- 1Shandong Provincial No.4 Institute of Geological and Mineral Survey, Weifang, China
- 2Key Laboratory of Coastal Geological Environment Protection of Shandong Bureau of Geology and Mineral Resources, Weifang, China
- 3School of Earth Sciences and Engineering, Nanjing University, Nanjing, China
The distribution of heavy metals in topsoil can have a significant impact on human health. A total of 1, 556 samples were collected from the topsoil of Weifang, China. Various indices, including the pollution index (PI), the Nemerow integrated pollution index (PN), and the potential ecological risk index (RI), were used to analyze the heavy metal pollution levels. The sources of heavy metals were analyzed using the positive matrix factorization (PMF) model. The results are as follows: (1) the ecological risk level of Cu, Pb, Zn, Ni, Cr, and As in the study area is relatively safe, but the ecological risk level of Cd and Hg is relatively high, leading to an increase in the ecological risk level of heavy metals in the study area and (2) the PMF results show that there are six main sources of the eight heavy metals. Cr and Ni come from soil parent material and nonferrous metal industrial activities; As is closely related to fossil fuel (coal)-related industrial activities; Cu originated from diesel fuel-related transportation and agricultural machinery activities; Pb is derived from gasoline transportation activities; Hg is closely related to the application of pesticides in agricultural production; Cd and Zn originated from organic fertilizers used in agricultural activities.
Highlights
• Environmental risk assessment of heavy metals in topsoil was performed.
• Spatial distributions of heavy metals in topsoil were characterized.
• Six main sources of eight heavy metals were determined by the PMF method.
Introduction
Environmental issues are getting more and more attention with population growth and urban development (Ambade et al., 2021a,b, c, 2022; Kurwadkar et al., 2022; Maharjan et al., 2021). In particular, heavy metal pollution in soil has attracted extensive attention all over the world (Lokeshwari and Chandrappa, 2006; Cao et al., 2010; Akbarpour et al., 2021). Heavy metal pollution in soil has the characteristics of a wide range and long duration (Hu et al., 2018; Chen et al., 2021). These pollutants can become enriched in soil and migrate to the human body through the food chain, posing a potential threat to the natural ecosystem and public health (Burges et al., 2015; Zhang et al., 2017; Li et al., 2019). Therefore, considering the hazards of heavy metals, the United States Environmental Protection Agency (USEPA) lists Cu, Pb, Zn, Ni, Cr, As, Cd, and Hg as priority pollutants (USEPA, 1986). The means to effectively evaluate the harm of heavy metals in soil has always been a research hotspot (Burges et al., 2015; Zhang et al., 2017; Li et al., 2019).
Determining the distribution pattern and pollution level of heavy metals in soil is the key to evaluating the potential risk of these metals (Lin al., 2010; Li et al., 2020). It is important to provide information about various heavy metal pollution levels in the soil to guide the formulation of relevant pollution control policies (Solomon et al., 2016). To assess the risk of heavy metals in soil, soil pollution sources have attracted the attention of many researchers (Chen et al., 2021; Yao et al., 2021). There are many indicators of heavy metal pollution in soil, including the enrichment coefficient (Buat-Menard and Chesselet, 1979), the pollution index (Wei and Yang, 2010), the Nemerow comprehensive pollution index (Cheng et al., 2007), the geological accumulation index (Hasan et al., 2013), and the potential ecological risk index (Sun et al., 2010).
The determination of heavy metal soil pollution sources is one of the important components of the comprehensive and effective evaluation of heavy metal soil pollution (Wu et al., 2018; Heidari et al., 2021). Generally, sources of heavy metals can be divided as coming from natural sources or human activities (Jiang et al., 2017; Wang et al., 2019; Cai et al., 2021a). Many scholars have adopted a variety of methods to determine heavy metal pollution sources in soil, such as geographic information systems, multivariate statistical analysis, positive matrix factorization (PMF), and chemical mass balance law (Facchinelli et al., 2001; Pekey et al., 2004; Cao et al., 2012). Among them, the PMF model reduces the dimension of multidimensional variables through correlation matrix and covariance matrix, which is a very effective method for source analysis of heavy metals (Fang et al., 2021), and, therefore, the PMF model has been widely used. In recent years, PMF models have been widely applied to atmospheric particles, water, soil, and sediments (Rodenburg et al., 2011; Tan et al., 2016).
Weifang, a regional center city in Shandong Province, is also an important vegetable growing area in China. Its soil environmental problems are closely related to urban development and people’s lives and health. However, there has been a lack of research on the distribution of heavy metals in the soil in this city. This study mainly carried out environmental risk assessment and determination of pollution sources in the soil of Weifang. The results of this study are expected to provide a useful reference for the control and management of heavy metal pollution in the soil of other cities of the world.
Materials and Methods
Regional Setting
The study area covers the whole of Weifang (Figure 1), with the geographic coordinates of 118°10′ E -120°01′E and 35°32′ N -37°26′ N. The total area is 15,859 km2 in August 2020. Weifang is located in the middle of the Shandong Peninsula and is adjacent to the Taiyi Mountains in the south, the Linyi and Rizhao cities in the south, Laizhou Bay in the Bohai Sea in the north, Qingdao and Yantai in the east, and Dongying and Zibo in the west. The land chokes the throat of the road from the hinterland of Shandong to the peninsula, and the Jiaoji Railway runs from the east to the west of the city. According to the census of 2020, the total population of Weifang is around 9.394 million. The urbanization of Weifang has also created numerous environmental problems, including ecosystem pollution (Hu et al., 2021), groundwater pollution (Gao et al., 2020), and air pollution (Li et al., 2020).
Analytical Methods
Based on the actual soil distribution in Weifang, a total of 1,556 surface samples were collected with a uniform distribution of 1 sample/10 km2. The sampling points were located by GPS (longitude and latitude), the surface sample collection depth was 0–20 cm, and the specific sampling distribution is shown in Figure 1. The data of all the sampling points were also recorded; the quantity of the original samples was more than 500 g each, and the weight of the processed samples was more than 250 g each. In the laboratory of the Fourth Geological and Mineral Exploration Institute of Shandong Province, inductively coupled plasma mass spectrometry (ICP-MS) was used to determine the content of heavy metal elements (Cu, Pb, Zn, Ni, Cr, As, Cd, and Hg) in the soil samples. During the test, GSS-4 and GSS-6 national standard soil samples were used for quality control throughout the entire process. A repeat sample is a measurement randomly selected from every five samples, and the error value of the analysis results was within ±10%. The pH value of the samples was measured using the potentiometric method.
Heavy Metal Evaluation Method
Pollution Evaluation Method
In this study, the pollution index (Pi), the Nemerow comprehensive pollution index (PN), and the potential ecological risk index (
PMF Analysis
PMF is a multivariate factor analysis tool, which is used to analyze different types of environmental pollution sources (Rodenburg et al., 2011; Tan et al., 2016; Guan et al., 2018). The PMF model decomposes the original matrix
The i, j, and k represent the i-th sample, j-th element, and k represents the k-th source, respectively. Minimizing the objective function Q can be calculated using Formula 2.
The PMF can weight each data point and provide an appropriate uncertainty for each data point. When the consent of the element is lower than or equal to the detection limit of the corresponding method (MDL), the uncertainty can be calculated using formula 3:
Otherwise, the uncertainty is calculated using formula 4:
The σ is the relative standard deviation, cij is the consent of elements.
Data Processing and Methods
Microsoft Excel 2016 and SPSS 22.0 were used for the statistical compilation and analysis of the pollution index, the ecological risk index, and the correlation and principal component of the heavy metals in the soil; ArcGIS 10.2 was used to analyze and map the distribution of soil sampling points and the spatial distribution of heavy metals. The EPA PMF5.0 software was used to quantitatively identify the source of metals in this study.
Results and Discussion
Descriptive Statistics of the Concentrations of Major Elements and REEs
The descriptive statistical results of eight heavy metals in the surface soil of Weifang are shown in Table 2. The average pH value is 7.85, indicating that the soil is alkaline. The mean content (ng/g) of Cu, Pb, Zn, Ni, Cr, As, Cd, and Hg are 22.71, 26.39, 76.29, 28.54, 82.37, 9.81, 0.09, and 0.03, respectively. The average values of the eight heavy metal elements are higher than their background values in the soil in Shandong Province, and there is a certain degree of heavy metal accumulation, but none exceeds the sorting and screening value of GB15618-2018. The coefficient of variation is the standardization of the dispersion of the probability distribution. The coefficient of variation can be divided into three levels: low (CV < 0.16), medium (0.16 < CV < 0.36), and high (CV > 0.36) (Wilding, 1985). The coefficient of variation of As is 0.261, and the degree of variation is medium; the coefficients of variation of Cu, Pb, Zn, Ni, Cr, and Cd were 0.542, 0.461, 1.495, 0.527, 0.369, 1.107, and 1.678, respectively. Overall, the heavy metals in the soil of the study area showed greater variability and greater spatial dispersion, and the concentration of heavy metals in the soil in the study area is likely to be affected by human activities.
Spatial Distribution of Heavy Metals
The results of the spatial interpolation of heavy metals using the ordinary Kriging method are shown in Figure 2. The pH value in the north of Weifang (Qingzhou, Shouguang, Hanting, Kuiwen, Fangzi District, and Weicheng District) and some areas of Changle County, Changyi City, Gaomi City, and Linqu County is higher than 8.2. The high-value Cu areas are mainly distributed in the western counties and in Weifang, Weicheng District, and the Gaomi City Center. The high-value Pb areas are mainly distributed in Weicheng District, Anqiu City, Zhucheng City, and Linqu County. The high-value Zn and Cd areas are mainly concentrated in the center of Zhucheng City. The high-value Ni areas are mainly in the western counties and Weifang. The high-value Cr areas are also mainly in the western counties and Weifang and the southeast of Gaomi. As and Hg are evenly distributed in Weifang.
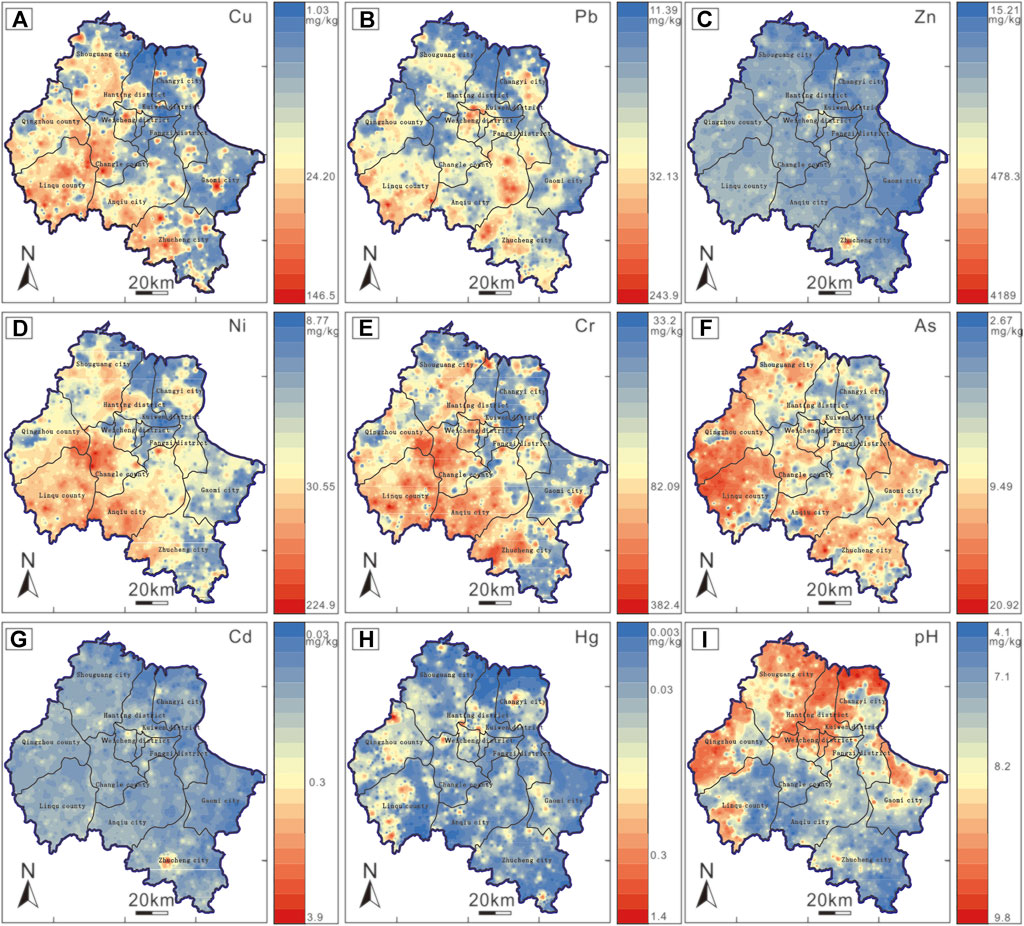
FIGURE 2. Spatial distribution of Cu (A), Pb (B), Zn (C), Ni (D), Cr (E), As (F), Cd (G), Hg (H), and pH (I) of surface soil in Weifang.
Environmental Risk Assessment of Heavy Metals
The proportion of heavy metal pollution levels was determined based on the Pi and PN evaluation standards of the 8 heavy metals (Table 3). The Pi results show that the pollution level at most of the sampling points is safe. The alert samples of Pb and As accounted for 0.13%, and the rest were safe. For Cu, the samples in the warning threshold, slight pollution, and safe levels accounted for 0.44, 0.58, and 98.97%, respectively. For Cr, the samples in the warning threshold, slight pollution, moderate pollution, and safe levels accounted for 3.66, 1.15, 0.06, and 95.13%, respectively. For Zn, the samples in the warning threshold, slight pollution, moderate pollution, severe pollution, and safe levels accounted for 1.15, 0.70, 0.19, 0.06, and 98.53%, respectively. For Ni, the samples in the warning threshold, slight pollution, moderate pollution, severe pollution, and safe levels accounted for 8.41, 4.63, 0.45, 0.19, and 86.36%, respectively. For Cd, the samples in the warning threshold, slight pollution, moderate pollution, severe pollution, and safe levels accounted for 0.84, 0.45, 0.06, 0.06, and 98.59% respectively. For Hg, the samples in the warning threshold, slight pollution, severe pollution, and safe levels accounted for 0.38, 0.13, 0.06, and 99.46%, respectively. The results of the PN value show that the samples in the safe level account for 92.67%, those in the warning threshold level account for 4.24%, those in the slight pollution level account for 2.70%, those in the moderate pollution level account for 0.26%, and those in the severe pollution level account for 0.13%, indicating that most of the soil in the entire study area is still relatively safe.
To further evaluate the ecological risk of heavy metals in the study area,
Source of Heavy Metals
Correlation Analysis
The results of the correlation analysis between the eight heavy metal elements and pH from the study area are shown in Table 4 and Figure 3. Usually, a strong correlation between elements indicates that they come from the same source or have similar geochemical behaviors, while an uncorrelation between elements indicates that they come from different sources or have certain antagonism (Cai et al., 2021b; Chai et al., 2021). Table 4 and Figure 3 show that the pH value does not correlate with most of the heavy metal elements; it has a significant negative correlation with Pb, Ni, and Cr. Cr and Ni (correlation coefficient = 0.792) and Zn and Cd (correlation coefficient = 0.995) show a high correlation with each other. The other heavy metals are uncorrelated from each other, indicating that they come from different sources.
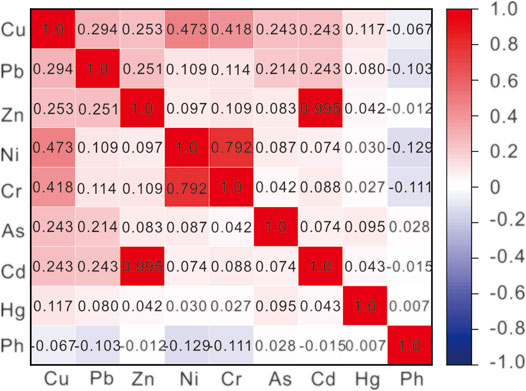
FIGURE 3. Pearson’s correlation coefficients among element concentrations of surface soil in Weifang.
PMF Analysis
The analysis in this study was done using the EPA-PMF 5.0 software. The factors were set to 5, 6, and 7, respectively. The model ran 20 times in each iteration. When the number of factors was 6, the difference between Qrobust and Qtrue was the smallest, and most of the residuals were between 3 and −3. The determination coefficients (R2) between the observed and predicted values of Cu, Pb, Zn, Ni, Cr, As, Cd, and Hg are 0.98, 0.99, 0.99, 0.93, 0.78, 0.99, 0.99, and 0.99 respectively (Figure 4), indicating that the PMF model uses a reasonable number of factors to fully interpret the information contained in the original data. The results of the contributions of six factors to each heavy metal in the soil in Weifang are shown in Figure 5.
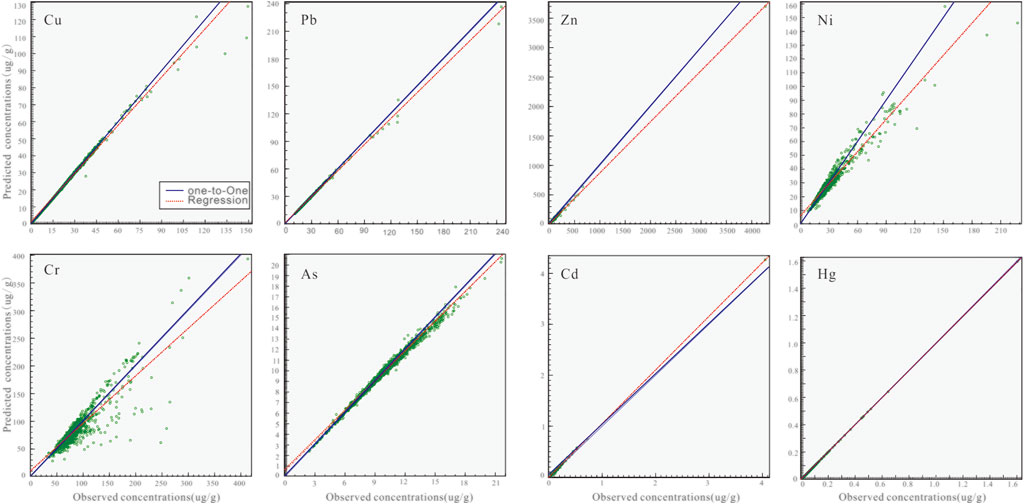
FIGURE 4. Comparisons of observed concentrations and predicted values for the surface soil in Weifang.
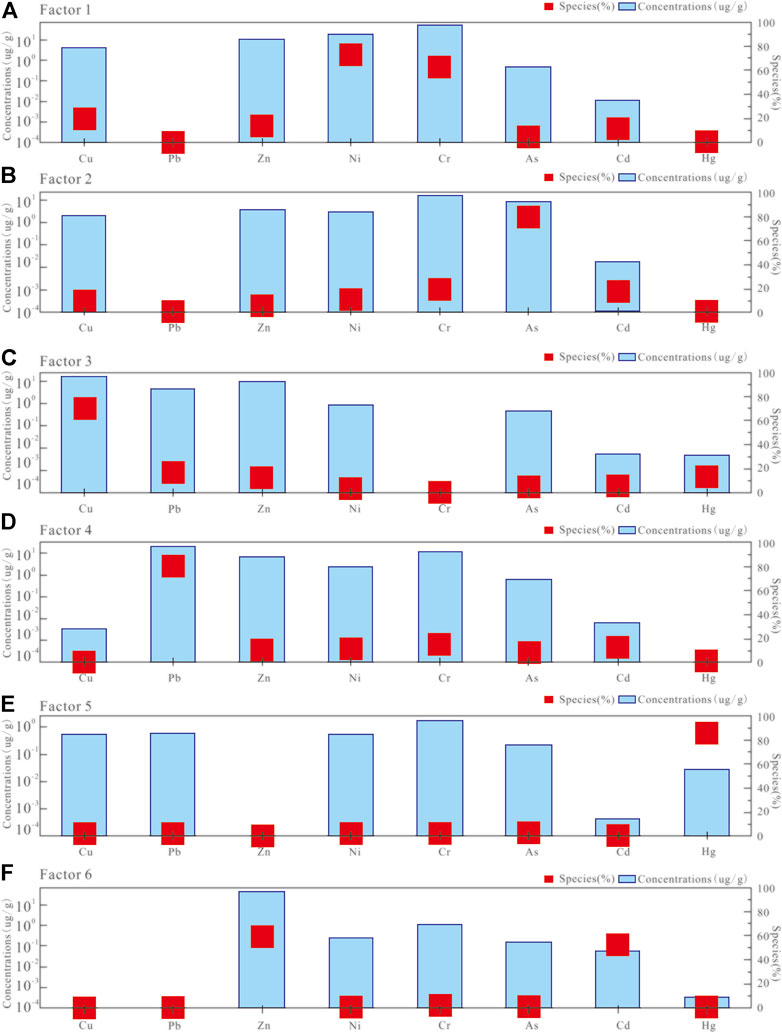
FIGURE 5. Contribution of factor 1 (A), factor 2 (B), factor 3 (C), factor 4 (D), factor 5 (E), and factor 6 (F) to each heavy metal of surface soil in Weifang.
Factor 1 has a high contribution to Ni and Cr elements, with contribution rates of 73.3 and 50.3%, respectively (Figure 5A). The results in Table 1 indicate that the average values of Ni and Cr in the samples are not higher than the national soil pollution risk screening value. However, the contents of Ni and Cr in some samples were at mild to medium pollution levels, and Ni in some samples was of medium ecological risk. Generally, Ni and Cr mainly come from soil parent material components (Manta et al., 2002; Cai et al., 2010; Nanos and Martin, 2012). However, studies have shown that due to the impact of human agricultural activities, Cr pollution in the soil can increase, especially when there is a shortage of water resources, and the rising cost of chemical fertilizers also plays a role (Luo et al., 2009; Zhang et al., 2016). In some instances, farmers also use untreated sewage to irrigate and fertilize farmland. However, as a coastal city, Weifang is rich in water resources, and sewage irrigation is not a source of Cr pollution in the study area. In addition, some studies show that Cr and Ni in the soil also come from industrial activities, such as perennial mining as well as smelting, coal consumption, steel production, and metal processing (Li et al., 2011; Chen et al., 2016). The nonferrous metal processing base in Weifang is mainly concentrated in Linqu County and Changle County, which is consistent with the regional characteristics of the high-value distribution of Cr and Ni in the western study area (Figure 2). Therefore, factor 1 is mainly the source of non-ferrous metals in the soil from industrial activities.
Factor 2 has the highest contribution to As, at 79% (Figure 5B). Some studies have shown that coal contains a lot of As (Raja et al., 2014). When it is burned, a large amount of containing fly ash will eventually precipitate into the soil. In the study area, cement plants and brick factories are distributed near the areas with high values of As. Therefore, factor 2 is related to coal-related industrial activities.
Factor 3 mainly contributes to Cu, with a contribution rate of 69.6% (Figure 5C). There is a high content of Cu in diesel vehicle exhaust (Aurélie et al., 2019). Weifang is an important industrial and agricultural production location in Shandong Province. Road transportation enables products to be sold in bulk all over the country. A large amount of tail gas emissions are also generated in the process of agricultural mechanization production. Cu is enriched in the surrounding soil through dust settlement. Therefore, factor 3 represents transportation and agricultural machinery activities related to diesel fuel.
Factor 4 mainly contributes to Pb, with a contribution rate of 80.7% (Figure 5D). Lead is a major indicator of traffic emissions and comes from the combustion of gasoline fuel and the use of engines and catalysts (Arditsoglou and Samara, 2005; Hjortenkrans et al., 2017). Motor vehicles, agricultural machinery, and equipment emit waste gas containing lead, resulting in soil pollution along the road. In addition, it can be seen from the high-value distribution map of Pb that the main distribution is related to the traffic activity in the urban area. Therefore, factor 4 is related to gasoline traffic activities.
Factor 5 mainly contributes to Hg, with a contribution rate of 85.4% (Figure 5E). One of the components of pesticides is Hg, which is volatile and mobile (Giersz et al., 2017). The heavy use of pesticides will increase the content of Hg in soil. As an important agricultural production city in Shandong Province, factor 5 should be related to the use of pesticides during agricultural production activities.
Factor 6 mainly contributes to Cd and Zn elements, with contribution rates of 52.8 and 59.3%, respectively (Figure 5F). It is generally believed that fertilizers, plastic films, atmospheric deposition, and sludge irrigation lead to the accumulation of Cd in soil (Younger et al., 2002; Kim et al., 2021). When it comes to agricultural activities, the long-term and large-scale use of heat stabilizers and Cd-containing fertilizers also lead to Cd enrichment in soil (Kim et al., 2021). The application of organic fertilizers, such as animal manure, is also an important source of Cd and Zn. Sampling investigation found that the application of organic fertilizers such as animal manure is common in the study area. Therefore, factor 6 is related to organic fertilizers used in agricultural and animal husbandry activities.
Conclusion
(1) The average values of the eight heavy metal elements analyzed in this study in the soil of Weifang are higher than the soil background value of Shandong Province, but they do not exceed the reference value of the national environmental quality agricultural land soil pollution risk control standard.
(2) According to the analysis of the ecological risk of heavy metals in the study area, the ecological risks of Cu, Pb, Zn, Ni, Cr, and As in the study area are relatively safe, but the ecological risks of Cd and Hg are relatively higher.
(3) The sources of heavy metals in the soil in the study area can be divided into six categories: Cr and Ni were attributed to soil parent material and nonferrous metal industrial activities, As was attributed to coal-related industrial activities, Cu was closely related to diesel fuel-related transportation and agricultural machinery activities, Pb was attributed to gasoline transportation activities, Hg was attributed to agricultural production activities and pesticide use, and Cd and Zn were attributed to organic fertilizers used in agricultural and animal husbandry activities.
Data Availability Statement
The original contributions presented in the study are included in the article/Supplementary Material, further inquiries can be directed to the corresponding author.
Author Contributions
YL: writing-original draft; ZX: software; HR: methodology, drawing; DW: investigation; JW: investigation; ZW: data curation; PC: writing–review and editing.
Funding
This work was supported by the Weifang City Coastal Zone Comprehensive Geological Survey Project under the grant Weidi Kanzi 2015-2 and the Weifang City Land Quality Geochemical Survey and Evaluation Project under the grant ZFCG-2020-200.
Conflict of Interest
The authors declare that the research was conducted in the absence of any commercial or financial relationships that could be construed as a potential conflict of interest.
Publisher’s Note
All claims expressed in this article are solely those of the authors and do not necessarily represent those of their affiliated organizations, or those of the publisher, the editors, and the reviewers. Any product that may be evaluated in this article, or claim that may be made by its manufacturer, is not guaranteed or endorsed by the publisher.
Acknowledgments
We are greatly indebted to Editor for efficient handling and constructive comments. We are also appreciating to the two reviewers for their insightful comments, which greatly improved our paper for better expression and quality.
References
Akbarpour, F., Gitipour, S., Baghdadi, M., and Mehrdadi, N. (2021). Correlation between Chemical Fractionation of Heavy Metals and Their Toxicity in the Contaminated Soils. Environ. Earth Sci. 80, 726. doi:10.1007/s12665-021-10024-x
Ambade, B., Kumar, A., Kumar, A., and Sahu, L. K. (2021b). Temporal Variability of Atmospheric Particulate-Bound Polycyclic Aromatic Hydrocarbons (PAHs) over central East India: Sources and Carcinogenic Risk Assessment. Air Qual. Atmos. Health 15, 115–130. doi:10.1007/s11869-021-01089-5
Ambade, B., Sankar, T. K., Kumar, A., Gautam, A. S., and Gautam, S. (2021). Covid-19 Lockdowns Reduce the Black Carbon and Polycyclic Aromatic Hydrocarbons of the Asian Atmosphere: Source Apportionment and Health hazard Evaluation. Environ. Dev. Sustainability (3), 1–20. doi:10.1007/s10668-020-01167-1
Ambade, B., Sankar, T. K., Panicker, A. S., Gautam, A. S., and Gautam, S. (2021c). Characterization, Seasonal Variation, Source Apportionment and Health Risk Assessment of Black Carbon over an Urban Region of East India. Urban Clim. 38, 100896. doi:10.1016/j.uclim.2021.100896
Ambade, B., Sethi, S. S., Giri, B., Biswas, J. K., and Bauddh, K. (2022). Characterization, Behavior, and Risk Assessment of Polycyclic Aromatic Hydrocarbons (PAHs) in the Estuary Sediments. Bull. Environ. Contam. Toxicol. 108, 243–252. doi:10.1007/s00128-021-03393-3
Arditsoglou, A., and Samara, C. (2005). Levels of Total Suspended Particulate Matter and Major Trace Elements in Kosovo: a Source Identification and Apportionment Study. Chemosphere 59 (5), 669–678. doi:10.1016/j.chemosphere.2004.10.056
Buat-Menard, P., and Chesselet, R. (1979). Variable Influence of the Atmospheric Flux on the Trace Metal Chemistry of Oceanic Suspended Matter. Earth Planet. Sci. Lett. 42, 399–411. doi:10.1016/0012-821x(79)90049-9
Burges, A., Epelde, L., and Garbisu, C. (2015). Impact of Repeated Single-Metal and Multi-Metal Pollution Events on Soil Quality. Chemosphere 120, 8–15. doi:10.1016/j.chemosphere.2014.05.037
Cai, L., Huang, L., Zhou, Y., Xu, Z., Peng, X., Yao, L. a., et al. (2010). Heavy Metal Concentrations of Agricultural Soils and Vegetables from Dongguan, Guangdong. J. Geogr. Sci. 20, 121–134. doi:10.1007/s11442-010-0121-1
Cai, P., Cai, G., Chen, X., Li, S., and Zhao, L. (2021b). The Concentration Distribution and Biohazard Assessment of Heavy Metal Elements in Surface Sediments from the continental Shelf of Hainan Island. Mar. Pollut. Bull. 166, 112254. doi:10.1016/j.marpolbul.2021.112254
Cai, P., Cai, G., Li, X., Chen, X., Lin, J., Li, S., et al. (2021a). Distribution and Source Determination of Rare Earth Elements in Sediment Collected from the continental Shelf off Hainan Island, China. Environ. Sci. Pollut. Res. 29, 3062–3071. doi:10.1007/s11356-021-15818-x
Cao, H., Chen, J., Zhang, J., Zhang, H., Qiao, L., and Men, Y. (2010). Heavy Metals in rice and Garden Vegetables and Their Potential Health Risks to Inhabitants in the Vicinity of an Industrial Zone in Jiangsu, China. J. Environ. Sci. 22 (11), 1792–1799. doi:10.1016/s1001-0742(09)60321-1
Cao, J.-j., Wang, Q.-y., Chow, J. C., Watson, J. G., Tie, X.-x., Shen, Z.-x., et al. (2012). Impacts of Aerosol Compositions on Visibility Impairment in Xi'an, China. Atmos. Environ. 59, 559–566. doi:10.1016/j.atmosenv.2012.05.036
Chai, L., Wang, Y., Wang, X., Ma, L., Cheng, Z., and Su, L. (2021). Pollution Characteristics, Spatial Distributions, and Source Apportionment of Heavy Metals in Cultivated Soil in Lanzhou, China. Ecol. Indicators 125, 107507. doi:10.1016/j.ecolind.2021.107507
Charron, A., Polo-Rehn, L., Besombes, J.-L., Golly, B., Buisson, C., Chanut, H., et al. (2019). Identification and Quantification of Particulate Tracers of Exhaust and Non-exhaust Vehicle Emissions. Atmos. Chem. Phys. 19 (7), 5187–5207. doi:10.5194/acp-19-5187-2019
Chen, H., Wang, L., Hu, B., Xu, J., and Liu, X. (2022). Potential Driving Forces and Probabilistic Health Risks of Heavy Metal Accumulation in the Soils from an E-Waste Area, Southeast China. Chemosphere 289, 133182. doi:10.1016/j.chemosphere.2021.133182
Chen, H., Teng, Y., Lu, S., Wang, Y., Wu, J., and Wang, J. (2016). Source Apportionment and Health Risk Assessment of Trace Metals in Surface Soils of Beijing Metropolitan, China. Chemosphere 144, 1002–1011. doi:10.1016/j.chemosphere.2015.09.081
Chen, M., Chen, X., Xing, Y., Liu, Y., Zhang, S., Zhang, D., et al. (2021). Arsenic and Cadmium in Soils from a Typical Mining City in Huainan, China: Spatial Distribution, Ecological Risk Assessment, and Health Risk Assessment. Bull. Environ. Contam. Toxicol. 107, 1080–1086. doi:10.1007/s00128-021-03278-5
Facchinelli, A., Sacchi, E., and Mallen, L. (2001). Multivariate Statistical and GIS-Based Approach to Identify Heavy Metal Sources in Soils. Environ. Pollut. 114, 313–324. doi:10.1016/s0269-7491(00)00243-8
Fang, H., Gui, H., Li, J., Yu, H., Wang, M., Jiang, Y., et al. (2021). Risks Assessment Associated with Different Sources of Metals in Abandoned Soil of Zhuxianzhuang Coal Mine, Huaibei coalfield (Anhui, China). Bull. Environ. Contam. Toxicol. 106 (2), 370–376. doi:10.1007/s00128-020-03069-4
Gao, L., Hu, X., Ma, C., Kuang, H., Qi, H., and He, Z. (2020). Geoenvironmental Risk Evaluation of High-Efficiency Eco-Economic Zone in Weifang City, China. Nat. Hazards Rev. 21 (3), 05020005. doi:10.1061/(asce)nh.1527-6996.0000384
Giersz, J., Bartosiak, M., and Jankowski, K. (2017). Sensitive Determination of Hg Together with Mn, Fe, Cu by Combined Photochemical Vapor Generation and Pneumatic Nebulization in the Programmable Temperature Spray Chamber and Inductively Coupled Plasma Optical Emission Spectrometry. Talanta 167, 279–285. doi:10.1016/j.talanta.2017.02.018
Guan, Q., Wang, F., Xu, C., Pan, N., Lin, J., Zhao, R., et al. (2018). Source Apportionment of Heavy Metals in Agricultural Soil Based on PMF: a Case Study in Hexi Corridor, Northwest China. Chemosphere 193, 189–197. doi:10.1016/j.chemosphere.2017.10.151
Hakanson, L. (1980). An Ecological Risk index for Aquatic Pollution control.a Sedimentological Approach. Water Res. 14, 975–1001. doi:10.1016/0043-1354(80)90143-8
Hasan, A. B., Kabir, S., Selim Reza, A. H. M., Nazim Zaman, M., Ahsan, A., and Rashid, M. (2013). Enrichment Factor and Geo-Accumulation index of Trace Metals in Sediments of the Ship Breaking Area of Sitakund Upazilla (Bhatiary-Kumira), Chittagong, Bangladesh. J. Geochemical Exploration 125, 130–137. doi:10.1016/j.gexplo.2012.12.002
Heidari, M., Darijani, T., and Alipour, V. (2021). Heavy Metal Pollution of Road Dust in a City and its Highly Polluted Suburb; Quantitative Source Apportionment and Source-specific Ecological and Health Risk Assessment. Chemosphere 273, 129656. doi:10.1016/j.chemosphere.2021.129656
Hjortenkrans, D., Bergbäck, B., and Häggerud, A. (2006). New Metal Emission Patterns in Road Traffic Environments. Environ. Monit. Assess. 117 (1–3), 85–98. doi:10.1007/s10661-006-7706-2
Hu, X., Ma, C., Huang, P., and Guo, X. (2021)., 125. China, 107464. doi:10.1016/j.ecolind.2021.107464Ecological Vulnerability Assessment Based on AHP-PSR Method and Analysis of its Single Parameter Sensitivity and Spatial Autocorrelation for Ecological protection - A Case of Weifang City, ChinaEcol. Indicators
Hu, Z., Wang, C., Li, K., and Zhu, X. (2018). Distribution Characteristics and Pollution Assessment of Soil Heavy Metals over a Typical Nonferrous Metal Mine Area in Chifeng, Inner Mongolia, china. Environ. Earth Sci. 77 (18), 638. doi:10.1007/s12665-018-7771-1
Jiang, Y., Chao, S., Liu, J., Yang, Y., Chen, Y., Zhang, A., et al. (2017). Source Apportionment and Health Risk Assessment of Heavy Metals in Soil for a Township in Jiangsu Province, China. Chemosphere 168, 1658–1668. doi:10.1016/j.chemosphere.2016.11.088
Kim, D.-M., Kwon, O.-H., Oh, Y. S., and Lee, J.-S. (2021). Determination of Soil Contamination Sources in Mining Area Using Zn/Cd Ratios with mobile Cd. Environ. Geochem. Health 43, 4061–4074. doi:10.1007/s10653-021-00820-6
Kurwadkar, S., Dane, J., Kanel, S. R., Nadagouda, M. N., Cawdrey, R. W., Ambade, B., et al. (2022). Per- and Polyfluoroalkyl Substances in Water and Wastewater: A Critical Review of Their Global Occurrence and Distribution. Sci. Total Environ. 809, 151003. doi:10.1016/j.scitotenv.2021.151003
Li, C., Dai, Z., Liu, X., and Wu, P. (2020). Transport Pathways and Potential Source Region Contributions of pm2.5 in Weifang: Seasonal Variations. Appl. Sci. 10 (8), 2835. doi:10.3390/app10082835
Li, M., Zhang, Q., Sun, X., Karki, K., Zeng, C., Pandey, A., et al. (2020). Heavy Metals in Surface Sediments in the Trans-Himalayan Koshi River Catchment: Distribution, Source Identification, and Pollution Assessment. Chemosphere 244, 125410. doi:10.1016/j.chemosphere.2019.125410
Li, X.-H., Tang, Z.-L., Chu, F.-Y., and Yang, L.-Y. (2011). Characteristics of Distribution and Chemical Speciation of Heavy Metals in Environmental Mediums Around Jinchang Mining City, Northwest China. Environ. Earth Sci. 64 (6), 1667–1674. doi:10.1007/s12665-009-0438-1
Li, X., Wang, X., Chen, Y., Yang, X., and Cui, Z. (2019). Optimization of Combined Phytoremediation for Heavy Metal Contaminated Mine Tailings by a Field-Scale Orthogonal experiment. Ecotoxicology Environ. Saf. 168, 1–8. doi:10.1016/j.ecoenv.2018.10.012
Lin, Y.-P., Cheng, B.-Y., Shyu, G.-S., and Chang, T.-K. (2010). Combining a Finite Mixture Distribution Model with Indicator Kriging to Delineate and Map the Spatial Patterns of Soil Heavy Metal Pollution in Chunghua County, central Taiwan. Environ. Pollut. 158, 235–244. doi:10.1016/j.envpol.2009.07.015
Lokeshwari, H., and Chandrappa, G. (2006). Impact of Heavy Metal Contamination of Bellandur Lake on Soil and Cultivated Vegetation. Curr. Sci. 91 (5), 622–627.
Luo, L., Ma, Y., Zhang, S., Wei, D., Zhu, Y.-G., and Zhu, Y. G. (2009). An Inventory of Trace Element Inputs to Agricultural Soils in China. J. Environ. Manag. 90 (8), 2524–2530. doi:10.1016/j.jenvman.2009.01.011
Maharjan, L., Tripathee, L., Kang, S. C., Ambade, B., Chen, P. F., Zheng, H. J., et al. (2021). Characteristics of Atmospheric Particle-Bound Polycyclic Aromatic Compounds over the Himalayan Middle Hills: Implications for Sources and Health Risk Assessment. Asian J. Atmos. Environ. 15 (4), 2021101. doi:10.5572/ajae.2021.101
Manta, D. S., Angelone, M., Bellanca, A., Neri, R., and Sprovieri, M. (2002). Heavy Metals in Urban Soils: a Case Study from the City of Palermo (Sicily), Italy. Sci. Total Environ. 300 (1–3), 229–243. doi:10.1016/s0048-9697(02)00273-5
Nanos, N., and Rodríguez Martín, J. A. (2012). Multiscale Analysis of Heavy Metal Contents in Soils: Spatial Variability in the Duero River basin (Spain). Geoderma 189-190, 554–562. doi:10.1016/j.geoderma.2012.06.006
Pekey, H., Karakaş, D., and Bakogˇlu, M. (2004). Source Apportionment of Trace Metals in Surface Waters of a Polluted Stream Using Multivariate Statistical Analyses. Mar. Pollut. Bull. 49, 809–818. doi:10.1016/j.marpolbul.2004.06.029
Qin, G., Niu, Z., Yu, J., Li, Z., Ma, J., and Xiang, P. (2021). Soil Heavy Metal Pollution and Food Safety in china: Effects, Sources and Removing Technology. Chemosphere 267, 129205. doi:10.1016/j.chemosphere.2020.129205
Raja, R., Nayak, A. K., Rao, K. S., Puree, C., Shahid, M., Panda, B. B., et al. (2014). Effect of Fly Ash Deposition on Photosynthesis, Growth, and Yield of rice. Bull. Environ. Contam. Toxicol. 93, 106–112. doi:10.1007/s00128-014-1282-x
Rodenburg, L. A., Du, S., Xiao, B., and Fennell, D. E. (2011). Source Apportionment of Polychlorinated Biphenyls in the New York/New Jersey Harbor. Chemosphere 83, 792–798. doi:10.1016/j.chemosphere.2011.02.058
Solomon, A., Rasheed, K., and Olanipekun, E. A. (2016). Concentration of Organophosphorous Pesticide Residues in Water and Sediment Samples from River Ilaje, Nigeria. Acsj 11, 1–9. doi:10.9734/acsj/2016/22077
Sun, Y., Zhou, Q., Xie, X., and Liu, R. (2010). Spatial, Sources and Risk Assessment of Heavy Metal Contamination of Urban Soils in Typical Regions of Shenyang, china. J. Hazard. Mater. 174 (1-3), 455–462. doi:10.1016/j.jhazmat.2009.09.074
Tan, J., Duan, J., Ma, Y., He, K., Cheng, Y., Deng, S.-x., et al. (2016). Long-term Trends of Chemical Characteristics and Sources of fine Particle in Foshan City, Pearl River Delta: 2008-2014. Sci. total Environ. 565, 519–528. doi:10.1016/j.scitotenv.2016.05.059
USEPA (1986). Guidelines for the Health Risk Assessment of Chemical Mixtures. Fed. Reg. 51, 34014–34025.
Wang, S., Cai, L.-M., Wen, H.-H., Luo, J., Wang, Q.-S., and Liu, X. (2019). Spatial Distribution and Source Apportionment of Heavy Metals in Soil from a Typical County-Level City of Guangdong Province, China. Sci. total Environ. 655, 92–101. doi:10.1016/j.scitotenv.2018.11.244
Wei, B., and Yang, L. (2010). A Review of Heavy Metal Contaminations in Urban Soils, Urban Road Dusts and Agricultural Soils from china. Microchemical J. 94 (2), 99–107. doi:10.1016/j.microc.2009.09.014
Wilding, L. (1985). , 30, 166–194.Spatial Variability: its Documentation, Accommodation and Implication to Soil Surveys. Soil Spatial VariabilityLas Vegas NV
Wu, J., Lu, J., Li, L., Min, X., and Luo, Y. (2018). Pollution, Ecological-Health Risks, and Sources of Heavy Metals in Soil of the Northeastern Qinghai-Tibet Plateau. Chemosphere 201, 234–242. doi:10.1016/j.chemosphere.2018.02.122
Yao, Y., Li, J., He, C., Hu, X., Yin, L., Zhang, Y., et al. (2021). Distribution Characteristics and Relevance of Heavy Metals in Soils and Colloids Around a Mining Area in Nanjing, China. Bull. Environ. Contam. Toxicol. 107, 996–1003. doi:10.1007/s00128-021-03350-0
Younger, P. L., Banwart, S. A., and Hedin, R. S. (2002). “Mine Water,” in Mine Water: Hydrology, Pollution, Remediation (Netherlands: Springer). doi:10.1007/978-94-010-0610-1
Zhang, J., Hua, P., and Krebs, P. (2017). Influences of Land Use and Antecedent Dry-Weather Period on Pollution Level and Ecological Risk of Heavy Metals in Road-Deposited Sediment. Environ. Pollut. 228, 158–168. doi:10.1016/j.envpol.2017.05.029
Keywords: heavy metals, soil, ecological risk, source, Weifang city
Citation: Li Y, Xu Z, Ren H, Wang D, Wang J, Wu Z and Cai P (2022) Spatial Distribution and Source Apportionment of Heavy Metals in the Topsoil of Weifang City, East China. Front. Environ. Sci. 10:893938. doi: 10.3389/fenvs.2022.893938
Received: 11 March 2022; Accepted: 05 April 2022;
Published: 29 April 2022.
Edited by:
Mohammad Saghir Khan, Aligarh Muslim University, IndiaReviewed by:
Balram Ambade, National Institute of Technology, Jamshedpur, IndiaYang Li, Anhui University of Science and Technology, China
Copyright © 2022 Li, Xu, Ren, Wang, Wang, Wu and Cai. This is an open-access article distributed under the terms of the Creative Commons Attribution License (CC BY). The use, distribution or reproduction in other forums is permitted, provided the original author(s) and the copyright owner(s) are credited and that the original publication in this journal is cited, in accordance with accepted academic practice. No use, distribution or reproduction is permitted which does not comply with these terms.
*Correspondence: Pengjie Cai, Y2FpcGVuZ2ppZUBuanUuZWR1LmNu