- 1Jiangsu Key Laboratory of Atmospheric Environment Monitoring and Pollution Control, Collaborative Innovation Center of Atmospheric Environment and Equipment Technology, Nanjing University of Information Science and Technology, Nanjing, China
- 2Tongxiang Meteorological Bureau, Jiaxing, China
- 3California Air Resources Board, Sacramento, CA, United States
This study utilizes the Weather Research and Forecasting model with a higher resolution (36 km × 36 km) to dynamically downscale the Community Earth System Model results forced by the three representative concentration pathways (RCP) scenarios (RCP4.5, RCP6.0, and RCP8.5) over China. The goal was to compare meteorological fields during the present (2006–2015) and future (2046–2055) climatological periods. An appropriate air stagnation judgment index was selected to explore the effect of climate change on air quality-related meteorological conditions. The results show that the occurrence of wintertime air stagnation over China in the middle of this century (2046–2055) will reduce slightly, with the largest reduction projected under the RCP8.5 scenario (−4 times). However, long-lasting air stagnation events (ASE) are projected to increase in the future, and this increasing trend is more obvious under the RCP8.5 scenario. The projected increase in the long-lasting ASE in different regions of China ranges from 3 to 11 times. Among these, Central China has the largest increase, followed by East and Northeast China, while South China has the lowest increase in ASE. Our results indicate that more attention should be dedicated to extreme pollution events that may potentially be caused by long-lasting air stagnation events in the future.
1 Introduction
With the rapid development of the Chinese economy and the accelerated process of industrialization, air pollution events of long durations and high concentrations occur frequently over China (Horton et al., 2012; Wang et al., 2019; Qin et al., 2021). The Chinese government has actively taken preventive measures to strictly control air pollutant emissions nationwide. Although the annual mean PM2.5 (fine particle matter with aerodynamic diameter ≤2.5 μm) concentrations have continued to decrease in recent years, severe haze pollution events still occur frequently during the winters, especially in North China (Chen and Wang, 2015; Sun et al., 2019). Therefore, in addition to strictly controlling emission sources, other factors, such as meteorological conditions, need to be considered to improve air quality. The interactions between climate change and air pollution have been hot topics in recent years. Climate and weather conditions strongly influence the spatial and temporal distribution of air pollutants concentrations (Kinney, 2018). When emission sources are relatively stable, diffusion, transmission, and transformation of atmospheric pollutants in a given region majorly depend on local meteorological conditions (Shi et al., 2020). The impact of meteorological conditions on the concentration of air pollutants can vary dozens of times (Zhang et al., 2010). Therefore, to improve air quality, it is not only necessary to control emission sources, but also to combine meteorological conditions and climate change.
A factor that causes deterioration in air quality is atmospheric stagnation under high-pressure systems and the resultant weak near-surface winds, compounded by stable vertical temperature profiles (inversion) (Lee et al., 2020). In addition to intensive emissions, stagnant meteorological conditions, characterized by slow winds, strong inversion, and shallow boundary layer, are key to the formation of severe haze pollution events (Mu and Liao, 2014; Zhang et al., 2014; Zhang et al., 2015; Cai et al., 2017; Wang et al., 2018; Xu et al., 2020). Many studies have assessed the association between air stagnation and haze pollution in China (Zhao et al., 2013; Wang et al., 2014; Zhang et al., 2014; Cai et al., 2017; Jing et al., 2017; Liao et al., 2018; Wang et al., 2018). Previous research has shown that a lower boundary layer creates covered pot-like conditions, limiting the vertical diffusion of pollutants (Yang et al., 2021). The weakening effect of quiet wind on the horizontal diffusion capacity of the atmosphere makes it difficult for pollutants to diffuse (Rigby and Toumi, 2008; Fu et al., 2014). The continuous absence of precipitation also reduces the wet deposition of pollutants, thereby deteriorating air quality (Guo et al., 2016). Extreme pollution events are thus often caused by long-lasting stagnation conditions (Cai et al., 2017).
To objectively measure stagnation conditions and their impacts on air pollution, several air stagnation indices (ASI) were proposed (Horton et al., 2012; Mu and Liao, 2014; Zhang et al., 2014; Huang et al., 2018; Li et al., 2020). The most used ASI was proposed by Horton et al. (2012), which is based on the wind speed at 10 m (WS10) and 500 hPa, while considering total precipitation (PCP). Collectively, these parameters are used as indicators of horizontal atmospheric dispersion capacity and wet deposition, respectively. However, this index has a weak correspondence with pollutants such as PM2.5 and O3 (Li et al., 2014; Kerr and Waugh, 2018; Garrido-Perez et al., 2019). Huang et al. (2018) replaced the 10 m and 500 hPa wind velocity fields with wind flux in the boundary layer and added the available potential energy based on the convective available potential energy (CAPE) and convective inhibition (CIN). Wang et al. (2018) used atmospheric planetary boundary layer height (PBLH) instead of 500 hPa wind speed to characterize the vertical mixing of pollutants. Garrido-Perez et al. (2021) compared the performance of the three ASIs (Horton et al., 2012; Huang et al., 2018; Wang et al., 2018) and found that all three revealed similar spatial patterns in the stagnation frequency. Furthermore, the response of PM10 concentrations to stagnation varied with ASI and depended on location and season. The main exceptions occurred in coastal areas, where the ASI defined by Wang et al. (2018) seemed to be more accurate than the other ASIs.
Climate change will lead to changes in air stagnation conditions, and global warming may increase the static stability of the lower troposphere by reducing near-surface wind speed, which means air stagnation events (ASE) will be more frequent in the future (Lee et al., 2020). Horton et al. (2012) reported that highly industrialized areas, such as eastern China, are more sensitive to climate warming and the incidence of air stagnation in such regions was expected to increase by 12%–25% in the late-21st century (2081–2100) as compared to the late-20th century (1981–2000). Wang et al. (2018) calculated ASI from observational data to find strong air stagnation conditions from autumn to winter over China, especially in the Sichuan basin and the Beijing-Tianjin-Hebei (BTH) region (more than 40% of the whole year).
The Global Climate Model (GCM) has been used widely in previous studies for climate change projections (Hewitson and Crane, 2006). However, the spatial resolution of GCMs is coarse and they cannot predict regional-scale weather patterns well, and thus, are not suitable for high-resolution regional climate, air quality, and health impact research. Dynamical downscaling is a kind of downscaling technique that converts coarse resolution outputs from GCM to fine spatial resolution by developing regional climate models (Lee et al., 2014). This study uses climate projections from the Community Earth System Model (CESM) provided by the Coupled Model Intercomparision Project 5 (CMIP5) to obtain initial and boundary conditions for the Weather Research and Forecasting (WRF) model. We have generated regional-scale meteorological fields for the present (2006–2015) and future (2046–2055) climatological periods under the three RCP scenarios (RCP4.5, RCP6.0, and RCP8.5). As mentioned above, we choose the ASI defined by Wang et al. (2018) to calculate atmospheric stagnation in this study and explore the impacts of climate change on air stagnation. This paper is organized as follows. Section 2 describes the data and methods used in this study, while the results are presented and discussed in Section 3. Section 4 comprises a summary and conclusions.
2 Methods
2.1 Data Description and Experimental Setup
Global bias-corrected data produced by CESM version 1 that participated in the CMIP5 was obtained from the website of the Research Data Archive (https://rda.ucar.edu/datasets/ds316.1/ #!description). The dataset was provided in the Intermediate File Format specific to WRF and contained all the variables needed for the initial and boundary conditions for WRF simulations. This dataset had already been bias-corrected using the European Centre for Medium-Range Weather Forecasts (ECMWF) Interim Reanalysis (ERA-Interim) fields for 1981–2005, following the method of Bruyère et al. (2014). All the variables had 26 pressure levels and were provided in files at 6-hourly intervals. Files were available for a simulation for the present (1951–2005) and three future scenarios (RCP4.5, RCP6.0, and RCP8.5) spanning 2006–2100.
We ran the WRF model to generate hourly meteorological fields of the “Basecase” (2006–2015) and the three RCP cases (RCP4.5, RCP6.0, RCP8.5) for the winter months (December, January, and February) of 2046–2055. The simulation domain covered the entirety of China with a spatial resolution of 36 km × 36 km (Figure 1). The physical options used to drive the WRF simulations are listed in Table 1 (Hu et al., 2016), including the new Thompson microphysics scheme (Thompson et al., 2008), Rapid Radiative Transfer Model (RRTM) longwave radiation (Mlawer et al., 1997), and Yonsei University PBL parameterization (Hong et al., 2006). Due to corrupted data caused by a modelling bug in CESM, bias-corrected data was unavailable for RCP2.6. Thus, we chose three scenarios (RCP4.5, RCP6.0, and RCP8.5), spanning the winter of 2046–2055, to drive WRF v3.8 for future analyses. Results from the CESM model using the RCP6.0 emission scenario were used as the meteorological boundary and initial fields of the WRF model for the 2006–2015 periods. The WRF model was configured with 44 vertical layers from surface to 50 hPa (Supplementary Figure S1). Because of the vast territory of China, climate and air quality vary in the different regions. To better compare and summarize changes in different regions, we divided China into seven regions based on differences in climate and administration (Figure 1), including East China (E), North China (N), Central China (C), South China (S), Southwest China (SW), Northwest China (NW), and Northeast China (NE).
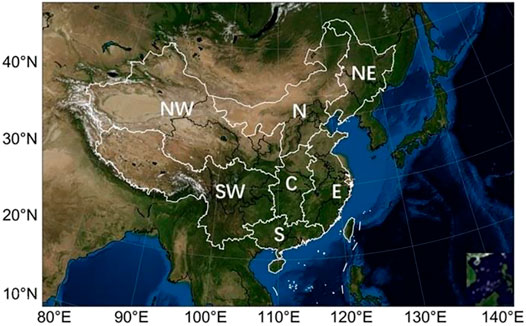
FIGURE 1. Model domain and sub-regions used for analysis. E: East China, N: North China, C: Central China, S: South China, SW: Southwest China, NW: Northwest China, NE: Northeast China.
2.2 Air Stagnation Days and Events
We adopt the ASI definition proposed by Wang et al. (2018), which is shown in Eq. 1. This parameterization is derived using PBLH and WS10 data to fit the normalized daily PM2.5 concentrations during the winter. This could better explain haze events and has been used in other studies (Zhang et al., 2019; Gao et al., 2020). For each grid cell (i, j), if the daily cumulative precipitation is <1 mm and the daily average PBLH and WS10 satisfy Eq. 1, the air is considered stagnant and the given day is considered to be an air stagnation day (ASD). We define an ASE as an event in which air stagnation lasts for 3 days or more.
2.3 Model Performance Evaluation
Evaluation of model performance is an important step to verify the reliability of modelling results and to establish confidence for further application. The WRF simulations were validated using the fifth-generation European Centre for Medium-Range Weather Forecasts (ECMWF) reanalysis data for global climate and weather (ERA5). Monthly averaged data (Hersbach et al., 2019) from ERA5 for 2006 to 2015 were obtained from the website https://cds.climate.copernicus.eu/cdsapp#!/dataset/. In this study, statistical metrics and benchmarks recommended by the United States EPA were used. The normalized mean deviation (NMB), normalized mean error (NME), and the Pearson correlation coefficient (R) of the observed and simulated values were calculated to quantitatively evaluate model performance (Emery et al., 2017). The corresponding equations are shown below:
3 Results and Discussion
3.1 Evaluation of Present Climatological Simulation
Figure 2 shows the spatial distribution of wintertime (DJF) meteorological fields from the Basecase and the corresponding ERA5 reanalysis data. Detailed statistical metrics are listed in Table 2. Note that PBLH, WS10, and T2 were averaged from 2006 to 2015, while PCP was calculated as the average of cumulative winter precipitation. As can be seen from Figure 2, the simulated PBLH in the Tibetan Plateau and its surrounding areas was significantly underestimated, which might be due to the complex terrain in the region (Wang et al., 2021). Combined with Table 2, the mean PBLH within the model domain was 490.4 m in the ERA5 data, while it was 477.92 m according to WRF. The WRF model underestimated PBLH with a mean bias of −12.48 m, while the RMSE was as large as 172.22 m. This indicated that model performances may vary a lot across the different regions. Nevertheless, the simulated PBLH showed a strong positive correlation of 0.84 with ERA5 data, suggesting that the overall model performance was acceptable. The WRF model successfully captured the general pattern of PCP as compared to the ERA5 data, but underestimated PCP in southern China. Previous studies have reported that ERA5 precipitation data are relatively higher than the ground observations over China in winters, with a mean bias of 35% (Jiang et al., 2021; Jiao et al., 2021), which partly explains the discrepancy between WRF simulations and ERA5 data. A comparison of the simulated PCP with the Tropical Rainfall Measuring Mission (TRMM) satellite data for 2006–2015 (Supplementary Figure S2) revealed that the WRF-simulated PCP had similar spatial patterns to that of TRMM data. The WRF-simulated mean PCP within the model domain was 15.0 mm, which was close to that of TRMM data (15.5 mm), giving us confidence in the simulated PCP.

TABLE 2. Statistical parameters for simulated meteorology at all grid cells within the model domain.
The WS10 and T2 (the temperature at 2 m) predicted by WRF showed relatively consistent spatial patterns with those from ERA5 data. WS10 was slightly overestimated with a mean bias of 0.95 m/s. The ME and RMSE of WS10 were both less than 2 m/s, and thus, met the performance criteria suggested by Emery et al. (2001). The model performance for T2 was the best among the selected meteorological factors with correlation coefficients as high as 0.99. To explore the differences in modelling results before and after downscaling, we compared the CESM output with ERA5 data and WRF simulations (Supplementary Figure S3; Supplementary Table S1). Because bias-corrected CESM output does not provide PBLH and PCP (Bruyère et al., 2014; Bruyère et al., 2015), only projected WS10 and T2 obtained. As Supplementary Figure S3 shows, both WRF and CESM exhibited good spatial agreement with the ERA5 data. As compared to CESM, WRF could reproduce more regional scale details for both WS10 and T2, especially in the Tibetan Plateau and the Sichuan Basin. Previous studies have indicated that CESM cannot capture topography-induced temperature patterns (Liu et al., 2013; Chen et al., 2018), which is consistent with our results. The biases between CESM and ERA5 were 1.07 m/s and 0.95 K for WS10 and T2, respectively, which was slightly larger than those between WRF and ERA5.
3.2 Changes in Meteorological Conditions Under Different Scenarios
The decadal mean values of PBLH, WS10, T2, and PCP under the three RCP scenarios were calculated and compared with the Basecase to obtain their spatial and temporal variations due to climate change. Note that PCP was calculated as the average cumulative winter precipitation. Figure 3 shows the WRF-projected changes in PBLH, WS10, T2, and PCP under RCP4.5, RCP6.0, and RCP8.5 scenarios relative to the present period; relative changes in these parameters in the different regions are depicted in Figure 4. Wintertime PBLH at the national scale was projected to increase for the 2046–2055 period under the three RCP scenarios. The increase in PBLH was more obvious for the RCP6.0 scenario (15.1 m), followed by RCP8.5 (6.4 m) and RCP4.5 (2.1 m). A significant increase mainly occurred in the Tibetan Plateau and some parts of North and Northeast China under the three RCP scenarios. For Central, East, and South China, the WRF-RCP4.5 and WRF-RCP8.5 projected generally shallow PBLH, while WRF-RCP6.0 showed a weak increase.
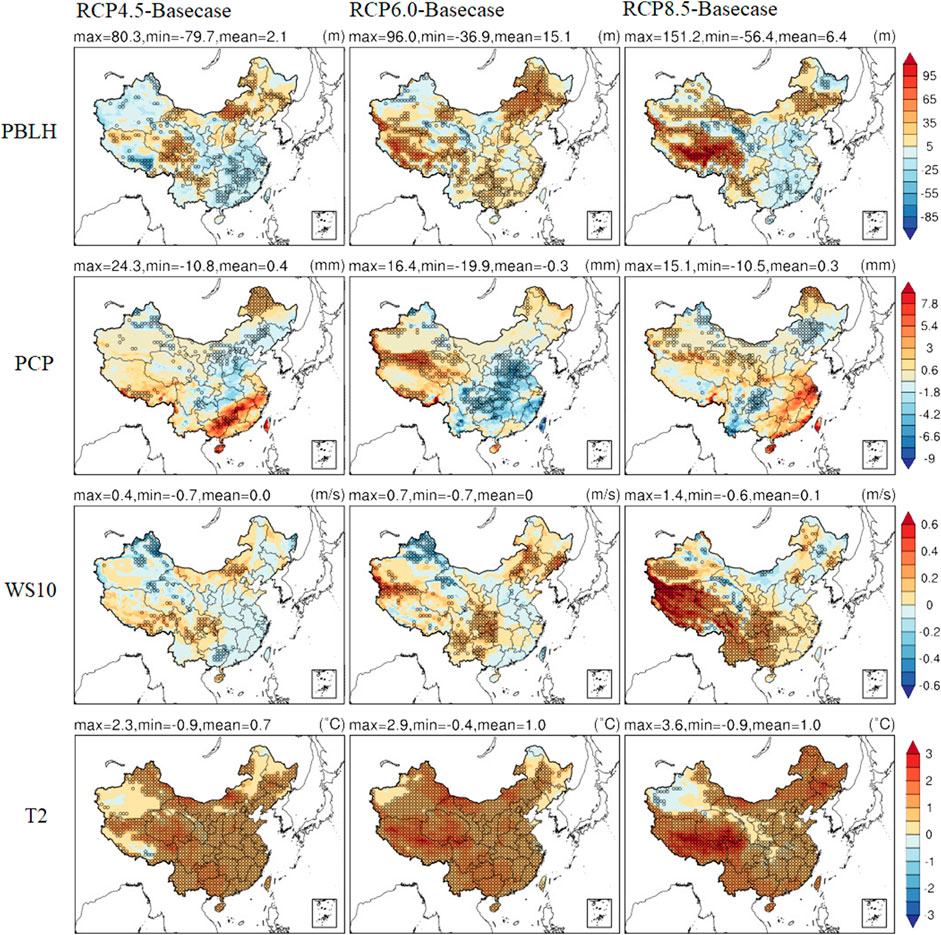
FIGURE 3. Spatial distributions of the projected changes in meteorological fields for the 2046–2055 period relative to the 2006–2015 period. Significant changes (confidence level of 95%) are marked with circles.
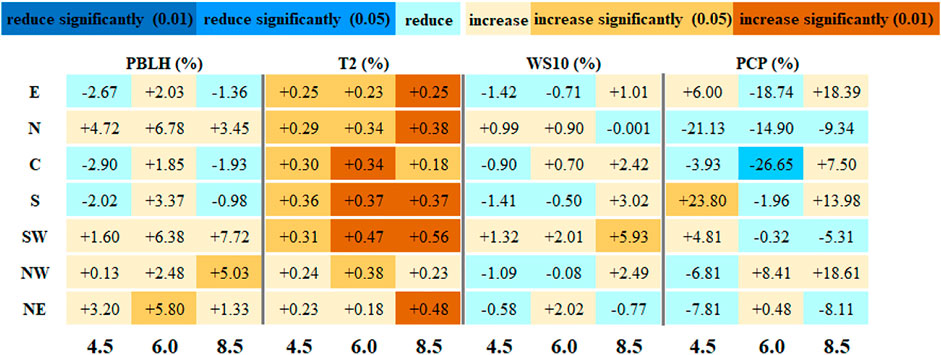
FIGURE 4. The relative changes (2046–2055 relative to 2006–2015) in meteorological fields for different regions of China. Statistical significance is marked with color.
The projected changes in PCP in China were more varied among different scenarios. The WRF-RCP4.5 projected wetter conditions in South and East China and slightly drier conditions in Central, North, and Northeast China for the 2046–2055 period. Under RCP6.0, PCP showed a decreasing trend in most of China except Northwest and Northeast China. A significant decrease was projected for Central China with a regional mean of 26.65% (−14.13 mm). Under RCP8.5, however, wetter conditions were projected for Central, East, and South China, with a maximum increase of 23.80% (15.1 mm). Significant decreases were found for Southwest and Northeast China, with the regional mean value changes of −2.35 and −1.38 mm, respectively. The PCP in North China showed a consistent decreasing trend under the three RCP scenarios, however, the changes were different for other regions.
For WS10, the national mean value for the 2046–2055 period remained largely unchanged relative to the present 2006–2015 period under the RCP4.5 and RCP6.0 scenarios, while it increased slightly under RCP8.5. The projected WS10 showed an increasing trend under all three scenarios over Southwest China, while the largest increase was found under RCP8.5 (5.93%). For other regions, the projected changes in WS10 were less than 2.02% under the RCP4.5 and RCP6.0 scenarios. As can be seen from Figure 3, the projected wintertime temperatures showed a significantly increasing trend over China under all three scenarios. Due to the highest anthropogenic greenhouse gas emissions in RCP8.5, the projected T2 showed the greatest increase, followed by RCP6.0 and RCP4.5. The projected changes in temperature presented obvious regional differences. The largest warming was found in the Tibetan Plateau, with a maximum of 3.6°C increase in average temperature under RCP8.5. The regional mean relative changes in T2 ranged from 0.18% to 0.56%, and the maximum occurred in Southwest China (0.56%).
The CESM-projected WS10 and T2 distributions over China under the three scenarios for the 2046–2055 period were also compared with distributions for the 2006–2015 period (Supplementary Figures S4, S5). Generally, there are no obvious differences between the CESM and WRF projections. The WRF-projected mean values of changes in WS10 and T2 under the three scenarios were comparable to those obtained from CESM; however, the latter covered a wider range, which is attributable to the finer spatial resolution of WRF that allowed it to better capture regional-scale climate patterns. As compared to the CESM simulations, the WRF projections showed a decrease in WS10 in South and Northeast China under the RCP6.0 and RCP8.5 scenarios.
3.3 Changes in Air Stagnation Under Different Scenarios
The spatial distributions of ASD and ASE in the Basecase and their changes under the three RCPs over China are shown in Figure 5. The projected total ASD during the 2006–2015 period was 167 days, while the changes during the 2046–2055 period under the three RCPs aere 6 days (RCP4.5), −9 days (RCP6.0), and −7 days (RCP8.5), respectively. The maximum (minimum) changes projected were 55 (−43), 37 (−83), and 49 (−68) days under RCP4.5, RCP6.0, and RCP8.5, respectively. Under the RCP4.5 scenario, the projected number of ASD increased in most of China, while it decreased significantly in Northwest, Southwest, and Northwest China under the RCP6.0 and RCP8.5 scenarios. Significant reductions in the number of ASD were also found in Sichuan Basin under RCP8.5, with a minimum of about −68 days. The projected ASE exhibited an increasing trend from south to north during the 2006–2015 period, with a national mean value of 56.9. The changes in ASE under the three scenarios showed similar spatial distributions to those of ASD. Positive changes were mainly projected for East and Central China, and the eastern part of Northwest and Southwest China under RCP4.5. Negative changes were found in most of China under the RCP6.0 and RCP8.5 scenarios. At the national scale, the projected ASE decreased in the future for the three scenarios, with the maximum decrease occurring in RCP8.5 (−4.0), followed by RCP6.0 (−2.9) and RCP4.5 (−0.5).
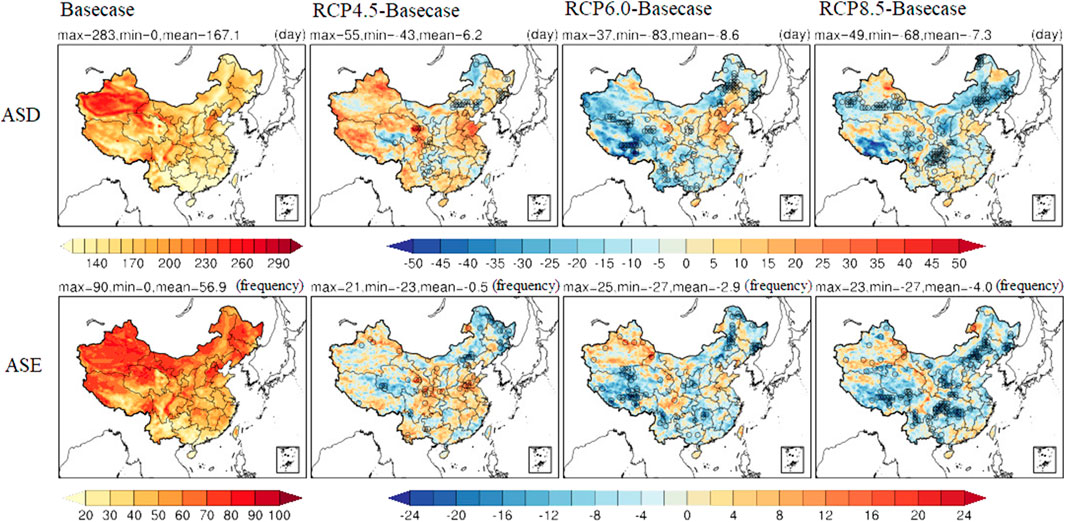
FIGURE 5. Spatial distribution of atmospheric stagnation days, (ASD) and air stagnation events (ASE) in Basecase and their changes under the three RCPs over China. Significant changes (confidence level of 95%) are marked with circles.
The probability distribution of ASE and the changes in ASE over the 2046–2055 period relative to 2006–2015 was calculated using the total ASE during the winter of the 10 years for each grid cell in China; the distribution is shown in Figure 6 to identify the changes of air stagnation under the different scenarios. The numbers of ASE occurrence in China during the winter of the 10 years were mainly concentrated in 30–75 events (for points with a frequency greater than 1%). The projected frequency of ASE occurrence exhibited a bimodal distribution, with a major peak at around 65–75 times and a minor peak at around 40–55 times. Both the peaks shifted to a less frequent ASE under the three RCP scenarios, which means that the number of wintertime ASE occurrences in China may decrease in the future. As can be seen from Figure 6B, the changes in ASE in the 2046–2055 period relative to the 2006–2015 period follow a normal distribution under the three RCP scenarios. The mean changes of winter ASE are −1, −3, and −4 times for RCP4.5, RCP6.0, and RCP8.5, respectively. This is related to the fact that the mean value of PBLH shows an increasing trend in the future, while the mean values of WS10 and PCP change slightly.
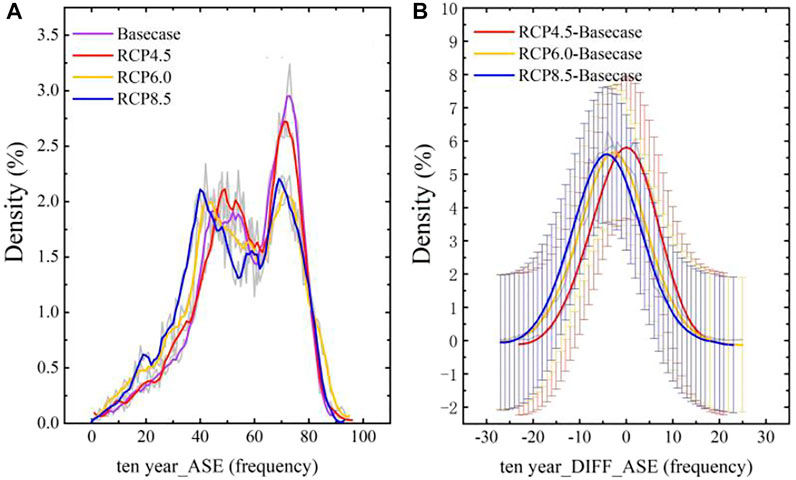
FIGURE 6. Probability distribution of ASE (A) and ASE difference (2046–2055 relative to 2006–2015) (B).
Long-lasting air stagnation events can increase the risk of public exposure to high concentrations of air pollutants. Figure 7 shows the relative frequency distribution of the duration of ASD under the different scenarios for all grid cells over China. For example, assuming that an ASE would last for 3 days, the occurrence under different scenarios was in the order of Basecase (28%) > RCP6.0 (26%) > RCP8.5 (23%) > RCP4.5 (22%). As can be seen from Figure 7, the longest duration of winter ASE in China was projected to be 14 days. The frequency of ASE lasting 2–4 days was the highest (27%–29%) in the Basecase, but with the increase in duration, the proportion of Basecase gradually decreased. The proportion of the RCP scenario gradually increases, especially under the RCP8.5 scenario, which means that the probability of the occurrence of long-lasting ASE will increase in the future.
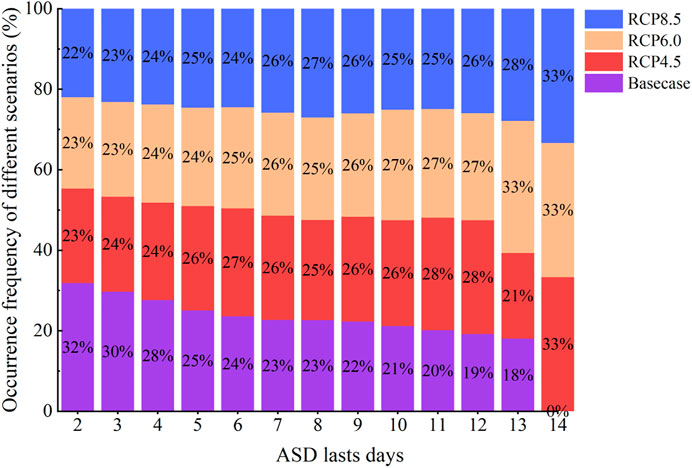
FIGURE 7. The probability distribution of the number of days that ASE lasted under different representative concentration pathway, (RCP) scenarios.
The changes in ASE lasted for more than 5 days for the 2046–2055 period relative to the present 2006–2015 period in different regions are given in Figure 8. Generally, the occurrence of long-lasting ASE in different regions of China increased by 3–11 times in the mid-century decade. The largest increase was projected unser the RCP4.5 scenario for most regions. Central China was found to have the largest increase among the different regions, followed by East China and Northeast China. The lowest increase was projected for South China and was majorly ascribed to a significant increase in PCP.
4 Discussion
Our results indicate that long-lasting ASE will increase in the mid-century winter (2046–2055) over China due to climate change. Zou et al. (2017) projected that the forcing effect of arctic sea ice will cause more extreme atmospheric static stability in China. Using CMIP5 single-forcing experiments, Lee et al. (2020) suggested that global warming caused by anthropogenic greenhouse gases has likely increased the static stability of the lower troposphere, implying more stagnant conditions in the future. Huang et al. (2017) assessed changes in China’s atmospheric stagnation from 1985 to 2014 and reported an upward trend. Based on a multi-model ensemble analysis under RCP8.5, Gao et al. (2020) found that by the end of this century, climate change may lead to an increase in both the duration and frequency of wintertime stagnation events over the North China Plain. Our results are consistent with the above-mentioned studies, providing confidence in our in-depth research on future projections.
This study used a dynamic downscaling method to generate high-resolution regional climate projects based on the outputs from the GCM. Such methods can provide more detailed information about regional or local synoptic patterns as compared to CESM data (Supplementary Figure S3). The results from the dynamic downscaling method predicted air stagnation in various regions over China more suitably. However, results also depend on the choice of ASI as different ASIs may lead to different conclusion for different regions (Garrido-Perez et al., 2021). The ASI used in this study was based on empirical statistical analysis methods (Wang et al., 2018). To compare the differences between ASIs, we calculated the ASI based on atmospheric dynamic methods (Feng et al., 2018). The results are shown in Supplementary Figure S7. The projected ASI exhibited an increasing trend under RCP4.5, while it decreased under RCP6.0 and RCP8.5, which is consistent with changes in the projected ASD (Figure 5).
Note that only one model is used in this study, although use of multiple model sets may be more representative. In future work, we will evaluate the results of other models to make our findings more representative. In addition, the accuracy of the simulations also influences the credibility of the projections of air stagnation but considering that we have used data from 10 years, we believe that trends delineated in our results will not be affected. Moreover, due to limitations in computation and storage resources, nested simulations were not conducted in our study, such simulations could provide finer spatial resolution. In our follow-up study, we aim to apply the nested WRF simulations with a 12- or 4-km horizontal resolution for key regions, such as the North China Plain and the Yangtze River Delta.
5 Conclusion
This study uses future climate projections under three RCP scenarios (RCP4.5, RCP6.0, and RCP8.5) provided by the CESM model based on CMIP5 to drive the WRF model to obtain regional climate projections with a higher resolution (36 km × 36 km) over China. The ERA5 reanalysis data were used to evaluate the performance of WRF projections. The results indicate that the dynamic downscaling method used in this study can generate reasonable regional climate processes. The simulated WS10 and T2 were in good agreement with the ERA5 data. However, PBLH and PCP were underestimated in the Tibetan Plateau and South China, respectively. According to our projections, the occurrence of wintertime ASE will reduce slightly in the future, with the largest reduction (−4 times) expected under the RCP8.5 scenario. Nonetheless, the occurrence of long-lasting ASE will increase in the future, where the increasing trend is more obvious under the RCP8.5 scenario. The projected increase in long-lasting ASE ranged from 3 to 11 times in the different regions, among which Central China had the largest increase, followed by East and Northeast China. The increase in ASE frequency was projected to be the lowest in South China, which is likely attributed to a significant increase in PCP. Our results suggest that atmospheric stagnation in Central and East China should be given more attention in future research and analysis.
Data Availability Statement
The original contributions presented in the study are included in the article/Supplementary Material, further inquiries can be directed to the corresponding author.
Author Contributions
AH, XX, and JH designed the research. AH wrote the first draft of the manuscript. XX and JH reviewed and modified the manuscript. KG and YH analyzed the results. ZZ downloaded CMIP5 data and set up models. All authors contributed to manuscript revision, and read and approved the submitted version.
Funding
This work was supported by the National Key R&D Program of China (2019YFA0606802), the National Natural Science Foundation of China (41975162, 42021004), the Jiangsu Environmental Protection Research Project (2016015).
Conflict of Interest
The authors declare that the research was conducted in the absence of any commercial or financial relationships that could be construed as a potential conflict of interest.
Publisher’s Note
All claims expressed in this article are solely those of the authors and do not necessarily represent those of their affiliated organizations, or those of the publisher, the editors and the reviewers. Any product that may be evaluated in this article, or claim that may be made by its manufacturer, is not guaranteed or endorsed by the publisher.
Supplementary Material
The Supplementary Material for this article can be found online at: https://www.frontiersin.org/articles/10.3389/fenvs.2022.894887/full#supplementary-material
References
Bruyère, C. L., Done, J. M., Holland, G. J., and Fredrick, S. (2014). Bias Corrections of Global Models for Regional Climate Simulations of High-Impact Weather. Clim. Dyn. 43, 1847–1856. doi:10.1007/s00382-013-2011-6
Bruyère, C., Monaghan, A. J., Steinhoff, D. F., and Yates, D. (2015). Bias-Corrected CMIP5 CESM Data in WRF/MPAS Intermediate File Format. No. NCAR/TN-515+STR. Boulder, CO: NCAR. doi:10.5065/D6445JJ7
Cai, W., Li, K., Liao, H., Wang, H., and Wu, L. (2017). Weather Conditions Conducive to Beijing Severe Haze More Frequent under Climate Change. Nat. Clim. Change 7, 257–262. doi:10.1038/nclimate3249
Chen, H., and Wang, H. (2015). Haze Days in North China and the Associated Atmospheric Circulations Based on Daily Visibility Data from 1960 to 2012. J. Geophys. Res. Atmos. 120, 5895–5909. doi:10.1002/2015JD023225
Chen, L., Ma, Z., Li, Z., Wu, L., Flemke, J., and Li, Y. (2018). Dynamical Downscaling of Temperature and Precipitation Extremes in China under Current and Future Climates. Atmos. Ocean 56, 55–70. doi:10.1080/07055900.2017.1422691
Emery, C., Tai, E., and Yarwood, G. (2001). Enhanced Meteorological Modeling and Performance Evaluation for Two Texas Ozone Episodes. Report to the Texas Natural Resources Conservation Commission. Novato, CA: ENVIRON International Corporation.
Emery, C., Liu, Z., Russell, A. G., Odman, M. T., Yarwood, G., and Kumar, N. (2017). Recommendations on Statistics and Benchmarks to Assess Photochemical Model Performance. J. Air Waste Manag. Assoc. 67, 582–598. doi:10.1080/10962247.2016.1265027
Feng, J., Quan, J., Liao, H., Li, Y., and Zhao, X. (2018). An Air Stagnation Index to Qualify Extreme Haze Events in Northern China. J. Atmos. Sci. 75, 3489–3505. doi:10.1175/JAS-D-17-0354.1
Fu, G. Q., Xu, W. Y., Yang, R. F., Li, J. B., and Zhao, C. S. (2014). The Distribution and Trends of Fog and Haze in the North China Plain over the Past 30 Years. Atmos. Chem. Phys. 14, 11949. doi:10.5194/acp-14-11949-2014
Gao, Y., Zhang, L., Zhang, G., Yan, F., Zhang, S., Sheng, L., et al. (2020). The Climate Impact on Atmospheric Stagnation and Capability of Stagnation Indices in Elucidating the Haze Events over North China Plain and Northeast China. Chemosphere 258, 127335. doi:10.1016/j.chemosphere.2020.127335
Garrido-Perez, J. M., Ordóñez, C., García-Herrera, R., and Schnell, J. L. (2019). The Differing Impact of Air Stagnation on Summer Ozone across Europe. Atmos. Environ. 219, 117062. doi:10.1016/j.atmosenv.2019.117062
Garrido-Perez, J. M., García-Herrera, R., and Ordóñez, C. (2021). Assessing the Value of Air Stagnation Indices to Reproduce PM10 Variability in Europe. Atmos. Res. 248, 105258. doi:10.1016/j.atmosres.2020.105258
Guo, J., Deng, M., Lee, S. S., Wang, F., Li, Z., Zhai, P., et al. (2016). Delaying Precipitation and Lightning by Air Pollution over the Pearl River Delta. Part I: Observational Analyses. J. Geophys. Res. Atmos. 121, 6472–6488. doi:10.1002/2015jd023257
Hersbach, H., Bell, B., Berrisford, P., Biavati, G., Horányi, A., Muñoz Sabater, J., et al. (2019). ERA5 Monthly Averaged Data on Single Levels from 1979 to Present. Copernicus Climate Change Service (C3S) Climate Data Store (CDS). doi:10.24381/cds.f17050d7
Hewitson, B. C., and Crane, R. G. (2006). Consensus between GCM Climate Change Projections with Empirical Downscaling: Precipitation Downscaling over South Africa. Int. J. Climatol. 26, 1315–1337. doi:10.1002/joc.1314
Hong, S. Y., Noh, Y., and Dudhia, J. (2006). A New Vertical Diffusion Package with an Explicit Treatment of Entrainment Processes. Mon. Weather Rev. 134, 2318. doi:10.1175/mwr3199.1
Horton, D. E., Harshvardhan, H., and Diffenbaugh, N. S. (2012). Response of Air Stagnation Frequency to Anthropogenically Enhanced Radiative Forcing. Environ. Res. Lett. 7, 044034. doi:10.1088/1748-9326/7/4/044034
Hu, J., Chen, J., Ying, Q., and Zhang, H. (2016). One-year Simulation of Ozone and Particulate Matter in China Using WRF/CMAQ Modeling System. Atmos. Chem. Phys. 16, 10333–10350. doi:10.5194/acp-16-10333-2016
Huang, Q., Cai, X., Song, Y., and Zhu, T. (2017). Air Stagnation in China (1985-2014): Climatological Mean Features and Trends. Atmos. Chem. Phys. 17, 7793–7805. doi:10.5194/acp-17-7793-2017
Huang, Q., Cai, X., Wang, J., Song, Y., and Zhu, T. (2018). Climatological Study of the Boundary-Layer Air Stagnation Index for China and its Relationship with Air Pollution. Atmos. Chem. Phys. 18, 7573–7593. doi:10.5194/acp-18-7573-2018
Jiang, Q., Li, W., Fan, Z., He, X., Sun, W., Chen, S., et al. (2021). Evaluation of the ERA5 Reanalysis Precipitation Dataset over Chinese Mainland. J. Hydrol. 595, 125660. doi:10.1016/j.jhydrol.2020.125660
Jiao, D., Xu, N., Yang, F., and Xu, K. (2021). Evaluation of Spatial-Temporal Variation Performance of ERA5 Precipitation Data in China. Sci. Rep. 11, 20216. doi:10.1038/s41598-021-97432-y
Jing, P., Lu, Z., and Steiner, A. L. (2017). The Ozone-Climate Penalty in the Midwestern U.S. Atmos. Environ. 170, 130–142. doi:10.1016/j.atmosenv.2017.09.038
Kerr, G. H., and Waugh, D. W. (2018). Connections between Summer Air Pollution and Stagnation. Environ. Res. Lett. 13, 084001. doi:10.1088/1748-9326/aad2e2
Kinney, P. L. (2018). Interactions of Climate Change, Air Pollution, and Human Health. Curr. Envir Health Rpt. 5, 179–186. doi:10.1007/s40572-018-0188-x
Lee, J.-W., Hong, S.-Y., Chang, E.-C., Suh, M.-S., and Kang, H.-S. (2014). Assessment of Future Climate Change over East Asia Due to the RCP Scenarios Downscaled by GRIMs-RMP. Clim. Dyn. 42, 733–747. doi:10.1007/s00382-013-1841-6
Lee, D., Wang, S.-Y., Zhao, L., Kim, H. C., Kim, K., and Yoon, J.-H. (2020). Long-term Increase in Atmospheric Stagnant Conditions over Northeast Asia and the Role of Greenhouse Gases-Driven Warming. Atmos. Environ. 241, 117772. doi:10.1016/j.atmosenv.2020.117772
Li, L., Qian, J., Ou, C.-Q., Zhou, Y.-X., Guo, C., and Guo, Y. (2014). Spatial and Temporal Analysis of Air Pollution Index and its Timescale-dependent Relationship with Meteorological Factors in Guangzhou, China, 2001-2011. Environ. Pollut. 190, 75–81. doi:10.1016/j.envpol.2014.03.020
Li, J., Zhang, H., Ying, Q., Wu, Z., Zhang, Y., Wang, X., et al. (2020). Impacts of Water Partitioning and Polarity of Organic Compounds on Secondary Organic Aerosol over Eastern China. Atmos. Chem. Phys. 20, 7291–7306. doi:10.5194/acp-20-7291-2020
Liao, T., Gui, K., Jiang, W., Wang, S., Wang, B., Zeng, Z., et al. (2018). Air Stagnation and its Impact on Air Quality during Winter in Sichuan and Chongqing, Southwestern China. Sci. Total Environ. 635, 576–585. doi:10.1016/j.scitotenv.2018.04.122
Liu, S., Gao, W., and Liang, X.-Z. (2013). A Regional Climate Model Downscaling Projection of China Future Climate Change. Clim. Dyn. 41, 1871–1884. doi:10.1007/s00382-012-1632-5
Mlawer, E. J., Taubman, S. J., Brown, P. D., Iacono, M. J., and Clough, S. A. (1997). Radiative Transfer for Inhomogeneous Atmospheres: RRTM, a Validated Correlated-K Model for the Longwave. J. Geophys. Res. 102, 16663–16682. doi:10.1029/97JD00237
Mu, Q., and Liao, H. (2014). Simulation of the Interannual Variations of Aerosols in China: Role of Variations in Meteorological Parameters. Atmos. Chem. Phys. 14, 9597–9612. doi:10.5194/acp-14-9597-2014
Qin, Y., Li, J., Gong, K., Wu, Z., Chen, M., Qin, M., et al. (2021). Double High Pollution Events in the Yangtze River Delta from 2015 to 2019: Characteristics, Trends, and Meteorological Situations. Sci. Total Environ. 792, 148349. doi:10.1016/j.scitotenv.2021.148349
Rigby, M., and Toumi, R. (2008). London Air Pollution Climatology: Indirect Evidence for Urban Boundary Layer Height and Wind Speed Enhancement. Atmos. Environ. 42, 4932–4947. doi:10.1016/j.atmosenv.2008.02.031
Shi, Z., Huang, L., Li, J., Ying, Q., Zhang, H., and Hu, J. (2020). Sensitivity Analysis of the Surface Ozone and Fine Particulate Matter to Meteorological Parameters in China. Atmos. Chem. Phys. 20, 13455–13466. doi:10.5194/acp-20-13455-2020
Sun, J., Liang, M., Shi, Z., Shen, F., Li, J., Huang, L., et al. (2019). Investigating the PM2.5 Mass Concentration Growth Processes during 2013-2016 in Beijing and Shanghai. Chemosphere 221, 452–463. doi:10.1016/j.chemosphere.2018.12.200
Thompson, G., Field, P. R., Rasmussen, R. M., and Hall, W. D. (2008). Explicit Forecasts of Winter Precipitation Using an Improved Bulk Microphysics Scheme. Part II: Implementation of a New Snow Parameterization. Mon. Weather Rev. 136, 5095–5115. doi:10.1175/2008MWR2387.1
Wang, Y., Yao, L., Wang, L., Liu, Z., Ji, D., Tang, G., et al. (2014). Mechanism for the Formation of the January 2013 Heavy Haze Pollution Episode over Central and Eastern China. Sci. China Earth Sci. 57, 14–25. doi:10.1007/s11430-013-4773-4
Wang, X., Dickinson, R. E., Su, L., Zhou, C., and Wang, K. (2018). PM2.5 Pollution in China and How it Has Been Exacerbated by Terrain and Meteorological Conditions. Bull. Am. Meteorol. Soc. 991, 105–119. doi:10.1175/BAMS-D-16-030110.1175/bams-d-16-0301.1
Wang, P., Guo, H., Hu, J., Kota, S. H., Ying, Q., and Zhang, H. (2019). Responses of PM2.5 and O3 Concentrations to Changes of Meteorology and Emissions in China. Sci. Total Environ. 662, 297–306. doi:10.1016/j.scitotenv.2019.01.227
Wang, X., Tolksdorf, V., Otto, M., and Scherer, D. (2021). WRF‐based Dynamical Downscaling of ERA5 Reanalysis Data for High Mountain Asia: Towards a New Version of the High Asia Refined Analysis. Int. J. Climatol. 41, 743–762. doi:10.1002/joc.6686
Xu, Z., Chen, S. X., and Wu, X. (2020). Meteorological Change and Impacts on Air Pollution: Results from North China. J. Geophys. Res. Atmos. 125, e2020JD032423. doi:10.1029/2020jd032423
Yang, H., Fang, Z., Xie, C., Cohen, J., and Cao, Y. (2021). Two Trans-boundary Aerosol Transport Episodes in the Western Yangtze River Delta, China: A Perspective from Ground-Based Lidar Observation. Atmos. Pollut. Res. 12, 370–380. doi:10.1016/j.apr.2021.01.004
Zhang, Y., Wen, X.-Y., and Jang, C. J. (2010). Simulating Chemistry-Aerosol-Cloud-Radiation-Climate Feedbacks over the Continental U.S. Using the Online-Coupled Weather Research Forecasting Model with Chemistry (WRF/Chem). Atmos. Environ. 44, 3568–3582. doi:10.1016/j.atmosenv.2010.05.056
Zhang, R., Qiang, L. I., and Zhang, R. N. (2014). Meteorological Conditions for the Persistent Severe Fog and Haze Event over Eastern China in January 2013. Sci. China Earth Sci. 57, 26–35. doi:10.1007/s11430-013-4774-3
Zhang, H., Wang, Y., Hu, J., Ying, Q., and Hu, X.-M. (2015). Relationships between Meteorological Parameters and Criteria Air Pollutants in Three Megacities in China. Environ. Res. 140, 242–254. doi:10.1016/j.envres.2015.04.004
Zhang, Q., Zheng, Y., Tong, D., Shao, M., Wang, S., Zhang, Y., et al. (2019). Drivers of Improved PM2.5 Air Quality in China from 2013 to 2017. Proc. Natl. Acad. Sci. U.S.A. 116, 24463–24469. doi:10.1073/pnas.1907956116
Zhao, X. J., Zhao, P. S., Xu, J., Meng, W., Pu, W. W., Dong, F., et al. (2013). Analysis of a Winter Regional Haze Event and its Formation Mechanism in the North China Plain. Atmos. Chem. Phys. 13, 5685–5696. doi:10.5194/acp-13-5685-2013
Keywords: climate change, air stagnation events, downscaling, RCP scenarios, China
Citation: Hu A, Xie X, Gong K, Hou Y, Zhao Z and Hu J (2022) Assessing the Impacts of Climate Change on Meteorology and Air Stagnation in China Using a Dynamical Downscaling Method. Front. Environ. Sci. 10:894887. doi: 10.3389/fenvs.2022.894887
Received: 12 March 2022; Accepted: 13 April 2022;
Published: 29 April 2022.
Edited by:
Yuqiang Zhang, University of North Carolina at Chapel Hill, United StatesReviewed by:
Yang Gao, Ocean University of China, ChinaJian Sun, University Corporation for Atmospheric Research (UCAR), United States
Copyright © 2022 Hu, Xie, Gong, Hou, Zhao and Hu. This is an open-access article distributed under the terms of the Creative Commons Attribution License (CC BY). The use, distribution or reproduction in other forums is permitted, provided the original author(s) and the copyright owner(s) are credited and that the original publication in this journal is cited, in accordance with accepted academic practice. No use, distribution or reproduction is permitted which does not comply with these terms.
*Correspondence: Xiaodong Xie, xiaodong.xie@nuist.edu.cn