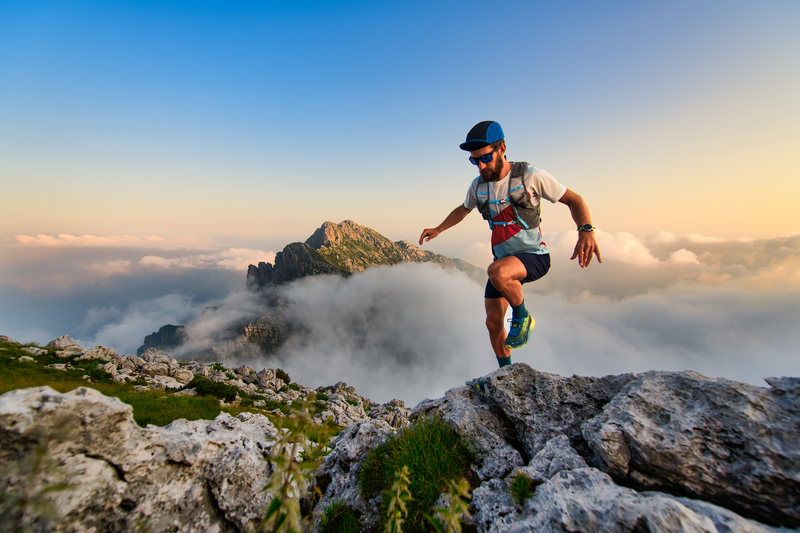
94% of researchers rate our articles as excellent or good
Learn more about the work of our research integrity team to safeguard the quality of each article we publish.
Find out more
ORIGINAL RESEARCH article
Front. Environ. Sci. , 25 May 2022
Sec. Environmental Economics and Management
Volume 10 - 2022 | https://doi.org/10.3389/fenvs.2022.893947
This article is part of the Research Topic Climate Risk, ESG Integration and Economic Growth View all 11 articles
The emissions trading policy is considered a key measure for China to achieve its “double carbon” goal. In this study, two types of Tapio carbon decoupling models are constructed, panel data for 30 provinces and cities in China from 2004 to 2017 are selected, and the difference-in-differences (DID) model is used to evaluate the role of carbon trading policies in carbon decoupling. The study shows that carbon emissions trading policies can significantly promote carbon decoupling in China and that the formulation and implementation of such environmental regulations promote carbon decoupling with the help of two intermediary variables: gray technology innovation and clean technology innovation. Based on the findings, it is concluded that China should actively build a unified national carbon trading market, ensure the synergistic coupling of emission reduction targets and economic growth targets, and effectively play the role of the carbon trading market in inducing and promoting low-carbon technology innovation to help decouple carbon.
Since the 18th National Congress, China has incorporated the construction of ecological civilization into the Five-in-One overall layout, and “Beautiful China” has become the shared vision for all Chinese people. China has committed to reducing CO2 emissions per unit of GDP by 60–65% by 2030, compared to 2005, as part of the Paris Agreement. The establishment of a national carbon emissions trading market is considered one of the most critical steps toward China’s 2030 carbon reduction target. After years of research, the National Development and Reform Commission (NDRC) launched a carbon trading pilot in seven provinces across the country in 2011, followed by the official launch of the national trading market at the end of 2017. In the framework of the Chinese economy’s new normal, China must address the relationship between economic development and carbon emission while enacting a policy of carbon emission reduction. Carbon emission decoupling refers to the relationship between the change in CO2 emissions and economic growth. When economic growth is realized, the negative growth rate of CO2 emissions, or a growth rate less than the economic growth rate, can be regarded as decoupling. Its essence is to measure whether economic growth comes at the cost of resource consumption and environmental damage. The major issues to be explored in the development of the carbon trading market are whether China’s carbon emissions trading scheme (ETS) can enhance carbon emission decoupling and how the contradiction between economic growth and carbon emission reduction can be balanced. The Chinese government has put forward the goal of “double carbon”: at the General Debate of the 75th United Nations General Assembly in September 2020, China proposed for the first time that its carbon dioxide emissions should reach their peak before 2030 and stated that it was striving to achieve the goal of carbon neutrality by 2060. Low-carbon technological innovation is, in fact, seen as a win–win solution both for economic development and emission reduction (Kurtzman, 2015). The NDRC and the National Energy Administration (NEA) jointly issued the Energy Technology Revolution Innovation Action Plan (2016–2030), which identifies 15 key tasks for technological innovation and proposes that, by 2023, energy technology will be close to advanced world standards, with China joining the ranks of powerful countries in terms of energy technology. Most of the current ETS research for low-carbon technological innovation has been concentrated on the EU’s carbon trading market (Rubashkina et al., 2015), with very little research on China. Therefore, the fact that the China’s carbon trading scheme can achieve a win–win situation regarding economic growth and the ETS through an innovation-driven mechanism is crucial to China’s low-carbon transition success. Accordingly, this study uses the quasi-natural experiment with a difference-in-differences (DID) model to evaluate the effect of China’s carbon trading pilot on carbon emission decoupling, subsequently discussing the driving mechanism based on low-carbon technological innovation and the specific path of low-carbon technological innovation to advance carbon decoupling, with the aim of providing insights into the construction and promotion of the national carbon emission trading market.
Carbon emissions trading aims to balance the contradiction between economic development and carbon emissions by using market instruments to reduce the total amount of carbon dioxide emissions (Burnett et al., 2013). Current studies on the effect of carbon trading have mainly focused on the economic and environmental effects of carbon emissions trading at the regional and national levels. In the context of economic effects, Wang et al. (2015) constructed a general equilibrium model with Guangdong province as the study target to assess the economic effects of carbon trading. This study revealed that strict targets for carbon emission reduction led to significant economic losses; however, carbon trading using market instruments can successfully mitigate such losses. Rose et al. (2006) conducted a cost–benefit analysis, concluding that the cost-saving effect of the carbon trading market would increase as the number of carbon trading subjects increases. Some researchers, however, have disagreed, such as Commins et al. (2011), who investigated EU enterprises from 1996 to 2007. Their results indicated that carbon trading drastically reduced the capital return for EU enterprises. In the context of environmental effects, Ren and Fu (2019) investigated the synergistic emission reduction effects of carbon trading policies on regional pollutants in China using panel data for 30 provinces from 2008 to 2015. Li and Zhang (2017) used panel data for 30 Chinese provinces to perform an empirical analysis of the effects of carbon trading on industrial carbon emissions and carbon intensity. Most studies in the literature, however, have focused on the effect of carbon trading from a single perspective instead of the policy’s combined economic and environmental implications. The majority of studies on China’s ETS have used simulation methods; hence, empirical research using econometric methods is lacking. Carbon emission decoupling is considered an idealized process of continuously weakening, or even removing, the relationship between economic growth and greenhouse gas (GHG) emissions. Therefore, it is a significant instrument for measuring the process of low-carbon development as well as a good example of the region’s synergistic control of economic development and carbon emission reduction.
Carbon trading policy is a type of market-incentive environmental regulation that differs significantly from other kinds of environmental regulation. The heterogeneity of environmental regulatory instruments imposes various cost limitations on enterprises, influencing the policy’s “economic effect” and “environmental effect.” As previously stated, carbon decoupling refers to the relationship between the change in CO2 emissions and economic growth, such that when economic growth is realized, the negative growth rate of CO2 emissions, or a growth rate less than the economic growth rate, can be regarded as decoupling. Therefore, it is worthwhile studying the rational portfolio of policy instruments. In view of this, the present research uses a quasi-natural experiment to examine whether carbon trading pilots improve carbon emission decoupling and whether the portfolio of policy instruments has an effect on the decoupling of carbon emissions in the context of the interaction between the ETS and environmental regulations.
One of the important mechanisms for carbon trading is to promote carbon reduction and technological progress. In line with the Porter hypothesis, environmental regulations can trigger innovation and generate net benefits for enterprises instead of increasing the costs, thus improving enterprises’ international competitive advantages. Carbon trading is considered an environmental regulatory instrument in the context of perfect competition. Through carbon quotas and associated costs, carbon trading can increase the price of carbon emission factors. Subsequently, enterprises with carbon emissions are forced to re-examine the costs of paying for a carbon quota and new technology R & D, which in turn induces the progress of low-carbon technological innovation. However, there are disagreements regarding the effect of carbon trading on enterprises’ innovation activities. Cong and Wei (2010) suggested that carbon trading stimulates the development of environmentally friendly technology through carbon pricing by adjusting the relative costs of different power-generation technologies. In contrast, Grubb et al. (2005) argued that restrictions on market emission quotas seriously damage enterprises’ overall innovation activities. Huang et al. (2015), taking the Shenzhen coal power industry as the study subject, found that carbon trading facilitated investment in emission reduction technology in the short term; however, the incentive was not evident in the long term.
Considering the heterogeneity within low-carbon technological innovations, the present study draws on study to classify low-carbon technological innovations into two types from the function perspective: gray and clean. It is generally assumed that gray technological innovation is aimed at improving the utilization efficiency of fossil-fuel energy with potential energy-saving effects. However, gray technological innovation improves energy utilization and the total energy consumption, which might lead to a “rebound effect” (Shao et al., 2013) in CO2 emissions. Jin et al. (2014) explored the role of technological progress on carbon emissions using panel data for 35 industries in China from 1999 to 2011. The results indicated that the gains in emission reduction caused by improved energy efficiency could not offset the CO2 growth resulting from economic growth. Shen et al. (2010) used panel data from 1997 to 2009 to examine the effect of technological progress on CO2 emissions in China as a whole, specifically in the eastern, central, and western regions. The results showed that the direct effect of technological progress was negative, which was not sufficient to offset the indirect positive effect of technological progress on CO2 emissions. On the other hand, clean technology is intended to achieve zero carbon production and consumption, which has a significant inhibitory effect on carbon intensity. Based on the world patent database and panel data for 15 economies from 1996 to 2011, analyzed the effect of different types of technological innovation on carbon intensity, and the findings showed that clean technological innovation has a significant inhibitory effect on carbon intensity and can achieve economic growth without increasing carbon emissions. In light of the aforementioned literature, it is necessary to conduct an empirical analysis of the specific paths of different types of low-carbon technological innovations to reduce carbon intensity and achieve carbon decoupling and subsequently to explore how to promote synergies to achieve a win–win situation for economic development and carbon emission reduction comparing two kinds of low-carbon technological innovations in the context of the ETS.
The purpose of this study was to accurately assess the effect of implementing a carbon emissions trading policy on the decoupling of China’s economic growth from CO2 emissions. Previous studies have usually used the DID approach to evaluate the effects of policy. In the process of empirical analysis, DID takes both ex ante difference and time difference into consideration, with the addition of other control variables affecting the dependent variable, in order to identify treatment effects, reduce errors, and improve the explanatory power of the model. Compared with general regression models, this approach can effectively avoid endogeneity issues and is currently the main method used to assess the utility of public policies.
According to the basic form of the DID model, the regression model is constructed in this study as follows:
where
The Chinese government formally established the carbon emissions trading pilot in 2011, including seven pilot areas (Beijing, Tianjin, Shanghai, Hubei, Guangdong, Shenzhen, and Chongqing); therefore, the present study designates 2011 as the policy implementation year. Since Guangdong province includes the pilot city Shenzhen, this study combines the data from the two regions mentioned previously with a total of six pilot regions as the experimental group and the non-pilot regions as the reference group. The ETS is used to denote the carbon trading policy, where one is implementation and 0 otherwise.
In recent years, the concept of carbon emission decoupling has been used to describe the relationship between economic growth and carbon dioxide emissions. Generally speaking, there is a positive relationship between economic growth and carbon dioxide emissions. However, with the adoption of an appropriate emission reduction policy and the application of low-carbon technological innovation, high-quality economic development can be achieved at a low carbon level, i.e., “carbon decoupling.” Based on the Tapio decoupling model, this study constructs decoupling indicators for China’s economic growth and carbon emissions as follows:
where E is a decoupling indicator of China’s economic growth and carbon emissions,
Tapio (2001) divided the decoupling values into eight categories based on the value size. The smaller the value is, the greater the decoupling degree between two variables. Due to the positive economic growth in China, four categories of carbon emission decoupling were selected in this study: strong decoupling, weak decoupling, expansionary linkage, and expansionary negative decoupling. A decoupling value below 0 denotes strong decoupling, representing positive economic growth and negative carbon emission growth. A decoupling value between 0 and 0.8 represents weak decoupling, showing that the growth rate of carbon emissions is smaller than the economic growth rate. A decoupling value between 0.8 and 1.2 represents expansive linkage, which means that the economic growth rate is similar to the growth rate of carbon emissions. Finally, a decoupling value greater than 1.2 represents expansive negative decoupling, indicating that the growth rate of carbon emissions is faster than the economic growth rate (Figure 1).
To present a more visual image of the distribution of carbon emission decoupling in China, this study uses the indicator of point elastic carbon emission decoupling to make the spatial distribution for types of carbon emission decoupling before (2004–2010) and after (2011–2017) China launched the carbon trading policy.
For carbon emissions (
Here,
Regarding gray technological innovation (gray) and clean technological innovation (clean), referring to the Cooperative Patent Classification (CPC) jointly issued by the US and the UK in 2013, this study uses the number of Chinese patent applications in the Y02 classification to indicate innovation in low-carbon technology. In view of the internal heterogeneity of low-carbon technological innovation, Y02 patents are divided into two sub-categories: gray technology (gray) and clean technology (clean). Gray technology is aimed at improving energy efficiency; thus, it is not completely carbon-free. Clean technology, in contrast, aims to achieve zero-carbon production or consumption.
The industrial structure (STR) is the ratio of the proportion of tertiary industry to the proportion of secondary industry in the region. Advanced industrial structure and its upgrading are helpful to eliminate the crude economic development model that relies on high energy consumption and high pollution, providing impetus for low-carbon technological innovation.
Regarding opening up (FDI), the use of foreign direct investment (FDI) is used to indicate the extent of openness. There exists disagreement on the effect of the extent of openness on the ecological environment of the host country. The “pollution paradise” hypothesis suggests that FDI is accompanied by the transfer of highly polluting and energy-intensive industries, thus aggravating the damage to the ecological environment of the host country. In contrast, the “pollution halo” hypothesis indicates that foreign investment can upgrade the production technology and management level of the host country, thus improving its environmental quality.
The urbanization rate (UR) is the ratio of the regional urban population to the total population. Generally speaking, the rapid growth in population resulting from urbanization will lead to a substantial increase in carbon emissions; thus, the resulting agglomeration effect contributes to carbon decoupling to some extent.
For command-and-control environmental regulation (IER) and voluntary environmental regulation (VER), referring to the calculation method of domestic scholars (Ghisetti et al., 2015), environmental regulation is used to construct an inverse index of comprehensive pollution. VER is measured by the number of environmental petitions in each province. Public environmental awareness induces technological innovation activities by exerting environmental pressure on governments and enterprises.
This study used the data from 30 provinces as the research objects (Tibet, Hong Kong, Macao, and Taiwan data are missing and therefore not included), with 2004–2017 being the research interval. Socioeconomic data were obtained from the China Statistical Yearbook as well as provincial and municipal yearbooks; Y02 patent data were obtained from the incoPat patent database; and energy consumption data were taken from the China Energy Statistical Yearbook. All variables for economic price were treated as constant prices to eliminate the effect of price fluctuations. Descriptive statistics are shown in Table 1.
On the basis of the design of the DID model, the explanatory variables for the reference and experimental groups need to meet the parallel trend test before the implementation of the policy pilot. The parallel trend test plots in Figure 2 show that the data both for the reference and experimental groups have relatively consistent trends in 2010, as well as the previous years, regardless of whether the indicator of point elasticity carbon emission decoupling or arc elasticity carbon emission decoupling is used as the explanatory variable. However, there was a wide divergence when the policy was implemented in 2011. The indicator for carbon emission decoupling in the experimental group continued to decline after 2011, while the indicator for carbon emission decoupling in the reference group still maintained an upward trend in 2011, compared to 2010, indicating that the hypothesis of the parallel trend test was met.
FIGURE 2. Parallel trend test. (A) Point elastic carbon decoupling index. (B) Arc elastic carbon decoupling index.
First, a benchmark regression on the effect of the carbon trading policy on carbon emission decoupling indicators was conducted, in which the explained variables include two types of carbon emission decoupling indicators: point elasticity and arc elasticity. The results are shown in Table 2.
TABLE 2. Regression results for the carbon trading policy on two carbon emission decoupling indicators.
As shown in Table 2, the results of models (1) and (3) indicated that there was a significantly negative correlation between the ETS and the indicator of point elasticity carbon emission decoupling, and between the ETS and the indicator of arc elasticity decoupling, which meant that the pilot of carbon emission rights policy significantly promoted the carbon emission decoupling process in the pilot areas of China. After introducing control variables in models (2) and (4), the China’s urbanization rate has a negative coefficient on both types of carbon emission decoupling indicators, passing the significance test at the 1% level. The results indicate that China’s current urbanization can have a better control over carbon emissions and promote economic development to achieve a benign coordination between the aforementioned two indicators. On the contrary, opening up significantly inhibits China’s carbon emission decoupling and pollutes the environment. The coefficients of the industrial structure on both types of carbon emission decoupling indicators are negative, but the coefficient of the point elasticity indicator only passes the significance test at the 10% level, while the coefficient of the arc elasticity indicator fails the significance test. Thus, the effect of China’s economic structural transformation has not been fully revealed yet, and transformation from an industrial-based economy to a service-based economy is needed.
Most studies have used counterfactual tests for the robustness of the results of DID model (Ye, 2018). The main idea is to change the time of policy implementation through the “counterfactual” tests to generate new policy shock variables for regression analysis and to verify the results by comparison. If the policy shock variable is not significant in the new regression analysis, the original model passes the robustness test. In this study, the year of policy implementation is set to 2008, and the new policy shock variables are then put into the model for regression to judge the robustness of the original regression results.
Table 3 shows that both coefficients of the counterfactual policy shock variables in 2008 and 2009 do not pass the significance test. Thus, the counterfactual policy shock variables do not have an effect on carbon decoupling, indicating that the original policy variables are valid and that the original model passes the robustness test.
As a market-incentive environmental regulation, a carbon trading policy is often implemented simultaneously with other environmental regulations as a policy portfolio; therefore, it is necessary to explore the interaction of heterogeneous environmental regulation on carbon emission decoupling in China. Based on the aforementioned literature review, regressions were conducted on the interaction between carbon trading policy and command-and-control and between carbon trading policy and voluntary environmental regulations, respectively. The results are presented in Tables 4, 5.
TABLE 4. Interaction between carbon trading and command-and-control environmental regulations on carbon decoupling.
TABLE 5. Interaction between carbon trading and voluntary environmental regulation on carbon decoupling.
Before the introduction of the carbon trading policy, the coefficient of the effect of command-and-control environmental regulation (IER) on carbon emission decoupling is positive but not significant, indicating that it does not contribute to carbon decoupling. After introducing the carbon trading policy, the interaction with command-and-control environmental regulation remains insignificant. This suggests that the government often adopts a “one-size-fits-all” coercive approach to administrative regulation, and enterprises are forced to reduce production and emissions in the face of transition cost constraints, resulting in the loss of economic growth. Furthermore, the interaction with command-and-control environmental regulation is significantly negative, effectively promoting carbon decoupling. The carbon trading policy internalizes the cost of carbon emissions into the production cost of enterprises through market incentives. With the dual constraints of pollution control from mandatory environmental regulations and energy consumption from market-based environmental regulations, enterprises tend to invest in low-carbon technology R & D and upgrade management skills to achieve both economic benefits and emission reduction.
Before introducing carbon trading, the coefficients of the effects of voluntary environmental regulation on both kinds of carbon emission decoupling indicators pass the significance test at the 5% level. The results show the weak public awareness of environmental protection in most regions and insufficient pressure on enterprises for environmental protection. After the introduction of carbon trading, the interaction with voluntary environmental regulation has a significant negative effect on the carbon emission decoupling index. This indicates that the ETS, as a market-based incentive system, can guide the green transformation of enterprises and help regions in carbon decoupling through the dual paths of cost constraint of carbon excess fines and the incentive of carbon sale proceeds.
Referring to Fang et al. (2014), this study adopted a mediating effect regression model based on the DID model and the Sobel test to explore the driving mechanism of low-carbon technological innovation in the process of a carbon trading policy promoting carbon decoupling. The three-step regression model is set up as follows:
The first step is to test path a: whether the coefficient α1, the effect of the carbon trading policy on two indicators of carbon emission decoupling, is significant. The second step is to test path b: whether the coefficient γ1, the effect of the carbon trading policy on two types of low-carbon technological innovation, is significant. The final step is to test c: whether the coefficients μ1 and μ2, the interaction between carbon trading policy and low-carbon technological innovation on carbon decoupling indicators, are significant.
Based on the explanatory law of the mediating effect model, the regression coefficient α1 in path a, γ1 in path b, and μ2 in path c are all significant, which meets the prerequisite of the mediating effect. When the regression coefficient μ1 in path c is not significant and the Z-value of the Sobel test is significant, the model is fully mediated. When the regression coefficient μ1 in path a is significant but lower than the corresponding coefficient α1, with a significant Z-value for the Sobel test, the model presents a partial mediation effect.
According to the regression results shown in Table 6, the coefficients α1 for path a and γ1 for path b are both significant when the mediating variable is gray technological innovation, and the coefficients in path c and the Z-values of the Sobel test are consistent with a full mediation effect when the explanatory variable is the indicator of point elasticity decoupling. When the explanatory variable is the indicator of arc elastic decoupling, the coefficient in path c and the Z-value of the Sobel test show partial mediation effects. Both coefficients, α1 in path a and γ1 in path b , are significant when the mediating variable is clean technological innovation. The coefficients in path c and the Z-values of the Sobel test show partial mediation when the explanatory variables are indicators of point elasticity decoupling and arc elasticity decoupling.
In summary, both gray and clean technological innovation can be considered critical mediating variables in the process of carbon emission decoupling boosted by the carbon trading policy. Based on the driving mechanism of low-carbon technological innovation, a carbon trading policy can encourage enterprises to increase their innovation efforts and then gradually achieve low carbon and clean production to reduce CO2 emissions while ensuring economic development at the same time.
To further explore the intrinsic paths of low-carbon technological innovations to promote carbon emission decoupling in China and different mechanisms of heterogeneous technological innovations, this study examines the emission reduction effects and economic effects of two kinds of low-carbon technological innovations on the basis of the nature of carbon decoupling, in which the explanatory variables are carbon emissions (carbon) and the level of economic development (lnGDP).
The results of the regression model (1) in Table 7 show that the coefficients of correlation between gray technological innovation and carbon emissions are negative. The results of the regression model (2) in Table 7 show that the coefficients of correlation between clean technological innovation and carbon emissions are also negative. The results of regression model (3) indicate that the coefficient of the effect of gray technological innovation remains negative and the coefficient of clean technological innovation is positive when both gray and clean technological innovations are put in the regression equation. Therefore, gray technological innovation improves energy efficiency and promotes more energy consumption, leading to an increase in CO2 emissions instead of a decrease, i.e., the “rebound effect.” In contrast, clean technological innovation is committed to zero-carbon production and consumption, which promotes an overall reduction in CO2.
Model (4) introduces the interaction effect of the carbon trading policy and discovers that the interaction with gray technological innovation remains positive but not significant, whereas the interaction with clean technological innovation is negative and larger than the corresponding coefficient of model (3). The findings show that the “carbon-increasing” effect of gray technological innovation is effectively suppressed in the context of the carbon trading policy, and the “carbon-reducing” effect of clean technological innovation is further enhanced.
Table 8 illustrates the economic effects of the two kinds of low-carbon technological innovations. The results of models (1) and (2) suggest that the coefficients both of gray and clean technological innovation on economic development are positive when the two kinds of low-carbon technological innovation are examined separately. The results of model (3) imply that the coefficient of gray technological innovation on economic development is significantly positive and the coefficient of clean technological innovation on economic development is significantly negative. Therefore, under the premise that both kinds of technologies are applied simultaneously with production activities in real situations, gray technological innovation can significantly contribute to economic growth. In contrast, clean technological innovation cannot boost economic development in a short period of time, and it might inhibit the economic development due to high R & D investment and slow implementation.
After the introduction of the carbon trading policy, the interaction coefficient with both types of technological innovation is not significant, indicating that the carbon trading policy has no significant effect on the economic effect of both kinds of low-carbon technological innovation.
To sum up, the elements of the heterogeneous environmental regulatory portfolio can interact with each other. In addition, it is worth noting that innovation is an important mechanism for the carbon trading policy to boost carbon decoupling. The results of the mediating effect and the Sobel test reveal that the carbon trading policy significantly increases two types of low-carbon technological innovations; in turn, low-carbon technological innovation is a significant driver for the carbon trading policy. After decomposing the paths of the two kinds of technological innovation, gray technological innovation reveals an “economic effect” with the increase of CO2 emissions, while clean technological innovation shows an “emission reduction effect” but does not have advantages in terms of economic cost-effectiveness. The mechanism of gray technological innovation promoting carbon emission decoupling increases economic growth, but it triggers a “rebound effect” for CO2 emissions. Clean technological innovation has great potential for reducing carbon emissions, although it has not promoted GDP growth. Since the introduction of the carbon trading policy, the interaction with the two types of low-carbon technological innovations has significantly facilitated the carbon emission reduction effect but not the economic effect.
The critical criteria for checking the effectiveness of implementing a carbon trading policy lie in whether the China’s carbon emission trading market can help decouple carbon and achieve a win–win situation between economic development and carbon emission reduction. Based on the panel data for 30 provinces across China from 2004 to 2017, this study has analyzed the underlying mechanisms through which the China’s carbon trading pilot policy facilitates carbon emission decoupling with the methods of a DID quasi-natural experiment, a mediating effect model, and the Sobel test. The main conclusions are presented as follows:
First, the carbon trading policy pilots can boost carbon emission decoupling in China. Regardless of whether using point or arc elasticity calculations, carbon trading policies have effectively promoted carbon emission decoupling in the pilot regions, with a significantly negative indicator for carbon emission decoupling.
Second, the elements of a heterogeneous environmental regulatory portfolio can interact with each other. Considering that the carbon trading policy has entailed for enterprises, the dual constraints of carbon costs and market incentives, both the underperforming command-and-control environmental regulation and voluntary environmental regulation, facilitate the decoupling of carbon emission.
Third, innovation is an important mechanism for the carbon trading policy to boost carbon decoupling. The results of the mediating effect and the Sobel test reveal that the carbon trading policy significantly increases two types of low-carbon technological innovations; in turn, low-carbon technological innovation is a significant driver for the carbon trading policy.
Fourth, after decomposing the paths for the two kinds of technological innovation, gray technological innovation revealed an “economic effect” with the increase of CO2 emissions, while clean technological innovation showed an “emission reduction effect” but did not have advantages in terms of economic cost-effectiveness.
Based on the aforementioned findings, the following policy recommendations are suggested:
First, the construction of the China’s carbon trading market is conducive to the win–win goal of carbon emission reduction and economic development. China should actively build a national unified carbon trading market and a scientific market trading system. Moreover, the focus should be on the synergistic coupling of emission reduction and economic growth and on the dynamic adjustment of the carbon market.
Second, the government needs to effectively manage different types of environmental regulations in a synergistic manner and actively guide both market-incentive environmental regulations and voluntary environmental regulations. Command-and-control environmental regulations should be prudently implemented. The conflicts among different environmental regulations need to be balanced in order to bring into play the synergy of the heterogeneous environmental regulatory portfolio.
Third, the carbon market should play an active role in promoting the progress of low-carbon technology and ensure a scientific carbon quota. China should speed up the construction of the national carbon emission trading market and guide enterprises in carrying out low-carbon innovation through reasonable carbon element costs and carbon trading income.
Finally, in developing countries such as China, it is necessary to encourage R & D in clean and low-carbon technology through various policy tools, such as tax, green finance, and financial subsidies. Moreover, it is also important to strive to reduce the R & D costs of, and barriers to, applying clean technological innovation in order to popularize the application of clean technology and achieve a boost to economic growth and the reduction of carbon emissions at an early date. For gray technological innovation, the government should gradually weaken the support to accelerate its transition to clean technology.
In conclusion, it must be noted that this study has some limitations, which suggest avenues for further research. It is reasonable to use patents as an index for innovation, which can bring convenience to research and reveal certain issues, but it is easy to ignore the complex relationship between patents and innovation, thus resulting in errors. Further research can broaden the meaning of innovation, especially green innovation, and explore the deeper impact of environmental policies such as carbon trading on one of the complex behaviors of innovation.
The raw data supporting the conclusion of this article will be made available by the authors, without undue reservation.
All authors listed have made a substantial, direct, and intellectual contribution to the work and approved it for publication.
This work was supported by the National Natural Science Foundation of China (72104091), the Humanities and Social Science Research Youth Foundation of Ministry of Education in China (21YJCZH097), and the Jiangsu Funds for Social Science (17GLB020).
The authors declare that the research was conducted in the absence of any commercial or financial relationships that could be construed as a potential conflict of interest.
All claims expressed in this article are solely those of the authors and do not necessarily represent those of their affiliated organizations, or those of the publisher, the editors, and the reviewers. Any product that may be evaluated in this article, or claim that may be made by its manufacturer, is not guaranteed or endorsed by the publisher.
Burnett, J. W., Bergstrom, J. C., and Wetzstein, M. E. (2013). Carbon Dioxide Emissions and Economic Growth in the U.S. J. Policy Model. 35 (6), 1014–1028. doi:10.1016/j.jpolmod.2013.08.001
Commins, N., Lyons, S., Schiffbauer, M., and Tol, R. (2011). Climate Policy & Corporate Behavior. Energy J. 32 (4), 51–68. doi:10.5547/issn0195-6574-ej-vol32-no4-4
Cong, R.-G., and Wei, Y.-M. (2010). Potential Impact of (CET) Carbon Emissions Trading on China's Power Sector: A Perspective from Different Allowance Allocation Options. Energy 35 (9), 3921–3931. doi:10.1016/j.energy.2010.06.013
Fang, J., Wen, Z., Zhang, M., and Sun, P. (2014). The Analyses of Multiple Mediation Effects Based on Structural Equation Modeling. J. Psychol. Sci. 37 (3), 735–741.
Ghisetti, C., Marzucchi, A., and Montresor, S. (2015). The Open Eco-Innovation Mode. An Empirical Investigation of Eleven European Countries. Res. Policy 44 (5), 1080–1093. doi:10.1016/j.respol.2014.12.001
Grubb, M., Azar, C., and Persson, U. M. (2005). Allowance Allocation in the European Emissions Trading System: A Commentary. Clim. Policy 5 (1), 127–136. doi:10.1080/14693062.2005.9685545
Huang, Y., Liu, L., Ma, X., and Pan, X. (2015). Abatement Technology Investment and Emissions Trading System: A Case of Coal-Fired Power Industry of Shenzhen, China. Clean. Techn Environ. Policy 17 (3), 811–817. doi:10.1007/s10098-014-0854-0
Jin, P.-Z., Zhang, Y.-B., and Peng, X. (2014). The Double-Edged Effect of Technological Progress in Carbon Dioxide Emissions Reduction: Empirical Evidence from 35 Sub-industrial Sectors in China. Stud. Sci. Sci. 32 (5), 706–716.
Kurtzman, J. (2015). Unleashing the Second American Century: Four Forces for Economic Dominance. Dalian: Dongbei University of Finance & Economics Press. Translated by Zhou, Y.
Li, G.-M., and Zhang, W.-J. (2017). Research on Industrial Carbon Emissions and Emissions Reduction Mechanism in China’s ETS. China Popul. Resour. Environ. 27 (10), 141–148.
Ren, Y.-Y., and Fu, J.-Y. (2019). Research on the Effect of Carbon Emissions Trading on Emission Reduction and Green Development. China Popul. Resour. Environ. 29 (5), 11–20.
Rose, A., Peterson, T. D., and Zhang, Z.-X. J. P. S. E. L. R. (2005). Regional carbon dioxide permit trading in the United States: coalition choices for Pennsylvania. 14, 203.
Rubashkina, Y., Galeotti, M., and Verdolini, E. (2015). Environmental Regulation and Competitiveness: Empirical Evidence on the Porter Hypothesis from European Manufacturing Sectors. Energy Policy 83, 288–300. doi:10.1016/j.enpol.2015.02.014
Shao, S., Yang, L., and Huang, T. (2013). Theoretical Model and Experience from China of Energy Rebound Effect. Econ. Res. J. 48 (2), 96–109.
Shen, M., Li, K.-J., and Qu, R.-X. (2010). Technological Progress, Economic Growth and CO2 Emissions: A Theoretical and Empirical Study. J. World Econ. 7, 85–102.
Tapio, P. (2001). Towards a Theory of Decoupling: Degrees of Decoupling in the EU and the Case of Road Traffic in Finland between 1970 and 2001. Transp. Policy 12 (2), 137–151. doi:10.1016/j.tranpol.2005.01.001
Wang, P., Dai, H.-c., Ren, S.-y., Zhao, D.-q., and Masui, T. (2015). Achieving Copenhagen Target through Carbon Emission Trading: Economic Impacts Assessment in Guangdong Province of China. Energy 79 (1), 212–227. doi:10.1016/j.energy.2014.11.009
Keywords: carbon emission decoupling, differences-in-difference, carbon trading, technology innovation, carbon emission
Citation: Lyu B, Ni W, Ostic D, Yu H and Qureshi NA (2022) Can Carbon Trading Policy Promote China’s Decoupling of Carbon Emission From the Perspective of Technology-Driven Innovation?. Front. Environ. Sci. 10:893947. doi: 10.3389/fenvs.2022.893947
Received: 11 March 2022; Accepted: 25 April 2022;
Published: 25 May 2022.
Edited by:
Mihaela Onofrei, Alexandru Ioan Cuza University, RomaniaCopyright © 2022 Lyu, Ni, Ostic, Yu and Qureshi. This is an open-access article distributed under the terms of the Creative Commons Attribution License (CC BY). The use, distribution or reproduction in other forums is permitted, provided the original author(s) and the copyright owner(s) are credited and that the original publication in this journal is cited, in accordance with accepted academic practice. No use, distribution or reproduction is permitted which does not comply with these terms.
*Correspondence: Dragana Ostic, ZG9zdGljQHVqcy5lZHUuY24=
Disclaimer: All claims expressed in this article are solely those of the authors and do not necessarily represent those of their affiliated organizations, or those of the publisher, the editors and the reviewers. Any product that may be evaluated in this article or claim that may be made by its manufacturer is not guaranteed or endorsed by the publisher.
Research integrity at Frontiers
Learn more about the work of our research integrity team to safeguard the quality of each article we publish.