- 1School of Management, Henan University of Science and Technology, Luoyang, China
- 2Institute of Resources, Environment and Ecology, Tianjin Academy of Social Sciences, Tianjin, China
Population and industry are closely related to CO2 emissions in Cities. However, few studies have explored the joint influence of population size and industrial structure on CO2 emissions. This paper examined the nonlinear influence of population size and industrial structure on CO2 emissions by using a threshold-STIRPAT model with the latest available data in 2001–2017 from 255 cities in China. Results indicated that the promotion effect of urban population size on CO2 emissions increased in the first two stages and then decreased in the third stage when the industrial structure exceeded the threshold value of 1.22. Meanwhile, the industrial structure had a positive impact on CO2 emissions if the urban population was less than 1.38 million. However, the previous promotional effect became an inhibitory effect when the urban population exceeded 1.38 million. According to the above findings, it is necessary to find a reasonable match between urban population size and industrial structure. Specifically, China should formulate differentiated urban population policies in cities with different industrial structures. In addition, for cities with a population size of more than 1.38 million, adjusting the industrial structure to give priority to the tertiary industry will be an effective way to reduce CO2 emissions.
1 Introduction
The rise of greenhouse gases, especially anthropogenic emissions of CO2, is the main cause of global climate change (Wang et al., 2019b; Sun et al., 2022). In 2021, global CO2 emissions from energy consumption and industrial processes rebounded to reach an amount of 36.3 gigatonnes (Gt), the highest ever annual level (IEA, 2022). According to World Economic Forum (WEF), without stronger action, global capacity to mitigate and adapt to climate change will be diminished, eventually leading to a “hot house world scenario” (WEF, 2022).
Cities are responsible for about 75% of the world’s consumption of resources (Pacione, 2009). Cities are the gathering place of population and industries (Wang and Wu, 2012; Mori, 2017) and the centers of CO2 emissions (Grimm et al., 2008; Dhakal, 2009; Glaeser and Kahn, 2010). As the world’s largest CO2 emitter, China’s urban area contributed approximately 75.15 and 85% of the total energy consumption and CO2 emissions (Shan et al., 2018; Tian and Zhou, 2019), both higher than the global average level (Cui et al., 2019). Thus, the urban CO2 emission reduction and the construction of a low-carbon city have become the strategic goals of China. As scheduled, China achieved the phased goal of carbon intensity reduction in 2020 (Cao and Gao, 2021). As a responsible country, China has promised to reach the peak of its carbon emissions by 2030 and achieve carbon neutrality by 2060. According to the previous studies, the CO2 emission reduction targets must be eventually decomposed at the city level (Liu et al., 2012; Reuter and Men, 2014). So, cities are the basic units for implementing CO2 abatement policies in China (Liu et al., 2021).
The rise and fall of cities are always accompanied by the change of population size and the evolution of industrial structure (Yuan et al., 2019). On the one hand, the industrial structure depended on population size and market demand (Abdel-Rahman and Anas, 2004). In other words, population dominates the evolution direction of industrial structure (Ba and Zheng, 2012; He, 2015; Sarkar et al., 2018). On the other hand, the industrial structure gives rise to population size change (Wu, 2012; Han and Li, 2019; Zheng et al., 2021; Guo, 2021). Also, “Regulation population through industrial restructuring” was an important urban population policy in China (Wang and Tong, 2015). The interaction between population size and industrial structure determines the production and consumption patterns (Su and Liao, 2015), which changes the energy efficiency and energy consumption, thus affecting CO2 emissions (Wang and Wu, 2012; Zhang et al., 2021a). Therefore, the interaction between urban population size and industrial structure cannot be ignored in the study of CO2 emissions. From the perspective of interactive influence of urban population size and industrial structure, the impacts of both on CO2 emissions were innovatively explored. The results would help policymakers formulate feasible differentiated policies to achieve CO2 abatement.
2 Literature Review
The influence of population size on CO2 emissions is an important issue (Zhou and Wang, 2018; Cui et al., 2019; Tian and Zhou, 2019). With the rapid expansion of urbanization, increasingly urbanized populations and corresponding consumption changes produced more CO2 emissions (Wiedenhofer et al., 2013; Zhang et al., 2014; Wang et al., 2019b). A great deal of literature confirmed that the population size was an important driving factor of CO2 emissions (Birdsall, 1992; Dietz and Rosa, 1994; Lonngren and Bai, 2008; Jiang and Hardee, 2011; Wang et al., 2017a; Zhou and Wang, 2018; Jung et al., 2021; Namahoro et al., 2021). Anser et al. (2020) found that the urban population size increased residential CO2 emissions in South Asian Association for Regional Cooperation (SAARC) member countries (Anser et al., 2020). Some literature found staged heterogeneity in the relationship between population size and CO2 emissions (Wu, 2012; Chen et al., 2018). According to the size of the urban population, Li et al. (2018) divided 50 cities in China into five kinds and found that the medium-sized cities (population size between 1 million and 2 million) produced the lowest CO2 emissions (Li et al., 2018). Cui et al. (2019) reported that urban population size in eastern China had a significant double-threshold effect on CO2 emissions, and the driving effect first decreased and then increased (Cui et al., 2019). Brought the quadratic term of urban population size in the STIRPAT model, Zhang et al. (2021b) found that the impact of the population size on CO2 emissions per capita presented an inverted U shape (Zhang et al., 2021a). However, only focusing on the impact of urban population size on CO2 emissions with the change of population size, the above studies failed to reveal the relationship between urban population size and CO2 emissions comprehensively.
With the evolution of industrial structure, the proportion of services gradually increased while manufacturing decreased (Kolko, 1999; He and Mao, 2016). But the demands and structures of energy consumption brought by the primary, secondary, and tertiary industries were quite different, leading to different impacts of the three industries on CO2 emissions (Li and Zhou, 2021). Thus, the industrial structure was used as a “monitor” of energy efficiency (Lu et al., 2021). A large body of studies considered that the adjustment in industrial structure played a key role in CO2 emission mitigation (Zhang et al., 2019b; Chuai and Feng, 2019). Some studies concluded that by shrinking the scale of the secondary industry but vigorously developing the tertiary industry, China could maintain economic development and reduce CO2 emissions (Zhixin and Qiao, 2011; Yu et al., 2018; Zhu and Shan, 2020; Lin and Wang, 2021). It is widely acknowledged that the optimal population size has a dependence on industrial structure (Au and Henderson, 2006; Wang et al., 2017b). Based on the STIRPAT model, Wang (2018) analyzed 9 cities in Fujian and found that the relationship between urban population density and per capita CO2 emissions was U-shaped in cities with different industrial structures, but cities with a higher proportion of secondary industry accelerated the arrival of the turning point (Wang, 2018). Owing to the vastness of its territory, China’s regional resource endowments differed greatly, leading to the differences in industrial structure between cities (Cao et al., 2017). However, whether the influence of urban population size on CO2 emissions varied at different stages of industrial structure was not discussed yet. Given this, the nonlinear influence of urban population size on CO2 emissions in cities with different industrial structures was studied in this paper so as to help policymakers formulate differentiated policies in cities at different stages of industrial structure.
Some studies found that the adjustment in industrial structure did not always achieve CO2 abatement. Using the panel data of 281 cities in China, Zhang and song (2020) found that the industrial restructuring to the tertiary industry had no significant effect on CO2 emission mitigation (Zhang and song, 2020). A new puzzle emerged, recklessly rushing to services sometimes failed to achieve CO2 emission mitigation (Zhang and Song, 2020; Zheng et al., 2020). It was found that cities with a population below a specific size could not simultaneously realize the linkage effect of upstream-downstream industries, so the growth of the proportion of services in these cities did not help improve economic efficiency (Ke and Zhao, 2014). Cities in China are diverse, and the industrial restructuring needs to adapt to the characteristics of cities. Significantly, the industrial structure should reasonably match the size of the urban population. It was found that the elasticity of CO2 emission to industrial structure was more than 0.8 in large cities, while it was not significant in small and medium-sized cities (Chen et al., 2018). Then, were there reasonable industrial structures in the cities with different population sizes? So, in the cities with varying population sizes, the nonlinear effects of the industrial structure on CO2 emissions were studied in this paper, which could provide a solution to the question.
In summary, the impacts of urban population size and industrial structure on CO2 emissions were generally uncertain. The nonlinear impacts of urban population size and industrial structure on CO2 emissions were not fully revealed in the existing literature. There was a linear effect in a specific sample or in a specific period, but it was often nonlinear when the sample was large enough and the investigation period was long enough. Considering the great changes of urban population size and industrial structure in the past 20 years in China, it was reasonable to assume that the influences were nonlinear. Moreover, the nonlinear impacts remained to be further tested in this paper. In addition, previous studies ignored the interaction between urban population size and industrial structure, which may also affect the applicability of the conclusions obtained. To investigate the nonlinear impacts of urban population size and industrial structure on CO2 emissions, it was necessary to divide the urban population size and industrial structure stages. The combination of the threshold model and the STIRPAT model, namely the threshold-STIRPAT model, was widely used to estimate thresholds of variables affecting CO2 emissions (Wang, 2016; Wang et al., 2019a; Huo et al., 2021). By dividing variable stages using a strict statistical inference method, the threshold-STIRPAT model avoided the estimation bias brought by the methods such as by adding a quadratic term, an interactive term, and empirically judged in previous studies (Li and Lin, 2015; Cui et al., 2019; Dong et al., 2019).
Thus, based on the available data from 2001 to 2017 in China’s 255 cities, this paper used the threshold-STIRPAT model to investigate the nonlinear influence of the urban population size and industrial structure on CO2 emissions. The contributions of this paper were three folded. First, the staged effects of urban population size on CO2 emissions in different stages of population size were studied. Secondly, the effects of population size in cities at different stages of industrial structure on CO2 emissions were discussed. Thirdly, the phased effects of industrial structure on CO2 emissions in cities with different population sizes were analyzed.
3 Methods and Data
3.1 Threshold-STIRPAT Model
STIRPAT model, one of the most representative methods in environmental impact assessment, is widely used to study CO2 emissions and the influencing factors (Li et al., 2016; Yi et al., 2019; Yue et al., 2022; Ziyuan et al., 2022). STIRPAT model overcomes the limitations of single elasticity in the IPAT model (Dietz and Rosa, 1994; Huo et al., 2021). Compared with the LMDI method, the STIRPAT model allows estimating the impact of each factor as a parameter, which makes up for the deficiency that the LMDI model cannot measure elasticity (Cai et al., 2017; Chen et al., 2018). The STIRPAT model is as follows:
Where
The fixed-effect panel threshold model is proposed by Hansen, (1999), which is used to describe the jumping character or structural break in the relationship between variables (Hansen, 1999; Hansen, 2000). The setting of the single-threshold model is as follows:
Where
In this study, the extended STIRPAT model, including the urban population size, industrial structure, technical level, per capita GDP, and foreign direct investment, are used to investigate their effects on CO2 emissions. By choosing the urban population size and industrial structure as the threshold variables, respectively, the threshold-STIPPAT models are as follows:
Where
3.2 Two-Way Fixed Effects Panel Model
To investigate whether the influence of urban population size on CO2 emissions presented U shape obtained in previous studies, this paper adds the quadratic term of urban population size to construct the following two-way fixed effects panel model:
Where,
3.3 Variable Description and Data Sources
3.3.1 Dependent Variable
CO2 emissions (
3.3.2 Core Explanatory Variables
Urban population size (
Industrial structure (
3.3.3 Control Variables
The control variables were technical level, per capita GDP, and foreign direct investment. The technical factor
3.3.4 Data Sources
CO2 emissions data were collected from CEADs (https://www.ceads.net.cn/). By removing those cities with incomplete statistical data (the Statistical Yearbooks lacked statistics data of certain indicators), 255 prefecture-level cities with different types were selected as research samples (there were 265 prefecture-level cities in China whose administrative divisions was not adjusted over the period 2001–2017), which made the data entire and coherent to ensure the comprehensiveness of the research. The data of other variables were collected from the China City Statistical Yearbooks (2002–2018)and the China National Bureau of Statistics.
3.4 Descriptive Statistics
Figure 1 and Figure 2 show the scatter plots of urban population size and CO2 emissions, industrial structure, and CO2 emissions, respectively.
As shown in Figure 1, CO2 emission increased markedly with the growth of urban population size. As shown in Figure 2, in the process of industrial structure adjustment, the proportion of the secondary industry with high energy consumption decreased, and the proportion of the tertiary industry dominated by services, emerging industry, and low-carbon industry increased, the CO2 emissions gradually decreased.
However, the scatter plot and linear regression were difficult to show the staged heterogeneity of the influence of urban population size and industrial structure on CO2 emissions. Therefore, it was necessary to use the threshold-STIRPAT model to study the phased effects of urban population size and industrial structure on CO2 emissions.
4 Results and Discussion
4.1 Impact of Urban Population Size on CO2 Emissions
For the sake of analyzing the impact of urban population size on CO2 emissions, the xtscc command in the Stata software was used to estimate the two-way fixed effects panel model. The results are listed in Table 1.
As shown in Table 1, Model 1 showed that the impact of urban population size on CO2 emissions was positive. The variance inflation factor (VIF) was 2.26 in Model 2, indicating no severe multicollinearity. In Model 2, the coefficient of the quadratic term of the urban population size was negative, but it was not statistically significant. The coefficient of urban population size was significantly positive, indicating the relationship between urban population size and CO2 emissions was linear.
At the same time, using the urban population size as the threshold variable, Eq. 4 examined whether the impact of urban population size on CO2 emissions differed with the change of urban population size. In the process of threshold estimation, two tests are needed (Huang et al., 2018). The first is whether there is a threshold, and the second is whether the estimated threshold value is equal to the actual threshold value. The existence of the urban population size threshold was tested by using the Bootstrap method. The test’s F statistic and p value are shown in Table 2.
As shown in Table 2, the p value of the single threshold was 0.1110, failing to pass the significant test. Thus, the null hypothesis H0 that there was no single threshold effect was accepted. In other words, urban population size had a significantly positive effect on CO2 emissions, and there was no staged heterogeneity in such effect.
Model 1 indicated that a 1% rise in urban population size could increase the CO2 emissions by 0.1479%. The impact of industrial structure on CO2 emissions was significantly negative. Increasing the proportion of the tertiary industry reduced CO2 emissions significantly, which was consistent with the previous conclusion (Shan et al., 2017). Overall, the high-carbon development mode can be avoided by guiding the adjustment in the industrial structure to the tertiary industry with high added value and low energy consumption.
The influence of technical factors on CO2 emissions was positive, inconsistent with previous literature (Sheng et al., 2019). Compared with the developed countries, technological innovation in most developing countries increased CO2 emissions (Kumar and Managi, 2009). As the world’s largest developing country, China’s research and development spending focused on technology that facilitated production rather than reduced energy consumption and CO2 emissions (Yu and Du, 2019; Lin and Wang, 2021). As such, China’s technical factor tended to increase CO2 emissions, which was confirmed in this study.
A 1% increase in per capita GDP could bring the rise of CO2 emissions by 0.1476%. A 1% increase in foreign direct investment led to a 0.0038% reduction in CO2 emissions. The pollution paradise was not confirmed in this study, which was consistent with the viewpoints of other studies (Yi et al., 2019; Yu and Du, 2019).
4.2 The Relationship Between Urban Population Size and CO2 Emissions at Different Thresholds of Industrial Structure
Using Eq. 5, the phased effects of urban population size on CO2 emissions at different stages of industrial structure were investigated. The results of the industrial structure threshold test are shown in Table 3.
As shown in Table 3, the number of thresholds was determined. From the p value in Table 3, the single threshold test of industrial structure rejected the original hypothesis of linear model (
As shown in Table 4, the industrial structures in different cities were divided into three intervals by two threshold values: interval 1 (lnis ≤ −1.3774), interval 2 (−1.3774 < lnis ≤ 0.1992), and interval 3 (lnis > 0.1992).
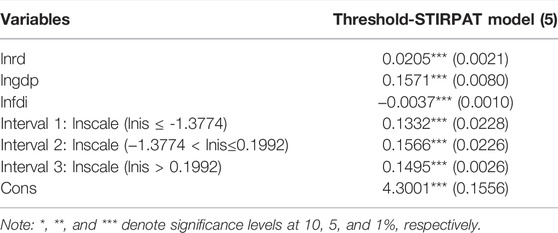
TABLE 4. Influence of urban population size on CO2 emissions at different thresholds of industrial structure.
Table 4 indicated that the impact of urban population size on CO2 emissions had nonlinear characteristics at different stages of industrial structure.
Then, the likelihood ratio (LR) statistic was used to test whether the estimated threshold value was equal to the actual value.
As shown in Figure 3, the LR statistics of the two threshold values were both significantly less than the critical value of 7.35 (marked with red dotted line in the figure). In other words, the threshold estimator for the industrial structure was true and effective.
In the three stages of industrial structure, the driving effect of urban population size on CO2 emissions first increased and then decreased. When the industrial structure was below the threshold of 0.25 (i.e., lnis ≤ −1.3774), the coefficient was 0.1332; When the industrial structure was between 0.25 and 1.22 (i.e., −1.3774 < lnis ≤ 0.1992), the coefficient was 0.1566; When the industrial structure was higher than the threshold value of 1.22 (i.e., lnis > 0.1992), the coefficient was 0.1495. Most of the cities in the sample were in the second stage (i.e., −1.3774 < lnis ≤ 0.1992), where the secondary industry led the economy. And most of the heavy industries in the secondary industry were energy-intensive industries (Hao et al., 2020; Li and Zhou, 2021). The expansion of urban population size in this stage provided human capital for industrial division, which would lead to further increase in energy consumption. In the third stage (i.e., lnis > 0.1992), the tertiary industry with low energy consumption and high added value led the economy. The service industries such as information, tourism, finance and logistics in the tertiary industry consumed less energy (Zhang et al., 2019b; Zheng et al., 2020; Yuan and Zhou, 2021). Taking Taiyuan as an example, its industrial structure exceeded 1.22 since 2012. From 2012 to 2017, the increase of Taiyuan’s population size did not lead to a significant increase in CO2 emissions.
The actual situations in Anyang and Haikou were also consistent with the results. The industrial structure of Anyang was between 0.25 and 1.22, while the industrial structure of Haikou was higher than 1.22 over the period 2001–2017. Moreover, the increment difference of the two cities’ population size was relatively small (an increase of 1.02 million people in Anyang and an increase of 1.11 million people in Haikou). Over the period, Anyang’s CO2 emissions increased by 24.25 million tons, while Haikou’s CO2 emissions increased by just 9.57 million tons. The effects of urban population size change on CO2 emissions were quite different in the two cities. The same increase of urban population size brought higher CO2 emissions when the city’s industrial structure was in the second stage.
4.3 The Relationship Between Industrial Structure and CO2 Emissions at Different Thresholds of Urban Population Size
In Eq. 6, the urban population size was selected as the threshold variable to examine whether the impact of industrial structure on CO2 emissions had a population size threshold effect. Table 5 displayed the results of the threshold effect test.
As shown in Table 5, there was a single threshold effect. The estimated results of Eq. 6 are listed in Table 6.
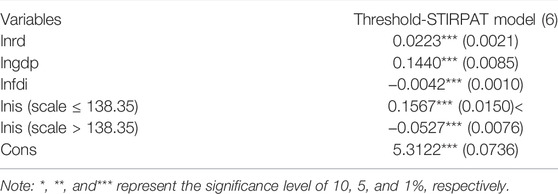
TABLE 6. Influence of industrial structure on CO2 emissions at different thresholds of urban population size.
Table 6 showed that the threshold value was 1.3835 million people. Urban population sizes were divided into two stages and the influence coefficients of industrial structure on CO2 emissions were 0.1567 and −0.0527, respectively.
From Figure 4, it could be seen that the threshold value of urban population size was true and effective.
The influence of industrial structure on CO2 emissions was opposite when it exceeded the threshold, first promoting and then restraining. When the urban population size was below the threshold value of 1.3835 million people, an increase in the ratio of the tertiary industry to the secondary industry failed to achieve CO2 emission reduction. The results also answered why industrial restructuring towards tertiary industry sometimes failed to achieve CO2 emission reduction.
When the urban population size exceeded 1.3835 million people, the influence coefficient of industrial structure on CO2 emissions was significantly negative. Such as Maanshan, a city dominated by secondary industry, exceeded the threshold value of 1.3835 million people in 2011. Moreover, its population size barely changed in 2011–2017. However, the ratio of the tertiary industry to the secondary industry increased rapidly (from 0.38 in 2011 to 0.83 in 2017). We found that its CO2 emissions declined from 15.66 million tons in 2011 to 14.33 million tons in 2017. Similar for Daqing, with a population size more than 1.3835 million people in 2001–2017, its tertiary industry increased significantly from 2014, which was helpful to reduce CO2 emissions. In cities with a population size larger than 1.3835 million people, adjusting the industrial structure to increase the tertiary industry and decrease the secondary industry was helpful to reduce CO2 emissions.
This conclusion was consistent with the viewpoints of the previous studies from the perspectives of CO2 emission intensity and economic performance. It was found that the inhibition effect of industrial structure on CO2 emission intensity was enhanced when the urbanization level was improved (Yu et al., 2022). By introducing the corss term of urban population size and industrial agglomeration, Zhang et al. (2021a) found that the cities with a population more than 1.52 million was conducive to play the CO2 emission reduction effect brought by industrial agglomeration (Zhang et al., 2021a). Cao (2017) deliberated the panel data of 277 cities and concluded that diversification in industry development patterns enhanced economic performance only when the population size exceeded 1.27 million people (Cao, 2017). From the perspective of CO2 emission reduction, this paper concluded that the industrial structure should be adjusted according to the urban population size. The threshold value of 1.3835 million people was almost consistent with the previous literature.
5 Conclusion and Policy Recommendations
In this study, the threshold-STIRPAT method was applied to investigate the nonlinear influence of urban population size and industrial structure on CO2 emissions in China’s 255 cities over the period of 2001–2017. The main findings were as follows: Urban population size positively affected CO2 emissions. Such driving effects did not vary with the change of urban population size but varied across different industrial structure stages. And the degree of the driving effects first increased when industrial structure exceeded the threshold value of 0.25, then decreased when industrial structure exceeded the threshold value of 1.22.
Furthermore, the impact of industrial structure on CO2 emissions was heterogeneous in different stages of urban population size. When the urban population exceeded 1.3835 million people, the industrial adjustment by expanding the tertiary industry and reducing the secondary industry was conducive to reducing CO2 emissions. However, when the urban population has not yet crossed 1.3835 million people, the above industrial restructuring strategies failed to produce lower CO2 emissions.
In the progress toward “carbon peak and carbon neutrality”, China should more scientifically coordinate population development and industrial development. From the perspective of population, a sound green consumption system should be established. By advocating and promoting a low-carbon lifestyle, the promoting effect of the urban population size on CO2 emissions will be gradually reduced. From the perspective of industry, most Chinese cities’ industrial structures are in the second stage, so the government should give priority to the tertiary industry while reducing the industrial CO2 emission intensity.
From the perspective of cities, the industrial structure and the urban population should be reasonably matched. The population development and the adjustment of industrial structure in cities should be differentiated. According to the conclusions obtained in this paper, it is necessary to explore the differential CO2 emission reduction paths in accordance with the size of urban population and heterogeneous industrial structure. For cities with industrial structure lower than the threshold value of 0.25 and higher than the threshold value of 1.22, the local government should appropriately expand urban population size to achieve economies of scale, which will be a helpful way to mitigate CO2 emissions. However, for cities with industrial structure between 0.25 and 1.22, the local government should implement a compact development mode to mitigate CO2 emissions.
In addition, the local government should formulate industrial adjustment policies according to the population size. In cities with a population larger than 1.38 million, adjusting industrial structure and prioritizing tertiary development will effectively reduce CO2 emissions. However, for cities with a population lower than 1.38 million, recklessly running to the tertiary will increase CO2 emissions. For these cities, strengthening the research and development of low-carbon technologies and introducing foreign advanced technologies to improve energy efficiency can be helpful to reduce CO2 emissions.
Data Availability Statement
The raw data supporting the conclusion of this article will be made available by the authors, without undue reservation.
Author Contributions
XZ: Conceptualization, Methodology, Writing-review and editing. YX: Conceptualization, Data curation, and Formal analysis.
Funding
This research was funded by the Project of Humanities and Social Science of Henan Province (No. 2022-ZZJH-037), the Basic Research Project of Philosophy and Social Science in Colleges and Universities of Henan Province (No. 2021JCZD04) and Key Research and Promotion Project of Henan Province (Soft Science Research) (No. 222400410001).
Conflict of Interest
The authors declare that the research was conducted in the absence of any commercial or financial relationships that could be construed as a potential conflict of interest.
Publisher’s Note
All claims expressed in this article are solely those of the authors and do not necessarily represent those of their affiliated organizations, or those of the publisher, the editors and the reviewers. Any product that may be evaluated in this article, or claim that may be made by its manufacturer, is not guaranteed or endorsed by the publisher.
References
Abdel-Rahman, H. M., and Anas, A. (2004). Theories of systems of cities. In Handbook of regional and urban economics. Amsterdam: Elsevier, 4, 2293–2339. doi:10.1016/S1574-0080(04)80009-9
Anser, M. K., Alharthi, M., Aziz, B., and Wasim, S. (2020). Impact of Urbanization, Economic Growth, and Population Size on Residential Carbon Emissions in the SAARC Countries. Clean. Tech. Environ. Pol., 1–14. doi:10.1007/s10098-020-01833-y
Au, C.-C., and Henderson, J. V. (2006). Are Chinese Cities Too Small? Rev. Econ. Stud. 73, 549–576. doi:10.1111/j.1467-937x.2006.00387.x
Ba, S., and Zheng, J. (2012). Motivation and Direction of Chinese Industrial Transformation: Based on a post Structural Economics Perspective. J. Cent. Univ. Finance Econ., 45–52.
Birdsall, N. (1992). Another Look at Population and Global Warming. New York, NY: World Bank Publications.
Cai, B., Wang, J., Yang, S., Mao, X., and Cao, L. (2017). Carbon Dioxide Emissions from Cities in China Based on High Resolution Emission Gridded Data. Chin. J. Popul. Resour. Environ. 15, 58–70. doi:10.1080/10042857.2017.1286143
Cao, C. (2017). Industry Development Patterns, Coordinated Development of City Size and Urban Economic Performance. Soft Sci. 31, 6–10. doi:10.13956/j.ss.1001-8409.2017.05.02
Cao, L., Cai, B., and Wang, J. (2017). Coupling Relationship between Industrial Structure and CO2 Emissions of Cities in China. China Popul. Resour. Environ. 27, 10–14. doi:10.3969/j.issn.1002-2104.2017.02.003
Cao, X., and Gao, Y. (2021). Has the Policy of Low-Carbon City Pilot Led to a green Life? China Popul. Resour. Environ. 31, 93–103. doi:10.12062/cpre.20210620
Chen, J., Gao, M., Cheng, S., Hou, W., Song, M., Liu, X., et al. (2020). County-level CO2 Emissions and Sequestration in China during 1997-2017. Sci. Data 7, 1–12. doi:10.1038/s41597-020-00736-3
Chen, Z., Wu, S., Ma, W., Liu, X., Cai, B., and Liu, J. (2018). Driving Forces of Carbon Dioxide Emission for China's Cities:empirical Analysis Based on Extended STIRPAT Model. China Popul. Resour. Environ. 28, 45–54. doi:10.12062/cpre.20180729
Chuai, X., and Feng, J. (2019). High Resolution Carbon Emissions Simulation and Spatial Heterogeneity Analysis Based on Big Data in Nanjing City, China. Sci. Total Environ. 686, 828–837. doi:10.1016/j.scitotenv.2019.05.138
Cui, P., Xia, S., and Hao, L. (2019). Do different Sizes of Urban Population Matter Differently to CO2 Emission in Different Regions? Evidence from Electricity Consumption Behavior of Urban Residents in China. J. Clean. Prod. 240, 118207. doi:10.1016/j.jclepro.2019.118207
Dhakal, S. (2009). Urban Energy Use and Carbon Emissions from Cities in China and Policy Implications. Energy policy 37, 4208–4219. doi:10.1016/j.enpol.2009.05.020
Dietz, T., and Rosa, E. A. (1994). Rethinking the Environmental Impacts of Population, Affluence and Technology. Hum. Ecol. Rev. 1, 277–300.
Dong, F., Wang, Y., Su, B., Hua, Y., and Zhang, Y. (2019). The Process of Peak CO2 Emissions in Developed Economies: A Perspective of Industrialization and Urbanization. Resour. Conservation Recycling 141, 61–75. doi:10.1016/j.resconrec.2018.10.010
Gan, C., Zheng, R., and Yu, D. (2011). An Empirical Study on the Effects of Industrial Structure on Economic Growth and Fluctuations in China. Econ. Res. J., 4–16.
Geng, Z., Liu, X., and Yang, C. (2012). The Effect of Strategic Orientation and External Knowledge Acquisition on Organizational Creativity. Nankai Business Rev. 15, 15–27.
Glaeser, E. L., and Kahn, M. E. (2010). The Greenness of Cities: Carbon Dioxide Emissions and Urban Development. J. Urban Econ. 67, 404–418. doi:10.1016/j.jue.2009.11.006
Grimm, N. B., Faeth, S. H., Golubiewski, N. E., Redman, C. L., Wu, J., Bai, X., et al. (2008). Global Change and the Ecology of Cities. science 319, 756–760. doi:10.1126/science.1150195
Guo, Y. (2021). Influence of industrial development on population agglomeration in the regional difference, Master's thesis. Jinan: Shandong University.
Han, F., and Li, Y. (2019). Industrial agglomeration, public service supply and urban expansion. Econ. Res. J. 11, 1–16.
Hansen, B. E. (2000). Sample Splitting and Threshold Estimation. Econometrica 68, 575–603. doi:10.1111/1468-0262.00124
Hansen, B. E. (1999). Threshold Effects in Non-dynamic Panels: Estimation, Testing, and Inference. J. Econom. 93, 345–368. doi:10.1016/s0304-4076(99)00025-1
Hao, Y., Zheng, S., Zhao, M., Wu, H., Guo, Y., and Li, Y. (2020). Reexamining the Relationships Among Urbanization, Industrial Structure, and Environmental Pollution in China-New Evidence Using the Dynamic Threshold Panel Model. Energ. Rep. 6, 28–39. doi:10.1016/j.egyr.2019.11.029
He, C., and Mao, X. (2016). Population Dynamics and Regional Development in China. Cambridge J. Regions, Economy Soc. 9, 535–549. doi:10.1093/cjres/rsw020
He, J. (2015). Urban Industrial Composition and Sizes of Cities. Res. Econ. Manag., 85–90. doi:10.13502/j.cnki.issn1000-7636.2015.08.011
Huang, J., Liu, Q., Cai, X., Hao, Y., and Lei, H. (2018). The Effect of Technological Factors on China's Carbon Intensity: New Evidence from a Panel Threshold Model. Energy Policy 115, 32–42. doi:10.1016/j.enpol.2017.12.008
Huo, T., Cao, R., Du, H., Zhang, J., Cai, W., and Liu, B. (2021). Nonlinear Influence of Urbanization on China's Urban Residential Building Carbon Emissions: New Evidence from Panel Threshold Model. Sci. Total Environ. 772, 145058. doi:10.1016/j.scitotenv.2021.145058
Jiang, L., and Hardee, K. (2011). How Do Recent Population Trends Matter to Climate Change? Popul. Res. Pol. Rev 30, 287–312. doi:10.1007/s11113-010-9189-7
Jung, T. Y., Kim, Y.-G., and Moon, J. (2021). The Impact of Demographic Changes on CO2 Emission Profiles: Cases of East Asian Countries. Sustainability 13, 677. doi:10.3390/su13020677
Ke, S., and Zhao, Y. (2014). Industrial Structure, City Size and Urban Productivity in China. Econ. Res. J., 76–88.
Kolko, J. (19991999). Information Technology, Service Industries, and the Future of Cities.Can I Get Some Service Here? Information Technology, Service Industries, and the Future of Cities
Kumar, S., and Managi, S. (2009). Energy price-induced and Exogenous Technological Change: Assessing the Economic and Environmental Outcomes. Resource Energ. Econ. 31, 334–353. doi:10.1016/j.reseneeco.2009.05.001
Li, K., and Lin, B. (2015). Impacts of Urbanization and Industrialization on Energy consumption/CO2 Emissions: Does the Level of Development Matter? Renew. Sust. Energ. Rev. 52, 1107–1122. doi:10.1016/j.rser.2015.07.185
Li, L., Lei, Y., He, C., Wu, S., and Chen, J. (2016). Prediction on the Peak of the CO2 Emissions in China Using the STIRPAT Model. Adv. Meteorol.. doi:10.1155/2016/5213623
Li, L., Lei, Y., Wu, S., He, C., Chen, J., and Yan, D. (2018). Impacts of City Size Change and Industrial Structure Change on CO2 Emissions in Chinese Cities. J. Clean. Prod. 195, 831–838. doi:10.1016/j.jclepro.2018.05.208
Li, Z., and Zhou, Q. (2021). Does the Upgrading of Industrial Structure Help Promote Carbon Emission Reduction: an Empirical Research Based on the Yangtze River Economic belt. Ecol. Economy 37, 34–40.
Lin, B., and Wang, M. (2021). The Role of Socio-Economic Factors in China's CO2 Emissions from Production Activities. Sustainable Prod. Consumption 27, 217–227. doi:10.1016/j.spc.2020.10.029
Liu, Q., Wu, S., Lei, Y., Li, S., and Li, L. (2021). Exploring Spatial Characteristics of City-Level CO2 Emissions in China and Their Influencing Factors from Global and Local Perspectives. Sci. Total Environ. 754, 142206. doi:10.1016/j.scitotenv.2020.142206
Liu, Z., Shi, Y., Yan, J., Ou, X., and Lieu, J. (2012). Research on the Decomposition Model for China's National Renewable Energy Total Target. Energy policy 51, 110–120. doi:10.1016/j.enpol.2012.04.080
Lonngren, K. E., and Bai, E.-W. (2008). On the Global Warming Problem Due to Carbon Dioxide. Energy Policy 36, 1567–1568. doi:10.1016/j.enpol.2007.12.019
Lu, J., Liu, J., and Zhang, X. (2021). Research on the Economic Growth Path of Energy Efficiency and Industrial Structure Upgrading from the Perspective of Population. J. China Univ. Geosciences (Social Sci. Edition ) 21, 82–100. doi:10.16493/j.cnki.42-1627/c.2021.05.008
Ma, X., Li, Q., and Guo, Y. (2013a). The Impact of Population on Carbon Dioxide Emissions in China: an Analysis Based on STIRPAT Model. Popul. Econ., 44–51.
Mori, T. (2017). Evolution of the Size and Industrial Structure of Cities in Japan between 1980 and 2010: Constant Churning and Persistent Regularity. Asian Dev. Rev. 34, 86–113. doi:10.1162/adev_a_00096
Namahoro, J. P., Wu, Q., Xiao, H., and Zhou, N. (2021). The Impact of Renewable Energy, Economic and Population Growth on CO2 Emissions in the East African Region: Evidence from Common Correlated Effect Means Group and Asymmetric Analysis. Energies 14, 312. doi:10.3390/en14020312
Sarkar, S., Phibbs, P., Simpson, R., and Wasnik, S. (2018). The Scaling of Income Distribution in Australia: Possible Relationships between Urban Allometry, City Size, and Economic Inequality. Environ. Plann. B: Urban Analytics City Sci. 45, 603–622. doi:10.1177/0265813516676488
Shan, Y., Guan, D., Hubacek, K., Zheng, B., Davis, S. J., Jia, L., et al. (2018). City-level Climate Change Mitigation in China. Sci. Adv. 4, eaaq0390. doi:10.1126/sciadv.aaq0390
Shan, Y., Guan, D., Liu, J., Mi, Z., Liu, Z., Liu, J., et al. (2017). Methodology and Applications of City Level CO 2 Emission Accounts in China. J. Clean. Prod. 161, 1215–1225. doi:10.1016/j.jclepro.2017.06.075
Sheng, Y., Miao, Y., Song, J., and Shen, H. (2019). The Moderating Effect of Innovation on the Relationship between Urbanization and CO2 Emissions: Evidence from Three Major Urban Agglomerations in China. Sustainability 11, 1633. doi:10.3390/su11061633
Su, S., and Liao, B. (2015). Urban Scale, Urban Structure, Industrial Agglomeration and Urban Energy Consumption. J. Ind. Technol. Econ., 153–160. doi:10.3969/j.issn.1004-910X.2015.04.020
Sun, Y., Zhang, X., Ding, Y., Chen, D., Qin, D., and Zhai, P. (2022). Understanding Human Influence on Climate Change in China. Natl. Sci. Rev. 9, nwab113. doi:10.1093/nsr/nwab113
Sun, Y., and Zhou, M. (2016). Impact of Urbanization and Higher Performance Industrial Structure on CO2 Emissions in China: Independent and Coupling Effects. Resour. Sci. 38, 1846–1860. doi:10.18402/resci.2016.10.03
Tang, J., and Guo, S. (2021). Industrial Agglomeration, Population Size and Environmental Pollution. Stat. Decis., 46–51. doi:10.13546/j.cnki.tjyjc.2021.24.010
Tian, Y., and Zhou, W. (2019). How Do CO2 Emissions and Efficiencies Vary in Chinese Cities? Spatial Variation and Driving Factors in 2007. Sci. Total Environ. 675, 439–452. doi:10.1016/j.scitotenv.2019.04.239
Wang, G., and Wu, J. (2012). Study on Industry Agglomeration, Urban Scale and Carbon Emission. J. Ind. Technol. Econ., 68–80. doi:10.3969/j.issn.1004-910X.2012.06.010
Wang, J. (2018). Impact of Urban Population on Carbon Emissions: Empirical Study Based on DMSP/OLS Night Light Data in Fujian Province, Economic School. Xiamen: Xiamen University.
Wang, Y., Kang, Y., Wang, J., and Xu, L. (2017a). Panel Estimation for the Impacts of Population-Related Factors on CO 2 Emissions: A Regional Analysis in China. Ecol. Indicators 78, 322–330. doi:10.1016/j.ecolind.2017.03.032
Wang, Y., Nian, M., and Wang, C. (2017b). Industrial Structure, Optimal Scale and the Urbanization Path in China. China Econ. Q. 16, 441–462. doi:10.13821/j.cnki.ceq.2017.01.01
Wang, Y. (2016). Study on Approaches to Energy-Carbon Emissions Modeling under the Background of Urbanization and Carbon Reduction targetCollege of New Energy and Environment. Jinlin: Jinlin University.
Wang, Y., and Tong, Y. (2015). The Impact of Industrial Agglomeration and Structural Sophistication on Beijing Population Size: Expansion or Convergence? Popul. J., 5–13. doi:10.16405/j.cnki.1004-129X.2015.06.001
Wang, Y., Xu, Z., and Zhang, Y. (2019a). Influencing Factors and Combined Scenario Prediction of Carbon Emission Peaks in Megacities in China: Based on Threshold-STIRPAT Model. Acta Scientiae Circumstantiae 39, 4284–4292. doi:10.13671/j.hjkxxb.2019.0290
Wang, Z., Cui, C., and Peng, S. (2019b). How Do Urbanization and Consumption Patterns Affect Carbon Emissions in China? A Decomposition Analysis. J. Clean. Prod. 211, 1201–1208. doi:10.1016/j.jclepro.2018.11.272
Wiedenhofer, D., Lenzen, M., and Steinberger, J. K. (2013). Energy Requirements of Consumption: Urban Form, Climatic and Socio-Economic Factors, Rebounds and Their Policy Implications. Energy policy 63, 696–707. doi:10.1016/j.enpol.2013.07.035
Yi, Y., Gao, S., and Guan, W. (2019). Industrial Agglomeration. Urban Population and CO2 missions in Chinese. Prefert. Northwest Popul. 40, 50–60. doi:10.15884/j.cnki.issn.1007-0672.2019.01.006
Yu, S., Zheng, S., Li, X., and Li, L. (2018). China Can Peak its Energy-Related Carbon Emissions before 2025: Evidence from Industry Restructuring. Energ. Econ. 73, 91–107. doi:10.1016/j.eneco.2018.05.012
Yu, X., and Tao, X. (2015). An Inverted U-Shape Relationship between Entrepreneurial Failure Experiences and New Product Development Performance: The Multiple Effects of Entrepreneurial Orientation. J. Manag. Sci. 28, 1–14.
Yu, Y., and Du, Y. (2019). Impact of Technological Innovation on CO2 Emissions and Emissions Trend Prediction on 'New Normal' Economy in China. Atmos. Pollut. Res. 10, 152–161. doi:10.1016/j.apr.2018.07.005
Yu, Z., Fan, Y., and Luo, H. (2022). Research on the Influence of Industrial Structure Upgrading on Carbon Emission Intensity in China. East China Econ. Manag. 36, 78–87. doi:10.19629/j.cnki.34-1014/f.210630001
Yuan, D., Xin, C., and Yuan, D. (2019). Agglomeration Mode and Urban Population Size: Empirical Evidence from 285 Cities at Prefecture-Level and above. Chin. J. Popul. Sci., 47–58.
Yuan, Y., and Zhou, J. (2021). Influence of Multi-Dimensional Characteristics and Evolution of Industrial Structure on Carbon Emissionsat Provincial Scale in China. J. Nat. Resour. 36, 3186–3202. doi:10.31497/zrzyxb.20211213
Yue, J., Zhu, H., and Yao, F. (2022). Does Industrial Transfer Change the Spatial Structure of CO2 Emissions?—Evidence from Beijing-Tianjin-Hebei Region in China. Int. J. Environ. Res. Public Health 19, 322.
Zhang, H., Yuan, P., and Zhu, Z. (2021a). City Population Size, Industrial Agglomeration and CO2 Emission in Chinese Prefectures. China Environ. Sci. 41, 2459–2470.
Zhang, H., Yuan, P., and Zhu, Z. (2021b). City Population Size, Industrial Agglomeration and CO2 Emission in Chinese Prefectures. China Environ. Sci. 41, 2459–2470.
Zhang, J., Lin, Q., Wu, X., and Wang, J. (2019a). Research on the Influence of Sophistication of Industrial Structure on Carbon Productivity: Based on Spatial Durbin Model Journal of South China University of Technology (Social Sciences Edition), 21, 1–13.
Zhang, L., and Song, Y. (2020). Impacts of Beijing-Tianjin-Hebei (JJJ)Industrial Structural Optimization on Carbon Emission Based on Dynamic Panel GMM Model and VAR Model. Resour. Industries 22.
Zhang, S., Li, H., Zhang, Q., Tian, X., and Shi, F. (2019b). Uncovering the Impacts of Industrial Transformation on Low-Carbon Development in the Yangtze River Delta. Resour. Conservation Recycling 150, 104442. doi:10.1016/j.resconrec.2019.104442
Zhang, Y.-J., Liu, Z., Zhang, H., and Tan, T.-D. (2014). The Impact of Economic Growth, Industrial Structure and Urbanization on Carbon Emission Intensity in China. Nat. Hazards 73, 579–595. doi:10.1007/s11069-014-1091-x
Zhang, Y., and Rajagopalan, N. (2010). Once an Outsider, Always an Outsider? CEO Origin, Strategic Change, and Firm Performance. Strat. Mgmt. J. 31, 334–346. doi:10.1002/smj.812
Zheng, X., Wang, R., and Du, Q. (2020). How Does Industrial Restructuring Influence Carbon Emissions: City-Level Evidence from China. J. Environ. Manage. 276, 111093. doi:10.1016/j.jenvman.2020.111093
Zhixin, Z., and Qiao, X. (2011). Low-carbon Economy, Industrial Structure and Changes in China's Development Mode Based on the Data of 1996-2009 in Empirical Analysis. Energ. Proced. 5, 2025–2029. doi:10.1016/j.egypro.2011.03.349
Zhou, C., and Wang, S. (2018). Examining the Determinants and the Spatial Nexus of City-Level CO2 Emissions in China: A Dynamic Spatial Panel Analysis of China's Cities. J. Clean. Prod. 171, 917–926. doi:10.1016/j.jclepro.2017.10.096
Zhu, B., and Shan, H. (2020). Impacts of Industrial Structures Reconstructing on Carbon Emission and Energy Consumption: A Case of Beijing. J. Clean. Prod. 245, 118916. doi:10.1016/j.jclepro.2019.118916
Ziyuan, C., Yibo, Y., Simayi, Z., Shengtian, Y., Abulimiti, M., and Yuqing, W. (2022). Carbon Emissions index Decomposition and Carbon Emissions Prediction in Xinjiang from the Perspective of Population-Related Factors, Based on the Combination of STIRPAT Model and Neural Network. Environ. Sci. Pollut. Res., 1–16. doi:10.1007/s11356-021-17976-4
Keywords: urban population size, industrial structure, CO2 emissions, threshold-STIRPAT model, threshold effects
Citation: Zhao X and Xi Y (2022) Threshold Effects of Urban Population Size and Industrial Structure on CO2 Emissions in China. Front. Environ. Sci. 10:894442. doi: 10.3389/fenvs.2022.894442
Received: 11 March 2022; Accepted: 08 April 2022;
Published: 25 April 2022.
Edited by:
Zhen Wang, Huazhong Agricultural University, ChinaReviewed by:
Sha Peng, Hubei University Of Economics, ChinaChen Zeng, Huazhong Agricultural University, China
Copyright © 2022 Zhao and Xi. This is an open-access article distributed under the terms of the Creative Commons Attribution License (CC BY). The use, distribution or reproduction in other forums is permitted, provided the original author(s) and the copyright owner(s) are credited and that the original publication in this journal is cited, in accordance with accepted academic practice. No use, distribution or reproduction is permitted which does not comply with these terms.
*Correspondence: Yanling Xi, eGl5YW5saW5nMzIzQDE2My5jb20=