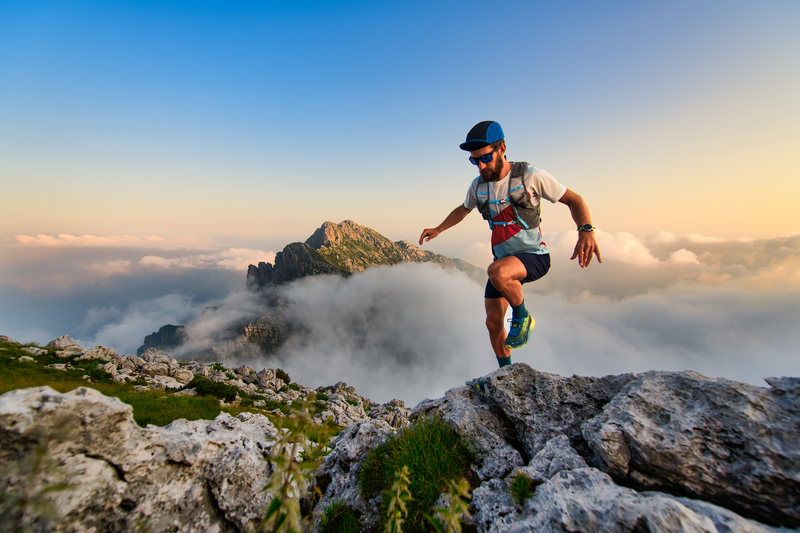
95% of researchers rate our articles as excellent or good
Learn more about the work of our research integrity team to safeguard the quality of each article we publish.
Find out more
ORIGINAL RESEARCH article
Front. Environ. Sci. , 04 May 2022
Sec. Environmental Economics and Management
Volume 10 - 2022 | https://doi.org/10.3389/fenvs.2022.901472
This article is part of the Research Topic The Role of Fiscal Decentralization in Achieving Environmental Sustainability in Developing and Emerging Economies View all 19 articles
This study investigates how carbon emissions and energy consumption related to the life satisfaction of Chinese households over the 2010–2018 period. The China Family Panel Studies (CFPS) data from 25 Chinese provinces shows that the effect of provincial carbon emissions on life satisfaction is positive, and increases in fuel and electricity consumption also predict a higher life satisfaction level. Fuel consumption, especially, has a greater impact on life satisfaction among older people with lower income or education levels. In addition, an increase in relative energy consumption negatively affects Chinese households’ life satisfaction. Heterogeneity analysis demonstrates that the relative fuel consumption change has a larger negative life satisfaction effect for younger, less educated or rural people. In developed regions (including Beijing and Shanghai), a strong inverse relationship between carbon emissions and the residents’ life satisfaction exists in China, but the effects of residents’ own and relative energy consumption are insignificant or slight.
In recent decades, carbon emissions have become a vital environmental issue of widespread concern, as excessive carbon emissions have the potential to be sources of both greenhouse effects and depletion of natural resources. Yet, the implications of proposed carbon emissions reduction schemes in developing countries remain a matter of debate, since nations need to extensively rely on fossil energy to fuel their current development demands (Costa et al., 2011). In addition, the carbon emissions dimension is still not well understood in the life satisfaction related literature. Life satisfaction reflects the subjective feelings in our daily lives, which is a vital indicator to measure people’s quality of life (Tonon, 2013) and is highly related to social progress (Li and Raine, 2013). With the deepening of reform and opening-up in the past decades, China has undergone remarkable economic growth and societal environment change. At the same time, the Chinese people’s requirements for a better life have been gradually increasing, which conflicts with the inadequate development in China (Qiu, 2017; Zhang et al., 2022). Due to higher goals for quality of life, despite the economic prosperity, the Chinese people’s life satisfaction is steadily declining (Li and Raine, 2013). Therefore, both carbon emissions and life satisfaction are emerging as public concerns. Understanding how carbon emissions related factors influence people’s satisfaction with life can help get a better picture of how to reduce carbon emissions while ensuring the improvement of people’s life satisfaction (Li and Chen, 2021), and further promote long-term social development. Recently, studies have called for much closer attention to be given to the role of the carbon emissions in human well-being literature (Fanning and O’Neill, 2019; Sugiawan et al., 2019), as it allows us to have a deeper understanding of how to reconcile a low-carbon lifestyle with a higher level of life satisfaction, which can promote the sustainable implementation of related carbon emissions reduction policies.
Another important issue related to carbon emissions to be addressed is energy consumption. Household energy consumption is largely dominant in the case of per capita carbon dioxide emissions (Sugiawan et al., 2019). In China, the household share of total carbon emissions is increasing (Zheng et al., 2010). Whether and to what extent energy consumption is associated with life satisfaction? And how do individual characteristics moderate the relationship between energy consumption and life satisfaction? Since a household’s energy consumption is like the bridge connecting the relationship between human well-being and carbon emissions (Li and Chen, 2021), a better understanding of these questions can help inform policies aimed at carbon emission reduction and enhancing people’s well-being. When evaluating life satisfaction, in addition to their own energy consumption level, individuals also draw comparisons with reference groups who share similar characteristics to themselves (Roychowdhury, 2019; Sun and Wang, 2013; Kaus, 2013; Maurer and Meier, 2008). The link between relative consumption and happiness has been one of the most discussed and debated topics in the literature on subjective well-being (Wu, 2019). Studies also shed light on the importance of reference groups and routine behavior for pro-environmental consumption (Welsch and Kühling, 2009; Wolske et al., 2020), however, there has been relatively little research on the impact of reference groups’ energy consumption on the well-being literature. Considering relative energy consumption as a potential mechanism may provide a new perspective in explaining people’s life satisfaction change, especially in China, a typical “human relationship society” (Zhang et al., 2022). In this context, the reference group’s energy consumption patterns are integrated into the framework to explore the mechanism through which the energy consumption of the reference group affects the Chinese households’ life satisfaction.
China has a vast territory, and carbon emissions vary from region to region due to discrepancies in climate, infrastructure, and energy consumption structure (Sun and Wang, 2021). Different provinces in China have different resource endowments, energy structures, and economic development levels; for example, the GDP per capita of some cities such as Beijing and Shanghai has reached the level of developed countries, while other regions are still at the level of developing countries (Hong et al., 2021). China provides a natural experiment in which current relationships among provincial carbon emissions, energy use, and household members’ life satisfaction; thus, this study is situated in China. With the mainstream goal of “green growth,” China has signed up to reduce its carbon emissions and has a plan to peak the carbon emissions within the next decade. The Chinese government began developing a route map that focused on emissions reductions. However, there is still uncertainty regarding the dependence of carbon reductions on people’s quality of life. In addition, the Chinese government has made various efforts to improve people’s life satisfaction, including implementing some support policies aimed at poverty alleviation. Now that the Chinese government needs to improve people’s quality of life and achieve the goal of peaking carbon emissions by 2030, a better understanding of the relationship between carbon emissions, energy consumption, and life satisfaction is needed. This research is among the first to simultaneously evaluate the carbon emission, energy consumption, and relative energy consumption in explaining Chinese people’s life satisfaction change.
Correspondingly, this present study makes several contributions. First, this study extends the emerging literature on the relationships among provincial carbon emissions, energy use, and life satisfaction levels within one country covering extended periods. Some research linking greenhouse gases and well-being is often conducted based on the overall index of countries (Apergis and Majeed, 2021). Nevertheless, the effect of environmental factors might vary in broad societal contexts. Focusing on one country makes it possible to test the sensitivity of the effect of carbon emissions and energy consumption on life satisfaction to the choices of geographic factors and control variables (Hou, 2013). Second, as the determinants of life satisfaction involving “internal” factors of personality traits and individuals’ socio-demographic characteristics are embedded within “external” factors in individuals’ environments, the perspectives of “top-down” and “bottom-up” methods are combined. Not only are macro factors like total provincial carbon emissions and GDP included, but micro factors such as income, socio-economic status, and other demographic characteristics are also examined in the model using a large micro panel data set, which reveals the detailed factors that are related to individuals’ life satisfaction and the trade-offs they face. Third, the findings are novel in that the importance of energy consumption comparisons for people’s life satisfaction is also underscored, and determine how residents’ own and relative energy consumption influence people’s life satisfaction in China.
The rest of the paper is organized as follows: Section 2 comprises a literature review of life satisfaction, carbon emissions, energy consumption, and relative energy consumption studies. Section 3 presents the data set, variables, and methods. Section 4 presents the empirical results and estimations. Section 5 discusses the results and makes policy suggestions.
As a kind of cognitive evaluation individual has regarding their life status, life satisfaction is the most common and reliable measure used in research on individuals’ happiness (Hou, 2013; Fanning and O’Neill, 2019). Easterlin (1995) and Hirsch (1976) laid the theoretical foundation for happiness and well-being-related studies. Easterlin (1995) found that more wealth does not guarantee greater happiness, the so-called “happiness paradox.” Following-up studies found that other factors ignored in early research may play a vital role in influencing people’s life satisfaction, including environment, energy use, health, subjective factors, etc. (Betti et al., 2020; Apergis and Majeed, 2021; Kumari et al., 2021). At the same time, scholars have also realized that aspects of the external factors such as the natural environment or ecosystem are vital to people’s life satisfaction (Summers et al., 2012; Cuñado and de Gracia, 2013; Zhang et al., 2017; Brereton et al., 2008), including air pollution, noise, and climate. Thus, it is necessary to identify influential environmental and energy-related factors to improve people’s life satisfaction.
Most previous studies of life satisfaction are confined to Western countries (Li and Raine, 2013). However, in recent years, some research focused on China, and has found that family characteristic factors such as household income, consumption, housing, and environmental factors such as air pollution are highly related to Chinese people’s life satisfaction (Zhang et al., 2022; Zhu et al., 2020; Cheng, et al., 2020; Zhang et al., 2017). Specifically, Zhang et al. (2017) investigated the association between air pollution and well-being using China Family Panel Studies data and found that the increase in the air pollution index reduced happiness and increased depression symptoms.
A large literature has also evolved on the relationship between carbon emissions and life satisfaction, but the results are mixed. Some scholars argue that carbon emissions have a negative effect on people’s life satisfaction since it is not only one of the main causes of global warming and pollution (Zhang et al., 2017), but also brings some health risks such as the increased risk of cardiovascular disease and lung cancer (Lelieveld et al., 2019; Betti et al., 2020). Further, an increase in carbon emissions might lead to increased anxiety and concern about the possibility that they will be unable to adapt or otherwise protect themselves in the climate crisis caused by carbon emissions (El Zoghbi and El Ansari, 2014). As household consumption plays a key role in energy use and carbon emissions (Liu et al., 2016; Sun and Wang, 2021; Ivanova, et al., 2016; Kerkhof et al., 2009; Guo et al., 2021), especially in developing countries, some scholars considered the carbon emissions issue from a “bottom-up” perspective, that is, using the method of the basic goods basket to link human well-being and carbon emissions. They argued that the “bottom-up” human well-being index is more appropriate to study the relationship between individuals’ lifestyles and carbon emissions (Li and Chen, 2021). Consistent with such predictions, Höysniemi and Salonen (2019) focused on the carbon emission-related behavioral intentions related to Finnish citizens’ life satisfaction and found that the limited use of private cars also significantly predicts increased life satisfaction. Vita et al. (2020) compared the carbon footprints of non-members and members of sustainability-oriented grassroots initiatives and found that members show higher life satisfaction compared to non-members.
In contrast, a positive relationship between life satisfaction and carbon emissions has also been found in many studies. Concretely, Rao and Baer (2012) believed that increased use of energy and the accompanying emissions are needed to provide people with a decent living standard. Energy use serves to alleviate extreme temperatures, provide light and shelter, etc., and presently the most readily available sources of energy produce carbon emissions. When its convenience outweighs its costs, increased use of carbon-based energy sources, and the associated emissions, will increase people’s life satisfaction.
However, other scholars have noted that carbon emissions and life satisfaction can be decoupled, or can have a non-linear relationship. Sulkowski and White (2015) found that carbon emissions are not correlated perfectly with either development or happiness. Between two clusters with the highest average levels of development, income, and happiness, there was a 43% difference in carbon emissions. A highly developed cluster had roughly the same mean carbon emissions as a cluster with 83% less income, and the least developed cluster had 93% of the happiness level of the most developed cluster, yet 86% fewer carbon emissions. They also reported a happiness Kuznets curve (HKC), implying that average happiness may decrease as countries begin to develop but eventually improves as countries move from being moderately developed to highly developed. In contrast, using panel data on 58 nations, Dietz et al. (2012) examined the environmental intensity of human well-being, that is, the ratio of a nation’s per capita ecological footprint to its average life expectancy at birth, and found a U-shaped relationship between gross domestic product per capita and EIWB, not the predicted inverted-U.
Why do previous studies focused on the relationship between carbon emissions and life satisfaction show different results? One potential explanation for this question could be the regional disparities, since carbon emissions are associated with the development and urbanization of a region, people living in developing regions who produce more carbon emissions through driving more and consuming more electricity might be happier than others (Glaeser and Kahn, 2010; Zheng et al., 2010). Some research has employed a “top-down” approach, that is, examining the relationship between macro-level measures of development and energy use from a macro level to expand the research focus of energy use from the economy or technology to society (Li and Chen, 2021). Costa et al. (2011) used the human development index (HDI) as the main indicator to evaluate human well-being and demonstrated the existence of a positive and time-dependent relationship between HDI and CO2 emissions per capita from fossil fuel combustion. They also proposed that until HDI reached the threshold of 0.8, the developing countries are not suggested to reduce carbon emissions. Using national-level panel data for the 1970–2009 period, Jorgenson (2014) found that economic growth improved human well-being but at the cost of an increasing amount of carbon emissions. The carbon intensity of human well-being (CIWB) indicator, that is, the level of anthropogenic carbon emissions per unit of human well-being, was utilized in this research. For nations in Asia and South and Central America, development increased CIWB throughout the 40 years of the study. Sugiawan et al. (2019) suggested that the commitment to carbon emissions reduction needs to be evaluated and should be different between developed and developing economies because it is important to consider its impact on inter-generational well-being. The developed countries were encouraged to set up a more ambitious target of carbon emissions reduction.
In China, the demand for energy consumption and households’ share of total carbon emissions is increasing as a result of China’s rapid urbanization and transition to being a service economy (Zheng et al., 2010). The corresponding outputs that the energy produces, such as food, heat, industrial objects, or service, are not only related to humans’ basic needs and well-being (Jorgenson et al., 2014) but also are a key driver of carbon emissions (Okulicz-Kozaryn and Altman, 2019). To this point, energy consumption is like the bridge connecting the relationship between human well-being and carbon emissions (Li and Chen, 2021); thus, it is necessary to take energy consumption into account in carbon emissions and life satisfaction-related research.
A fundamental trade-off exists in energy consumption between the environmental impact and self-interest at the individual and national levels (Okulicz-Kozaryn and Altman, 2019). It is generally believed that energy use, such as electric power might offer an important material foundation for individuals’ happiness and socio-economic development, which brings convenience to their lives and improves their overall quality of life, especially for the rural residents (Guo et al., 2016). Kumari et al. (2021) analyzed the effect of energy consumption and environmental quality on subjective well-being in G20 countries from 2006 to 2019. The findings reveal that renewable energy consumption and environmental quality significantly enhance well-being in G20 countries, while non-renewable energy consumption reduces subjective well-being. Arto et al. (2016) found that the relationship between energy demand and HDI shows a strong positive correlation in 40 developed and developing countries for the period 1995–2008; however, beyond a certain point, HDI is not associated with GDP. Yet, in contrast, some research has also found that subjective well-being and life satisfaction are unrelated to energy use. Okulicz-Kozaryn and Altman (2019) integrated data from multiple sources and found that no evidence of increasing energy consumption substantially increases well-being. Arto et al. (2016) also found that in developed countries, the relationship is decoupled, because international trade enables countries to maintain their development level while goods and services are produced abroad without causing domestic energy consumption to increase. Similarly, Akizu-Gardoki et al. (2020) found that there is a well-being turning point, that is, there is a logarithmic positive relationship between well-being and energy consumption up to a certain point, but a negative correlation between energy consumption and well-being after the well-being turning point has been reached.
Relative consumption is defined as consumption in comparison to that of the reference group (Frank, 1985; Sun and Wang, 2013). Individuals live in society and observe others’ behaviors; thus, the consumption patterns of others in comparison to oneself are taken into account in individuals’ self-reported life satisfaction and well-being (Guillen-Royo, 2011; Wang et al., 2017). The reference group is the social group that consumers compare themselves to and, as a result, make judgments about themselves (Tajfel, 2003). Some research has found that the reference group has a value-expressive influence: it helps consumers express themselves and meets their needs for self-elevation (Hammerl et al., 2016). Through observations and communications with the reference groups, individuals’ self-evaluated life satisfaction will be affected accordingly.
Reference groups’ spending on different types of commodities might have different externalities. The early work of Veblen (1899) put forward the conspicuous consumption theory, which indicated that people pursued conspicuous consumption to demonstrate that they were better than others, and to help them maintain or raise their position in the social hierarchy. Previous work has also distinguished between positional/status goods and non-positional/non-status goods (Frank, 1985; Hirsch, 1976) to understand the nature of social comparisons regarding consumption behavior. Positional/status goods are the visible things that can signal the owners’ place or rank in the society, while non-positional/non-status goods are not used to make such comparisons and are consumed for their utilitarian value. Heffetz (2012) pointed out that different commodities have different degrees of visibility; Strangers notice an individual’s expenditures with higher visibility more than other expenditures, such as clothing and cars. Wu (2019) hypothesized that individuals’ positional consumption relative to that of others in one’s reference group increases their life satisfaction in all income groups, while higher levels of basic expenditures have a negative influence on people in the lowest income quartile.
Energy consumption is a kind of non-positional and basic good. Much of the previous research on energy consumption focuses on how individuals’ energy consumption decisions are influenced by the behavior of reference groups (Welsch and Kühling, 2009; Mi et al., 2019; Wolske et al., 2020). Welsch and Kühling (2009) hypothesized that the reference groups’ pro-environmental consumption patterns are relevant to consumers’ consumption, because social comparison may serve the purpose of encouraging social compatibility within ones’ reference group. Wolske et al. (2020) explored two potential ways in which peer effects on energy use and clean technology adoption could occur. The first is interpersonal communication and persuasion. Such communication may raise awareness of pro-environmental behaviors and the potential benefits of new energy technologies, and it may convey important procedural information (Berger, 2014). The second peer effect involves changes in social norms. Some research examined respondents’ perceptions of peer influences and found that communicating with peers can reduce uncertainty and confirm peer adopters’ beliefs that photovoltaics can work as intended without hassle (Palm, 2017). These two processes represent complementary ways in which social influence may manifest itself in peer effects. Some research has also indicated that a reference group’s norms serve as a way to stimulate residents to implement low-carbon consumption behaviors (Mi et al., 2019). Based on the theory of social interaction and embeddedness, Yin and Shi (2021) found that having a strong relationship with one’s reference group can directly promote low carbon consumption behavior among Chinese residents, while network size has an indirect effect through social norms. To date, however, there have been very few empirical studies that consider the relationship between the reference group’s energy consumption and life satisfaction directly. This study extends this line of research by investigating whether individuals feel more satisfied when their reference groups engage in more energy consumption.
Most household survey data do not have accurate social interaction variables and lack direct survey questions about the composition of someone’s social circles, considering the availability of data, some studies would take reference groups as given exogenous variables. Many scholars assume that people living in the same region constitute a membership reference group (Wang et al., 2017; Cheng, et al., 2020; Roychowdhury, 2019; Sun and Wang, 2013; Kaus, 2013). As argued by Kaus, (2013), consumables are prominently visible in the process of social interaction, and a large proportion of social interactions occur in residential environments. Other researchers assume that individuals primarily compare themselves with others who share certain demographic characteristics (e. g., age, gender, degree, or occupation) (Maurer and Meier, 2008).
To date, although there are mixed findings concerning the impact of carbon emissions and energy consumption on life satisfaction, most research linking carbon emissions and well-being is often conducted based on the overall index of countries (Fanning and O’Neill, 2019; Apergis and Majeed, 2021), few studies test the regional disparities focusing on one country. In addition, little substantive empirical research has addressed the link between reference groups’ energy consumption and household members’ life satisfaction. To address this research gap, this study analyzes the effects of carbon emissions, as well as consumers’, and also underscores the importance of their references groups’ energy consumption on life satisfaction in China, using top-down and bottom-up methods.
In this study, for every household member i in year t, life satisfaction is predicted from provincial carbon emissions, household income, energy consumption, and its ratio to the household member’s reference group, etc. The following model represents this relationship:
Where
CFPS data administered by the Institute of Social Science Survey at Peking University is used from 2010 to 2018 and the project aims to conduct regular and systematic surveys on the three levels of communities, families, and family members (individuals). CFPS is a nationally representative household survey in China. Up to now, CFPS has released tracking survey data for 2010, 2012, 2014, 2016, and 2018, containing a wide range of detailed economic, social, and demographic information about Chinese households. After data combining and cleaning, 133,204 samples covering 25 provinces and municipalities are obtained.
Some macro-level data such as provincial GDP and provincial population are drawn from the China National Bureau of Statistics Database, and carbon emissions data came from the Carbon Emission Accounts and Datasets (CEADs). CFPS micro-data is also matched with these macro data, which covers 25 provinces in China and spans 2010–2018.
The life satisfaction level of the respondents is measured through self-reported satisfaction levels on a 5-point scale, in response to the question: “Are you satisfied with your life?” Responses ranged from 1 (most dissatisfied) to 5 (most satisfied). Life satisfaction is the most common and reliable measure used in subjective well-being-related literature (Hou, 2013; Fanning and O’Neill, 2019).
Carbon emissions per capita (in metric tons) are the key independent variable, with data for 25 provinces from 2010 to 2018 from the CEADs. The detailed computation process is available in Shan et al. (2020)’s research. Each province’s carbon emissions level is divided by the provincial population to get the carbon emissions per capita variable.
Household energy consumption is also a vital independent variable since it is not only related to well-being (Jorgenson et al., 2014) but also is a key driver of carbon emissions (Okulicz-Kozaryn and Altman 2019). Based on data availability, households’ energy consumption mainly contains two parts: electricity expenditures and fuel expenditures, respectively measured through these two questions: “How much does your family spend on electricity?” and “How much does your family spend on fuel (including coal gas, liquefied gas, coal, firewood, charcoal, etc.)?” These two questions are only asked starting in 2012. As the expense is monthly and household data, these two variables are multiplied by 12 and divided by family size. For households with no electricity or fuel expenses, the values are replaced with 0.01, then transformed logarithmically.
The relative electricity consumption and relative fuel consumption independent variables are used to measure the positionality of household energy consumption, defined as the ratio between an individual’s electricity consumption, fuel consumption, and their reference group’s average levels respectively. The reference group is assumed to consist of people of similar age and similar income levels living in the same province. The reference-level energy consumption is first defined at the provincial level as revealed in the literature (Wang et al., 2017; Cheng, et al., 2020). Within each province, further reference groups are constructed along the dimensions of age and household income. Age is divided into six categories: 18–24, 25–34, 35–44, 45–54, 55–65, and 65 or above. Household income is divided into 1–5 quintiles from low to high. Thus, there are 30 reference groups in total within each province in 1 year (5*6 = 30). The electricity consumption ratio is measured by their own electricity expense divided by the average electricity expense of ones’ reference group and introduced in logarithmic form. Similarly, the fuel consumption ratio variable is measured by their own fuel expense divided by the average level of reference-group fuel expense and introduced in logarithmic form.
To measure the economic development levels of each province over time, provincial real GDP per capita is selected as the indicator. GDP measures the total market value of all final goods and services purchased within a province. Each province’s GDP level is divided by the provincial population to get the GDP per capita. And the natural logarithm form of real GDP per capita is used.
In addition, some key micro-level covariates are also included in the model based on their relevance to life satisfaction as revealed in the literature, including household income and individual demographic variables. Household income is defined as the sum of total money income for all members of the household. Total income includes income from wages and operating income, property income, and transfer income, and is divided by family size, and the natural logarithm calculated. Households with no income are assigned a value of zero. A series of demographic variables are treated as control variables including education, gender, age, marital status, and residence (urban or rural). Among them, the level of education received is calculated according to years of education. Other demographic variables are dummy-coded. For gender, female is 0 and male is 1; for marital status, married or cohabitating is 1, and unmarried, not cohabiting, divorced, or widowed residents are 0; for residence, 1 = living in an urban area and 0 = living in a rural area.
The provincial population (according to the China National Bureau of Statistics Database) is chosen as the macro-level control variable and is log-transformed. The categories of household energy consumption, income, and provincial GDP have been deflated by the consumer price index (CPI), using 2010 as the base year.
Descriptive statistics for the above variables are presented in Table 1. The mean value of life satisfaction increased from 3.652. And the mean value of provincial GDP per capita is 18742.89. However, the standard deviation value of GDP is large, which indicates that China’s regional economic development is unbalanced. In addition, reference-group electricity expenditures and reference-group fuel expenditures are defined at the provincial age-income level, and their standard deviation values indicate that residents’ energy expenditures are also heterogeneous in different groups or regions.
In line with previous research, the changes in the coefficients across linear models can be compared, but for logit or probit models, one cannot straightforwardly compare the coefficient changes (Mood, 2009; Hou, 2013). Therefore, life satisfaction was first treated as a continuous variable and employed OLS models. Considering that CFPS data has a multi-level nature, in all OLS models, standard errors are clustered at the province level. The OLS model with cluster-robust standard errors is identical to a random intercept with higher-level predictors and a fixed-slope model with level-1 covariates within the framework of hierarchical linear models (HLM) (Arceneaux and Nickerson, 2017; Hou, 2013). To make the results robust, the results are also replicated in ordered logit models. To test relationship heterogeneity across different regions, the data sample was further segregated into first-tier cities (including Beijing and Shanghai) and other province categories. Finally, a series of heterogeneity analyses across several dimensions: individuals’ income, education level, age, and residence type (urban or rural) are conducted.
Life satisfaction and carbon emissions may show heterogeneity in China. Therefore, these two macro variables are plotted in Figure 1. The x-axis indicates each province’s average carbon emissions per capita, and the y-axis indicates the life satisfaction level. Across these provinces in China, the quadratic fit shows that there is a clear positive association between carbon emissions and life satisfaction. The higher carbon emissions are within a province, the higher life satisfaction is for its households. Still, the variance across provinces is large. Guangxi (GX), Jiangxi (JX), and Guangdong (GD) have both the lowest carbon emissions levels and lowest average life satisfaction. Some provinces, such as Tianjin (TJ), have both higher carbon emissions per capita and higher life satisfaction. Still, there are some exceptions, such as Shanxi (SX) and Liaoning (LN), which have higher carbon emissions per capita, but lower life satisfaction.
FIGURE 1. The relationship between carbon emissions and life satisfaction. Notes: Quadratic fit is shown with 95% confidence intervals. All data are averaged over the 2010–2018 period.
In addition, Beijing and Shanghai, as two first-tier municipalities of China, are the economic leaders in comparison to other provinces and cities. Thus, these municipalities’ carbon emissions and residents’ life satisfaction might exhibit unique features that need to be studied. Figures 2, 3 depict the relationship between carbon emissions and life satisfaction in Beijing and Shanghai respectively. As can be seen in Figure 2, contrary to Figure 1, the trend in life satisfaction is negatively related to carbon emissions for residents in Beijing, which is somewhat in line with Zhang et al. (2017), who argue for a negative correlation between air pollution and life satisfaction.
FIGURE 3. The relationship between carbon emissions and life satisfaction in Shanghai. Note: Quadratic fit is shown with 95% confidence intervals. All data are averaged over the 2010–2018 period.
The self-reported levels of life satisfaction in Shanghai are shown in Figure 3, which illustrates that there is an inverted U-shaped relationship between carbon emissions and life satisfaction levels. Life satisfaction is highest at the intermediate level of carbon emissions per capita, around 0.078–0.079. As carbon emissions increase further, life satisfaction declines. Thus, people who experience the extremes of carbon emissions have the lowest life satisfaction. This is partly in line with Costa et al. (2011), who found that carbon emissions result in more life satisfaction only up to the point that is used to satisfy basic human needs. When essential human needs for energy are met, more carbon emissions will result in decreased life satisfaction.
In Table 2, the results from OLS with life satisfaction as the dependent variable are presented. Column 1 shows whether changes in carbon emissions and other macro variables are relevant to explaining changes in life satisfaction across provinces. The coefficient of carbon emissions implies that a 1% increase in provincial carbon emissions per capita is associated with a 2.122% increase in life satisfaction, and other macro factors such as provincial GDP and population only have some potential insignificant effects on life satisfaction.
In Column 2, alongside other macro determinants of life satisfaction, the model introduces some micro variables, including household income per capita, gender, registered residence condition, age, level of education, marital status, and family size. Among the micro indicators, household income, gender, age, and family size variables have positive and statistically significant coefficients, suggesting that older, female individuals with higher household income and larger family sizes tend to have higher levels of life satisfaction. It can be seen that these findings are consistent with previous research findings (Alesina et al., 2004; Hou, 2013; Zhu et al., 2020). Elders usually have lower expectations for future life and stress compared with younger people, thus having higher life satisfaction. In particular, the coefficient of the provincial population becomes significantly positive when the micro variables are introduced.
As for the economic factors, the coefficient of lnGDP indicates that provincial GDP, the macro factor, fails to explain people’s life satisfaction when incorporating the carbon emissions factor, which is consistent with Easterlin’s (1995). However, on a micro-economic level, increased household income is still associated with a higher level of life satisfaction, suggesting that higher income not only satisfies people’s requirements of higher-level life quality but provides opportunities for higher relative income level, which is also a positive determinant of life satisfaction, consistent with previous research (Frank, 2012; Yuan, 2015; Wang et al., 2019).
In Columns 4 and 5, the model incorporates the energy consumption factor: electricity expenses and fuel expenses. As these two variables are recorded only for the years 2012, 2014, 2016 and 2018 in CFPS, the sample size has changed. To raise the comparability of the coefficients, all the variables are regressed from the year 2012–2018 in Column 3. The regression of life satisfaction scores on carbon emissions of the variable for carbon emissions is 1.886 in Column 3, but this effect is reduced to 1.546 and 1.775 and becomes less significant in Columns 4 and 5. As for energy consumption, in Column 4, holding other factors fixed, a 1% increase in electricity expenses and fuel expenses are associated with 0.029% and 0.024% increases in life satisfaction, respectively; that is, any reduction of energy expenditures may have a negative effect on people’s life satisfaction. Since appliance usage has become a part of daily life, there is usually certain inertia for household appliance usage (Guo, et al., 2016). Using more electric and gas-powered appliances, such as refrigerators, TVs, and cooking appliances, can facilitate people’s daily lives, thus improving their life satisfaction. Furthermore, column 5 extends the set of regressors to include the electricity expense ratio and fuel expense ratio. In this case, the coefficients of electricity expense and fuel expense increased to 0.102 and 0.037. As for the referent ratio factors, higher ratio levels tend to be associated with lower life satisfaction levels. The fuel consumption ratio is negative and significant; a 1% increase in fuel consumption ratio leads to about a 0.024% decrease in life satisfaction. Though the electricity consumption ratio is less significant than the fuel consumption ratio, a 1% increase in it is associated with a 0.102% decline in life satisfaction, suggesting that people feel more satisfied or happier if they consume less electricity and fuel than their neighbors or peers.
The robustness of the results is checked and reported in Table 3. To explain the possible non-cardinality of life satisfaction data, Column 1 reports the results from estimating the models from Column 5 of Table 2 using an ordered logit instead of the OLS model. Column 2 presents a full model with fixed effect results, which provide within-person interpretations. And Column 3 shows the random effect results.
Comparing the results, the OLS model, ordered logistic model, fixed-effect model, and random effect model show similar patterns of how life satisfaction is determined. Though the coefficients of carbon emissions in Table 3 become significant, the other coefficients, including electricity expenses, fuel expenses, relative electricity expenses, and relative fuel expenses are similar in significance and influence direction to that of variables in Column 5 of Table 2. These results imply that the model is robust.
For the population as a whole, carbon emissions have a significant positive relationship with life satisfaction. The impact of carbon emissions on people’s life satisfaction may vary among different areas, given the significant differences in the economic development levels and natural resource endowments of different provinces. Among all provinces, Beijing and Shanghai are the first-tier municipalities, and the per capita GDP has reached the level of developed countries, while most of the other regions are still at the level of developing countries. As their economic and political resources and people’s living conditions are significantly different from other provinces and municipalities, the effects on life satisfaction should also be different.
To test for this, in Table 4, split-sample analyses are conducted. OLS models are run separately for the first-tier municipalities (Beijing and Shanghai) and other provinces. Results shown in Table 4 reveal some significant changes for the regional differences in the effects of provincial carbon emissions, reference groups, and individuals’ energy consumption. The first-tier municipalities’ sample results are reported in Columns 1–2, and those of other provinces in Columns 3–4. For individuals living in the first-tier municipalities, the carbon emissions variable is negatively related to their life satisfaction, which has also confirmed the conclusion from Figure 2, that carbon emissions have a significant negative coefficient. As Beijing and Shanghai are the most developed first-tier cities in China, the results presented so far suggest that how the changes in carbon emissions explain changes in life satisfaction across provinces depends on the degree of development of the region, which reinforces the conclusion of the previous research (Dietz et al., 2012). In addition, the effects of relative and individual energy consumption for Beijing and Shanghai are statistically different from the effect for other provinces. While the coefficients significantly influence life satisfaction levels in other provinces, they are not statistically significant for residents in Beijing and Shanghai.
TABLE 4. Impact of carbon emissions, energy consumption on Life satisfaction in Beijing and Shanghai.
Some individuals, depending on their characteristics, might be more prone than others to respond to carbon emissions or energy consumption. To obtain a better understanding of how these characteristics correlate with it, a series of heterogeneity analyses across several dimensions are conducted: individuals’ income, education level, age, and residence type (urban or rural).
In the heterogeneity analysis, main independent variables, including carbon emissions, electricity consumption, fuel consumption, relative electricity consumption, and relative fuel consumption, are interacted with individual characteristic variables. In Table 5, the results show that the carbon emissions variable does not exhibit different effects across an individual’s income, education level, age, and residence type.
For electricity, fuel consumption, and relative consumption, their interactions with individuals’ income are first assessed, the results of which are shown in Column 1. Among all the interaction terms, only the coefficient of the interaction term of fuel expense is negative and significant. This suggests that fuel expenditures have significantly positive effects on life satisfaction for all people, but the effects for higher-income individuals are less than for others.
A similar heterogeneity analysis is conducted as above for the interaction of carbon emissions, electricity consumption, fuel consumption, relative electricity consumption, and relative fuel consumption with individuals’ education level, the results of which are shown in Column 2. The fuel interaction term and relative fuel consumption interaction term have significant effects, and the other relevant interaction terms did not have a significant influence. Thus, for individuals who have higher educational attainment, increased fuel expenses will bring less increase in their life satisfaction than for others, and they are also less likely to compare themselves to their reference groups on fuel consumption.
People’s reactions to energy consumption could vary with their age. In Column 3, electricity, fuel consumption and relative consumption regressors by themselves have significant influences. The fuel consumption interaction and electricity consumption interaction terms have significant and positive effects, which means that older individuals are more sensitive to their energy consumption than younger individuals when evaluating their life satisfaction. And the relative electricity consumption interaction shows a significant negative influence, and relative fuel consumption interaction terms are significantly positive. The results indicate that older people care more about their relative electricity consumption, but care less about their relative fuel consumption than younger ones.
To check for residence type differences in the relationship between life satisfaction and energy consumption, the interactions with residence dummies are computed and are shown in Column 4. In this regression, the relative fuel consumption interaction term has a significant and positive effect, and the other three relevant interaction terms are not significant. This suggests that if urban people consume more fuel than their reference group, the negative effect is less on life satisfaction than it is for rural people.
This study is conducted to expand the body of knowledge by simultaneously extrapolating the carbon emissions, energy consumption, and relative energy consumption factors in the life satisfaction literature. Using CFPS data from 2010 to 2018, we find that the increase in provincial carbon emissions over time predicts increased household life satisfaction, but when the model adds energy consumption-related factors, the effect of carbon emissions becomes less significant. As for energy consumption, increased household energy consumption can proportionally increase life satisfaction. In addition, the results also present that energy consumption is subject to positional concerns, indicating that having more electricity and fuel expenditures than their reference group decreases people’s life satisfaction.
Therefore, the possible reason for the positive relationship between people’s self-reported life satisfaction and carbon emissions is partly due to the demand for more energy consumption. Higher energy consumption brings a higher-carbon form of lifestyle, because some direct and indirect energy consumption, such as lighting, heating, cooling, etc., are major sources of carbon emissions (Lamb and Steinberger, 2017), and these behaviors facilitate individuals’ daily life and enable them to obtain higher life satisfaction (Guo et al., 2016). Thus, the result is in line with previous research indicating that the relationship between life satisfaction and energy consumption reflects the relationship between people’s life satisfaction and carbon emissions (Li and Chen, 2021). In heterogeneity analyses, it is also found that the electricity consumption change has a larger life satisfaction effect for older people. Since fuel is a kind of cheap energy resource (Zheng et al., 2010), for people with lower income, lower education, or older age, fuel consumption increase has a higher life satisfaction effect.
The ratios of individuals’ and references groups’ energy consumption might have social and ethical impacts on people’s life satisfaction, which indicates that the effects of reference energy behaviors are mostly external and relevant to group affiliation characteristics (Chen and Peng, 2014; Mi et al., 2019). The heterogeneity analysis results demonstrate that the relative fuel consumption change has a larger negative effect on life satisfaction for younger, less educated, or rural people. And older people have a higher relative electricity consumption effect on life satisfaction. Therefore, social comparison with the reference group can effectively elicit Chinese residents’ attention to the energy consumption of others, and further influence their life satisfaction. Fuel and electricity expenditures can be categorized as non-positional or basic expenditures, and excess levels of energy consumption might also bring excess carbon emissions and resource depletion. Especially, fuel consumption (such as coal, firewood, charcoal, etc.) also represents traditional energy use and relatively backward lifestyles. Compared to electricity use, this kind of expenditure is not environmentally friendly and produces more carbon emissions (Huang et al., 2021). Therefore, possible explanations of this finding are as follows: first, the explanation of the social comparison, which means people often observe and compare themselves to their reference group, by gathering information or accidentally witnessing other’s lives (Heffetz, 2012), due to their aspiration to be evaluated positively by others and themselves. Fuel and electricity expenditures are kinds of basic consumption, which have not been tied to social status and showing off to others. Veblen’s conspicuous consumption (Veblen, 2005) also indicates that superfluous consumption might not satisfy human needs but simply only demonstrate that one consumes more than others, which does not make people any happier (Okulicz-Kozaryn and Altman 2019). On the contrary, spending more on energy might squeeze on other household expenditures tied to higher social status, such as clothing or cars, especially for lower-income households. Hence, unlike the positional commodities, spending on more energy consumption than others might decrease people’s life satisfaction. The results are in line with Wu (2019), who found that increases in basic consumption expenditures have a negative influence on people’s life satisfaction. Second, the guilty explanation states that a higher level of reference groups’ fuel and electricity expenditure brings happiness to the people because they feel less guilty about the impact of their actions on the environment. According to Burger et al. (2022), people’s behaviors influenced by climate change largely result from feelings of guilt, an ecological worldview, and a desire to play a positive role in society. Regarding the mechanism of a guilty conscience, consuming more energy than the reference group might lead people to feel guilty about their higher energy consumption. But if an individual observes an increase in the reference group’s fuel consumption compared to their own, this might generate lower pressure to reduce their use of energy, and thus increased spending on fuels might lead higher level of life satisfaction. Thus, the experienced guilt about the reference group's energy consumption can be a crucial influence on people’s life satisfaction. These two explanations demonstrate the potential mechanisms of relative energy consumption effects in addition to the other mechanisms discussed in this context.
Given that different regions may exhibit different resource endowments, energy structures, and economic development levels in China, samples in first-tier municipalities are separated from other provinces. According to Guo et al. (2021), as economically developed cities, Beijing and Shanghai have transitioned to service sector-oriented economics, the producer service industries can lead to relatively low carbon emissions. In addition, the energy resources in Beijing and Shanghai are highly reliant on domestic and foreign imports, Beijing also implements local restrictions on enterprises with heavy energy consumption and emissions, which result in a notable energy cost to other provinces. The results show that people living in Beijing and Shanghai have reduced life satisfaction when carbon emissions increase, which reinforces the conclusions of previous research (Dietz et al., 2012), indicating that for developed regions, there is a strong inverse relationship between carbon emissions and individuals’ life satisfactions. In these cities, the energy consumption of themselves and others is less relevant to people’s life satisfaction, since the basic needs for energy consumption in these areas have already been met to a large extent. But for other provinces, energy consumption still plays an important role in bringing about convenience and improving people’s quality of life despite a high level of carbon emissions. This result for these provinces is consistent with Rao and Baer (2012), who indicated that energy and the accompanying emissions are needed to provide people with a decent living standard. Therefore, low-carbon forms of lifestyle should first meet people’s demand for convenience and higher quality of life, and the regional development should be viewed as a necessary condition for adopting a low-carbon policy.
The results for the links and pathways are illustrated in Figure 4.
Future policies should attempt to reconcile environmental and well-being agendas. First, carbon emissions reduction and energy policies should focus on their impact on residents’ life satisfaction, since environmental factors such as carbon emissions, and energy consumption are playing the prescribed roles in people’s quality of life as well. Second, carbon reduction and energy policies should address the regional synergy and the characteristics of different regions across China. For Beijing and Shanghai, a more ambitious target of carbon emissions reduction should be encouraged, since reducing carbon emission meet people’s requirement for higher life quality in these cities. For other provinces, as the increased energy consumption can increase life satisfaction and carbon emissions, the economic structure needs to shift to renewable energy (Huang et al., 2021), to keep life satisfaction unchanged or increasing, while reducing carbon emissions. Third, as life satisfaction is highly influenced by relative energy consumption, to take advantage of energy consumption externalities, advanced publicity can be used to make people believe that low-carbon and energy-saving lifestyles are becoming more and more popular among their reference groups, especially for rural and less educated people, as they are more sensitive to others’ energy consumption. Such policies can enhance residents’ endogenous motivation to save energy and live a low carbon life.
There are some limitations to this research. First, in CFPS data, some psychological factors (such as people’s environmental attitudes, etc.) that might impact the relationship between carbon emissions, energy consumption, and life satisfaction were not included in the analysis, and this may have led to some deviations in the research results. Second, it is assumed that the reference group consists of people with similar ages and similar income levels living in the same province, but this method is to some extent post-inference, although most studies have used the same post-inference strategy (Hou, 2013; Quintana-Domeque and Wohlfart, 2016). For this reason, future research should collect reference group data about respondents’ subjective judgments, environmental attitudes, or other psychological factors, to elaborate more precisely on the mechanisms of influence of relative energy consumption on life satisfaction. Third, the conclusions drawn from this research focusing on China might not be applicable to other countries. In future studies, the sample can include other countries to test the relationship between carbon emissions, energy expenditures, and life satisfaction in other developed countries and developing countries, and a comparative study can be conducted.
The original contributions presented in the study are included in the article/Supplementary Material, further inquiries can be directed to the corresponding author.
JL contributed to conceptualization and writing. FC contributed to formal analysis and methodology. All authors contributed to the article and approved the submitted version.
The authors declare that the research was conducted in the absence of any commercial or financial relationships that could be construed as a potential conflict of interest.
All claims expressed in this article are solely those of the authors and do not necessarily represent those of their affiliated organizations, or those of the publisher, the editors and the reviewers. Any product that may be evaluated in this article, or claim that may be made by its manufacturer, is not guaranteed or endorsed by the publisher.
Akizu-Gardoki, O., Kunze, C., Coxeter, A., Bueno, G., Wiedmann, T., and Lopez-Guede, J. M. (2020). Discovery of a Possible Well-Being Turning Point within Energy Footprint Accounts Which May Support the Degrowth Theory. Energy Sustain. Dev. 59, 22–32. doi:10.1016/j.esd.2020.09.001
Alesina, A., Di Tella, R., and MacCulloch, R. (2004). Inequality and Happiness: Are Europeans and Americans Different? J. Public Econ. 88 (9–10), 2009–2042. doi:10.1016/j.jpubeco.2003.07.00610.1016/j.jpubeco.2003.07.006
Apergis, N., and Majeed, M. T. (2021). Greenhouse Gas Emissions and Cross-National Happiness: a Global Perspective. Air Qual. Atmos. Health 14 (8), 1289–1300. doi:10.1007/s11869-021-01019-5
Arceneaux, K., and Nickerson, D. W. (2017). Modeling Certainty with Clustered Data: A Comparison of Methods. Polit. Anal. 17 (2), 177–190. doi:10.1093/pan/mpp004
Arto, I., Capellán-Pérez, I., Lago, R., Bueno, G., and Bermejo, R. (2016). The Energy Requirements of a Developed World. Energy Sustain. Dev. 33, 1–13. doi:10.1016/j.esd.2016.04.001
Berger, J. (2014). Word of Mouth and Interpersonal Communication: A Review and Directions for Future Research. J. Consumer Psychol. 24 (4), 586–607. doi:10.1016/j.jcps.2014.05.002
Betti, G., Neri, L., Lonzi, M., and Lemmi, A. (2020). Objective Environmental Indicators and Subjective Well-Being: Are They Directly Related? Sustainability 12 (6), 2277. doi:10.3390/su12062277
Brereton, F., Clinch, J. P., and Ferreira, S. (2008). Happiness, Geography and the Environment. Ecol. Econ. 65 (2), 386–396. doi:10.1016/j.ecolecon.2007.07.008
Burger, A. M., Schuler, J., and Eberling, E. (2022). Guilty Pleasures: Moral Licensing in Climate-Related Behavior. Glob. Environ. Change 72, 102415. doi:10.1016/j.gloenvcha.2021.102415
Cheng, Z., Prakash, K., Smyth, R., and Wang, H. (2020). Housing Wealth and Happiness in Urban China. Cities 96, 102470. doi:10.1016/j.cities.2019.102470
Costa, L., Rybski, D., and Kropp, J. P. (2011). A Human Development Framework for CO2 Reductions. PLoS One 6 (12), e29262. doi:10.1371/journal.pone.0029262
Cuñado, J., and de Gracia, F. P. (2013). Environment and Happiness: New Evidence for Spain. Soc. Indic. Res. 112 (3), 549–567. doi:10.1007/s11205-012-0038-4
Dietz, T., Rosa, E. A., and York, R. (2012). Environmentally Efficient Well-Being: Is There A Kuznets Curve? Appl. Geogr. 32 (1), 21–28. doi:10.1016/j.apgeog.2010.10.011
Easterlin, R. A. (1995). Will Raising the Incomes of All Increase the Happiness of All? J. Econ. Behav. Organ. 27, 35–47. doi:10.1016/0167-2681(95)00003-B
El Zoghbi, M. B., and El Ansari, W. (2014). Ethical Concerns and Contributions in Response to Climate Change and the Links to Well-Being: A Study of University Students in the Netherlands. Cent. Eur. J. Public Heal. J. Public Health 22 (2), 118–124. doi:10.21101/cejph.a3998
Fanning, A. L., and O'Neill, D. W. (2019). The Wellbeing-Consumption Paradox: Happiness, Health, Income, and Carbon Emissions in Growing versus Non-growing Economies. J. Clean. Prod. 212, 810–821. doi:10.1016/j.jclepro.2018.11.223
Frank, R. H. (2012). The Easterlin Paradox Revisited. Emotion 12 (6), 1188–1191. doi:10.1037/a0029969
Frank, R. H. (1985). The Demand for Unobservable and Other Nonpositional Goods. Am. Econ. Rev. 75 (1), 101–116. Available at: http://www.jstor.org/stable/1812706
Glaeser, E. L., and Kahn, M. E. (2010). The Greenness of Cities: Carbon Dioxide Emissions and Urban Development. J. Urban Econ. 67 (3), 404–418. doi:10.1016/j.jue.2009.11.006
Guillen-Royo, M. (2011). Reference Group Consumption and the Subjective Wellbeing of the Poor in Peru. J. Econ. Psychol. 32 (2), 259–272. doi:10.1016/j.joep.2009.12.001
Guo, S., Li, Y., He, P., Chen, H., and Meng, J. (2021). Embodied Energy Use of China's Megacities: A Comparative Study of Beijing and Shanghai. Energy Policy 155, 112243. doi:10.1016/j.enpol.2021.112243
Guo, S., Zhao, H., Li, C., Zhao, H., and Li, B. (2016). Significant Factors Influencing Rural Residents' Well-Being with Regard to Electricity Consumption: An Empirical Analysis in China. Sustainability 8 (11), 1132. doi:10.3390/su8111132
Hammerl, M., Dorner, F., Foscht, T., and Brandstaetter, M. (2016). Attribution of Symbolic Brand Meaning: the Interplay of Consumers, Brands and Reference Groups. Journal of Consumer Marketing 33 (1), 32–40. doi:10.1108/jcm-12-2014-1243
Heffetz, O. (2012). Who Sees what? Demographics and the Visibility of Consumer Expenditures. J. Econ. Psychol. 33 (4), 801–818. doi:10.1016/j.joep.2012.02.005
Hong, M., Chen, S., and Zhang, K. (2021). Impact of the "Low-Carbon City Pilot" Policy on Energy Intensity Based on the Empirical Evidence of Chinese Cities. Front. Environ. Sci. 9. doi:10.3389/fenvs.2021.717737
Hou, F. (2013). Keep up with the Joneses or Keep on as Their Neighbours: Life Satisfaction and Income in Canadian Urban Neighbourhoods. J. Happiness Stud. 15 (5), 1085–1107. doi:10.1007/s10902-013-9465-4
Höysniemi, S., and Salonen, A. O. (2019). Towards Carbon-Neutral Mobility in Finland: Mobility and Life Satisfaction in Day-To-Day Life. Sustainability 11 (19), 5374. doi:10.3390/su11195374
Huang, Y., Xue, L., and Khan, Z. (2021). What Abates Carbon Emissions in China: Examining the Impact of Renewable Energy and Green Investment. Sustain. Dev. 29 (5), 823–834. doi:10.1002/sd.2177
Ivanova, D., Stadler, K., Steen-Olsen, K., Wood, R., Vita, G., Tukker, A., et al. (2016). Environmental Impact Assessment of Household Consumption. J. Industrial Ecol. 20, 526–536. doi:10.1111/jiec.12371
Jorgenson, A. K., Alekseyko, A., and Giedraitis, V. (2014). Energy Consumption, Human Well-Being and Economic Development in Central and Eastern European Nations: A Cautionary Tale of Sustainability. Energy Policy 66, 419–427. doi:10.1016/j.enpol.2013.11.020
Jorgenson, A. K. (2014). Economic Development and the Carbon Intensity of Human Well-Being. Nat. Clim. Change 4 (3), 186–189. doi:10.1038/nclimate2110
Kaus, W. (2013). Conspicuous Consumption and “Race”: Evidence from South Africa. J. Dev. Econ. 100 (1), 63–73. doi:10.1016/j.jdeveco.2012.07.004
Kerkhof, A. C., Benders, R. M. J., and Moll, H. C. (2009). Determinants of Variation in Household CO2 Emissions between and within Countries. Energy Policy 37 (4), 1509–1517. doi:10.1016/j.enpol.2008.12.013
Kumari, N., Kumar, P., and Sahu, N. C. (2021). Do Energy Consumption and Environmental Quality Enhance Subjective Wellbeing in G20 Countries? Environ. Sci. Pollut. Res. 28 (42), 60246–60267. doi:10.1007/s11356-021-14965-5
Lamb, W. F., and Steinberger, J. K. (2017). Human Well‐being and Climate Change Mitigation. WIREs Clim. Chang. 8 (6). doi:10.1002/wcc.485
Lelieveld, J., Klingmüller, K., Pozzer, A., Pöschl, U., Fnais, M., Daiber, A., et al. (2019). Cardiovascular Disease Burden from Ambient Air Pollution in Europe Reassessed Using Novel Hazard Ratio Functions. Eur. Heart J. 40 (20), 1590–1596. doi:10.1093/eurheartj/ehz135
Li, J., and Raine, J. W. (2013). The Time Trend of Life Satisfaction in China. Soc. Indic. Res. 116 (2), 409–427. doi:10.1007/s11205-013-0300-4
Li, Q., and Chen, H. (2021). The Relationship between Human Well-Being and Carbon Emissions. Sustainability 13 (2), 547. doi:10.3390/su13020547
Liu, X. Y., Wang, L. M., and Zhu, S. W. (2016). Urban Sprawl and Residential Carbon Emission: Panel Data on Southern Cities in China. J. Southeast Univ. Philos. Soc. Sci. 18, 101–108+148. doi:10.13916/j.cnki.issn1671-511x.2016.05.014
Maurer, J., and Meier, A. (2008). Smooth it like the 'Joneses'? Estimating Peer‐Group Effects in Intertemporal Consumption Choice. Econ. J. 118 (527), 454–476. doi:10.1111/j.1468-0297.2007.02129.x
Mi, L., Zhu, H., Yang, J., Gan, X., Xu, T., Qiao, L., et al. (2019). A New Perspective to Promote Low-Carbon Consumption: The Influence of Reference Groups. Ecol. Econ. 161, 100–108. doi:10.1016/j.ecolecon.2019.03.019
Mood, C. (2009). Logistic Regression: Why We Cannot Do What We Think We Can Do, and What We Can Do about it. Eur. Sociol. Rev. 26 (1), 67–82. doi:10.1093/esr/jcp006
Okulicz-Kozaryn, A., and Altman, M. (2019). The Happiness-Energy Paradox: Energy Use Is Unrelated to Subjective Well-Being. Appl. Res. Qual. Life 15 (4), 1055–1067. doi:10.1007/s11482-019-09719-y
Palm, A. (2017). Peer Effects in Residential Solar Photovoltaics Adoption-A Mixed Methods Study of Swedish Users. Energy Res. Soc. Sci. 26, 1–10. doi:10.1016/j.erss.2017.01.008
Roychowdhury, P. (2019). Peer Effects in Consumption in India: an Instrumental Variables Approach Using Negative Idiosyncratic Shocks. World Dev 114, 122–137. doi:10.1016/j.worlddev.2018.09.028
Qiu, Y. (2017). Deeply Understands the Changes of the Main Social Contradictions in China. Available at: http://theory.people.com.cn/GB/n1/2017/1216/c40531-29710999.html (Accessed November 15, 2021).
Quintana-Domeque, C., and Wohlfart, J. (2016). "Relative Concerns for Consumption at the Top": An Intertemporal Analysis for the UK. J. Econ. Behav. Organ. 129, 172–194. doi:10.1016/j.jebo.2016.06.005
Rao, N. D., and Baer, P. (2012). "Decent Living" Emissions: A Conceptual Framework. Sustainability 4 (4), 656–681. doi:10.3390/su4040656
Shan, Y., Huang, Q., Guan, D., and Hubacek, K. (2020). China CO2 Emission Accounts 2016-2017. Sci. Data 7 (1), 54. doi:10.1038/s41597-020-0393-y
Sugiawan, Y., Kurniawan, R., and Managi, S. (2019). Are Carbon Dioxide Emission Reductions Compatible with Sustainable Well-Being? Appl. Energy 242, 1–11. doi:10.1016/j.apenergy.2019.03.113
Sulkowski, A., and White, D. S. (2015). A Happiness Kuznets Curve? Using Model-Based Cluster Analysis to Group Countries Based on Happiness, Development, Income, and Carbon Emissions. Environ. Dev. Sustain. 18 (4), 1095–1111. doi:10.1007/s10668-015-9689-z
Summers, J. K., Smith, L. M., Case, J. L., and Linthurst, R. A. (2012). A Review of the Elements of Human Well-Being with an Emphasis on the Contribution of Ecosystem Services. Ambio 41 (4), 327–340. doi:10.1007/s13280-012-0256-7
Sun, W., and Wang, X. (2013). Do Relative Income and Income Inequality Affect Consumption? Evidence from the Villages of Rural China. J. Dev. Stud. 49 (4), 533–546. doi:10.1080/00220388.2012.740017
Sun, X., and Wang, Z. (2021). Can Chinese Families Achieve a Low-Carbon Lifestyle? an Empirical Test of China's Low-Carbon Pilot Policy. Front. Energy Res. 9. doi:10.3389/fenrg.2021.655733
Tajfel, H. (2003). Social Psychology of Intergroup Relations. Annu. Rev. Psychol 33, 1–39. doi:10.1146/annurev.ps.33.020182.000245
Tonon, G., and Joseph Sirgy, M. (2021). A Review of the Psychology of Quality of Life: Hedonic Well-Being, Life Satisfaction, and Eudaimonia. Appl. Res. Qual. Life 8 (2), 263–264. doi:10.1007/s11482-013-9228-8
Veblen, T. (2005). The Theory of the Leisure Class; an Economic Study of Institutions. New York: Aakar Books.
Vita, G., Ivanova, D., Dumitru, A., García-Mira, R., Carrus, G., Stadler, K., et al. (2020). Happier with Less? Members of European Environmental Grassroots Initiatives Reconcile Lower Carbon Footprints with Higher Life Satisfaction and Income Increases. Energy Res. Soc. Sci. 60, 101329. doi:10.1016/j.erss.2019.101329
Wang, H., Cheng, Z., and Smyth, R. (2017). Consumption and Happiness. J. Dev. Stud. 55 (1), 120–136. doi:10.1080/00220388.2017.1371294
Wang, J., Yan, W., and Zhang, J. (2019). Relative Income and Subjective Well-Being of Urban Residents in China. J. Fam. Econ. Iss 40, 673–680. doi:10.1007/s10834-019-09636-0
Welsch, H., and Kühling, J. (2009). Determinants of Pro-environmental Consumption: The Role of Reference Groups and Routine Behavior. Ecol. Econ. 69 (1), 166–176. doi:10.1016/j.ecolecon.2009.08.009
Wolske, K. S., Gillingham, K. T., and Schultz, P. W. (2020). Peer Influence on Household Energy Behaviours. Nat. Energy 5 (3), 202–212. doi:10.1038/s41560-019-0541-9
Wu, F. (2019). An Examination of the Effects of Consumption Expenditures on Life Satisfaction in Australia. J. Happiness Stud. 21 (8), 2735–2771. doi:10.1007/s10902-019-00161-3
Yin, J., and Shi, S. (2021). Social Interaction and the Formation of Residents′ Low-Carbon Consumption Behaviors: An Embeddedness Perspective. Resour. Conservation Recycl. 164, 105116. doi:10.1016/j.resconrec.2020.105116
Yuan, H. (2015). Structural Social Capital, Household Income and Life Satisfaction: The Evidence from Beijing, Shanghai and Guangdong-Province, China. J. Happiness Stud. 17 (2), 569–586. doi:10.1007/s10902-015-9622-z
Zhang, K., Pei, J., Wang, S., Rokpelnis, K., and Yu, X. (2022). Life Satisfaction in China, 2010-2018: Trends and Unique Determinants. Appl. Res. Qual. Life. doi:10.1007/s11482-021-10031-x
Zhang, X., Zhang, X., and Chen, X. (2017). Happiness in the Air: How Does a Dirty Sky Affect Mental Health and Subjective Well-Being? J. Environ. Econ. Manag. 85, 81–94. doi:10.1016/j.jeem.2017.04.001
Zheng, S., Wang, R., Glaeser, E. L., and Kahn, M. E. (2010). The Greenness of China: Household Carbon Dioxide Emissions and Urban Development. J. Econ. Geogr. 11 (5), 761–792. doi:10.1093/jeg/lbq031
Keywords: carbon emissions, energy consumption, life satisfaction, reference group, relative energy consumption
Citation: Li J and Chen F (2022) The Impacts of Carbon Emissions and Energy Consumption on Life Satisfaction: Evidence From China. Front. Environ. Sci. 10:901472. doi: 10.3389/fenvs.2022.901472
Received: 22 March 2022; Accepted: 19 April 2022;
Published: 04 May 2022.
Edited by:
Haikun Wang, Nanjing University, ChinaReviewed by:
Yasir Khan, Anhui Polytechnic University, ChinaCopyright © 2022 Li and Chen. This is an open-access article distributed under the terms of the Creative Commons Attribution License (CC BY). The use, distribution or reproduction in other forums is permitted, provided the original author(s) and the copyright owner(s) are credited and that the original publication in this journal is cited, in accordance with accepted academic practice. No use, distribution or reproduction is permitted which does not comply with these terms.
*Correspondence: Jiaying Li, amFjcXVlbGluZWxqeUAxMjYuY29t
Disclaimer: All claims expressed in this article are solely those of the authors and do not necessarily represent those of their affiliated organizations, or those of the publisher, the editors and the reviewers. Any product that may be evaluated in this article or claim that may be made by its manufacturer is not guaranteed or endorsed by the publisher.
Research integrity at Frontiers
Learn more about the work of our research integrity team to safeguard the quality of each article we publish.