technology innovation (Preeker and De Giovanni, 2018).
Conventionally, specific solutions related to manufacturing have been reported as consistent, reliable, and robust for operational excellence. Among them, the philosophy of lean is the most utilized and followed (Jasti and Kodali, 2015; Buer et al., 2018). Lean manufacturing philosophy has been explained as a combination of multiple operations and practices deployed to eliminate non-value-added activities and all kinds of wastes, including human motion, inventory, process duplication, and so on (Womack et al., 1990). In lean philosophy, the organization aims to enhance their productivity and efficiency, performance, sales, customer value, and satisfaction (Yang et al., 2011) through implementing best practices of lean; organizations tend to attain the leanness at a certain level (Genc and De Giovanni 2018).
In terms of environmental friendliness, lean practices should positively affect environmental quality; however, scholars have a certain level of disagreement regarding this relationship (Giovanni and Cariola, 2021). Researchers in favor of positive relationships highlight that implementing lean practices reduces wastage and excessive operations that negatively affect the environment (Genc and De Giovanni, 2017). However, researchers also argue that both phenomena can have negative relationships. This is because a lean organization which is principally operated as customer-focused and incorporates customization by customers will have production batches that are relatively larger with small sizes, leading to additional startup and processing costs, and in turn to the destruction of the environment (Venkat and Wakeland, 2006). And this situation does not just reside with the manufacturing firm itself; it follows along the whole supply chain (Giovanni and Cariola, 2021). Therefore, there is a need to explore these relationships better.
In addition, the regular worsening and destruction of ecology and human health is motivating individuals to consume responsibly (Najmi et al., 2021a) and demanding organizations fulfil their extended responsibilities (Najmi et al., 2022). Moreover, not just customers but also government institutions are forcing organizations to increase compliance with the rules and directives governing environmental safeguarding (Ahmed et al., 2021). Therefore, organizations are transforming their supply chain towards more environmentally friendly decisions and moving towards having practices oriented to Green Supply Chain Management (GSCM) (Ahmed et al., 2018). It does not just include the incorporation of environment orientation in the forward supply chain, sourcing, manufacturing, and selling, but also includes the disposition of product when it is consumed (Najmi et al., 2021b), calling back products (Najmi et al., 2019), and recycling (Razzaq et al., 2021). Nevertheless, implementation of GSCM is not easy, and requires sound financial investment and participation of all related stakeholders (Ahmed et al., 2020a).
Innovation is a term that reflects novelty, recency, advancement, and high efficiency, and is considered an important determinant of organizational performance and competitiveness (Ahmed et al., 2020a). Therefore, despite the association of lean GSCM with organizational performances, there will still be a need for innovation to assist in achieving operational, economic, and environmental excellence (Ahmed et al., 2020a; Giovanni and Cariola, 2021). Especially in recent times, when the technological solution of IR 4.0 powers involve innovation, there is the highest probability that organizational performance excellence will be easily achieved. However, these require empirical investigation to back the arguments with practical justification and reasoning. Hence, this study seeks the answers to the following research questions:
RQ1: To what extent do lean and GSCM contribute to excelling organizational performances (including operational, economic, and environmental)?
RQ2: How does IR 4.0 powered process innovation enhance the relationships of lean and GSCM with organizational performances (including operational, economic, and environmental)?
The operationalization of lean practices to acquire a competitive advantage is integral, especially in current manufacturing in challenging business environments (Giovanni and Cariola, 2021). Moreover, it has been reported in a study that more than 80% of the studied sample agree to adopt the lean strategy in their manufacturing operations for the attainment of a competitive advantage (Dombrowski and Mielke, 2012). Whenever there is a discussion of lean philosophy, there are some crucial elements that form its foundations. These include: reduction in the generation of waste and non-value added activities, reduction in excess inventory and lead times, and the transformation of the overall organizational culture where continuous improvement and innovation are encouraged (Martínez-Jurado and Moyano-Fuentes, 2013; Giovanni and Cariola, 2021). Similarly, lean manufacturing has been explained as a value addition process in which there is a transformation of raw material into a value-added product after following the principles of lean as discussed earlier (Jasti and Kodali, 2015; Buer et al., 2018; Genc and De Giovanni, 2018). In addition to this, when a firm follows the lean philosophy and reduces the generation of waste by improving resource optimization, it eventually contributes to the greenness of the environment, and such practices and operations are considered environment-friendly operations (Giovanni and Cariola, 2021).
According to Dües et al. (2013), there is a high correlation between lean and green when operationalizing and integrating them at different levels, including fulfilling customer demands, reducing lead times, and improving product design. On the other hand, companies that implement lean practices move towards green philosophy (Franchetti et al., 2009; Inman and Green, 2018). The concept of Green Supply Chain Management (GSCM) revolves around the ideology of collaboration and coordination among the supply chain partners for environmental betterment and well-being (Wong et al., 2015; Ahmed et al., 2018). Hence, for the coordination, there is a need to have synergy among the partners and a common understanding of environmental protection. GSCM has pushed the philosophies beyond the boundaries of a single organization (Vachon and Klassen 2006). Researchers agree that for an environment-friendly operation that follows the GSCM principles, the manufacturing concerns need to be efficient (De Giovanni, 2016; Colicchia et al., 2017). Hence it is proposed that:
H1a: Leanness of the organization directly enhances the level of organizational GSCM.
In the current study, there are three kinds of organizational performances studied. These are environmental, operational, and economic performances. Considering the relationship between leanness and ecological performance, despite the positive attributes that leanness contributes to environment and ecology, there is a disagreement in terms of the nature of the relationships (Giovanni and Cariola, 2021). For instance, Dhingra et al. (2014) reported that firms could easily improve their corporate reputation and profile in terms of greenness through leanness. However, not all leanness necessarily contributes to the environment. For instance, removing excessive inventory is one of the principles of leanness, and by doing that, there will be the production of small batches; however, due to such small batches, there will be more frequent transportation expenses across the supply chain, thus leading to environmental pollution through carbon emissions (Venkat and Wakeland, 2006; Ramani and De Giovanni, 2017). Hence, there will be a tradeoff on whether the organization should opt for leanness or greenness as products and resources optimization are often done at the cost of the environment (Inman and Green, 2018). Despite this, leanness foundations are built on the principles of waste elimination; thus, it will also be beneficial for the environment. Hence it is proposed that:
H2a: Leanness of the organization directly enhances organizational environmental performance.
H2b: Leanness of the organization indirectly enhances organizational environmental performance.
There should not be any doubt that whichever firms opt to have leanness in their operations is doing it to improve their operational and financial performance (Martínez-Jurado and Moyano-Fuentes, 2013). For instance, when the firm is willing to reduce the lead time without impairing any other efficiencies and eventually do it successfully, it will improve both operational and economic performances (De Giovanni, 2017). However, this is not the case in every situation. For example, for customer service, if a firm offers customized products, it is challenging to handle operationally. In contrast, when the firms implement a continuous improvement philosophy, frequent changes adversely affect economic and operational performances (Giovanni and Cariola, 2021). Additionally, during these changes, there will be an additional expenditure of training, monitoring, sacrifice of product quality, and so on (Duhaylongsod and De Giovanni, 2019). However, leanness is expected to enhance the organizational operational and economic performance in the long-term. Hence it is proposed that:
H3a: Leanness of the organization directly enhances the level of organizational operational performance.
H3b: Leanness of the organization indirectly enhances the level of organizational operational performance.
H4a: Leanness of the organization directly enhances the level of organizational economic performance.
H4b: Leanness of the organization indirectly enhances the level of organizational economic performance.
As already discussed, the concept of GSCM encompasses all of the aspects of the typical supply chain (Ahmed et al., 2018) and enhances the level of collaboration between the supply chain partners (Ahmed et al., 2021) for environmental betterment and well-being (Ahmed et al., 2020b). In addition to this, for successful implementation of GSCM, firms need to have suppliers on board (Najmi et al., 2020), transformation of internal operations towards green (Ahmed et al., 2019), and the concerns of the relevant stakeholders (Ahmed et al., 2021). Through the implementation of GSCM, there are several benefits that a firm can extract. These include improvement in profits because of resources’ optimization (Ahmed et al., 2021), an increase in sales due to improved corporate image and reputation (Giovanni and Cariola, 2021), and extending competitive advantage (Rao and Holt 2005). In addition to this, researchers have documented the positive association between GSCM and competitiveness and environmental excellence (Chen et al., 2015), economic performance (Bowen et al., 2003), and operational performance (De Giovanni and Ramani, 2017). However, certain researchers are against these findings (see De Giovanni and Vinzi, 2014; De Giovanni (2017); Rao 2002). Hence, despite the contrasting evidence, it has been assumed that:
H5a. GSCM directly enhances the level of organizational Environmental Performance
H6a. GSCM directly enhances the level of organizational Operational Performance
H6b. GSCM indirectly enhances the level of organizational Operational Performance
H7a. GSCM directly enhances the level of organizational Economic Performance
H7b. GSCM indirectly enhances the level of organizational Economic Performance
The different aspects of organizational performances have interesting relationships within themselves. For instance, for environmental performance, firms opt for consuming green inputs that normally are not up to the mark in terms of quality, which adversely affect operational performance and economic performance (Kirchoff et al., 2016). On the other hand, implementing green initiatives for environmental performance, which are comparatively dearer than conventional investments, leads to adverse effects financially, but the benefits are reaped over a longer period of time (Ahmed et al., 2018). Thus, despite the contrasting evidence, it has been assumed that:
H8a. Environmental performance directly enhances the level of organizational Economic Performance
H9a. Environmental performance directly enhances the level of organizational Operational Performance
H9b. Environmental performance indirectly enhances the level of organizational Operational Performance
In addition to this, theoretically, operational performance directly correlates with the firms’ economic performance. This is because, for improving operational performance, an organization takes certain initiatives, like improving productivity, optimizing resources, eliminating non-value-added activities, improving sales, enhancing market share, attaining competitive advantage, and so on (De Giovanni and Zaccour 2019; Ahmed et al., 2021). And by doing that, it is more likely that this will improve the organization’s financial position, profits, and economic performance.
H10a. Organizational Operational Performance enhances the level of organizational Economic Performance.
Another objective of the current study was to explore the role of Industry revolution (IR) 4.0 powered process innovation in better organizational outcomes. Though the organizations are quite aware of their environmental responsibilities and are taking necessary initiatives to meet their environmental targets, they are either reluctant or not sure about the benefits and scalability of innovation through IR 4.0 powered technologies (Chiarini et al., 2020). On the other hand, Liu and De Giovanni (2019) confirms the association between GSCM, leanness, and organizational performance. Furthermore, Buer et al. (2018) highlighted that IR-based innovation is being made to improve productivity and efficiency, which aligns with the principles and foundations of lean. With the help of investing in IR 4.0, firms will be in a position to incorporate smart technologies like the internet of things, robotics, big data, cyber-physical systems, and so on (Kanapathy et al., 2022), which improves the operational, economic, and environmental outcome of the organization. However, due to the stakeholders’ reluctance, rigidness, and non-cooperation, firms resist taking such initiatives. Hence, the present study intends to compare whether that companies are willing to implement process innovation powered by IR 4.0 technologies are better in their operations than those companies that resist. Therefore, it is proposed that:
H1–H10c: IR 4.0 powered process innovation enhances the earlier proposed hypotheses (H1–10).
The conceptual framework is presented in Figure 1, following the above discussion.
To empirically investigate the proposed hypotheses (discussed in the previous section), the current study utilizes the Quantitative research approach. It involves the numeric data drawn by the outcome, logic, and results. After incorporating specific statistical analysis, it should also be noted that because of this kind of research approach, the logical conclusions and outcome can be drawn by utilizing the primary data collected from the potential samples and that such findings are treated as generalizable for the whole population, of which such a sample is representative of (Cooper et al., 2006). Moreover, within the quantitative research approach, there are various research methodologies that can be selected depend upon the objectives and nature of the study. Likewise, following the current study’s objectives, the survey research design was chosen. In such a research design, a survey questionnaire is developed that reflects the studied phenomena and upon which the potential respondents’ responses are sought. However, this methodology needs to be followed by addressing certain precautions as, otherwise, the collected data and the generated outcome could be biased and inferior. Therefore, following the guidelines of Hulland et al. (2018), there was a development of a survey questionnaire that intends to ask the questions measuring the studied phenomena and the queries catering for the demographic information of the respondents discussed in the following section.
As mentioned earlier, for the purpose of collecting the data, a survey questionnaire was designed, which was self-administered and intended to measure the studied phenomena, along with the queries catering for the demographic information of the respondents. The researchers relied on the existing developed scales for measuring the studied phenomena as they have already been tested and validated in other studies. Therefore, the researchers adapted the measuring questions from the sources listed in Table 1. Moreover, on all of these questions, the respondents were asked about their level of agreement on the 5-point Likert Scale, which stated that “1 represents Strongly Disagree,” “2 represents Disagree,” “3 represents neither Disagree nor Agree,” “4 represents agree,” and “5 represents Strongly Agree.” Furthermore, for the demographic questions, respondents were asked about their gender, age, size, and nature of the organization they belonged to. In addition to this, following the objective of understanding the potential role of process innovation through IR 4.0 technologies in improving the overall supply chain performances, an additional question was added to the questionnaire seeking the respondent’s willingness to implement such technologies for process innovation. Such a question is intended to act as the moderator and hence requires additional application of Multi-Group Analysis (discussed in Section 4.3.3).
Apart from relying on the same statements, the researchers also validate the understanding of the questions through the experts about the face and the content. The purpose behind this is to remove confusion and ambiguity that may arise in the respondents’ minds when responding to the survey questions. Since the current study is based on the data collected from the Chinese respondents, and as the respondents’ first language is not supposed to be English, therefore the comprehension of the statements of the measurement scale needs to be easy to comprehend and understand. Therefore, a panel of five experts comprising of two languages and three subject-related individuals was consulted. The comments and improvements suggested by the individuals were incorporated, and then the questionnaire was made available for the data collection.
Since the geographical context of the current study resides in China and the study’s objectives are focused on resource optimization and environmental well-being, only the supply chain professionals from those companies were approached for data collection through the questionnaire that is, ISO14001 certified. Initially, 1,000 questionnaires were circulated, and 390 respondents responded, leading to a response rate of 39%. Among those 390, the responses having missing values and identified as univariate and multivariate outliers were eliminated following the discussions by Hair et al. (2010), leading to the useable sample of 314 respondents upon which the statistical analysis was applied.
In the research involving survey design, there is a significant likelihood of having methodological and operational biases, which accordingly need to be controlled; otherwise, they lead to distorted and inaccurate outcomes (Podsakoff et al., 2012). Such kinds of unexpected and unwanted variance, which have the potential to inflate the outcome, are termed as “Common Method Variance” (CMV) (Podsakoff et al., 2003). Hence, for the exploration, the study employs Harman’s (1967) single factor test also applied in other related studies (see Najmi and Ahmed, 2018). In addition to this, another test was applied to gauge the level of CMV proposed by Kock (2015) and is named Full Collinearity Assessment. Both of the tests nullify the presence of CMV.
The final data sample for the current study comprised 314 respondents that belong to the ISO 14001 certified companies from China. The majority of the respondents were male, accounting for 58%, and were aged between 31 and 40 years, accounting for 45% of the data. In terms of the size of the companies the respondents belong to, 90 (29%) were from companies with less than 100 employees, 102 (32%) were from companies with employees between 101–250, 79 (25%) were from companies with employees between 251–450, and 43 (14%) were from companies with greater than 450 employees. In terms of the nature of the business, 74 (24%) were from the automobile industry, 119 (38%) were from the electronics industry, 49 (16%) were from the chemical industry, 45 (14%) were from the Pharmaceutical industry, and 27 (9%) were from industries other than those mentioned earlier. The demographic profile of the respondents are listed in Table 2.
Based on the objective and the conceptual framework proposed in Figure 1 and exploring the direct relationships of predictors with the criterion variables, the current study also explained the indirect and the exploration of the role of technological innovation as moderator. Therefore, because of the complexity of the research framework, the current study employs the application of Partial Least Square-Structural Equation Modelling (PLS-SEM) as its technique belongs to the second generation and is known for explaining greater variance from the data when the models are complex (Hair et al., 2019). However, the application of PLS-SEM was made through SmartPLS, which was designed by Ringle et al. (2015). Additionally, the guidelines discussed by Hair et al. (2016) were followed, which suggested applying PLS-SEM in two stages. The first stage involves assessing the outer model, and the later stage consists in evaluating the inner model.
As per Hair et al. (2016) guidelines, the outer model is also referred to as the measurement model. It involves examining the relatedness of the measuring items with their respective construct, referred to as convergent validity. The level of un-relatedness that the measuring items of a construct possess with the measuring items of another construct is denoted as discriminant validity (Mehmood and Najmi, 2017).
Firstly, the assessment of convergent validity is done using three criteria. The first is that factor loading must be larger than 0.7 (Hair et al. (2016); the second is that internal consistency, which is assessed by Cronbach’s Alpha and Composite Reliability, should also be larger than 0.7; and Average Variance Extracted (AVE) which according to Hair et al. (2016) must be larger than 0.5. Table 3 summarizes the assessment of convergent validity.
For the discriminant validity, three criteria have been utilized in the current study. Firstly, according to Gefen and Straub (2005), the loading of a factor into its respective construct must be higher, and the difference between the higher respective loadings and the loadings into other constructs, referred to as cross-loadings, must be greater than 0.1. Table 4 summarizes the assessment of discriminant validity through the criteria of cross-loadings.
The second criterion for assessing discriminant validity is Fornell and Larcker (1981) criterion. According to this measure, the square root of AVE of a particular construct should be higher than all of the values representing correlations of a construct with all of the other constructs. Referring to Table 5, the value which is highlighted and placed at the diagonal line is the square root of AVE, whereas the values other than the diagonal values are the correlations among the constructs. It is evident that based on the criteria proposed by Fornell and Larcker (1981), the outcome listed in Table 5 reflects the meeting of the discriminant validity.
The third criteria utilized for the assessment of discriminant validity is “Heterotrait-Monotrait ratio of correlations” (HTMT). The criteria is the latest one that Henseler proposes, and, since its proposition, has been used in many studies. Henseler et al. (2015) proposed that the threshold for HTMT is 0.85. The outcome listed in Table 6 summarizes the HTMT correlations and confirms the accomplishment of the said criteria.
In the inner model, the predictability, relevancy, and accuracy of the predictor variables while linking them with the criterion variables is assessed, which is necessary in accordance with the guidelines proposed by Hair et al. (2016). Though for this assessment, the nature and operationalization of the predictor variables are extremely important, as their nature, number, and theoretical connectivity with the criterion variables decide the level of explanation that is, made of criterion variable. Still, there are certain thresholds that provide an interpretation to the generated outcome. For instance, Cohen (1988) suggested that with R-Square, which is the measure of “coefficient of determination”, any value which is greater than 0.25 is substantial. In contrast, values found between 0.02 and 0.25 are said to be between weak and moderate. On the other hand, Hair et al. (2016), suggested that with the Q-Square, which is the measure of Stone Geisser’s Cross-Validated Redundancy, that a value is acceptable if found to be more than 0. The generated outcome for these measures is listed in Table 7.
After assessing both the inner and outer models, the hypotheses testing was proposed in Section 2 of the current study. Another advantage of using PLS-SEM is that it computes the significance by following bootstrapping, which involves generating multiple subsets from the data. According to Hair et al. (2016), the recommended number of sub-samples should be 5000. This led to the generation of robust and reliable significance.
Firstly, the effects of leanness were studied with the rest of the variables, as shown in Figure 1. The impact of Leanness on GSCM is found to be positive and significant
Secondly, the role of GSCM in improving organizational performances was evaluated against three aspects: environmental, operational, and economic. All of these relationships were found to be significant and positive at 1% level of significance, which means that enhancing the level of GSCM will improve all of these performances. An improvement in GSCM by 1% will improve the ENP by 17.4%, upgrading in GSCM by 1% will improve the OPR by 15.4%, and improving GSCM by 1% will improve the ECP by 26.1%. These relationships are justified when the organization strives for greenness in its operations and performance. There will be an improvement in the quality of environment as there will be a lesser level of pollution and carbon emissions, thus improving environmental performance. Similarly, due to greenness, there will be less consumption of resources due to its optimization, leading to improved operational performance. Since there will be a reduction in the consumption of resources, it will lead to improved financial performance, which is the organization’s economic performance.
Thirdly, the relationships among the three kinds of performances were evaluated and environmental performance was found to improve operational performance through less pollution and carbon emissions. This is because of the improved environmental performance because of the efficient utilization of resources, which leads to improved operational efficiency performance. Similarly, environmental performance is also found to improve the economic performance, as less pollution and carbon emissions will reap more financial benefits, thus increasing economic performance. Likewise, such operational performance will also lead to improved economic performance because when there is comparatively a lesser investment in resources, there will be an improvement in economic performance. The outcome of the direct relationships is listed in Table 8.
Similar to the outcome of the direct effects listed in Table 8, the indirect effects were also statistically significant for all of the studied relationships (Table 9). According to Giovanni and Cariola (2021), this kind of relationship is evidence of the presence of a long-term effects. This is because regular operations within the organization will continue improvement, elimination of waste, and reduction in non-value-added activities. These improvements in an organization’s environment, operational, and economic performances are already discussed in the direct relationships. The same is seen for the association of GSCM with operational and economic performance and environment with economic performance. Thus, for organizations, the investments made in the lean and GSCM will continue to have benefits over a long period.
In the current study, one of the objectives was to evaluate the role of process innovation through investing in IR 4.0 technologies. Moreover, since that question is categorical, a Multi-Group Analysis was performed. The group is divided into two based on the categories and their path coefficients, and the explanation of variation is compared. If there is a significant difference between the groups, then the role of process innovation is said to be integral; otherwise, its implementation is not recommended.
The outcome generated revealed the role of process innovation in improving GSCM through Lean and improving operational performance through lean. This reflects that through lean, when non-value-added activities are eliminated, standardization in the operations is being done, and delivery time is optimized; it will improve the greenness of the supply chain, whereas these improvements in operations also lead to improving the operational performance. Additionally, the role of process innovation in operational and economic performance is also significant. This reflects that technology innovation in the process also enhances economic performance as it will optimize the consumption of resources and reap enhanced financial and economic benefits. The generated outcome of multi-group analysis for direct effects is listed in Table 10.
The long-term benefits of lean toward improving the operational performance are also reported through the outcome listed in Table 11, which remains legitimate when process innovation through technology is implemented. This suggested that organizations need to invest in the IR 4.0 technologies as it continues to reap benefits over a more extended period. Similarly, this also assists in transforming environmental performance into economic performance. Hence, improving environmental performance for a long time through process innovation powered by IR technologies will enhance financial performance. The generated outcome of multi-group analysis for indirect effects is listed in Table 11.
The surge in competition urges market organizations to instigate innovation and remain relevant and competitive. For that, it is of the utmost importance that organizations have increased productivity and efficiency, which eventually will assist them in attaining and sustaining competitive advantage. Following the restrictions by government agencies and pressure from customers and other related stakeholders, a firm needs to think beyond its own financial gains and benefits. Moreover, the gradual destruction to the quality of the environment and ecology demands firms to have environmental excellence in addition to economic and operational well-being.
The conventional solution to address the operational, economic, and environmental problems is implementing lean and green practices within the supply chains. For operational and economic excellence, firms heavily rely on implementing lean practices. In contrast, to mitigate the adverse repercussions to the environment, the implementation of GSCM practices is beneficial. However, both lean and GSCM share a significant portion of similarity as both philosophies urge to mitigate wastages and elimination of non-value-added activities. However, having the right fit between the two needs to be attained by firms. This is because if a firm opts for improving operational performance and targets the elimination of excessive stock, it will, through reducing the amount of inventory and production, but it increases the startup of the machinery and the level of pollution due to such a rapid startup. On the other hand, when firms opt to consume green inputs, they are likely to increase their financial expenditure as such green products are relatively more expensive than conventional products. Hence, the right strategic fit between leanness and GSCM must be maintained.
To attain the strategic fit, firms need to have technological innovation that are deployed to improve the supply chain processes. Additionally, in the era of IR 4.0 there are several potential solutions available to firms through which organizational excellence in terms of performance can be achieved. However, firms still seem resistant when they are told about innovation, technological advancements, and business operation changes. Therefore, the present study is conducted to explore the role of Industry Revolution 4.0 powered process innovation in enhancing the Leanness, Green Supply Chain Management, and Performance of the Organization (including operational, economic, and environmental). Through the employment of a survey research design, a self-administered questionnaire was formulated, through which 314 responses from supply chain professionals working in ISO 14001 certified Chinese manufacturing companies were received. With the collected data, the application of PLS-SEM is made to assess the nature and significance of direct and indirect relationships, whereas Multi-group Analysis was applied to assess the presence of difference of variances between the relationships of firms willing to implement the process innovation powered by IR 4.0 and the firms not willing to implement. The generated outcome reported significant and positive relationships among the studied hypotheses, whereas, while assessing the interaction effect of technological process innovation, a few of the relationships were found to be significant and supported, which are thoroughly discussed in Section 4.
Based on the findings, the current study offers multiple policy implications. Firstly, the empirical evidence suggests that there is no one-size-fits-all solution regarding the generic level of strategic fit. Firms need to obtain an equilibrium point according to the nature of their business and respective operations. Secondly, both leanness and GSCM enhance the performance; therefore, firms need to promote a culture of innovation, advancements, and continuous improvements through which any sort of structural and/or operational changes are welcomed by the internal and external stakeholders of the supply chain. Thirdly, since the cost of technology and advancements is expensive and firms used to have a low return of investments in the short run, any decision regarding technology implementation should not be backed by short-sightedness and must be given enough time to reap the expected financial, operational, and environmental benefits. Lastly, firms need to encourage the other supply chain partners in terms of their support, acceptance, and transformation towards more environment-friendly initiatives.
Based on the limitations, there are also various future research recommendations. Firstly, firms need to explore the determinants and success factors that can assist in implementing lean and green initiatives. Relevant literature has urged the exploration of the role of top management support, employee commitment, collaboration and integration among the partners, etc. Secondly, the current study is based on data from multiple companies, which may have a certain level of heterogeneity that is, quite obvious because of the change in the nature of the business. Therefore, there is a need to have industry-specific studies to draw precise conclusions and recommendations. The current study explains only linear relationships among the variables in terms of methodology. Thus, exploration through machine learning-based techniques can assist in exploring non-linear variance among the studied phenomena. Lastly, the current study is based on the operationalization of lean and GSCM as generic constructs. Hence, it is recommended to explore the relationships of the practices precisely and separately like eco-design, elimination of waste, reverse logistics, and integrated environmental management.
The data analyzed in this study is subject to the following licenses/restrictions: The data used in this study is a part of funded project and not allowed to share. However, required information can be obtained from Corresponding author. Requests to access these datasets should be directed to the data can be obtained from Corresponding author.
HS and XC equally contributed and completed all thesis together.
The authors declare that the research was conducted in the absence of any commercial or financial relationships that could be construed as a potential conflict of interest.
All claims expressed in this article are solely those of the authors and do not necessarily represent those of their affiliated organizations, or those of the publisher, the editors and the reviewers. Any product that may be evaluated in this article, or claim that may be made by its manufacturer, is not guaranteed or endorsed by the publisher.
Ahmed, W., Ahmed, W., and Najmi, A. (2018). Developing and Analyzing Framework for Understanding the Effects of GSCM on Green and Economic Performance. Manag. Environ. Qual. 29 (4), 740–758. doi:10.1108/meq-11-2017-0140
Ahmed, W., Najmi, A., Arif, M., and Younus, M. (2019). Exploring Firm Performance by Institutional Pressures Driven Green Supply Chain Management Practices. Sasbe 8 (5), 415–437. doi:10.1108/sasbe-04-2018-0022
Ahmed, W., Najmi, A., and Ikram, M. (2020a). Steering Firm Performance through Innovative Capabilities: A Contingency Approach to Innovation Management. Technol. Soc. 63, 101385. doi:10.1016/j.techsoc.2020.101385
Ahmed, W., Najmi, A., and Khan, F. (2020b). Examining the Impact of Institutional Pressures and Green Supply Chain Management Practices on Firm Performance. Manag. Environ. Qual. Int. J. 31 (5), 1261–1283. doi:10.1108/meq-06-2019-0115
Ahmed Khan, S., Kusi-Sarpong, S., Gupta, H., Kow Arhin, F., Nguseer Lawal, J., Mehmood Hassan, S., et al. (2021). Critical Factors of Digital Supply Chains for Organizational Performance Improvement. IEEE Trans. Eng. Manage., 1–15. doi:10.1109/tem.2021.3052239
Bowen, F. E., Cousins, P. D., Lamming, R. C., and Faruk, A. C. (2003). The Role of Supply Management Capabilities in Green Supply. Prod. Operations Manag. 10 (2), 174–189. doi:10.1111/j.1937-5956.2001.tb00077.x
Buer, S.-V., Strandhagen, J. O., and Chan, F. T. S. (2018). The Link between Industry 4.0 and Lean Manufacturing: Mapping Current Research and Establishing a Research Agenda. Int. J. Prod. Res. 56 (8), 2924–2940. doi:10.1080/00207543.2018.1442945
Chen, Y. J., Wu, Y. J., and Wu, T. (2015). Moderating Effect of Environmental Supply Chain Collaboration: Evidence from Taiwan. Int. J. Phys. Distribution Logist. Manag. 45 (9–10), 959–978. doi:10.1108/ijpdlm-08-2014-0183
Chiarini, A., Belvedere, V., and Grando, A. (2020). Industry 4.0 Strategies and Technological Developments. An Exploratory Research from Italian Manufacturing Companies. Prod. Plan. Control, 1–14. doi:10.1080/09537287.2019.1710304
Cohen, J. (1988). Statistical Power Analysis for the Behavioral Sciences. 2nd ed. New York: Academic Press.
Colicchia, C., Creazza, A., and Dallari, F. (2017). Lean and Green Supply Chain Management through Intermodal Transport: Insights from the Fast Moving Consumer Goods Industry. Prod. Plan. Control 28 (4), 321–334. doi:10.1080/09537287.2017.1282642
Cooper, D. R., Schindler, P. S., and Sun, J. (2006). Business Research Methods, 9. New York: McGraw-Hill, 1–744.
De Giovanni, P., and Cariola, A. (2021). Process Innovation Through Industry 4.0 Technologies, Lean Practices and Green Supply Chains. Res. Transp. Econ. 90, 100869. doi:10.1016/j.retrec.2020.100869
De Giovanni, P. (2017). Closed-loop Supply Chain Coordination through Incentives with Asymmetric Information. Ann. Oper. Res. 253 (1), 133–167. doi:10.1007/s10479-016-2334-x
De Giovanni, P. (2016). Coordination in a Distribution Channel with Decisions on the Nature of Incentives and Share-Dependency on Pricing. J. Operational Res. Soc. 67 (8), 1034–1049. doi:10.1057/jors.2015.118
De Giovanni, P., and Ramani, V. (2017). Product Cannibalization and the Effect of a Service Strategy. J. Operational Res. Soc., 1–17. doi:10.1057/s41274-017-0224-5
De Giovanni, P., and Vinzi, V. (2014). The Benefits of the Emissions Trading Mechanism for Italian Firms: a Multi-Group Analysis. Int. J. Phys. Distribution Logist. Manag. 44, 305–324. doi:10.1108/ijpdlm-06-2012-0188
De Giovanni, P., and Zaccour, G. (2019). Optimal Quality Improvements and Pricing Strategies with Active and Passive Product Returns. Omega 88, 248–262. doi:10.1016/j.omega.2018.09.007
Dhingra, R., Kress, R., and Upreti, G. (2014). Does Lean Mean Green? J. Clean. Prod. 85, 1–7. doi:10.1016/j.jclepro.2014.10.032
Dombrowski, U., and Mielke, T. (2012). Lean Leadership - Nachhaltige Führung in Ganzheitlichen Produktionssystemen. ZWF - Z. für Wirtsch. Fabr. 107, 697–701. doi:10.3139/104.110840
Dües, C. M., Tan, K. H., and Lim, M. (2013). Green as the New Lean: How to Use Lean Practices as a Catalyst to Greening Your Supply Chain. J. Clean. Prod. 40, 93–100. doi:10.1016/j.jclepro.2011.12.023
Duhaylongsod, J. B., and De Giovanni, P. (2019). The Impact of Innovation Strategies on the Relationship between Supplier Integration and Operational Performance. Int. J. Phys. Distribution Logist. Manag. 49, 156–177. doi:10.1108/ijpdlm-09-2017-0269
Fornell, C., and Larcker, D. F. (1981). Evaluating Structural Equation Models with Unobservable Variables and Measurement Error. J. Mark. Res. 18 (1), 39–50. doi:10.1177/002224378101800104
Franchetti, M., Bedal, K., Ulloa, J., and Grodek, S. (2009). Lean and Green: Industrial Engineering Methods Are Natural Stepping Stones to Green Engineering. Ind. Eng. IEEE 41 (9), 24–29.
Gefen, D., and Straub, D. (2005). A Practical Guide to Factorial Validity Using PLS-Graph: Tutorial and Annotated Example. Commun. Assoc. Inf. Syst. 16 (1), 91–105. doi:10.17705/1cais.01605
Genc, T. S., and Giovanni, P. D. (2017). Trade-in and Save: A Two-Period Closed-Loop Supply Chain Game with Price and Technology Dependent Returns. Int. J. Prod. Econ. 183, 514–527. doi:10.1016/j.ijpe.2016.07.012
Genc, T. S., and De Giovanni, P. (2018). Closed-loop Supply Chain Games with Innovationled Lean Programs and Sustainability. Int. J. Prod. Econ. 219, 440–456. (in press). doi:10.1016/j.ijpe.2018.05.026
Hair, J. F., Black, B., Babin, B., and Anderson, R. E. (2010). Multivariate Data Analysis 7th. Upper Saddle River, NJ: Pearson Prentice Hall.
Hair, J. F., Hult, G. T. M., Ringle, C., and Sarstedt, M. (2016). A Primer on Partial Least Squares Structural Equation Modeling (PLS-SEM). London, United Kingdom: Sage Publications.
Hair, J. F., Risher, J. J., Sarstedt, M., and Ringle, C. M. (2019). When to Use and How to Report the Results of PLS-SEM. Eur. Bus. Rev. 31 (1), 2–24. doi:10.1108/ebr-11-2018-0203
Henseler, J., Ringle, C. M., and Sarstedt, M. (2015). A New Criterion for Assessing Discriminant Validity in Variance-Based Structural Equation Modeling. J. Acad. Mark. Sci. 43 (1), 115–135. doi:10.1007/s11747-014-0403-8
Hulland, J., Baumgartner, H., and Smith, K. M. (2018). Marketing Survey Research Best Practices: Evidence and Recommendations from a Review of JAMS Articles. J. Acad. Mark. Sci. 46 (1), 92–108. doi:10.1007/s11747-017-0532-y
Inman, R. A., and Green, K. W. (2018). Lean and Green Combine to Impact Environmental and Operational Performance. Int. J. Prod. Res. 56, 1–17. doi:10.1080/00207543.2018.1447705
Ivanov, D., Dolgui, A., and Sokolov, B. (2019). The Impact of Digital Technology and Industry 4.0 on the Ripple Effect and Supply Chain Risk Analytics. Int. J. Prod. Res. 57 (3), 829–846. doi:10.1080/00207543.2018.1488086
Jasti, N. V. K., and Kodali, R. (2015). Lean Production: Literature Review and Trends. Int. J. Prod. Res. 53 (3), 867–885. doi:10.1080/00207543.2014.937508
Kanapathy, K., Aziz, A. A., and Najmi, A. (2022). Industry 4.0 and its Enablers and Barriers: A Systematic and Bibliometric Review of Literature. Int. J. Logist. Syst. Manag. Forthcom. 1, 1. doi:10.1504/ijlsm.2021.10044571
Kirchoff, J. F., Tate, W. L., and Mollenkopf, D. A. (2016). The Impact of Strategic Organizational Orientations on Green Supply Chain Management and Firm Performance. Int. J. Phys. Distribution Logist. Manag. 46 (3), 269–292. doi:10.1108/ijpdlm-03-2015-0055
Kock, N. (2015). Common Method Bias in PLS-SEM. Int. J. e-Collaboration (ijec) 11 (4), 1–10. doi:10.4018/ijec.2015100101
Liu, B., and De Giovanni, P. (2019). Green Process Innovation through Industry 4.0 Technologies and Supply Chain Coordination. WP ESSECBS2019#32.
Martínez-Jurado, P. J., and Moyano-Fuentes, J. (2013). Lean Management, Supply Chain Management and Sustainability: A Literature Review. J. Clean. Prod. 85, 134–150. doi:10.1016/j.jclepro.2013.09.042
Mehmood, S. M., and Najmi, A. (2017). Understanding the Impact of Service Convenience on Customer Satisfaction in Home Delivery: Evidence from Pakistan. Int. J. Electron. Cust. Relatsh. Manag. 11 (1), 23–43. doi:10.1504/ijecrm.2017.10007745
Najmi, A., and Ahmed, W. (2018). Assessing Channel Quality to Measure Customers' Outcome in Online Purchasing. Int. J. Electron. Cust. Relatsh. Manag. 11 (2), 179–201. doi:10.1504/ijecrm.2018.10011240
Najmi, A., Kanapathy, K., and Aziz, A. A. (2019). Prioritising Factors Influencing Consumers' Reversing Intention of E-Waste Using Analytic Hierarchy Process. Int. J. Electron. Cust. Relatsh. Manag. 12 (1), 58–74. doi:10.1504/ijecrm.2019.098981
Najmi, A., Maqbool, H., Ahmed, W., and Rehman, S. A. U. (2020). The Influence of Greening the Suppliers on Environmental and Economic Performance. Int. J. Bus. Perform. Supply Chain Model. 11 (1), 69–90. doi:10.1504/ijbpscm.2020.108888
Najmi, A., Kanapathy, K., and Aziz, A. A. (2021a). Exploring Consumer Participation in Environment Management: Findings from Two‐staged Structural Equation Modelling‐artificial Neural Network Approach. Corp. Soc. Responsib. Environ. Manag. 28 (1), 184–195. doi:10.1002/csr.2041
Najmi, A., Kanapathy, K., and Aziz, A. A. (2021b). Understanding Consumer Participation in Managing ICT Waste: Findings from Two-Staged Structural Equation Modeling-Artificial Neural Network Approach. Environ. Sci. Pollut. Res. 28 (12), 14782–14796. doi:10.1007/s11356-020-11675-2
Najmi, A., Kanapathy, K., and Aziz, A. A. (2022). A Pathway to Involve Consumers for Exchanging Electronic Waste: a Deep Learning Integration of Structural Equation Modelling and Artificial Neural Network. J. Mater Cycles Waste Manag. 24 (1), 410–424. doi:10.1007/s10163-021-01332-2
Podsakoff, P. M., MacKenzie, S. B., Lee, J.-Y., and Podsakoff, N. P. (2003). Common Method Biases in Behavioral Research: a Critical Review of the Literature and Recommended Remedies. J. Appl. Psychol. 88 (5), 879–903. doi:10.1037/0021-9010.88.5.879
Podsakoff, P. M., MacKenzie, S. B., and Podsakoff, N. P. (2012). Sources of Method Bias in Social Science Research and Recommendations on How to Control it. Annu. Rev. Psychol. 63, 539–569. doi:10.1146/annurev-psych-120710-100452
Preeker, T., and De Giovanni, P. (2018). Coordinating Innovation Projects with High Tech Suppliers through Contracts. Res. Policy 47 (6), 1161–1172. doi:10.1016/j.respol.2018.04.003
Ramani, V., and De Giovanni, P. (2017). A Two-Period Model of Product Cannibalization in an Atypical Closed-Loop Supply Chain with Endogenous Returns: The Case of DellReconnect. Eur. J. Operational Res. 262 (3), 1009–1027. doi:10.1016/j.ejor.2017.03.080
Rao, P. (2002). Greening the Supply Chain: A New Initiative in South East Asia. Int Jrnl Op Prod Mnagemnt 22 (6), 632–655. doi:10.1108/01443570210427668
Rao, P., and Holt, D. (2005). Do green Supply Chains Lead to Competitiveness and Economic Performance? Int. J. Operations Prod. Manag. 25 (9), 898–916. doi:10.1108/01443570510613956
Razzaq, A., Sharif, A., Najmi, A., Tseng, M.-L., and Lim, M. K. (2021). Dynamic and Causality Interrelationships from Municipal Solid Waste Recycling to Economic Growth, Carbon Emissions and Energy Efficiency Using a Novel Bootstrapping Autoregressive Distributed Lag. Resour. Conservation Recycl. 166, 105372. doi:10.1016/j.resconrec.2020.105372
Ringle, C. M., Wende, S., and Becker, J. M. (2015). SmartPLS 3. SmartPLS GmbH, Boenningstedt. J. Serv. Sci. Manag. 10 (3), 32–49.
Vachon, S., and Klassen, R. D. (2006). Extending Green Practices across the Supply Chain. Int. J. Operations Prod. Manag. 26 (7), 795–821. doi:10.1108/01443570610672248
Venkat, K., and Wakeland, W. (2006). “Is Lean Necessarily Green?; Pdf from Website,” in Proceedings of the 50th annual meeting of the ISSS, Sonoma, CA, USA, June 2006.
Womack, J. P., Jones, D. T., and Ross, D. (1990). The Machine that Changed the World. New York: Rawson Associates.
Wong, C. Y., Wong, C. W., and Boon-Itt, S. (2015). Integrating Environmental Management into Supply Chains. Int. J. Phys. Distribution Logist. Manag. 45 (1/2), 43–68. doi:10.1108/ijpdlm-05-2013-0110
Keywords: industry revolution 4.0, COVID-19, technology innovation, green supply chain, environmental performance
Citation: Sun H and Chu X (2022) Industry 4.0 Powered Process Technology Innovation, Firm’s Leanness, and Eco-Environmental Performance During the COVID-19 Phase. Front. Environ. Sci. 10:902182. doi: 10.3389/fenvs.2022.902182
Received: 22 March 2022; Accepted: 12 April 2022;
Published: 31 August 2022.
Edited by:
Asif Razzaq, Dalian University of Technology, ChinaReviewed by:
Mohsin Shahzad, Dalian University of Technology, ChinaCopyright © 2022 Sun and Chu. This is an open-access article distributed under the terms of the Creative Commons Attribution License (CC BY). The use, distribution or reproduction in other forums is permitted, provided the original author(s) and the copyright owner(s) are credited and that the original publication in this journal is cited, in accordance with accepted academic practice. No use, distribution or reproduction is permitted which does not comply with these terms.
*Correspondence: Xiao Chu, Y2h1eGlhbzk5OTlAc2luYS5jb20=
Disclaimer: All claims expressed in this article are solely those of the authors and do not necessarily represent those of their affiliated organizations, or those of the publisher, the editors and the reviewers. Any product that may be evaluated in this article or claim that may be made by its manufacturer is not guaranteed or endorsed by the publisher.
Research integrity at Frontiers
Learn more about the work of our research integrity team to safeguard the quality of each article we publish.