- 1Key Laboratory of Middle Atmosphere and Global Environment Observation, Institute of Atmospheric Physics, Chinese Academy of Sciences, Beijing, China
- 2Collaborative Innovation Center on Forecast and Evaluation of Meteorological Disasters, Nanjing University of Information Science & Technology, Nanjing, China
- 3Shenzhen National Climate Observatory, Meteorological Bureau of Shenzhen Municipality, Shenzhen, China
Much attention has been paid to the rapid variation of aerosol loading in the urban areas of the Pearl River Delta (PRD) region. The baseline of aerosol loading in this rapidly developing region is critical in evaluating how and why the aerosol level has evolved, which absolutely requires long-term observations. Based on long-term observations of aerosol optical depth (AOD), visibility, and particulate matter (PM) mass concentrations at Xichong (114.56°E, 22.49°N), a background site in the PRD region, the variabilities of aerosol loading at multiple temporal scales are revealed. The means (±σ) of AOD, visibility, PM10, PM2.5, and PM1 are 0.38 ± 0.07, 12.6 ± 2.3 km, 23.7 ± 12.6 μg/m3, 19.7 ± 11.0 μg/m3, and 16.1 ± 10.1 μg/m3, respectively, which show that aerosol loading at the Xichong site is much lower than that in urban and suburban sites. Significant decreases in PM10, PM2.5, and PM1 mass concentrations are observed with magnitudes up to −2.13, −1.82, and −1.37 yr−1, respectively, at a 95% confidence level. The decrease in aerosol loadings at Xichong is attributed to the strict environmental regulations for improving air quality. Higher AOD and PM (lower visibility) values are observed during the early spring months as a result of long-range transport of biomass burning from Southeastern Asia. Diurnal variations of PM and visibility are dominantly determined by those of boundary layer height and relative humidity. PM mass concentrations show a generally negative (positive) correlation with visibility (AOD) at Xichong, but the correlations are weak with the R2 of 0.22 and 0.54, respectively. Low visibility and high aerosol loading are generally associated with very weak easterly and southerly winds. Understanding of variability of surface particle concentration and column-integrated aerosol loading at this background site in the PRD region would provide a scientific basis for the adoption of pollution prevention and control measures.
Introduction
The Pearl River Delta (PRD) region has experienced a rapid urbanization process for the past 40 years. High population densities and developed industries have resulted in severe air pollution in the PRD region. Analysis of the long-term (1954–2006) trend of visibility in an urban site in the PRD region showed that visibility has dramatically deteriorated since the 1980s as a result of a rapid increase in fine particle loading (Wu et al., 2007; Deng et al., 2008a).
To characterize the air pollution and improve the understanding of chemical and radiative processes in the atmosphere of the PRD, a couple of intensive field campaigns were performed, for example, the Program of Regional Integrated Experiments on Air Quality over the PRD of China (PRIDE-PRD) was conducted in 12 urban sites and 2 super sites from 4 Oct. to 5 Nov. 2004 (Hu et al., 2008; Liu et al., 2008; Zhang et al., 2008), in Jul. 2006 (Liu et al., 2010; Yue et al., 2013), and from 19 Oct. to 18 Nov. 2008 (Ma et al., 2017). The major contributor to air pollution is particulate matter (PM) in PRD, mainly fine particulate matter (PM2.5) emitted from fossil fuel, biomass burning, and urban construction (Cao et al., 2003; Wu et al., 2007). In addition to local anthropogenic emissions which are major driving forces for poor air quality, the smoke plume of agricultural residue burning in Southeastern Asia is also transported to the downwind PRD that often occurs during dry seasons such as early spring (Deng et al., 2008b).
The air pollution in the PRD region has attracted attention from both the scientific community and policymakers. A series of air pollution control measures have been undertaken, and the air quality has been improved since 2014 (Yu et al., 2009; Wang G. et al., 2019; Wu et al., 2019). These previous studies mainly focused on observations of surface PM mass concentration (Xia et al., 2017; Kong et al., 2020), carbonaceous aerosol properties (Cao et al., 2003; Lan et al., 2011), and related chemical apportionment (Liu et al., 2008; Huang et al., 2011) based on some short-term intensive campaigns. Recently, Fang et al. (2019) investigated the spatial-temporal characteristics of PM concentrations in the Guangdong–Hong Kong–Macao Greater Bay Area (GBA) of China using daily observations data during 2015–2017. It was emphasized that there are different major air quality issues in the GBA cities due to the differences in pollution sources, meteorological factors, and synergic pollution control policy.
Previous studies are mainly based on observations of short-term field campaigns (Ansmann et al., 2005; Cheng et al., 2008; Hu et al., 2008; Xiao et al., 2011; Yue et al., 2013; Kong et al., 2020), which shed new light on the processes of the formation, maintenance, and dilution of air pollution as a result of comprehensive observations of air quality. Long-term operational observation is also of high significance in showing how air quality evolves as a result of anthropogenic and natural processes, especially recording potential changes in air quality as a result of the implementation of air pollution control measures. Strict implementation of the “Air Pollution Prevention and Control Action Plan” took effect in China in 2013, which provided us a good opportunity to study how PM concentration was impacted by this huge change in anthropogenic emissions. PM2.5 concentration in the PRD region was observed to gradually decrease with an average reduction rate of 21% from 2013 to 2017 (Zhang et al., 2019; Lu et al., 2021). The objective of this study is to present a closer look at aerosol evolution during 2011–2018. In order to fulfill this goal, we used three aerosol datasets, i.e., aerosol optical depth (AOD), PM concentration, and visibility at a background site in the PRD. A detailed analysis of variation of AOD, PM, and visibility was presented. Furthermore, we also investigated the influence of meteorological factors and estimated the relationships of AOD, PM, and visibility. The results are necessary for the understanding of the baseline of aerosol properties in PRD and will provide the scientific basis for the adoption of pollution prevention and control measures.
Site, Data, and Method
Site
Shenzhen is a rising urban metropolis in the PRD region, with a total area of 1997.47 km2 and a population of 17.56 million by the end of 2020. It lies along the coast of the South China Sea and is located immediately to the north of Hong Kong. Shenzhen has been experiencing rapid economic development and population growth that probably results in complicated air pollution problems. The sampling site in the study is located at the Shenzhen Xichong Astronomical Observatory (114.56°E, 22.49°N), at the top of a seaside hill with an elevation of 155 m above sea level (ASL). The Xichong observatory is far away from the urban and industrial regions of the PRD, therefore, representing the background level of air quality in the PRD (Figure 1).
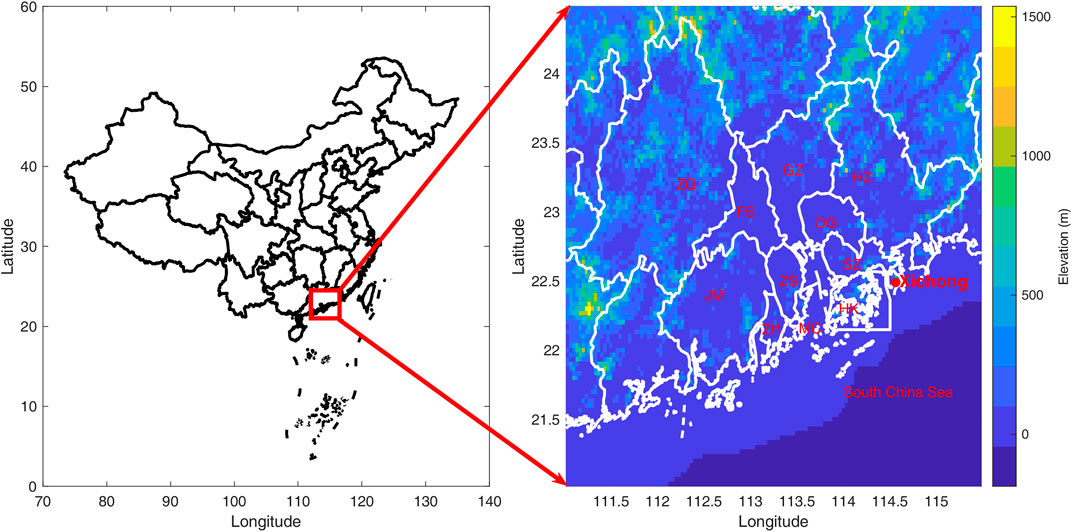
FIGURE 1. Topography map of the Pearl River Delta region with 11 cities [Zhaoqing (ZQ), Guangzhou (GZ), Huizhou (HZ), Foshan (FS), Dongguan (DG), Jiangmen (JM), Zhongshan (ZS), Shenzhen (SZ), Hong Kong (HK), Zhuhai (ZH), and MC (Macau)] and the location of the Xichong site.
Observation Data
Aerosol optical depth data are derived from the direct and diffuse solar spectral radiance measured by a CIMEL CE318 sun photometer. The sun photometer measures direct sun and diffuse sky radiances in 15 and 30 min intervals between the spectral ranges of 340–1020 nm, respectively, with a 1.2° field of view (Holben et al., 1998). The AOD products are automatically cloud cleared and quality assured, and have a low uncertainty of 0.01–0.02 in the visible and near-infrared wavelengths (Dubovik et al., 2000). The AOD at 550 nm used in the study was computed using the quadratic fit of AOD to wavelength on a log-log scale. Hourly PM mass concentration is observed by the beta-ray method. An automatic meteorological station is equipped with a set of sensors measuring 1-min visibility (km), temperature (T, °C), relative humidity (RH, %), wind speed (WS, m·s−1), and precipitation (PR, mm). To eliminate the contamination of precipitation, data obtained under rainy conditions (PR > 0) are removed.
European Centre for Medium-Range Weather Forecasts Atmospheric Reanalysis Data
The boundary layer heights (BLH) and wind vectors at pressure levels from European Centre for Medium-Range Weather Forecasts (ECMWF) reanalysis data (ERA5) are used to analyze the impacts of BLH and wind on the suspended particulate matter and the horizontal visibility. ERA5 is the fifth generation ECMWF atmospheric reanalysis of the global climate, covering the period from January 1950 to the present (https://cds.climate.copernicus.eu/). The data resolve the atmosphere using 137 levels from the surface up to a height of 80 km and has been regreded to a regular grid of 0.25 degrees. The u- and v-components of wind are the eastward and northward components at pressure levels, which indicate the horizontal speed of air moving toward the east and north, respectively. The wind speed (s) in meters per second is calculated using s =
Results and Discussions
Temporal Variability of Aerosol Optical Depth, PM, and Visibility
The temporal variations in daily PM mass concentrations are presented in Figure 2A. Daily averaged PM10 (PM2.5, PM1) in Figure 2A varies from 1.14 (1.06, 0.22) to 138 (90.8, 80.02) μg/m3. According to the Chinese ambient air quality standards (GB 3095-2012), the first-level (second-level) limits of daily PM10 and PM2.5 are 50 μg/m3 (150 μg/m3) and 35 μg/m3 (75 μg/m3), respectively. It is shown that almost all the daily PM10 and PM2.5 at Xichong are below the second-level limit but the days over the first-level limit of PM10 and PM2.5 account for about 8.7% (228 days) and 15.2% (399 days) of the entire study period (2641 days). It is remarkable that a significant reduction in the daily PM mass concentrations occurs after Apr. 2015 at Xichong. Since then, the number of days over the first-level limit of PM10 and PM2.5 is 22, and 60, respectively, mostly occurring during the winter and spring months.
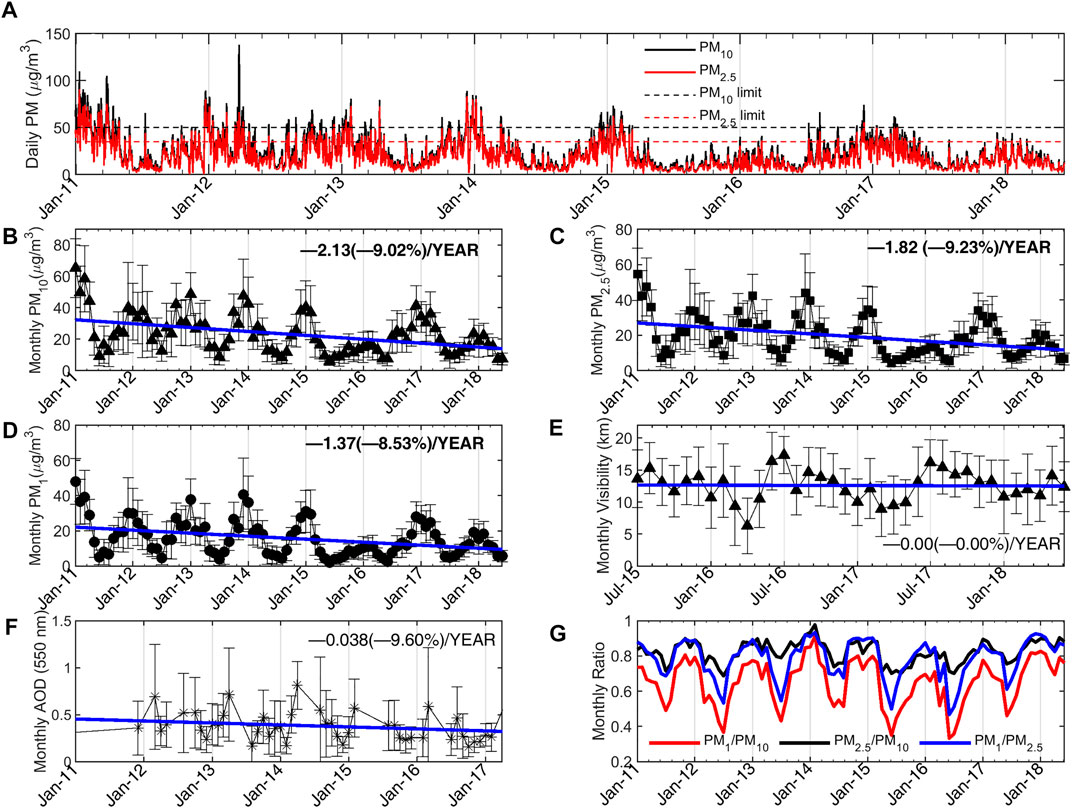
FIGURE 2. Time series of daily PM10 and PM2.5 mass concentrations from Jan. 2011 to June 2018 (A), variability of monthly PM concentrations (B–D), visibility (E), AOD (F), and ratios of PM (G) at Xichong site. The statistical parameters in the figures are linear trends of monthly PM, visibility and AOD. Bold font indicates trends are statistically significant at the 95% confidence level.
Figures 2B–G depict the variability of monthly PM mass concentrations, AOD, visibility, and the ratio of PM1, PM2.5, and PM10. Annual means of PM concentrations reached the minimum in 2016, and the values of PM10, PM2.5, and PM1 are 16.5 ± 11.0 μg/m3, 13.3 ± 10.1 μg/m3, and 10.8 ± 9.7 μg/m3, respectively. According to the observational data during 2015–2017 in the PRD, the minima of yearly mean PM2.5 (<28 μg/m3), PM10 (<46 μg/m3), and AQI (<56) were also found in Shenzhen in 2016 (Fang et al., 2019). The mean AOD in 2016 is 0.30 ± 0.14, lower than in other years possibly because of the following reasons: First, the observation data is not available in April when the highest aerosol loading of the year generally occurs (Figure 3A). Second, the higher wind speed (>3.5 m/s) at 850 hPa (Supplementary Figure S2B) lasted throughout the spring and summer except August and September, which are favorable for the dispersion of aerosols and result in a lower AOD. Finally, the precipitation amount (>200 mm) during the wet season except July is larger than that in other years (Supplementary Figure S2A), which implies a stronger wet removal of aerosols.
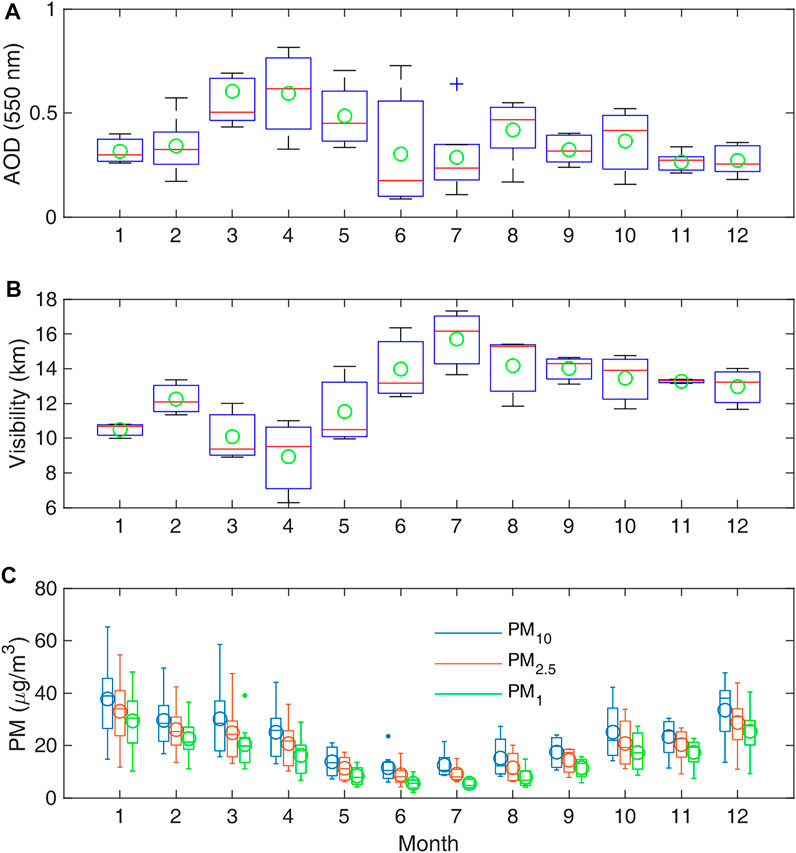
FIGURE 3. Boxplot of monthly averaged AOD (A) from Jan. 2011 to Jun. 2017, visibility (B) from Jul. 2015 to Jun. 2018, and PM mass concentrations (C) from Jan. 2011 to Jun. 2018 at Xichong. The ends of the boxes, the ends of the whiskers, and the short line across each box represent the 25th and 75th percentiles, the 5th and 95th percentiles, and the median, respectively. The means are represented by the circles.
The linear trends in PM mass concentrations, visibility, and AOD during the study period are also given in Figures 2B–F. Negative trends in PM10, PM2.5, and PM1 are significant at a 95% confidence level with the magnitudes of −2.13, −1.82, and −1.37 yr−1, respectively. Moreover, a downward trend of −0.038 yr−1 in AOD is also found in Figure 2F although it is statistically insignificant. The decrease in aerosol loadings at Xichong is attributed to the strict environmental regulations for improving air quality (http://www.mee.gov.cn/ywgz/fgbz/fl/201404/t20140425_271040.shtml), including the regulations and measures of emission reduction in power plants, combustion facilities, vehicles, ports, and ships (http://www.sz.gov.cn/zfgb/2013/gb852/201309/t20130929_2217840.htm). The relatively short-term observations of visibility might not be enough to handle the interannual variation. The trend in visibility cannot be derived from the study.
Table1 listed the means of PM mass concentrations at different sites in the PRD during the same period. The data of PM mass concentration are available from Dec.2013 to Jun. 2018 (https://www.aqistudy.cn/historydata). The multi-year averaged mass concentrations of PM10, PM2.5, and PM1 at Xichong during the study period are 23.7 ± 12.6 μg/m3, 19.7 ± 11.0 μg/m3, and 16.1 ± 10.1 μg/m3, respectively. The averaged mass concentrations of PM10 and PM2.5 observed in 19 ground-based monitoring sites around Shenzhen during Mar. 2013–Feb. 2014 were 85 and 43 μg/m3, respectively (Xia et al., 2017). The averaged mass concentrations of PM10 and PM2.5 during the same period at Xichong were 26.7 ± 13.0 μg/m3 and 23.2 ± 11.9 μg/m3, which indicate that the polluting levels of particulate matters at Xichong were much lower than those of urban sites in Shenzhen. The PM mass concentrations at Xichong are the lowest in all the sites in the PRD region (Table 1), which suggested that the air masses are relatively clean because the Xichong site is far away from the urban and industrial emission.
The averaged PM1/PM10, PM1/PM2.5, and PM2.5/PM10 ratios from Jan. 2011 to Jun. 2018 are 0.65, 0.78, and 0.82, respectively. The extents of variation in the monthly mean of PM1/PM10, PM1/PM2.5, and PM2.5/PM10 ratios are 0.33–0.91, 0.47–0.93, and 0.69–0.98. The mean ratio of PM2.5 to PM10 at the Xichong site is the highest in all the observations in the PRD (Table 1). In addition to primary emission sources, the ratio of PM10 and PM2.5 can be significantly affected by secondary aerosol formation in the atmosphere (Kong et al., 2017; Munir, 2017; Fan et al., 2021). Secondary aerosols contribute significantly to PM mass concentrations, especially PM2.5 concentrations (Zhao et al., 2018; Fan et al., 2020; Spandana et al., 2021). The high ratios of PM2.5 to PM10 suggest that aerosol pollution at Xichong is mainly caused by fine particles more from anthropogenic sources (Chu et al., 2015; Kong and Yi, 2015). The site is relatively far away from the downtown of Shenzhen, but the air quality at Xichong is still affected by human activities. The PM1/PM10 and PM1/PM2.5 ratios exhibit a remarkable reduction in summer because the hygroscopic growth of a large fraction of submicrometer particles under higher RH may increase the proportion of PM2.5 (Zhao et al., 2018; Wang et al., 2020; Fan et al., 2021).
All the PM ratios in Figure 2G have distinct seasonal characteristics, with lower values in the summer but higher values during the autumn and winter months. The mean ratios of PM1 to PM2.5 are greater than 0.83 during the autumn and winter months, which means the mass of PM2.5 is dominated by particles with a diameter of <1.0 μm. The PM1/PM10, PM1/PM2.5, and PM2.5/PM10 ratios exhibit a remarkable reduction in summer with the seasonal means of 0.52, 0.67, and 0.76 due to the hygroscopic growth of a large fraction of submicrometer particles under higher RH.
Figure 3 exhibits the statistical distribution of AOD 1) from Jan. 2011 to Jun. 2017, visibility 2) from Jul. 2015 to Jun. 2018, and PM mass concentrations 3) from Jan. 2011 to Jun. 2018 at Xichong. AOD reached its maximum during the spring months with the mean values of 0.61, 0.59, and 0.49 in March, April, and May, respectively. The monthly mean visibility varies from 6.3 ± 4.3 to 17.3 ± 2.9 km. The mean (±σ) of visibility during the study period is 12.6 ± 2.3 km at Xichong. The PM concentrations were higher in the winter and spring months but lower during the summer and autumn months. The peak values of PM mass concentrations were observed in January when the monthly mean of PM10, PM2.5, and PM1.0 was 37.8, 33.0, and 29.4 μg/m3, respectively. The PM concentrations reached the lowest values in June when the monthly mean of PM10, PM2.5, and PM1.0 was 11.6, 8.8, and 5.6 μg/m3, respectively.
The seasonality of the visibility is inversed to those of PM mass concentrations and AOD. The visibility was higher during the summer and autumn months and lower during the winter and spring months. The highest AODs in spring correspond to the lowest visibility in March and April with the monthly means of 10.1 and 8.9 km. The highest visibility appeared in July with a monthly mean value of 15.7 km, which corresponds to the lowest AOD of 0.29 and the lowest PM1 and PM2.5 concentrations.
Diurnal Variation of PM, Visibility, RH, and Boundary Layer Heights
The height of the boundary layer (BLH) determines the volume available for pollution dispersion and transport in the atmosphere (Yang et al., 2012). Figure 4 shows the diurnal variations in multi-year averaged visibility, PM10, PM2.5, PM1, RH, and BLH for four seasons at Xichong. The visibility, relative humidity, and BLH show significant diurnal cycles. The visibility reaches the maximum value (13.6 km) at noon and then decreases from 14:00 LT and drops to the minimum (11.8 km) during the night. Relative humidity shows an opposite diurnal cycle to that of visibility, with a higher RH (∼86%) during the night and a lower RH at noon (76%). Most atmospheric aerosols are externally mixed with respect to hygroscopicity and consist of more and less hygroscopic sub-fractions (Cheng et al., 2008; Cubison et al., 2008; Swietlicki et al., 2008; Meier et al., 2009; Yan et al., 2009). Water, absorbed by hygroscopic or deliquescent aerosols, makes a substantial contribution to visibility reduction (Swietlicki et al., 2008; Chen et al., 2014; Li et al., 2017). Therefore, in addition to the chemical composition of the particulate matter, the ambient RH also has a substantial impact on visibility (Deng et al., 2008a; Yang et al., 2015; Wang X. et al., 2019).
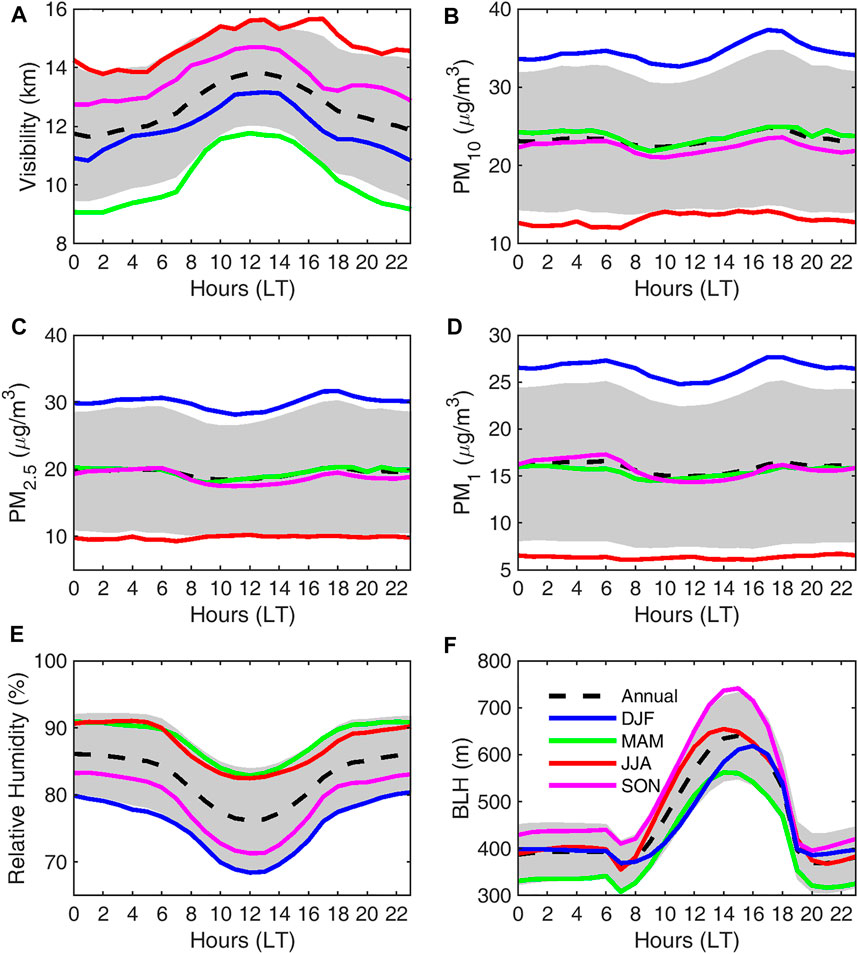
FIGURE 4. The diurnal variations in multi-year averaged visibility (A), PM10 (B), PM 2.5 (C), PM1 (D), RH (E), and BLH (F) for four seasons at Xichong.
The diurnal variations of PM concentrations show a two-peak pattern. The first peak occurs at 6:00 LT, and the mean values of PM10, PM2.5, and PM1 are 23.4, 20.1, and 16.6 μg/m3, respectively; the second peak occurs at 18:00 LT, and the mean values of PM10, PM2.5, and PM1 are 24.9, 20.3, and 16.5 μg/m3. The diurnal variation of PM pollutants can be controlled by many factors, including emission, chemical reactions, and meteorological conditions (Sun et al., 2015; Tao et al., 2015; Du et al., 2020). Zhang and Cao (2015) used a long-term dataset of surface PM2.5 concentrations measured at 190 cities of China and found that the diurnal variation of the PM2.5-to-CO ratio (an excellent tracer for excluding the influence of primary combustion emissions) consistently displayed a pronounced peak during the afternoon (about 16:00 LT). This indicates that the secondary formation process plays an important role in PM concentrations, especially in the afternoon when the photochemical activities are relatively strong (Huang et al., 2011; Zhou et al., 2014; Du et al., 2020). A more detailed explanation of the diurnal variation of PM concentrations is subject to further studies including more comprehensive observations of the chemical of PM and its precursors. The diurnal variations of PM2.5 and PM1 concentrations are flattened in summer compared to those of other seasons.
In addition to pollutant emissions and topographic conditions, the spatial and temporal distribution of PM is mainly affected by meteorological conditions in the troposphere, especially in the atmospheric boundary layer (ABL) (Li et al., 2017; Song et al., 2017; Chen et al., 2018; Su et al., 2018; Wei et al., 2018). Pollutants within this layer are fully mixed and vertically dispersed due to convection or mechanical turbulence (Seibert et al., 2000). The BLH begins increasing after sunrise (7:00 LT) and reaches a peak with a value of 640 m at 14:00 LT and then decreases to 370 m at 20:00 LT. The BLH determines the volume available for pollution dispersion and transport in the atmosphere. Low BLH and weak turbulence strengthen the accumulation of air pollutants (Petaja et al., 2016; Miao et al., 2018). When the BLH is lower, higher concentrations of pollutants can accumulate, which result in lower visibility. So the diurnal cycles of the BLH exhibit similar patterns to those of visibility.
Relating Aerosol Optical Depth, Visibility, and PM Concentrations
The increase in PM concentration generally leads to reduced visibility (Deng et al., 2008a; Cheng et al., 2008; Li et al., 2017; Wang G. et al., 2019; Kong et al., 2020) and rising AOD (Xiao et al., 2016; Shahzad et al., 2013; 2018). However, the relationship between PM concentration and visibility as well as AOD is complex and nonlinear because of the variability in the particle size distribution, mixing state, and chemical composition of aerosols (Wang X. et al., 2019). The relationship between the AOD and PM can be used to derive the PM concentrations from satellite observations of AOD (Li et al., 2005; Green et al., 2009; van Donkelaar et al., 2010). Figure 5A showed the scatter plots of hourly-averaged AOD vs. PM2.5. The correlation between AOD and PM is very low, with the coefficients of determination being only 0.11. AOD is the column-integrated aerosol extinction coefficient, while PM observations represent near-surface PM concentration. The relationship between AOD and PM concentrations is, therefore, influenced by the relative humidity, the BLH, and the vertical distribution of aerosol (Dehghan et al., 2017; Shahzad et al., 2018; Filonchyk et al., 2019). The vertical and humidity corrections should be carefully considered in order to better characterize the relationship between AOD and PM. Following the previous studies (Wang et al., 2010; Zheng et al., 2013; Zheng et al., 2017), the column-integrated PM concentrations are first corrected by the BLH as follows:
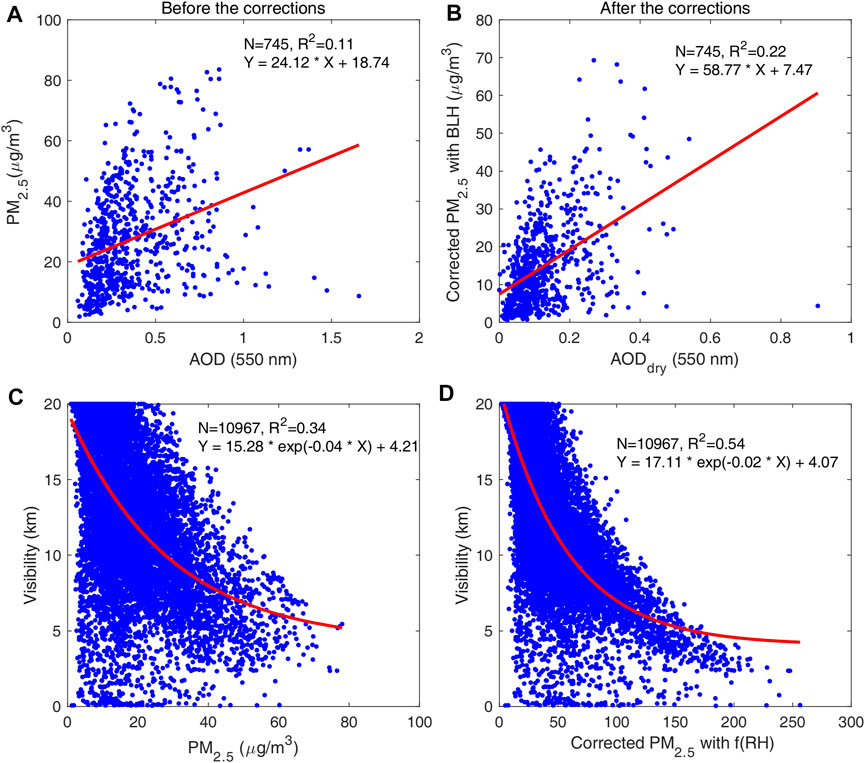
FIGURE 5. Scatter plots of AOD vs. PM2.5 (A,B) and PM2.5 vs. visibility (C,D) at Xichong. The left panel is before the corrections of RH and BLH; the right panel is after the corrections of RH and BLH.
In addition, PM concentrations are measured under a dry condition with the RH <35%. But the hygroscopic growth of particles significantly affects AOD. The effect of relative humidity on AOD has to be considered in order to get a more reliable AOD and PM relationship. Hygroscopic growth factor, f (RH), is one of the most used parameters in describing variations of the aerosol sizes at different ambient RHs (Kotchenruther et al., 1999; Zheng et al., 2013). So, a dehydration adjustment proposed by Zheng et al. (2017) is applied to get the dry condition AOD as follows:
f (RH) can be expressed as follows:
where a and b are empirical coefficients determined by the aerosol types. The empirical coefficients are based on the previous studies of hygroscopic growing factors in the PRD region (Liu et al., 2008). In the atmosphere, the RH often increases with height within PBL (Zheng et al., 2017; Zhou et al., 2020). This could definitely affect the dehydration adjustment of AOD in Eqs 2, 3. Currently, the surface RH is used to do the adjustment, and the vertical variation in RH has not been considered, which could cause the dry condition AOD to be somehow overestimated compared to its true value.
Figure 5B exhibited the linear regression between hourly-averaged AOD and PM2.5 mass concentrations with the vertical and humidity corrections. There is a moderate correlation with an R value of 0.47 between PM2.5 and AOD after these corrections, with the coefficients of determination ∼0.22 at a 95% confidence level. The RMSE decreased from 14.7 (without corrections) to 8.8 (with correction). There are several factors resulting in the weak correlation between PM and AOD at Xichong. The aerosol loading is relatively low in the background site, and the variation ranges of PM and AOD are narrower compared to those in the urban sites. Generally, higher correlation coefficients were observed at urban sites than at suburban sites (Zheng et al., 2013). In addition, the AODdry is adjusted based on surface RH, and the vertical variation in RH has not been considered. The AODdry obtained here could somehow deviate from its true value (Zheng et al., 2017). After the vertical and humidity corrections, the correlative coefficients R between PM10, PM1, and AOD are 0.44, and 0.46 at a 95% confidence level, respectively.
PM mass concentrations are also corrected using the hygroscopic growth factor in order to consider the effect of humidity on the PM mass concentrations. Figures 5C, D showed the scatter plots of PM2.5 vs. visibility before and after humidity correction. There is a significant anti-correlation between PM and visibility because the increase of surface PM concentrations impairs the horizontal visibility. There are significant improvements in the correlations between PM and visibility after humidity corrections. The data points are more compact with the determination coefficient R2 increasing from 0.34 (without correction) to 0.54 (with correction). The relationship between PM10, PM1, and visibility is similar to that of PM2.5 and visibility.
It is known that relative humidity is the most influencing factor on the visual impairment in the context of high aerosol mass loading (Chen et al., 2014). The formation of secondary aerosol species could be enhanced under high-humidity conditions (Yu et al., 2005; Hennigan et al., 2008). Additionally, fine hydrophilic aerosols could increase to a larger size by taking ambient water vapor, resulting in a higher extent of light extinction and visibility deterioration (Xiao et al., 2011; Liu et al., 2012). Figure 6 shows the variation of visibility with relative humidity and the PM mass concentrations. RH was classified into three ranges: RH ≥90%, 90% > RH ≥ 80%, and RH <80%. It can be seen that the visibility decreases significantly with increasing RH. Moreover, the visibility decreases in a nonlinear tendency with the increase of PM mass concentrations. The highest correlation between visibility and PM concentrations is observed in the RH range of 80–90%, with the coefficients of determination being 0.49, 0.54, and 0.60 for visibility vs. PM10, PM2.5, and PM1, respectively. Under excessively high RH (>90%), the variation of visibility becomes not significant when the mass concentrations of PM10, PM2.5, and PM1 are greater than 40, 30, and 20 μg/m3.
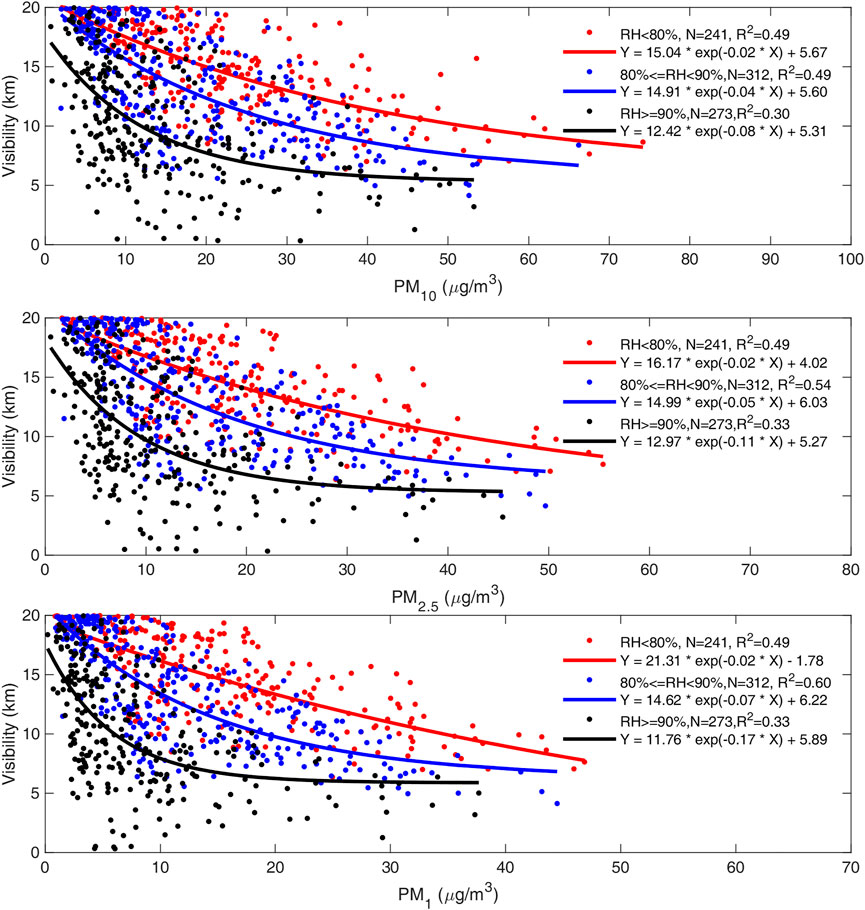
FIGURE 6. The exponential function fitting between visibility and PM mass concentrations in different RH ranges at Xichong.
The relationship between AOD and visibility is illustrated in Figure 7. There is a clear anti-correlation between AOD and visibility, although the coefficient of determination of exponential fitting was only 0.17. The higher aerosol loading in the atmosphere results in increased extinction of light and hence decreases visibility. In addition to the aerosol loading, the effect of meteorological conditions on visibility is also very important (Wu et al., 2007; Deng et al., 2008b). The effects of humidity and BLH on visibility are significant as previous discussion. We also investigated the effects of ambient temperature and surface pressure on visibility, AOD, and PM mass concentrations. Visibility, AOD, and PM mass concentrations show strong independence on ambient temperature and surface pressure with R2 less than 0.03 at a 95% confidence level.
Effect of Wind on Aerosol Optical Depth, PM, and Visibility
Wind direction and speed have an important effect on the transport of air pollutants (Yang et al., 2017; Meng et al., 2019; Liu et al., 2020). The influence of surface wind speed and direction on AOD, PM, and visibility is investigated in the section. Figure 8 shows the relationships of surface wind speed, direction, and daily visibility (a), AOD (b), PM10 (c), and PM2.5 (d). At Xichong, low visibility and high aerosol loading tend to appear at a low wind speed. About 80% of the low visibility (VIS <5 km), high PM mass concentrations (PM10 > 50 μg/m3, PM2.5 > 50 μg/m3) and 100% of high aerosol loading (AOD>1.0) occur on days when the wind speed is less than 5 m/s. The dominant wind direction is concentrated at easterly and southerly wind on low-visibility and high-AOD days.
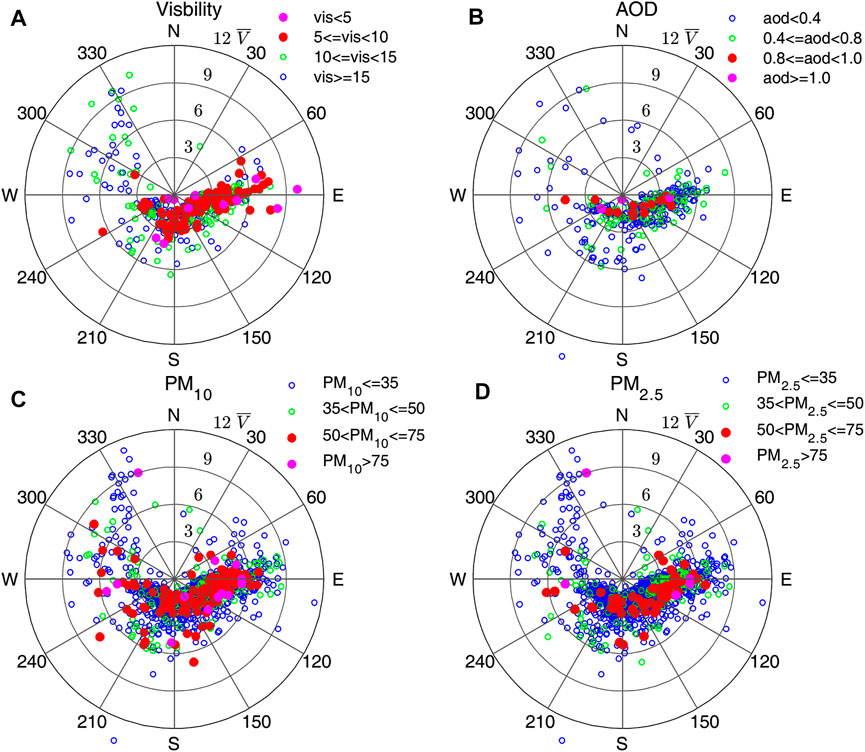
FIGURE 8. Wind rose as scatter plots with different ranges of daily visibility (A), AOD (B), PM10 (C), and PM2.5 (D) at Xichong.
Backward trajectory analysis is an effective method to clarify the transport of air parcels in the atmosphere (Cheng et al., 2015). We calculated and clustered backward trajectories of air mass using the Hybrid Single-Particle Lagrangian Trajectory (HYSPLIT) model developed by the Air Resources Laboratory of the National Oceanic and Atmospheric Administration (NOAA), United States (Stein et al., 2015). Figure 9 shows the clustered 72-h backward trajectories ending at 1500 m, 1000 m, and 500 m in January (a–c), April (d–e), July (f–h), and October (i–l) calculated by the HYSPLIT model. The airflows from the west and northwest directions occupy about 80% at Xichong in winter. The airflows from the south directions increase to over 50% during the spring and summer months. The airflows from the southwest increase significantly in spring and summer, especially at higher heights (1000 m and 1500 m), which verify the effects of air pollutants transported from Southeastern Asia on the air quality at Xichong. The air mass mainly from northeast directions in autumn, especially at lower heights (500 m), contributes to the degradation of visibility and enhancement of PM mass concentrations at Xichong.
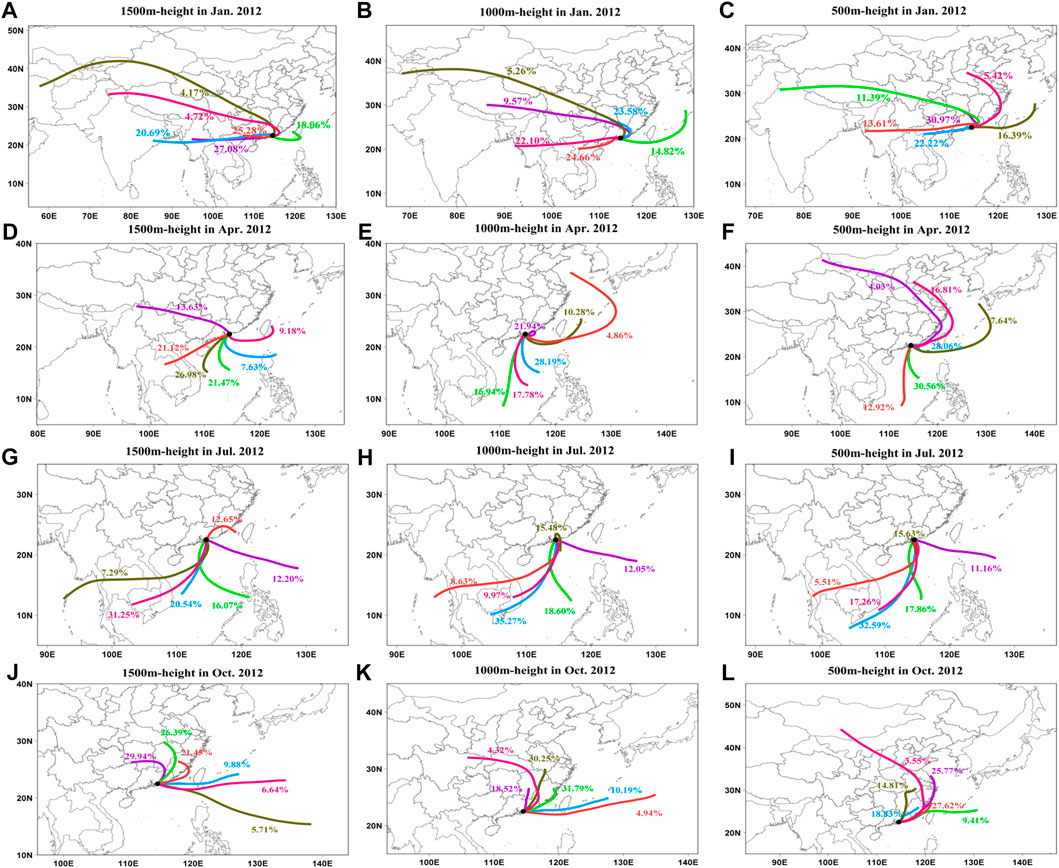
FIGURE 9. Clustered 72-h backward trajectories of air mass ending at 1500 m (left panel), 1000 m (middle panel), and 500 m (right panel) in January (A–C), April (D–F), July (G–I), and October (J–L) 2012, calculated by the Hybrid Single-Particle Lagrangian Trajectory (HYSPLIT) model.
Conclusion
Based on the observations of AOD, visibility, and PM mass concentrations during the period of 2011–2018 at Xichong, a long-term analysis of the parameters relative to the air quality is performed. The mean (±σ) of visibility and AOD during the study period is 12.6 ± 2.3 km and 0.38 ± 0.07, respectively. The multi-year averaged mass concentrations of PM10, PM2.5, and PM1 during the study period are 23.7 ± 12.6 μg/m3, 19.7 ± 11.0 μg/m3, and 16.1 ± 10.1 μg/m3, respectively. The daily PM10 and PM2.5 at Xichong are lower than those in other sites in the PRD because the air masses are relatively cleaner; almost all the daily PM concentrations are below the second-level limit of Chinese ambient air quality standards (GB 3095-2012), which suggested that they may represent the air quality of the background level in the PRD region. Negative trends in PM10, PM2.5, and PM1 are significant at a 95% confidence level with the magnitudes of −2.13, −1.82, and −1.37 yr−1, respectively. The decrease in aerosol loadings at Xichong is attributed to the strict environmental regulations for improving air quality.
AOD reached its maximum during the spring months with the mean values of 0.61, 0.59, and 0.49 in March, April, and May, respectively. The peak and the lowest values of PM mass concentrations were observed in January and June, respectively. The averaged PM1/PM10, PM2.5/PM10, and PM1/PM2.5 ratios during the study period are 0.65, 0.78, and 0.82, respectively. The ratio of PM10 and PM2.5 can be significantly affected by secondary aerosol formation in the atmosphere (Kong et al., 2017; Munir, 2017; Fan et al., 2021). Secondary aerosols contribute significantly to PM mass concentrations, especially PM2.5 concentrations (Zhao et al., 2018; Fan et al., 2020; Spandana et al., 2021). The high ratios of PM2.5 to PM10 suggest that aerosol pollution at Xichong is mainly caused by fine particles more from anthropogenic sources (Chu et al., 2015; Kong and Yi, 2015). The PM1/PM10 and PM1/PM2.5 ratios exhibit a remarkable reduction in summer because the hygroscopic growth of a large fraction of submicrometer particles under higher RH may increase the proportion of PM2.5 (Zhao et al., 2018; Wang et al., 2020; Fan et al., 2021). The seasonality of the visibility is inversed to those of PM mass concentrations and AOD at Xichong. The visibility was higher during the summer and autumn months and lower during the winter and spring months.
The visibility and PM concentrations show significant diurnal cycles. The visibility reaches its maximum values at noon and then drops to its minimum during the night, which is similar to the diurnal variation of BLH. The diurnal variations of PM concentrations show a two-peak pattern possibly caused by the more active photochemistry process due to the increasing solar radiation during the daytime. A more detailed explanation of the diurnal variation of PM concentrations is subject to further studies including more comprehensive observations of the chemical of PM and its precursors.
AOD, visibility, and PM mass concentrations are physically related. There is a clear anti-correlation between AOD and visibility at the Xichong site, although the coefficient of determination of exponential fitting was only 0.17. The highest correlation between visibility and PM concentrations is observed in the RH range of 80–90%, with the coefficients of determination being 0.49, 0.54, and 0.60 for visibility vs. PM10, PM2.5, and PM1, respectively. Under excessively high RH (>90%), the variation of visibility becomes insignificant when the mass concentrations of PM10, PM2.5, and PM1 are greater than 40, 30, and 20 μg/m3.
At Xichong, low visibility and high aerosol loading tend to appear with a low wind speed. About 80% of the low visibility (VIS <5 km), high PM mass concentrations (PM10 > 50, PM2.5 > 50) and 100% of high aerosol loading (AOD >1.0) occur on days when the wind speed is less than 5 m/s. The dominant wind direction is concentrated at easterly and southerly wind in low-visibility and high-AOD days. Understanding of variability of surface particle concentration and column-integrated aerosol loading at multiple temporal scales at this background site in the PRD region would provide a scientific basis for the adoption of pollution prevention and control measures.
Data Availability Statement
The original contributions presented in the study are included in the article/Supplementary Material, further inquiries can be directed to the corresponding author.
Author Contributions
XF, XX, and HC contributed to the conceptualization. XF contributed to methodology, formal analysis, and writing—original draft preparation. XX and HC contributed to reviewing and editing. YZ contributed to software. JL contributed to validation. HY and HL contributed to data curation. All authors have read and agreed to the published version of the manuscript.
Funding
This research was supported by the National Natural Science Foundation of China granting (42030708, 41775033), the Strategic Priority Research Program of the Chinese Academy of Sciences (XDA17040511), and the Opening Foundation of Key Laboratory of Atmosphere Sounding, China Meteorological Administration and CMA Research Centre on Meteorological Observation Engineering Technology (U2021Z03).
Conflict of Interest
The authors declare that the research was conducted in the absence of any commercial or financial relationships that could be construed as a potential conflict of interest.
Publisher’s Note
All claims expressed in this article are solely those of the authors and do not necessarily represent those of their affiliated organizations, or those of the publisher, the editors, and the reviewers. Any product that may be evaluated in this article, or claim that may be made by its manufacturer, is not guaranteed or endorsed by the publisher.
Acknowledgments
We appreciate the open-access data released by European Centre for Medium-Range Weather Forecasts. We also thank Yaqiang Wang for developing the GIS software for meteorological data (MeteoinfoMap, http://www.meteothink.org).
Supplementary Material
The Supplementary Material for this article can be found online at: https://www.frontiersin.org/articles/10.3389/fenvs.2022.893408/full#supplementary-material
References
Ansmann, A., Engelmann, R., Althausen, D., Wandinger, U., Hu, M., Zhang, Y. H., et al. (2005). High Aerosol Load Over the Pearl River Delta, China, Observed with Raman Lidar and Sun Photometer. Geophys. Res. Lett. 32 (13), L13815. doi:10.1029/2005gl023094
Cao, J., Lee, S. C., Ho, K. F., Zhang, X. Y., Zou, S. C., Fung, K., et al. (2003). Characteristics of Carbonaceous Aerosol in Pearl River Delta Region, China during 2001 Winter Period. Atmos. Environ. 37 (11), 1451–1460. doi:10.1016/s1352-2310(02)01002-6
Chen, J., Qiu, S., Shang, J., Wilfrid, O. M. F., Liu, X., Tian, H., et al. (2014). Impact of Relative Humidity and Water Soluble Constituents of PM2.5 on Visibility Impairment in Beijing, China. Aerosol Air Qual. Res. 14 (1), 260–268. doi:10.4209/aaqr.2012.12.0360
Chen, Y., An, J., Sun, Y., Wang, X., Qu, Y., Zhang, J., et al. (2018). Nocturnal Low-Level Winds and Their Impacts on Particulate Matter over the Beijing Area. Adv. Atmos. Sci. 35 (12), 1455–1468. doi:10.1007/s00376-018-8022-9
Cheng, Y. F., Wiedensohler, A., Eichler, H., Heintzenberg, J., Tesche, M., Ansmann, A., et al. (2008). Relative Humidity Dependence of Aerosol Optical Properties and Direct Radiative Forcing in the Surface Boundary Layer at Xinken in Pearl River Delta of China: An Observation Based Numerical Study. Atmos. Environ. 42 (25), 6373–6397. doi:10.1016/j.atmosenv.2008.04.009
Cheng, T., Xu, C., Duan, J., Wang, Y., Leng, C., Tao, J., et al. (2015). Seasonal Cariation and Difference of Aerosol Optical Properties in Columnar and Surface Atmospheres Over Shanghai. Atmos. Environ. 123, 315–326. doi:10.1016/j.atmosenv.2015.05.029
Chu, B., Liu, T., Zhang, X., Liu, Y., Ma, Q., Ma, J., et al. (2015). Secondary Aerosol Formation and Oxidation Capacity in Photooxidation in the Presence of Al2O3 Seed Particles and SO2. Sci. China Chem. 58 (9), 1426–1434. doi:10.1007/s11426-015-5456-0
Cubison, M. J., Ervens, B., Feingold, G., Docherty, K. S., Ulbrich, I. M., Shields, L., et al. (2008). The Influence of Chemical Composition and Mixing State of Los Angeles Urban Aerosol on CCN Number and Cloud Properties. Atmos. Chem. Phys 8 (18), 5649–5667. doi:10.5194/acp-8-5649-2008
Dehghan, M., Omidvar, K., Mozafari, K., and Mazidi, A. (2017). Estimation of Relationship Between Aerosol Optical Depth, PM10 and Visibility in Separation of Synoptic Codes, as Important Parameters in Researches Connected to Aerosols; Using Genetic Algorithm in Yazd. Int. J. Environ. Sci. Nat. Res. 7 (4). doi:10.19080/IJESNR.2017.07.5557
Deng, X., Tie, X., Wu, D., Zhou, X., Bi, X., Tan, H., et al. (2008a). Long-term Trend of Visibility and its Characterizations in the Pearl River Delta (PRD) Region, China. Atmos. Environ. 42 (7), 1424–1435. doi:10.1016/j.atmosenv.2007.11.025
Deng, X., Tie, X., Zhou, X., Wu, D., Zhong, L., Tan, H., et al. (2008b). Effects of Southeast Asia Biomass Burning on Aerosols and Ozone Concentrations over the Pearl River Delta (PRD) Region. Atmos. Environ. 42 (36), 8493–8501. doi:10.1016/j.atmosenv.2008.08.013
Du, Q., Zhao, C., Zhang, M., Dong, X., Chen, Y., Liu, Z., et al. (2020). Modeling Diurnal Variation of Surface PM<sub>2.5</sub> Concentrations over East China with WRF-Chem: Impacts from Boundary-Layer Mixing and Anthropogenic Emission. Atmos. Chem. Phys. 20 (5), 2839–2863. doi:10.5194/acp-20-2839-2020
Dubovik, O., Smirnov, A., Holben, B. N., King, M. D., Kaufman, Y. J., Eck, T. F., et al. (2000). Accuracy Assessments of Aerosol Optical Properties Retrieved from Aerosol Robotic Network (AERONET) Sun and Sky Radiance Measurements. J. Geophys. Res. 105 (D8), 9791–9806. doi:10.1029/2000jd900040
Fan, H., Zhao, C. F., and Yang, Y. K. (2020). A Comprehensive Analysis of the Spatio-Temporal Variation of Urban Air Pollution in China during 2014-2018. Atmos. Environ. 220, 117066. doi:10.1016/j.atmosenv.2019.117066
Fan, H., Zhao, C. F., Yang, Y. K., and Yang, X. C. (2021). Spatio-Temporal Variations of the PM2.5/PM10 Ratios and its Application to Air Pollution Type Classification in China. Front. Environ. Sci. 9. doi:10.3389/fenvs.2021.692440
Fang, X., Fan, Q., Liao, Z., Xie, J., Xu, X., and Fan, S. (2019). Spatial-temporal Characteristics of the Air Quality in the Guangdong−Hong Kong−Macau Greater Bay Area of China during 2015-2017. Atmos. Environ. 210, 14–34. doi:10.1016/j.atmosenv.2019.04.037
Filonchyk, M., Yan, H. W., Zhang, Z. R., Yang, S. W., Li, W., and Li, Y. M. (2019). Combined Use of Satellite and Surface Observations to Study Aerosol Optical Depth in Different Regions of China. Sci. Rep. 9, 6174. doi:10.1038/s41598-019-42466-6
Green, M., Kondragunta, S., Ciren, P., and Xu, C. (2009). Comparison of GOES and MODIS Aerosol Optical Depth (AOD) to Aerosol Robotic Network (AERONET) AOD and IMPROVE PM2.5Mass at Bondville, Illinois. J. Air & Waste Manag. Assoc. 59 (9), 1082–1091. doi:10.3155/1047-3289.59.9.1082
Hennigan, C. J., Bergin, M. H., Dibb, J. E., and Weber, R. J. (2008). Enhanced Secondary Organic Aerosol Formation Due to Water Uptake by Fine Particles. Geophys. Res. Lett. 35 (18). doi:10.1029/2008gl035046
Holben, B. N., Eck, T. F., Slutsker, I., Tanre, D., Buis, J. P., Setzer, A., et al. (1998). AERONET - A Dederated Instrument Network and Data Archive for Aerosol Characterization. Remote Sens. Environ. 66 (1), 1–16. doi:10.1016/S0034-4257(98)00031-5
Hu, M., Wu, Z., Slanina, J., Lin, P., Liu, S., and Zeng, L. (2008). Acidic Gases, Ammonia and Water-Soluble Ions in PM2.5 at a Coastal Site in the Pearl River Delta, China. Atmos. Environ. 42 (25), 6310–6320. doi:10.1016/j.atmosenv.2008.02.015
Huang, X.-F., He, L.-Y., Hu, M., Canagaratna, M. R., Kroll, J. H., Ng, N. L., et al. (2011). Characterization of Submicron Aerosols at a Rural Site in Pearl River Delta of China Using an Aerodyne High-Resolution Aerosol Mass Spectrometer. Atmos. Chem. Phys. 11 (5), 1865–1877. doi:10.5194/acp-11-1865-2011
Kong, L., Hu, M., Tan, Q., Feng, M., Qu, Y., An, J., et al. (2020). Aerosol Optical Properties under Different Pollution Levels in the Pearl River Delta (PRD) Region of China. J. Environ. Sci. 87, 49–59. doi:10.1016/j.jes.2019.02.019
Kong, L., Xin, J., Liu, Z., Zhang, K., Tang, G., Zhang, W., et al. (2017). The PM2.5 Threshold for Aerosol Extinction in the Beijing Megacity. Atmos. Environ. 167, 458–465. doi:10.1016/j.atmosenv.2017.08.047
Kong, W., and Yi, F. (2015). Convective Boundary Layer Evolution From Lidar Backscatter and its Relationship with Surface Aerosol Concentration at a Location of a Central China Megacity. J. Geophys. Res. Atmos. 120 (15), 7928–7940. doi:10.1002/2015jd023248
Kotchenruther, R. A., Hobbs, P. V., and Hegg, D. A. (1999). Humidification Factors for Atmospheric Aerosols off the Mid-Atlantic Coast of the United States. J. Geophys. Res. 104 (D2), 2239–2251. doi:10.1029/98jd01751
Lan, Z.-J., Chen, D.-L., Li, X., Huang, X.-F., He, L.-Y., Deng, Y.-G., et al. (2011). Modal Characteristics of Carbonaceous Aerosol Size Distribution in an Urban Atmosphere of South China. Atmos. Res. 100 (1), 51–60. doi:10.1016/j.atmosres.2010.12.022
Li, C., Lau, A. K.-H., Mao, J., and Chu, D. A. (2005). Retrieval, Validation, and Application of the 1-km Aerosol Optical Depth from MODIS Measurements Over Hong Kong. IEEE Trans. Geosci. Remote Sens. 43 (11), 2650–2658. doi:10.1109/tgrs.2005.856627
Li, Y., Huang, H. X. H., Griffith, S. M., Wu, C., Lau, A. K. H., and Yu, J. Z. (2017). Quantifying the Relationship between Visibility Degradation and PM2.5 Constituents at a Suburban Site in Hong Kong: Differentiating Contributions from Hydrophilic and Hydrophobic Organic Compounds. Sci. Total Environ. 575, 1571–1581. doi:10.1016/j.scitotenv.2016.10.082
Liu, X., Cheng, Y., Zhang, Y., Jung, J., Sugimoto, N., Chang, S.-Y., et al. (2008). Influences of Relative Humidity and Particle Chemical Composition on Aerosol Scattering Properties during the 2006 PRD Campaign. Atmos. Environ. 42 (7), 1525–1536. doi:10.1016/j.atmosenv.2007.10.077
Liu, X., Zhang, Y., Cheng, Y., Hu, M., and Han, T. (2012). Aerosol Hygroscopicity and its Impact on Atmospheric Visibility and Radiative Forcing in Guangzhou during the 2006 PRIDE-PRD Campaign. Atmos. Environ. 60, 59–67. doi:10.1016/j.atmosenv.2012.06.016
Liu, X., Zhang, Y., Wen, M., Wang, J., Jung, J., Chang, S.-y., et al. (2010). A Closure Study of Aerosol Hygroscopic Growth Factor during the 2006 Pearl River Delta Campaign. Adv. Atmos. Sci. 27 (4), 947–956. doi:10.1007/s00376-009-9150-z
Liu, Y., He, J., Lai, X., Zhang, C., Zhang, L., Gong, S., et al. (2020). Influence of Atmospheric Circulation on Aerosol and its Optical Characteristics in the Pearl River Delta Region. Atmos. 11 (3). doi:10.3390/atmos11030288
Lu, M., Zheng, J., Huang, Z., Wu, C., Zheng, C., Jia, G., et al. (2021). Insight into the Characteristics of Carbonaceous Aerosols at Urban and Regional Sites in the Downwind Area of Pearl River Delta Region, China. Sci. Total Environ. 778, 146251. doi:10.1016/j.scitotenv.2021.146251
Ma, Y., Lu, K., Chou, C. C.-K., Li, X., and Zhang, Y. (2017). Strong Deviations from the NO-NO2-O3 Photostationary State in the Pearl River Delta: Indications of Active Peroxy Radical and Chlorine Radical Chemistry. Atmos. Environ. 163, 22–34. doi:10.1016/j.atmosenv.2017.05.012
Meier, J., Wehner, B., Massling, A., Birmili, W., Nowak, A., Gnauk, T., et al. (2009). Hygroscopic Growth of Urban Aerosol Particles in Beijing (China) during Wintertime: a Comparison of Three Experimental Methods. Atmos. Chem. Phys. 9 (18), 6865–6880. doi:10.5194/acp-9-6865-2009
Meng, C., Cheng, T., Bao, F., Gu, X., Wang, J., Zuo, X., et al. (2020). The Impact of Meteorological Factors on Fine Particulate Pollution in Northeast China. Aerosol. Air Qual. Res. 20, 1618–1628. doi:10.4209/aaqr.2019.10.0534
Miao, Y., Liu, S., Guo, J., Huang, S., Yan, Y., and Lou, M. (2018). Unraveling the Relationships between Boundary Layer Height and PM2.5 Pollution in China Based on Four-Year Radiosonde Measurements. Environ. Pollut. 243, 1186–1195. doi:10.1016/j.envpol.2018.09.070
Munir, S. (2017). Analysing Temporal Trends in the Ratios of PM2.5/PM10 in the UK. Aerosol Air Qual. Res. 17 (1), 34–48. doi:10.4209/aaqr.2016.02.0081
Petäjä, T., Järvi, L., Kerminen, V. M., Ding, A. J., Sun, J. N., Nie, W., et al. (2016). Enhanced Air Pollution via Aerosol-Boundary Layer Feedback in China. Sci. Rep. 6, 18998. doi:10.1038/srep18998
Seibert, P., Beyrich, F., Gryning, S. E., Joffre, S., Rasmussen, A., and Tercier, P. (2000). Review and Intercomparison of Operational Methods for the Determination of the Mixing Height. Atmos. Environ. 34 (7), 1001–1027. doi:10.1016/s1352-2310(99)00349-0
Shahzad, M. I., Nichol, J. E., Campbell, J. R., and Wong, M. S. (2018). Assessment of MODIS, OMI, MISR and CALIOP Aerosol Products for Estimating Surface Visual Range: A Mathematical Model for Hong Kong. Remote Sens. 10 (9). doi:10.3390/rs10091333
Shahzad, M. I., Nichol, J. E., Wang, J., Campbell, J. R., and Chan, P. W. (2013). Estimating Surface Visibility at Hong Kong From Ground-Based LIDAR, Sun Photometer and Operational MODIS Products. J. Air Waste Manag. Assoc. 63 (9), 1098–1110. doi:10.1080/10962247.2013.801372
Song, C., Wu, L., Xie, Y., He, J., Chen, X., Wang, T., et al. (2017). Air Pollution in China: Status and Spatiotemporal Variations. Environ. Pollut. 227, 334–347. doi:10.1016/j.envpol.2017.04.075
Spandana, B., Srinivasa Rao, S., Upadhya, A. R., Kulkarni, P., and Sreekanth, V. (2021). PM2.5/PM10 Ratio Characteristics over Urban Sites of India. Adv. Space Res. 67 (10), 3134–3146. doi:10.1016/j.asr.2021.02.008
Stein, A. F., Draxler, R. R., Rolph, G. D., Stunder, B. J. B., Cohen, M. D., and Ngan, F. (2015). NOAA’s HYSPLIT Atmospheric Transport and Dispersion Modeling System. Bull. Amer. Meteor. Soc. 86, 2059–2077. doi:10.1175/BAMS-D-14-00110.1
Su, T., Li, Z., and Kahn, R. (2018). Relationships between the Planetary Boundary Layer Height and Surface Pollutants Derived from Lidar Observations over China: Regional Pattern and Influencing Factors. Atmos. Chem. Phys. 18 (21), 15921–15935. doi:10.5194/acp-18-15921-2018
Sun, Y., Du, W., Wang, Q., Zhang, Q., Chen, C., Chen, Y., et al. (2015). Real-Time Characterization of Aerosol Particle Composition above the Urban Canopy in Beijing: Insights into the Interactions between the Atmospheric Boundary Layer and Aerosol Chemistry. Environ. Sci. Technol. 49 (19), 11340–11347. doi:10.1021/acs.est.5b02373
Swietlicki, E., Hansson, H.-C., Hämeri, K., Svenningsson, B., Massling, A., McFiggans, G., et al. (2008). Hygroscopic Properties of Submicrometer Atmospheric Aerosol Particles Measured with H-TDMA Instruments in Various Environments-A Review. Tellus B Chem. Phys. Meteorology 60 (3), 432–469. doi:10.1111/j.1600-0889.2008.00350.x
Tao, J., Zhang, L., Gao, J., Wang, H., Chai, F., and Wang, S. (2015). Aerosol Chemical Composition and Light Scattering during a Winter Season in Beijing. Atmos. Environ. 110, 36–44. doi:10.1016/j.atmosenv.2015.03.037
van Donkelaar, A., Martin, R. V., Brauer, M., Kahn, R., Levy, R., Verduzco, C., et al. (2010). Global Estimates of Ambient Fine Particulate Matter Concentrations from Satellite-Based Aerosol Optical Depth: Development and Application. Environ. Health Perspect. 118 (6), 847–855. doi:10.1289/ehp.0901623
Vogelezang, D. H. P., and Holtslag, A. A. M. (1996). Evaluation and Model Impacts of Alternative Boundary-Layer Height Formulations. Boundary-Layer Meteorol. 81 (3-4), 245–269. doi:10.1007/bf02430331
Wang, G., Deng, X.-J., Wang, C.-L., Zhang, X.-Y., Yan, H.-H., Chen, D.-H., et al. (2019a). A New and Detailed Assessment of the Spatiotemporal Characteristics of the SO2 Distribution in the Pearl River Delta Region of China and the Effect of SO2 Emission Reduction. Aerosol Air Qual. Res. 19 (8), 1900–1910. doi:10.4209/aaqr.2019.03.0135
Wang, J., Xu, X. G., Spurr, R., Wang, Y. X., and Drury, E. (2010). Improved Algorithm for MODIS Satellite Retrievals of Aerosol Optical Thickness Over Land in Dusty Atmosphere: Implications for Air Quality Monitoring in China. Remote Sens. Environ. 114 (11), 2575–2583. doi:10.1016/j.rse.2010.05.034
Wang, M., Chen, Y., Fu, H., Qu, X., Li, B., Tao, S., et al. (2020). An Investigation on Hygroscopic Properties of 15 Black Carbon (BC)-containing Particles from Different Carbon Sources: Roles of Organic and Inorganic Components. Atmos. Chem. Phys. 20 (13), 7941–7954. doi:10.5194/acp-20-7941-2020
Wang, X., Zhang, R., and Yu, W. (2019b). The Effects of PM 2.5 Concentrations and Relative Humidity on Atmospheric Visibility in Beijing. J. Geophys. Res. Atmos. 124 (4), 2235–2259. doi:10.1029/2018jd029269
Wei, W., Zhang, H., Wu, B., Huang, Y., Cai, X., Song, Y., et al. (2018). Intermittent Turbulence Contributes to Vertical Dispersion of PM<sub>2.5</sub> in the North China Plain: Cases from Tianjin. Atmos. Chem. Phys. 18 (17), 12953–12967. doi:10.5194/acp-18-12953-2018
Wu, D., Deng, X., Li, F., and Tan, H. (2007). Effect of Atmospheric Haze on the Deterioration of Visibility over the Pearl River Delta. J. Meteorological Res. 21 (2), 215–223.
Wu, Z., Zhang, Y., Zhang, L., Huang, M., Zhong, L., Chen, D., et al. (2019). Trends of Outdoor Air Pollution and the Impact on Premature Mortality in the Pearl River Delta Region of Southern China during 2006-2015. Sci. Total Environ. 690, 248–260. doi:10.1016/j.scitotenv.2019.06.401
Xia, X., Qi, Q., Liang, H., Zhang, A., Jiang, L., Ye, Y., et al. (2017). Pattern of Spatial Distribution and Temporal Variation of Atmospheric Pollutants during 2013 in Shenzhen, China. Ijgi 6 (2), 2. doi:10.3390/ijgi6010002
Xiao, R., Takegawa, N., Zheng, M., Kondo, Y., Miyazaki, Y., Miyakawa, T., et al. (2011). Characterization and Source Apportionment of Submicron Aerosol with Aerosol Mass Spectrometer during the PRIDE-PRD 2006 Campaign. Atmos. Chem. Phys. 11 (14), 6911–6929. doi:10.5194/acp-11-6911-2011
Xiao, Z. Y., Jiang, H., and Song, X. D. (2016). Aerosol Optical Thickness Over Pearl River Delta Region, China. Int. J. Remote Sens. 38 (1), 258–272. doi:10.1080/01431161.2016.1264024
Yan, P., Pan, X., Tang, J., Zhou, X., Zhang, R., and Zeng, L. (2009). Hygroscopic Growth of Aerosol Scattering Coefficient: A Comparative Analysis between Urban and Suburban Sites at Winter in Beijing. Particuology 7 (1), 52–60. doi:10.1016/j.partic.2008.11.009
Yang, J. X., Lau, A. K. H., Fung, J. C. H., Zhou, W., and Wenig, M. (2012). An Air Pollution Episode and its Formation Mechanism during the Tropical Cyclone Nuri's Landfall in a Coastal City of South China. Atmos. Environ. 54, 746–753. doi:10.1016/j.atmosenv.2011.12.023
Yang, W., Ji, Y., Lin, H. T., Yang, Y., Kang, S. B., and Yu, J. Y. (2015). “Ambient Occlusion via Compressive Visibility Estimation,” in 2015 Ieee Conference on Computer Vision and Pattern Recognition (Cvpr), Boston, MA, USA, 7-12 June 2015, 3882–3889.
Yang, Y., Russell, L. M., Lou, S. J., Liao, H., Guo, J. P., Liu, Y., et al. (2017). Dust-Wind Interactions Can Intensify Aerosol Pollution Over Eastern China. Nat. Comm. 8, 15333. doi:10.1038/ncomms15333
Yu, L. E., Shulman, M. L., Kopperud, R., and Hildemann, L. M. (2005). Characterization of Organic Compounds Collected during Southeastern Aerosol and Visibility Study: Water-Soluble Organic Species. Environ. Sci. Technol. 39 (3), 707–715. doi:10.1021/es0489700
Yu, M., Zhu, Y., Lin, C. J., Wang, S., Xing, J., Jang, C., et al. (2009). Effects of Air Pollution Control Measures on Air Quality Improvement in Guangzhou, China. J. Environ. Manage 244, 127–137. doi:10.1016/j.jenvman.2019.05.046
Yue, D. L., Hu, M., Wang, Z. B., Wen, M. T., Guo, S., Zhong, L. J., et al. (2013). Comparison of Particle Number Size Distributions and New Particle Formation between the Urban and Rural Sites in the PRD Region, China. Atmos. Environ. 76, 181–188. doi:10.1016/j.atmosenv.2012.11.018
Zhang, Q., Zheng, Y., Tong, D., Shao, M., Wang, S., Zhang, Y., et al. (2019). Drivers of Improved PM 2.5 Air Quality in China from 2013 to 2017. Proc. Natl. Acad. Sci. U.S.A. 116 (49), 24463–24469. doi:10.1073/pnas.1907956116
Zhang, Y. H., Hu, M., Zhong, L. J., Wiedensohler, A., Liu, S. C., Andreae, M. O., et al. (2008). Regional Integrated Experiments on Air Quality over Pearl River Delta 2004 (PRIDE-Prd2004): Overview. Atmos. Environ. 42 (25), 6157–6173. doi:10.1016/j.atmosenv.2008.03.025
Zhang, Y. L., and Cao, F. (2015). Fine Particulate Matter (PM 2.5) in China at a City Level. Sci. Rep. 5, 14884. doi:10.1038/srep14884
Zhao, C., Li, Y., Zhang, F., Sun, Y., and Wang, P. (2018). Growth Rates of Fine Aerosol Particles at a Site Near Beijing in June 2013. Adv. Atmos. Sci. 35 (2), 209–217. doi:10.1007/s00376-017-7069-3
Zheng, C., Zhao, C., Zhu, Y., Wang, Y., Shi, X., Wu, X., et al. (2017). Analysis of Influential Factors for the Relationship between PM<sub>2.5</sub> and AOD in Beijing. Atmos. Chem. Phys. 17 (21), 13473–13489. doi:10.5194/acp-17-13473-2017
Zheng, J., Che, W., Zheng, Z., Chen, L., and Zhong, L. (2013). Analysis of Spatial and Temporal Variability of PM10 Concentrations Using MODIS Aerosol Optical Thickness in the Pearl River Delta Region, China. Aerosol Air Qual. Res. 13 (3), 862–876. doi:10.4209/aaqr.2012.09.0234
Zhou, S., Wang, T., Wang, Z., Li, W., Xu, Z., Wang, X., et al. (2014). Photochemical Evolution of Organic Aerosols Observed in Urban Plumes from Hong Kong and the Pearl River Delta of China. Atmos. Environ. 88, 219–229. doi:10.1016/j.atmosenv.2014.01.032
Zhou, S., Wu, L., Guo, J., Chen, W., Wang, X., Zhao, J., et al. (2020). Measurement Report: Vertical Distribution of Atmospheric Particulate Matter within the Urban Boundary Layer in Southern China - Size-Segregated Chemical Composition and Secondary Formation through Cloud Processing and Heterogeneous Reactions. Atmos. Chem. Phys. 20 (11), 6435–6453. doi:10.5194/acp-20-6435-2020
Keywords: air quality, visibility, PM mass concentration, AOD, background site, Pearl River Delta
Citation: Fan X, Xia X, Chen H, Zhu Y, Li J, Yang H and Luo H (2022) Baseline of Surface and Column-Integrated Aerosol Loadings in the Pearl River Delta Region, China. Front. Environ. Sci. 10:893408. doi: 10.3389/fenvs.2022.893408
Received: 10 March 2022; Accepted: 19 April 2022;
Published: 30 May 2022.
Edited by:
Jianhuai Ye, Southern University of Science and Technology, ChinaReviewed by:
Shunyao Wang, Shanghai University, ChinaLei Shu, Southern University of Science and Technology, China
Copyright © 2022 Fan, Xia, Chen, Zhu, Li, Yang and Luo. This is an open-access article distributed under the terms of the Creative Commons Attribution License (CC BY). The use, distribution or reproduction in other forums is permitted, provided the original author(s) and the copyright owner(s) are credited and that the original publication in this journal is cited, in accordance with accepted academic practice. No use, distribution or reproduction is permitted which does not comply with these terms.
*Correspondence: Xuehua Fan, fxh@mail.iap.ac.cn
†ORCID ID: Yanliang Zhu orcid.org/0000-0002-9898-5375