- 1College of Economics, Zhejiang University, Hangzhou, China
- 2School of Finance, Zhejiang University of Finance and Economics, Hangzhou, China
- 3School of Oriental and African Studies (SOAS), University of London, London, United Kingdom
- 4College of International Students, Wuxi University, Wuxi, China
The electricity crisis in Pakistan has been triggering grid power outages (load shedding) for many decades, which has not only affected the commercial and industrial sectors but also the domestic sector, specifically the livelihood of rural areas of the country. However, the extant literature advocates that renewable energy technologies (RETs), such as solar photovoltaic (PV) can be the remedy. Given the abundant availability of solar energy in Pakistan that can be converted into electrical energy using a solar PV system, this study examines the determinants of solar PV adoption in rural areas of Pakistan. Our preliminary investigations—using government/official publications—indicate that despite the huge potential of solar energy in Pakistan, the usage of solar PV systems at the household level in rural areas is still untapped, which makes this research agenda more appealing and provocative. In doing so, this study first conducts surveys, face-to-face comprehensive interviews, and questionnaires in four different districts of Pakistan and then implements a stepwise two-stage novel approach on a sample of 1,140 selected rural households. The first stage focuses on the determinants of solar PV system adoption, whereas the second stage focuses on the determinants of the type of solar PV system adopted. Using logistic regression, this study finds that age, education, children in school, income level, access to credit, gender (female), and price of a solar PV system are the factors significantly affecting the solar PV system adoption. In the second stage, we use a multivariate probit model and find that among these significant factors, the former five are significantly positive for the uptake of solar home-system, whereas the latter two are significant for both solar shed-lighting and solar panel-kit systems. In addition to these factors, landholding and access-to-road are significant for solar home systems, whereas household size, distance-to-market, and access-to-grid-electricity are significant for both solar shed-lighting and solar panel-kit systems. Since burning fossil fuels and solid biomass fuels for domestic energy needs are common in rural areas globally and cause carbon emissions and several severe health issues, the findings of this study are useful in many ways. In specific, we contribute to the literature examining the determinants of RETs in rural communities in developing countries.
1 Introduction
Fossil fuels are considered a major source of energy supply but intensively emit carbon (CO2) (Jefferson 2006). Many countries are heavily dependent on an unceasing stream of fossil fuels due to energy security. In the recent age, fossil fuels are not only getting harder to extract but are growing insufficient to shrink the gap of demand and supply, and additionally the growing imports of fossil fuels are deemed an economic injury specifically for countries which have undersized energy reserves and resources of their own (IEA, 2017). Furthermore, in nonurban regions, particularly in developing countries such as South Asia and sub-Saharan Africa, the basic energy consumption and reliance on unclean sources (e.g. biomass) is pullulating and inevitable (Karekezi 2002).
Being listed as an energy-deficient economy, Pakistan as a developing country is facing a severe energy crisis for many decades, and its energy demand does not match the existing stream of supply. The energy crisis in Pakistan is one of the biggest drains on its economy, and among many other reasons, one of the daunting factors of energy (electricity) supply scarcity is a substantial dependence on thermal-based power plants (Baloch et al., 2016), which is a costly source of electricity generation yet unavoidable to slake the country’s energy demand cycle. However, the downside of this significant reliance on thermal-based energy sources is plaguing the energy sector to face circular debt and confines it from continuous use and operation (Sheikh 2010). The reason behind this inter-corporate circular debt is the number of subsidies provided by the government to control the tariff. Alongside this kind of circular debt, the issues of less-functional and mismanaged power plants are major facets that drag down economic growth, the social system, and life in general under intense pressure (Rauf et al., 2015). However, the said situation of energy deficiency could be handled by shifting the electricity generation dependence away from single grid-based (conventional energy) sources to RETs sources.
The energy generation from solar PV technology is one of the most unique inventions in the line of RETs. Solar photovoltaic energy is recognized as a clean energy source that has an upheld advantage—i.e., reduction in greenhouse gas (GHG) emission—over non-renewable fossil fuel-based energy. In the recent age, the advancement of solar PV-based power generation technology has gained a real attraction globally, particularly for economies to elevate their energy portfolio and attain green development (Carlisle et al., 2014; Othman et al., 2021). Solar PV technology is increasingly the most expedient substitute, a viable solution to mitigate the requirement of electricity, especially in off-grid or under-electrified areas, and provides a facility to an entity for producing and self-consuming electricity with less maintenance (Palm 2017). At the household or small end-user level, the solar PV system has also uplifted the prominence of RETs and enabled its participation in the so-called “energy ladder” (Hiemstra-Van der Horst and Hovorka, 2008; Karytsas, Polyzou, and Karytsas 2019).
The International Energy Agency (IEA) has estimated 16% of global energy needs, which is proximately 6,000 TWh, could be generated through solar PV technology by 2050 (Othman et al., 2021). Globally, the total solar PV installed capacity has already crossed more than 500 GW in 2019. Conventional solar PV adoption at household level and in solar farms is promisingly trending upward since the last decade (Peng and Lu 2013). At the household level, in the product line of solar PV technology, solar shed lighting, solar panel kits, and solar home systems (SHS) are commonly known types and commercially available for customers, which are capable of providing the specific capacity of energy in form of lighting or electricity for the use of electric appliances. (Azimoh et al., 2017; Das et al., 2017).
Since the adoption of solar PV systems has boosted worldwide attention for energy security, attainment of sustainable development, and socio-economic growth, Pakistan is also seeking solar PV-based energy development projects to strengthen its energy security and overcome the long-lasting energy crisis. However, Pakistan has relatively a great advantage of its location which lies in an excellent solar belt range, and its subtropical zone makes it favorable for solar energy (Adnan et al., 2012). The annual average sunshine of the country is 8 h a day, which is favorable, and on average will generate 5.2 kWh/m2/day of electricity production on a horizontal surface of solar photovoltaic panels (Khan et al., 2020). Apart from its geographical advantage for solar PV energy production and among other projects, the adoption of solar PV systems on the household-level remains mostly untapped, specifically on the rural side, and comparative very slower than other developing Asian countries (Ali et al., 2016; Qureshi et al., 2017). Previous literature on solar PV adoption has documented the influential factors to uptake RETs in Pakistan. The increasing energy demand is being managed through exceptionally high load-shedding—a supply-cut from households to fulfill the need of the industrial sector—in Pakistan, consequently, the energy demand of households has increased from 35% to 46.5% during the past 2 decades (Aqeeq et al., 2018). A growing body of literature and actual concerns about energy security suggests that residential solar PV adoption has a significant impact on both households’ energy needs as well as on the environment.
It is largely debated that the general public is more concerned about the ease of using technology as compared to the usefulness of the technology, such factors play an important role to affect the intentions of households towards technology use and adoption. Consequently, numerous studies shed light on installation costs, maintenance, and repair services (Rai et al., 2016; Qureshi et al., 2017) information and awareness (Qureshi et al., 2017; Jabeen et al., 2019; Jan et al., 2020), the demographic, socio-economic, and infrastructural factors such as age, sex, education level, household income, household size, assets and landowning, ease of access to transport, and credit facilities, play an important role and influence the adoption of solar PV systems at household level (Guta 2014; De Groote et al., 2016; Rahut et al., 2018). Regarding the determinants of a household’s adoption of a solar PV system, the studies by (Sommerfeld et al., 2017) comprehensively illustrate the assessment of demographic variables and their influences on household solar PV system adoption. (Bashiri and Alizadeh 2018). brought out the findings regarding factors affecting household choices of solar PV system in Tehran. Similarly, (Kurata et al., 2018), inspected the determinants of Solar Home System (SHS) adoption specifically focusing on the resemblance and variances between households and micro-enterprises levels. The extant literature (Chowdhury et al., 2014; Vasseur and Kemp 2015; Qureshi et al., 2017) show that the influencing factors, such as financial position, awareness, technical knowledge, social impacts, and public policy, significantly impact the households’ behavior towards adoption of solar PV systems.
The majority of studies exploring household adoption of solar PV systems in Pakistan have mainly focused on the determinants of adoption by comparing the differences between the characteristics of adopters and non-adopters; however, this straightaway ignores the types of solar PV systems adopted by the households. Therefore, the main contribution of this research is to comprehensively understand the determinants of both the adoption of solar PV systems and the types of solar PV systems adopted by households in rural areas of Pakistan.
This study uncovers the potential attributes, such as economic motivations, environmental considerations, demographic characteristics, and infrastructural and institutional aspects which can address the determinants of households’ adoption of solar PV systems and more hypothetically the types of solar PV system preferences in Pakistan. In doing that, the research starts with conducting surveys in selected villages comprising the total sample size of 1,140 households across four districts of Pakistan—namely Dera Ismail Khan, Bhakkar, Tank, and Lakki Marwat. This study is unique in two ways; the first part attempts to analyze the factors affecting the household’s adoption of a solar PV system, and the second part aims to find the factors affecting the household’s (users) choice among three common types of solar PV system, which are 1) solar shed lighting, 2) solar panel kit, and 3) solar home system. The factors associated with the adoption of solar PV system includes age, gender, education, family size of household head, annual net income, access to a credit facility, access to electricity, children in school, landholding size (space availability), access to road, distance to market of household, price of the solar PV system, and the location (districts wise).
In the first stage of empirical analysis, the logistic regression approach is employed. The results show that, among 13 selected variables, the variables; gender (female), age, level of education, children in school, family size, access to credit, net annual income, and space availability are found positive and significant; only the factor ‘price of solar PV system’ is negative, but significantly affects the household’s adoption of a solar PV system. In contrast, access to permanent road, access to gird electricity, distance to the nearest market, and location (district-wise differences) factors are found to be not significantly associated with household adoption of a solar PV system.
In the second stage of empirical analysis—the determinants of household choices associated with the types of solar PV system—an appropriate statistical approach is used; the Multivariate Probit Model (MVP). The results illustrate that the variables; age, education level, school-going children, higher income level, having access to credit facilities, and easy access to a road, significantly affect the households’ adoption towards favoring SHS. In contrast, being a female house-head, family size, the increase in the distance to market, access to electricity, and high solar PV system prices are factors pursuing the households’ choice towards solar shed lighting and solar panel kits. This study also performs a comparative analysis by investigating district-wise bifurcation and suggests that infrastructural development, promotional activities, solar PV system services, RETs substitutes, and other social factors play important roles in the adoption decision of different types of the solar PV system.
The remainder of the studies is organized as follows: Section 2 provides a detailed description of the proposed methodology, theoretical framework, and empirical modeling, section 3 illustrates the results efficacy of demographic, socio-economic, and infrastructural parameters in detail, and section 4 concludes this study and offers few recommendations.
2 Methodology and Framework of the Study
2.1 Sampling Procedures and Techniques
This study is undertaken in the four districts of the northwest side of Pakistan as shown in Figure 1, the districts: Dera Ismail Khan, Bhakkar, Tank, and Lakki Marwat are selected due to their cumulative 85% area comprising rural livelihood. Administratively, the districts are subdivided into Tehsils, Union Councils (UCs), and UC-Wards. District Dera Ismail Khan spreads over 9,334 km2, and the proportion of its urban area is 21.27%, and 78.73% rural area. It contains five Tehsils, 47 UCs, and 174 UC-Wards with a ratio of 48.4% men and 51.5% women of a total 1,693,594 population, and the literacy rate is nearly 44.52%. District Bhakkar’s total area is 8,153 km2 and contains four Tehsils, 64 UCs, and 220 UC-wards. Out of district Bhakkar’s total population of 1,647,852, 51.16% are men and 48.84% women with a proportion of 15.76% urban and 84.24% rural livelihood, having a literacy rate of about 51.82%. District Tank holds a total area of around 2,900 km2–11.02% urban and 88.98% rural—and contains one Tehsil, 16 UCs, and 87 UC-Wards. The literacy rate is proximately 40.98%; its total population is 427,044 (52.19% men, 47.81% women). District Lakki Marwat is scattered over 3,296 km2–9.89% urban and 90.11% rural—and contains two Tehsil, seven UCs, and 94 UC-Wards. The literacy rate is proximately 44.13% of its total population of 902,138 (52.19% men, 47.81%women). All four districts are geographically connected to Dera Ismail Khan and entail wide plan agriculture land which is substantially suitable for solar PV technology (LGCD, 2021; LGKP 2021).
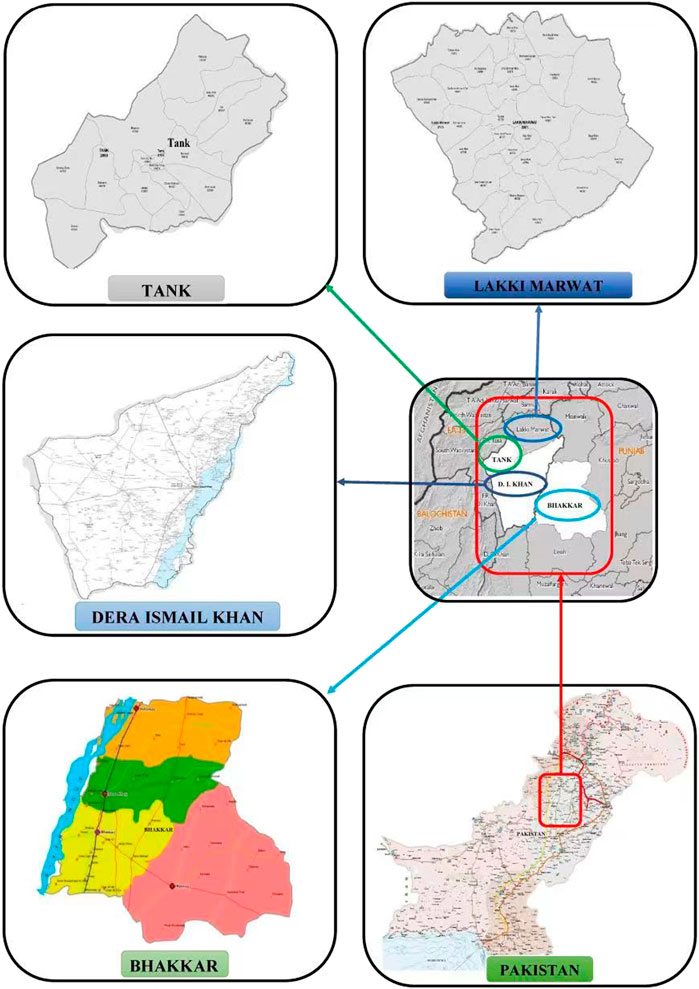
FIGURE 1. Map of the target site (District Dera Ismail Khan, Bhakkar, Tank, Lakki Marwat), Pakistan. Source: (IVNM.net, 2021; LGCD, 2021; LGKP 2021).
2.2 Questionnaire and Survey
A study starts with a scheme of cross-sectional survey and semi-structured questionnaire for data collection from the households. The cross-sectional data information is based on socio-economic, demographic, institutional, and infrastructural characteristics of inhabitants of the four districts; Dera Ismail Khan, Bhakkar, Tank, and Lakki Marwat. The precision, reliability, and range of the data have been immensely refined with the key informants’ help, local languages’ privilege, the cross-sectional loop of open/close questions, and face-to-face interviews sessions. Consequently, the outline of our survey—conducted between September 2020 and August 2021—imperatives also involved information which is collected from solar PV system experts at the village/district level for further affirmation and supplement of the data curation. Secondary data which is used in this study has been collected from several sources, such as the official reports of government offices (LGCD, 2021; LGKP 2021), published research material (Ali et al., 2017; Ali et al., 2021; Luo et al., 2021), public survey data information of different NGOs (unpublished), online data available from prestigious institutions, and scientific organizations and associations (NTDC 2021; PBS 2021).
The random stratification sampling method for data collection is based on insights, whether the households use solar PV technology or not, and what type of solar PV system the households mainly use. Out of the total 134 UCs of all four districts, the 114 UCs contain rural areas villages that are proposed for the study target site with consideration of taking 10 households from each UC as the total sample size. Therefore, a random selection of a total 1,140 households is proposed for the sample size. The data in Figure 2 demonstrate that 611 households are found as potential users of solar PV systems and the remaining 529 households are found as non-solar PV system users.
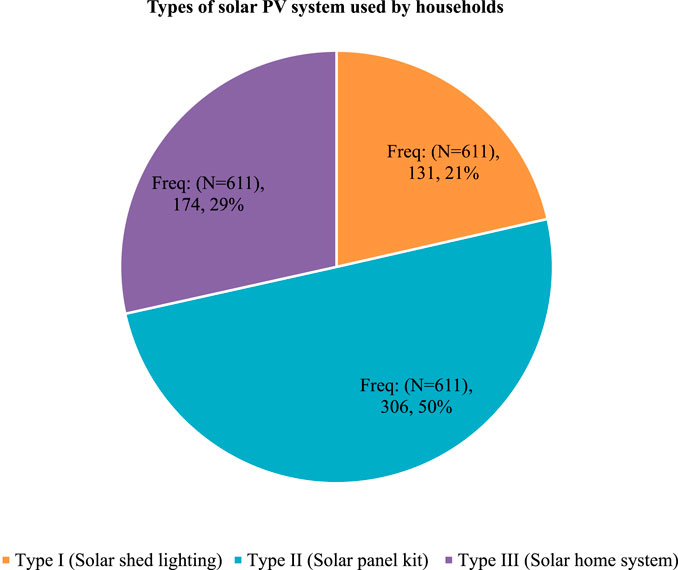
FIGURE 2. Types of solar PV system used by households, Source: Own survey data September 2020- August 2021.
2.3 Theatrical Framework
2.3.1 Consumer Behavior of Technology Adoption
The fundamental theoretical framework designed for the first part of this study is based on consumer behavior in the context of economic theory. The households are subjective to have an expected inclination toward solar PV technology adoption, which is generally fetched from the characteristics that a given technology represents the effect of the adoption decisions (Adesina and Baidu-Forson 1995; Somda et al., 2002). It is supposed that energy needs and related issues are categorized by the households and then ranked according to their preferences (categorically) i.e. socio-economic, demographic, environmental, infrastructural, and other evident traits. Therefore, technology adoption can be treated as a preference between two alternatives, i.e., RETs and traditional ones (Qureshi et al., 2017; Tareen et al., 2018). In this scenario, a preference—for technology that is likely to generate a higher utility—will expectedly be required more by households’ inhabitants. This study supposes that households choose to adopt solar PV technology which can be denoted by
A utility-maximizing household would only adopt the new technology
2.1.2 Household Energy Choice and Transition
The theoretical framework is designed for the second part of this study, based on the energy transition process the “energy stacking” and the “energy ladder” (Campbell et al., 2003; Heltberg 2004). They are two mainstream theories often presented by researchers that explain household energy choices (Masera et al., 2000; Li et al., 2013; Wassie et al., 2021). The energy ladder model (ELM) explains that the choice of the household switches from one type to another due to socio-economic factors (Masera et al.,2000). Climbing up the energy portfolio from the bottom like a ladder, ELM divides the household energy choices into three rungs: 1) Primitive/basic level, 2) Transitional level, 3) Advanced or modern level (Masera et al., 2000; Andadari et al., 2014). The energy ladder model explains that household energy choices are primarily determined by socioeconomic e.g. income levels. Due to the ‘income’ factor, the households experience linear energy swapping as their income level changes.
Conversely, the energy-stacking model (ESM) explains a household’s diversification of energy portfolio (use of multiple energy sources), instead of completely jumping from one source to another source, or in other words, households would rather expand the energy portfolio and use of mix fuels due to influencing factors (Masera et al., 2000). According to (Muller and Yan 2018), the ESM describes the household energy’s transition as associated with socio-economic, cultural, demographical, social, and infrastructural factors instead of a solely income-based unidirectional energy-switching. Theoretical and empirical evidence suggests that a household’s energy choices are greatly influenced by several factors (Campbell et al., 2003; Heltberg 2004).
2.4 Empirical Modeling
2.4.1 Logistic Regression (Logit) Model
For empirical analysis of the study, in the first step, the logistic regression model is employed to determine factors affecting solar PV technology adoption. The model applies maximum likelihood estimation after transforming the dependent into a logit variable (Swider et al., 2008). Logistic regression is widely used—when the dependent variable is dichotomous and the independent variables are of any type “it estimates the odds of a certain event occurring, and the dependent variable is binary, which is the natural log of the odds (logit)”—and considered as the most appropriate model. The model can be described as follows:
A dummy variable is used to categorize whether the household is a potential solar PV system user or a non-user.
The contracted form of formula is used in this logistic regression model, as shown in Eq 3.
Since the probability of non-event occurring is (1−prob (event)) the new equation is as follows:
where
The dependent variable represents as
2.4.2 Multivariate Probit (MVP) Model
In the second step, our findings unfold that the households use different types of solar PV systems as shown in Table 3 which represents Type I (solar shed lighting), Type II (solar panel kit), and Type III (solar home system). The households’ choice of adoption of the three different solar PV system types depends on demographic, socio-economic, institutional, and infrastructural factors that are shown in Table 1. To find that we use the MVP which is an appropriate method to analyze correlated multivariate binary outcomes. In contrast, the single equation Probit and multinomial Probit models do not predict joint interdependence of binary outcomes however the MVP model is capable of assessing joint prediction (Asfaw et al., 2016; Wassie et al., 2021). The MVP model is based on the random utility model (Mcfadden 1974). In this model, each participant makes an adoption decision to maximize one’s utility. The utility function
where
Note that if the expected utility is larger than 0, then individual
Further, the choice probability
Since the MVP model can be applied to multiple choice situations, the choice probability is adjusted in Eq 7.
where.
More specifically, the model considers three dependent variables and takes the following form in our study (Behera et al., 2015; Ali et al., 2019).
And
where X is a vector of the explanatory variables;
2.5 Explanatory Variables for the Adoption of a Solar PV System and Its Types
The range of explanatory variables we have used in this study is based on experiencing the practical field observations and considerations of preceding similar literature. The findings documented by (Qureshi et al., 2017) refer to the adoption behavior that explains the socio-economic, demographic, and infrastructural factors are the main determinants linked to the adoption process. In our study, the variety of households’ characteristics based on similar–demographic, socio-economic, institutional, and infrastructural—factors were considered for the logistic regression model and MVP model. The list of explanatory variables taken in this study is summarized in Table 1 by defining the characteristics of each variable. Likewise, the three common types of solar PV system (binary dependent variables) used by the households in all four districts are shown in Table 2 by defining the descriptions of each technology type.
2.6 Profiles of Sampled Households
Table 3 presents the frequency and average stats values of the demographic, socio-economic institutional, and infrastructural parameters of the households. In the total sample size of 1,140 households, the proportionate respondents of districts Dera Ismail Khan, Bhakkar, Tank, and Lakki Marwat are 349, 294, 236, and 261 households (in numbers) respectively. We found 91.3% of households are male-headed and 8.7% are female-headed families. The average age of the household is 47.6 (in years) and the family size is 6.44 (in numbers). On average household education level is 7.93 (in years), school-going children are 2.72 (in numbers), and space availability is 449 (in square meters). The average annual net income of the household is 696,500 (Pakistani Rupee). Out of the total sample size, 397 households own the credit facility, 731 households have a grid electricity connection and 321 households have easy/direct access to roads. The round trip (distance) to the nearest market is proximately 65.20 min (6.5 km) on average.
3 Results
3.1 Determinants of Households’ Adoption of Solar PV System
The results of binary logistic regression in Table 4 show the fitness of the model (Prob > chi2 = 0.0000), which is indicating the results of the model are useful and cannot be considered sporous. Although the Pseudo R2 is not very high (45.28%), it does not affect the quality of the model given that the pseudo R2 in logistic regression illustrates a different meaning than the R2 in Ordinary Least Squares (OLS) regression (Kabir et al., 2013).
Age: The results of Table 4 show that the age of the household head is significant (p-value = 0.0186) and positively associated with solar PV system adoption. It shows that in the rural areas, the aged inhabitants heading their families probably adopt solar PV systems more than their younger counterparts. Table 4 further shows that as the age ratio increases year-wise, the odds ratio of adoption also rises factor-wise (1.049). Usually, the old age villagers are comparatively wealthier, have higher economic status, more experience, and have the ability to partake in financing the latest technologies. Therefore, for them the initial cost investment decisions are easy. The previous literature indicates the link between age and RETs adoption is sensitive to factors variations. Thus, the exertion of age on technology adoption cannot be driven as deducible, such as (Kabir et al., 2013) and (Bekele and Drake 2003) report positive association, while (Smith and Urpelainen 2014) and (Walekhwa et al., 2009) report negative associations between age and adoption of RETs.
Household size: The results (p-value = 0.0002) indicate that household size has a substantially positive effect on solar PV system adoption. The odds ratio increases by a factor of aproximately 1.27. It might be due to the reason that a larger family often requires more energy as compared to a smaller family. In the survey, we found the larger families choose solar PV systems as a substitute energy source and a better option to fulfill their daily need in a time of electricity load-shedding. This finding can also be linked with studies of (Kelebe et al., 2017; Jan et al., 2020), but in contrast to the findings of (Kabir et al., 2013).
Gender: The female-headed households as compared to male counterparts have a high drive to adopt solar PV technology, which is statistically significant (p-value = 0.0115). Practically, women are the real victims of energy deficiency as they spend most of their time working at home and suffer from electricity load-shedding problems, thus such circumstances drive women’s willingness toward solar PV system adoption in comparison with their male counterparts, assuming other factors are constant. Interestingly, (Mwirigi et al., 2014; Mengistu et al., 2016), reported that male-headed households are more likely to adopt new technologies as compared to female-headed households in Ethiopia and Kenya, respectively.
Education level: The association between the household head’s education (in years) and solar PV system adoption is positive and significant (p-value = 0.043). An increase of 1 year in education level increases the system PV system adoption by the odd ratio 1.42, which is the highest among all the odd ratios in the demographic category. It is perhaps considered that a better education helps quick decision-making towards RETs adoption, the low grade of literacy generally averts the working flow of facts. The findings of (Guta 2014; Urpelainen and Yoon 2015; Kelebe et al., 2017) reveal a positive association between RETs adoption and level of education.
Children in school: The results (p-value = 0.0102) show a significant and positive relationship between the factor ‘children in school’ and households’ solar PV system adoption. Since school-going children of households not only represent educated and the wealthier families but also push their parents to adopt solar PV systems as they get to know about the advantages of technological innovation (e.g., solar PV technology) through educational institutes. Further, it is also revealed from the survey that upon unavailability of electricity, the solar PV system is a suitable option among other RETs for the households to provide clean/bright lighting energy sources to children which can be helpful for their studies.
Income: Income is one of the most crucial factors in making any kind of decision, especially in rural communities of developing countries (Karytsas et al., 2019; Wassie et al., 2021), since households are typically financially unsound in rural areas of developing countries. However, face-to-face interviews with households reveal that the wealthier households prefer to choose solar PV systems with their energy mix portfolio. The results (p value = 0.0000) and (odds ratio = 5.001)—which is the highest among all the odd ratios in the socio-economic category—also validate that income is a positive and significant factor for households’ solar PV system adoption. Conversely, the households with low annual income either cannot afford the solar PV system or rely on cheap kinds of energy sources. The findings of (Scarpa and Willis 2010) are inconsonant with studies an increase in the income level increases the adoption of RETs as energy substitutes.
Space availability: The large space availability is more condign for the installation of a solar PV system. The results of Table 4 show statistically significant (p value = 0.0273) and positive for household solar PV system adoptions. Usually, in the rural villages, households have larger and single-story houses as compared to urban areas, besides, most rural inhabitants do crop field farming business and reside near fields so they have enough space available for the installation of a solar PV system, that link the likelihood of the households with solar PV system adoption. The authors (Ali et al., 2016; Powell et al., 2021) stated a similar finding.
Access to credit: Table 4 results (p-value = 0.0004) and (odds ratio = 2.47) infer the information that access to credit allows the village inhabitants at first to shield the initial investment cost of a solar PV system. The positive association hence shows that the credit facility significantly affects the households’ willingness to adopt solar PV systems. The other most important reason is that the rural households have seasonal income that is based on agriculture harvesting seasons. Therefore, access to credit can help them to cover the initial expense of a solar PV system in meantime. The (Gwavuya et al., 2012; Mengistu et al., 2016) findings are somehow identical to our studies, the results show a positive association between the access to credit and the adoption of RETs.
Price of solar PV system: Higher prices of RETs, such as solar PV technology are considered one of the most decisive factors that affect the decision and willingness of the rural households towards owning the solar PV system, specifically in the village communities of developing countries. The results (p-value = 0.0124) in Table 4 show negative but significant associations between the prices of solar and households’ adoption. Previous findings (Wassie et al., 2021) are congruent to our findings that a huge proportion of the village households still rely on unclean sources and cannot afford such expensive technologies in the rural area. The authors (Scarpa and Willis 2010) also reveal the high prices of RETs influence the household’s willingness to adopt.
Location: This study particularly highlights the location factor, whether, the adoption decision of households for a solar PV system is influenced by numerous differences exist between districts (114 UC’s villages). In terms of location-based solar PV system adoption at the household level, our results are insignificant for all districts; Dera Ismail Khan, Bhakkar, Tank, and Lakki Marwat. It is indicating that the solar PV system adoption behavior is similar in all districts. It is interesting to note that—during the survey and collection of data—the district Dera Ismail Khan is better in prospects of availability of the variety of RETs, infrastructure, and has a higher literacy rate, but still, the results are found insignificant. However, the households’ choices between the types of solar PV systems are relatively distinguished across all districts, which is comprehensively discussed in the following section of MVP results.
Access to road, market, and electricity: Easy access to roads helps in easy transportation of goods, in our case the road infrastructure of the rural villages in all four districts is not good, so the result (p-value = 0.1105) of factor ‘easy access to road’ is found insignificant for households’ solar PV system adoption. In previous studies (Wassie et al., 2021; Kelebe et al., 2017; Karytsas et al., 2019), it is found that the easy access to roads increases the willingness of households towards the adoption of clean energy and RETs. Similarly, the households residing near the market have the convenience of easy access to RETs but in our case, the villages’ markets have limited availability of solar PV systems across all four districts. Furthermore, a household residing near the market has easy access to a grid electricity connection, which affects the willingness of household solar PV system adoption as results show (p-value = 0.064) are insignificant. According to (Michelsen and Madlener 2012; Kelebe et al., 2017) easy access to the market increases the willingness of household RETs adoption. Consequently, in Table 4, the factor ‘access to grid electricity’ result (p-value = 0.0893) is found insignificant for the rural inhabitants’ adoption of solar PV systems. It suggests that easy access to electricity affects the household’s adoption of solar PV systems, as is similar to (Smith and Urpelainen 2014)’s findings, that the households having grid electricity connections are less likely to adopt solar PV systems. Another related reason might be because the solar PV system is suitable to be used in daylight and (in most the cases) it is not powerful enough as compared to grid electricity to provide sufficient electric power, in contrast, the grid electricity system provides a stream of electricity which can fulfill the need of households after sunset thus reduce the chances of solar PV system adoption.
The above logistics regression results interpret the overall scenario of demographic, socio-economic, institutional, and infrastructural factors affecting the household’s adoption of solar PV systems. The following section provides a comprehensive explanation of the determinants of households’ choices associated with the three different types of solar PV systems.
3.2 Determinants for Adoption Decision of Different Types of Solar PV System
In this section, we analyze what type of solar PV system among three different options—type-I (solar shed lighting), type-II (solar panel kit), and the type-III (solar home system)—the households choose to adopt. Choosing the MVP model with robust standard errors fits for the analysis of the key factors influencing households’ selection among different types of solar PV systems. For imperial analysis, it is important to know the multicollinearity between explanatory variables, which can misdirect the findings. Hence, the Variance Inflation Factor (VIF) test is commonly exercised to examine whether the values are within the acceptance range or not (i.e., the threshold value of VIF <10). All variables are found below the threshold value of 10. In addition, to normalize the data and drop outliers, Z-scores are calculated to reduce biases, and a cut-off value of ±3 is used (as suggested by the relevant literature (Vu et al., 2015). Note that the higher values of the Z-score indicate more unusual observations, whereas 0 indicates a value that equals the mean.
Table 5 provides the estimation of coefficients (βi), whereas Table 6 illustrates the marginal probability effect (Yi = 1) of factors explaining households’ choices between solar PV system types. The Wald Chi2 45) = 317.63 (Prob > Chi2 = 0.0000) is statistically significant at any commonly referred conventional significance levels (e.g., at the 1% level; α = 0.01). Thus, the results of the model can be considered reliable.
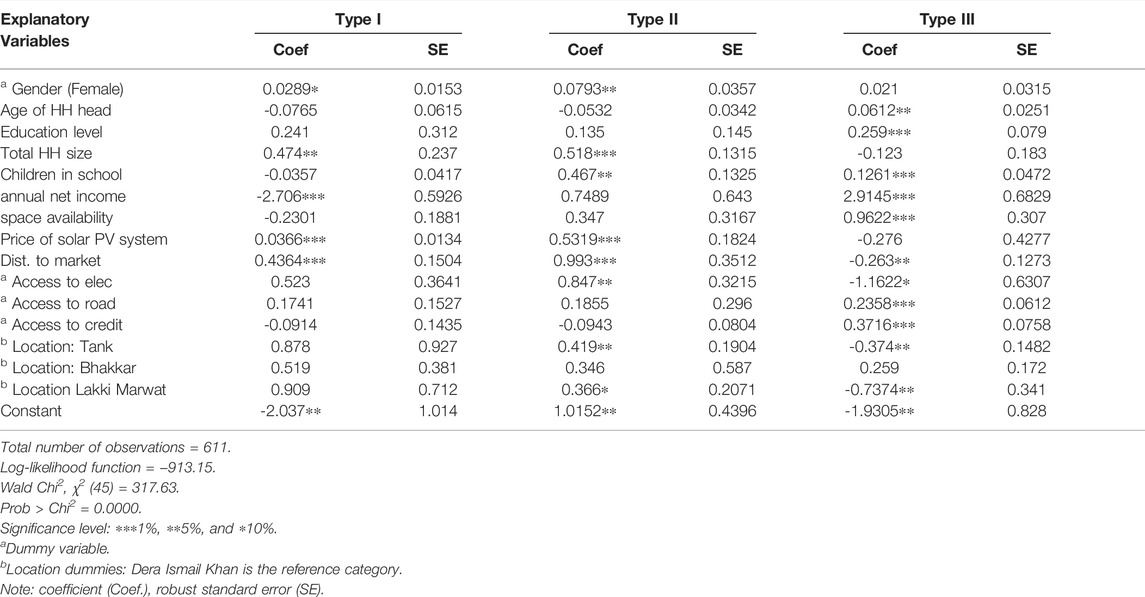
TABLE 5. Factors affecting the household’s choice for different solar PV system types: MVP model estimation.
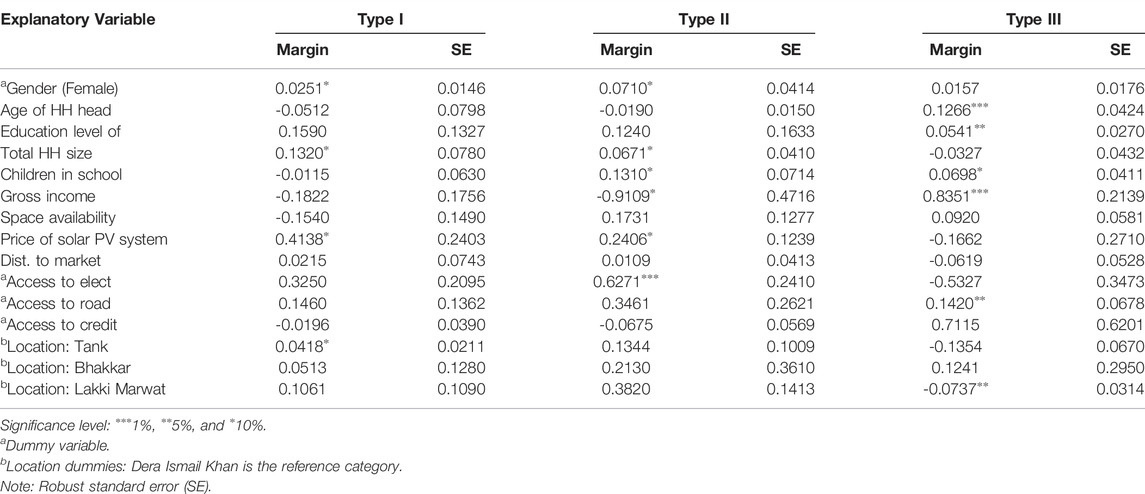
TABLE 6. Marginal effects of explanatory variables affecting the household’s adoption of solar PV system types.
Gender: In Table 5, Table 6, both the coefficients and marginal probability estimations of gender (female) are found statistically positive and significant for type I and type II. It enunciates that in rural areas female-headed households have a higher tendency toward solar shed lighting and solar PV kit. It is because the mobility, maintenance, and repair of solar shed lighting and solar PV kit are relatively easy as compared to the solar home system. Furthermore, in surveys, the female-headed households are found comparatively less wealthy and have lower economic status, hence can not afford SHS.
Age: The coefficients and probability estimations of the household head’s age are positive and significant for type III. The former relationship indicates that the elder households may prioritize comfort and require palliative care with their living standards. In addition to that, the elder households have more resources and savings and can bear the cost of expensive RETs i.e. solar home systems as compared to the younger households in the villages. The findings of (Wassie et al., 2021) stated that young rural villagers have a high obsession with advanced RETs as compared to old-age villagers.
Education level: Table 5, Table 6 illustrates that the education level of the household head has a positive and significant association with the type III solar PV system. This clearly indicates the importance of literacy for adopting and using the better option of the modern energy system. However, the insignificant results associated with solar shed lighting and solar PV kit infer from the survey information that the educated farmers’ households residing near field farms (in off-grid areas) are leaning towards SHS. A substantially large number of studies are assaying that the educational level plays a significant role in the adoption of clean energy sources (Islam 2014; Aarakit et al., 2021).
Household size: The results of both coefficients and marginal probability estimates of the household size are positive for Type I and type II. Our survey findings and previous literature (Lodhi et al., 2021) affirm that most of the families live in a joint family system in one big house, which is very common in Pakistan’s village livelihood. It is undeniable that households with larger family sizes need more sources that can provide a sufficient amount of energy to satisfy their daily need (Bhandari and Jana 2010; Karytsas et al., 2019; Aarakit et al., 2021). During the survey, in face-to-face question sessions, it is assessed that a solar home system (type III) is not a suitable option for such large households’ families, and is conceivably very expensive. Larger families using multiple sources in the energy mix and owning a solar panel kit (type II), or multiple ones, is a suitable option.
Children in school: Table 5 shows positive and significant relationships between school-going children and type II and Type III. Although the educational institutes play a significant role in the way that the parents (households) are acknowledged through their school-going children learning about advantages of RETs e.g. solar PV technology that are more user-friendly, clean, and brighter lighting options (apart from the electricity), as compared to unclean sources like kerosene lamps, generators, and similar substitutes (corban gas emission). The parents (households) both rich and poor—off-grid and grid-connected—are prudent about their childrens educations and prefer to adopt solar PV systems, which can help in providing efficient, clean, and brighter lighting for school going children’s studies. In India (Sharma et al., 2019) and Zambia (Gustavsson 2007) findings displayed that solar PV technology plays a significant role and benefits children’s education.
Household income level: Both the coefficients and marginal probability estimates are positive and significant for type III. The grid-connected wealthier households are found to have a solar grid-tied system (SHS) that allows the eco-power energy (electricity) to fulfill the households’ need not only in time of grid electricity load-shedding but also in reducing the annual marginal cost of electricity. Similarly, in off-grid areas, the rich households also own the facility of an off-grid Solar System (SHS) that could provide 24 h energy efficiently. In contrast, the coefficient and marginal probability of the household’s income portray a negative but significant relationship with solar shed lighting (type I). The findings by (Jan et al., 2020; Aarakit et al., 2021) are inconsonant with our studies, that high-income households lean toward a reliable RETs energy portfolio.
Space availability: The coefficient of factor ‘space availability’ is positive and significant for type III. Such results are in favor of those households occupying larger land that relatively provide more space availability (in most of the cases) for installing a large solar PV system (type III). In contrast, the coefficient results in table 5 illustrate space is insignificant for type I and type II solar PV systems, it is probably that households that own smaller land have small space availability or incompatible rooftop, thus select compact or smaller solar PV systems (type I or type II) that are compatibly fit in small available space. The finding (Carlisle et al., 2014; Othman et al., 2021) paraded that the availability of land substantially supports the adoption of solar PV technology.
Price of solar PV system: Table 5, Table 6 demonstrate positive and significant associations between the prices of solar PV and Type I and Type II. This means that an increase in the prices of solar PV systems impels rural households either to choose solar shed lighting or solar PV kit that are comparatively cheaper than SHS. The negative and significant results associated with type III illustrate that the high cost of SHS, its installation, repair, and maintenance affect the village household willingness to own such a system. In the villages, a huge proportion of the households cannot afford such an expensive system. our findings are consonant with the study of (Mukisa et al., 2022) “SHS adoption in Sub-Saharan African countries”, interpreting the influence of high prices of SHS on household willingness of adoption.
Access to credit: The relationship between access to credit and SHS (type III) is found positive and significant. In the rural area, most of the households are professionally crop-farmers and crop-dusters, those households are often encouraged for credit offers (loans) by private banks as agriculture loans which is auspicious to cover the initial cost of the solar PV system. Similarly, the government entities also encourage the use of RETs at the provincial level by giving special rebates (offers) to farmer households (those engaged in agricultural activities). The prevailing literature unfolds the aspect of access to credit facilities positively and significantly influences the households’ solar PV system adoption (Ali et al., 2016; Kizilcec et al., 2021; Wassie et al., 2021).
Access to near market: The coefficient is found negative and insignificant for type III, indicating that the easy access to the market does not uplift the households’ adoption of SHS. During our survey—face-to-face interview sessions–households revealed that the SHS businesses are not eminent in the rural area market as compared to urban areas and the maintenance and repair services of SHS are not easily available in rural area markets. The households residing near rural markets have easy access to solar shed lighting and solar PV kit. This is further acclaimed in results that the coefficient and marginal probability estimates are positive and significant for type I and type II. According to (Doner 2007; McEachern and Hanson 2008; Qureshi et al., 2017), access to the near market is an influencing factor that affects the household’s willingness of adoption of solar PV systems.
Access to electricity. The negative and significant coefficient results associated with type III infer that the grid-electricity-connected households do not prefer to own SHS. In previous literature, it is revealed that rural inhabitants’ decisions for solar PV system adoption are greatly influenced by easy access to grid electricity (Aarakit et al., 2021). However, it is also found that grid electricity services with supplementary RETs e.g., solar PV system is considered a workable strategy for rural inhabitants’ energy mix portfolio (Urpelainen and Yoon 2015). Table 5, Table 6 results portray that access to electricity coefficient and probability estimates are positive and significant for type II only, it is because, in the rural villages the solar PV kit is more suitable for daytime use for multiple purposes—lighting, fan, charging batteries, and ordinary energy needs—while rest of energy need of household might be covered with grid-based electricity or other types of energy-mix (in off-grid areas) after sunset.
Access to road: The coefficient and probability estimates both are positive and significant for type III only. The households residing near the main or link road are in great favor for easy access to transportation facilities of SHS (type III), alongside the SHS service providers including installation, maintenance, and repair. The insignificant results for solar shed lighting and solar PV kit show that these types of solar PV systems can easily be transported to any location whether having easy access to a road or not.
Location: The district-wise adoption of the households associated with solar PV system types is found uneven. The households of district Dera Ismail Khan are the highest among all the districts to choose solar PV system type III. It is perhaps the spill-over effect of its urban side area which is an indirect influence on the rural households to choose such an option. The choices of the villagers of districts Tank and Lakki Marwat opted more towards type II (solar kits system). This infers that the villages under UCs of said districts are rather more remote or SHS is adequately not that promoted as compared to district Dera Ismail Khan. Consequently, Table 5, Table 6 insignificant results of factor ‘location’ illustrate that the proportion of solar PV system type I usage is similar across all four districts, however, insignificant results of location district Bhakkar illuminate that the tendency of households’ RETs adoption is more pronounced towards biogas systems. The authors (Iqbal et al., 2013; Jabeen et al., 2019; Yasmin and Grundmann 2019) mentioned that the government of Punjab is adeptly encouraging its rural residents to install biogas systems, thus somehow influencing the adoption of solar PV systems.
4 Conclusion and Recommendation
Choosing the right and neccessary option in energy generation technologies is becoming significantly important due to environmental pollution and the economy. In the midst of all the economic and infrastructural development issues, one prominent and clean source of energy generation is solar PV technology, which has the ability to scale down the long-standing electricity shortage in the country, in addition, standalone solar PV technologies indulge as an instantaneous workable alternative to electricity at the household level. However, solar PV system adoption is still unelevated in the rural livelihood of Pakistan.
This study unfolds the determinants of household adoption of solar PV systems and their types. An appropriate scheme of study through surveys, face to face interview sessions, and questionnaires in four different districts of Pakistan, the first step assents the logistic regression model and portrays the results of—demographic, socio-economic, institutional, and infrastructural—factors: gender (female), age, level of education, children in school, family size, access to credit, net annual income, space availability are found positive and significant, except the single factor ‘price of solar PV system’ which is negative but significantly affecting the households’ adoption. The second step enacts the multivariate probit model and finds the factors that affect the households’ (users) choices associated with three different types of solar PV systems: solar shed lighting, solar panel kit, and solar home system. The results show that the household head’s age, education, children in school, income, land availability, access to road, access to credit are the factors resulting positive and significant for the SHS, however, the factors; gender (female), family size, price of solar PV system, distance to market, and access to electricity are significantly affecting households’ choices for solar shed lighting and solar panel kit. The users of SHS in district Dera Ismail Khan are highest across other districts while the villagers of districts Tank and Lakki Marwat are more opt towards solar panel kit.
The study finds that demographic, socio-economic, and institutional factors are major traits that suggestively affect the adoption of solar PV system decisions of rural inhabitants. The infrastructural and environmental factors also play an important role, however, they are contingent on policy analysis concerning the national, and domestic energy supply and developments scenario. All factors are significantly important for solar PV technology elevation. The diffusion process ought to be planned in a way that ensembles local circumstances instead of following the hypothetical strategy for all settings.
Due to weak financial positions, the inhabitants of rural villages are more sensitive to higher costs (especially initial costs). Therefore, it may not be possible for poor households to buy comparatively expensive solar PV systems and this may delay them in adopting the technology. Hence, there is a need for local manufacturing of solar PV technology, encouraging local investors to invest in solar-based projects, motivating households toward solar PV technology adoption through a drop in the solar PV prices by either controlling the exchange rate against USD or by providing subsidies for solar PV technology by the government, etc strategies which might help to slump the chronic energy crises.
Data Availability Statement
The raw data supporting the conclusions of this article will be made available by the authors, without undue reservation.
Ethics Statement
Ethical review and approval was not required for this study in accordance with the local legislation and institutional requirements.
Author Contributions
MA: Conceptualization, Data curation, Software, Formal analysis, Methodology, Writing—original draft and review and editing; FA: Conceptualization, Formal analysis, Supervision, Investigation, Writing—original draft and review and editing; YJ: Conceptualization, Supervision, Investigation, Validation, Writing—review and editing; YW: Investigation, Validation, Funding; KI: Writing—review and editing.
Funding
YW acknowledges the funding from SOAS, University of London.
Conflict of Interest
The authors declare that the research was conducted in the absence of any commercial or financial relationships that could be construed as a potential conflict of interest.
Publisher’s Note
All claims expressed in this article are solely those of the authors and do not necessarily represent those of their affiliated organizations, or those of the publisher, the editors and the reviewers. Any product that may be evaluated in this article, or claim that may be made by its manufacturer, is not guaranteed or endorsed by the publisher.
Acknowledgments
We thank the Editor, the Associate Editor, the Subject Editor, the Special Issue Editors, and the reviewers. We also acknowledge the participants of the Asian Economic Community (AEC) Forum, held in South Korea, for their helpful suggestions and comments, specifically Prof. Josef C. Brada (Arizona State University, United States), Prof. Willem Thorbecke (RIETI, Japan), and Prof. Jehoon Park (AEC Foundation and Incheon National University, Korea). All remaining errors are our own.
References
Aarakit, S. M., Ntayi, J. M., Wasswa, F., Adaramola, M. S., and Ssennono, V. F. (2021). Adoption of Solar Photovoltaic Systems in Households: Evidence from Uganda. J. Clean. Prod. 329, 129619. doi:10.1016/j.jclepro.2021.129619
Adesina, A. A., and Baidu‐Forson, J. (1995). Farmers' Perceptions and Adoption of New Agricultural Technology: Evidence from Analysis in Burkina Faso and Guinea, West Africa. Agric. Econ. 13 (1), 1–9. doi:10.1111/j.1574-0862.1995.tb00366.x
Adesina, A. A., Mbila, D., Nkamleu, G. B., and Endamana, D. (2000). Econometric Analysis of the Determinants of Adoption of Alley Farming by Farmers in the Forest Zone of Southwest Cameroon. Agric. Ecosyst. Environ. 80 (3), 255–265. doi:10.1016/s0167-8809(00)00152-3
Adnan, S., Hayat Khan, A., Haider, S., and Mahmood, R. (2012). Solar Energy Potential in Pakistan. J. Renew. Sustain. Energy 4 (3), 032701. doi:10.1063/1.4712051
IEA (2017). “ Energy Access Outlook 2017. From Poverty to Prosperity, IEA: Paris, Available at: https://doi.org/10.1787/9789264285569-en (Accessed Febuary 28, 2022).
Ali, A., Bahadur Rahut, D., and Behera, B. (2016). Factors Influencing Farmers׳ Adoption of Energy-Based Water Pumps and Impacts on Crop Productivity and Household Income in Pakistan. Renew. Sustain. Energy Rev. 54, 48–57. doi:10.1016/j.rser.2015.09.073
Ali, A., Rahut, D. B., Mottaleb, K. A., and Aryal, J. P. (2019). Alternate Energy Sources for Lighting Among Rural Households in the Himalayan Region of Pakistan: Access and Impact. Energy & Environ. 30 (7), 1291–1312. doi:10.1177/0958305x19842960
Ali, F., Jiang, L., and Khan, K. (2017). Feasibility analysis of renewable based hybrid energy system for the remote community in Pakistan. Proceedings of the 2017 IEEE International Conference on Industrial Engineering and Engineering Management (IEEM), 348–348. doi:10.1109/IEEM.2017.8289909
Ali, F., Ahmar, M., Jiang, Y., and AlAhmad, M. (2021). A Techno-Economic Assessment of Hybrid Energy Systems in Rural Pakistan. Energy 215, 119103. doi:10.1016/j.energy.2020.119103
Andadari, R. K., Mulder, P., and Rietveld, P. (2014). Energy Poverty Reduction by Fuel Switching. Impact Evaluation of the LPG Conversion Program in Indonesia. Energy Policy 66, 436–449. doi:10.1016/j.enpol.2013.11.021
Aqeeq, M. A., Hyder, S. I., Shehzad, F., and Tahir, M. A. (2018). On the Competitiveness of Grid-Tied Residential Photovoltaic Generation Systems in Pakistan: Panacea or Paradox? Energy Policy 119, 704–722. doi:10.1016/j.enpol.2018.04.071
Asfaw, S., Di Battista, F., and Lipper, L. (2016). Agricultural Technology Adoption under Climate Change in the Sahel: Micro-evidence from Niger. J. Afr. Econ. 25 (5), 637–669. doi:10.1093/jae/ejw005
Azimoh, C. L., Klintenberg, P., Mbohwa, C., and Wallin, F. (2017). Replicability and Scalability of Mini-Grid Solution to Rural Electrification Programs in Sub-saharan Africa. Renew. Energy 106, 222–231. doi:10.1016/j.renene.2017.01.017
Baloch, M. H., KaloiKaloi, G. S., and Memon, Z. A. (2016). Current Scenario of the Wind Energy in Pakistan Challenges and Future Perspectives: A Case Study. Energy Rep. 2, 201–210. doi:10.1016/j.egyr.2016.08.002
Bashiri, A., and Alizadeh, S. H. (2018). The Analysis of Demographics, Environmental and Knowledge Factors Affecting Prospective Residential PV System Adoption: A Study in Tehran. Renew. Sustain. Energy Rev. 81, 3131–3139. doi:10.1016/j.rser.2017.08.093
Behera, B., Rahut, D. B., Jeetendra, A., and Ali, A. (2015). Household Collection and Use of Biomass Energy Sources in South Asia. Energy 85 (null), 468–480. doi:10.1016/j.energy.2015.03.059
Bekele, W., and Drake, L. (2003). Soil and Water Conservation Decision Behavior of Subsistence Farmers in the Eastern Highlands of Ethiopia: A Case Study of the Hunde-Lafto Area. Ecol. Econ. 46 (3), 437–451. doi:10.1016/s0921-8009(03)00166-6
Bhandari, A. K., and Jana, C. (2010). A Comparative Evaluation of Household Preferences for Solar Photovoltaic Standalone and Mini-Grid System: An Empirical Study in a Costal Village of Indian Sundarban. Renew. Energy 35 (12), 2835–2838. doi:10.1016/j.renene.2010.05.006
Campbell, B. M., Vermeulen, S. J., Mangono, J. J., and Mabugu, R. (2003). The Energy Transition in Action: Urban Domestic Fuel Choices in a Changing Zimbabwe. Energy Policy 31 (6), 553–562. doi:10.1016/S0301-4215(02)00098-8
Carlisle, J. E., KaneKane, S. L., Solan, D., and Joe, J. C. (2014). Support for Solar Energy: Examining Sense of Place and Utility-Scale Development in California. Energy Res. Soc. Sci. 3, 124–130. doi:10.1016/j.erss.2014.07.006
Chowdhury, S., Sumita, U., Islam, A., and Bedja, I. (2014). Importance of Policy for Energy System Transformation: Diffusion of PV Technology in Japan and Germany. Energy Policy 68, 285–293. doi:10.1016/j.enpol.2014.01.023
Das, B. K., Hoque, N., Mandal, S., Pal, T. K., and Raihan, M. A. (2017). A Techno-Economic Feasibility of a Stand-Alone Hybrid Power Generation for Remote Area Application in Bangladesh. Energy 134, 775–788. doi:10.1016/j.energy.2017.06.024
De Groote, O., Pepermans, G., and Verboven, F. (2016). Heterogeneity in the Adoption of Photovoltaic Systems in Flanders. Energy Econ. 59, 45–57. doi:10.1016/j.eneco.2016.07.008
Doner, J. (2007). Barriers to Adoption of Renewable Energy Technology Barriers to Adoption of Renewable Energy Technology Table of Contents. Illinois State University, Institute for Regulatory Policy StudiesAvailable at: https://irps.illinoisstate.edu/downloads/research/documents/IRPSDonerWorkingPaper050707.pdf (Accessed March 23, 2022).
Edwards, Y. D., and Allenby, G. M. (2003). Multivariate Analysis of Multiple Response Data. J. Mark. Res. 40 (3), 321–334. doi:10.1509/jmkr.40.3.321.19233
Gustavsson, M. (2007). Educational Benefits from Solar Technology-Access to Solar Electric Services and Changes in Children's Study Routines, Experiences from Eastern Province Zambia. Energy Policy 35 (2), 1292–1299. doi:10.1016/j.enpol.2006.03.019
Guta, D. D. (2014). Effect of Fuelwood Scarcity and Socio-Economic Factors on Household Bio-Based Energy Use and Energy Substitution in Rural Ethiopia. Energy Policy 75, 217–227. doi:10.1016/j.enpol.2014.09.017
Gwavuya, S. G., Abele, S., Barfuss, I., Zeller, M., and Müller, J. (2012). Household Energy Economics in Rural Ethiopia: A Cost-Benefit Analysis of Biogas Energy. Renew. Energy 48, 202–209. doi:10.1016/j.renene.2012.04.042
Heltberg, R. (2004). Fuel Switching: Evidence from Eight Developing Countries. Energy Econ. 26 (5), 869–887. doi:10.1016/j.eneco.2004.04.018
Hiemstra-Van der Horst, G., and Hovorka, A. J. (2008). Reassessing the "energy Ladder": Household Energy Use in Maun, Botswana. Energy Policy 36 (9), 3333–3344. doi:10.1016/j.enpol.2008.05.006
Iqbal, S., Anwar, S., Akram, W., and Irfan, M. (2013). Factors Leading to Adoption of Biogas Technology: A Case Study of District Faisalabad, Punjab, Pakistan. Int. J. Acad. Res. Bus. Soc. Sci. 3 (11), 571. doi:10.6007/ijarbss/v3-i11/376
Islam, T. (2014). Household Level Innovation Diffusion Model of Photo-Voltaic (PV) Solar Cells from Stated Preference Data. Energy Policy 65, 340–350. doi:10.1016/j.enpol.2013.10.004
IVNM.net (2021). Maps of Pakistan. Available at: http://www.maps-of-the-world.net/maps-of-asia/maps-of-pakistan/.
Jabeen, G., Yan, Q., Ahmad, M., Fatima, N., and Qamar, S. (2019). Consumers' Intention-Based Influence Factors of Renewable Power Generation Technology Utilization: A Structural Equation Modeling Approach. J. Clean. Prod. 237, 117737. doi:10.1016/j.jclepro.2019.117737
Jan, I., Ullah, W., and Ashfaq, M. (2020). Social Acceptability of Solar Photovoltaic System in Pakistan: Key Determinants and Policy Implications. J. Clean. Prod. 274, 123140. doi:10.1016/j.jclepro.2020.123140
Jefferson, M. (2006). Sustainable Energy Development: Performance and Prospects. Renew. Energy 31 (5), 571–582. doi:10.1016/j.renene.2005.09.002
Kabir, H., Yegbemey, R. N., and Bauer, S. (2013). Factors Determinant of Biogas Adoption in Bangladesh. Renew. Sustain. Energy Rev. 28, 881–889. doi:10.1016/j.rser.2013.08.046
Karekezi, S. (2002). Renewables in Africa—Meeting the Energy Needs of the Poor. Energy Policy 30 (11–12), 1059–1069. doi:10.1016/s0301-4215(02)00058-7
Karytsas, S., Polyzou, O., and Karytsas, C. (2019). Factors Affecting Willingness to Adopt and Willingness to Pay for a Residential Hybrid System that Provides Heating/Cooling and Domestic Hot Water. Renew. Energy 142, 591–603. doi:10.1016/j.renene.2019.04.108
Kelebe, H. E., Ayimut, K. M., Berhe, G. H., and Hintsa, K. (2017). Determinants for Adoption Decision of Small Scale Biogas Technology by Rural Households in Tigray, Ethiopia. Energy Econ. 66, 272–278. doi:10.1016/j.eneco.2017.06.022
Khan, S., Rashid, M., Husain, M. A., Rahim, A., and Shah, S. U. (2020). Potential & Current Status of Solar Energy in Pakistan Policy, Planning & Strategy. Ijew 07 (02), 98–108. doi:10.34259/ijew.20.70298108
Kizilcec, V., Parikh, P., and Bisaga, I. (2021). Examining the Journey of a Pay-As-You-Go Solar Home System Customer: A Case Study of Rwanda. Energies 14, 330. doi:10.3390/en14020330
Kurata, M., Matsui, N., Ikemoto, Y., and Tsuboi, H. (2018). Do Determinants of Adopting Solar Home Systems Differ between Households and Micro-enterprises? Evidence from Rural Bangladesh. Renew. Energy 129, 309–316. doi:10.1016/j.renene.2018.06.015
LGCD (2021). The Local Government & Community Development (LG&CD) Department. Punjab Information Technology Board: Punjab. Available at: https://lgcd.punjab.gov.pk/ (Accessed Febuary 28, 2022).
LGKP (2021). Local Government , Elections, and Rural Development Departments. Pakistan: KPK. Available at: https://www.lgkp.gov.pk/districts/.
Li, X., Li, H., and Wang, X. (2013). Farmers' Willingness to Convert Traditional Houses to Solar Houses in Rural Areas: A Survey of 465 Households in Chongqing, China. Energy Policy 63, 882–886. doi:10.1016/j.enpol.2013.09.004
Lodhi, F. S., Rabbani, U., Khan, A. A., Raza, O., Holakouie-Naieni, K., Yaseri, M., et al. (2021). Factors Associated with Quality of Life Among Joint and Nuclear Families: A Population-Based Study. BMC Public Health 21 (1), 234. doi:10.1186/s12889-021-10265-2
Luo, B., Khan, A. A., Ali, M. A. S., and Yu, J. (2021). An Evaluation of Influencing Factors and Public Attitudes for the Adoption of Biogas System in Rural Communities to Overcome Energy Crisis: A Case Study of Pakistan. Sci. Total Environ. 778, 146208. doi:10.1016/j.scitotenv.2021.146208
Masera, O. R., Saatkamp, B. D., and Kammen, D. M. (2000). From Linear Fuel Switching to Multiple Cooking Strategies: A Critique and Alternative to the Energy Ladder Model. World Dev. 28 (12), 2083–2103. doi:10.1016/s0305-750x(00)00076-0
McEachern, M., and Hanson, S. (2008). Socio-Geographic Perception in the Diffusion of Innovation: Solar Energy Technology in Sri Lanka. Energy Policy 36 (7), 2578–2590. doi:10.1016/j.enpol.2008.03.020
Mcfadden, D. (1974). “Conditional Logit Analysis of Qualitative Choice Behaviour,” in Frontiers in Econometrics. Editor P. Zarembka (Academic Press), 1, 105–142.
Mengistu, M. G., Simane, B., Eshete, G., and Workneh, T. S. (2016). Factors Affecting Households' Decisions in Biogas Technology Adoption, the Case of Ofla and Mecha Districts, Northern Ethiopia. Renew. Energy 93, 215–227. doi:10.1016/j.renene.2016.02.066
Michelsen, C. C., and Madlener, R. (2012). Homeowners' Preferences for Adopting Innovative Residential Heating Systems: A Discrete Choice Analysis for Germany. Energy Econ. 34 (5), 1271–1283. doi:10.1016/j.eneco.2012.06.009
Mukisa, N., Manitisa, M. S., Nduhuura, P., Tugume, E., and Chalwe, C. K. (2022). Solar Home Systems Adoption in Sub-saharan African Countries: Household Economic and Environmental Benefits Assessment. Renew. Energy 189, 836–852. doi:10.1016/j.renene.2022.03.029
Muller, C., and Yan, H. (2018). Household Fuel Use in Developing Countries: Review of Theory and Evidence. Energy Econ. 70, 429–439. doi:10.1016/j.eneco.2018.01.024
Mwirigi, J., Balana, B. B., Mugisha, J., Walekhwa, P., Melamu, R., Nakami, S., et al. (2014). Socio-Economic Hurdles to Widespread Adoption of Small-Scale Biogas Digesters in Sub-saharan Africa: A Review. Biomass Bioenergy 70, 17–25. doi:10.1016/j.biombioe.2014.02.018
NTDC (2021). NTDC. Daily Operational Energy Data. Available at: http://www.ntdc.com.pk/doed.php.
Palm, A., Lantz, B., Abdul-Rahim, A. S., Shahwahid Othman, M., Radzi, M. A. M., Hizam, H., et al. (2020). Information Dissemination and Residential Solar PV Adoption Rates: The Effect of an Information Campaign in Sweden. Energy Policy 142 (3), 111540. doi:10.1016/j.enpol.2020.111540
Palm, A. (2017). Peer Effects in Residential Solar Photovoltaics Adoption-A Mixed Methods Study of Swedish Users. Energy Res. Soc. Sci. 26, 1–10. doi:10.1016/j.erss.2017.01.008
PBS (2021). Pakistan Bureau of Statistics - PBS. Available at: https://www.pbs.gov.pk/population-tables.
Peng, J., and Lu, L. (2013). Investigation on the Development Potential of Rooftop PV System in Hong Kong and its Environmental Benefits. Renew. Sustain. Energy Rev. 27, 149–162. doi:10.1016/j.rser.2013.06.030
Powell, J. W., Welsh, J. M., Pannell, D., and Kingwell, R. (2021). Factors Influencing Australian Sugarcane Irrigators' Adoption of Solar Photovoltaic Systems for Water Pumping. Clean. Eng. Technol. 4, 100248. doi:10.1016/j.clet.2021.100248
Qureshi, T. M., Ullah, K., and Arentsen, M. J. (2017). Factors Responsible for Solar PV Adoption at Household Level: A Case of Lahore, Pakistan. Renew. Sustain. Energy Rev. 78, 754–763. doi:10.1016/j.rser.2017.04.020
Rahut, D. B., Mottaleb, K. A., Ali, A., and Aryal, J. (2018). The Use and Determinants of Solar Energy by Sub-saharan African Households. Int. J. Sustain. Energy 37 (8), 718–735. doi:10.1080/14786451.2017.1323897
Rai, V., Reeves, D. C., and Margolis, R. (2016). Overcoming Barriers and Uncertainties in the Adoption of Residential Solar PV. Renew. Energy 89, 498–505. doi:10.1016/j.renene.2015.11.080
Rauf, O., Wang, S., Yuan, P., and Tan, J. (2015). An Overview of Energy Status and Development in Pakistan. Renew. Sustain. Energy Rev. 48, 892–931. doi:10.1016/j.rser.2015.04.012
Scarpa, R., and Willis, K. (2010). Willingness-to-pay for Renewable Energy: Primary and Discretionary Choice of British Households' for Micro-generation Technologies. Energy Econ. 32 (1), 129–136. doi:10.1016/j.eneco.2009.06.004
Sharma, R., Choudhary, D., Kumar, P., Venkateswaran, J., and Solanki, C. S. (2019). Do Solar Study Lamps Help Children Study at Night? Evidence from Rural India. Energy Sustain. Dev. 50, 109–116. doi:10.1016/j.esd.2019.03.005
Sheikh, M. A. (2010). Energy and Renewable Energy Scenario of Pakistan. Renew. Sustain. Energy Rev. 14 (1), 354–363. doi:10.1016/j.rser.2009.07.037
Smith, M. G., and Urpelainen, J. (2014). Early Adopters of Solar Panels in Developing Countries: Evidence from Tanzania. Rev. Policy Res. 31 (1), 17–37. doi:10.1111/ropr.12061
Somda, J., Joseph Nianogo, A., Nassa, S., and Sanou, S. (2002). Soil Fertility Management and Socio-Economic Factors in Crop-Livestock Systems in Burkina Faso: A Case Study of Composting Technology. Ecol. Econ. 43 (2–3), 175–183. doi:10.1016/s0921-8009(02)00208-2
Sommerfeld, J., Buys, L., and Vine, D. (2017). Residential Consumers' Experiences in the Adoption and Use of Solar PV. Energy Policy 105, 10–16. doi:10.1016/j.enpol.2017.02.021
Swider, D. J., Beurskens, L., Davidson, S., Twidell, J., Pyrko, J., Prüggler, W., et al. (2008). Conditions and Costs for Renewables Electricity Grid Connection: Examples in Europe. Renew. Energy 33 (8), 1832–1842. doi:10.1016/j.renene.2007.11.005
Tareen, W. U. K., Anjum, Z., Yasin, N., Siddiqui, L., Farhat, I., Malik, S. A., et al. (2018). The Prospective Non-conventional Alternate and Renewable Energy Sources in Pakistan-A Focus on Biomass Energy for Power Generation, Transportation, and Industrial Fuel. Energies 11 (9), 2431. doi:10.3390/en11092431
Urpelainen, J., and Yoon, S. (2015). Solar Home Systems for Rural India: Survey Evidence on Awareness and Willingness to Pay from Uttar Pradesh. Energy Sustain. Dev. 24, 70–78. doi:10.1016/j.esd.2014.10.005
Vasseur, V., and Kemp, R. (2015). The Adoption of PV in the Netherlands: A Statistical Analysis of Adoption Factors. Renew. Sustain. Energy Rev. 41, 483–494. doi:10.1016/j.rser.2014.08.020
Vu, D. H., Muttaqi, K. M., and Agalgaonkar, A. P. (2015). A Variance Inflation Factor and Backward Elimination Based Robust Regression Model for Forecasting Monthly Electricity Demand Using Climatic Variables. Appl. Energy 140, 385–394. doi:10.1016/j.apenergy.2014.12.011
Walekhwa, P. N., Mugisha, J., and Drake, L. (2009). Biogas Energy from Family-Sized Digesters in Uganda: Critical Factors and Policy Implications. Energy Policy 37 (7), 2754–2762. doi:10.1016/j.enpol.2009.03.018
Wassie, Y. T., Rannestad, M. M., and AdaramolaAdaramola, M. S. (2021). Determinants of Household Energy Choices in Rural Sub-saharan Africa: An Example from Southern Ethiopia. Energy 221, 119785. doi:10.1016/j.energy.2021.119785
Keywords: solar photovoltaic, determinants of adoption, multivariate probit model, binary logit model, rural households, Pakistan
Citation: Ahmar M, Ali F, Jiang Y, Wang Y and Iqbal K (2022) Determinants of Adoption and the Type of Solar PV Technology Adopted in Rural Pakistan. Front. Environ. Sci. 10:895622. doi: 10.3389/fenvs.2022.895622
Received: 14 March 2022; Accepted: 30 March 2022;
Published: 19 May 2022.
Edited by:
Munir Ahmad, Zhejiang University, ChinaReviewed by:
Gul Jabeen, Shenzhen University, ChinaHaitao Wu, Beijing Institute of Technology, China
Copyright © 2022 Ahmar, Ali, Jiang, Wang and Iqbal. This is an open-access article distributed under the terms of the Creative Commons Attribution License (CC BY). The use, distribution or reproduction in other forums is permitted, provided the original author(s) and the copyright owner(s) are credited and that the original publication in this journal is cited, in accordance with accepted academic practice. No use, distribution or reproduction is permitted which does not comply with these terms.
*Correspondence: Yichu Wang, d2FuZ3lpY2h1MTk5M0AxNjMuY29t
†These authors have contributed equally to this work and share first authorship