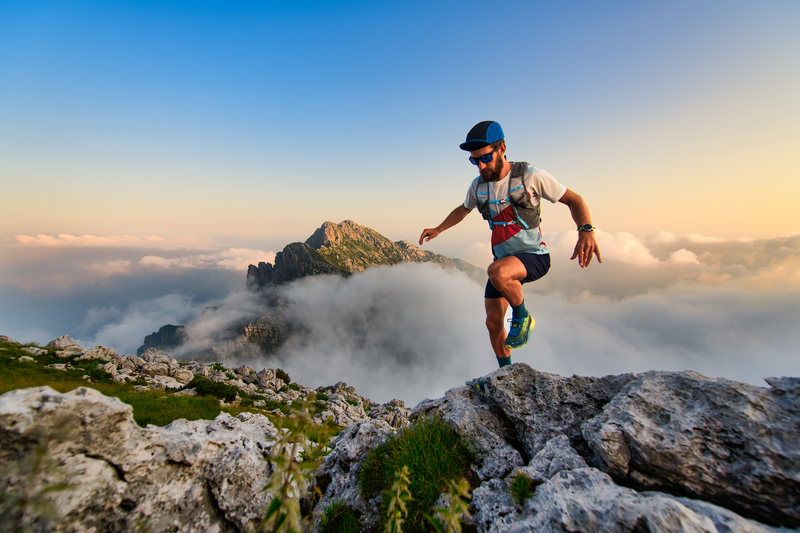
94% of researchers rate our articles as excellent or good
Learn more about the work of our research integrity team to safeguard the quality of each article we publish.
Find out more
ORIGINAL RESEARCH article
Front. Environ. Sci. , 19 May 2022
Sec. Environmental Economics and Management
Volume 10 - 2022 | https://doi.org/10.3389/fenvs.2022.901844
This article is part of the Research Topic Green Innovation and Industrial Ecosystem Reconstruction in Achieving Environmental Sustainability View all 34 articles
Improving farmers’ green production behavior can guarantee food safety at the source. In recent years, a rising number of studies have focused on food safety management and have provided general regulation recommendations. Unlike many studies, this study aimed to find targeted policy recommendations according to different spatial aggregations of non-green production behavior. In the current study, more than 800 tea farmers located in the Qinba and Huangshan Mountain regions of China were investigated. An order logit model was employed to evaluate the impact of government regulation and community governance on ignorant or unkind non-green tea production behavior. Furthermore, a multi-valued treatment effect model was also recruited to demonstrate the average treatment effect of government regulation and community governance. The results show that the difference in farmers’ ignorant or unkind non-green production behavior between regions is substantial but is similar in the same region. Farmers’ non-green production behavior is negatively impacted by community governance but not significantly affected by government regulation. Government regulation can effectively inhibit the occurrence of farmers’ unkind behavior, while community governance can effectively cause farmers’ unkind behavior. Thus, some measures to reduce local farmers’ non-green production behavior should be put forward according to the differences in the spatial distribution of non-green production behavior and the differences in the effect of government regulation and community governance.
Improving food safety and protecting the agricultural environment is widely deemed as an important mission, which is close to the true life of the smallholders. By governments and local communities, vigorous efforts are being made to reduce the pesticides usage in agricultural activities (Liu et al., 2015; Kumar et al., 2019). Currently, the residues of these chemicals have been detected in various food, such as vegetables, fruits, even tea (Seenivasan and Muraleedharan, 2011; Amirahmadi et al., 2013). Thus, strict food safety standards have become an important measure to ensure food safety (Fan et al., 2014). According to the statistics of the Food and Agriculture Organization (FAO), China was the largest consumer of the pesticides globally. The annual average pesticide use is approximately 0.067 kg/ha, which is more than twice consumption compared to some developed countries. Meanwhile, the rate of effective pesticide use is only 35%, which is well below developed countries’ average of 50∼60% (Grung et al., 2015).
For tea and its culture in China, there was a long history since tea originated. The Qinba Mountains and Huangshan Mountains are the central areas for tea cultivation. According to survey, various diseases and pests will be encountered in tea planting, such as small green leafhoppers, tea stink bugs, tea coal diseases, etc. Therefore, excessive pesticides have been applied in the actual production process to ensure tea production. According to the report from Greenpeace (Greenpeace, 2016), nearly 60% of normal tea was detected with prohibited pesticides, while 85% of normal tea was evaluated as overproof pesticide residue, and this will seriously threat the reputation of Chinese tea in the global market. As an essential part of the agricultural industry in China, the tea sector is supposed to thrive as an endogenous industry and, therefore, enrich the environment and reduce the rural poverty level in the different tea regions of China. According to the FAO, China exported 355,258 tons of tea in 2017, which is significantly higher than any other tea-exporting countries nearby, such as Sri Lanka, India, and Vietnam. In addition, China is also the second largest tea exporter in the world, following Kenya. Thus, it is essential to regulate tea farmers’ overuse of pesticides to guarantee food safety. For the Chinese management system of agricultural products, regulation development generates an important role in the supply chains, while the safety and quality control of agricultural products is also essential (Dou et al., 2015). The Chinese government has adopted a series of measures to regulate farmers’ non-green production behavior. For example, the Ministry of Agriculture implemented the “Year of Supervision on Agricultural Food Safety and Quality” in 2014, carrying out strict supervision and special rectification on pesticide applications. Furthermore, the Ministry of Agriculture of China introduced “Zero Growth of Chemical Fertilizer and Pesticide Use”, such action aimed to achieve a 40% pesticide utilization rate, as well as a zero increase in pesticide and fertilizer use in the year of 2020 (Shuqin and Fang, 2018). Similarly, in 2019, several major departments represented by the “Ministry of Agriculture and Rural Affairs” formulated a national food quality standard for agricultural products, achieving a 98% detection pass rate and a 41% pesticide utilization rate until the year 2020. Based on agricultural fitness cultivation and ecological control, and chemical control as an emergency prevention and control strategy, the local government coordinated the application of physical control, immune inducement and biological control measures starting from the tea garden ecosystem. Farmers’ non-green production behavior can be caused by ignorance and unkind psychology, which influenced by community and government regulation, respectively. Adhere to local conditions and zoning management, focusing on the prevention and control of major diseases and pests such as tea net bugs. To ensure the quality and safety of tea products as well as the ecological environment of tea gardens, scientific, safe and reasonable methods were advocated.
Government regulation plays an important role in boosting farmers’ pesticide reduction behavior (Yu, 2012). Government regulation is mainly implemented for the control of pesticide use, including detecting residual pesticides, punishing the use of illegal pesticides, and prohibiting the use of highly toxic pesticides. However, due to the special model of small-scale family management in China, the cost of government regulation will be substantial. Community governance is expected to enhance farmers’ access to more food safety and green production rule knowledge, to help farmers use new green production technology (Ostrom, 1996). However, without a healthy institutional environment provided by government, the community cannot well develop their ability (Lam, 1996). Therefore, to regulate the pesticide use in tea farmers, it is important to explore the cooperation between governments and community in China.
According to the published studies, many different factors affect farmers’ pesticide overuse behaviors (Ferrier and Lamb, 2007; Hafezi and Zolfagharinia, 2018). We made an attempt to determine the impact of government regulation on farmers’ pesticide overuse, and found that controlling government regulation is a direct method to regulate farmers’ pesticide overuse, for which the government in China bears a substantial regulation cost at the small-scale household level (Lamichhane, 2017; Liu and Lan, 2018). Many published studies have found that the regulation cost is greater than the regulation gain because the regulatory outcomes deviate from the regulatory objectives, showing the inefficiency in the regulations (Antle, 2000; Lichtenberg, 2013; Miewald et al., 2013). There is a strong link between reputation, quality, another characteristic etc. and agriculture products in Geographical Indication region (Barham, 2003; Likudis et al., 2013), Thus, in the Geographical Indication regions with pesticide overuse, stricter standards on agricultural products have been applied by the government and community (Drogue and DeMaria, 2012). In addition, to ensure the quality and value of public agricultural product brand, the community and local government are more motivated to attach importance to supervising farmers’ pesticide overuse behavior and urging farmers to abide strictly by the technical specifications for the quality control of Geographical Indication products (Meredith and Willer, 2016). This paper provides a detailed description, explanation, and prediction on farmers’ green production behavior in two regions. Based on the above descriptive analysis and regression results, we also provide targeted policy recommendations for the government and community.
Current published researches primarily focused on the aspects mentioned above. However, the farmers’ psychological state and location condition were ignored, resulting in the low regulatory efficiency with a one-size-fits-all approach. According to farmers’ psychological state, farmers’ non-green production behavior in the present study was divided into ignorant and unkind behavior. In addition, according to the differences in spatial distribution, the ArcGIS10.4.1 software was employed to draw ignorant and unkind pesticide use maps. Finally, an order logit model was recruited to verify the effect of government regulation and local community governance on ignorant and unkind pesticide use, and a multi-valued treatment effect model was also conducted to analyze the average treatment effect of government regulation and community governance. Based on the evaluated results, a targeted policy was obtained.
By affecting the cost and benefit from the production of smallholder farmers, government regulation and community governance can influence agricultural performance. This study conducted the Institutional Analysis and Development (IAD) framework for reference. The analytical framework of potential pathways was illustrated in Figure 1. The IAD framework provides a means for decision-making relative to the integrated tea farmers reducing their pesticide usage and the outcome of this beyond the local level, where actors such as the government, the community, and the market make decisions regarding local government institutions and local natural resources (Ostrom, 2005).
In this multilevel arena, the actors (e.g., the government, community, and market) come together to determine what regulation should be made in a particular situation. This determines a result (a combination of farmers’ pesticide use) that feeds back into exogenous variables (e.g., natural resources, governance institutions, and rule markets) in an action situation formulated by the actors themselves and an action situation that is fitted (Figure 1).
The action arena is a virtual platform where actors make interactions in a particular action situation. An action situation usually remains relatively stable with time in comparison to participating actors. Based on the roles of actors (the government, the community, and the market) who directly affect farmers’ non-green production behavior, this study aimed to analyze how actors’ interactions in an action situation cause farmers’ pesticide overuse. Regardless of the specific goal of farmers reducing pesticide use, the actions taken or not taken affect food safety, common brand value, and the agricultural environment (Minten et al., 2013).
Farmers’ pesticide overuse can be decomposed into two steps: the first step is to determine non-green production behavior, which means determining whether farmers have used overuse pesticides in the last 5 years; the second step is to determine whether these farmers are ignorant or unkind. Such classification aims to explore the underlying reason for non-green production behavior and to take suitable measurements of local conditions according to the spatial agglomeration of different methods of pesticide use in different exogenous environments.
In addition to actors influencing farmers’ behavior through their norms, values, and rules, exogenous variables can generally drive an action situation (Ostrom, 2005). Actors’ governance measures or rules can exert a different effect in a particular action arena due to different exogenous environments (Basurto et al., 2013). The categorization of these exogenous variables helps to efficiently analyze actors’ measures and actions (Figure 1). One strategy is to determine which natural resource allocations, institutions of the government and community, and market rules form the action situation’s components (Whaley and Weatherhead, 2014).
Natural resources refer to the foundational abiotic environment, such as the altitude, the geographic location, or the history of planting tea in a certain area, where actors and farmers perform their work. How a natural resource affects the action arena largely depends on the governance institution (Carazo-Rojas et al., 2018), which consists of local government regulation, committee publicity, and supervision from other farmers. Therefore, the action situation is the action arena in which actors can easily combine exogenous variables using an interaction model and subsequently have an effect on farmers’ non-green production behavior (Arias et al., 2014). This study combined informal rules with formal rules to regulate these farmers’ behavior. Informal rules are similar to formal rules in that they guide actors in deciding what is allowed (Ostrom et al., 1993). It is necessary to address a strategy for how governance power can be distributed so as to shape farmers’ pesticide use and how an efficient regulation of farmers’ behavior can be achieved, aimed specifically at different regions.
1) Order Logit Model
It is particularly important to identify government and community goverance effects that may arise in the farmers’ green production. An order logit model is adopted as the predict model used to estimated the influence of government and community goverance effects on farmers’ green production. This model is constructed as follows:
Where
2) Multi-Valued Treatment Effect Model
According to Linden et al. (Linden and Yarnold, 2016) multiple estimation strategies were provided by multivalued treatment effect models, including regression adjustment (RA)、inverse-probability weighting (IPW)、augmented inverse probability weighting (AIPW) and inverse probability weighted regression adjustment (IPWRA). From the perspective of promoting farmers’ green production behavior by government and community, this paper further analyzes the effect of policies more accurately, following Cattaneo (Cattaneo, 2010), a multi-valued treatment effect model (IPWRA) was used to analyze the average treatment effect of government regulation and community governance. The model is as follows:
Where
The data used in this study were taken from a survey of household tea farming between July and August 2018 in China. A multistage sampling procedure was used to collect the data. First, we selected two regions with long traditions of tea cultivation and that are home to the most famous common brands: the Qinba Mountain region (Shaanxi and Sichuan provinces; Figure 2A) and the Huangshan Mountain region (Anhui and Zhejiang provinces; Figure 2B). Almost all farmers in these regions are small-scale tea producers and are engaged in tea production and marketing for their livelihoods. Importantly, these regions show high densities of common tea brands and Geographical Indication Protection. They are also leading regions in the production of tea because they are endowed with the same climatic conditions. The first region, the Qinba Mountain region, located in the west of China, is a low-economic-status and poverty-stricken area in China. The second region, the Huangshan Mountain region, located in the east of China, is well known for its high-economic-status areas. Second, seven districts with a high density of tea production at the provincial level were selected, including the Ziyang, Xixiang, and Nanzheng counties in Shaanxi; the Wanyuan and Qingchuan counties in Sichuan; the Kaihua county in Zhejiang; and the Huangshan and Qimen counties in Anhui. Third, six villages from each county were randomly selected. Finally, approximately 16–20 farmers from each village were randomly selected according to their income level. In total, a sample of 818 farmers was collected using the household survey.
Before conducting questionnaire-based interviews, we trained the investigators. Face-to-face interviews were conducted by the investigators who spoke both the local language and Mandarin. The questions covered family information, labor input, land input, pesticide and fertilizer input, outcome variables, Geographical Indication Protection, and numerous other topics. The final dataset comprised 498 farmers from the Qinba Mountain region and 320 farmers from the Huangshan region.
(1) Green and Non-Green Production Behavior
According to tea farmers’ spatial data with the “PRA+3 S” methods, ArcGIS10.4.1 was used to draw a spatial aggregate of farmers’ green and non-green production behavior, shown in Figure 3. The figure shows that farmers’ green and non-green production behavior presents obvious spatial aggregates in different regions. If the farmers have no overuse pesticides in recently 5 years, we defined it as green production behavior. If the farmers have overuse pesticides in recently 5 years, we defined it as non-green production behavior. The red points represent more pesticide overuse, and the green points represent more pesticide reduction behavior. The findings suggest that farmers’ non-green production behavior is similar in the same region but is substantially different between different regions. The most interesting result shown in Figure 3 is that farmers located in Shaanxi province engage in more green production. In contrast, farmers located in Sichuan, Anhui, and Zhejiang have more non-green production behavior.
2) Farmers’ Psychological State
FIGURE 3. Spatial aggregates of farmers’ green or non-green production behavior: (A) Qinba Mountain and (B) Huangshan Mountain.
To explore the reason for farmers’ non-green production behavior more in-depth, we divided them in to two groups (behavior of ignorance or behavior of unkindness) according to famers’ psychological behavior. Firstly, farmers’ green production knowledge and green or no-green production behaviors were captured in investigation. According to the deviation of knowledge and behaviors, if the famers have a low level of green production knowledge and have non-green production behavior, we defined it as the behavior of ignorance. But if the famers have a high level of green production knowledge and still have non-green production behavior, we defined it as the behavior of unkindness. Figure 4 presents the spatial aggregates of farmers’ ignorant and unkind behavior. The red points represent unkind behavior, and the green points represent more ignorant behavior. This shows that behavior also presents obvious spatial aggregates in different regions, especially in the county. Figure 4 shows that farmers located in Shaanxi and Sichuan are less unkind and that farmers located in Anhui and Zhejiang are unkinder. This paper provides a detailed analysis of why there is a substantial difference between similar regions, how governance power is distributed to shape farmers’ non-green production behavior, and how efficient regulation of farmers’ behavior can be achieved. We tried to design a strategy that adapts to local conditions.
3) Government Regulation
FIGURE 4. Spatial aggregates of farmers’ ignorant or unkind non-green production behavior: (A) Qinba Mountain and (B) Huangshan Mountain.
Government regulation means government takes measures (e.g., laws, punishment policy or quality supervision) on the whole production process of farmers. In this article, “if government regulates farmers’ pesticide use” is used as a variable description of government regulations to explore the impact of government regulations on farmers’ green production behaviors. According to previous research, government regulation plays a key role in changing farmers’ crop production behavior, particularly non-green production behavior (Yue et al., 2010). Many countries have proposed government regulation policies to ensure food safety and to reduce environment pollution (Bhandari et al., 2019). As shown in Figure 5, the survey data indicate that 48.8% of farmers in Shaanxi, 20.9% in Sichuan, 17.4% in Anhui, and only 1.6% in Zhejiang are regulated by the government. Therefore, government regulation in Shaanxi and Sichuan is significantly higher than that in Anhui and Zhejiang, and that is similar to the spatial aggregates of farmers’ ignorant and unkind behavior. Based on the empirical statistical analysis, we can hypothesize that government regulation can influence farmers’ ignorant and unkind behavior. This hypothesis is empirically verified in the next section.
4) Community Governance
Community governance refers to an institutional system in which multiple entities such as village committees and farmers participate in the governance of farmers’ production behaviors. In this article, community governance refers to the process in which the village committee, farmers, and assembly jointly supervise the production of farmers. Currently, community governance is one of the most important actors of the rural revitalization strategy. Community governance can effectively regulate farmers’ behavior, and it can share the government’s financial pressure. Figure 6 shows the community governance performance in different provinces. According to Figure 6, farmers in Shaanxi show the most advanced management, which is similar to the spatial aggregate of farmers’ non-green production behavior. That is to say, there appear to be similar trends among community governance and non-green production behavior. This presents the hypothesis that community governance has a significantly negative effect on farmers’ non-green production behavior. This hypothesis is empirically verified in the next section.
5) Farmers’ Total Characteristics
Supplementary Table S1 reports the descriptive statistics for the variables used in this study. The farmers engaging in green production behavior show a green production value of 1.4866, which means that the level of green production of farmers is normal. Twenty-seven percent of farmers have regulated their pesticide use due to the government. This suggests that the government regulation of pesticide use is still in its initial stage. It is expected that community governance will do its best to increase low regulator levels. Community governance includes farmers’ supervision, community publicity, and assembly supervision. Farmers’ supervision represents the degree of supervision between farmers. Supplementary Table S1 shows that the farmers’ supervision is approximately 3.2983, which means that farmers’ supervision is at a high level and can regulate farmers’ behavior in most cases. Only 26% of farmers have been accepted for public training by the community. Meanwhile, only 29% of farmers need to be checked for tea quality by the assembly. Therefore, the statistics show that community public training and assembly supervision are both at a low level and need to be reinforced in the next step.
This section shows the impacts of government regulation and community governance on farmers’ pesticide use. Farmers’ pesticide use is divided into two parts: green production behavior and non-green production behavior, which is either ignorant or unkind. To explore how governance power and community governance can shape pesticide use and how the efficient regulation of farmers’ behavior can be achieved, order logit models were used. The results are shown in Supplementary Table S2.
Supplementary Table S2 shows the effect of government regulation and community governance on green production behavior. The variable representing government regulation has a positive and significant impact on green production behavior. As expected, the variable for government regulation has a significant negative impact on farmers’ unkind behavior, suggesting that government regulation can prevent unkind behavior. However, the variable representing community governance has a positive and statistically significant effect on unkind non-green production behavior, suggesting that community governance could lead to more unkind non-green production behavior than green production. The reason for this is that, though the community can improve farmers’ knowledge of green production, there is no strict constraint on farmers’ behavior. Farmers whose goal is to maximize their interests will naturally choose unkind behaviors that benefit them. They contribute to diverse governance measures that adapt to different regions according to the differences in non-green production behavior, ignorant or unkind. This is associated with less non-green production behavior, which contributes to providing a strategy that improves the degree of green production in agriculture (Kayhan, 2015).
Moreover, the variables representing party members have a positive and significant impact on farmers’ green production behavior. The reason for this is that a farmer who is a member of the party is more politically inclined to produce green products. This positive impact of being a party member and the degree of information on reducing farmers’ non-green production behavior is in line with previous studies (Schreinemachers et al., 2016). The degree of information has a positive and significant impact on farmers’ unkind non-green production behavior. This supports findings that information can improve farmers’ knowledge of green production in terms of the convenience of the acquisition of said knowledge as well as multi-party market information. Furthermore, a higher degree of information could reduce speculation costs and influence farmers’ values. This shows that farmers’ correct values and positive attitudes on green production would effectively reduce farmers’ unkind behavior. The findings further confirm that the cost of acquired information is associated with unkind behavior. In addition, the variables representing the brand traceability system and the rate of tea income significantly accelerate green production behavior. Meanwhile, the tea income of farmers is the biggest motivations throughout the production process. Ensuring higher tea income is the key to ensuring green production for farmers.
The differential promotion effect of government regulation and community governance on farmers’ green production was estimated using the order logit model. Aimed at assessing the impact of policies more accurately, a multi-valued treatment effect model was used to analyze the average treatment effect of government regulation and community governance. This model was also used to check the robustness of the obtained results. Inverse probability weighted regression adjustment (IPWAR) was adopted to analyze the average treatment effect of government regulation and community governance. The results are shown in Supplementary Tables S3, S4.
Supplementary Table S3 shows that farmers’ green production behavior is significantly promoted by government regulation. The ATE value is 0.1393, and the level of significance is 5%. This means that government regulation can promote farmers’ green production behavior and significantly restrain farmers’ unkind behavior.
Supplementary Table S4 shows that community governance has a negative effect on farmers’ green production behavior. This means that community governance can promote farmers’ non-green production behavior. Moreover, this could lead to farmers’ unkind behavior. The green production behavior of surrounding farmers has a significant positive average treatment effect on farmers’ unkind behavior. If there is a higher level of green production among the surrounding farmers, more farmers engage in unkind behavior. This is consistent with the above research conclusion. However, community publicity and assembly supervision have no significant average treatment effect on farmers’ green production behavior.
Farmers’ pesticide use is affected by various factors. Among them, government regulation and community governance have a greater impact. However, targeted government regulation and community governance institutions are necessary to guarantee food safety at low financial costs.
The results obtained from the descriptive statistics suggest that there is a substantial difference in pesticide use between county regions, regardless of the green production behavior or ignorant and unkind behavior. Sun, H et al. (Sun et al., 2020) also indicated that the behavior of club clusters would be formed in a sub sample of every decade. As shown in Figure 3, farmers’ behavior shows an obvious spatial agglomeration, which means that green or non-green production behavior is similar in the same geographically indicated protected areas. There is a considerable difference in spatial accumulation in the different geographically indicated protected areas. Therefore, it is particularly important to formulate differentiated regulatory policies for different geographically indicated protected areas. This lends credit to the idea that community governance has a significant inhibiting effect on farmers’ pesticide overuse. Therefore, in Geographical Indication Protection areas with serious pesticide overuse, attention should be paid to the regulatory role of community governance. However, empirical research also shows that community governance aggravates the occurrence of unkindness. Among the farmers who apply excess pesticides, the above discussion also shows that government regulation can effectively restrain the unkind behavior of farmers.
Formal and informal institutions (e.g., government regulation, community governance, and market rules) create these governance power relationships. Moreover, government actors directly design the formal institutions that are subsequently implemented and then distributed by the community, which plays a complementary role. Formal and informal institutions are both identified as core driving forces for improving farmers’ green production in agriculture (Casson et al., 2010). For instance, the empirical result shows that, given the positive and significant role of community governance in inhibiting farmers’ non-green production behavior, the power of community governance should be further enhanced and distributed while government regulation can significantly prevent farmers’ unkind behavior. However, community governance can significantly exacerbate unkind behavior. The main reason for this is that, while community governance can improve farmers’ knowledge, there is no strict law constraint on farmers’ behavior. Consequently, community governance should not be enhanced in regions where farmers’ unkind behavior is aggregated.
Government regulation plays an essential role in smallholders’ behavior in many developing countries. While numerous studies have estimated the impact of government regulation on non-green production behavior, little attention has been paid to exploring how community governance with government regulation affects pesticide use. There is also a lack of awareness of efficient measures according to different spatial agglomeration. In addition, there is no literature on the subdivision of pesticide overuse into ignorant and unkind behavior. The existing literature has found many interaction patterns and outcomes among actors (local governments, community, and the market) within institutions created with national resources and local governance systems. By applying the IAD theoretical concepts, this study analyzed these interactions in a coherent manner. The results of the case study in China showed that, although the measures and relationships of governments and communities vary, farmers’ pesticide use decisions are shaped by a similar set of exogenous variables that result in the use of different governance approaches. Both the institution’s role and rules are core drivers of these various behaviors (Brodrechtova et al., 2018). The description of the sample and the behaviors’ spatial aggregates indicate a difference in national resources.
Combined with the descriptive statistics and empirical results, formulating adjusted measures to local conditions is necessary. For instance, in Shaanxi and Zhejiang, more attention should be paid to community governance. However, the pesticide use of farmers located in Sichuan and Anhui should be regulated by the government due to a higher probability of unkind behavior. Community governance can efficiently prevent farmers’ non-green production behavior, and government regulation can efficiently prevent farmers’ unkind behavior if farmers present non-green production behavior.
One of the key steps in distributing governance power to shape farmers’ pesticide use was achieved by empirical analysis. As indicated in Section 3.1 and Section 3.2, another key step is the efficient regulation of farmers’ non-green production behavior, making adjustments based on local conditions. Therefore, based on the spatially distributed differences in pesticide use and in the ignorant and unkind behavior of farmers, policy suggestions suitable for different Geographical Indication reserves would be put forward.
Most scholars have only used a single mothed to analyze farmers’ green production. However, this study applied an order logit model, a multi-value treatment model, and the “PRA+3 S” method to analyze Qinba Mountain and Huangshan Mountain data. Aimed at exploring the differences in farmers’ green or non-green production behavior between the two mountain regions, this study further explored the effect of government regulation and local community governance on reduced pesticide use behavior. Another essential objective of this study was to assess how the local government can cooperate with the local community to achieve the objective of reduced pesticides and to formulate government and community policies that vary according to resource endowments in different regions.
Geographically, the research on the spatial agglomeration behavior of farmers is relatively extensive, but it is rare in management. This paper studies the cross-integration of geography and management Figure 3 clearly shows that farmers located in Shaanxi and Zhejiang have a greater probability of reducing pesticides under the present governance. Moreover, Figure 4 shows another spatially aggregated phenomenon that suggests that farmers located in Sichuan and Anhui have a greater probability of engaging in non-green production behavior due to unkindness.
According to the results of the IAD framework, the effects of the actors (e.g., the government, community, and market) come together to determine what regulation should be made in a particular situation. Other studies that only attention on one of the actors, for instance, Ziheng Niu et al. (Niu et al., 2022) found that community peer effects could improve farmers’ adoption of cleaner production technology, Fuduo Li et al. (Li F. et al., 2020) found that government compensates does let farmers’ aware of more environmental value of the Green Manure Planting Program. Meanwhile, no scholar divided non-green production behaviors into ignorant and unkind behavior for in-depth research. However, the relative impact of government and community governance across studies, which is expected to propose targeted policies to improve farmers’ green production behavior efficiently based on the spatially aggregated phenomenon and mental behavior. Our results indicate that farmers’ non-green production behavior is negatively impacted by community supervision but are not significantly impacted by government regulation. Although some references found that community supervision had a greater impact on the perceived value-green production willingness path of farmers’ agricultural green production than the variable non-community supervision (Li M. et al., 2020). Our results also show that unkind intentions cause most farmers to increase their application of pesticides. Farmers’ unkind behavior is negatively impacted by government regulation and positively impacted by community supervision. Community supervision improves farmers’ knowledge and thus reduces the possibility of ignorance.
A one-size-fits-all policy can significantly increase fiscal costs. On the contrary, the proposal of targeted policies can not only reduce the cost of government governance, but also effectively improve the efficiency of regulation. Therefore, it is necessary to propose targeted policy in this study. Targeted policy can be formulated by combining descriptive statistics and empirical results, for instance, the finding that, in Shaanxi, community governance should be paid more attention than in Zhejiang province. However, the pesticide use of farmers in Sichuan and Anhui should be regulated by the government due to a higher probability of unkind behavior. More precisely, community governance can efficiently prevent farmers’ non-green production behavior and government regulation can efficiently prevent farmers’ unkind behavior. Similarly, Ataei et al. (Ataei et al., 2021) noted that farmers’ mental behavior (perceived benefits, perceived susceptibility, moral norms and self-identity) influenced the intention to use green pesticides. Therefore, our findings show that the government and community are associated with pesticide use. Hence, government regulation and other policy incentives for smallholder farmers to choose green and eco-friendly production methods in agriculture contribute to inclusive food safety and environmental protection for China.
Government should strengthen monitoring and statistics of agricultural production data, and construct a more scientific and rational agricultural green production measurement system (Liu et al., 2020). Our results have essential policy implications. The results of this study show important factors that affect farmers’ green production behavior and find efficient governance measures that adapt to local conditions. Therefore, our findings show that formal and informal institutions are associated with pesticide use. Multi-valued treatment effect analysis results show that the ATE value of government regulation on farmers’ green production is 0.1393, and the level of significance is 5%. This means the probability of farmers with government regulation to adopt green production behaviors increases by 13.93% and significantly restrain farmers’ unkind behavior. Hence, government regulation and other supplemental policies serve as incentives for smallholder farmers to adopt green production technology and to contribute to the objective of inclusive food safety and environmental protection for China (Orcos et al., 2018). Meanwhile, long-term policies with increased technological progress can address green production issue (Sun et al., 2021). Furthermore, government regulation and extension policy (e.g., community governance) could allow for farmer training and supervisory programs to enhance farmers’ knowledge on pesticide reduction. These are necessary conditions to ensure rural food safety and environmental protection and to develop the economy of tea farmers in a rapidly changing environment.
The original contributions presented in the study are included in the article/Supplementary Material, further inquiries can be directed to the corresponding author.
Conceptualization, YY and HL. Methodology, YY and YH. Formal analysis, YY and TL. Investigation, YY. Data curation, YY and HL. Writing—original draft preparation, YY. Writing—review and editing, YY and KM. Supervision, HL. Project administration, HL. Funding acquisition, HL. All authors read and agreed to the published version of the manuscript.
This work was financially supported by the Humanities and Social Science Foundation of the Ministry of Education (21YJC630158).
The authors declare that the research was conducted in the absence of any commercial or financial relationships that could be construed as a potential conflict of interest.
All claims expressed in this article are solely those of the authors and do not necessarily represent those of their affiliated organizations, or those of the publisher, the editors and the reviewers. Any product that may be evaluated in this article, or claim that may be made by its manufacturer, is not guaranteed or endorsed by the publisher.
The Supplementary Material for this article can be found online at: https://www.frontiersin.org/articles/10.3389/fenvs.2022.901844/full#supplementary-material
Amirahmadi, M., Shoeibi, S., Abdollahi, M., Rastegar, H., Khosrokhavar, R., and Hamedani, M. P. (2013). Monitoring of Some Pesticides Residue in Consumed Tea in Tehran Market. J. Environ. Health Sci. Eng. 10 (1), 9. doi:10.1186/1735-2746-10-9
Antle, J. M. (2000). No Such Thing as a Free Safe Lunch: The Cost of Food Safety Regulation in the Meat Industry. Am. J. Agric. Econ. 82 (2), 310–322. doi:10.1111/0002-9092.00027
Arias, L. A., Bojacá, C. R., Ahumada, D. A., and Schrevens, E. (2014). Monitoring of Pesticide Residues in Tomato Marketed in Bogota, Colombia. Food C 35 (1), 213–217. doi:10.1016/j.foodcont.2013.06.046
Ataei, P., Gholamrezai, S., Movahedi, R., and Aliabadi, V. (2021). An Analysis of Farmers' Intention to Use Green Pesticides: The Application of the Extended Theory of Planned Behavior and Health Belief Model. J. Rural Stud. 81, 374–384. doi:10.1016/j.jrurstud.2020.11.003
Barham, E. (2003). Translating Terroir: The Global Challenge of French AOC Labeling. J. Rural Stud. 19 (1), 127–138. doi:10.1016/S0743-0167(02)00052-9
Basurto, X., Gelcich, S., and Ostrom, E. (2013). The Social-Ecological System Framework as a Knowledge Classificatory System for Benthic Small-Scale Fisheries. Glob. Environ. Change 23 (6), 1366–1380. doi:10.1016/j.gloenvcha.2013.08.001
Bhandari, G., Zomer, P., Atreya, K., Mol, H. G. J., Yang, X., and Geissen, V. (2019). Pesticide Residues in Nepalese Vegetables and Potential Health Risks. Environ. Res. 172, 511–521. doi:10.1016/j.envres.2019.03.002
Brodrechtova, Y., Navrátil, R., Sedmák, R., and Tuček, J. (2018). Using the Politicized IAD Framework to Assess Integrated Forest Management Decision-Making in Slovakia. Land Use Policy 79, 1001–1013. doi:10.1016/j.landusepol.2016.10.015
Carazo-Rojas, E., Pérez-Rojas, G., Pérez-Villanueva, M., Chinchilla-Soto, C., Chin-Pampillo, J. S., Aguilar-Mora, P., et al. (2018). Pesticide Monitoring and Ecotoxicological Risk Assessment in Surface Water Bodies and Sediments of a Tropical Agro-Ecosystem. Environ. Pollut. 241, 800–809. doi:10.1016/j.envpol.2018.06.020
Casson, M. C., Della Giusta, M., and Kambhampati, U. S. (2010). Formal and Informal Institutions and Development. World Dev. 38 (2), 137–141. doi:10.1016/j.worlddev.2009.10.008
Cattaneo, M. D. (2010). Efficient Semiparametric Estimation of Multi-Valued Treatment Effects under Ignorability. J. Econ. 155 (2), 138–154. doi:10.1016/j.jeconom.2009.09.023
Dou, L., Yanagishima, K., Li, X., Li, P., and Nakagawa, M. (2015). Food Safety Regulation and its Implication on Chinese Vegetable Exports. Food Policy 57, 128–134. doi:10.1016/j.foodpol.2015.09.007
Drogué, S., and DeMaria, F. (2012). Pesticide Residues and Trade, the Apple of Discord? Food Policy 37 (6), 641–649. doi:10.1016/j.foodpol.2012.06.007
Fan, Y., Lai, K., Rasco, B. A., and Huang, Y. (2014). Analyses of Phosmet Residues in Apples with Surface-Enhanced Raman Spectroscopy. Food control. 37, 153–157. doi:10.1016/j.foodcont.2013.09.014
Ferrier, P., and Lamb, R. (2007). Government Regulation and Quality in the US Beef Market. Food Policy 32 (1), 84–97. doi:10.1016/j.foodpol.2006.01.004
Greenpeace (2016). Investigation of Pesticides Used in Tea on 2016 in China [Online]. Available: http://www.360doc.com/content/17/0317/23/4450299_637792288.shtml (Accessed April 4th, 2021).
Grung, M., Lin, Y., Zhang, H., Steen, A. O., Huang, J., Zhang, G., et al. (2015). Pesticide Levels and Environmental Risk in Aquatic Environments in China - A Review. Environ. Int. 81, 87–97. doi:10.1016/j.envint.2015.04.013
Hafezi, M., and Zolfagharinia, H. (2018). Green Product Development and Environmental Performance: Investigating the Role of Government Regulations. Int. J. Prod. Econ. 204, 395–410. doi:10.1016/j.ijpe.2018.08.012
Kayhan, V. O. (2015). The Nature, Dimensionality, and Effects of Perceptions of Community Governance. Inf. Manag. 52 (1), 18–29. doi:10.1016/j.im.2014.10.004
Kumar, A., Mishra, A. K., Saroj, S., and Joshi, P. K. (2019). Impact of Traditional Versus Modern Dairy Value Chains on Food Security: Evidence from India's Dairy Sector. Food Policy 83, 260–270. doi:10.1016/j.foodpol.2019.01.010
Lam, W. F. (1996). Institutional Design of Public Agencies and Coproduction: A Study of Irrigation Associations in Taiwan. World Dev. 24 (6), 1039–1054. doi:10.1016/0305-750x(96)00020-4
Lamichhane, J. R. (2017). Pesticide Use and Risk Reduction in European Farming Systems with IPM: An Introduction to the Special Issue. Crop Prot. 97, 1–6. doi:10.1016/j.cropro.2017.01.017
Li, F., Ren, J., Wimmer, S., Yin, C., Li, Z., and Xu, C. (2020a). Incentive Mechanism for Promoting Farmers to Plant Green Manure in China. J. Clean. Prod. 267, 122197. doi:10.1016/j.jclepro.2020.122197
Li, M., Wang, J., Zhao, P., Chen, K., and Wu, L. (2020b). Factors Affecting the Willingness of Agricultural Green Production from the Perspective of Farmers' Perceptions. Sci. Total Environ. 738, 140289. doi:10.1016/j.scitotenv.2020.140289
Lichtenberg, E. (2013). “Economics of Pesticide Use and Regulation,” in Encyclopedia of Energy, Natural Resource, and Environmental Economics. Editor J.F. Shogren (Waltham: Elsevier), 86–97. doi:10.1016/b978-0-12-375067-9.00092-9
Likudis, Z., Costarelli, V., Vitoratos, A., and Apostolopoulos, C. (2013). Determination of Pesticide Residues in Olive Oils with Protected Geographical Indication or Designation of Origin. Int. J. Food Sci. Technol. 49 (2), 484–492. doi:10.1111/ijfs.12326
Linden, A., and Yarnold, P. R. (2016). Combining Machine Learning and Propensity Score Weighting to Estimate Causal Effects in Multivalued Treatments. J. Eval. Clin. Pract. 22 (6), 875–885. doi:10.1111/jep.12610
Liu, Y., Liu, F., Zhang, J., and Gao, J. (2015). Insights into the Nature of Food Safety Issues in Beijing through Content Analysis of an Internet Database of Food Safety Incidents in China. Food control. 51, 206–211. doi:10.1016/j.foodcont.2014.11.017
Liu, Y., Sun, D., Wang, H., Wang, X., Yu, G., and Zhao, X. (2020). An Evaluation of China's Agricultural Green Production: 1978-2017. J. Clean. Prod. 243, 118483. doi:10.1016/j.jclepro.2019.118483
Liu, Z., and Lan, J. (2018). The Effect of the Sloping Land Conversion Programme on Farm Household Productivity in Rural China. J. Dev. Stud. 54 (6), 1041–1059. doi:10.1080/00220388.2017.1324145
Meredith, S., and Willer, H. (2016). Organic in Europe: Prospects and Developments 2016. Brussels, Belgium: IFOAM EU Group.
Miewald, C., Ostry, A., and Hodgson, S. (2013). Food Safety at the Small Scale: The Case of Meat Inspection Regulations in British Columbia's Rural and Remote Communities. J. Rural Stud. 32, 93–102. doi:10.1016/j.jrurstud.2013.04.010
Minten, B., Singh, K. M., and Sutradhar, R. (2013). Branding and Agricultural Value Chains in Developing Countries: Insights from Bihar (India). Food Policy 38, 23–34. doi:10.1016/j.foodpol.2012.09.003
Niu, Z., Chen, C., Gao, Y., Wang, Y., Chen, Y., and Zhao, K. (2022). Peer Effects, Attention Allocation and Farmers' Adoption of Cleaner Production Technology: Taking Green Control Techniques as an Example. J. Clean. Prod. 339, 130700. doi:10.1016/j.jclepro.2022.130700
Orcos, R., Pérez-Aradros, B., and Blind, K. (2018). Why Does the Diffusion of Environmental Management Standards Differ across Countries? The Role of Formal and Informal Institutions in the Adoption of ISO 14001. J. World Bus. 53 (6), 850–861. doi:10.1016/j.jwb.2018.07.002
Ostrom, E., Calvert, R., and Eggertsson, T. (1993). Governing the Commons: The Evolution of Institutions for Collective Action. Am. Political Sci. Rev. 86 (1), 279–249. doi:10.2307/1964069
Ostrom, E. (1996). Crossing the Great Divide: Coproduction, Synergy, and Development. World Dev. 24 (6), 1073–1087. doi:10.1016/0305-750X(96)00023-X
Schreinemachers, P., Wu, M.-H., Uddin, M. N., Ahmad, S., and Hanson, P. (2016). Farmer Training in Off-Season Vegetables: Effects on Income and Pesticide Use in Bangladesh. Food Policy 61, 132–140. doi:10.1016/j.foodpol.2016.03.002
Seenivasan, S., and Muraleedharan, N. (2011). Survey on the Pesticide Residues in Tea in South India. Environ. Monit. Assess. 176 (1-4), 365–371. doi:10.1007/s10661-010-1589-y
Shuqin, J., and Fang, Z. (2018). Zero Growth of Chemical Fertilizer and Pesticide Use: China's Objectives, Progress and Challenges. J. Resour. Ecol. 9 (1), 50–58. doi:10.5814/j.issn.1674-764x.2018.01.006
Sun, H., Edziah, B. K., Sun, C., and Kporsu, A. K. (2021). Institutional Quality and its Spatial Spillover Effects on Energy Efficiency. Socio-Economic Plan. Sci. 101023, 101023. doi:10.1016/j.seps.2021.101023
Sun, H., Kporsu, A. K., Taghizadeh-Hesary, F., and Edziah, B. K. (2020). Estimating Environmental Efficiency and Convergence: 1980 to 2016. Energy 208, 118224. doi:10.1016/j.energy.2020.118224
Whaley, L., and Weatherhead, E. K. (2014). An Integrated Approach to Analyzing (Adaptive) Comanagement Using the “Politicized” IAD Framework. Ecol. Soc. 19 (1), 10. doi:10.5751/ES-06177-190110
Yu, X. (2012). Productivity, Efficiency and Structural Problems in Chinese Dairy Farms. China Agric. Econ. Rev. 4 (2), 168–175. doi:10.1108/17561371211224755
Keywords: government regulation, community governance, green production behavior, targeted agricultural policy, Qinba and Huangshan mountain regions of China
Citation: Yu Y, Lu T, Hu YG, Meng K and Li H (2022) How to Improve Farmers’ Green Production Level in a Targeted Manner?. Front. Environ. Sci. 10:901844. doi: 10.3389/fenvs.2022.901844
Received: 22 March 2022; Accepted: 25 April 2022;
Published: 19 May 2022.
Edited by:
Huaping Sun, Jiangsu University, ChinaReviewed by:
Jinna Yu, Guizhou Minzu University, ChinaCopyright © 2022 Yu, Lu, Hu, Meng and Li. This is an open-access article distributed under the terms of the Creative Commons Attribution License (CC BY). The use, distribution or reproduction in other forums is permitted, provided the original author(s) and the copyright owner(s) are credited and that the original publication in this journal is cited, in accordance with accepted academic practice. No use, distribution or reproduction is permitted which does not comply with these terms.
*Correspondence: Hua Li, bGlodWE3NDg1QDE2My5jb20=
Disclaimer: All claims expressed in this article are solely those of the authors and do not necessarily represent those of their affiliated organizations, or those of the publisher, the editors and the reviewers. Any product that may be evaluated in this article or claim that may be made by its manufacturer is not guaranteed or endorsed by the publisher.
Research integrity at Frontiers
Learn more about the work of our research integrity team to safeguard the quality of each article we publish.