- 1School of Humanities and Law, Northeastern University, Shenyang, China
- 2Jangho Architecture College, Northeastern University, Shenyang, China
- 3Human Settlements Research Center, Liaoning Normal University, Dalian, China
- 4Key Laboratory of Regional Sustainable Development Modeling, Institute of Geographic Sciences and Natural Resources Research, CAS, Beijing, China
- 5School of Geography and Information Engineering, China University of Geosciences, Wuhan, China
- 6Department of Microbiology and Plant Biology, Center for Spatial Analysis, University of Oklahoma, Norman, OK, United States
- 7School of Earth and Planetary Sciences (EPS), Curtin University, Perth, WA, Australia
Resource-based urban agglomerations often encounter greater challenges in the sustainable development of human settlements. The aim of this study is to propose an approach to the coordinated development of competitiveness by analyzing the interaction of human settlements competitiveness (HSC) in resource-based urban agglomerations. Through the compound evaluation model of HSC and urban network analysis, this study finds: 1) the HSC measure increased from 35.12 in 1990 to 52.15 in 2015 and showed a downward trend from 2015 to 2019, with an average value of 47.82 in 2019; 2) The change trend of the relevance network density is the same as that of the HSC, while the difference network density reaches the lowest value of 0.441 when the HSC is the highest, indicating that the HSC of the urban agglomerations has improved to a certain extent but is more unsustainable, and 3) Communities in the relevance network are obviously bounded by the borders of provinces or urban agglomerations, while the communities in the difference network are differentiated into two types: high-competitiveness and low-competitiveness. Using the theory of “co-opetition” to analyze the sustainable development path of resource-based urban agglomerations, the study believes that a coordination mechanism and a guarantee mechanism for benefit distribution should be established between urban agglomerations to curb local protectionism, and promote regional dislocation development. The development gradient level also should be established within the urban agglomeration to narrow the gap between HSC of cities, and innovative development should be the core of promoting industrial transformation and upgrading.
1 Introduction
In the process of trade liberalization and economic globalization, urban agglomeration is a new regional unit for countries to participate in global competition and international division of labor (Ma et al., 2019), and its agglomeration benefit has become the key factor of economic development (Meijers and van der Wouw, 2019). As a spatial form with high concentration of resources, urban agglomeration has caused great pressure on regional land and ecosystem. Building compact cities is an effective way to improve land efficiency and curb disorderly spread (Yao et al., 2022). High-density and intensive model has a positive impact on economic sustainability, but has a negative impact on environmental and social sustainability (Lin and Yang, 2006). Therefore, it is necessary to consider the quality of human settlements in order to achieve three sustainable considerations (Westerink et al., 2013). In addition, the economic hinterland of cities within urban agglomerations must overlap with each other, the large demand for production factors such as free capital and high-level labor makes the competition between cities inevitable (Wang and Shen, 2017). However, under the guidance of “co-opetition” theory and “positive sum game” thinking, cities tend to establish a regional coordinated development model of cooperation in competition (Esty and Geradin, 2000; Li and Zhong, 2020). To understand the important role of human settlements in urban competition and promote the cooperation of urban agglomerations in competition to maximize regional interests, it is necessary to clarify the relationship between urban comparative competitive advantage and regional competition based on human settlements science.
Competitiveness was originally conceived as an economic concept to understand the competitive relationship between firms, whereas in urban studies, competitiveness is defined as a city’s comparative advantage relative to other cities, and a city’s ability to optimize its allocation to promote development (Song and Xie, 2021). In 1990s, the “Diamond model” (Portergoff, 1990) was developed to measure the relationship between national competitiveness and national economy and then to evaluate urban competitiveness (Rogerson, 1999). Ni et al. (2014), Ni et al. (2017) also regarded the economic performance of cities as a core factor to evaluate competitiveness and analyzed the driving factors of competitiveness using urban hard and soft factors. Since the ecological environment has a significant effect on human survival and development (Li et al., 2021; Liu D et al., 2021), competition between cities depends on the attractiveness of the urban environment, and thus ecological factors have started to be included in the construction of theoretical urban competitiveness models. Webster and Muller (2000) added territorial endowment factors to their competitiveness model, and Oensel et al. (2008) introduced environmental factors into their nation competitiveness model. Rapid and extensive urbanization and industrialization create severe problems for megacities, while small and medium-sized cities are facing population loss and lack of economic vitality (Bell and Gonzalez, 2011)The urban quality of life has become an important factor affecting the population’s willingness to stay in cities (Sørensen and Sørensen, 2015; Zhao et al., 2021) as reflected by the livability of cities (Liu et al., 2017). There is a symbiotic relationship between the urban quality of life and urban competitiveness (Esmaeilpoorarabi et al., 2016), and improving the quality of life has become the ultimate goal of enhancing urban competitiveness (Zhang and Corrie, 2018). Creating livable cities is the main goal of modern urban development (Liu and Wang, 2013), and the basis of urban competition has gradually shifted from economic strength to the sustainable development of human settlements. However, there is limited research on urban competitiveness based on the science of human settlements.
The high concentration of population and the rapid development of social economy in urban agglomerations lead to problems such as resource depletion, environmental pollution, traffic congestion and housing difficulties (Li et al., 2009), so in the long-term development planning, more attention should be paid to its sustainability (Ma et al., 2019). Human settlements refer to the unity of natural, humanistic, and spatial elements and to the geographical spaces where residents live, work, study, and carry out other activities (Mamat, 2012). The Sustainable Development Goals put forward the construction of sustainable cities and human settlements, which also causes global scholars to pay more attention to the human settlement environment. Wu (2001) first advocated human settlement science in China, and studied the spatial structure of human settlements from systematic and hierarchical points of view. Quantitative evaluation of human settlements is an important part of human settlement research, and data sources are key factors for evaluation. Statistical data reflect the macro-scale development of cities, and comprehensive evaluation of human settlements can be realized using the panel data of statistical yearbooks. Zhang and Fan, (2020) analyzed the driving mechanism of human settlements at the township level, and Tian et al. (2016) established a risk model to analyze the spatial-temporal evolution of human settlements at the provincial level in China. Multi-source big data, including remote sensing and meteorological data, can reflect the complex characteristics of human settlements, and grid-based accurate assessment of human settlements can be realized with the support of geographic information systems and spatial analysis methods. Based on this method, the natural suitability of urban human settlement environment has been effectively evaluated (Chen et al., 2021; Luo et al., 2021). The adjustment and optimization of urban internal structure can also effectively improve the living environment (Guo et al., 2020; Yang Y.et al., 2020; Ren et al., 2022). Yang Z. et al. (2020) found that residents seem to attach the greatest importance to quality of life facilities when choosing their residence. Patias et al. (2021) thought that the increase of urban walking space was the main reason for the improvement of urban structural sustainability. In addition, questionnaire data can directly reflect the subjective feelings of urban residents about their living environment, and quantitative analysis of the questionnaire results can reveal the livability score of human settlements (Simis et al., 2016; Yu et al., 2019). Current research on human settlement assessment reflects the spatial and temporal characteristics of human settlements but cannot directly measure the external impact of urban human settlements (Zachary, 2012). In this study, an urban network model was developed to explore the competitiveness of urban human settlements. Network models are popular in urban attraction and diffusion studies (Yuan et al., 2017). The GaWC research team was committed to establishing the network of major cities in the world and revealing the hierarchical characteristics of the global economy by analyzing changes in network integrity and node centrality caused by changes in urban competitiveness (Derudder and Taylor, 2016; Taylor and Derudder, 2021). Network modeling and analysis are widely used to describe the relative attractiveness of cities in terms of local economy (Huang et al., 2020), population growth (Yang et al., 2020), and transportation infrastructure (Wang et al., 2020).
Resource-based and industrial-based urban agglomerations are facing more severe problems in the process of sustainable development of human settlements. Urban agglomerations in Northeast China used to be the most important heavy industry base of China, with the most complete industrial system in Northeast Asia. With the advancement of economic and trade globalization, planned economic systems, and imbalance between supply and demand, the urban agglomerations have gradually produced “northeast problems,” including land disputes and societal conflicts (You et al., 2021), transformation of resource-based cities (Fu et al., 2020; Chen and Zhang, 2021), and urban environmental pollution, resulting in great resistance to the development of human settlements (H. Zhao et al., 2021; Zhao et al., 2013).
To help solve the “northeast problem” and enhance the attractiveness of human settlements in the urban agglomerations, we aim to address the following two research questions with empirical data: 1) how to establish a competitiveness model to reflect the attraction of human settlement environment to population and capital? and 2) how to understand the impact of the relevance and difference of human settlements on sustainable development in urban agglomerations and the wider region? Specifically, 1) a compound evaluation model was constructed to quantify the HSC of urban agglomerations over a long period, and spatial and temporal differentiation of human settlements in urban agglomerations was analyzed based on the evaluation results; and 2) a social network model was constructed to describe interactions between cities, and the relevance and difference coefficients of HSC were determined to explore the network spatial structure and evolution process of urban HSC in urban agglomerations. The study has reference value for promoting resource-based and industrial urban agglomerations to enhance their competitiveness of human settlements.
2 Data and Methods
2.1 Data
2.1.1 Study Area
The urban agglomerations in Northeast China are located at 38.72–49.44°N and 119.21°–135.09°E and cover a total area of 5.372 × 1011 km2. The area consists of five regional-level urban agglomerations and 29 prefecture-level cities, and the terrain is primarily plain and mountainous. The five regional urban agglomerations in Northeast China are Liaoning Coastal Urban Agglomeration, including Dalian, Dandong, Yingkou, Panjin, Jinzhou, and Huludao; Central Liaoning Urban Agglomeration, including Shenyang, Anshan, Fushun, Benxi Liaoyang, Tieling, and Fuxin; Central Jilin Urban Agglomeration, including Changchun and surrounding cities Jilin, Liaoyuan, Songyuan, and Siping; Harbin-Daqing-Qiqihar Urban Agglomeration including Harbin, Daqing, Qiqihar, and Suihua; and the planned Eastern Heilongjiang Urban Agglomeration including Mudanjiang, Jiamusi, Qitaihe Jixi, Shuangyashan, Hegang, and Yichun. As of 2019, the population of the region was 9.085 × 107, and per capita gross domestic product was 5.15×104 yuan. The administrative division map of the region is shown in Figure 1.
2.1.2 Index System
The scientific of human settlements proposed by Wu (2001) includes five subsystems: population, social, environmental, supporting, and housing. Based on these subsystems, we constructed an evaluation index system of HSC composed of multiple indices (Table 1). The population subsystem reflects changes in the urban population and employment structure, the social subsystem reflects urban economic growth and expansion, the environmental subsystem reflects urban greening and control of industrial pollution, the supporting subsystem reflects the development and construction of urban infrastructure and public services, and the residential subsystem reflects housing density and support.
2.1.3 Data Sources
We evaluated the HSC of the urban agglomerations in Northeast China for the years 1990, 1995, 2000, 2005, 2010, 2015, and 2019. The sources of statistical data, nighttime light data, and administrative division data are listed in Table 2. The statistical data were obtained from the China Urban Statistical Yearbook, Liaoning Statistical Yearbook, Jilin Statistical Yearbook, and Heilongjiang Statistical Yearbook from 1990 to 2020, which were downloaded from the National Bureau of Statistics of China. The nighttime light data were obtained from the National Oceanic and Atmospheric Administration (NOAA) National Centers for Environmental Information (NCEI) (formerly National Geophysical Data Center, NGDC). Administrative boundary data were obtained from the Resources and Environmental Science and Data Center, Institute of Geographical Sciences and Resources, Chinese Academy of Sciences, and the collection year was 2015.
2.2 Methods
2.2.1 Evaluation Model of HSC
In this study, we constructed a compound HSC evaluation model whose architecture is shown in Figure 2. Principal component analysis was used to reduce the number of initial indices and generate principal component indices (Meng and Chen, 2021). Using SPSS 26 software, 30 original indices were classified and dimensional reduction of the five subsystems was performed in the scientific of human settlements. Each subsystem formed two principal components, and then the original 30 indices were synthesized into 10 principal components. By analyzing the proportion of compound index components, the 10 principal components were named
Subsequently, the constant weight of the principal component set
The Lagrange multiplier method was used to combine the subjective and objective weights. The obtained constant weight
Considering the influence of index data on index weight, the penalty incentive variable weighting method was adopted to increase the weight of the principal component indices that are lower than the average value. The obtained variable weight matrix
where
The GRA-TOPSIS method was used to evaluate the HSC (Shi and Gan, 2020). The method can be used to reduce the influence of small sample size and extreme value effect on the evaluation results.
where
2.2.2 Urban Network Analysis
2.2.2.1 Network Metrics
The relevance coefficient of HSC (HSCR) constructed in this study is an extension of the economic gravity model (Fan et al., 2018). For any two cities in the network, the HSCR can be expressed by using Eq. 4:
where
The difference coefficient of HSC (HSCD) constructed in this study is based on the Gini coefficient, considering the spatial distance (Zhang et al., 2020). For any two cities in the network, HSCD can be expressed by using Eq. 5:
where
2.2.2.2 Characteristic Network Parameters
The complete social network was processed using the binary method, and the median of HSCR and HSCD in all time nodes was calculated as basis for screening. There will be a connection between the two cities, if either the HSCR between two cities is greater than the median, or if the HSCD between the two cities is lesser than the median.
The spatial-temporal patterns of the network structure are analyzed by calculating the network density, centrality, and community characteristics of social networks (Fan et al., 2018). Network density describes the closeness of connections between nodes in a social network and can be expressed as the ratio of the number of connections in the network to the maximum number of connections possible. Centrality is used to describe the central characteristics of nodes, including degree centrality, closeness centrality, and betweenness centrality, which represent the direct, indirect, and intermediary degrees between nodes, respectively. Community detection can measure the aggregation behavior of nodes in social networks and is used here to detect the aggregation patterns of cities in the HSC network.
3 Results
3.1 HSC
The HSC was evaluated using the GRA-TOPSIS comprehensive evaluation method combined with the weight matrix
Each urban agglomeration showed different development trends of HSC. As shown in Figure 4, the HSC of each city in the Eastern Heilongjiang Urban Agglomeration grew slowly, and the difference in HSC among cities was small; the polarization effect between Harbin-Daqing-Qiqihar Urban Agglomeration and Central Jilin Urban Agglomeration was evident. Daqing and Changchun were dominant in terms of HSC, whereas Suihua and Siping were in the inferior position in terms of HSC. Liaoning Coastal Urban Agglomeration and Central Liaoning Urban Agglomeration gradually developed into HSC hot spots, and the coordinated development ability of human settlements in urban agglomerations was satisfactory, Dalian and Shenyang have the highest HSC in their urban agglomerations.
3.2 Relevance Network Characteristics
The relevance coefficient matrix of HSC is shown in Figure 5. The relevance coefficient within urban agglomerations was higher than that between urban agglomerations. Central Liaoning Urban Agglomeration had high relevance of HSC with Liaoning Coastal Urban Agglomeration and Central Jilin Urban Agglomeration due to its location and competitive advantage. The structural characteristics of the relevance network of the HSC are shown in Figure 6. The density of the HSC relevance network showed an upward trend, reflecting that the relevance of the urban human settlements in northeastern cities was getting closer, but the upward trend gradually slowed down, and even negative growth occurred. The years with a density higher than the average of 0.5 include 2010, 2015, and 2019. The network density was the highest in 2015 (0.569). The average values of degree centrality, closeness centrality, and betweenness centrality of the HSC relevance network were 14, 0.72, and 9.45, respectively. The order of relevance of urban agglomerations was Central Jilin Urban Agglomeration > Central Liaoning Urban Agglomeration > Liaoning Coastal Urban Agglomeration > Harbin-Daqing-Qiqihar Urban Agglomeration > Eastern Heilongjiang Urban Agglomeration, and betweenness centrality measure showed that Central Jilin Urban Agglomeration and Harbin-Daqing-Qiqihar Urban Agglomeration had significant intermediary capabilities, with averages of 21.23 and 22.49, respectively. Central Liaoning Urban Agglomeration, Liaoning Coastal Urban Agglomeration, and Eastern Heilongjiang Urban Agglomeration had low intermediary capabilities (for Liaoning Coastal Urban Agglomeration, it is only 0.67). Community detection was carried out on the relevance network of HSC with the resolution of 1.0. The results showed that there are two main communities in the HSC relevance network, and the community boundaries coincided with the boundaries of urban agglomerations and provinces to a great extent, indicating that the association between HSC were significant in urban agglomerations within the same province, but not significant among inter-provincial urban agglomerations.
The relevance network of HSC in each urban agglomeration presents the following characteristics. 1) The degree centrality and closeness centrality of cities in Liaoning Coastal Urban Agglomeration were close to the average value, but the intermediary ability was lacking. Cities were closely related to HSC within urban agglomerations, while the HSC relevance between urban agglomerations was relatively low. 2) The cities in Central Liaoning Urban Agglomeration showed the central characteristics of high point-high closeness-low betweenness, and the competitiveness of human settlements was highly correlated, but the role of cities as a “bridge” for human settlements was weak; Fushun had the highest average degree centrality and closeness centrality among all cities, which were 19 and 0.833, respectively. 3) The cities in Central Jilin Urban Agglomeration showed the centrality characteristics of high degree-high closeness-high betweenness, and the centrality of cities was average. The betweenness centrality values of Changchun and Jilin were 29.10 and 29.51, respectively, which are important intermediary nodes. 4) The centrality polarization of cities in Harbin-Daqing-Qiqihar Urban Agglomeration was significant: the values for Harbin and Daqing were slightly higher than the average value, whereas those for Suihua and Qiqihar were lower than the average value. The betweenness centrality in Harbin was 61.85, which was the core intermediary node of the relevance network. 5) The cities in Eastern Heilongjiang Urban Agglomeration showed the centrality characteristics of low degree-low closeness-low betweenness, and Mudanjiang had the strongest centrality in this urban agglomeration and played the role of an intermediary node.
3.3 Difference Network Characteristics
The difference coefficient matrix of HSC is shown in Figure 7. The difference coefficient within urban agglomerations was lower than that between urban agglomerations, and HSC in Liaoning Coastal Urban Agglomeration was different from that of Harbin-Daqing-Qiqihar Urban Agglomeration and Eastern Heilongjiang Urban Agglomeration. The structural characteristics of the difference network of HSC are shown in Figure 8, and the difference in network density shows a wavy downward trend. The lowest network density value of 0.441 was achieved in 2015 with the highest average value of HSC, reflecting the uncoordinated development of HSC in urban agglomerations, with average values of degree centrality, closeness centrality, and betweenness centrality of the difference network were 14, 0.72, and 9.45, respectively. The order of difference of urban agglomerations was Harbin-Daqing-Qiqihar Urban Agglomeration > Eastern Heilongjiang Urban Agglomeration > Central Jilin Urban Agglomeration > Liaoning Coastal Urban Agglomeration > Central Liaoning Urban Agglomeration; Central Jilin Urban Agglomeration and Central Liaoning Urban Agglomeration had significant intermediary ability with average values of 14.74 and 13.13, respectively, whereas Harbin-Daqing-Qiqihar Urban Agglomeration, Eastern Heilongjiang Urban Agglomeration, and Liaoning Coastal Urban Agglomeration showed low intermediary ability. Community detection was carried out on the difference network of HSC with a resolution of 1.0. The results show two main communities in urban agglomerations (high-competitiveness and low-competitiveness communities). Overall, the number of cities in highly competitive communities increased.
The difference network of HSC in each urban agglomeration presents the following characteristics. 1) The degree centrality and closeness centrality of each city in the Central Liaoning Urban Agglomeration were higher than the average value, showing the centrality characteristics of high degree-high closeness-high betweenness; degree centrality, closeness centrality, betweenness centrality of Tieling were 21, 0.87, and 23.15, respectively, which were higher than those of other cities. 2) In the Liaoning Coastal Urban Agglomeration, the degree centrality and closeness centrality of Dandong, Jinzhou, Panjin, and Yingkou were higher than the average, whereas Dalian and Panjin had lower centrality than the average because of their high HSC. Dandong is an important intermediary node with betweenness centrality of 11.39. 3) The Central Jilin Urban Agglomeration shows the centrality characteristic of high degree-high closeness-high betweenness. The centrality of Changchun and Liaoyuan was higher than the average and showed a characteristic of low difference. All cities in the urban agglomeration except Jilin had significant intermediary function. 4) The cities in Harbin-Daqing-Qiqihar Urban Agglomeration showed the centrality characteristics of low degree-low closeness-low betweenness, and there were differences in human settlements inside and outside the urban agglomeration, where Suihua and Daqing experienced solitary point, and Harbin had the highest centrality in the urban agglomeration, with degree centrality, closeness centrality, and betweenness centrality values of 12.29, 0.70, and 2.48, respectively. 5) The cities in the Eastern Heilongjiang Urban Agglomeration showed the central characteristics of low degree-low closeness-low betweenness, and the urban agglomeration showed the characteristics of low internal difference and high external difference. Mudanjiang was the city with the highest centrality in the urban agglomeration, with degree centrality, closeness centrality, and betweenness centrality values of 16.29, 0.78, and 12.64, respectively.
4 Discussion
4.1 Scientificity and Accuracy of HSC
In terms of scientificity, different research frameworks have diversified the index system that reflects human settlements. Based on the scientific of human settlements proposed by Wu, (2001), we constructed a “five-in-one” index system of HSC, which takes “human” as its internal core factor, “economy” as its key factor, and “environment” as its important influencing factor. While considering natural factors, we highlight the key role played by social and economic factors in urban competition. Compared with previous research that mainly focused on the suitability and risk of human settlements (Tian et al., 2016; Li et al., 2018; Luo et al., 2021), the index system of HSC in our study reflects the attraction of human settlements to population and capital. In terms of accuracy, the evaluation model of HSC constructed in this study is an organic combination of the methods widely used in human settlement evaluation (Ma et al., 2016; Tang et al., 2017). We applied principal component analysis to reduce the overlapping effect of indices, the analytic hierarchy process and entropy weight method to realize the comprehensive consideration of subjective and objective decision-making factors, and the positive and negative ideal solutions and gray correlation degree to explore the synchronous changes in human settlements among cities to form HSC evaluation results.
In order to further prove the accuracy of HSC evaluation model, we used the floating population in the sixth national census of China (2010) to verify the extent to which HSC could reflect the attraction effect of cities on population. Specifically, the proportion of floating population to resident population was used to represent the relative population attraction capacity of cities based on their population carrying capacity, Figure 9A showed the correlation between HSC and the proportion of floating population. Pearson correlation coefficient of HSC and floating population was 0.771, showing a significant positive correlation between HSC and floating population, and R square was 0.579, indicating that there was a good linear correlation between HSC and floating population proportion. To sum up, the evaluation results of HSC by the evaluation model established in this study can significantly reflect the influence of the advantages or disadvantages of urban living environment on the residence willingness of floating population.
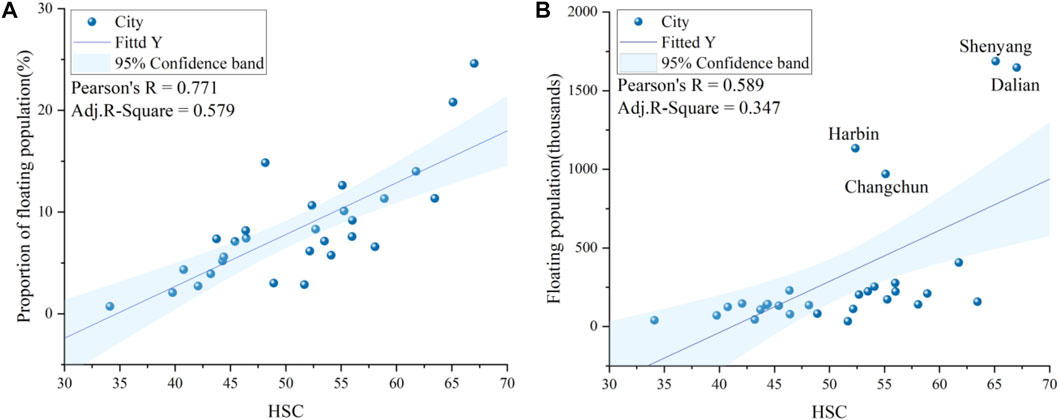
FIGURE 9. Correlation between floating population and HSC. (A) correlation between HSC and the proportion of floating population; (B) correlation between HSC and the number of floating population.
In addition, this study used the number of floating population to represent the absolute population attraction of cities. Figure 9B showed the correlation between HSC and the number of floating population. Pearson correlation coefficient between them was 0.589, and R square was 0.347. Although the correlation between HSC and the number of floating population also verified the effectiveness of HSC evaluation results to a certain extent, compared with the proportion of floating population, the interpretation strength declined. The reason lies in the existence of Changchun, Harbin, Dalian and Shenyang, which actually attract more floating population than their HSC should attract. These four cities are the core cities in the urban agglomeration where they are located, and they are also the provincial capitals and sub-provincial cities of the Northeast China. The advantages of employment, medical care and education resources brought by their political status are obviously more attractive to the floating population than those embodied by HSC, which attaches importance to the quality of ecological environment.
4.2 HSC Networks Evolution Mechanism
For HSC relevance networks, the network structure was most obviously improved between 2005–2010 (Figure 10A). This is mainly due to the implementation of the strategy of revitalizing the old industrial bases in Northeast China in 2003. Due to the promotion of policies, the social and economic development of cities in the Northeast region has been rapid, and the infrastructure has been further improved. Between 2010–2015, the upgrading rate of HSC network structure in Northeast urban agglomeration slowed down, and there was even a contraction between 2015–2019. This is because after the implementation of the strategy, most of the urban development in the urban agglomerations is still highly dependent on the development and utilization of resources and lacks the ability to innovate. The urban agglomerations pursue the rapid growth of the regional economy through over-exploitation of resources, resulting in a sharp decline in resource stock and extraction efficiency, and has not achieved the transformation and upgrading of the industrial structure. At the same time, this development model leads to rising costs and environmental degradation, which finally caused the global financial crisis in 2008 to have a serious impact on its development.
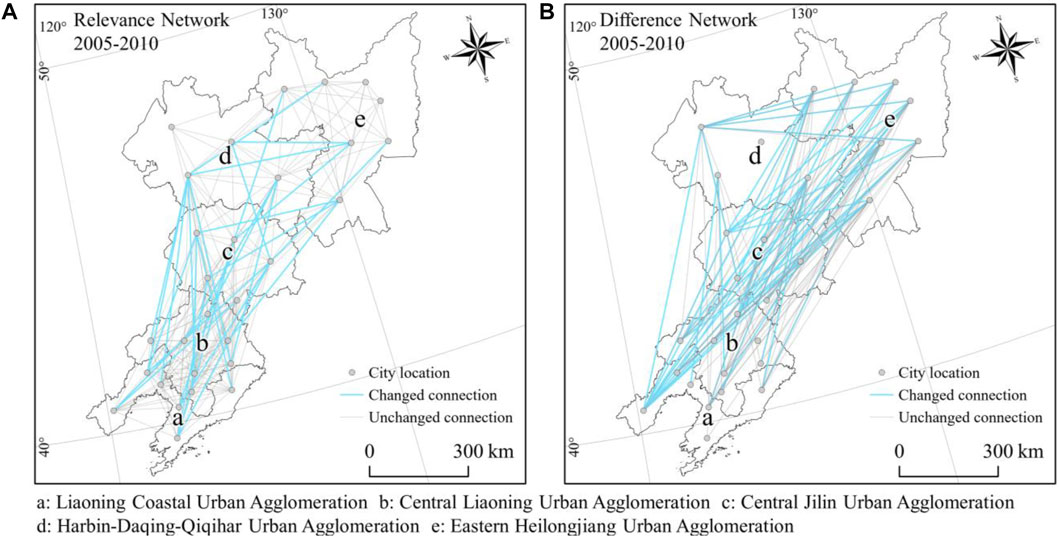
FIGURE 10. HSC networks evolution. (A) HSC relevance networks evolution; (B) HSC difference networks evolution.
In addition, we found that all the cities at both ends of the newly connected of relevance network have come from different urban agglomerations since 1995. Community detection results of relevance network showed that the influence of the two urban agglomerations in Liaoning province was increasing. This is mainly due to the fact that the Central Liaoning Urban Agglomeration and the Liaoning Coastal Urban Agglomeration have gradually developed into a window for the northeast region to communicate with the south of China and foreign countries. Especially, the coastal cities represented by Dalian have made great efforts to improve the ecological environment and develop diversified economy, and the competitive advantages brought by their human settlement environment have gradually enhanced the radiation ability. This result is similar to the related research on the evolution of the economic network structure of the urban agglomerations in Northeast China (Li, 2019; Jia, 2021).
For HSC difference network, we found that there were huge HSC differences inside and outside urban agglomerations. Moreover, HSC difference connection was more unstable (Figure 10B), indicating that the core cities in urban agglomerations failed to form a stable radiation-driven effect, and the development of human settlements in urban agglomerations was uncoordinated and unsustainable. The reason is that there are many resource-based cities in urban agglomerations, which are highly dependent on resources and weak in driving the surrounding cities. Restricted by regional economic development level, talents, labor and other factors, the overall differences in HSC between cities are huge. Shenyang, Dalian, Changchun and Harbin, the core cities of the four urban agglomerations in Northeast China, have strong regional influence and gather a lot of resources. Under the long-term effect of siphon effect in the four cities, Matthew effect is formed, in which the stronger the strong and the weaker the weak.
4.3 Sustainable Development of Human Settlements
From the evolution mechanism of HSC networks, we found that the relationship between human settlements in Northeast urban agglomerations at present was mostly competition rather than competition and cooperation, which was not conducive to the sustainable development of resource-based urban agglomerations. But in fact, the Northeast urban agglomeration has many competing conditions, but it has not been well utilized. First of all, the resources of each urban agglomeration are rich and complementary. Among them, Harbin-Daqing-Qiqihar and Eastern Heilongjiang urban agglomerations are rich in forest, cultivated land and oil resources, Central Jilin urban agglomeration are rich in natural gas, water and mountain resources, and Liaoning Coastal and Central Liaoning urban agglomerations are rich in marine resources and non-ferrous metal resources; Secondly, the industrial development pattern has made the infrastructure of the Northeast urban agglomerations increasingly perfect, especially the Northeast urban agglomeration has formed a comprehensive transportation system with staggered water, land and air, which has laid a good foundation for the urban agglomeration to enhance cooperation; Finally, the Northeast urban agglomerations is being supported by the second round of national policies such as “Revitalizing the Old Industrial Base in Northeast China,” and the policy opportunities are obvious.
Based on the theory of “co-opetition,” we believe that the competitiveness of human settlements in Northeast urban agglomerations can be promoted synergistically from the following aspects. First of all, coordination mechanism and benefit distribution guarantee mechanism should be established among urban agglomerations to curb local protectionism. The theory of “co-opetition” emphasizes that conflicts do not necessarily occur between different regions. As long as the interests of both sides are improved and local governments can make profits from such competition and cooperation, there are inherent incentives for competition and cooperation. In the early development process of Northeast urban agglomerations, the planning positioning was highly overlapping, which not only increased the cost, but also reduced the efficiency of resource allocation. Good division of labor and coordination can reduce the conflicts of interests among urban agglomerations, so that all urban agglomerations can obtain higher benefits, and then realize the sustainable development model.
Secondly, from the perspective of internal development of urban agglomerations, all urban agglomerations in Northeast China had different degrees of HSC polarization. The analysis of competition and cooperation shows that the agglomeration of cities with low HSC is not conducive to the improvement of competitiveness, and polarization will also have a negative impact on the balanced pattern (Liu R et al., 2021). For urban agglomerations in Northeast China, it is necessary to gradually narrow the HSC gap within urban agglomerations by establishing urban development gradient levels. In addition, considering the long-term competition and cooperation between cities, urban agglomerations should take innovation and development as the core, promote industrial transformation and upgrading, improve labor efficiency and factor allocation efficiency, and pay attention to ecological protection and environmental sustainable development, so as to drive regional HSC promotion.
4.4 Limitations
In this study, the urban agglomerations in Northeast China were taken as the research area, and the competitiveness of the urban human settlements was quantitatively evaluated from 1990 to 2019. The characteristics of the HSC network structure and its driving factors were analyzed. However, this study has some limitations. 1) The research time scale of this study is relatively macro, which reduces the availability of data. A better index system can be constructed in the future to cover the scientific of human settlements. 2) In this study, the Euclidean distance between urban administrative centers was used to express the urban interaction ability, and follow-up research can describe the interaction ability between cities more accurately.
5 Conclusion
In this study, a HSC compound evaluation model is established. Compared with the existing models, this model introduces the penalty variable weight method to increase the penalty for extreme disadvantage index, and introduces GRA-TOPSIS method to reduce the influence of small sample size and extreme value effect on the basis of considering subjective and objective factors. Using this model, this study evaluates the HSC in the urban agglomerations in Northeast China from 1990 to 2019 and quantifies the temporal and spatial patterns of the relevance and difference network structure based on the overall characteristics, node characteristics, and community characteristics. The main conclusions are as follows.
1) The HSC increased from 35.12 in 1990 to 52.15 in 2015 and showed a downward trend from 2015 to 2019, with an average value of 47.82 in 2019. The HSC of cities in the Eastern Heilongjiang Urban Agglomeration grew slowly, the difference in HSC among cities was small, and the polarization effect between Harbin-Daqing-Qiqihar Urban Agglomeration and Central Jilin Urban Agglomeration was evident. Liaoning Coastal Urban Agglomeration and Central Liaoning Urban Agglomeration gradually developed into HSC hot spots, and the coordinated development ability of human settlements in urban agglomerations was satisfactory.
2) The relevance coefficient within urban agglomerations was higher than that between urban agglomerations. The density of the HSC relevance network showed an upward trend, reflecting that the relevance of the urban human settlements in northeastern cities was getting closer, and the network density was the highest in 2015 (0.569). Central Jilin Urban Agglomeration and Harbin-Daqing-Qiqihar Urban Agglomeration had significant intermediary capabilities, with betweenness centrality values of 21.23 and 22.49, respectively. Community detection showed that the association between HSC exists are obviously bounded by the borders of provinces or urban agglomerations
3) The difference coefficient within urban agglomerations was lower than that between urban agglomerations. The difference in network density showed a wavy downward trend. The lowest network density value of 0.441 was achieved in 2015 with the highest average value of HSC, reflecting the uncoordinated development of HSC in urban agglomerations. Central Jilin Urban Agglomeration and Central Liaoning Urban Agglomeration had significant intermediary ability with betweenness centrality values of 14.74 and 13.13, respectively. Community detection showed two main communities in the urban agglomerations: high-competitiveness and low-competitiveness communities.
This study believes that the Northeast urban agglomeration has not yet formed an effective HSC development path of co-opetition. In order to promote the sustainable development of the human settlement environment of the Northeast urban agglomerations, a coordination mechanism and a benefit distribution guarantee mechanism should be established among urban agglomerations to curb local protectionism, break down institutional barriers and promote regional dislocation development. Urban development gradient levels should be established within urban agglomerations to narrow the gap between HSCs within urban agglomerations, and at the same time, innovation and development should be taken as the core to promote industrial transformation and upgrading.
Data Availability Statement
The raw data supporting the conclusion of this article will be made available by the authors, without undue reservation.
Author Contributions
WY performed the data analyses and wrote the manuscript; JY contributed to the conception of the study; HY contributed significantly to analysis and manuscript preparation; DS and XX helped perform the analysis with constructive discussions. YY and JX reviewed the manuscript and provided valuable review comments.
Funding
This research study was supported by the National Natural Science Foundation of China (grant no’s 41771178, 42030409, and 41671151), the Fundamental Research Funds for the Central Universities (grant no. N2111003), Basic Scientific Research Project (Key Project) of the Education Department of Liaoning Province (grant no. LJKZ0964), Natural Science Foundation of Guizhou Province (grant no. (2019)1150), the Second Tibetan Plateau Scientific Expedition and Research Program (STEP) (grant no. 2019QZKK1004).
Conflict of Interest
The authors declare that the research was conducted in the absence of any commercial or financial relationships that could be construed as a potential conflict of interest.
Publisher’s Note
All claims expressed in this article are solely those of the authors and do not necessarily represent those of their affiliated organizations, or those of the publisher, the editors and the reviewers. Any product that may be evaluated in this article, or claim that may be made by its manufacturer, is not guaranteed or endorsed by the publisher.
Acknowledgments
The authors would like to acknowledge all colleagues and friends who have voluntarily reviewed the translation of the survey and the manuscript of this study.
References
Bell, G., and Gonzalez, A. (2011). Adaptation and Evolutionary Rescue in Metapopulations Experiencing Environmental Deterioration. Science 332, 1327–1330. doi:10.1126/science.1203105
Chen, W., Zhu, K., Wu, Q., Cai, Y., Lu, Y., and Wei, J. (2021). Adaptability Evaluation of Human Settlements in Chengdu Based on 3S Technology. Environ. Sci. Pollut. Res. 29, 5988–5999. doi:10.1007/s11356-021-15998-6
Chen, Y., and Zhang, D. (2021). Multiscale Assessment of the Coupling Coordination between Innovation and Economic Development in Resource-Based Cities: A Case Study of Northeast China. J. Clean. Prod. 318, 128597. doi:10.1016/j.jclepro.2021.128597
Cong, Y., Xia, B., and Zhang, J. (2010). Associated Evaluation of Subjective and Objective of Human Settlement on a City Scale: A Case Study of Guangzhou City. Trop. Geogr. 30, 183–187. doi:10.13284/j.cnki.rddl.001349
Derudder, B., and Taylor, P. (2016). Change in the World City Network, 2000-2012. Prof. Geogr. 68, 624–637. doi:10.1080/00330124.2016.1157500
Esmaeilpoorarabi, N., Yigitcanlar, T., and Guaralda, M. (2016). Place Quality and Urban Competitiveness Symbiosis? A Position Paper. IJKBD 7, 4–21. doi:10.1504/IJKBD.2016.075444
Esty, D., and Geradin, D. (2000). Regulatory Co-opetition. J. Int. Econ. Law 3, 235–255. doi:10.1093/jiel/3.2.235
Fan, Y., Zhang, S., He, Z., He, B., Yu, H., Ye, X., et al. (2018). Spatial Pattern and Evolution of Urban System Based on Gravity Model and Whole Network Analysis in the Huaihe River Basin of China. Discrete Dyn. Nat. Soc. 2018, 1–11. doi:10.1155/2018/3698071
Fu, J., Xiao, G., and Wu, C. (2020). Urban Green Transformation in Northeast China: A Comparative Study with Jiangsu, Zhejiang and Guangdong Provinces. J. Clean. Prod. 273, 122551. doi:10.1016/j.jclepro.2020.122551
Guo, A., Yang, J., Xiao, X., Xia, , Jin, C., and Li, X. (2020). Influences of Urban Spatial Form on Urban Heat Island Effects at the Community Level in China. Sust. Cities Soc. 53, 101972. doi:10.1016/j.scs.2019.101972
Huang, Y., Hong, T., and Ma, T. (2020). Urban Network Externalities, Agglomeration Economies and Urban Economic Growth. Cities 107, 102882. doi:10.1016/j.cities.2020.102882
Jia, Z., and Gu, G. (2021). Is Local Government Intervention Effective in Promoting the tfp of an old Industrial Area? — a Spatial Econometric Analysis of Northeast China. Singap. Econ. Rev. 66, 933–951. doi:10.1142/S0217590820500319
Khorrami, Z., Ye, T., Sadatmoosavi, A., Mirzaee, M., Fadakar Davarani, M. M., and Khanjani, N. (2021). The Indicators and Methods Used for Measuring Urban Liveability: a Scoping Review. Rev. Environ. Health 36, 397–441. doi:10.1515/reveh-2020-0097
Li, F., Liu, X., Hu, D., Wang, R., Yang, W., Li, D., et al. (2009). Measurement Indicators and an Evaluation Approach for Assessing Urban Sustainable Development: A Case Study for China's Jining City. Landsc. Urban Plan. 90, 134–142. doi:10.1016/j.landurbplan.2008.10.022
Li, P., and Zhong, W. (2020). An Optimization Management Model for Countries with Mutually Competitive Regions. Sustainability 12, 2326. doi:10.3390/su12062326
Li, Q. (2019). Research on the Spatial Correlation Structure of Urban Agglomeration Economic Growth and its Effects in Northeast China. China: Northeast Normal University.
Li, X., Li, S., Gao, J., Zhao, P., and Li, H. (2018). Human Settlement Quality Evaluation Based on Air Quality in Major Cities of China. Adv. Meteorology 2018, 1–9. doi:10.1155/2018/4914760
Li, Z., Wang, J., Kong, X., Zhang, B., Liu, J., Ding, S., et al. (2021). Effects of Ecosystems Preservation on Economic Growth in China's Coastal Region: Multilevel Modelling and Exploration. Ecol. Indic. 132, 108224. doi:10.1016/j.ecolind.2021.108224
Lin, J.-J., and Yang, A.-T. (2006). Does the Compact-City Paradigm Foster Sustainability? an Empirical Study in Taiwan. Environ. Plann B Plann Des. 33, 365–380. doi:10.1068/b31174
Liu, D., Huang, A., Yang, D., Lin, J., and Liu, J. (2021). Niche-driven Socio-Environmental Linkages and Regional Sustainable Development. Sustainability 13, 1331. doi:10.3390/su13031331
Liu, R., Dong, X., Wang, X.-c., Zhang, P., Liu, M., and Zhang, Y. (2021). Study on the Relationship Among the Urbanization Process, Ecosystem Services and Human Well-Being in an Arid Region in the Context of Carbon Flow: Taking the Manas River Basin as an Example. Ecol. Indic. 132, 108248. doi:10.1016/j.ecolind.2021.108248
Liu, J., Nijkamp, P., Huang, X., and Lin, D. (2017). Urban Livability and Tourism Development in China: Analysis of Sustainable Development by Means of Spatial Panel Data. Habitat Int. 68, 99–107. doi:10.1016/j.habitatint.2017.02.005
Liu, K., and Wang, C. (2013). Progress and Prospect of Livable City Researches in China. J. Landsc. Res. 5, 2530–2626.
Liu, S., Li, W., and Wang, Q. (2018). Zoning Method for Environmental Engineering Geological Patterns in Underground Coal Mining Areas. Sci. Total Environ. 634, 1064–1076. doi:10.1016/j.scitotenv.2018.04.060
Luo, X., Yang, J., Sun, W., and He, B. (2021). Suitability of Human Settlements in Mountainous Areas from the Perspective of Ventilation: A Case Study of the Main Urban Area of Chongqing. J. Clean. Prod. 310, 127467. doi:10.1016/j.jclepro.2021.127467
Ma, J., Wang, J., and Szmedra, P. (2019). Economic Efficiency and its Influencing Factors on Urban Agglomeration-An Analysis Based on China's Top 10 Urban Agglomerations. Sustainability 11, 5380. doi:10.3390/su11195380
Ma, R., Wang, T., Zhang, W., Yu, J., Wang, D., Chen, L., et al. (2016). Overview and Progress of Chinese Geographical Human Settlement Research. J. Geogr. Sci. 26, 1159–1175. doi:10.1007/s11442-016-1320-1
Mamat, A. (2012). Evaluations of Living Environment Suitability of Hotan Prefecture in Xinjiang Based on GIS. Arid. Land Geogr. 35, 155–163. doi:10.13826/j.cnki.cn65-1103/x.2012.05.003
Meijers, E., and van der Wouw, D. (2019). Struggles and Strategies of Rural Regions in the Age of the 'urban Triumph'. J. Rural Stud. 66, 21–29. doi:10.1016/j.jrurstud.2019.01.027
Meng, Y., and Chen, L.-S. (2021). Evaluation of Urban Competitiveness in Environmental Protection Zones Based on Principal Component Analysis (Pca) and Entropy Method. J. Environ. Prot. Ecol. 22, 182–188.
Ni, P., Kamiya, M., and Ding, R. (2017). “Global Urban Competitiveness: Comparative Analysis from Different Perspectives,” in Cities Network along the Silk Road. Singapore: Springer Singapore), 51–64. doi:10.1007/978-981-10-4834-0_4
Ni, P., Kresl, P., and Li, X. (2014). China Urban Competitiveness in Industrialization: Based on the Panel Data of 25 Cities in China from 1990 to 2009. Urban Stud. 51, 2787–2805. doi:10.1177/0042098013514461
Önsel, Ş., Ülengin, F., Ulusoy, G., Aktaş, E., Kabak, Ö., and Topcu, Y. İ. (2008). A New Perspective on the Competitiveness of Nations. Socio-Economic Plan. Sci. 42, 221–246. doi:10.1016/j.seps.2007.11.001
Rogerson, R. J. (1999). Quality of Life and City Competitiveness. Urban Stud. 36, 969–985. doi:10.1080/0042098993303
Ren, J., Yang, J., Zhang, Y., Xiao, X., Li, X., Wang, S., et al. (2022). Exploring Thermal Comfort of Urban Buildings Based on Local Climate Zones. J. Clean Prod. 340, 130744. doi:10.1016/j.jclepro.2022.130744
Shi, H., and Gan, L. (2020). “Evaluation for Village Development Based on GRA-TOPSIS Method,” in Proceedings of the 6th International Conference on Environmental Science and Civil Engineering, Bristol, 455, 012194. doi:10.1088/1755-1315/455/1/012194
Simis, M., Awang, A., and Arifin, K. (2016). From Ex-Landfill to Public Park: Impact on Local Community's Quality of Life and Living Environment. Procedia - Soc. Behav. Sci. 222, 763–771. doi:10.1016/j.sbspro.2016.05.157
Song, M., and Xie, Q. (2021). Evaluation of Urban Competitiveness of the Huaihe River Eco-Economic Belt Based on Dynamic Factor Analysis. Comput. Econ. 58, 615–639. doi:10.1007/s10614-019-09952-5
Sørensen, J. F. L., and Sørensen, L. (2015). The Impact of Residential Environment Reputation on Residential Environment Choices. J Hous Built Environ 30, 403–425. doi:10.1007/s10901-014-9419-0
Tang, L., Ruth, M., He, Q., and Mirzaee, S. (2017). Comprehensive Evaluation of Trends in Human Settlements Quality Changes and Spatial Differentiation Characteristics of 35 Chinese Major Cities. Habitat Int. 70, 81–90. doi:10.1016/j.habitatint.2017.10.001
Taylor, P. J., and Derudder, B. (2021). NY‐LON 2020: The Changing Relations between London and New York in Corporate Globalisation. Trans Inst Br. Geog 47, 257–270. doi:10.1111/tran.12498
Tian, S., Li, X., Li, H., Zhang, Y., and Bao, T. (2016). Initial Evaluation of Provincial-Level Environmental Risks from the Perspective of Human Settlements. Sustainability 8, 1259. doi:10.3390/su8121259
Wang, J., Du, D., and Huang, J. (2020). Inter-city Connections in China: High-Speed Train vs. Inter-city Coach. J. Transp. Geogr. 82, 102619. doi:10.1016/j.jtrangeo.2019.102619
Wang, L., and Shen, J. (2017). Comparative Analysis of Urban Competitiveness in the Yangtze River Delta and Pearl River Delta Regions of China, 2000-2010. Appl. Spat. Anal. 10, 401–419. doi:10.1007/s12061-016-9210-6
Webster, D., and Muller, L. (2000). “Urban Competitiveness Assessment in Developing Country Urban Regions: The Road Forward,” in Paper Prepared for Urban Group, INFUD. Washington D.C: The World Bank), 47.
Westerink, J., Haase, D., Bauer, A., Ravetz, J., Jarrige, F., and Aalbers, C. B. E. M. (2013). Dealing with Sustainability Trade-Offs of the Compact City in Peri-Urban Planning across European City Regions. Eur. Plan. Stud. 21, 473–497. doi:10.1080/09654313.2012.722927
Wu, L. (2001). Introduction to Sciences of Human Settlements. Beijing: China Architecture and Building Press.
Xu, Y., Sun, J., Zhang, J., Xu, Y., Zhang, M., and Liao, X. (2012). Combining AHP with GIS in Synthetic Evaluation of Environmental Suitability for Living in China's 35 Major Cities. Int. J. Geogr. Inf. Sci. 26, 1603–1623. doi:10.1080/13658816.2011.642800
Yang, J., Wang, Y., Xiu, C., Xiao, X., Xia, J., and Jin, C. (2020). Optimizing Local Climate Zones to Mitigate Urban Heat Island Effect in Human Settlements. J. Clean Prod. 275, 123767. doi:10.1016/j.jclepro.2020.123767
Yang, Z., Gao, W., Zhao, X., Hao, C., and Xie, X. (2020). Spatiotemporal Patterns of Population Mobility and its Determinants in Chinese Cities Based on Travel Big Data. Sustainability 12, 4012. doi:10.3390/su12104012
Yao, Y., Pan, H., Cui, X., and Wang, Z. (2022). Do compact Cities Have Higher Efficiencies of Agglomeration Economies? A Dynamic Panel Model with Compactness Indicators. Land Use Policy 115, 106005. doi:10.1016/j.landusepol.2022.106005
You, H., Yang, J., Xue, B., Xiao, X., Xia, J., Jin, C., et al. (2021). Spatial Evolution of Population Change in Northeast China during 1992-2018. Sci. Total Environ. 776, 146023. doi:10.1016/j.scitotenv.2021.146023
Yu, S., Liu, Y., Cui, C., and Xia, B. (2019). Influence of Outdoor Living Environment on Elders' Quality of Life in Old Residential Communities. Sustainability 11, 6638. doi:10.3390/su11236638
Yuan, Z., Zheng, X., Zhang, L., and Zhao, G. (2017). Urban Competitiveness Measurement of Chinese Cities Based on a Structural Equation Model. Sustainability 9, 666. doi:10.3390/su9040666
Zachary, N. (2012). The Connected City: How Networks Are Shaping the Modern Metropolis. New York: Routledge.
Zhang, H., Geng, Z., Yin, R., and Zhang, W. (2020). Regional Differences and Convergence Tendency of Green Development Competitiveness in China. J. Clean. Prod. 254, 119922. doi:10.1016/j.jclepro.2019.119922
Zhang, X., and Corrie, B. P. (2018). “Urban Competitive Analysis,” in Investing in China and Chinese Investment Abroad (Singapore: Springer Singapore), 115–125. doi:10.1007/978-981-10-7983-2_10
Zhang, Y., and Fan, Q. (2020). The Application of the Fuzzy Analytic Hierarchy Process in the Assessment and Improvement of the Human Settlement Environment. Sustainability 12, 1563. doi:10.3390/su12041563
Zhao, H., Che, H., Zhang, X., Ma, Y., Wang, Y., Wang, X., et al. (2013). Aerosol Optical Properties over Urban and Industrial Region of Northeast China by Using Ground-Based Sun-Photometer Measurement. Atmos. Environ. 75, 270–278. doi:10.1016/j.atmosenv.2013.04.048
Zhao, H., Gui, K., Ma, Y., Wang, Y., Wang, Y., Wang, H., et al. (2021). Climatological Variations in Aerosol Optical Depth and Aerosol Type Identification in Liaoning of Northeast China Based on MODIS Data from 2002 to 2019. Sci. Total Environ. 781, 146810. doi:10.1016/j.scitotenv.2021.146810
Keywords: urban network analysis, compound evaluation model, relevance and difference coefficient, network evolution analysis, northeast China urban agglomerations
Citation: Yu W, Yang J, Sun D, Yu H, Yao Y, Xiao X and Xia J (2022) Spatial-Temporal Patterns of Network Structure of Human Settlements Competitiveness in Resource-Based Urban Agglomerations. Front. Environ. Sci. 10:893876. doi: 10.3389/fenvs.2022.893876
Received: 11 March 2022; Accepted: 27 April 2022;
Published: 30 May 2022.
Edited by:
Edyta Laszkiewicz, University of Łódź, PolandReviewed by:
Haozhi Pan, Shanghai Jiao Tong University, ChinaAdam Radzimski, Adam Mickiewicz University, Poland
Copyright © 2022 Yu, Yang, Sun, Yu, Yao, Xiao and Xia. This is an open-access article distributed under the terms of the Creative Commons Attribution License (CC BY). The use, distribution or reproduction in other forums is permitted, provided the original author(s) and the copyright owner(s) are credited and that the original publication in this journal is cited, in accordance with accepted academic practice. No use, distribution or reproduction is permitted which does not comply with these terms.
*Correspondence: Jun Yang, yangjun8@mail.neu.edu.cn; Dongqi Sun, sundq@igsnrr.ac.cn