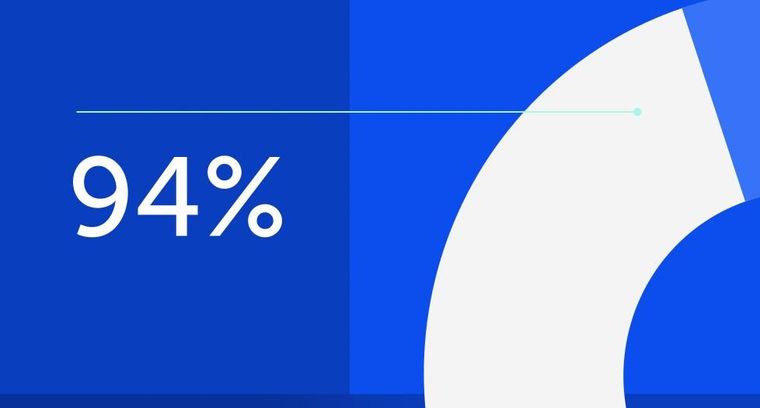
94% of researchers rate our articles as excellent or good
Learn more about the work of our research integrity team to safeguard the quality of each article we publish.
Find out more
ORIGINAL RESEARCH article
Front. Environ. Sci., 26 April 2022
Sec. Environmental Economics and Management
Volume 10 - 2022 | https://doi.org/10.3389/fenvs.2022.895648
This article is part of the Research TopicGreen Innovation and Industrial Ecosystem Reconstruction in Achieving Environmental SustainabilityView all 34 articles
China’s carbon emissions are a major global concern. China has proposed a defined “dual-carbon” aim, with the first target being to attain the carbon emissions peak by 2,030. To address this issue, this study provides a two-stage method for forecasting China’s annual carbon emissions, which is paired with pertinent carbon emissions data to predict China’s annual carbon emissions. We discovered the associated aspects affecting China’s carbon emissions through the research of this article, and we predicted the carbon emissions data from 2017 to 2020 using the two-stage technique based on these factors. When compared to the actual data of China’s annual emissions from 2017 to 2020, the prediction intervals from this method encompass the actual data well. This method, on the one hand, identifies the main affecting factors for estimating carbon emissions data, and on the other hand, it validates the method’s performance. It provides support for further policy development and change based on the outcome of this method.
In response to the scarcity of resources and the deterioration of the global environment, Chinese President Xi Jinping set a “dual-carbon” target in 2020, with carbon emissions peaked in 2030 and eventually neutralized in 2060, urging China to contribute to environmental conservation. In January 2022, he re-emphasized the importance of the carbon-reduction process. The key to reaching the “dual carbon” goal is the establishment of a low-carbon economy. Developing a low-carbon economy requires a shift in China’s development model, which now includes not only pursuing economic growth but also pursuing economic development while considering the environment. China’s development of a low-carbon economy will have a major impact on the world’s ecological and economic fields as the world’s second-biggest economy and largest carbon emitter. To achieve President Xi Jinping’s goal of a low-carbon and efficient Chinese economy as soon as possible, the Chinese government should formulate a reasonable development plan based on a combination of its own national conditions and relevant low-carbon economy conditions (Cheng et al., 2020; Chen et al., 2021; Du et al., 2021; Meng et al., 2021).
The primary concept is to define the process of its own carbon emissions and future changes in carbon emissions in order to better coordinate carbon reduction efforts according to local conditions and establish a low-carbon economy. Governments at all levels will be able to support a low-carbon economy only when they have clarified their own carbon emissions. The two-stage model is used in this study to fit and forecast China’s annual carbon emissions data successfully, meaning that the actual data is highly consistent with the fitted data, and the predicted value intervals completely cover the true value from 2018 to 2020. It is expected that the prediction will encourage the government to make more scientific decisions. It will also provide a powerful drive for achieving the “dual-carbon” target and developing a low-carbon economy.
This paper is organized as follows: Section Literature Review is the Literature Review. In the section, we sort out the previous research, clarifying the process and shortcomings of the previous research, and providing a reference for the research of this paper. Section Methodology is the methodology. In the section, we introduce the specific method used in this paper, and give the expression. Section Data is the data. In the section, we describe the types and reasons for data selection, the sources of data, and the data processing software used throughout the article. Section Empirical Results is the empirical results. This section contains graphs of empirical results and corresponding interpretations of model fit results. Section Conclusion and Policy Implications is the conclusions and policy implications. In this section, we summarize the research in this paper, and give the corresponding policy recommendations based on the empirical results.
In recent years, academics have focused on low-carbon economic research, hoping to discover the secret to sustaining economic growth while conserving the environment. We discovered that research on the low-carbon economy primarily begins with quantitative research after evaluating these literatures. The foundation of low-carbon economic research is quantitative study. Scholars mostly focus on mechanism analysis, influencing factor analysis, carbon emissions forecasting, and so on in quantitative research.
In the mechanism analysis, scholars have studied the carbon trading mechanism (Hu et al., 2019; Pan et al., 2020; Jin et al., 2020; Chen and Lin, 2021), discussing the quality of the carbon compensation mechanism (Gao et al., 2020; Lv and Bai, 2020), analyzing the government mechanism of the low-carbon economy (Marsden and Rye, 2010; Wright, 2013; Wu et al., 2016; Sun et al., 2019; Liu et al., 2021), and seeking efficient low-carbon economic governance methods (Zhao et al., 2011; Chen and Zhu, 2019; Keivani et al., 2020).
In the study of influencing factors, scholars mainly focus on the impact of various factors on carbon emissions. Some papers research the influencing factors of carbon emissions by country and region, such as China (Wang et al., 2016; Wang and Liu, 2017; Cao et al., 2019; Wen and Shao, 2019; Ma et al.,2019), America (Mallia et al., 2015), India (Li et al., 2018), Pakistan (Azam et al., 2021) and other regions (Sun et al., 2021); some scholars conduct research on the influencing factors of carbon emissions by industry, such as agriculture (Tian et al., 2014; Han et al., 2018; Tian et al., 2020), construction (Lu et al., 2016; Shi et al., 2017; Li et al., 2019), manufacturing (Chontanawat et al., 2020), transportation (Zhang and Wei, 2015), etc. These studies all contribute to the search for the secret to an efficient low-carbon economy.
In the exploration of carbon emissions forecasting, the main difference lies in the application of research methods. Different methods have different forecasting effects, such as ARIMA model (Ning et al., 2021; Yang et al., 2020), a New Information Priority Accumulated Grey Model with Simpson (Xiang et al., 2020), inclusive multiple model (Shabani et al., 2021), LSTM (Huang et al., 2018; Li, 2020). In this type of research, scholars strive to find a high-accuracy method that can predict future carbon emissions and closely match historical data.
By examining these literatures, we discovered that research on carbon emissions prediction is critical for the development of a low-carbon economy. However, the existing approaches for carbon emissions prediction are mainly point estimation, and the data fitting is not very close to the actual data. For this purpose, we collect data from two aspects, resource and environmental data and economic and social data, to use a two-stage model (Gamma regression and ARIMA model’s residual processing) to fit and estimate the annual carbon emissions data of China from 1999 to 2020. The result is an interval estimation rather than a point estimation. The prediction interval can cover the real data effectively by fully extracting data information, which is useful to the development of a low-carbon economy.
The two-stage method to analyze the annual carbon emissions of China includes the trend analysis and the residuals analysis. After getting these parameters of the models of the trend and residuals, we can use these two models to predict the trend and residual respectively.
Let Zt represent the annual carbon emissions at the tth year (t = 1, 2, ..., T = 20) from 1998 to 2017. These data are left-skewed continuous in Figure 1. Since a left-skewed continuous response has a constant upper bound 12,000 in this data set, we can then analyze the corresponding right-skewed response 12,000−Zt by using some well-known distributions. Let Yt = 12,000−Zt follow the gamma distribution. For simplicity of notation, we let Gamma (μ, σ2) stand for the gamma distribution, where µ and σ denote it’s mean and coefficient of variation, respectively. Thus, if a random variable Y follows the gamma distribution, that is Y ∼ Gamma (μ, σ2), then E (Y) = μ and Var(Y) = σ2μ2. In this paper, the Gamma regression models for Yt is given by
but with the mean trend μt defined as
where xt includes intercept term and six indicators are forest coverage (x1), total energy consumption (x2), energy consumption intensity (x3), GDP (x4), industrial structure (x5) and employment structure (x6), as given in Section Data, and β is a 6-dimensional vectors of regression parameters.
FIGURE 1. The histogram of carbon emission and six covariates distributions skewed to the left, and the last four being positive and skewed to the right. The kurtosis for these data is −1.605, −1.130, −1.487, −1.705, −1.255, −0.712, and −1.285, all negative, meaning the distributions are flatter than the normal distribution. The data histograms in Figure 1 and the model presented in this study do not appear to contradict each other, indicating that the model is plausible.
After the trend analysis of the annual carbon emissions of China, we could get the raw residuals Rt of the model 1, which is given by
Then, we use the Autoregressive Integrated Moving Average (ARIMA for short) model to fit the
We can get trend forecast values based on the trend analysis model by applying the six indicators, and the ARIMA model can produce forecasts with 95% prediction limitations at the same time. The predicted values of trend and residuals are then summed to generate the yearly carbon emissions data forecasted values (Zhang and Ma, 2021).
To investigate the low-carbon economy, we sought for data from two perspectives (Wu, 2021). On the one hand, it is concerned with resources and the environment, and we seek information on carbon emissions, forest coverage, total energy consumption, and energy consumption intensity in this regard. Carbon emissions reduction is a major goal in the transition to a low-carbon economy. Total energy consumption is a resource consumption measure that is used to reflect energy usage. The ratio of forest area to total land area, which is used to illustrate the availability of environmental resources, is known as forest coverage. Energy consumption intensity is defined as the ratio of total energy consumption to GDP, which represents the intensity of energy consumption. On the other hand, we were looking for information on the economy and society’s GDP, industrial structure, and employment structure. The gross domestic product, or GDP, is an inescapable metric for analyzing economic concerns. Because the tertiary industry is such an important supporting industry when the government is focusing on developing a low-carbon economy, the industrial structure is expressed by the contribution rate of the tertiary industry, and the employment structure is expressed by the proportion of employment in the tertiary industry. The preceding data comes from the China Statistical Yearbook and CEADs, and it spans the years 1998–2020. At the same time, this study processes the data with R software. Table 1 shows the descriptive statistics of the data, whereas Figure 1 shows the histogram of the variables.
From the descriptive statistics presented in Table 1, it can be known that the means of the variables are 7198.272, 19.400, 325049.600, 1.006, 435509.300, 47.113, and 35.526, respectively. The standard deviations are 2850.107, 2.561, 123607.700, 0.394, 312836.800, 8.386, and 6.918, respectively. The skewness is −0.299, −0.244, −0.235, 0.084, 0.473, 0.478, and 0.402, with the first three being negative and the.
First, we performed Gamma regression fitting on the indicators’ data from 1998 to 2016, and generated parameter estimates within the 95% confidence interval using R software. Table 2 shows the results of the parameters.
With the parameter estimated by model (1), the covariates from 1998 to 2016 are used to fit the annual carbon emissions of China from 1999 to 2017, we can obtain the fitted values, and by comparing the fitted values with the corresponding real data, Figure 2 can be drawn. From Figure 2, we can see that by collecting two aspects of data, economic and social data and resource and environmental data, as indicators, and then performing Gamma regression on the data, our model fully considers the factors affecting carbon emissions in a low-carbon economy, so that the fitted data with the real data are highly consistent, and the fitting effect is good.
The variables from 1998 to 2016 are used to fit the annual carbon emissions of China from 1999 to 2017 using the parameter obtained by the model (1), and by comparing the fitted values with the corresponding real data, Figure 2 can be created. As shown in Figure 2, our model fully considers the factors affecting carbon emissions in a low-carbon economy by collecting two types of data as indicators, economic and social data, and resource and environmental data, and then performing Gamma regression on the data. As a result, the fitted data and the real data are highly consistent, and the fitting effect is good.
We evaluated the model residuals after the trend analysis with the Gamma model and found that they were correlated, as shown in Figure 3. The autocorrelation and partial autocorrelation graphs in Figure 3 show that using the ARIMA model to handle the data is more reasonable. Finally, to fit the residuals, we chose ARIMA (4, 1, 0). We used the Ljung-Box test on the residuals of ARIMA (4, 1, 0) to check the ARIMA model’s effect, and plotted autocorrelation and partial autocorrelation graphs, as shown in Figure 4. The p-value of the Ljung-Box test is greater than 0.05, which is 0.354, that is, the residual sequence does not violate the assumption of white noise, and the results shown in Figure 4 also meet the application requirements of the ARIMA model.
FIGURE 4. Processed autocorrelation and partial autocorrelation plots of the residuals of the ARIMA (4, 1, 0).
The performance of the fitted data is not, however, the primary topic of this essay. If the predicted data is unsatisfactory, the article’s meaning will be substantially diminished. As a result, we did the following step in order to increase forecast accuracy and test it quickly: It is impossible to test whether impending carbon emissions are accurate in a short period of time because future data has not yet been obtained.
Considering the time lag in the impact of indicators on carbon emissions, we separated the data and utilized the indicators’ data from 1998 to 2016 to match the carbon emissions data from 1999 to 2017. As shown in Section Trend Analysis of the Annual Carbon Emissions From 1998 to 2017, the fitted values are similar to the real values. The covariates from 2017 to 2019 are then used to forecast carbon emissions data from 2018 to 2020, and the predicted value is compared to the actual value. This divide is obviously advantageous for swiftly testing the accuracy of the prediction algorithm in this research.
Because the information provided by point prediction is restricted, we cannot see the particular difference between the predicted and actual value. As a result, instead of just proposing point estimation findings to improve prediction accuracy, this work reprocesses the ARIMA prediction results to obtain an appropriate estimation interval. We give upper and lower bounds in this estimation range, and if the real data is substantially covered by the estimation interval, our prediction impact will be thoroughly confirmed.
During the trend prediction phase, we employ the six covariates to calculate the values of China’s carbon emissions from 2018 to 2020, after which the ARIMA model for residuals may provide the prediction value as well as the upper and lower intervals. These two components are combined from the trend prediction and residuals parts. Finally, the forecast obtained by combining these two parts can provide the prediction as well as the prediction intervals for the years 2018–2020.
Through the above empirical steps, we finally got the most representative graph in this paper, Figure 5, which contains two parts of fitting and prediction, corresponding to the Gamma regression and ARIMA model treating process in the two-stage model respectively.
Figure 5 shows that our fitted results are in good agreement with the real data during the fitting stage, indicating that we have fully considered when selecting indicators and have divided the relevant data of the low-carbon economy into two parts, resources and environment, economic and social, in a scientific and reasonable manner. Simultaneously, we can see that in the prediction stage, the median line of our interval estimate (that is, the yellow line in the figure) is consistent with the real data, and the upper and lower bounds perfectly cover the real data, fully respecting the fact that real data fluctuations are caused by random events. As a result, the two-stage model utilized in this research has proven to be effective in fitting and forecasting carbon emissions in a low-carbon economy.
The findings in this report will help governments at all levels control pollution more scientifically and correctly, reduce carbon emissions, and establish a low-carbon economy more efficiently. At the same time, in relevant prediction research, the two-stage method has a great promotion value. Predictive research in various domains can use the ideas included in the two-stage approach as a reference.
We used a two-stage model to analyze and forecast China’s annual carbon emissions data in this article. Furthermore, we projected China’s carbon emissions from 2018 to 2020 based on the relation between the six covariates and carbon emissions. The findings suggest that this method can more accurately fit and forecast China’s annual carbon emissions data. This makes it easier for decision-makers to set more specific targets in the process of reaching the 2030 carbon peak. At the same time, the model’s forecasted value continues to rise, indicating that China faces a complex challenge in meeting the carbon peak target in the current mode. As a result, it is critical to encourage the development of a low-carbon economy. Finally, the key factors to pay attention to in China’s carbon emissions management process are offered based on the research of the relationship between the six covariates and carbon emissions.
Publicly available datasets were analyzed in this study. This data can be found here: http://www.stats.gov.cn, https://www.ceads.net.cn.
The first author XZ is responsible for model construction and data analysis. The second author JX is responsible for data collection and paper writing. The corresponding author JS is responsible for the framework design.
This work was supported by the Yunnan Philosophy and Social Science Planning Project Fund (No. HX2019082760), Yunnan Provincial Postgraduate Quality Course Project: Yunnan Normal University Postgraduate Core Curriculum Construction Project (No.YH2020-C08), and Doctoral Research Initiation Project Fund (No. 2019BSXM11).
The authors declare that the research was conducted in the absence of any commercial or financial relationships that could be construed as a potential conflict of interest.
All claims expressed in this article are solely those of the authors and do not necessarily represent those of their affiliated organizations, or those of the publisher, the editors and the reviewers. Any product that may be evaluated in this article, or claim that may be made by its manufacturer, is not guaranteed or endorsed by the publisher.
Azam, M., Nawaz, S., Rafiq, Z., and Iqbal, N. (2021). A Spatial-Temporal Decomposition of Carbon Emission Intensity: a Sectoral Level Analysis in Pakistan. Environ. Sci. Pollut. Res. 28 (17), 21381–21395. doi:10.1007/s11356-020-12088-x
Cao, Q., Kang, W., Xu, S., Sajid, M. J., and Cao, M. (2019). Estimation and Decomposition Analysis of Carbon Emissions from the Entire Production Cycle for Chinese Household Consumption. J. Environ. Manage. 247, 525–537. doi:10.1016/j.jenvman.2019.06.044
Chen, X., and Lin, B. (2021). Towards Carbon Neutrality by Implementing Carbon Emissions Trading Scheme: Policy Evaluation in China. Energy Policy 157, 112510. doi:10.1016/j.enpol.2021.112510
Chen, Y., Chen, M., and Li, T. (2021). China's CO2 Emissions Reduction Potential: A Novel Inverse DEA Model with Frontier Changes and Comparable Value. Energ. Strategy Rev. 38, 100762. doi:10.1016/j.esr.2021.100762
Chen, Y., and Zhu, J. (2019). A Graph Theory-Based Method for Regional Integrated Energy Network Planning: A Case Study of a China-U.S. Low-Carbon Demonstration City. Energies 12 (23), 4491. doi:10.3390/en12234491
Cheng, S., Fan, W., Chen, J., Meng, F., Liu, G., Song, M., et al. (2020). The Impact of Fiscal Decentralization on CO2 Emissions in China. Energy 192, 116685. doi:10.1016/j.energy.2019.116685
Chontanawat, J., Wiboonchutikula, P., and Buddhivanich, A. (2020). Decomposition Analysis of the Carbon Emissions of the Manufacturing and Industrial Sector in Thailand. Energies 13 (4), 798. doi:10.3390/en13040798
Du, G., Yu, M., Sun, C., and Han, Z. (2021). Green Innovation Effect of Emission Trading Policy on Pilot Areas and Neighboring Areas: An Analysis Based on the Spatial Econometric Model. Energy Policy 156, 112431. doi:10.1016/j.enpol.2021.112431
Gao, Y., Li, M., Xue, J., and Liu, Y. (2020). Evaluation of Effectiveness of China's Carbon Emissions Trading Scheme in Carbon Mitigation. Energ. Econ. 90, 104872. doi:10.1016/j.eneco.2020.104872
Han, H., Zhong, Z., Guo, Y., Xi, F., and Liu, S. (2018). Coupling and Decoupling Effects of Agricultural Carbon Emissions in China and Their Driving Factors. Environ. Sci. Pollut. Res. 25 (9), 25280–25293. doi:10.1007/s11356-018-2589-7
Hu, Y., Ren, S., Wang, Y., and Chen, X. (2020). Can Carbon Emission Trading Scheme Achieve Energy Conservation and Emission Reduction? Evidence from the Industrial Sector in China. Energ. Econ. 85, 104590. doi:10.1016/j.eneco.2019.104590
Huang, Y., Shen, L., and Liu, H. (2019). Grey Relational Analysis, Principal Component Analysis and Forecasting of Carbon Emissions Based on Long Short-Term Memory in China. J. Clean. Prod. 209, 415–423. doi:10.1016/j.jclepro.2018.10.128
Jin, Y., Liu, X., Chen, X., and Dai, H. (2020). Allowance Allocation Matters in China's Carbon Emissions Trading System. Energ. Econ. 92, 105012. doi:10.1016/j.eneco.2020.105012
Keivani, E., Abbaspour, M., Abedi, Z., and Ahmadian, M. (2020). Promotion of Low-Carbon Economy through Efficiency Analysis: A Case Study of a Petrochemical Plant. Int. J. Environ. Res. 15 (15), 45–55. doi:10.1007/s41742-020-00282-1
Li, B., Han, S., Wang, Y., Wang, Y., Li, J., and Wang, Y. (2020). Feasibility Assessment of the Carbon Emissions Peak in China's Construction Industry: Factor Decomposition and Peak Forecast. Sci. Total Environ. 706, 135716. doi:10.1016/j.scitotenv.2019.135716
Li, Y. (2020). Forecasting Chinese Carbon Emissions Based on a Novel Time Series Prediction Method. Energy Sci Eng 8, 2274–2285. ese3. doi:10.1002/ese3.662
Li, Y., Su, B., and Dasgupta, S. (2018). Structural Path Analysis of India's Carbon Emissions. EnergyEconomics 76, S0140988318304316. doi:10.1016/j.eneco.2018.10.029
Liu, T., Wang, Y., Li, H., and Qi, Y. (2021). China's Low-Carbon Governance at Community Level: A Case Study in Min'an Community, Beijing. J. Clean. Prod. 311, 127530. doi:10.1016/j.jclepro.2021.127530
Lu, Y., Cui, P., and Li, D. (2016). Carbon Emissions and Policies in China's Building and Construction Industry: Evidence from 1994 to 2012. Building Environ. 95, 94–103. doi:10.1016/j.buildenv.2015.09.011
Lv, M., and Bai, M. (2021). Evaluation of China's Carbon Emission Trading Policy from Corporate Innovation. Finance Res. Lett. 39, 101565. doi:10.1016/j.frl.2020.101565
Ma, X., Wang, C., Dong, B., Gu, G., Chen, R., Li, Y., et al. (2019). Carbon Emissions from Energy Consumption in China: Its Measurement and Driving Factors. Sci. Total Environ. 648, 1411–1420. doi:10.1016/j.scitotenv.2018.08.183
Mallia, D. V., Lin, J. C., Urbanski, S., Ehleringer, J., and Nehrkorn, T. (2015). Impacts of Upwind Wildfire Emissions on CO, CO2, and PM2.5 Concentrations in Salt Lake City, Utah. J. Geophys. Res. Atmos. 120 (1), 147–166. doi:10.1002/2014JD022472
Marsden, G., and Rye, T. (2010). The Governance of Transport and Climate Change. J. Transport Geogr. 18 (6), 669–678. doi:10.1016/j.jtrangeo.2009.09.014
Meng, F., Chen, S., Cheng, S., Chen, B., Li, Z., Wang, F., et al. (2021). Analysis of Subnational CO2 Mitigation Policy Pressure in the Residential Sector in China. J. Clean. Prod. 293, 126203. doi:10.1016/j.jclepro.2021.126203
Ning, L., Pei, L., and Li, F. (2021). Forecast of China's Carbon Emissions Based on ARIMA Method. Discrete Dyn. Nat. Soc. 2021, 1–12. 1441942. doi:10.1155/2021/1441942
Pan, X., Li, M., Xu, H., Guo, S., Guo, R., and Lee, C. T. (2020). Simulation on the Effectiveness of Carbon Emission Trading Policy: A System Dynamics Approach. J. Oper. Res. Soc. 72, 1447–1460. doi:10.1080/01605682.2020.1740623
Shabani, E., Hayati, B., Pishbahar, E., Ghorbani, M. A., and Ghahremanzadeh, M. (2021). A Novel Approach to Predict CO2 Emission in the Agriculture Sector of Iran Based on Inclusive Multiple Model. J. Clean. Prod. 279, 123708. doi:10.1016/j.jclepro.2020.123708
Shi, Q., Chen, J., and Shen, L. (2017). Driving Factors of the Changes in the Carbon Emissions in the Chinese Construction Industry. J. Clean. Prod. 166 (17), 615–627. doi:10.1016/j.jclepro.2017.08.056
Sun, H., Edziah, B. K., Kporsu, A. K., Sarkodie, S. A., and Taghizadeh-Hesary, F. (2021). Energy Efficiency: The Role of Technological Innovation and Knowledge Spillover. Technol. Forecast. Soc. Change 167, 120659. doi:10.1016/j.techfore.2021.120659
Sun, H., Edziah, B. K., Sun, C., and Kporsu, A. K. (2019). Institutional Quality, green Innovation and Energy Efficiency. Energy policy 135, 111002. doi:10.1016/j.enpol.2019.111002
Tian, P., Li, D., Lu, H., Feng, S., and Nie, Q. (2021). Trends, Distribution, and Impact Factors of Carbon Footprints of Main Grains Production in China. J. Clean. Prod. 278 (20), 123347–123348. doi:10.1016/j.jclepro.2020.123347
Tian, Y., Zhang, J.-b., and He, Y.-y. (2014). Research on Spatial-Temporal Characteristics and Driving Factor of Agricultural Carbon Emissions in China. J. Integr. Agric. 13 (6), 1393–1403. doi:10.1016/s2095-3119(13)60624-3
Wang, S., and Liu, X. (2017). China's City-Level Energy-Related CO 2 Emissions: Spatiotemporal Patterns and Driving Forces. Appl. Energ. 200, 204–214. doi:10.1016/j.apenergy.2017.05.085
Wang, W., Zhao, D., and Kuang, Y. (2016). Decomposition Analysis on Influence Factors of Direct Household Energy-Related Carbon Emission in Guangdong Province-Based on Extended Kaya Identity. Environ. Prog. Sustain. Energ. 35 (1), 298–307. doi:10.1002/ep.12219
Wen, L., and Shao, H. (2019). Analysis of Influencing Factors of the CO2 Emissions in China: Nonparametric Additive Regression Approach. Sci. Total Environ. 694, 133724. doi:10.1016/j.scitotenv.2019.133724
Wright, T. (2013). The Governance of Energy in China: Transition to a Low-Carbon Economy. Philip Andrews-Speed. Houndmills, UK: Palgrave Macmillan, 2012. Xvi + 259 Pp. £57.50 ISBN 978-0-230-28224-7. China Q. 213, 217–218. doi:10.1017/S0305741013000143
Wu, C. (2021). Research on the Synergistic Effect of Low-Carbon Economy in China. Manage. World 37 (8), 12. doi:10.19744/j.cnki.11-1235/f.2021.0110
Wu, Z., Tang, J., and Wang, D. (2016). Low Carbon Urban Transitioning in Shenzhen: A Multi-Level Environmental Governance Perspective. Sustainability 8 (8), 720. doi:10.3390/su8080720
Xiang, X., Cai, Y., and Xie, S. (2020). Application of a New Information Priority Accumulated Grey Model with Simpson to Forecast Carbon Dioxide Emission. Jamcs 35 (2, 70–83. doi:10.9734/jamcs/2020/v35i230250
Xingang, Z., Tiantian, F., Lu, L., Pingkuo, L., and Yisheng, Y. (2011). International Cooperation Mechanism on Renewable Energy Development in China - A Critical Analysis. Renew. Energ. 36 (12), 3229–3237. doi:10.1016/j.renene.2011.05.035
Yang, H., O’Connell, J. F., and John, F. (2020). Short-term Carbon Emissions Forecast for Aviation Industry in Shanghai. J. Clean. Prod. 275, 122734. doi:10.1016/j.jclepro.2020.122734
Zhang, N., and Wei, X. (2015). Dynamic Total Factor Carbon Emissions Performance Changes in the Chinese Transportation Industry. Appl. Energ. 146, 409–420. doi:10.1016/j.apenergy.2015.01.072
Keywords: carbon emissions, forecasting, two-stage model, China, low-carbon economy
Citation: Zhang X, Xiong J and Song J (2022) Forecast of China’s Annual Carbon Emissions Based on Two-Stage Model. Front. Environ. Sci. 10:895648. doi: 10.3389/fenvs.2022.895648
Received: 14 March 2022; Accepted: 25 March 2022;
Published: 26 April 2022.
Edited by:
Huaping Sun, Jiangsu University, ChinaReviewed by:
Pengyi Liu, Yunnan University of Finance and Economics, ChinaCopyright © 2022 Zhang, Xiong and Song. This is an open-access article distributed under the terms of the Creative Commons Attribution License (CC BY). The use, distribution or reproduction in other forums is permitted, provided the original author(s) and the copyright owner(s) are credited and that the original publication in this journal is cited, in accordance with accepted academic practice. No use, distribution or reproduction is permitted which does not comply with these terms.
*Correspondence: Jianqi Song, MjgwNzI3MDE4QHFxLmNvbQ==
Disclaimer: All claims expressed in this article are solely those of the authors and do not necessarily represent those of their affiliated organizations, or those of the publisher, the editors and the reviewers. Any product that may be evaluated in this article or claim that may be made by its manufacturer is not guaranteed or endorsed by the publisher.
Research integrity at Frontiers
Learn more about the work of our research integrity team to safeguard the quality of each article we publish.