- 1Interdisciplinery Centre for Water Research, Indian Institute of Science, Bangalore, India
- 2Athabasca River Basin Research Institute (ARBRI), Athabasca University, Edmonton, AB, Canada
- 3Institute of Bio- and Geosciences, Agrosphere (IBG-3), Forschungszentrum Jülich GmbH, Jülich, Germany
The natural carbon dioxide (CO2) emission from the ecosystem, also termed as the ecosystem respiration (Reco), is the primary natural source of atmospheric CO2. The contemporary models rely on empirical functions to represent decomposition of litter with multiple soil carbon pools decaying at different rates in estimating Reco variations and its partitioning into autotrophic (Ra) (originating from plants) and heterotrophic (originating mostly from microorganisms) respiration (Rh) in relation to variation in temperature and soil water content. Microbially-mediated litter decomposition scheme representation are not very popular yet. However, microbial enzymatic processes play integral role in litter as well as soil organic matter (SOM) decomposition. Here we developed a mechanistic model comprising of multiple hydro-biogeochemical modules in the soil and water assessment tool (SWAT) code to explicitly incorporate microbial-enzymatic litter decomposition and decomposition of SOM for separately estimating regional-scale Ra, Rh and Reco. Modeled annual mean Reco values are found varying from 1,600 to 8,200 kg C ha−1 yr−1 in 2000–2013 within the boreal forest covered sub-basins of the Athabasca River Basin (ARB), Canada. While, for the 2000–2013 period, the annual mean Ra, Rh and soil CO2 emission (Rs) are varying within 800–6,000 kg C ha−1 yr−1, 700–4,200 kg C ha−1 yr−1 and 1,200–5,000 kg C ha−1 yr−1, respectively. Rs generally dominates Reco with nearly 60–90% contribution in most of the sub-basins in ARB. The model estimates corroborate well with the site-scale and satellite-based estimates reported at similar land use and climatic regions. Mechanistic modeling of Reco and its components are critical to understanding future climate change feedbacks and to help reduce uncertainties particularly in the boreal and subarctic regions that has huge soil carbon store.
Introduction
The natural carbon dioxide (CO2) emission from the ecosystem, also termed as the ecosystem respiration (Reco) is the primary natural source of CO2 throughout the globe (Ciais et al., 2014). There is an urgent need to completely understand the soil carbon conversion processes and the associated land-surface gas exchange in estimating climate change feedback, which ultimately leads to the release of soil carbon as CO2 to the atmosphere (Mitchard, 2018). In natural ecosystems, Reco can be partitioned into the soil heterotrophic respiration (Rh) that mostly resulting from soil microorganisms and the autotrophic respiration (Ra) resulting from plant species (both from aboveground components and roots) (Hicks Pries et al., 2013; Hicks Pries et al., 2016). Globally, Ra is the predominant component of the terrestrial carbon budget with photosynthetic carbon consumption rate of 54–71% (Ryan et al., 1997). In permafrost regions, Ra accounts for 40–70% of the total ecosystem respiration (Hicks Pries et al., 2013).
The Ra and Rh have different adaptation mechanisms in response to climate change. For example, rising temperature can accelerate microbial enzymatic activities and thus enhance Rh and facilitate nutrient dynamics based on substrate availability (Davidson and Janssens, 2006; Manzoni et al., 2008). On the other hand, rising temperature has different influence in plant growth. The aboveground component on Ra shows positive response while root shows near neutral response (Hick Pries et al., 2013). For example, 2.3°C warming of soil leads to 20% increase in aboveground productivity at an Alaskan site (Natali et al., 2012). With an increase of 2°C, root respiration didn’t show any significant changes, while heterotrophic respiration did 21% increase linked with increased microbial activities (Wang et al., 2014). Therefore, quantifying the distinct contributions of Reco, Ra, and Rh is necessary to estimate climate feedbacks and sensitivity in the rapidly changing subarctic regions but state of the art monitoring techniques, such as eddy covariance and remote sensing techniques, are still unable to directly partition Ra and Rh from Reco (Davidson and Janssens, 2006; Konings et al., 2019). In addition to the distinct characteristics and feedbacks to climate change processes by Ra and Rh, respiration partitioning is particularly important as it has been reported that global land carbon sink has been increasing in recent years (Ciais et al., 2019).
Climate change-linked future projections of ecosystem respiration are challenging because estimating litter and soil organic matter (SOM) decomposition rates and anticipated soil derived CO2 feedbacks resulting from anthropogenic warming are both seen as being highly uncertain (Collins et al., 2013; Crowther et al., 2016). Many experimental and modelling studies have been performed to determine the Reco and its partitioning and gross primary production (GPP) at different land use types (Hardie et al., 2009; Hicks Pries et al., 2013; Senapati et al., 2018). On the other hand, predicting the net ecosystem exchange (NEE), and Reco require the development of sophisticated land-surface models. These modeling approaches can be categorized into: 1) agroecosystem models (models used to simulate agricultural system functioning), and 2) the Earth System Models (ESMs - that are used to simulate Earth system processes). Both modelling approaches commonly use empirical formula of multiple soil C pools decaying at different rates to calculate Reco (Del Grosso et al., 2005; Davison and Janssens, 2006; Oleson et al., 2010; Clark et al., 2011). The CENTURY model used simplified functions of soil temperature and moisture for estimating soil respiration and its partition to autotrophic and heterotrophic components (Del Grosso et al., 2005). The decaying module of multiple soil C pools in Daily CENTURY model (DayCent) has also been incorporated into Organising Carbon and Hydrology In Dynamic Ecosystems (ORCHIDEE) (Qiu et al., 2018) and Community Land Model (CLMs) (Lawrence et al., 2019). The microbial processes in ESMs are simplified into linear, empirical equations (Crowther et al., 2014; Crowther et al., 2019). It is found that contemporary ESMs cannot reproduce grid-scale variation in soil C due to missing key processes and the predicted global carbon stocks in the fifth Coupled Model Intercomparison Project (CMIP), leading to 6-fold difference in predicted data (Todd-Brown et al., 2013). Microbial activity responses (as reflected in soil heterotrophic respiration) are expected to increase with warming (Karhu et al., 2014; Walker et al., 2018). However, the relationship between warming and soil carbon loss, as well as overall ecosystem respiration, is not straightforward as the microbially mediated litter and SOM decomposition processes are not linearly correlated with temperature (Melillo et al., 2017). Additionally, microbially-mediated decomposition of SOM is not only an important biological-driven process for carbon conversion but also play a major role in overall global nutrient dynamics (Manzoni et al., 2008).
Apart from the environmental variables, the turnover of organic material is directly controlled by soil microbes (Crowther et al., 2019). As a result, there is a need to improve microbial processes for global carbon modeling estimates (Wieder et al., 2013; Crowther et al., 2019). Microbial abundances are relatively larger in arctic and subarctic regions (Serna-Chavez et al., 2013; Xu et al., 2013). They are also responsible for biogeochemical cycling of nutrients (Crowther et al., 2019). However, different types of microbes require favorable soil redox conditions for their growth (DeAngelis et al., 2010). Thus, soil redox condition is an essential measure for the dynamics of nutrients as well as soil organic matter, which microbes used as a substrate (Bhanja et al., 2019a; Bhanja et al., 2019b). It has been found that nutrient availability directly controls soil organic matter stock and terrestrial carbon sink (Wieder et al., 2015). Alteration of hydrological processes has profound impact in soil carbon mineralization (Anthony et al., 2018). Therefore, integration of hydrological processes along with redox/biogeochemical and microbial processes would definitely improve model estimates (Bhanja et al., 2019a; Bhanja et al., 2019b). Global-scale respiration studies continue to be sought for further improving the modeling processes and soil respiration estimates (Todd-Brown et al., 2013; Luo et al., 2016; Wang et al., 2020; Wang et al., 2021). A decomposition feedback to warming requires accounting explicitly for not only temperature but also nutrient availability and microbial activities (Davidson et al., 2012).
Substantial amount of soil organic carbon can be released due to enhanced microbial activities associated with climate warming in arctic, subarctic regions (Schuur et al., 2015). The arctic and subarctic regions are the most sensitive regions to the combined climate change impacts due to declining permafrost, glacial retreat and change in freeze-thaw cycles on its ecosystems (Bates et al., 2008). An abrupt thawing in lakes due to global warming can lead to faster mobilization of deeper stored carbon (Anthony et al., 2018). Therefore, in these regions, modeling the carbon mobilization processes and their feedbacks are much sought after (Schuur et al., 2009). The present study describes the development of a mechanistic model to separately simulate regional-scale, autotrophic, heterotrophic and total ecosystem respirations. This being achieved through an explicitly integrated inclusion of microbially-mediated decomposition of litter as well as SOM, redox processes and hydrological processes. The model performance was assessed at the boreal forest covered Athabasca River Basin (ARB), Canada (Fig. S1) using both site-scale, as well as remote-sensing data.
Methodology
Model Description
The Soil and Water Assessment Tool (SWAT) is widely used for simulating regional-scale hydrology due to its comparatively lower computational requirements (Arnold et al., 1998). Integrated hydro-biogeochemical modeling was performed at a daily time step. Our microbial kinetics and thermodynamics (MKT) model is integrated within SWAT framework, known as SWAT-MKT (Bhanja et al., 2019a; Bhanja et al., 2019b; Bhanja and Wang, 2020). While the SWAT calculates hydrological processes, MKT evaluates microbially-mediated dynamics of biogeochemical processes in soils. At daily time step, two models exchange data and update their variables. In this process, MKT rely on daily soil moisture and temperature simulation from SWAT and independently simulate its biogeochemical processes. The oxygen diffusion and related oxidizing reactions are also modelled. Oxygen diffusion processes are described in supplementary information.
In order to simulate the processes of multiple chemical reactions, microbial kinetics and thermodynamics are simultaneously considered in SWAT-MKT model. The energy balance and sequence of the chemical reaction to be simulated is modeled through thermodynamics approach following the Nernst equation. Considering a chemical reaction:
Where, A, A- are the normal and reduced form of the chemical component modeled, respectively. D and D+ are the chemical component that donate electron in the reaction and its oxidized form, respectively. x, y, p and q, are the stoichiometric coefficients of reactants and products.
The redox potential (Eh) dynamics was modeled following the Nernst’s equation (Stumm and Morgan, 1996).
Where, Eh and E0 are the redox potential (mV) and the standard electrode potential (mV), respectively. R is the universal gas constant; T is the ambient temperature in Kelvin; n is the number of electrons transferred in the chemical reactions; F and Q are the Faraday’s constant and the reaction quotient, respectively. ‘[ ]’ represents concentration in molality and ‘γ’ is the activity coefficients in molality.
The chemical reactions in soil-water zone occur at a sub-daily time step catalyzed by various microbes. Otherwise, if the reactions would have happen in thermodynamic control only, the rates would be much slower e.g. in microbe limiting conditions, in groundwater systems. The microbes catalyzed the reactions after taking energy from the system. We have considered this non equilibrium condition by after computing and removing the microbial energy requirement from the system and updated the net redox potential at a daily time step (for details, please check Bhanja et al., 2019a).
The mass balance of different chemical species is achieved through modeling microbial kinetics for estimating the reaction rates of individual reactions at a daily time step. Dual Michaelis-Menten kinetics is followed here, the reaction rate can be represented as:
Where, rate of the reaction is represented by R in molal/day; specific microbial activity as Qmax in mol/mol of biomass per day; B is the microbial biomass in mole biomass/l of water; [D] is the concentration of the electron donor species in Eq (1) in molal; [A] is the concentration of the electron acceptor species in molal, and KD and KA are the half saturation constants for the electron donor and acceptor species, respectively in mole/l. KD and KA are otherwise termed as the Michaelis-Menten constants.
Major soil oxidation-reduction reactions considered in this approach were given in Supplementary Table S1 and their reaction quotient values are provided in Supplementary Table S2. The reactions are shown in Supplementary Figure S3 and further details are provided in Bhanja et al. (2019a); Bhanja et al. (2019b) and Bhanja and Wang (2020).
New carbon cycle capabilities are incorporated into SWAT to simulate ecosystem respiration components from litter decomposition, root respiration, above ground respiration and respiration component from the dissolved organic carbon transformation by enzymatic processes (Supplementary Figures S2, 3). The entire chemical processes considered in the new version of SWAT-MKT are shown in Supplementary Figure S3.
Various spatial and meteorological datasets are used to built-up the SWAT model for the ARB. Shuttle Radar Topography Mission (SRTM)-based Digital Elevation Model (DEM) data, at a resolution of 90 m × 90 m, are obtained from the Consultative Group on International Agricultural Research (CGIAR) (Jarvis et al., 2008). Land-use data are obtained at 1 km × 1 km, from the International Geosphere-Biosphere Programme, Data and Information Systems (IGBP-DIS) initiative archived at USGS (Loveland et al., 2000). The soil map is obtained from the Agriculture and Agri-Food Canada at 1:1 million resolution (SLC, 2010). Elevation ranges from 207 to 3,669 m in the ARB. We have defined 320 soil classes and 11 land-use classes. Watershed delineation through SWAT with a 200 km2 threshold gives rise to 131 sub-basins within the ARB. Hydrologic Response Units (HRU) are defined using four slope classes: 5, 10, 15 and 20%, and with 10, 5 and 10% thresholds for land-use, soil and slope, respectively. A total of 1,370 HRUs are obtained through this process in this the region. Meteorological data are used at a daily scale for precipitation, minimum and maximum temperature, were obtained from the GoC (2016) database. Solar radiation, relative humidity and wind speed data are obtained for 230 stations from CFSR (CFSR, 2016). Details on these data processing and model built-up can be found in Shrestha et al. (2017).
Soil Mineralization and Litter Decomposition
The equations should be inserted in editable format from the equation editor.
Soil mineralization module is modeled considering two carbon pools: active and passive. The active pool represents fraction of active litter including microbial biomass (Fujita et al., 2014). We used microbial enzymatic litter transformation approach that is an advancement of the CENTURY model’s simple, first order kinetics-based litter decomposition approach (Parton et al., 1987; Parton et al., 1994; Fujita et al., 2014):
Where, litter decomposition rate originally adopted in CENTURY model:
Litter decomposition can also be modeled through microbial enzymatic approach following one-substrate Michaelis-Menten kinetics (Fujita et al., 2014). Thus, the new decomposition rate becomes:
Where, Kmi is the half-saturation constant or Michaelis-Menten constant.
Where,
If considering microbial biomass being an active component of the litter, microbial biomass decomposition can directly be assumed to be proportional to the litter decomposition. The new litter decomposition rate (
Actual soil respiration rates (RLD) from litter transformation is estimated as follows (Fujita et al., 2014).
Where, ei,m represents the growth efficiency of microbes during assimilation of either active or passive substrates and its value is estimated as 0.45 (Fujita et al., 2014). Im,c is an decomposition inhibition factor (its value varies from 0 for full to one for no inhibition) and at present its value taken as one also resembles the CENTURY model parameterization (Fujita et al., 2014). Om,c is the overflow of carbon due to limiting nitrogen concentration and its value is taken as 0 without proper data to represent the processes.
Root Respiraton
Root respiration (Rr) is an essential component of soil respiration. However, SWAT does not have the ability to simulate root respiration. To simulate root respiration, we have developed a new sub-module within SWAT following Li et al. (1994):
Where, CO2 produced by roots for nitrogen uptake: Rn (13.8 mg C meq−1 N; Veen, 1981; Li et al., 1994). Nitrogen uptake rates of plant is represented as Un (kg N ha−1 d−1). CO2 produced by roots due to their growth: Rrg (19.19 mg C g−1 dry matter; Veen, 1981; Li et al., 1994). Root biomass growth at a day: BGr (g dry matter ha−1). CO2 produced as a function of root maintenance: Rrm (0.288 mg C g−1 dry matter d−1; Veen, 1981; Li et al., 1994). Blr is the living root biomass (g dry matter ha−1).
Above-Ground Autotrophic Respiration
Above ground respiration (Rabv) is estimated following the equation (Ryan et al., 1994):
Where, aboveground foliar biomass growth at a day: BGabvf (g dry matter ha−1 d−1). CO2 produced as a function of aboveground foliar biomass growth: Rabvf (1.767 mgC g−1 dry matter d−1; Ryan et al., 1994). Aboveground woody biomass growth at a day: BGabvw (g dry matter ha−1 d−1). CO2 produced as a function of aboveground woody biomass growth: Rabvw (0.12 mgC g−1 dry matter d−1; Ryan et al., 1994).
Satellite-Based Estimates of Autotrophic Respiration
We used gross primary production (GPP) and net primary productivity (NPP) data from the observation of the Moderate Resolution Imaging Spectroradiometer (MODIS) sensors (Zhao et al., 2005; Zhao et al., 2006; Zhao and Running, 2010). Annual mean MOD17 products are used at a spatial resolution of 30 arcsec. MOD17 is the first satellite derived continuous data product for vegetation productivity at the global-scale (Zhao et al., 2006). The algorithm includes several satellite derived parameters such as the land cover, fractional photosynthetically active radiation and leaf area index along with the meteorological variables (Zhao et al., 2006). NCEP/DOE reanalysis II outputs are used for meteorological parameters (Zhao and Running, 2010). Detailed descriptions of the MOD17 products can be found in Zhao et al. (2005); Zhao et al. (2006). Satellite-based Ra is estimated by subtracting NPP from the GPP data (Bond-Lamberty et al., 2018).
Assumptions and Limitations
In order to compute the total soil respiration, we only used the heterotrophic and autotrophic components. Here, we have not considered the geological CO2 emission (Andrews and Schlesinger, 2001). Ecosystem respiration also includes animal respiration. However, due to the cold climatic conditions and presence of very low number of animals at the ARB (Weber et al., 2015), the respiration from animals are not considered at present in our approach. Other assumptions and limitations associated with the basic version of the model can be obtained from Bhanja et al. (2019a); Bhanja et al. (2019b) and Bhanja and Wang (2020). In natural conditions, all of the soil microbes do not produce enzymes or produce at a slower rate to take part in the decomposition activities. These types of microbes restrict/slow down the decomposition process (Kaiser et al., 2015).
Results and Discussion
Heterotrophic, Autotrophic and Total Ecosystem Respiration
Annual mean ecosystem respiration (Reco) did show spatial variability, however, most of the predicted values for the 2000–2013 period are in the range of 1,600–8,200 kg C ha−1 yr−1 (Figure 1). The Reco estimates were compared with the available site-scale measurements from the Canadian boreal forest locations data retrieved from FLUXNET 2015 (Pastorello et al., 2017). Most of the site-scale Reco values vary within 4,000–10,000 kg C ha−1 yr−1, but with values on three sites exceeding 11,000 kg C ha−1 yr−1 (Supplementary Table S3). The modeled Reco estimates were in lower range compared to the other boreal forest Reco observations shown in Supplementary Table S3. These reported sites are however located either at more southern or the same latitude as our study area. Therefore, overall the prevailing climatic conditions at the ARB are more nudging towards a arctic-like climate, with more limited respiration rates. In general, the respiration values were lower during winter months, both climatic factors and the presence of deciduous trees do account for these lower rates (Cumming, 2001). The mean heterotrophic respiration (Rh) values did mostly vary from 700 to 4,200 kg C ha−1 yr−1 in 2000–2013 at the ARB (Figure 1). The data showed strong seasonality with highest Rh values occurring during the summer months. Although, no actual measurements are available within Athabasca River basin (ARB), estimates from other Canadian boreal forests are well within the range of our modelled estimates in all of the studied locations except the Quebec sites and one Saskatchewan site (Supplementary Table S4) retrieved from the SRDB archives (Bond-Lamberty and Thomson, 2010). The Quebec sites are located far southern areas with little warmer climate and thus exhibiting higher Rh. Most of these site-scale values varied between 1700 and 5,900 kg C ha−1 yr−1, but on a few occasions (Quebec sites) with values of >10,000 kg C ha−1 yr−1 were recorded (Supplementary Table S4). Mean root respiration (Rr) values (300–2,800 kg C ha−1 yr−1) were however found to be lower than the mean Rh values in our study at ARB (Figure 1). In general, Rh is found to be higher than Rr in global boreal sites (Bond-Lamberty and Thomson, 2010) and at an Alaskan site (Hicks Pries et al., 2013). Rh is not only contributing higher toward Rs, the contribution rate has been increased from 54 to 63% over the years during 1990–2014 at a global study (Bond-Lamberty et al., 2018). Annual mean autotrophic respiration from above-ground vegetation components (Rabv) did vary from 200 to 2,900 kg C ha−1 yr−1. In general, the combination of Rr and Rabv that is the mean autotrophic respiration (Ra) is estimated to be higher than the mean Rh (Figure 1). Annual mean Ra varies within 800–6,000 kg C ha−1 yr−1 (Figure 1). Most of the ARB is covered by forests (Bhanja et al., 2018), which may account for the higher magnitude of autotrophic respiration compared to its heterotrophic counterpart. Contribution of above-ground canopy respiration to Reco is significant at boreal forests (Ryan et al., 1997) and at arctic climates (Hick Pries et al., 2013). Thus Rabv along with the Rr, makes Ra dominant over Rh in forest. The Ra dominancy was also observed in savanna and grasslands (Ma et al., 2007), and in peatland ecosystems (Hardie et al., 2009). The autotrophic and heterotrophic respiration estimates are well in line with the data reported in Goulden et al. (2011) from Canadian boreal forests with values reported for Ra was estimated at 2000–4,500 kg C ha−1 yr−1 and Rh at ∼2000 kg C ha−1 yr−1. Ra reported by Ryan et al. (1997) at eight Canadian boreal forest sites with values between 3,120 and 6,110 kg C ha−1 yr−1 are also matching our modelled estimates. Bond-Lamberty et al. (2010) reported Rh within 200–6,000 kg C ha−1 yr−1 at boreal locations and 100–900 kg C ha−1 yr−1 at arctic locations across the globe. In general, mean soil respiration (Rs) also show spatial patterns with values from 1,200 to 5,000 kg C ha−1 yr−1 at the ARB (Figure 1). The lower values of Rs were mainly found at the Southern ARB sub-basins dominated by mountains. The Rs values do also well comparable with the site-scale estimates from many of the other Canadian boreal sites except those at Quebec (located in southern latitude with warmer climate) and some Saskatchewan sites (Supplementary Table S4).
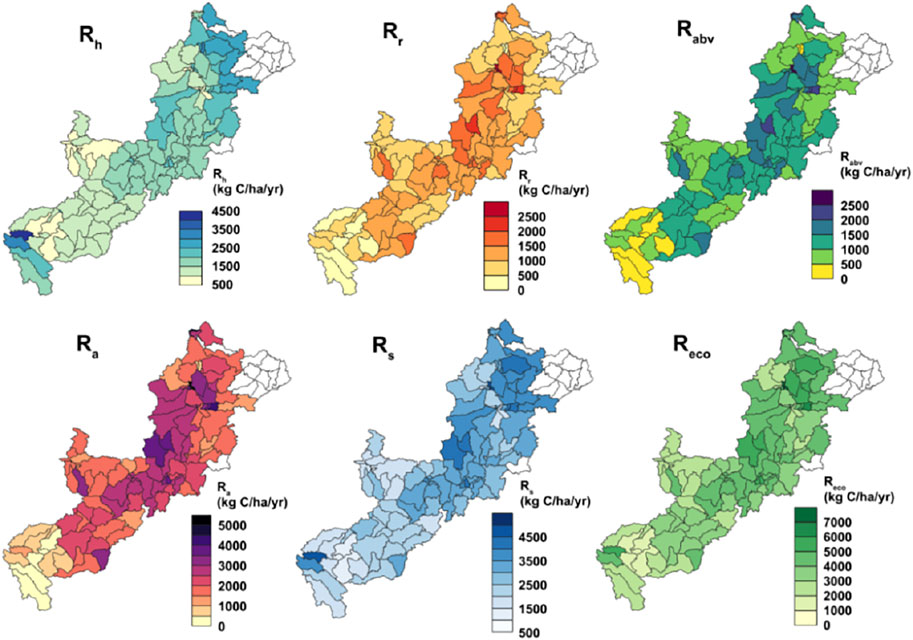
FIGURE 1. Subbasin-wise, long-term modeled (2000–2013) annual average maps of soil heterotrophic respiration (Rh), root respiration (Rr), autotrophic respiration from above-ground autotrophic respiration (Rabv), autotrophic respiration (Ra), soil respiration (Rs), ecosystem respiration (Reco) in kg C ha−1 yr−1. The northernmost regions are not modelled and are shown blank.
Relationships Between the Respiration Components
Relationship between Rh and Rs shows near equal contribution of soil autotrophic and heterotrophic respiration to total soil respiration in most of the sub-basins (Figure 2). Root contribution to total soil respiration (RC) values did varies from 0.1 to 0.6 (occasionally 0.7). This generally matches the field-scale estimates, which further increases our confidence in the modelled estimates (Supplementary Table S4). The ratio of Ra to Reco did show varying contribution of Ra to Reco from 30 to 80% in most of the sub-basins (Figure 2). The estimates are well within the ranges reported in previous studies (40–80% in Nowinski et al., 2010; 40–70% in Hicks Pries et al., 2013) at similar eco-climatic regions. Rs contributes to nearly 60–90% of Reco in most parts of the study area (Figure 2). Contribution of Rr to Reco lies within 10–45%. Results are consistent with the observation of Hicks Pries et al. (2013) at arctic climate (15–35% contribution reported).
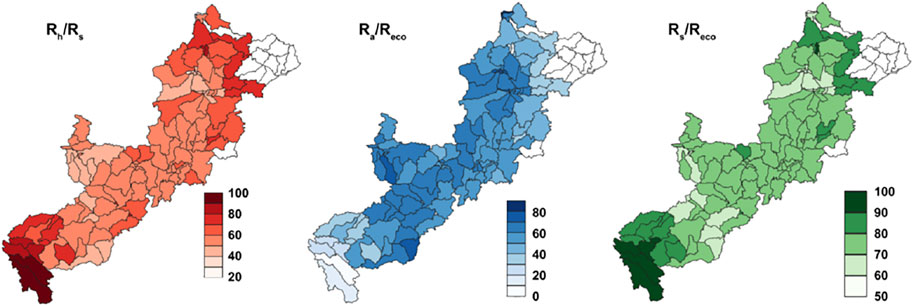
FIGURE 2. Maps of the average percentage (%) ratios of soil respiration (Rs) and heterotrophic respiration (Rh), autotrophic respiration (Ra) and ecosystem respiration (Reco), Rs and Reco, respectively in 2000–2013. The northernmost regions are not modelled and are shown blank.
ARB is mostly covered by forest (Supplementary Figure S4) and occurrence of comparatively lower annual mean soil temperature (<2°C, Supplementary Figure S5) are the two main reasons for the dominance of autotrophic respiration toward the total ecosystem respiration (Ryan et al., 1997; Hicks Pries et al., 2013; Crowther et al., 2016). The relative proportions of Rh to Reco were also found to be consistent with the values reported in previous studies (Hardie et al., 2009; Schuur et al., 2009; Hicks Pries et al., 2013).
The respiration partitioning and their ratio show some interesting facts. Our work shows that the relationships between Rs and Reco and Ra and Reco follow linear relationship (r2 > 0.74, p < 0.001, Figures 3B, C). Relationship between Rh and Rs follow linear pattern (r2 = 0.61, p < 0.001, Figure 3A). Similar relationships are also observed by Bond-Lamberty et al. (2004) in different locations across the globe.
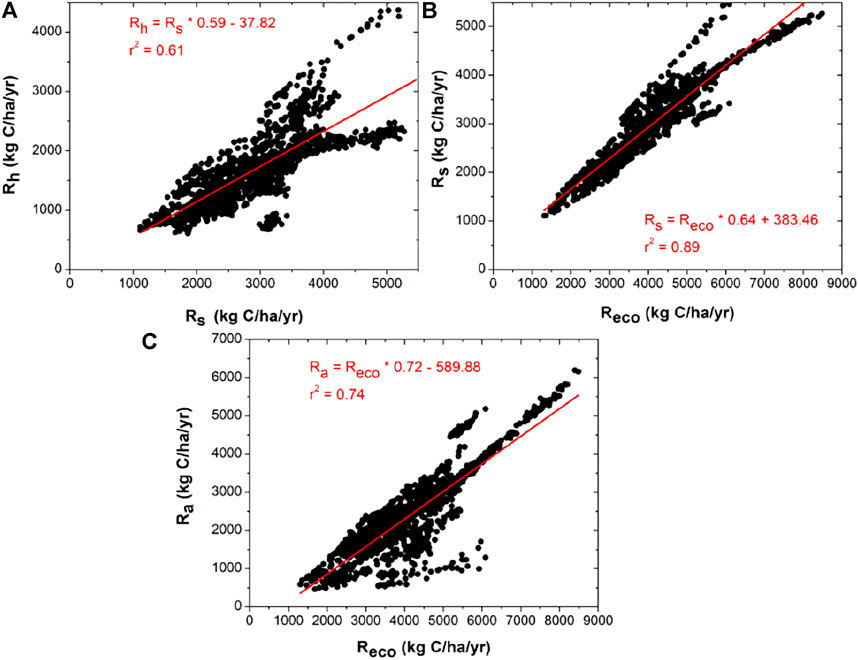
FIGURE 3. Relationships between (A) annual mean soil heterotrophic respiration (Rh) and total soil respiration (Rs); (B) Rs and ecosystem respiration (Reco); (C) autotrophic respiration (Ra) and Reco, respectively.
Global anthropogenic CO2 emissions (combination of fossil fuel and land use change) has been increased from 4.5 to 11 Gt C yr−1 from 1960–1969 to 2009–2018 (Friedlingstein et al., 2019). Global terrestrial ecosystem carbon sink has increased from 1.3 to 3.2 Gt C yr−1 from 1960–1969 to 2009–2018 and subsequently slowing down the atmospheric CO2 concentration increase (Friedlingstein et al., 2019). Terrestrial ecosystems are acting as a carbon sink for approximately 29% annual anthropogenic CO2 emissions during the last decade (2009–2018) and the magnitude is higher than the ocean sink rates (∼23%) (Friedlingstein et al., 2019). Although the direct link is unclear, it has been reported that atmospheric CO2 concentration is also sensitive to terrestrial water storage change at global-scale with declining values associated with rapid increase of CO2 concentration (Humphrey et al., 2018).
Modelled Estimates Comparison With Remote Sensing Data
Satellite-based estimates of Ra (Figure 4A) show similar spatial patterns on comparing with the modeled Ra (Figure 1). Satellite-based Ra varies from 800 kg C ha−1 yr−1 to as high as 3,943 kg C ha−1 yr−1 across the sub-basins of ARB. In general, modeled Ra is aligned with the satellite-based Ra in the mid-Ra region (1,500–4,000 kg C ha−1 yr−1; Figure 4B). The dissimilarity, in lower and higher Ra ranges are result of various well known issues with the satellite-based approach. Several studies have reported erroneous satellite-based NPP estimates (∼15% less estimates comparing the observations). These were found to be associated with the interference from the autotrophic respiration estimation from the neighboring areas (Ito, 2011). This make the satellite-based Ra value smaller than its real value. The GPP and NPP database developed using different available meteorological datasets are also showing an overestimation of the indices when comparing with the GPP (∼30% higher GPP reported using NCEP data) and NPP (15–20% higher NPP using NCEP data) developed using observed meteorological data (Zhao et al., 2006). The NCEP/DOE reanalysis II meteorological data were used to develop the global-scale GPP and NPP products (Zhao and Running, 2010)—this can also be a further reason for the overestimation. Turner et al. (2006) reported overestimation of MODIS NPP and GPP products at regions with comparatively lower productivity e.g. Boreal forest regions.
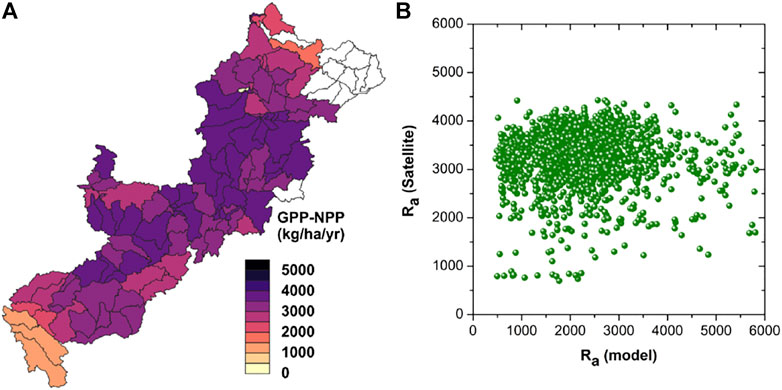
FIGURE 4. (A) Mean annual total autotrophic respiration (root and above ground biomass) from satellite-based estimates [Ra (Satellite)] in 2000–2013. (B) Relationship between autotrophic respirations from satellite and modeled estimates in kg C ha−1 yr−1 at ARB during 2000–2013.
Conclusion
Although the terrestrial ecosystem respiration is one of main components of climate change feedbacks, the sign and the magnitude of this feedback is highly uncertain in future. Our approach using a new integrated hydro-biogeochemical model show reasonable estimates of regional-scale Reco and its subsequent partitioning into Ra and Rh on comparing with the available data at the boreal forest covered Athabasca river basin, Canada. Annual mean Reco ranging between 1,600 and 8,200 kg C ha−1 yr−1 in 2000–2013. The Rs was dominated and contributed 60–90% toward Reco. The model estimates are in line with the site-scale measurements reported at similar land use and climatic regions. Satellite-based estimates of Ra also show similar patterns as of the modeled estimates.
Reasonable performance of our model for simulating regional-scale Reco and its components show that the bottom-up approach performs good for estimating the respiration components. Anthropogenic CO2 emission is a magnitude lower than the global ecosystem respiration. The accurate quantification of respiration components warrants better understanding of their feedbacks associated with the anthropogenic warming. Separate estimation of the respiration components is getting more attention here due to their differential responses to warming. It would be interesting to integrate the microbial enzymatic kinetics-based approach with widely used ESMs as a core module of soil greenhouse gas emissions.
Data Availability Statement
This paper used open-source data from FLUXNET (https://fluxnet.org, last accessed on 1 August, 2020) and SRDB network (https://daac.ornl.gov/SOILS/guides/SRDB_V5.html, last accessed on 1 August, 2020). Satellite-based GPP and NPP MOD17 data were retrieved from MODIS land team (https://modis.gsfc.nasa.gov/data/dataprod/mod17.php, last accessed on 1 August, 2020). The raw data supporting the conclusion of this article will be made available by the authors, without undue reservation.
Author Contributions
SB acquired the data, developed the model code and completed the analyses with inputs from JW and SB wrote the manuscript with inputs from JW and RB.
Conflict of Interest
The authors declare that the research was conducted in the absence of any commercial or financial relationships that could be construed as a potential conflict of interest.
Publisher’s Note
All claims expressed in this article are solely those of the authors and do not necessarily represent those of their affiliated organizations, or those of the publisher, the editors and the reviewers. Any product that may be evaluated in this article, or claim that may be made by its manufacturer, is not guaranteed or endorsed by the publisher.
Acknowledgments
This is a short text to acknowledge the contributions of specific colleagues, institutions, or agencies that aided the efforts of the authors. SB. acknowledges support from the Indian Institute of Science in the form of C. V. Raman Postdoctoral Fellowship for partly carrying out the study. JW. would like to thank the Alberta Economic Development and Trade for the Campus Alberta Innovates Program Research Chair (No. RCP-12-001-BCAIP). The authors acknowledge FLUXNET and SRDB network for making their data available to public. We appreciate the MODIS land team for the MOD17 GPP and NPP data.
Supplementary Material
The Supplementary Material for this article can be found online at: https://www.frontiersin.org/articles/10.3389/fenvs.2022.898199/full#supplementary-material
References
Allison, S. D., Wallenstein, M. D., and Bradford, M. A. (2010). Soil-carbon Response to Warming Dependent on Microbial Physiology. Nat. Geosci. 3, 336–340. doi:10.1038/ngeo846
Andrews, J. A., and Schlesinger, W. H. (2001). Soil CO2 Dynamics, Acidification, and Chemical Weathering in a Temperate Forest with Experimental CO2 Enrichment. Glob. Biogeochem. Cycles 15, 149–162. doi:10.1029/2000gb001278
Anthony, K. W., Schneider von Deimling, T., Nitze, I., Frolking, S., Emond, A., Daanen, R., et al. (2018). 21st-century Modeled Permafrost Carbon Emissions Accelerated by Abrupt Thaw beneath Lakes. Nat. Commun. 9, 3262. doi:10.1038/s41467-018-05738-9
Arnold, J. G., Srinivasan, R., Muttiah, R. S., and Williams, J. R. (1998). Large Area Hydrologic Modeling and Assessment Part I: Model Development. J. Am. Water Resour. Assoc. 34, 73–89. doi:10.1111/j.1752-1688.1998.tb05961.x
Bates, B., Kundzewicz, Z., and Wu, S. (2008). Climate Change and Water. Geneva: Intergovernmental Panel on Climate Change Secretariat.
Bhanja, S. N., and Wang, J. (2020). Estimating Influences of Environmental Drivers on Soil Heterotrophic Respiration in the Athabasca River Basin, Canada. Environ. Pollut. 257, 113630. doi:10.1016/j.envpol.2019.113630
Bhanja, S. N., Wang, J., Shrestha, N. K., and Zhang, X. (2019a). Microbial Kinetics and Thermodynamic (MKT) Processes for Soil Organic Matter Decomposition and Dynamic Oxidation-Reduction Potential: Model Descriptions and Applications to Soil N2O Emissions. Environ. Pollut. 247, 812–823. doi:10.1016/j.envpol.2019.01.062
Bhanja, S. N., Wang, J., Shrestha, N. K., and Zhang, X. (2019b). Modelling Microbial Kinetics and Thermodynamic Processes for Quantifying Soil CO2 Emission. Atmos. Environ. 209, 125–135. doi:10.1016/j.atmosenv.2019.04.014
Bhanja, S. N., Zhang, X., and Wang, J. (2018). Estimating Long-Term Groundwater Storage and its Controlling Factors in Alberta, Canada. Hydrol. Earth Syst. Sci. 22, 6241–6255. doi:10.5194/hess-22-6241-2018
Bond‐Lamberty, B., Wang, C., and Gower, S. T. (2004). A Global Relationship between the Heterotrophic and Autotrophic Components of Soil Respiration? Glob. Change Biol. 10, 1756–1766. doi:10.1111/j.1365-2486.2004.00816.x
Bond-Lamberty, B., Bailey, V. L., Chen, M., Gough, C. M., and Vargas, R. (2018). Globally Rising Soil Heterotrophic Respiration over Recent Decades. Nature 560, 80–83. doi:10.1038/s41586-018-0358-x
Bond-Lamberty, B., and Thomson, A. M. (2010). A Global Database of Soil Respiration Measurements. Biogeosciences 7, 1321–1344. doi:10.5194/bg-7-1915-2010
CFSR (2016). Global Weather Data for SWAT. Climate Forecast System Reanalysis. Available at: http://globalweather.tamu.edu/ (Accessed January 31, 2019).
Ciais, P., Sabine, C., Bala, G., Bopp, L., Brovkin, V., Canadell, J., et al. (2014). “Carbon and Other Biogeochemical Cycles,” in Climate Change 2013: The Physical Science Basis. Contribution of Working Group I to the Fifth Assessment Report of the Intergovernmental Panel on Climate Change (New York, NY, USA: Cambridge University Press), 465–570.
Ciais, P., Tan, J., Wang, X., Roedenbeck, C., Chevallier, F., Piao, S.-L., et al. (2019). Five Decades of Northern Land Carbon Uptake Revealed by the Interhemispheric CO2 Gradient. Nature 568, 221–225. doi:10.1038/s41586-019-1078-6
Clark, D. B., Mercado, L. M., Sitch, S., Jones, C. D., Gedney, N., Best, M. J., et al. (2011). The Joint UK Land Environment Simulator (JULES), Model Description - Part 2: Carbon Fluxes and Vegetation Dynamics. Geosci. Model Dev. 4, 701–722. doi:10.5194/gmd-4-701-2011
Cleveland, C. C., and Liptzin, D. (2007). C:N:P Stoichiometry in Soil: Is There a “Redfield Ratio” for the Microbial Biomass? Biogeochemistry 85 (3), 235–252. doi:10.1007/s10533-007-9132-0
Collins, M., Knutti, R., Arblaster, J., Dufresne, J.-L., Fichefet, T., Friedlingstein, P., et al. (2013). “Long-term Climate Change: Projections, Commitments and Irreversibility,” in Climate Change 2013: The Physical Science Basis. Contribution of Working Group I to the Fifth Assessment Report of the Intergovernmental Panel on Climate Change. Editors T. F. Stocker, D. Qin, G.-K. Plattner, M. Tignor, S. K. Allen, J. Boschunget al. (Cambridge, United Kingdom and New York, NY, USA: Cambridge University Press).
Crowther, T. W., Todd-Brown, K. E., Rowe, C. W., Wieder, W. R., Carey, J. C., Machmuller, M. B., et al. (2016). Quantifying Global Soil Carbon Losses in Response to Warming. Nature 540, 104–108. doi:10.1038/nature20150
Crowther, T. W., Van den Hoogen, J., Wan, J., Mayes, M. A., Keiser, A. D., Mo, L., et al. (2019). The Global Soil Community and its Influence on Biogeochemistry. Science 365 (6455), eaav0550. doi:10.1126/science.aav0550
Crowther, T. W., Maynard, D. S., Crowther, T. R., Peccia, J., Smith, J. R., and Bradford, M. A. (2014). Untangling the Fungal Niche: the Trait-Based Approach. Front. Microbiol. 5, 579. doi:10.3389/fmicb.2014.00579
Cumming, S. G. (2001). Forest Type and Wildfire in the Alberta Boreal Mixedwood: what Do Fires Burn? Ecol. Appl. 11, 97–110. doi:10.1890/1051-0761(2001)011[0097:ftawit]2.0.co;2
Davidson, E. A., and Janssens, I. A. (2006). Temperature Sensitivity of Soil Carbon Decomposition and Feedbacks to Climate Change. Nature 440, 165–173.
Davidson, E. A., Samanta, S., Caramori, S. S., and Savage, K. (2012). The Dual Arrhenius and Michaelis-Menten Kinetics Model for Decomposition of Soil Organic Matter at Hourly to Seasonal Time Scales. Glob. Change Biol. 18, 371–384. doi:10.1111/j.1365-2486.2011.02546.x
DeAngelis, K. M., Silver, W. L., Thompson, A. W., and Firestone, M. K. (2010). Microbial Communities Acclimate to Recurring Changes in Soil Redox Potential Status. Environ. Microbiol. 12, 3137–3149. doi:10.1111/j.1462-2920.2010.02286.x
Del Grosso, S. J., Parton, W. J., Mosier, A. R., Holland, E. A., Pendall, E., Schimel, D. S., et al. (2005). Modeling Soil CO2 Emissions from Ecosystems. Biogeochemistry 73 (1), 71–91. doi:10.1007/s10533-004-0898-z
Friedlingstein, P., Jones, M. W., O’Sullivan, M., Andrew, R. M., Hauck, J., Peters, G. P., et al. (2019). Global Carbon Budget 2019. Earth Syst. Sci. Data 11, 1783–1838. doi:10.5194/essd-11-1783-2019
Fujita, Y., Witte, J.-P. M., and van Bodegom, P. M. (2014). Incorporating Microbial Ecology Concepts into Global Soil Mineralization Models to Improve Predictions of Carbon and Nitrogen Fluxes. Glob. Biogeochem. Cycles 28, 223–238. doi:10.1002/2013gb004595
GoC (2016). Environment and Natural Resources; Weather, Climate and Hazard. Available at: http://climate.weather.gc.ca/ (Accessed January 31, 2019).
Goulden, M. L., Mcmillan, A. M. S., Winston, G. C., Rocha, A. V., Manies, K. L., Harden, J. W., et al. (2011). Patterns of NPP, GPP, Respiration, and NEP during Boreal Forest Succession. Glob. Change Biol. 17, 855–871. doi:10.1111/j.1365-2486.2010.02274.x
Hardie, S. M. L., Garnett, M. H., Fallick, A. E., Ostle, N. J., and Rowland, A. P. (2009). Bomb-14C Analysis of Ecosystem Respiration Reveals that Peatland Vegetation Facilitates Release of Old Carbon. Geoderma 153, 393–401. doi:10.1016/j.geoderma.2009.09.002
Hicks Pries, C. E., Schuur, E. A. G., and Crummer, K. G. (2013). Thawing Permafrost Increases Old Soil and Autotrophic Respiration in Tundra: Partitioning Ecosystem Respiration Using δ13C and ∆14C. Glob. Change Biol. 19, 649–661. doi:10.1111/gcb.12058
Hicks Pries, C. E., Schuur, E. A. G., Natali, S. M., and Crummer, K. G. (2016). Old Soil Carbon Losses Increase with Ecosystem Respiration in Experimentally Thawed Tundra. Nat. Clim. Change 6, 214–218. doi:10.1038/nclimate2830
Humphrey, V., Zscheischler, J., Ciais, P., Gudmundsson, L., Sitch, S., and Seneviratne, S. I. (2018). Sensitivity of Atmospheric CO2 Growth Rate to Observed Changes in Terrestrial Water Storage. Nature 560, 628–631. doi:10.1038/s41586-018-0424-4
Ito, A. (2011). A Historical Meta-Analysis of Global Terrestrial Net Primary Productivity: Are Estimates Converging? Glob. Change Biol. 17, 3161–3175. doi:10.1111/j.1365-2486.2011.02450.x
Jarvis, A., Reuter, H. I., Nelson, A., and Guevara, E. (2008). Hole-filled SRTM for the Globe Version 4. Montpellier, France: CGIAR. Available from the CGIAR-CSI SRTM90m Database http://srtm.csi.cgiar.org.
Kaiser, C., Franklin, O., Richter, A., and Dieckmann, U. (2015). Social Dynamics within Decomposer Communities Lead to Nitrogen Retention and Organic Matter Build-Up in Soils. Nat. Commun. 6, 8960. doi:10.1038/ncomms9960
Karhu, K., Auffret, M. D., Dungait, J. A. J., Hopkins, D. W., Prosser, J. I., Singh, B. K., et al. (2014). Temperature Sensitivity of Soil Respiration Rates Enhanced by Microbial Community Response. Nature 513, 81–84. doi:10.1038/nature13604
Konings, A. G., Bloom, A. A., Liu, J., Parazoo, N. C., Schimel, D. S., and Bowman, K. W. (2019). Global Satellite-Driven Estimates of Heterotrophic Respiration. Biogeosciences 16 (11), 2269–2284. doi:10.5194/bg-16-2269-2019
Lawrence, D., Fisher, R., Koven, C., Oleson, K., Swenson, S., and Bonan, G. (2019). The Community Land Model Version 5: Description of New Features, Benchmarking, and Impact of Forcing Uncertainty. J. Adv. Model. Earth Syst.. doi:10.1029/2018MS001583
Li, C., Frolking, S., and Harriss, R. (1994). Modeling Carbon Biogeochemistry in Agricultural Soils. Glob. Biogeochem. Cycles 8, 237–254. doi:10.1029/94gb00767
Loveland, T. R., Reed, B. C., Brown, J. F., Ohlen, D. O., Zhu, Z., Yang, L. W. M. J., et al. (2000). Development of a Global Land Cover Characteristics Database and IGBP DISCover from 1 Km AVHRR Data. Int. J. Remote Sens. 21 (6-7), 1303–1330. doi:10.1080/014311600210191
Luo, Y., Ahlström, A., Allison, S. D., Batjes, N. H., Brovkin, V., Carvalhais, N., et al. (2016). Toward More Realistic Projections of Soil Carbon Dynamics by Earth System Models. Glob. Biogeochem. Cycles 30, 40–56. doi:10.1002/2015gb005239
Ma, S., Baldocchi, D. D., Xu, L., and Hehn, T. (2007). Inter-annual Variability in Carbon Dioxide Exchange of an Oak/grass Savanna and Open Grassland in California. Agric. For. Meteorology 147, 157–171. doi:10.1016/j.agrformet.2007.07.008
Manzoni, S., Jackson, R. B., Trofymow, J. A., and Porporato, A. (2008). The Global Stoichiometry of Litter Nitrogen Mineralization. Science 321, 684–686. doi:10.1126/science.1159792
Melillo, J. M., Frey, S. D., DeAngelis, K. M., Werner, W. J., Bernard, M. J., Bowles, F. P., et al. (2017). Long-term Pattern and Magnitude of Soil Carbon Feedback to the Climate System in a Warming World. Science 358, 101–105. doi:10.1126/science.aan2874
Mitchard, E. T. A. (2018). The Tropical Forest Carbon Cycle and Climate Change. Nature 559, 527–534. doi:10.1038/s41586-018-0300-2
Natali, S. M., Schuur, E. A. G., and Rubin, R. L. (2012). Increased Plant Productivity in Alaskan Tundra as a Result of Experimental Warming of Soil and Permafrost. J. Ecol. 100, 488–498. doi:10.1111/j.1365-2745.2011.01925.x
Nowinski, N. S., Taneva, L., Trumbore, S. E., and Welker, J. M. (2010). Decomposition of Old Organic Matter as a Result of Deeper Active Layers in a Snow Depth Manipulation Experiment. Oecologia 163, 785–792. doi:10.1007/s00442-009-1556-x
Oleson, K. W., Lawrence, D. M., Bonan, G. B., Flanner, M. G., Kluzek, E., Lawrence, P. J., et al. (2010). Technical Description of Version 4.0 of the Community Land Model (CLM). Boulder, CO: NCAR Tech. Note NCAR/TN-478+STR. doi:10.5065/D6FB50WZ
Parton, W. J., Ojima, D. S., Cole, C. V., and Schimel, D. S. (1994). A General Model for Soil Organic Matter Dynamics: Sensitivity to Litter Chemistry, Texture and Management. Quantitative Model. soil Form. Process. 39, 147–167.
Parton, W. J., Schimel, D. S., Cole, C. V., and Ojima, D. S. (1987). Analysis of Factors Controlling Soil Organic Matter Levels in Great Plains Grasslands. Soil Sci. Soc. Am. J. 51, 1173–1179. doi:10.2136/sssaj1987.03615995005100050015x
Pastorello, G., Papale, D., Chu, H., Trotta, C., Agarwal, D. A., Canfora, E., et al. (2017). A New Data Set to Keep a Sharper Eye on Land-Air Exchanges. Eos, Trans. Am. Geophys. Union 98. doi:10.1029/2017eo071597
Qiu, C., Zhu, D., Ciais, P., Guenet, B., Krinner, G., Peng, S., et al. (2018). ORCHIDEE-PEAT (Revision 4596), a Model for Northern Peatland CO2, Water, and Energy Fluxes on Daily to Annual Scales. Geosci. Model Dev. 11, 497–519. doi:10.5194/gmd-11-497-2018
Richardson, A. D., Hollinger, D. Y., Aber, J. D., Ollinger, S.V., and Braswell, B. H. (2007). Environmental Variation is Directly Responsible for Short- but not Long-Term Variation in Forest-Atmosphere Carbon Exchange. Global Change Biol. 13, 788–803. doi:10.1111/j.1365-2486.2007.01330.x
Ryan, M. G., Linder, S., Vose, J. M., and Hubbard, R. M. (1994). Dark Respiration of Pines. Ecol. Bull. 43, 50–63.
Ryan, M. G., Lavigne, M. B., and Gower, S. T. (1997). Annual Carbon Cost of Autotrophic Respiration in Boreal Forest Ecosystems in Relation to Species and Climate. J. Geophys. Res. 102, 28871–28883. doi:10.1029/97jd01236
Schuur, E. A. G., McGuire, A. D., Schädel, C., Grosse, G., Harden, J. W., Hayes, D. J., et al. (2015). Climate Change and the Permafrost Carbon Feedback. Nature 520, 171–179. doi:10.1038/nature14338
Schuur, E. A. G., Vogel, J. G., Crummer, K. G., Lee, H., Sickman, J. O., and Osterkamp, T. E. (2009). The Effect of Permafrost Thaw on Old Carbon Release and Net Carbon Exchange from Tundra. Nature 459, 556–559. doi:10.1038/nature08031
Senapati, N., Chabbi, A., and Smith, P. (2018). Modelling Daily to Seasonal Carbon Fluxes and Annual Net Ecosystem Carbon Balance of Cereal Grain-Cropland Using DailyDayCent: A Model Data Comparison. Agric. Ecosyst. Environ. 252, 159–177. doi:10.1016/j.agee.2017.10.003
Serna‐Chavez, H. M., Fierer, N., and Van Bodegom, P. M. (2013). Global Drivers and Patterns of Microbial Abundance in Soil. Glob. Ecol. Biogeogr. 22, 1162–1172. doi:10.1111/geb.12070
Shrestha, N. K., Du, X., and Wang, J. (2017). Assessing Climate Change Impacts on Fresh Water Resources of the Athabasca River Basin, Canada. Sci. Total Environ. 601-602, 425–440. doi:10.1016/j.scitotenv.2017.05.013
SLC (2010). Soil Landscapes of Canada Version 3.2. Agriculture and Agri-Food Canada. Available at: http://sis.agr.gc.ca/cansis/nsdb/slc/v3.2/index.html (Accessed January 31, 2019).
Stumm, W., and Morgan, J. J. (1996). Aquatic Chemistry: Chemical Equilibria and Rates in Natural Waters. Wiley.
Todd-Brown, K. E. O., Randerson, J. T., Post, W. M., Hoffman, F. M., Tarnocai, C., Schuur, E. A. G., et al. (2013). Causes of Variation in Soil Carbon Simulations from CMIP5 Earth System Models and Comparison with Observations. Biogeosciences 10, 1717–1736. doi:10.5194/bg-10-1717-2013
Turner, D. P., Ritts, W. D., Cohen, W. B., Gower, S. T., Running, S. W., Zhao, M., et al. (2006). Evaluation of MODIS NPP and GPP Products across Multiple Biomes. Remote Sens. Environ. 102, 282–292. doi:10.1016/j.rse.2006.02.017
Veen, B. W. (1981). “Relation between Root Respiration and Root Activity,” in Structure and Function of Plant Roots (Dordrecht: Springer), 277–280. doi:10.1007/978-94-009-8314-4_53
Walker, T. W. N., Kaiser, C., Strasser, F., Herbold, C. W., Leblans, N. I. W., Woebken, D., et al. (2018). Microbial Temperature Sensitivity and Biomass Change Explain Soil Carbon Loss with Warming. Nat. Clim. Change 8, 885–889. doi:10.1038/s41558-018-0259-x
Wang, J., Li, Y., Bork, E. W., Richter, G. M., Eum, H.-I., Chen, C., et al. (2020). Modelling Spatio-Temporal Patterns of Soil Carbon and Greenhouse Gas Emissions in Grazing Lands: Current Status and Prospects. Sci. Total Environ. 739, 139092. doi:10.1016/j.scitotenv.2020.139092
Wang, J., Shrestha, N. K., Delavar, M. A., Meshesha, T. W., and Bhanja, S. N. (2021). Modelling Watershed and River Basin Processes in Cold Climate Regions: A Review. Water 13 (4), 518. doi:10.3390/w13040518
Wang, X., Liu, L., Piao, S., Janssens, I. A., Tang, J., Liu, W., et al. (2014). Soil Respiration under Climate Warming: Differential Response of Heterotrophic and Autotrophic Respiration. Glob. Change Biol. 20, 3229–3237. doi:10.1111/gcb.12620
Weber, M., Hauer, G., and Farr, D. (2015). Economic-ecological Evaluation of Temporary Biodiversity Offsets in Alberta's Boreal Forest. Envir. Conserv. 42, 315–324. doi:10.1017/s0376892915000181
Wieder, W. R., Bonan, G. B., and Allison, S. D. (2013). Global Soil Carbon Projections Are Improved by Modelling Microbial Processes. Nat. Clim. Change 3, 909–912. doi:10.1038/nclimate1951
Wieder, W. R., Cleveland, C. C., Smith, W. K., and Todd-Brown, K. (2015). Future Productivity and Carbon Storage Limited by Terrestrial Nutrient Availability. Nat. Geosci. 8, 441–444. doi:10.1038/ngeo2413
Zhao, M., Heinsch, F. A., Nemani, R. R., and Running, S. W. (2005). Improvements of the MODIS Terrestrial Gross and Net Primary Production Global Data Set. Remote Sens. Environ. 95, 164–176. doi:10.1016/j.rse.2004.12.011
Zhao, M., and Running, S. W. (2010). Drought-induced Reduction in Global Terrestrial Net Primary Production from 2000 through 2009. Science 329, 940–943. doi:10.1126/science.1192666
Keywords: ecosystem respiration, soil respiration, root respiration, litter decomposition, respiration modeling, SWAT (soil and water assessment tool)
Citation: Bhanja SN, Wang J and Bol R (2022) Soil CO2 Emission Largely Dominates the Total Ecosystem CO2 Emission at Canadian Boreal Forest. Front. Environ. Sci. 10:898199. doi: 10.3389/fenvs.2022.898199
Received: 17 March 2022; Accepted: 06 June 2022;
Published: 28 June 2022.
Edited by:
Jiang Helong, Nanjing Institute of Geography and Limnology (CAS), ChinaReviewed by:
Hyung Eum, Alberta Environment and Parks, CanadaMarwan Fahs, National School for Water and Environmental Engineering, France
Copyright © 2022 Bhanja, Wang and Bol. This is an open-access article distributed under the terms of the Creative Commons Attribution License (CC BY). The use, distribution or reproduction in other forums is permitted, provided the original author(s) and the copyright owner(s) are credited and that the original publication in this journal is cited, in accordance with accepted academic practice. No use, distribution or reproduction is permitted which does not comply with these terms.
*Correspondence: Soumendra N. Bhanja, soumendrabhanja@gmail.com; Roland Bol, r.bol@fz-juelich.de
†Present address: Soumendra N. Bhanja, Environmental Sciences Division, Oak Ridge National Laboratory, Oak Ridge, TN, United States