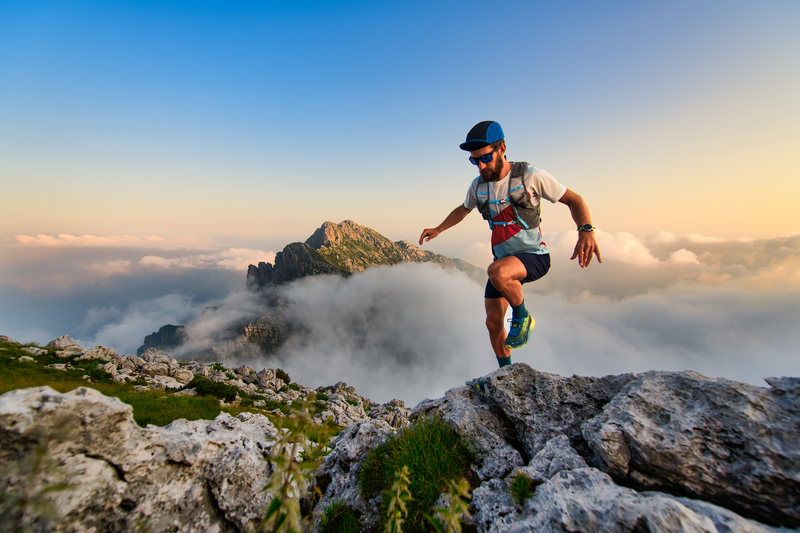
94% of researchers rate our articles as excellent or good
Learn more about the work of our research integrity team to safeguard the quality of each article we publish.
Find out more
ORIGINAL RESEARCH article
Front. Environ. Sci. , 06 July 2022
Sec. Interdisciplinary Climate Studies
Volume 10 - 2022 | https://doi.org/10.3389/fenvs.2022.894779
This article is part of the Research Topic Early Career Scientists’ Contributions to Tropical Pacific Ocean Dynamics and Its Interaction on Mid-Latitude Weather and Climate: Features, Mechanisms, and Prediction View all 5 articles
Carbon monoxide (CO) is an important trace gas in the troposphere, while the El Niño-Southern Oscillation (ENSO) phenomenon is the most important tropical climate variability. ENSO is known to influence interannual variation in meteorological variables on the global scale but its influence on atmospheric CO over large areas in a long term is uncertain. Here we report a strong positive teleconnection between the El Niño–Southern Oscillation (ENSO) in winter (November to February) to tropospheric CO over the North Atlantic European region (NAE) in the following spring (March to May). This ENSO teleconnection is evident in trajectory-mapped airborne CO data (In-service Aircraft for a Global Observing System, IAGOS) over 2002–2019. CO concentrations in El Niño years are 5–20 ppbv higher than those in La Niña years over the NAE troposphere. The regional mean difference from the surface to 300 hPa is 9.4 ppbv (7.6% of the mean). The correlation coefficient (r) between the ENSO index and detrended CO concentrations in the NAE is 0.67 at 400 hPa and 0.63 near the surface, both statistically significant at the 95% level. Such a teleconnection is also observed in independent surface observations, with r ranging from 0.57 to 0.74, all at 95% significance level. From analysis of fire emissions and atmospheric conditions, combined with tagged CO simulations using a chemical transport model, GEOS-Chem, we conclude that this teleconnection results from the combined effects of ENSO on both biomass burning and atmospheric transport. We find that in El Niño years, CO emissions from biomass burning are significantly enhanced in Northern Hemispheric South America, Southeast Asia, and North America due to warmer air temperatures and lowered precipitation. In addition, ENSO enhances CO transport from these regions to the NAE by enhancing upward and northeastward motions in the fire regions, accelerating westerlies over 20°N–40°N, and prompting ascents over the Atlantic and descents over Europe, while reducing CO outflow at the eastern boundary of Europe. The combined effect of ENSO on both CO emissions and CO transport leads to interannual variability in tropospheric CO over the NAE.
Carbon monoxide (CO) is a by-product of incomplete combustion of carbon-based fuels. In addition to being a major pollutant near the ground, CO also acts as the major sink for hydroxyl radicals (OH), responsible for about 75% of its removal (Thompson, 1992). CO therefore influences the oxidation of the atmosphere and the global climate by affecting the concentrations of OH. Under high NOx conditions, CO is a precursor of ozone (O3), while under low NOx conditions, CO participates in O3 destruction (Jacob, 1999; Holloway et al., 2000). O3 is important to radiative forcing as a short-lived climate pollutant and to tropospheric chemistry as a major oxidant (Ramaswamy et al., 2001); CO thus participates in radiative forcing and tropospheric chemistry. Depending on location and season, the atmospheric lifetime of CO varies from several weeks to several months. Therefore, CO is a good tracer for studying the transport of airmass (Edwards et al., 2006a; Liu et al., 2006; Osman et al., 2016; Han et al., 2018), and can help track co-emission pollutants that are more difficult to measure, such as black carbon (Arellano et al., 2010), aerosols and other trace gases (Edwards et al., 2004; Zhang et al., 2006; Bian et al., 2010; Pumphrey et al., 2011).
The El Niño-Southern Oscillation (ENSO) is generally characterized as an oscillation between El Niño and La Niña events, which usually lasts for 1–2 years and recurs every 3–7 years (Rasmusson and Carpenter, 1982; Neelin et al., 1998; Timmermann et al., 2018). The ENSO cycle is irregular (Kessler, 2002). Most El Niño events tend to decay rapidly after the peak while many La Niña events last around 2 years (Okumura and Deser, 2010; Ohba and Watanabe, 2012; Hu et al., 2013; Zhang et al., 2019). ENSO can greatly influence the interannual variation (IAV) of the global climate (Webster et al., 1998; Li and Hsu, 2018). El Niño events often lead to widespread changes in fires, wetland emissions, and atmospheric circulation (Feely et al., 1987; Jones et al., 2001; McPhaden et al., 2006). These effects are strongest in the tropics, but can also trigger atmospheric teleconnections far away from the Pacific tropics and thus have a global impact (Jones et al., 2001).
ENSO teleconnection to the North Atlantic European region (NAE) is currently an active topic of research (Lorenzo et al., 2010; Rodríguez-Fonseca et al., 2016; Joshi et al., 2021). El Niño usually impacts climate with a time lag (Hertig et al., 2015). Brönnimann (2007) suggested that ENSO influence on the NAE is strongest in late winter and early spring. For example, El Niño will increase precipitation in the following spring in Europe (van Oldenborgh and Burgers, 2005; Herceg-Bulić and Kucharski, 2012; Shaman, 2014; Herceg-Bulić et al., 2017). However, whether and how ENSO influences variability in atmospheric CO in the NAE remain unclear.
Biomass burning (BB) is an important source of tropospheric CO (Novelli et al., 2003; Liu et al., 2005; Duncan and Logan, 2008; Liu et al., 2013; Livesey et al., 2013; Cussac et al., 2020; Zhang et al., 2020). Voulgarakis et al. (2015) stated that biomass burning emissions are almost entirely responsible for global CO interannual variability. In the upper tropical troposphere, fire emissions from Indonesia during El Niño are the main drivers for interannual variability of CO (Huang et al., 2014). Previous studies have explored linkages among CO, biomass burning, and ENSO. Some of the studies reported that ENSO can influence atmospheric CO in the Arctic (Monks et al., 2012), Amazon (Ribeiro et al., 2020), the Southern Hemisphere (Buchholz et al., 2018), and the tropics (Edwards et al., 2006b), mainly by enhancing or suppressing biomass burning in their respective source regions. The scale of global fire activities is apparently related to ENSO phases (Doherty et al., 2006; Fuller and Murphy, 2006; Le Page et al., 2008; Fang et al., 2021). The largest CO releases from uncontrolled forest wildfires are often associated with ENSO-induced droughts (Duncan et al., 2003). During the 1997–1998 El Niño phase, global biomass burning emissions increased, resulting in a 40% increase in global CO concentrations and 9% decrease in OH concentrations (Rowlinson et al., 2019). Some studies attributed ENSO-induced CO IAV to the variability in climate and atmospheric circulation. Szopa et al. (2007) suggested that in the tropics, climatic variability can explain 50–90% of CO IAV, while in high latitudes, CO IAV is driven by variability in biomass burning and climate almost equally. Zhang (2011) suggested that negative (positive) anomalies in CO total column are associated with positive (negative) anomalies in precipitation during ENSO. Transport can also influence variations in CO globally (Stohl et al., 2002). The elevated CO concentrations in 1999 spring in Europe were linked to long-range transport of pollution from extremely large fires in China, Southeast Asia, and India (Koumoutsaris et al., 2008). Other studies found that in El Niño years, the global OH level has a downward tendency (Prinn et al., 2001). Anderson et al. (2021) pointed out that in the boundary layer, H2O increases during El Niño years; as OH production is dominated by the reaction of O1D with water, OH also increases. However, in the upper troposphere, OH is mainly controlled by NO, so that in El Niño years, NO decreases, and so does global tropospheric OH.
In view of the importance of CO as a major pollutant and precursor of ozone, it is desirable to investigate CO variability in different time scales, including IAV, and the associated controlling mechanisms. Investigating ENSO influence on IAV in atmospheric constituents, including CO, is challenging (Buchholz et al., 2018) because of the complexity of ENSO effects on atmospheric constituents and their interactions. Such investigation also requires long-term datasets. Previous studies are limited to model simulations (Szopa et al., 2007; Koumoutsaris et al., 2008; Monks et al., 2012; Voulgarakis et al., 2015; Rowlinson et al., 2019), or observations at the surface (Ribeiro et al., 2020) or from satellites (Zhang, 2011; Buchholz et al., 2018). Observations at the surface are scattered and affected by complex boundary-layer processes, while satellite data have low vertical sensitivity (Jacob et al., 2003; Ding et al., 2015). In recent years, a trajectory-mapped CO dataset (Osman et al., 2016) has been constructed based on airborne IAGOS data (In-service Aircraft for a Global Observing System, Nédélec et al., 2015). This global CO dataset is latitudinally, longitudinally, and vertically resolved with a vertical resolution of 1 km. It also covers decadal periods. This observation-based dataset provides an unprecedented opportunity to investigate ENSO influence over large areas and in fine vertical and horizontal resolutions that have not been available before. With this dataset, we aim to address the following scientific questions: 1) is there an ENSO teleconnection to IAV in CO concentrations over the NAE? 2) what are possible drivers for the teleconnection? To help interpret the observational CO data, we conduct CO simulations using a global chemical transport model, GEOS-Chem.
The paper is organized as follows. After this introduction, data and methods are described. Then, our analysis is presented in two sections: 1) an ENSO teleconnection to the IAV in springtime CO concentrations over the NAE and 2) underlying mechanisms for this ENSO connection. In the Discussion section, we further examine whether CO in the NAE is connected to other climate modes. Conclusions are provided in the last session. We also briefly discuss the new contributions of this study to the field.
The primary data for this study are the trajectory-mapped IAGOS CO data (Osman et al., 2016). We also supplement our analysis with ground-level CO observations and GEOS-Chem model simulations. To explore how ENSO affects the interannual variability of tropospheric CO in the NAE (30°N–60°N, 60°W–50°E, see Figure 1A), NCEP meteorological reanalysis, and Global Fire Emissions Data (GFED) are used.
FIGURE 1. (A) The seasonal mean CO distribution in March, April, and May (MAM, the same for the figures and tables below) at 400 hPa in El Niño years (2002, 2007, 2010, and 2016, the same for the figures and tables below). The dashed lines indicate the North Atlantic European region (NAE). The blue, magenta, and green stars indicate three ground-level sites: IZO (Izaña), WIS (Sede Boker) and JFJ (Jungfraujoch), respectively. (B) The same as (A), but in La Niña years (2006, 2008, 2009, 2011, 2012, and 2018, the same for the figures and tables below). (C) The longitude-altitude cross section of the CO difference between El Niño and La Niña years averaged over 30°N-60°N, MAM, overlaid with the corresponding wind difference between El Niño and La Niña years. The vertical wind velocity is enlarged by 1,000 times for illustration purposes. The dashed vertical lines at 60°W and 50°E indicate the longitudinal borders of the NAE. (D) Interannual variation during 2002–2019 in MAM CO concentrations averaged over the NAE (the boxed area in Figure 1A), varying with altitude. Red and blue lines denote El Niño and La Niña years, respectively.
The IAGOS airborne CO data are available from the European Research Infrastructures programme (https://www.iagos.org, Nédélec et al., 2015). The IAGOS data are unevenly distributed in space and time. Through trajectory mapping of the IAGOS data, Osman et al. (2016) constructed a three-dimensional CO dataset at 5 ° × 5 °×1 km (latitude, longitude, altitude), available monthly from 2002 to 2012 at ftp://es-ee.tor.ec.gc.ca/pub/ftpdt/MOZAIC_output_CO/. The trajectory-mapped CO dataset was carefully evaluated. The dataset is in good agreement with laboratory-analyzed NOAA aircraft flask data. The CO total column is 12–16% higher than that estimated by MOPITT (Osman et al., 2016).
In this study, the trajectory-mapped CO dataset is extended to 2019 to cover a study period of 18 years, from 2002 to 2019. The global monthly CO data fields after the trajectory-mapping still have data gaps, with 30–70% missing data depending on region and layer. A weighted average approach was used to interpolate data into these gaps, on each of the 1-km layers. The CO concentration for a gridpoint without CO data is determined by the mean of the CO values from the closest surrounding gridpoints where CO data are available, weighted by distance. The weight
where N is the number of trajectories at the gridpoint with CO data when generating the trajectory-mapped CO data for that grid. This value is available with the CO dataset. D is the distance between this gridpoint and the target (the gridpoint with no data), and R is the correlation length of CO (taken as 700 km in this study, the same as Osman et al. (2016)).
We use the bi-monthly Multivariate ENSO Index (MEI.v2, https://psl.noaa.gov/enso/mei/) to describe ENSO variations (Wolter and Timlin, 2011). The MEI.v2 is a leading principal component time series of the Empirical Orthogonal Function (EOF) of five different atmosphere ocean variables over the tropical Pacific basin (30°S–30°N and 100°E–70°W). The MEI.v2 allows for spatial variations in its key features with the seasonal cycle, and can reflect multiple characteristics of ENSO and has less vulnerability to errors.
Since the mature stage of ENSO often occurs in cold seasons, we define the average of MEI.v2 in November of year 0 to February of year 1 (NDJF) as the ENSO index of year 1 (Monks et al., 2012). In this study, an El Niño event is defined when the MEI. v2 > 0.5 for five consecutive months and the mean of MEI. v2 in NDJF > 0.5, while a La Niña event is defined when the MEI. v2 < −0.5 for five consecutive months and the mean of MEI. v2 in NDJF < −0.5. Over 2002−2019, there are four ENSO cycles with four El Niño and six La Niña phases. We use El Niño and La Niña years to describe the years affected by El Niño or La Niña events in the previous winters. Therefore, El Niño years include 2003, 2007, 2010, and 2016, while La Niña years include 2006, 2008, 2009, 2011, 2012, and 2018.
We also examine whether there are connections between the CO concentrations over the NAE and other climate modes, including the North Atlantic Oscillation (NAO), Pacific North American pattern (PNA), the Atlantic Multi-Decadal Oscillation (AMO), and sea surface temperature (SST). The NAO index and the PNA index are derived from the Rotated Principal Component Analysis technique. The tropical North Atlantic sea surface temperature (TNA SST) index is the anomaly of the average of the monthly SST over 5.5°N to 23.5°N and 15°W to 57.5°W. The NAO, PNA, and TNA SST indices can be downloaded from https://psl.noaa.gov/data/climateindices/list/. The AMO index is based on the average anomalies of SST in the North Atlantic basin, typically over 0°−80°N. To remove the climate change signal from the AMO index, the SST data need to be detrended by subtracting the global-mean SST anomaly time series from the spatially averaged time series. The AMO index can be downloaded from https://climatedataguide.ucar.edu/sites/default/files/amo_monthly.txt. The NINO 1 + 2 index is the area-averaged SST from 0° to 10°S and 90°W−80°W and the NINO 4 index is the area averaged SST from 5°S to 5°N and 160°E−150°W. SST Indices (NINO 1 + 2 and NINO 4) are downloaded from https://psl.noaa.gov/gcos_wgsp/Timeseries/.
We employ surface CO measurements from three sites in the NAE (Figure 1A). Flask measurements are made at IZO (Izaña) and WIS (Sede Boker) while in-situ measurements are made at JFJ (Jungfraujoch). Details of the stations and sampling network can be found in Dlugokencky et al. (2005), Novelli et al. (1998), and Yu et al. (2020). Data are available from the World Data Centre for Greenhouse Gases (WDCGG, https://gaw.kishou.go.jp/) and the NOAA Global Monitoring Laboratory (GML, https://gml.noaa.gov/ccgg/flask.html).
Data for CO emissions from biomass burning are from the GFED version 4.1 with small fires (GFED4.1s) from 1997 to 2021 (Giglio et al. (2013); van der Werf et al. (2017), available at https://globalfiredata.org/pages/data/). The gridded emission data have spatial resolution of 0.25° latitude × 0.25° longitude and monthly temporal resolution. Emissions of fire-generated trace gases are derived by combining satellite information on burned areas, biogeochemical model estimates of fuel burned, and estimated emission factors for each species.
The anthropogenic CO emission inventories are from the Community Emissions Data System (CEDS) database (v_2021_04_21, last access: 2022/02/10; Hoesly et al., 2018), available at https://doi.org/10.25584/PNNLDataHub/1779095. The spatial resolution of the inventory is 0.5° latitude × 0.5° longitude. Emissions are provided as monthly averages. The scaled emission estimates are extended back to 1750 by extending activity data and emission factors separately to produce a full trend from 1750 to 2019.
The observed precipitation data used are the National Oceanic and Atmospheric Administration (NOAA) Precipitation Reconstruction Dataset (PREC) (Chen et al., 2002). The PREC dataset is an analysis of monthly precipitation constructed on a 2.5° latitude × 2.5° longitude grid over the globe from 1948 to the present.
The reanalysis data of pressure, air temperature, sea level pressure, geopotential height, zonal wind, meridional wind, and vertical motion are provided by the National Centers for Environmental Prediction (NCEP, https://psl.noaa.gov/). The data have a horizontal resolution of 2.5° latitude × 2.5° longitude, and a vertical resolution of 17 layers from 1,000 hPa to 10 hPa.
Here we use the GEOS-Chem (v12-09-03, http://geos-chem.org) chemistry transport model to simulate tropospheric CO globally over 2002–2019 (using 2000–2001 for spin-up). The model is driven by the Modern-Era Retrospective Analysis for Research and Applications, Version 2 (MERRA2) meteorological data (Gelaro et al., 2017). To match the resolution of the IAGOS data, GEOS-Chem simulations were carried out at 4° latitude × 5° longitude grids. We ran two simulations: the standard and tagged CO simulations.
The results from the standard simulations are used to compare with observations. The standard simulations have 72 vertical layers, including about 35 levels in the troposphere from 1,000 to 100 hPa. GEOS-Chem uses global anthropogenic emissions from CEDS, supplemented by some regional emission inventories, such as Air Pollutant Emission Inventory (APEI) and monthly mean contiguous U.S. (CONUS) emissions from 2011 National Emissions Inventory (NEI 2011) inventory. The biomass burning emissions are from GFED monthly inventories. Emissions from other natural sources (e.g., lightning, volcanoes) are also included. The GEOS-Chem standard simulation considers most of the factors in climate change, and can accurately simulate the interannual variations of common atmospheric substances such as CO and O3. It is known that GEOS-Chem underestimates CO concentrations by ∼10% (Heald et al., 2003; Duncan et al., 2007; Kopacz et al., 2010; Ding et al., 2015). The underestimate is possibly due to biases in the CO inventory or model parametrization or both (Kopacz et al., 2010).
The tagged CO simulation can assess how much CO is transported from a defined domain to other regions. The tagged CO simulations can help analyze the impact of CO produced in one region on CO concentrations in other regions. Tagged CO simulation can separate the CO from biomass burning and from all sources, including anthropogenic and biofuel sources. The tagged CO simulation has 47 vertical layers, with 35 vertical layers (1,000–100 hPa) in the troposphere. GEOS-Chem tagged CO simulations use the same anthropogenic emission inventories as the standard simulations, including CEDS, APEI, and NEI 2011. The biomass burning emission inventory used for the tagged CO simulations is Quick Fire Emissions Database v2 (QFED2). The tagged CO simulation first defines CO source and receptor regions. This study divides the world into 10 areas (Supplementary Figure S1), namely the North Atlantic European region (NAE, the receptor region), North America (NA), Northern Hemispheric South America (NHSA), Southern Hemispheric South America (SHSA), Northern Hemispheric Africa (NHAF), Southern Hemispheric Africa (SHAF), Asia (AS), Southeast Asia (SEAS), Australia (AUST), and the rest of the world (ROW).
Because of the autocorrelation of climate variability, the effective degree of freedom (
where N is the sample size and
Figures 1A,B respectively show the spatial distribution of CO concentrations in El Niño and La Niña years at 400 hPa in spring (mean of March, April, and May (MAM)). Spatially, CO concentrations at this level are highest in northeastern Asia and northern North America, reaching more than 180 ppbv in El Niño years. With increasing latitude, solar radiation and OH radicals decrease (Lu and Khalil, 1991), leading to an increase of CO concentrations from the equator to the Arctic. CO concentrations in El Niño years are higher than those in La Niña years over most of the NH.
Figure 1C shows a latitudinal-vertical cross section of the difference in CO between El Niño and La Niña years, averaged over 30°N−60°N. The difference in CO concentrations over the NAE is evident throughout the troposphere. The maximum difference in CO concentrations between El Niño and La Niña years can reach 20 ppbv at some locations. On average (Table 1), CO concentrations over the NAE are about 9.4 ppbv (7.6% of the mean) higher in El Niño years than in La Niña years from the surface to 300 hPa. The difference in CO concentrations between El Niño and La Niña years at the latitudes in Table 1 reaches the 95% significance level from 1,000 to 400 hPa and the 90% significance level at 300 hPa.
TABLE 1. Vertical variations in CO concentrations, CO anomalies in El Niño and La Niña years, the relative differences of the anomalies, the coefficient of variation (CV = STD/mean), trend of CO concentrations, correlation coefficients (r) between the ENSO index and detrended CO concentrations in the North Atlantic European region (CODT_NAE). “ΔCO” is the difference in CO concentrations between El Nino and La Nina years.
Also shown in Figure 1C is the difference in wind between El Niño and La Niña years. As is well known, westerlies prevail over the NAE troposphere (Supplemenatry Figure S2A). Previous studies have suggested a pollution transport pathway from the east coast of North America in the lower troposphere to the west coast of Europe in the middle and upper troposphere (Li et al., 2002; Stohl and Eckhardt, 2004; Liu et al., 2006; Liu J. J. et al., 2009). When CO from other regions is transported to the western border of the NAE, airmass carrying CO ascends between 30°W and 60°W from the lower troposphere to the middle and upper troposphere, and then descends to the lower troposphere between 10°W and 30°E (Supplementary Figure S2B). Figure 1C shows that in El Niño years, the eastward and upward winds in the western NAE strengthen, increasing CO concentrations in the upper troposphere, while the subsidence becomes stronger around 10°W and 30°E, facilitating CO accumulation in the lower troposphere and enhancing ground-level CO concentrations in Europe. At the same time, CO outflow at the eastern border of Europe decreases. All these variations in wind fields are conductive to CO accumulation in Europe, which will be discussed in detail in Section 4.2.
Figure 1D shows the year-to-year variation in MAM CO concentrations over 2002–2019 in the NAE at different altitudes. Generally, the higher the altitude, the lower the CO concentrations, as the major CO sources are at the surface. At all altitudes, the four El Niño years are all associated with an increase in CO concentrations, while the five La Niña years are associated with a decrease in CO concentrations, except for 2018. There is a CO peak in 2014 that apparently has no connection with ENSO, suggesting other influences. In fact, the elevated CO in 2014 is related to an anomalous increase in biomass burning in Southeast Asia resulted from human activities (World Resources Institute, 2014). Some studies regard 2017 as a weak La Niña year (Setimela et al., 2018; Ferrer et al., 2019; Zhang et al., 2019), and CO concentrations in 2017 also decline. The overall magnitude for the IAV, as expressed by the coefficient of variation (CV), stays around 5–7% below 200 hPa (Table 1).
In addition to the IAV in CO concentrations, there is a downward trend in CO concentrations in the troposphere from 2002 to 2019. The declining trend is most significant in the lower troposphere (Table 1). To analyze IAV in CO, we first remove the decreasing trend in CO concentrations over 2002–2019 for the entire CO database. Figures 1A,B show that over the NAE, CO concentrations appear to be more sensitive to ENSO than in other regions: high in El Niño years and low in La Niña years. This is further confirmed in Figure 2A that shows correlations between the ENSO index in winter (November to February) and detrended CO concentrations (CODT) in the following spring (March to May) over 2002–2019 at 400 hPa. Positive correlations between CODT and the ENSO index are significant over the central Europe and the Mediterranean region, as well as over the central North Atlantic. In El Niño years, CODT in the NAE increases in spring, while in La Niña years, CODT in the NAE decreases in spring. On average over the entire NAE (Table 1), the two series correlate significantly from the surface to 300 hPa (all p < 0.10), with strongest correlation at 400 hPa (r = 0.67) and at the surface (r = 0.63). An example of these correlations is shown in the time series over 2002–2019 at 400 hPa (Figure 2B).
FIGURE 2. (A) Correlation between the ENSO index and detrended CO concentrations (CODT) at 400 hPa in MAM over 2002–2019, with asterisks indicating correlations at the 95% significance level. The dashed lines indicate the area of interest, the NAE. (B) Interannual variation in MAM CODT (blue line) in the NAE against the ENSO index in the previous winter (brown line, the mean of the ENSO index from November to February, the same in the figures and tables below). (C) The longitude-altitude cross section of the correlation between the ENSO index and MAM CODT, averaged over 30°N-60°N, with asterisks indicating the 95% significance level. The vertical dashed lines show the longitudinal borders of the NAE.
The variation in the correlation between ENSO and CODT at different altitudes is also of interest (Figure 2C). In the central North Atlantic, the correlation appears stronger in the lower troposphere, while over Europe, the correlation becomes stronger in the middle and upper troposphere. This can be explained by Figure 1C, which shows that the eastward and upward winds are strengthened in El Niño years over the North Atlantic. The variations in vertical and horizontal wind field with ENSO will be examined in detail in Section 4.2.
Figure 3 further shows the connection of ENSO to IAV in ground-level CODT in spring at three sites in the NAE (Figure 1A): Izaña (IZO, 16°W, 28°N, and 2,372 m), Sede Boker (WIS, 35°E, 29°N, and 151 m), and Jungfraujoch (JFJ, 7.9°E, 46°N, and 3,580 m). Despite their geographical differences, the correlations of the ENSO index and CODT are all positive and significant (p < 0.05). Similar positive correlations between CODT concentrations and the ENSO index can also be observed from MOPITT (Measurements Of Pollution In The Troposphere, Supplementary Material S1) CO data (Supplementary Table S1). This correlation is also supported by GEOS-Chem simulations (Supplementary Table S1): an example at 400 hPa is shown in Supplementary Figure S3A, although CO concentrations from GEOS-Chem are about 15% lower than IAGOS-based ones. The spatial distributions of the simulated MAM CO concentrations at 400 hPa in El Niño and La Niña years are shown in Supplementary Figures S3B,C, which compare well to Figures 1A,B. All the analysis indicates that the ENSO index in the cold season is positively and significantly correlated with the CO anomaly in the following spring over the NAE, and the correlation persists from the ground up to the upper troposphere.
FIGURE 3. Interannual variation in detrended CO concentrations in the surface layer (CODT, blue line) at three stations in spring and the ENSO index in the previous winter (brown line). The correlation coefficient (r) and its p-value (p) between the two series are also shown. The three ground-level sites are (A) IZO (Izaña), 16°W, 28°N, 2,372 m, (B) WIS (Sede Boker), 35°E, 29°N, 151 m, and (C) JFJ (Jungfraujoch), 7.9°E, 46°N, 3,580 m.
We assess CO emissions from biomass burning (COBB) in the NH from six regions (Figure 4A), including North America (NA; 160°W−60°W, 12.5°N−80°N), NH South America (NHSA; 82°W−50°W, 0°−12.5°N), Southeast Asia (SEAS; 90°E−130°E, 0°−25°N), NH Africa (NHAF; 20°W-50°E, 0°−30°N), the North Atlantic European region (NAE; 60°W-50°E, 30°N−60°N), and Asia (AS; 50°E−180°E, 25°N−80°N).
FIGURE 4. (A) Spatial distribution of MAM CO emissions from biomass burning (COBB_E, in Tg) averaged over 2002–2019, based on GFED4.1s data. COBB_E less than 0.001 Tg are not shown. (B) Time series of COBB_E in MAM from North America (NA), Northern Hemispheric South America (NHSA), and Southeast Asia (SEAS) over 2002–2019. The correlation coefficients (r) and the corresponding p-values (p) between the ENSO index and COBB_E are indicated. The vertical red and blue lines show El Niño and La Niña years respectively.
Figure 4B shows the IAV in COBB emissions in MAM from NHSA, SEAS, and NA. Chen et al. (2017) found that, following an El Niño episode, fires peaked in SEAS and NHSA from January to April and in central America from March to May. Figure 4B and Table 2 show that the ENSO index correlates well with COBB emissions in NHSA (r = 0.53) and SEAS (r = 0.50). Although the correlation coefficient for NHSA is higher, its effective degrees of freedom is lower than that for SEAS, so the ENSO connection to fire emissions from SEAS is more significant. The ENSO correlation with COBB emissions in NA is complex, and can be discussed individually for three sub-regions (Supplementary Figure S4): boreal North America (BONA, 40°N−80°N), temperate North America (TENA, 20°N−40°N), and central America (CEAM, 12.5°N−20°N). In BONA, the COBB emissions per unit area are small, but the total fire area is large, and COBB emissions in BONA are generally positively correlated with ENSO, while in TENA, the total fire area is small and COBB emissions per unit area are moderate, but ENSO is negatively correlated with COBB emissions. In CEAM, the total fire area is small, but COBB emissions per unit area are large, and COBB emissions are positively correlated with ENSO. Overall, the correlation coefficient between ENSO and COBB emissions over the entire NA reaches 0.31, positive and moderately strong, although not passing the 90% significance level. Although COBB emissions in NHSA, SEAS, and NA usually increases in El Niño years and decreases in La Niña years, they are not completely synchronized in every year. For example, 2003 is an El Niño year while 2004 is a neutral year; COBB emissions in NHSA and NA in 2003 are higher than those in 2004, but the opposite is true in SEAS. Similarly, 2010 is an El Niño year while 2011 is a La Niña year; COBB emissions in NHSA and SEAS in 2010 are higher than those in 2011, but the opposite is true in NA. None of the three regions individually corresponds to ENSO variations impeccably. However, the overall response of COBB emissions in the three regions corresponds well to the ENSO signal (r = 0.72, p < 0.02). In sum, ENSO apparently correlates to the IAV in COBB emissions from NHSA and SEAS positively and significantly. ENSO shows a positive impact on COBB emissions from NA, but not to a significance level. In contrast, ENSO impact on fires in the NAE, as well as in NHAF and AS, appears non-significant.
TABLE 2. CO emissions from biomass burning (COBB_E) in different regions based on GFED4.1s data. Correlation coefficients (r) between the ENSO index and COBB_E, air temperature (T), and precipitation (Precip) at the surface. See Figure 4A for the region definition.
Temperature and precipitation are the most important factors affecting IAV in COBB emissions (Field and Shen, 2008; Marlon et al., 2008; Pechony and Shindell, 2010; Daniau et al., 2012). It is known that ENSO can disturb normal climatic patterns in air temperature and precipitation around the world (Ropelewski and Halpert, 1986; Bradley et al., 1987; Magaña et al., 2003; Yu et al., 2012; Cai et al., 2020). Chen et al. (2017) suggested that on average, reductions in precipitation and terrestrial water storage during and after El Niño episodes increased fire emissions in pan-tropical forests by 133% relative to after La Niña episodes. Table 2 shows the mean air temperature and precipitation over 2002–2019 and their correlations with ENSO in different regions. In SEAS and NHSA, ENSO is positively correlated with air temperature (p < 0.05) and negatively correlated with precipitation (p < 0.05), more strongly in SEAS. These correlations explain why ENSO is positively correlated with COBB emissions in SEAS and NHSA. In AS, NAE, and NHAF, ENSO appears to be positively correlated to precipitation, significantly in AS and NHAF. This may be the reason for negative but non-significant correlation between ENSO and COBB emissions in these three regions. In NA, the relationship between the ENSO index and air temperature or precipitation can also be assessed in three sub-regions: BONA, TENA and CEAM (Supplementary Figure S5). Overall, in El Niño years, BONA and CEAM show an increase in air temperature and a decrease in precipitation, while TENA shows the opposite, consistent with the impact of ENSO on COBB emissions in BONA, TENA, and CEAM. Human activities are another factor driving IAV in COBB emission. In particular, large biomass burning in 2014 over SEAS was related to human activities (World Resources Institute, 2014), and clearing land for agriculture is the major cause of these fires (Earth Observatory, 2014).
We directly correlate CO variability in the NAE with COBB emissions in three major source regions (NHSA, SEAS, and NA) (Table 3). As in Table 1, detrended CO concentrations in the NAE are expressed CODT_NAE. The results show that the sum of COBB emissions in SEAS, NA, and NHSA is highly correlated with CODT_NAE throughout the troposphere from 850 hPa to 300 hPa (at least p < 0.10). The positive correlation between CODT_NAE and the sum of COBB emissions in SEAS, NA, and NHSA is largest at 400 hPa, consistent with the fact that CODT_NAE and ENSO show the largest correlation at 400 hPa. COBB emissions in NHSA are also correlated with CODT_NAE from 850 hPa to 300 hPa, while COBB emissions in NA and CODT_NAE are correlated significantly in the upper troposphere only (400 hPa and 300 hPa).
TABLE 3. Correlation coefficient (r) of detrended CO concentrations over the NAE (CODT_NAE) against fire-induced CO emissions (COBB_E) from North America (NA), NH South America (NHSA), and Southeast Asia (SEAS). See Figure 4A for the region definition.
How does the positive correlation between ENSO and COBB emissions in NHSA, SEAS, and NA (the source regions) affect the CO variability in the NAE (the receptor region)? The GEOS-Chem tagged CO simulation can help to address this question (Figures 5, 6). The simulation can separately assess the transport of fire-induced CO (COBB) from different regions to the NAE. Figure 5 shows an example of the transport pathways of COBB from individual source regions at 850 hPa. The COBB in SEAS (Figure 5A) is first uplifted and transported northeastward to around 30°N in Asia, then crosses the Pacific Ocean along the 30°N parallel to reach the west coast of North America. This airmass then flows across the United States, joining airmass carrying NA COBB (Figure 5B) to cross the Atlantic along the 30°N−60°N latitude belt, and to finally reach the NAE. COBB produced in NHSA (Figure 5C) is also uplifted to 850 hPa. A part of it is transported northeastwards and reaches the NAE, while the rest of it is transported southwestwards to the equatorial Pacific Ocean. Figure 6 further shows the difference in CO concentrations from individual source regions and along the transport pathways between El Niño and La Niña years. Clearly shown, COBB transported to the NAE from SEAS, NHSA, and NA is enhanced during El Niño years. Supplementary Table S2A shows COBB from several source regions that has reached the NAE, using the mean CO concentrations over the NAE (COBB_NAE) at 400 hPa as an example. As known, using the tagged CO simulation, COBB_NAE can be partitioned to COBB from different regions. Among all the regions, ENSO is most correlated to COBB_NAE from NHSA, SEAS, and NA.
FIGURE 5. GEOS-Chem tagged CO simulations at 850 hPa. (A) Spatial distribution of fire-induced CO (COBB, ppbv, in color) from Southeast Asia (SEAS) only, overlaid with the winds (arrows). (B) The same as (A), but for COBB from North America (NA) only. (C) The same as (A), but for COBB from Northern Hemispheric South America (NHSA) only. (D) The same as (A), but for COBB from Southeast Asia (SEAS), North America (NA), and NH Southern America (NHSA) in total. All values are averaged MAM CO concentrations over 2002–2019.
FIGURE 6. The same as Figure 5, but for the differences in COBB concentrations and wind between El Niño and La Niña years.
Switching from the receptor region to the various source regions, we examine the ENSO connection to COBB concentrations in the source regions at 400 hPa, termed as COBB_source. Comparing Supplementary Table S2B with Supplementary Table S2A, we note that ENSO correlates better with COBB_NAE in the receptor region that is transported from NHSA and SEAS than with COBB in the corresponding source regions (NHSA and SEAS). This suggests that ENSO can also modulate CO transport from the source regions to the NAE. This is addressed in Section 4.2.
Atmospheric transport can lead to variations in CO concentrations at regional and global scales (Stohl et al., 2002; Bowman, 2006; Liu et al., 2006; Ding et al., 2015; Han et al., 2018). For example, the increase in CO concentrations over Europe in the 1999 spring has been related to the long-distance transport of CO from large-scale fires in China, Southeast Asia, and India (Koumoutsaris et al., 2008). CO transport is driven by the global atmospheric circulation, which is sensitive to different ENSO signals. We find that ENSO can impact IAV in CO transport to the NAE through the following processes: 1) enhancing upward, northward, and eastward motions over the SEAS fire regions, 2) strengthening westerlies around 10°N−50°N, 3) enhancing northward motion over the NHSA fire region, 4) enhancing ascents over the Atlantic and descents over Europe, and 5) reducing outflow from the eastern border of the NAE. These processes are examined from the perspectives of sea level pressure (Figure 7A), geopotential height (Figure 7A), and horizontal and vertical winds (Figures 7B,C, Supplementary Figure S2). The connection between ENSO and the atmospheric circulation is illustrated from the differences in pressure and wind fields between El Niño and La Niña years (Figure 8, Supplementary Figures S6) and the correlations between the ENSO index and wind fields, including zonal wind (Figure 9), meridional wind (Supplementary Figure S7) and vertical wind (Supplementary Figure S8). Furthermore, GEOS-Chem tagged CO simulations help understand these processes by showing CO transport pathways to the NAE from different source regions (Figure 5) and the differences in the transport pathways between El Niño and La Niña years (Figure 6). Note that except for Figures 5, 6, and Supplementary Table S3, all the dynamic analysis in this section is based on the reanalysis data.
FIGURE 7. (A) Mean MAM sea level pressure (SLP, in color, unit: hPa) and geopotential height at 850 hPa (HGT, in contour, unit: m) over 2002–2019. (B) Horizontal wind fields (streamlines) averaged over 2002–2019 in MAM at 850 hPa. Overlaid is the corresponding vertical velocity (in color, Pa s−1). (C) The same as (B), but at 400 hPa. Note the upward velocity is negative (in blue).
FIGURE 8. (A) Difference in horizontal wind (arrows) between El Niño and La Niña years in MAM at 850 hPa. Overlaid is the corresponding difference in vertical velocity (in color, Pa s−1). (B) The same as (A), but at 400 hPa. Note the upward velocity is negative (in blue).
FIGURE 9. (A) Correlation between the ENSO index and wind in the U direction at 850 hPa in MAM over 2002–2019, with asterisks indicating the 95% significance level. (B) The same as (A), but at 400 hPa.
Figure 7A shows the mean status of sea level pressure (in color) and geopotential height (in contour) at 850 hPa in spring averaged over 2002–2019. The Pacific is under control of the Aleutian low and the Hawaiian high, forming a trans-Pacific airflow along the 30°N–45°N latitude belt (Figure 5A, Figure 7B,C), while the North Atlantic under control of the Icelandic low and the Azores high, forming a trans-Atlantic airflow along the 30°N–45°N latitude belt. SEAS, NHSA, and Mesoamerica are controlled by low pressure systems, leading to vertical updraft in the three regions (Figures 7B,C).
Figures 7B,C respectively show the mean status (2002–2019) of horizontal wind flows (streamlines) and vertical velocity (in color) at 850 hPa and 400 hPa in spring. At 850 hPa (Figure 7B; Figure 5A), updrafts over SEAS are evident, which facilitate transport of CO from the surface to the middle and upper troposphere. The horizontal wind there is dominated by southwesterlies, which transport CO northeastward across the Pacific Ocean to the west coast of North America along the 30°N–45°N latitude belt. On the west coast of North America, the airmass carrying SEAS CO is partially blocked by the Rocky Mountains and turns to the south. Some of this airmass crosses over the Rocky Mountains. Together with CO from central North America, SEAS CO ascends along the northern rim of the Azores high and flows northeastward along the 30°N-60°N latitude belt across the Atlantic to Europe (Figure 7B, 5A,B, also see Li et al., 2002; Stohl and Eckhardt, 2004). On the west coast of Europe, the air flow is divided into two branches, southward and northward, with the northward flow transporting CO to northern Europe and the southward flow transporting CO to the Mediterranean (Huntrieser and Schlager, 2004). As subsidence prevails over most of Europe (Supplementary Figure S2B over 10°W-30°E), CO from SEAS and NA is then transported downward to the surface. For CO from NHSA (Figure 7B and 5C), the transport pathway is rather different (Figure 5C): the NHSA CO can be uplifted to 850 hPa (Figure 7B) and then is transported northeastward across the Mesoamerica (the south rim of the Azores high), and then to the NAE. Overall, the spatial variation in wind field at 400 hPa (Figure 7C) is similar to that in 850 hPa, although zonal winds prevail more at 400 hPa. The airflow at 400 hPa is not blocked by the Rocky Mountains, so SEAS CO at this level can be more efficiently transported to North America and then to the NAE.
In El Niño years, both the Aleutian low and the Hawaiian high over the North Pacific intensify (Supplementary Figure S6A), which can strengthen the trans-Pacific flow from Asia to North America. Over the North Atlantic, a low-pressure anomaly over the east coast of North America can enhance updrafts, while a high-pressure anomaly over northern Europe would bring about more descents over Europe. High-pressure anomalies in western Africa and low-pressure anomalies in eastern North America will also enhance northward transport from the NHSA to Europe. The overall spatial distribution of sea level pressure and geopotential height anomalies in La Niña years is opposite to that in El Niño years (Supplementary Figure S6B).
ENSO anomalies in wind fields are shown in Figure 8A for 850 hPa and Figure 8B for 400 hPa. In El Niño years, upward motion is enhanced over northern SEAS (Figure 8A and Supplementary Figure S8A). In the meantime, eastward (Figure 9) and northward (Supplementary Figure S7A) motion is also promoted there (Process 1). Therefore, COBB from SEAS can be more effectively uplifted and transported northeastward to join the westerlies (Figure 6A). Process 2 is well illustrated in Figure 9A (for 850 hPa) and Figure 9B (for 400 hPa): westerlies over the 20°N–40°N latitude belt are generally enhanced, more significantly at 400 hPa than at 850 hPa. There are three regions where westerlies are significantly enhanced at 400 hPa (Figure 9B), including regions near 20°N over SEAS, over the Pacific around 10°N–40°N, and over the Atlantic between 20°N and 40°N. This process can facilitate more COBB transport from the major source regions to the NAE; the large enhancement in westerlies over the Atlantic is the main driver for positive anomalies in NA COBB to be transported to the NAE (Figure 6B). Process 3 is related to CO from NHSA. In El Niño years, eastward (Figure 9A) and upward (Figure 8A) motions are enhanced over NHSA, although the connection of ENSO to these enhancements is not statistically significant. The significant influence of ENSO is the elevated northward motion (Figure 8A and Supplementary Figure S7A) that transport COBB from NHSA to the NAE (Figure 6C). Process 4, enhancing vertical motion along the northern rim of the Azores, is evident in Figures 8A,B, Supplementary Figure S8A,B at both 850 hPa and 400 hPa. The enhancements are apparent over the west cost of Europe, the Mediterranean Sea, and the Black Sea at 400 hPa (Supplementary Figure S8B). These anomalies are significantly correlated with ENSO in southwest Europe at 850 hPa (Supplementary Figure S8A). Process 5, an ENSO effect of reducing outflow from the eastern border of the NAE, has been discussed for Figure 1C. We further examine this process in terms of CO flux. Supplementary Figure S9 shows the mean horizontal CO flux at the eastern and western borders of the NAE, and Figure 10 shows the corresponding flux differences between El Niño and La Niña years. As expected, eastward CO flux dominates. In El Niño years, the horizontal CO inflow at the western border of the NAE increases throughout the entire tropospheric column, with largest increase of 600 ppbv m s−1 around 400–300 hPa (Figure 10A), while the CO outflow from the eastern border of the NAE decreases by up to 500 ppbv m s−1 (Figure 10B). The combined effect of Processes 4 and 5 may explain the vertical ENSO-CO relationship over the NAE (Figure 2C).
FIGURE 10. (A) The latitude-altitude cross section of CO flux difference (in ppbv m s−1) between El Niño and La Niña years in MAM along the western border of the NAE (60°W). (B) The same as (A), but along the eastern border of the NAE (50°E). The dashed vertical lines at 30°N and 60°N indicate the latitudinal borders of the NAE. Positive flux is from west to east.
Supplementary Figure S10 shows the horizontal CO flux at 850 hPa. The arrows perpendicular to the four borders of the NAE indicate the direction of CO flux. On average (Supplementary Figure S10A), only the western border of NAE experiences CO inflow, while the other three borders experience CO outflow, their sum implying CO flux divergence over the NAE. However, when the differences between El Niño and La Niña years are considered, only the northern border experience CO outflow, while the other borders experience CO inflow. The total horizontal CO flux difference between El Niño and La Niña years is 28 ppbv m s−1 (about 5.0% of the mean over 2002–2019). Meanwhile, the upward CO flux over the North Atlantic (15 ppbv Pa s−1, about 241.3% of the mean over 2002–2019) and the downward CO flux over Europe (−6 ppbv Pa s−1, about 6.5% of the mean over 2002–2019) are also strengthened. The positive horizontal and vertical CO fluxes lead to CO accumulation over the NAE.
We further assess CO anomalies in the NAE under El Niño and La Niña conditions and partition the CO anomalies by source region, using GEOS-Chem simulations (Supplementary Table S3). At 850 hPa, the total of CO concentration from all regions is 109 ppbv at 850 hPa. CO anomalies are 2.5 ppbv (2.3% of the mean) in El Niño years and −1.1 ppbv (−1.0% of the mean) in La Niña years. Although of the same signs, the simulated CO anomalies are lower than the observed ones shown in Table 1. In the Northern Hemisphere, ENSO influences on CO transported from NHSA is largest (39% of the mean in El Niño years, the same as below), followed by that from SEAS (24%), and NA (7%). The case at 400 hPa is rather similar to that at 850 hPa.
Overall, positive ENSO events can enhance upward and northeastward transport of CO from SEAS to higher altitudes and middle latitudes, and increase northward transport of CO from NHSA toward the Atlantic. The westerlies between 20°N and 50°N become stronger, especially over the Pacific and the Atlantic at the upper troposphere. Furthermore, the upward motion along the transport pathway over the Atlantic is enhanced, and so is the downward motion over Europe. In addition, the outflow along the eastern border of the NAE is weakened. All these variations explain why the ENSO correlation with CO in the NAE is the highest in the middle to upper troposphere around 400 hPa (Table 1).
To identify the relative importance of ENSO impacts on CO emissions and CO transport, we partition CO flux into CO concentration and wind speed (Eq. 3). Also, CO concentration can be partitioned into the mean state CO (
We define
FIGURE 11. (A) CO flux difference (unit: ppbv m s−1) in CO anomaly between El Niño and La Niña years in MAM at 400 hPa. (B) CO flux difference in wind anomaly between El Niño and La Niña years in MAM at 400 hPa. (C) The same as (A), but at 850 hPa. (D) The same as (B), but at 850 hPa. See Eq. 3 for the term definitions. The white areas in 850 hPa are due to topography.
Besides ENSO, there are other climate modes that may affect CO concentrations in the NAE, such as NAO and AMO. Previous studies have found that NAO could affect pollution transport over the Atlantic (Li et al., 2002; Creilson et al., 2003; Christoudias et al., 2012; Bacer et al., 2016). Pope et al. (2018) stated that under the positive NAO, the enhanced westerlies will enhance the outflow of the primary pollutants such as CO from Europe, while during the negative NAO, more stable meteorological conditions will lead to a build-up of pollutants in Europe. However, secondary pollutants such as ozone show the opposite signal, the positive NAO will introduce more Atlantic ozone-enriched air into Europe, resulting in an increase in European ozone concentrations. Both NAO and AMO can affect the climate in the NAE (Rodó et al., 1997; Enfield et al., 2001; Knippertz et al., 2003; Sutton and Hodson, 2005; Kushnir et al., 2010) and may regulate or co-vary with ENSO (Huang et al., 1998; Knippertz et al., 2003; McCabe et al., 2004; García-García and Ummenhofer, 2015; Hardiman et al., 2019; Johnson et al., 2020). Table 4A shows correlations between NAO or AMO in winters to CO concentrations over the NAE in the following springs. The correlations are mostly positive for NAO and negative for AMO through the tropospheric column, but non-significant statistically. We also correlate the two data series without time lags and no significant correction is found (Table 4B).
TABLE 4. (A) Correlation coefficients (r) between climate mode (NAO, AMO, TNA SST, PNA, C-Index, and E-Index) indices and detrended CO concentrations in MAM over the NAE at different altitudes. All the climate indices are averaged from November of year 0 to February of year 1 (NDJF). (B) The same as (A), but all the climate indices are averaged from March to May (MAM) i.e. (A) Correlation between climate mode indices and CODT_NAE with a time lag. (B) Correlation between climate mode indices and CODT_NAE without a time lag.
PNA can greatly influence the climate and dynamics over the North America (Leathers et al., 1991; Guan et al., 2013; Harding and Snyder, 2015; Feng et al., 2016). The positive-PNA pattern is usually characterized as below-normal geopotential heights in the North Pacific and the south–eastern United States and above-normal geopotential heights over western Canada (Horel and Wallace, 1981). It has been suggested that ENSO-related circulation anomalies in mid-high latitudes in the middle troposphere resemble the PNA pattern (Horel and Wallace, 1981; van Loon and Madden, 1981). However, Straus and Shukla (2002) pointed out that ENSO is not just related to the PNA circulation pattern. Li et al. (2019) stated that ENSO can only explain 29% of the variance in PNA, while other processes, including atmospheric internal dynamics and sea surface temperature variations in the North Pacific, explain the remaining 71%. This study finds that in El Niño years, geopotential height anomalies resemble the positive-PNA pattern. ENSO can influence the atmospheric circulation over the western flank of the NAE (or the north eastern America) through PNA and introduce more CO from north America to the NAE (Supplementary Figure S10). Nevertheless, PNA is not fully determined by ENSO; ENSO impact on the NAE CO is not limited to enhanced CO transport over the western flank of NAE. Therefore, there is moderately positive but non-significant connection between wintertime PNA and the IAV in spring CO over the NAE (Table 4A).
Overall, although the NAO, AMO, PNA, and TNA SST can affect springtime CO concentrations over the NAE in some particular years, these modes show no clear connection to the CO IAV in spring over the NAE with or without a time lag. (Table 4).
Recent studies have paid attention to ENSO diversity and suggest that ENSO has two different types: eastern Pacific (EP) ENSO and central Pacific (CP) ENSO (Ashok et al., 2007; Kao and Yu, 2009; Takahashi et al., 2011), which may have distinct impacts on climate and atmospheric circulation (Yu et al., 2012; Zhang et al., 2014; Paek et al., 2016; Ding et al., 2017; Paek et al., 2017; Xie et al., 2018; Yu and Zhang, 2018; Peng et al., 2019; Peng et al., 2020). There appears a shift to a higher frequency of CP ENSO recently (Lee and McPhaden, 2010; Yu et al., 2012). By using Eqs 3 and 4 in Takahashi et al. (2011), we obtain C-index and E-index values to represent CP ENSO and EP ENSO respectively. The teleconnection of ENSO and NAE CO IAV over 2002–2019 is attributable to CP ENSO more than to EP ENSO (Table 4).
To examine if there are any differences in dynamical mechanisms for the ENSO-CO relationship between the two types of ENSO years, we extend the time series backward to 1983, thus obtain a study period from 1983 to 2019, to have more samples for EP/CP ENSO events, following the method in Jiang and Li (2022). In total, there are three EP El Niño years (1983, 1998, and 2016) and four CP El Niño years (1995, 2003, 2005, and 2010). The total COBB emissions from SEAS, NHSA and NA increased in both types of the ENSO years. In CP El Niño years (Supplementary Figure S11), all the five processes discussed for Figures 7–9 are present, with two differences. First, the upward, northward, and eastward motions over SEAS are elevated, but with lower magnitudes. Second, the inflows to the north eastern America related to PNA disappear, which may weaken CO inflows to the NAE from BONA. In EP El Niño years (Supplementary Figure S12), Processes 1–3, and 5 are similar to these in CP El Niño years. However, Process 4 (enhancing ascents over the Atlantic, descents over the Europe and horizontal transport from Florida to Europe) does not happen or becomes opposite, which may explain why the teleconnection of ENSO to the CO IAV in the NAE appears stronger in CP ENSO years than in EP ENSO years.
This study suggests that ENSO is an important factor that drivers IAVs in air temperature and precipitation, but not the only one. For example, Kushnir et al. (2010) found that during the cold season (October-March), the North American precipitation variability, associated with the TNA SST fluctuations, is considerably weaker than its association with ENSO. During the warm season (April-September), however, the Atlantic influence, per standard deviation of SST anomalies, is larger than that of ENSO. Moreover, similar to TNA SST, AMO, possibly covarying with ENSO (McCabe et al., 2004; García-García and Ummenhofer, 2015; Johnson et al., 2020), can also affect air temperature and precipitation over North America and Europe (Enfield et al., 2001; Sutton and Hodson, 2005; Kushnir et al., 2010). Seager et al. (2014) and Pu et al. (2016) found that precipitation in the Southern Great Plains or the southern United States and northern Mexico (TENA in this study) is affected by ENSO, NAO, and TNA SST. In TENA, El Niño episodes and warm TNA SST can co-occur, while La Niña episodes can co-occur with colder TNA SST. As precipitation responds to ENSO and TNA SST in opposite ways, the variation in precipitation in TENA is a compromise between ENSO and TNA SST. The La Niña phase, negative NAO, and warm TNA SST are all factors causing the 2011 TENA drought, resulting in the abnormally high COBB emission in NA in 2011 (Figure 4B).
The long-term variations in MAM CO concentrations at 850 hPa in the NAE show a significant downward trend (Supplementary Figure S13A, Table 1), which correlates well with the long-term variation in anthropogenic CO sources in the NAE. Declining trends in tropospheric CO have been reported on global and regional scales, i.e., Worden et al. (2013), Petetin et al. (2015), Osman et al. (2016), Jiang et al. (2017) and Zhang et al. (2020). Previous studies suggested that the decline of anthropogenic CO emissions may be one of the reasons for the decrease of tropospheric CO concentrations in recent decades (Granier, et al., 2011; Zheng, et al., 2019). We find that 74% of the variation in CO in the troposphere can be explained by anthropogenic emissions in the NAE alone, while the ENSO index explains less (13%). However, if the decreasing trend in the CO concentrations is removed, the ENSO effect becomes dominant and can explain 35% of the variation in CODT (Supplementary Figure S13B), while anthropogenic emission variations explain little (1%). Overall, the reduction in anthropogenic CO emissions in the NAE would be one of the main reasons for the declining trend in CO concentrations in the lower and middle troposphere over the NAE, while ENSO plays a dominant role in modulating the IAV in CO concentrations there.
We report an ENSO teleconnection to the IAV in tropospheric CO concentrations over the NAE in spring (MAM). Our finding is mainly based on the analysis of trajectory-mapped IAGOS CO data, with support from ground-level CO observations. Using a chemical transport model, GEOS-Chem, we track CO transport to the NAE from different regions with fire and anthropogenic sources. Through analysis of GFED4.1s fire emission data and NOAA /NCEP meteorological data, we investigate the underlying mechanisms for the teleconnection. The primary conclusions are summarized below.
The IAV in springtime CO concentrations over the NAE is positively correlated with the ENSO index in the previous winter from the surface to the upper troposphere with high confidence (on average over the NAE, r = 0.5–0.6, p < 0.1). CO concentrations in spring over the NAE increase in El Niño years and decrease in La Niña years. Among all altitudes, the highest correlation is around 400 hPa. In the lower troposphere, the positive correlation is strongest over the central North Atlantic, while in the upper troposphere, the maximum correlation shifts to central Europe and the Mediterranean. That is, moving from the surface to the upper troposphere, the positive correlation shifts eastward. The positive correlation between CO concentrations in the NAE and ENSO is also found to be significant in ground-level CO measurements, MOPITT satellite data, and GEOS-Chem model simulations. This teleconnection appears stronger in CP ENSO years than in EP ENSO years.
We find this ENSO-CO teleconnection over the NAE is established through ENSO modulation of: 1) CO emissions from fire regions and 2) atmospheric circulation that facilitates CO transport from the rest of the world, including fire-regions, to the NAE. In El Niño years, surface air temperature increases and precipitation decreases, leading to more fire activity, especially in SEAS, NA, and NHSA. Consequently, COBB emissions in SEAS, NA, and NHSA all increase. The sum of CO emissions in the three regions corresponds strongly to ENSO with r = 0.72 and p < 0.02. Directly, the sum of the COBB emissions in the three regions positively correlates with IAV in CO concentrations over the NAE, most strongly at 400 hPa. We identify the following processes that ENSO can modulate CO transport: 1) elevating upward, northward, and eastward motions in fire regions over SEAS, 2) strengthening westerlies around 10°N–50°N, 3) enhancing northward motion over NHSA fire region, 4) enhancing ascents over the Atlantic and descents over Europe, and 5) reducing outflow from the eastern border of the NAE. We further assess that ENSO-induced anomalies in CO emission and CO transport have different spatial variations in the Northern Hemispheric troposphere. The two anomalies are with the same magnitude at the upper troposphere. However, stronger ENSO influence on COBB emissions than on CO transport appears in the lower troposphere.
ENSO influence on IAV in atmospheric CO is complicated because of complexity of both ENSO and CO. ENSO-CO connections have been explored in the equator or the Southern Hemisphere (Huang et al., 2014; Buchholz et al., 2018; Ribeiro et al., 2020). Some studies were focused on one or few extreme events or within a short period of time (Duncan et al., 2003; Edwards et al., 2006b; Szopa et al., 2007; Seager et al., 2014). Some studies were based on surface observations at sites (Ribeiro et al., 2020) or satellite observations (Edwards et al., 2006b; Zhang, 2011; Buchholz et al., 2018). Atmospheric chemical models have also been used (Szopa et al., 2007; Koumoutsaris et al., 2008; Monks et al., 2012; Ding et al., 2015; Voulgarakis et al., 2015; Rowlinson et al., 2019). Observational data can be site-specific or have low vertical sensitivity, while models can suffer from biases induced by input data, coarse resolution, and model parameterization. Here, by using trajectory-mapped airborne CO data, we are able to show the ENSO-CO connection over a large area with unprecedented vertical and horizontal detail. This level of detail on CO variability, vertically and horizontally, is not generally available from observation-based datasets. As the analysis is based on an 18-years observational dataset, our conclusions are reasonably robust.
Previous studies often attributed ENSO modulation of CO concentrations of a region to local or nearby fires (Monks et al., 2012; Ribeiro et al., 2020). This study shows that in fact, ENSO induces little perturbation in local fire activity in the NAE. It is the ENSO influence on fires distant from SEAS, NHSA, and NA that is seen in CO IAV in the NAE. We find that ENSO affects both CO emissions from fires and CO transport from distant fire regions, not just one of them. These new understandings of ENSO regulation of CO interannual variability can be beneficial to CO prediction in mid to high latitudes when an ENSO event occurs.
The original contributions presented in the study are included in the article/Supplementary Material, further inquiries can be directed to the corresponding author.
YL conducted the research, prepared the figures and tables, and wrote the first draft of the manuscript. YL and JL developed the research ideas and methodology, and completed the analysis. JL conceived and supervised the study, and revised the manuscript. All coauthors contributed substantially to the data, methodology, and analysis. DT and all coauthors provided valuable input to the revision and approved the submission of the paper.
This research was funded by the Chinese Ministry of Science and Technology National Key R&D Program of China (Grant no. 2019YFA0606803) and National Natural Science Foundation of China (Grant no. 41971022).
The authors declare that the research was conducted in the absence of any commercial or financial relationships that could be construed as a potential conflict of interest.
All claims expressed in this article are solely those of the authors and do not necessarily represent those of their affiliated organizations, or those of the publisher, the editors and the reviewers. Any product that may be evaluated in this article, or claim that may be made by its manufacturer, is not guaranteed or endorsed by the publisher.
We thank the European Research Infrastructures programme for providing the IAGOS CO data, WDCGG and GML for the ground-level CO observations, GFED for the fire emission data, Pacific Northwest National Laboratory (PNNL) for the CEDS anthropogenic emission data, the National Oceanic and Atmospheric Administration (NOAA) and National Centers for Environmental Prediction (NCEP) for the meteorological reanalysis, the National Aeronautics and Space Administration (NASA)’s MOPITT team for the satellite CO data. We are grateful to Harvard University for their open-source GEOS-Chem model. We thank the comments and suggestions from the reviewers and editor, which greatly help us improve this paper. Nanjing University and Fujian Normal University provided computing resources.
The Supplementary Material for this article can be found online at: https://www.frontiersin.org/articles/10.3389/fenvs.2022.894779/full#supplementary-material
Anderson, D. C., Duncan, B. N., Fiore, A. M., Baublitz, C. B., Follette-Cook, M. B., Nicely, J. M., et al. (2021). Spatial and Temporal Variability in the Hydroxyl (OH) Radical: Understanding the Role of Large-Scale Climate Features and Their Influence on OH through its Dynamical and Photochemical Drivers. Atmos. Chem. Phys. 21 (8), 6481–6508. doi:10.5194/acp-21-6481-2021
Arellano, A. F., Hess, P. G., Edwards, D. P., and Baumgardner, D. (2010). Constraints on Black Carbon Aerosol Distribution from Measurement of Pollution in the Troposphere (MOPITT) CO. Geophys. Res. Lett. 37 (L17). doi:10.1029/2010gl044416
Ashok, K., Behera, S. K., Rao, S. A., Weng, H., and Yamagata, T. (2007). El Niño Modoki and its Possible Teleconnection. J. Geophys. Res. 112 (C11). doi:10.1029/2006jc003798
Bacer, S., Christoudias, T., and Pozzer, A. (2016). Projection of North Atlantic Oscillation and its Effect on Tracer Transport. Atmos. Chem. Phys. 16 (24), 15581–15592. doi:10.5194/acp-16-15581-2016
Bian, H., Chin, M., Kawa, S. R., Yu, H., Diehl, T., and Kucsera, T. (2010). Multiscale Carbon Monoxide and Aerosol Correlations from Satellite Measurements and the GOCART Model: Implication for Emissions and Atmospheric Evolution. J. Geophys. Res. 115 (D7). doi:10.1029/2009jd012781
Bowman, K. P. (2006). Transport of Carbon Monoxide from the Tropics to the Extratropics. J. Geophys. Res. 111 (D2). doi:10.1029/2005jd006137
Bradley, R. S., Diaz, H. F., Kiladis, G. N., and Eischeid, J. K. (1987). ENSO Signal in Continental Temperature and Precipitation Records. Nature 327 (6122), 497–501. doi:10.1038/327497a0
Bretherton, C. S., Widmann, M., Dymnikov, V. P., Wallace, J. M., and Bladé, I. (1999). The Effective Number of Spatial Degrees of Freedom of a Time-Varying Field. J. Clim. 12 (7), 1990–2009. doi:10.1175/1520-0442(1999)012<1990:tenosd>2.0.co;2
Brönnimann, S. (2007). Impact of El Niño-Southern Oscillation on European Climate. Rev. Geophys. 45 (3). doi:10.1029/2006rg000199
Buchholz, R. R., Hammerling, D., Worden, H. M., Deeter, M. N., Emmons, L. K., Edwards, D. P., et al. (2018). Links between Carbon Monoxide and Climate Indices for the Southern Hemisphere and Tropical Fire Regions. J. Geophys. Res. Atmos. 123 (17), 9786–9800. doi:10.1029/2018jd028438
Cai, W., McPhaden, M. J., Grimm, A. M., Rodrigues, R. R., Taschetto, A. S., Garreaud, R. D., et al. (2020). Climate Impacts of the El Niño-Southern Oscillation on South America. Nat. Rev. Earth Environ. 1 (4), 215–231. doi:10.1038/s43017-020-0040-3
Chen, M., Xie, P., Janowiak, J. E., and Arkin, P. A. (2002). Global Land Precipitation: A 50-yr Monthly Analysis Based on Gauge Observations. J. Hydrometeor 3 (3), 249–266. doi:10.1175/1525-7541(2002)003<0249:glpaym>2.0.co;2
Chen, Y., Morton, D. C., Andela, N., van der Werf, G. R., Giglio, L., and Randerson, J. T. (2017). A Pan-Tropical Cascade of Fire Driven by El Niño/Southern Oscillation. Nat. Clim. Change 7 (12), 906–911. doi:10.1038/s41558-017-0014-8
Christoudias, T., Pozzer, A., and Lelieveld, J. (2012). Influence of the North Atlantic Oscillation on Air Pollution Transport. Atmos. Chem. Phys. 12 (2), 869–877. doi:10.5194/acp-12-869-2012
Creilson, J. K., Fishman, J., and Wozniak, A. E. (2003). Intercontinental Transport of Tropospheric Ozone: a Study of its Seasonal Variability across the North Atlantic Utilizing Tropospheric Ozone Residuals and its Relationship to the North Atlantic Oscillation. Atmos. Chem. Phys. 3 (6), 2053–2066. doi:10.5194/acp-3-2053-2003
Cussac, M., Marécal, V., Thouret, V., Josse, B., and Sauvage, B. (2020). The Impact of Biomass Burning on Upper Tropospheric Carbon Monoxide: a Study Using MOCAGE Global Model and IAGOS Airborne Data. Atmos. Chem. Phys. 20 (15), 9393–9417. doi:10.5194/acp-20-9393-2020
Daniau, A. L., Bartlein, P. J., Harrison, S. P., Prentice, I. C., Brewer, S., Friedlingstein, P., et al. (2012). Predictability of Biomass Burning in Response to Climate Changes. Glob. Biogeochem. Cycles 26, Gb4007. doi:10.1029/2011gb004249
Ding, K., Liu, J., Ding, A., Liu, Q., Zhao, T. L., Shi, J., et al. (2015). Uplifting of Carbon Monoxide from Biomass Burning and Anthropogenic Sources to the Free Troposphere in East Asia. Atmos. Chem. Phys. 15 (5), 2843–2866. doi:10.5194/acp-15-2843-2015
Ding, S., Chen, W., Feng, J., and Graf, H.-F. (2017). Combined Impacts of PDO and Two Types of La Niña on Climate Anomalies in Europe. J. Clim. 30 (9), 3253–3278. doi:10.1175/jcli-d-16-0376.1
Dlugokencky, E. J., Myers, R. C., Lang, P. M., Masarie, K. A., Crotwell, A. M., Thoning, K. W., et al. (2005). Conversion of NOAA Atmospheric Dry Air CH4mole Fractions to a Gravimetrically Prepared Standard Scale. J. Geophys. Res. 110, D18306. doi:10.1029/2005jd006035
Doherty, R. M., Stevenson, D. S., Johnson, C. E., Collins, W. J., and Sanderson, M. G. (2006). Tropospheric Ozone and El Niño–Southern Oscillation: Influence of Atmospheric Dynamics, Biomass Burning Emissions, and Future Climate Change. J. Geophys. Res. 111 (D19), 1–21. doi:10.1029/2005jd006849
Duncan, B. N., Logan, J. A., Bey, I., Megretskaia, I. A., Yantosca, R. M., Novelli, P. C., et al. (2007). Global Budget of CO, 1988–1997: Source Estimates and Validation with a Global Model. J. Geophys. Res. 112 (D22). doi:10.1029/2007jd008459
Duncan, B. N., and Logan, J. A. (2008). Model Analysis of the Factors Regulating the Trends and Variability of Carbon Monoxide between 1988 and 1997. Atmos. Chem. Phys. 8 (24), 7389–7403. doi:10.5194/acp-8-7389-2008
Duncan, B. N., Martin, R. V., Staudt, A. C., Yevich, R., and Logan, J. A. (2003). Interannual and Seasonal Variability of Biomass Burning Emissions Constrained by Satellite Observations. J. Geophys. Res. 108 (D2), 1–22. doi:10.1029/2002jd002378
Earth Observatory (2014). Fires in Southeast Asia. Available at: https://earthobservatory.nasa.gov/images/83365/fires-in-southeast-asia (Accessed February 16, 2022).
Edwards, D. P., Emmons, L. K., Gille, J. C., Chu, A., Attié, J.-L., Giglio, L., et al. (2006a). Satellite-observed Pollution from Southern Hemisphere Biomass Burning. J. Geophys. Res. 111 (D14). doi:10.1029/2005jd006655
Edwards, D. P., Emmons, L. K., Hauglustaine, D. A., Chu, D. A., Gille, J. C., Kaufman, Y. J., et al. (2004). Observations of Carbon Monoxide and Aerosols from the Terra Satellite: Northern Hemisphere Variability. J. Geophys. Res. 109 (D24). doi:10.1029/2004jd004727
Edwards, D. P., Pétron, G., Novelli, P. C., Emmons, L. K., Gille, J. C., and Drummond, J. R. (2006b). Southern Hemisphere Carbon Monoxide Interannual Variability Observed by Terra/Measurement of Pollution in the Troposphere (MOPITT). J. Geophys. Res. 111 (D16). doi:10.1029/2006jd007079
Enfield, D. B., Mestas-Nuñez, A. M., and Trimble, P. J. (2001). The Atlantic Multidecadal Oscillation and its Relation to Rainfall and River Flows in the Continental U.S. Geophys. Res. Lett. 28 (10), 2077–2080. doi:10.1029/2000gl012745
Fang, K., Yao, Q., Guo, Z., Zheng, B., Du, J., Qi, F., et al. (2021). ENSO Modulates Wildfire Activity in China. Nat. Commun. 12 (1), 1764. doi:10.1038/s41467-021-21988-6
Feely, R. A., Gammon, R. H., Taft, B. A., Pullen, P. E., Waterman, L. S., Conway, T. J., et al. (1987). Distribution of Chemical Tracers in the Eastern Equatorial Pacific during and after the 1982-1983 El Niño/Southern Oscillation Event. J. Geophys. Res. 92 (C6), 6545. doi:10.1029/JC092iC06p06545
Feng, J., Liao, H., and Li, J. (2016). The Impact of Monthly Variation of the Pacific–North America (PNA) Teleconnection Pattern on Wintertime Surface-Layer Aerosol Concentrations in the United States. Atmos. Chem. Phys. 16 (8), 4927–4943. doi:10.5194/acp-16-4927-2016
Ferrer, N., Folch, A., Lane, M., Olago, D., Odida, J., and Custodio, E. (2019). Groundwater Hydrodynamics of an Eastern Africa Coastal Aquifer, Including La Niña 2016-17 Drought. Sci. Total Environ. 661, 575–597. doi:10.1016/j.scitotenv.2019.01.198
Field, R. D., and Shen, S. S. P. (2008). Predictability of Carbon Emissions from Biomass Burning in Indonesia from 1997 to 2006. J. Geophys. Res. 113 (G4). doi:10.1029/2008jg000694
Fuller, D. O., and Murphy, K. (2006). The ENSO-Fire Dynamic in Insular Southeast Asia. Clim. Change 74 (4), 435–455. doi:10.1007/s10584-006-0432-5
García-García, D., and Ummenhofer, C. C. (2015). Multidecadal Variability of the Continental Precipitation Annual Amplitude Driven by AMO and ENSO. Geophys. Res. Lett. 42 (2), 526–535. doi:10.1002/2014gl062451
Gelaro, R., McCarty, W., Suárez, M. J., Todling, R., Molod, A., Takacs, L., et al. (2017). The Modern-Era Retrospective Analysis for Research and Applications, Version 2 (MERRA-2). J. Clim. 30 (13), 5419–5454. doi:10.1175/JCLI-D-16-0758.1
Giglio, L., Randerson, J. T., and van der Werf, G. R. (2013). Analysis of Daily, Monthly, and Annual Burned Area Using the Fourth-Generation Global Fire Emissions Database (GFED4). J. Geophys. Res. Biogeosci. 118 (1), 317–328. doi:10.1002/jgrg.20042
Granier, C., Bessagnet, B., Bond, T., D’Angiola, A., Denier van der Gon, H., Frost, G. J., et al. (2011). Evolution of Anthropogenic and Biomass Burning Emissions of Air Pollutants at Global and Regional Scales during the 1980-2010 Period. Clim. Change 109 (1-2), 163–190. doi:10.1007/s10584-011-0154-1
Guan, B., Molotch, N. P., Waliser, D. E., Fetzer, E. J., and Neiman, P. J. (2013). The 2010/2011 Snow Season in California's Sierra Nevada: Role of Atmospheric Rivers and Modes of Large-Scale Variability. Water Resour. Res. 49 (10), 6731–6743. doi:10.1002/wrcr.20537
Han, H., Liu, J., Yuan, H., Jiang, F., Zhu, Y., Wu, Y., et al. (2018). Impacts of Synoptic Weather Patterns and Their Persistency on Free Tropospheric Carbon Monoxide Concentrations and Outflow in Eastern China. J. Geophys. Res. Atmos. 123 (13), 7024–7046. doi:10.1029/2017jd028172
Hardiman, S. C., Dunstone, N. J., Scaife, A. A., Smith, D. M., Ineson, S., Lim, J., et al. (2019). The Impact of Strong El Niño and La Niña Events on the North Atlantic. Geophys. Res. Lett. 46 (5), 2874–2883. doi:10.1029/2018gl081776
Harding, K. J., and Snyder, P. K. (2015). The Relationship between the Pacific–North American Teleconnection Pattern, the Great Plains Low-Level Jet, and North Central U.S. Heavy Rainfall Events*. J. Clim. 28 (17), 6729–6742. doi:10.1175/jcli-d-14-00657.1
Heald, C. L., Jacob, D. J., Fiore, A. M., Emmons, L. K., Gille, J. C., Deeter, M. N., et al. (2003). Asian Outflow and Trans-Pacific Transport of Carbon Monoxide and Ozone Pollution: An Integrated Satellite, Aircraft, and Model Perspective. J. Geophys. Res. 108 (D24). doi:10.1029/2003jd003507
Herceg-Bulić, I., and Kucharski, F. (2012). Delayed ENSO Impact on Spring Precipitation over North/Atlantic European Region. Clim. Dyn. 38 (11-12), 2593–2612. doi:10.1007/s00382-011-1151-9
Herceg-Bulić, I., Mezzina, B., Kucharski, F., Ruggieri, P., and King, M. P. (2017). Wintertime ENSO Influence on Late Spring European Climate: the Stratospheric Response and the Role of North Atlantic SST. Int. J. Climatol. 37, 87–108. doi:10.1002/joc.4980
Hertig, E., Beck, C., Wanner, H., and Jacobeit, J. (2015). A Review of Non-stationarities in Climate Variability of the Last Century with Focus on the North Atlantic-European Sector. Earth-Science Rev. 147, 1–17. doi:10.1016/j.earscirev.2015.04.009
Hoesly, R. M., Smith, S. J., Feng, L., Klimont, Z., Janssens-Maenhout, G., Pitkanen, T., et al. (2018). Historical (1750–2014) Anthropogenic Emissions of Reactive Gases and Aerosols from the Community Emissions Data System (CEDS). Geosci. Model Dev. 11 (1), 369–408. doi:10.5194/gmd-11-369-2018
Holloway, T., Levy, H., and Kasibhatla, P. (2000). Global Distribution of Carbon Monoxide. J. Geophys. Res. 105(D10), 12123–12147. doi:10.1029/1999jd901173
Horel, J. D., and Wallace, J. M. (1981). Planetary-scale Atmospheric Phenomena Associated with the Southern Oscillation. Mon. Wea. Rev. 109 (4), 813–829. doi:10.1175/1520-0493(1981)109<0813:psapaw>2.0.co;2
Hu, Z.-Z., Kumar, A., Xue, Y., and Jha, B. (2013). Why Were Some La Niñas Followed by Another La Niña? Clim. Dyn. 42 (3-4), 1029–1042. doi:10.1007/s00382-013-1917-3
Huang, J., Higuchi, K., and Shabbar, A. (1998). The Relationship between the North Atlantic Oscillation and El Niño-Southern Oscillation. Geophys. Res. Lett. 25 (14), 2707–2710. doi:10.1029/98gl01936
Huang, L., Fu, R., and Jiang, J. H. (2014). Impacts of Fire Emissions and Transport Pathways on the Interannual Variation of CO in the Tropical Upper Troposphere. Atmos. Chem. Phys. 14 (8), 4087–4099. doi:10.5194/acp-14-4087-2014
Huntrieser, H., and Schlager, H. (2004). “Air Pollution Export from and Import to Europe: Experimental Evidence” in The Handbook of Environmental Chemistry Vol. 4, Part G. Intercontinental Transport of Air Pollution, editor by A. Stohl. (Berlin, Heidelberg: Springer), 69–98. doi:10.1007/b10681
Jacob, D. J., Crawford, J. H., Kleb, M. M., Connors, V. S., Bendura, R. J., Raper, J. L., et al. (2003). Transport and Chemical Evolution over the Pacific (TRACE-P) Aircraft Mission: Design, Execution, and First Results. J. Geophys. Res. 108 (D20). doi:10.1029/2002jd003276
Jacob, D. J. (2000). Introduction to Atmospheric Chemistry. Princeton, New Jersey: Princeton University Press. doi:10.1515/9781400841547
Jiang, Z., and Li, J. (2022). Impact of Eastern and Central Pacific El Niño on Lower Tropospheric Ozone in China. Atmos. Chem. Phys. 22 (11), 7273–7285. doi:10.5194/acp-22-7273-2022
Jiang, Z., Worden, J. R., Worden, H., Deeter, M., Jones, D. B. A., Arellano, A. F., et al. (2017). A 15-year Record of CO Emissions Constrained by MOPITT CO Observations. Atmos. Chem. Phys. 17 (7), 4565–4583. doi:10.5194/acp-17-4565-2017
Johnson, Z. F., Chikamoto, Y., Wang, S.-Y. S., McPhaden, M. J., and Mochizuki, T. (2020). Pacific Decadal Oscillation Remotely Forced by the Equatorial Pacific and the Atlantic Oceans. Clim. Dyn. 55 (3-4), 789–811. doi:10.1007/s00382-020-05295-2
Jones, C. D., Collins, M., Cox, P. M., and Spall, S. A. (2001). The Carbon Cycle Response to ENSO: A Coupled Climate-Carbon Cycle Model Study. J. Clim. 14 (21), 4113–4129. doi:10.1175/1520-0442(2001)014<4113:tccrte>2.0.co;2
Joshi, M. K., Abid, M. A., and Kucharski, F. (2021). The Role of an Indian Ocean Heating Dipole in the ENSO Teleconnection to the North Atlantic European Region in Early Winter during the Twentieth Century in Reanalysis and CMIP5 Simulations. J. Clim. 34 (3), 1047–1060. doi:10.1175/jcli-d-20-0269.1
Kao, H.-Y., and Yu, J.-Y. (2009). Contrasting Eastern-Pacific and Central-Pacific Types of ENSO. J. Clim. 22 (3), 615–632. doi:10.1175/2008jcli2309.1
Kessler, W. S. (2002). Is ENSO a Cycle or a Series of Events? Geophys. Res. Lett. 29 (23), 40-1–40-44. doi:10.1029/2002gl015924
Knippertz, P., Ulbrich, U., Marques, F., and Corte-Real, J. (2003). Decadal Changes in the Link between El Niño and Springtime North Atlantic Oscillation and European-North African Rainfall. Int. J. Climatol. 23 (11), 1293–1311. doi:10.1002/joc.944
Kopacz, M., Jacob, D. J., Fisher, J. A., Logan, J. A., Zhang, L., Megretskaia, I. A., et al. (2010). Global Estimates of CO Sources with High Resolution by Adjoint Inversion of Multiple Satellite Datasets (MOPITT, AIRS, SCIAMACHY, TES). Atmos. Chem. Phys. 10 (3), 855–876. doi:10.5194/acp-10-855-2010
Koumoutsaris, S., Bey, I., Generoso, S., and Thouret, V. (2008). Influence of El Niño-Southern Oscillation on the Interannual Variability of Tropospheric Ozone in the Northern Midlatitudes. J. Geophys. Res. 113 (D19). doi:10.1029/2007jd009753
Kushnir, Y., Seager, R., Ting, M., Naik, N., and Nakamura, J. (2010). Mechanisms of Tropical Atlantic SST Influence on North American Precipitation Variability*. J. Clim. 23 (21), 5610–5628. doi:10.1175/2010jcli3172.1
Le Page, Y., Pereira, J. M. C., Trigo, R., da Camara, C., Oom, D., and Mota, B. (2008). Global Fire Activity Patterns (1996–2006) and Climatic Influence: an Analysis Using the World Fire Atlas. Atmos. Chem. Phys. 8 (7), 1911–1924. doi:10.5194/acp-8-1911-2008
Leathers, D. J., Yarnal, B., and Palecki, M. A. (1991). The Pacific/North American Teleconnection Pattern and United States Climate. Part I: Regional Temperature and Precipitation Associations. J. Clim. 4 (5), 517–528. doi:10.1175/1520-0442(1991)004<0517:tpatpa>2.0.co;2
Lee, T., and McPhaden, M. J. (2010). Increasing Intensity of El Niño in the Central-Equatorial Pacific. Geophys. Res. Lett. 37 (14). doi:10.1029/2010gl044007
Li, Q., Daniel, J. J., Bey, I., Palmer, P. I., Duncan, B. N., Field, B. D., et al. (2002). Transatlantic Transport of Pollution and its Effects on Surface Ozone in Europe and North America. J. Geophys. Res. 107 (D13), 1–21. doi:10.1029/2001jd001422
Li, T., and Hsu, P.-c. (2018). “Dynamics of El Niño-Southern Oscillation,” in Fundamentals of Tropical Climate Dynamics (Cham: Springer atmospheric sciences), 149–183. doi:10.1007/978-3-319-59597-9_5
Li, X., Hu, Z.-Z., Liang, P., and Zhu, J. (2019). Contrastive Influence of ENSO and PNA on Variability and Predictability of North American Winter Precipitation. J. Clim. 32 (19), 6271–6284. doi:10.1175/jcli-d-19-0033.1
Liu, G., Tarasick, D. W., Fioletov, V. E., Sioris, C. E., and Rochon, Y. J. (2009). Ozone Correlation Lengths and Measurement Uncertainties from Analysis of Historical Ozonesonde Data in North America and Europe. J. Geophys. Res. 114 (D4). doi:10.1029/2008jd010576
Liu, J., Drummond, J. R., Jones, D. B. A., Cao, Z., Bremer, H., Kar, J., et al. (2006). Large Horizontal Gradients in Atmospheric CO at the Synoptic Scale as Seen by Spaceborne Measurements of Pollution in the Troposphere. J. Geophys. Res. 111 (D2). doi:10.1029/2005jd006076
Liu, J., Drummond, J. R., Li, Q., Gille, J. C., and Ziskin, D. C. (2005). Satellite Mapping of CO Emission from Forest Fires in Northwest America Using MOPITT Measurements. Remote Sens. Environ. 95, 502–516. doi:10.1016/j.rse.2005.01.009
Liu, J. J., Jones, D. B. A., Worden, J. R., Noone, D., Parrington, M., and Kar, J. (2009). Analysis of the Summertime Buildup of Tropospheric Ozone Abundances over the Middle East and North Africa as Observed by the Tropospheric Emission Spectrometer Instrument. J. Geophys. Res. 114, D05304. doi:10.1029/2008JD010993
Liu, J., Logan, J. A., Murray, L. T., Pumphrey, H. C., Schwartz, M. J., and Megretskaia, I. A. (2013). Transport Analysis and Source Attribution of Seasonal and Interannual Variability of CO in the Tropical Upper Troposphere and Lower Stratosphere. Atmos. Chem. Phys. 13 (1), 129–146. doi:10.5194/acp-13-129-2013
Livesey, N. J., Logan, J. A., Santee, M. L., Waters, J. W., Doherty, R. M., Read, W. G., et al. (2013). Interrelated Variations of O3, CO and Deep Convection in the Tropical/subtropical Upper Troposphere Observed by the Aura Microwave Limb Sounder (MLS) during 2004–2011. Atmos. Chem. Phys. 13 (2), 579–598. doi:10.5194/acp-13-579-2013
Lorenzo, M. N., Taboada, J. J., Iglesias, I., and Gómez-Gesteira, M. (2010). Predictability of the Spring Rainfall in Northwestern Iberian Peninsula from Sea Surfaces Temperature of ENSO Areas. Clim. Change 107 (3-4), 329–341. doi:10.1007/s10584-010-9991-6
Lu, Y., and Khalil, M. A. K. (1991). Tropospheric OH: Model Calculations of Spatial, Temporal, and Secular Variations. Chemosphere 23 (3), 397–444. doi:10.1016/0045-6535(91)90194-i
Magaña, V., Vázquez, J. L., Pérez, J. L., and Pérez, J. B. (2003). Impact of El Niño on Precipitation in Mexico. Geofis. Int. 42 (3), 313–330. Available at: https://www.redalyc.org/articulo.oa?id=56842304
Marlon, J. R., Bartlein, P. J., Carcaillet, C., Gavin, D. G., Harrison, S. P., Higuera, P. E., et al. (2008). Climate and Human Influences on Global Biomass Burning over the Past Two Millennia. Nat. Geosci. 1 (10), 697–702. doi:10.1038/ngeo313
McCabe, G. J., Palecki, M. A., and Betancourt, J. L. (2004). Pacific and Atlantic Ocean Influences on Multidecadal Drought Frequency in the United States. Proc. Natl. Acad. Sci. U.S.A. 101 (12), 4136–4141. doi:10.1073/pnas.0306738101
McPhaden, M. J., Zebiak, S. E., and Glantz, M. H. (2006). ENSO as an Integrating Concept in Earth Science. Science 314 (5806), 1740–1745. doi:10.1126/science.1132588
Monks, S. A., Arnold, S. R., and Chipperfield, M. P. (2012). Evidence for El Niño-Southern Oscillation (ENSO) Influence on Arctic CO Interannual Variability through Biomass Burning Emissions. Geophys. Res. Lett. 39 (14). doi:10.1029/2012gl052512
Nédélec, P., Blot, R., Boulanger, D., Athier, G., Cousin, J.-M., Gautron, B., et al. (2015). Instrumentation on Commercial Aircraft for Monitoring the Atmospheric Composition on a Global Scale: the IAGOS System, Technical Overview of Ozone and Carbon Monoxide Measurements. Tellus B Chem. Phys. Meteorology 67 (1), 27791. doi:10.3402/tellusb.v67.27791
Neelin, J. D., Battisti, D. S., Hirst, A. C., Jin, F.-F., Wakata, Y., Yamagata, T., et al. (1998). ENSO Theory. J. Geophys. Res. 103 (C7), 14261–14290. doi:10.1029/97jc03424
Novelli, P. C., Masarie, K. A., and Lang, P. M. (1998). Distributions and Recent Changes of Carbon Monoxide in the Lower Troposphere. J. Geophys. Res. 103 (D15), 19015–19033. doi:10.1029/98jd01366
Novelli, P. C., Masarie, K. A., Lang, P. M., Hall, B. D., Myers, R. C., and Elkins, J. W. (2003). Reanalysis of Tropospheric CO Trends: Effects of the 1997-1998 Wildfires. J. Geophys. Res. 108 (D15). doi:10.1029/2002jd003031
Ohba, M., and Watanabe, M. (2012). Role of the Indo-Pacific Interbasin Coupling in Predicting Asymmetric ENSO Transition and Duration. J. Clim. 25 (9), 3321–3335. doi:10.1175/jcli-d-11-00409.1
Okumura, Y. M., and Deser, C. (2010). Asymmetry in the Duration of El Niño and La Niña. J. Clim. 23 (21), 5826–5843. doi:10.1175/2010jcli3592.1
Osman, M. K., Tarasick, D. W., Liu, J., Moeini, O., Thouret, V., Fioletov, V. E., et al. (2016). Carbon Monoxide Climatology Derived from the Trajectory Mapping of Global MOZAIC-IAGOS Data. Atmos. Chem. Phys. 16 (15), 10263–10282. doi:10.5194/acp-16-10263-2016
Paek, H., Yu, J.-Y., and Qian, C. (2017). Why Were the 2015/2016 and 1997/1998 Extreme El Niños Different? Geophys. Res. Lett. 44, 1848–1856. doi:10.1002/2016gl071515
Paek, H., Yu, J.-Y., Zheng, F., and Lu, M.-M. (2016). Impacts of ENSO Diversity on the Western Pacific and North Pacific Subtropical Highs during Boreal Summer. Clim. Dyn. 52 (12), 7153–7172. doi:10.1007/s00382-016-3288-z
Pechony, O., and Shindell, D. T. (2010). Driving Forces of Global Wildfires over the Past Millennium and the Forthcoming Century. Proc. Natl. Acad. Sci. U.S.A. 107 (45), 19167–19170. doi:10.1073/pnas.1003669107
Peng, Q., Xie, S.-P., Wang, D., Kamae, Y., Zhang, H., Hu, S., et al. (2020). Eastern Pacific Wind Effect on the Evolution of El Niño: Implications for ENSO Diversity. J. Clim. 33 (8), 3197–3212. doi:10.1175/jcli-d-19-0435.1
Peng, Q., Xie, S.-P., Wang, D., Zheng, X.-T., and Zhang, H. (2019). Coupled Ocean-Atmosphere Dynamics of the 2017 Extreme Coastal El Niño. Nat. Commun. 10 (1), 298. doi:10.1038/s41467-018-08258-8
Petetin, H., Thouret, V., Fontaine, A., Sauvage, B., Athier, G., Blot, R., et al. (2015). Characterizing Tropospheric Ozone and CO Around Frankfurt between 1994-2012 Based on MOZAIC-IAGOS Aircraft Measurements. Atmos. Chem. Phys. Discuss. 15, 23841–23891. doi:10.5194/acpd-15-23841-2015
Pope, R. J., Chipperfield, M. P., Arnold, S. R., Glatthor, N., Feng, W., Dhomse, S. S., et al. (2018). Influence of the Wintertime North Atlantic Oscillation on European Tropospheric Composition: an Observational and Modelling Study. Atmos. Chem. Phys. 18 (11), 8389–8408. doi:10.5194/acp-18-8389-2018
Prinn, R. G., Huang, J., Weiss, R. F., Cunnold, D. M., Fraser, P. J., Simmonds, P. G., et al. (2001). Evidence for Substantial Variations of Atmospheric Hydroxyl Radicals in the Past Two Decades. Science 292 (5523), 1882–1888. doi:10.1126/science.1058673
Pu, B., Fu, R., Dickinson, R. E., and Fernando, D. N. (2016). Why Do Summer Droughts in the Southern Great Plains Occur in Some La Niña Years but Not Others? J. Geophys. Res. Atmos. 121 (3), 1120–1137. doi:10.1002/2015jd023508
Pumphrey, H. C., Santee, M. L., Livesey, N. J., Schwartz, M. J., and Read, W. G. (2011). Microwave Limb Sounder Observations of Biomass-Burning Products from the Australian Bush Fires of February 2009. Atmos. Chem. Phys. 11 (13), 6285–6296. doi:10.5194/acp-11-6285-2011
Ramaswamy, V., Boucher, O., Haigh, J., Hauglustaine, D., Haywood, J., Myhre, G., et al. (2001). “Radiative Forcing of Climate Change,” in Climate Change 2001: The Scientific Basis. Contribution of Working Group I to the Third Assessment Report of the Intergovernmental Panel on Climate Change. J. T. Houghton, Y. Ding, D. J. Griggs, M. Noguer, P. J. van der Linden, X. Dai, K. Maskell,. Editorset al. (Cambridge, United Kingdom and New York: Cambridge University Press), 349–416.
Rasmusson, E. M., and Carpenter, T. H. (1982). Variations in Tropical Sea Surface Temperature and Surface Wind Fields Associated with the Southern Oscillation/El Niño. Mon. Wea. Rev. 110 (5), 354–384. doi:10.1175/1520-0493(1982)110<0354:vitsst>2.0.co;2
Ribeiro, I. O., do Santos, E. O., Batista, C. E., Fernandes, K. S., Ye, J., Medeiros, A. S., et al. (2020). Impact of Biomass Burning on a Metropolitan Area in the Amazon during the 2015 El Niño: The Enhancement of Carbon Monoxide and Levoglucosan Concentrations. Environ. Pollut. 260, 114029. doi:10.1016/j.envpol.2020.114029
Rodó, X., Baert, E., and Comín, F. A. (1997). Variations in Seasonal Rainfall in Southern Europe during the Present Century: Relationships with the North Atlantic Oscillation and the El Niño-Southern Oscillation. Clim. Dyn. 13 (4), 275–284. doi:10.1007/s003820050165
Rodríguez-Fonseca, B., Suárez-Moreno, R., Ayarzagüena, B., López-Parages, J., Gómara, I., Villamayor, J., et al. (2016). A Review of ENSO influence on the North Atlantic. A non-stationary signal. Atmosphere 7 (7), 87. doi:10.3390/atmos7070087
Ropelewski, C. F., and Halpert, M. S. (1986). North American Precipitation and Temperature Patterns Associated with the El Niño/Southern Oscillation (ENSO). Mon. Wea. Rev. 114 (12), 2352–2362. doi:10.1175/1520-0493(1986)114<2352:napatp>2.0.co;2
Rowlinson, M. J., Rap, A., Arnold, S. R., Pope, R. J., Chipperfield, M. P., McNorton, J., et al. (2019). Impact of El Niño-Southern Oscillation on the interannual variability of methane and tropospheric ozone. Atmos. Chem. Phys. 19 (13), 8669–8686. doi:10.5194/acp-19-8669-2019
Seager, R., Goddard, L., Nakamura, J., Henderson, N., and Lee, D. E. (2014). Dynamical Causes of the 2010/11 Texas-Northern Mexico Drought*. J. Hydrometeor 15 (1), 39–68. doi:10.1175/Jhm-D-13-024.1
Setimela, P., Gasura, E., Thierfelder, C., Zaman-Allah, M., Cairns, J. E., and Boddupalli, P. M. (2018). When the going gets tough: Performance of stress tolerant maize during the 2015/16 (El Niño) and 2016/17 (La Niña) season in southern Africa. Agric. Ecosyst. Environ. 268, 79–89. doi:10.1016/j.agee.2018.09.006
Shaman, J. (2014). The seasonal effects of ENSO on European precipitation: Observational analysis. J. Clim. 27 (17), 6423–6438. doi:10.1175/Jcli-D-14-00008.1
Stohl, A., Eckhardt, S., Forster, C., James, P., and Spichtinger, N. (2002). On the pathways and timescales of intercontinental air pollution transport. J.-Geophys.-Res. 107, 6–1. doi:10.1029/2001JD001396
Stohl, A., and Eckhardt, S. (2004), “Intercontinental transport of air pollution: An introduction”, in The Handbook of Environmental Chemistry Vol. 4, Part G. Intercontinental Transport of Air pollution, editor by A. Stohl. (Berlin, Heidelberg: Springer), 1–12. doi:10.1007/b10681
Straus, D. M., and Shukla, J. (2002). Does ENSO force the PNA? J. Clim. 15 (17), 2340–2358. doi:10.1175/1520-0442(2002)015<2340:deftp>2.0.co;2
Sutton, R. T., and Hodson, D. L. R. (2005). Atlantic Ocean forcing of North American and European summer climate. Science 309 (5731), 115–118. doi:10.1126/science.1109496
Szopa, S., Hauglustaine, D. A., and Ciais, P. (2007). Relative contributions of biomass burning emissions and atmospheric transport to carbon monoxide interannual variability. Geophys. Res. Lett. 34 (18). doi:10.1029/2007gl030231
Takahashi, K., Montecinos, A., Goubanova, K., and Dewitte, B. (2011). ENSO regimes: Reinterpreting the canonical and Modoki El Niño. Geophys. Res. Lett. 38 (10). doi:10.1029/2011gl047364
Thompson, A. M. (1992). The oxidizing capacity of the earth's atmosphere: probable past and future changes. Science 256 (5060), 1157–1165. doi:10.1126/science.256.5060.1157
Timmermann, A., An, S.-I., Kug, J.-S., Jin, F.-F., Cai, W., Capotondi, A., et al. (2018). El Niño-Southern Oscillation complexity. Nature 559 (7715), 535–545. doi:10.1038/s41586-018-0252-6
van der Werf, G. R., Randerson, J. T., Giglio, L., van Leeuwen, T. T., Chen, Y., Rogers, B. M., et al. (2017). Global fire emissions estimates during 1997-2016. Earth Syst. Sci. Data 9 (2), 697–720. doi:10.5194/essd-9-697-2017
van Loon, H., and Madden, R. A. (1981). The Southern Oscillation. part I: global associations with pressure and temperature in northern winter. Mon. Wea. Rev. 109 (6), 1150–1162. doi:10.1175/1520-0493(1981)109<1150:tsopig>2.0.co;2
van Oldenborgh, G. J., and Burgers, G. (2005). Searching for decadal variations in ENSO precipitation teleconnections. Geophys. Res. Lett. 32 (15). doi:10.1029/2005gl023110
Voulgarakis, A., Marlier, M. E., Faluvegi, G., Shindell, D. T., Tsigaridis, K., and Mangeon, S. (2015). Interannual variability of tropospheric trace gases and aerosols: The role of biomass burning emissions. J. Geophys. Res. Atmos. 120 (14), 7157–7173. doi:10.1002/2014jd022926
Webster, P. J., Magaña, V. O., Palmer, T. N., Shukla, J., Tomas, R. A., Yanai, M., et al. (1998). Monsoons: Processes, predictability, and the prospects for prediction. J. Geophys. Res. 103 (C7), 14451–14510. doi:10.1029/97jc02719
Wolter, K., and Timlin, M. S. (2011). El Niño/Southern Oscillation behaviour since 1871 as diagnosed in an extended multivariate ENSO index (MEI.ext). Int. J. Climatol. 31 (7), 1074–1087. doi:10.1002/joc.2336
Worden, H. M., Deeter, M. N., Frankenberg, C., George, M., Nichitiu, F., Worden, J., et al. (2013). Decadal record of satellite carbon monoxide observations. Atmos. Chem. Phys. 13 (2), 837–850. doi:10.5194/acp-13-837-2013
World Resources Institute (2014). Fires in Indonesia spike to highest levels since June 2013 haze emergency. Available at: https://www.wri.org/insights/fires-indonesia-spike-highest-levels-june-2013-haze-emergency (Accessed February 16, 2022).
Xie, S.-P., Peng, Q., Kamae, Y., Zheng, X.-T., Tokinaga, H., and Wang, D. (2018). Eastern Pacific ITCZ dipole and ENSO diversity. J. Clim. 31 (11), 4449–4462. doi:10.1175/jcli-d-17-0905.1
Yu, H., and Zhang, M. (2018). Explaining the year-to-year variability of the eastern Pacific intertropical convergence zone in the boreal spring. J. Geophys. Res. Atmos. 123 (8), 3847–3856. doi:10.1002/2017jd028156
Yu, J.-Y., Zou, Y., Kim, S. T., and Lee, T. (2012). The changing impact of El Niño on US winter temperatures. Geophys. Res. Lett. 39 (15). doi:10.1029/2012gl052483
Yu, L., Harris, E., Henne, S., Eggleston, S., Steinbacher, M., Emmenegger, L., et al. (2020). The isotopic composition of atmospheric nitrous oxide observed at the high-altitude research station Jungfraujoch, Switzerland. Atmos. Chem. Phys. 20 (11), 6495–6519. doi:10.5194/acp-20-6495-2020
Zhang, C., Luo, J. J., and Li, S. (2019). Impacts of Tropical Indian and Atlantic Ocean Warming on the Occurrence of the 2017/2018 La Niña. Geophys. Res. Lett. 46 (6), 3435–3445. doi:10.1029/2019gl082280
Zhang, L., Jacob, D. J., Bowman, K. W., Logan, J. A., Turquety, S., Hudman, R. C., et al. (2006). Ozone-CO correlations determined by the TES satellite instrument in continental outflow regions. Geophys. Res. Lett. 33 (18). doi:10.1029/2006gl026399
Zhang, W., Wang, L., Xiang, B., Qi, L., and He, J. (2014). Impacts of two types of La Niña on the NAO during boreal winter. Clim. Dyn. 44 (5-6), 1351–1366. doi:10.1007/s00382-014-2155-z
Zhang, X., Liu, J., Han, H., Zhang, Y., Jiang, Z., Wang, H., et al. (2020). Satellite-observed variations and trends in carbon monoxide over Asia and their sensitivities to biomass burning. Remote Sens. 12 (5), 830. doi:10.3390/rs12050830
Zhang, Y. (2011). Mean global and regional distributions of MOPITT carbon monoxide during 2000-2009 and during ENSO. Atmos. Environ. 45 (6), 1347–1358. doi:10.1016/j.atmosenv.2010.11.044
Keywords: carbon monoxide, North Atlantic European region, biomass burning, transport, ENSO (El nino/southern oscillation)
Citation: Liu Y, Liu J, Xie M, Fang K, Tarasick DW, Wang H, Meng L, Cheng X, Han H and Zhang X (2022) ENSO Teleconnection to Interannual Variability in Carbon Monoxide Over the North Atlantic European Region in Spring. Front. Environ. Sci. 10:894779. doi: 10.3389/fenvs.2022.894779
Received: 12 March 2022; Accepted: 15 June 2022;
Published: 06 July 2022.
Edited by:
Xiaolin Zhang, University of Hamburg, GermanyReviewed by:
Zachary Freitag Johnson, Purdue University, United StatesCopyright © 2022 Liu, Liu, Xie, Fang, Tarasick, Wang, Meng, Cheng, Han and Zhang. This is an open-access article distributed under the terms of the Creative Commons Attribution License (CC BY). The use, distribution or reproduction in other forums is permitted, provided the original author(s) and the copyright owner(s) are credited and that the original publication in this journal is cited, in accordance with accepted academic practice. No use, distribution or reproduction is permitted which does not comply with these terms.
*Correspondence: Jane Liu, amFuZWpqLmxpdUB1dG9yb250by5jYQ==
Disclaimer: All claims expressed in this article are solely those of the authors and do not necessarily represent those of their affiliated organizations, or those of the publisher, the editors and the reviewers. Any product that may be evaluated in this article or claim that may be made by its manufacturer is not guaranteed or endorsed by the publisher.
Research integrity at Frontiers
Learn more about the work of our research integrity team to safeguard the quality of each article we publish.