- 1Water, Environment and Agriculture Program, Department of Infrastructure Engineering, University of Melbourne, Melbourne, VIC, Australia
- 2Arthur Rylah Institute for Environmental Research, Department of Environment, Land Water and Planning Heidelberg, Melbourne, VIC, Australia
- 3Streamology Pty. Ltd, Bright, VIC, Australia
Ecological responses to changing riverine flows are often evaluated by describing the relationship between river discharge and response. However, aquatic organisms experience the hydraulics (i.e. velocity, shear stress, depth) of a river, not its discharge. Hydraulic characterizations of riverine habitats may improve our ability to predict ecological responses. We used two-dimensional hydraulic models to translate river discharge into flow velocity. We used discharge and reach-averaged velocity, along with water temperature and 8 years of field observations of fish spawning, to develop predictive models of the spawning of golden perch (Macquaria ambigua) in the Goulburn River, south-east Australia. Probability of spawning was positively related to both discharge and reach-averaged velocity. Water temperature was critical for enabling the flow response, and antecedent flows prior to spawning had a weak positive effect. Against expectations, there was little difference in predictive uncertainty for the effect of flows when reach-averaged velocity was used as the main predictor rather than discharge. The lower Goulburn River has a relatively simple channel and so discharge and velocity are monotonically related over most flows. We expect that in a more geomorphically complex environment, improvement in predictive ability would be substantial. This research only explores one example of a hydraulic parameter being used as a predictor of ecological response; many others are possible. The extra effort and expense involved in hydraulic characterization of river flows is only justified if our understanding of flow-ecology relationships is substantially improved. Further research to understand which environmental responses might be best understood through different hydraulic parameters, and how to better characterize hydraulic characteristics relevant to riverine biota, would help inform decisions regarding investment in hydraulic models. Regardless, hydraulics offers a more process-based assessment of ecological responses to changing flows, has the potential to facilitate mechanistic understanding rather than just associations, and provides the opportunity to translate hydraulic metrics that drive ecological responses across river systems of differing sizes. However, while considering ecological responses in terms of river hydraulics is more physically realistic, our results suggest that average hydraulic conditions may not result in an improved ability to predict the effects of changing flows.
Introduction
Regulation of the majority of the world’s rivers has caused myriad environmental impacts on the biotas of the world’s freshwater ecosystems (Vorosmarty et al., 2010; Horne et al., 2017). In response, environmental flows are being increasingly used to partly address these negative impacts. Environmental flows are defined as “the quantity, timing, and quality of freshwater flows and levels necessary to sustain aquatic ecosystems which, in turn, support human cultures, economies, sustainable livelihoods, and well-being” (Arthington et al., 2018). Environmental flows can be controversial in systems that are heavily allocated for agriculture and other consumptive purposes (Poff et al., 2003), and often represent a substantial monetary value of water that could otherwise be used for “productive” purposes (Jorda-Capdevila & Rodríguez-Labajos, 2017). Governments and management agencies must therefore monitor the outcomes of environmental flows to demonstrate return on this investment in the environment and also to incrementally improve management of the environmental water asset and efficiency of its use through adaptive management (Webb et al., 2017b; Webb et al., 2018a).
Many environmental flows assessment methods recommend the delivery of flow components (Poff et al., 2017) of specific timing, duration and volume to emulate aspects of the natural flow regime that are important for ecological functions (Poff et al., 1997). The required flow components may be derived from empirical flow-response models (e.g. Arthington et al., 2012; McManamay et al., 2013), based on an expert panel process, or some combination of both. While the flow components are usually defined in hydrologic terms (e.g. m3s−1), those volumes are often calculated to deliver a specific habitat outcome via some form of hydraulic model. These can be simple or complex. For example, EarthTech (2003) used the one-dimensional hydraulic modelling software HECRAS to calculate the flows required to provide minimum navigable depth for large bodied fish. Conversely MESOHABSIM (Parasiewicz, 2007) creates a fine scale three-dimensional model of the different hydraulic habitats generated by different flows. Some flow component recommendations also directly consider the effects of flow on other aspects of an organism’s life history. For example, Horne et al. (2020, Table 10) recommends elevated spring flows to cue fish migration through systems, thereby dispersing populations. However, despite the frequent consideration of hydraulics during the environmental flows assessment process, during evaluation of the outcomes of environmental flows and adaptive management, the occurrence or not of an ecological response is often solely assessed against the hydrologic description of the flow component (e.g. Webb et al., 2010).
While it is convenient to describe flow components and their relationships to ecological outcomes in terms of hydrology, riverine biotas actually experience the hydraulics of the environment (Maddock, 2013; Mallen-Cooper & Zampatti, 2018). Biological responses are driven by such aspects as depth, water velocity, shear stress or turbulence (e.g. Wheaton et al., 2018). It therefore makes sense to use hydraulic descriptors of flow when evaluating ecological responses to environmental flows. Moreover, hydraulic descriptors of flow may be non-linearly (or even non-monotonically) related to discharge, and so they may be more useful for predicting effects of potential environmental flow deliveries (Turner & Stewardson, 2014). Finally, not only can this approach improve the ecological realism of the driver against which responses are evaluated, hydraulic evaluations offer the potential to extrapolate findings of monitoring and evaluation among multiple river systems with different channel sizes. This is because, while the responses to discharge will vary greatly among different sized rivers, responses to the hydraulic environment are more likely to be similar (Webb et al., 2017a).
Despite these advantages, hydraulic evaluations of ecological responses to flow regimes (i.e. quantity, timing, volume and duration of flow events; Arthington et al., 2018) that have been restored with environmental flows are rare in the literature (but for an example see Bice et al., 2017). This may be because of the interdisciplinary expertise required to address such ecohydraulic questions (Nestler et al., 2016). However, this connection has been made in other contexts. Hydraulic models have been used in making predictions of ecological responses under climate change (e.g., Poff et al., 2016), to help design individual environmental flow events (Stuart & Sharpe, 2021), used to assess biotic habitat and retention in rivers (Vietz et al., 2013), and been proposed as tools for generalized improved management of rivers and catchments (Harby et al., 2017). Their lack of use in direct evaluation of environmental flow responses appears to be an oversight.
Here, we report upon work in the Goulburn River, south-eastern Australia, examining spawning of the native fish species golden perch (Macquaria ambigua). Golden perch is generally acknowledged as showing positive spawning responses to elevated flow volumes during spring-summer (Zampatti & Leigh, 2013; Koster et al., 2014; Koster et al., 2017). This behavior is consistent across rivers of very different sizes (Stuart & Sharpe, 2020), and there is little evidence that other habitat characteristics (e.g., depth) are important for spawning on their own. We used two-dimensional (2D) hydraulic models to translate discharge into reach-averaged velocity—one hydraulic parameter that is a more likely direct cause of spawning behaviour. We present results for analyses that used the traditional hydrologic descriptor of discharge as the independent variable, along with an alternate model that employed water velocity, and evaluate the relative performance of both. Based on these analyses, we discuss the value of considering hydraulic descriptors in evaluations of environmental flow outcomes in the future.
Methods
Study area and background to monitoring
The Goulburn River is a major tributary of the River Murray in south-eastern Australia. It runs north from the Great Dividing Range and enters the River Murray upstream of Echuca (Figure 1). The lower Goulburn River, approximately 220 river km downstream of Goulburn Weir, is the focus of monitoring and evaluation of the effects of environmental flows funded by the Commonwealth Environmental Water Office (CEWO). The monitoring began with Short-Term Intervention Monitoring (STIM) projects from 2012-14 (Webb et al., 2015). These projects were replaced by the much larger scale and coordinated Long Term Intervention Monitoring (LTIM) Project (Gawne et al., 2020). On its completion, the LTIM Project was replaced with the Monitoring Evaluation and Research (MER) Program, which is running in the first instance from 2019-22 (Webb et al., 2019b). Monitoring in these programs has covered a wide variety of endpoints, including physical habitat, water quality and ecosystem metabolism, macroinvertebrates, vegetation and native fish assemblages (Webb et al., 2019b). The programs monitor effects of environmental water being released under The Basin Plan—an ambitious federal government program to restore environmental flows of some 2750 GL (2.75 × 109 m3) annually to the rivers of the Murray-Darling Basin. The goal of the Basin Plan is to improve ecological function of river and floodplain ecosystems, and provide a more sustainable foundation for irrigated agriculture (Hart, 2016).
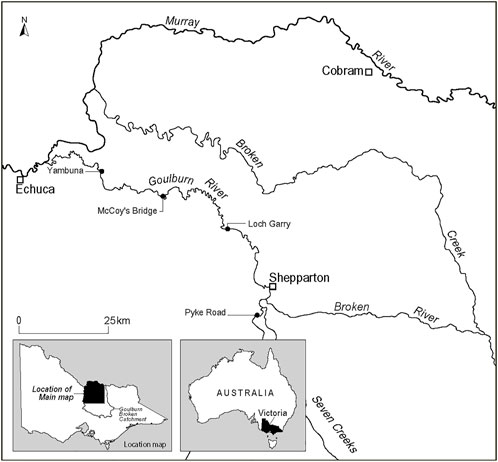
FIGURE 1. Map of the lower Goulburn River, showing study sites used in this research. The lower Goulburn is generally defined as beginning downstream of Goulburn Weir, which is to the south of the mapped portion of the river. Insets illustrate the location of the Goulburn-Broken catchment within the state of Victoria and within Australia.
Golden perch data
We are monitoring spawning of golden perch at four sites in the lower Goulburn River. Golden perch is a native species of Australian inland waters that is threatened by human development of waterways. It is one of only two species in the Murray-Darling Basin with well-demonstrated spawning responses to change in flows (King et al., 2009; Zampatti & Leigh, 2013; Koster et al., 2017), and hence is a frequent target of environmental flows monitoring programs. The fish generally spawn on high flow events in the Austral spring-summer when water temperatures are getting warmer (Humphries & Walker, 2013), and there is some indication that smaller flow increases in the weeks prior to spawning also have a positive effect (Cockayne et al., 2013). Golden perch is a pelagic broadcast spawner (Koster et al., 2017); gametes are released directly into the water column and eggs drift downstream on river currents (Stuart & Sharpe, 2020). For this paper, spawning data for 8 years—2013-20 are included. This encompasses one of the STIM projects, 5 years of the LTIM Project, plus the first 2 years of data collected under the MER Program.
Monitoring of spawning was conducted weekly using drift net sampling during spring-summer (October-December) at four sites (Figure 1), with occasional extra sampling arranged specifically around managed flow events (water released deliberately by managers to attempt to stimulate spawning). For the LTIM and MER projects, data collection methods were identical. Three drift nets (50 cm diameter, 1.5 m taper to sample bottle, 500 μm mesh size) were deployed overnight in locations within the stream that were initially chosen using a stratified random approach, and which have then been used for each year of sampling. For the 2013 STIM data, sampling was not conducted at Loch Garry or McCoy’s Bridge; samples were taken at Pyke Road and Yambuna, and data from an additional site, Cable Hole, were excluded because this was the only year of sampling for that site. Also for 2013, only two nets were deployed per site. Water temperature was recorded at each site at the time of deployment. Drift samples were inspected briefly in the field to obtain fertilized eggs so that these could be taken to the laboratory for hatching to assist identification. The remainder of each sample was immersed in an overdose concentration solution of anaesthetic, preserved in 90% ethanol, and taken to the laboratory for processing and identification. Although catch-per-unit-effort of eggs/larvae was calculated from the raw data, the analysis in this paper only examines the presence or absence of spawning. See (Webb et al., 2019b) for more details on the larval sampling.
Hydraulic models
At three of the four sites where golden perch spawning is being monitored (Darcy’s Track—near Pyke Road, Loch Garry, McCoy’s Bridge), we have developed 2-dimensional hydraulic models (Webb et al., 2016). There is no model for the site at Yambuna. We used the model for McCoy’s Bridge to predict reach-averaged velocity at Yambuna based on the following argument (See Supplementary Material S1 for more detail). First, a morphometric analysis of the four sites shows that Yambuna is physically very similar to McCoy’s Bridge. Second, in consideration of this, and given that the relationship between discharge and reach-averaged velocity is very similar for the other three sites (see Results below), it was reasonable to use McCoy’s Bridge. Third, Yambuna is relatively close (∼20 km) to McCoy’s Bridge in a low-gradient section of the river where channels change little and there are no tributary inputs between the two sites. Finally, we are using reach-averaged velocity as the predictor of spawning, rather than the fine scale hydraulics that are more likely to vary among sites.
The models were built on a topographic layer generated from aerial lidar surveys of above-water bench and bank features, aligned with boat-mounted sonographic surveys of underwater features. The models themselves were developed using River 2D software (www.river2d.ca). Each modelled site encompassed approximately 0.5–1.0 km of river channel, with the topographic mesh having an approximate 2 m2 triangular cell size within the river channel. We undertook field calibration and verification of model performance at two difference discharges (discharge data for validation and data analysis sourced from https://data.water.vic.gov.au/; velocity data from Acoustic Doppler Current Profiling (ADCP) collected in the field). See Supplementary Material S1 for more details on the development and validation of the hydraulic models.
Data analysis
We hypothesize that golden perch spawning is primarily caused by high flow events (Q)—quantified as either discharge or reach-averaged velocity on the day of sampling, but that water temperature (Te) provides a threshold that ‘enables’ spawning to occur (King et al., 2016). In addition, we hypothesize that antecedent flows prior to monitoring affect the probability of spawning. Over several years of analyses, we have experimented with different periods of antecedent flow for this secondary hypothesis; for the analyses in this paper, we include the effect of antecedent flows of the 5 weeks (Q5wk) prior to monitoring. This linked set of hypotheses was tested using the Bayesian hierarchical logistic regression below, where Bern implies the Bernoulli distribution and logit refers to a logit-transformation of the probability of spawning (see Gelman & Hill, 2007; Webb et al., 2010 for background on hierarchical Bayesian modelling). This analysis calculated the probability of spawning under different conditions of flow on the day of sampling, water temperature, and antecedent flow conditions.
The random effects were drawn from a normal distribution with mean zero and standard deviation estimated during model fitting. The site-level estimates of eff. Q and eff. Q5wk were modelled hierarchically and drawn from a hyper-parameter distribution. All prior distributions for parameters were assigned as minimally informative, with N(0,10) for means and U[0,10] for standard deviations (Gelman et al., 2013). As described above, flow on the day of sampling (Q) was included as both discharge (in Australia, it is common to use Megalitres per day (1 m3 s−1 = 86.4 ML d−1) in water management and we adopt this convention in this paper) and reach-averaged velocity (m∙s−1) in separate analyses of the effects of flow. Discharge and reach-averaged velocity data were log-transformed to improve normality of the data set. The discharge and reach-averaged velocity (after transformation), and the water temperature data were then standardized by subtracting the mean and dividing by the standard deviation of the entire data set. This ensured comparability of the resulting parameter estimates from the different analyses. Four models were fitted altogether. There were two models that used discharge as the main predictor variable for eff. Q and two that used reach-averaged velocity. This was crossed with two models that incorporated the effect of antecedent flows (eff.Q5wk) and two that did not.
The models were fitted using Stan statistical software (Carpenter et al., 2017), via the RStan interface for the R language (Stan Development Team, 2018). Four independent Markov chains were employed, each with 50,000 iterations (including 25,000 iterations employed as a burn-in) with a thinning rate of one in 10 for parameter estimation. This resulted in a total of 10,000 Markov chain iterations for parameter estimation. Model convergence was verified using the
Results
Hydraulic models
Average velocities across the ADCP data—the most important comparison for the analyses conducted in this paper—revealed little evidence of substantial bias in the modelled reach-averaged velocities. The greatest difference was a 0.08 m s−1 overestimate of modelled average velocity at McCoy’s Bridge for the high flow event (Supplementary Material S1; Supplementary Table S1.9). At finer scales, the differences were larger. Cross section-scale velocities as measured by ADCP, showed minor differences compared to the modelled velocities after calibration of the models, with occasional sections showing greater deviations (e.g. Hydraulic models, Supplementary Table S1.5). Within cross-sections, there were occasional large differences between point-wise modelled and measured velocities (e.g. Supplementary Figure S1.18). Such differences were often explained by the presence of local blockages (e.g. large woody debris) within the channel that was not included in the model. Overall, however, we were satisfied that the models were giving sufficiently accurate estimates of the reach-averaged velocities used in the data analysis.
The hydraulic models produce a range of hydraulic metrics that provide greater insight into the flow environment than the simple measures of discharge. In the example provided, point-wise velocity illustrates a substantial change (e.g. much greater area of yellow, orange and red, indicating point-wise flows of >0.40 m s−1) in the spatial pattern of depth-averaged velocities at McCoy’s Bridge when moving from 930 ML d−1–5600 ML d−1, but then little additional change in velocities with a further jump to 8000 ML d−1 (Figure 2). For all discharges, highest velocities are observed at narrowest points in the channel.
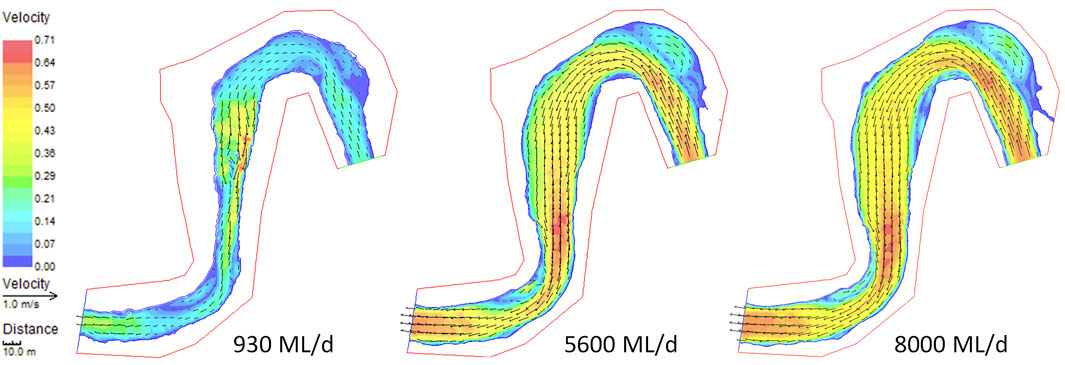
FIGURE 2. Velocity outputs for the 2D hydraulic model at the McCoy’s Bridge site. Plots show depth-averaged velocities at three different discharges as per the inset labels. Blue colours are slow velocities, through to red being the highest. Red border is the limit of the channel area modelled and is close to bankfull elevation.
Such calculations at different discharges provide an export of nodes, which within GIS were used to analyse hydraulic characteristics and to generate rating curves relating hydraulic parameters to discharge. These curves can then be converted to equations or lookup tables to allow easy translation of discharge to hydraulic metrics. For the velocity-based analyses presented below, we used the curves shown in Figure 3 to convert daily discharge to reach-averaged velocity.
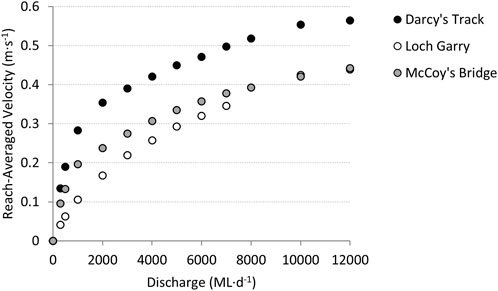
FIGURE 3. ‘Rating curves’ relating discharge to reach-averaged velocity for the three hydraulic models used in this study.
Golden perch spawning
Across 285 total sampling events (site x date) over the 8 years of monitoring, we collected golden perch eggs or larvae on 29 occasions. Spawning was more common moving from upstream to downstream, with 10 of the 29 detections occurring at Yambuna and nine at McCoy’s Bridge (Figures 4C,D). Spawning was generally detected under combinations of elevated discharge and temperature. There was an anomalous detection of a small number of larvae at baseflow levels at two sites (Pyke Road—927 ML d−1; Loch Garry—1032 ML d−1) on 8 November 2018 (Figures 4A,B). These detections occurred shortly after the end of a high flow event—discharges had been back at baseflow levels for only 5 days - and so it is possible that we detected larvae that were stimulated by these earlier high flows. Apart from these two observations, spawning was only detected under conditions of elevated flow, with the next lowest discharge at which spawning was detected being 2,771 ML d−1 at Loch Garry on 2 November 2016 (Figure 4B). This is ∼3 times target baseflows for the lower Goulburn River. All other detections of spawning were at discharges above 3,500 ML d−1. The lowest temperature at which we detected spawning was 17.1°C at McCoy’s Bridge on 20 October 2020 (Figure 4C). However, this was also somewhat anomalous, with 25 of the 29 detected spawning events occurring at temperature of 19°C or greater. Elevated flow and temperature did not guarantee that spawning would be detected (e.g. Figure 4D).
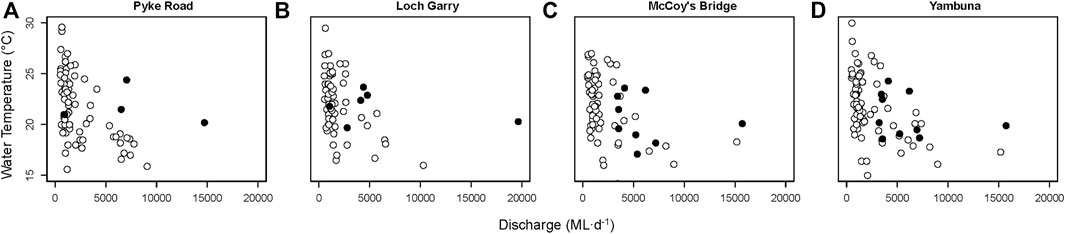
FIGURE 4. Temperature and discharge conditions of the spawning samples. Each point is the combination of discharge and water temperature on the day of sampling. Black markers are those samples for which golden perch eggs or larvae were detected, with white circles being no detection. Panels are arranged from upstream [(A)-Pyke Road] to [(D)-Yambuna] downstream.
Statistical results
All models demonstrated the importance of flows for stimulating spawning. All 95% credible intervals for the effects of flow (eff.Q) were above the 0 line of ‘no effect’ (Figure 5). The smallest median effect was observed at Pyke Road for the discharge-based models and at Loch Garry for the reach-averaged velocity-based models, consistent with the less frequent occurrent of spawning at these sites (Figures 4A,B). The greatest median effect was seen at McCoy’s Bridge for all models. Uncertainty regarding the effect of flow was slightly greater for the two reach-averaged velocity-based models compared to the discharged-based models at all sites other than Loch Garry.
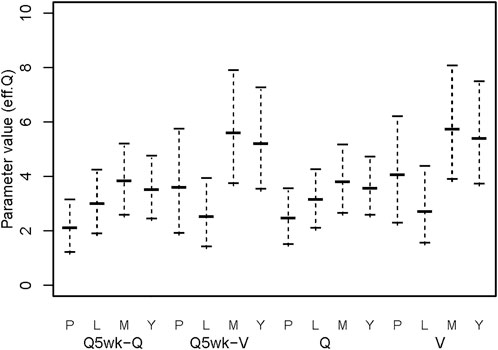
FIGURE 5. Effects of flow on spawning probability. The graph shows the estimated parameter values for the effect of flow (eff.Q) for each site and each of the four models developed. Horizontal bars show the median parameter estimate with whiskers encompassing the 95% credible interval for the estimate. Sites are arranged from upstream to downstream (P = Pyke Road, L = Loch Garry, M = McCoy’s Bridge, Y = Yambuna). The first two sets of results are those that included antecedent flows (Q5wk) as an additional driver of spawning; the second two sets of results did not include Q5wk. Q implies that flow effects were modelled using discharge; V implies that reach-averaged velocity was used.
Water temperature was an important threshold variable and was estimated very precisely by all models. Distributions of the threshold spawning temperature (Te.crit) were almost identical for the four models (Figure 6), with the medians close to 18.56°C for all four models, and with 95% credible intervals spanning just over 0.1°C.
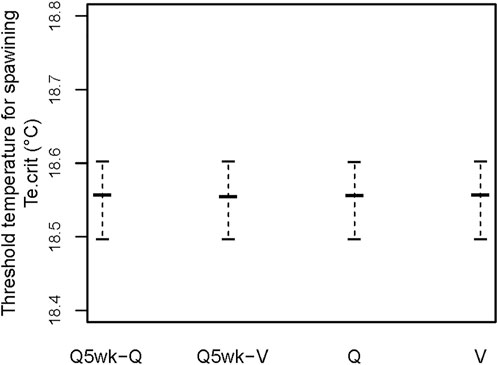
FIGURE 6. Effects of temperature on ability to spawn. The graph shows the estimated threshold temperature for spawning (Te.crit) for each of the four model structures as described for Figure 5. Horizontal bars show the median parameter estimate with whiskers encompassing the 95% credible interval for the estimate.
In contrast, effects of antecedent flows on spawning probability were weakly positive. The median effect sizes for eff. Q5wk were above zero for both models that considered antecedent flows, but the credible intervals extended below zero (Figure 7).
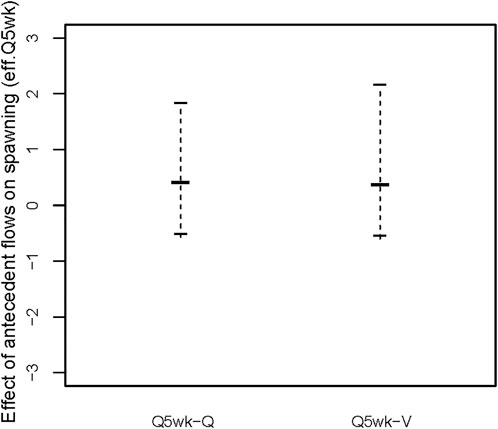
FIGURE 7. Effects of 5-week antecedent flows (eff.Q5wk) on ability to spawn, for each of the two model structures that include the antecedent flows, as described for Figure 5. The plot shows the hyperparameter estimate for eff. Q5wk across the four sites, but all site-level estimates show similar patterns. Horizontal bars show the median parameter estimate with whiskers encompassing the 95% credible interval for the estimate.
Predictions of spawning
We compared the relative abilities of the two antecedent flow models to predict the probability of spawning. These predictions under different combinations of flow and temperature (Figure 8) reveal several characteristics of golden perch spawning in the lower Goulburn River. First, spawning is possible at all sites at 18.56°C (the approximate median estimates of the critical threshold for temperature—Te.crit), but this is highly uncertain, with the 95% credible intervals spanning the full probability space from 0-1 (Figures 8A–H). With this temperature being close to the median estimates for the Te.crit threshold, around half of model iterations will not have the flow-related effect of spawning ‘enabled’ and so the result is not surprising. Second, spawning at 20°C is least likely at the upstream site Pyke Road, but even at this site median estimates of the probability of spawning exceed 0.8 at high discharges and velocities (Figure 8I,M). This reflects the relative rarity of spawning at this site (4 instances over the 8 years). Conversely, spawning is most likely at the downstream sites at McCoy’s Bridge and Yambuna (Figures 8K,L,O,P), also consistent with our observations of spawning. Third, we calculated spawning probabilities at 21.5°C and they were identical to those for 20.0°C (results not shown). This is because both temperatures are well beyond the estimate for Te.crit. An examination of the Te.inc parameter showed that it was one for every iteration of the Markov chains for both temperatures. Finally, although it is difficult to directly compare the two x-axes for discharge and velocity, we can see that estimates of the probability of spawning are not systematically different or more precise when modelled using the velocity-based model rather than the discharge-based model (Figure 8I vs. M, J vs. N, K vs. O, L vs. P). The estimate is less precise at Loch Garry for the velocity-based model, but otherwise the sites show very little difference in the predictions of the two models.
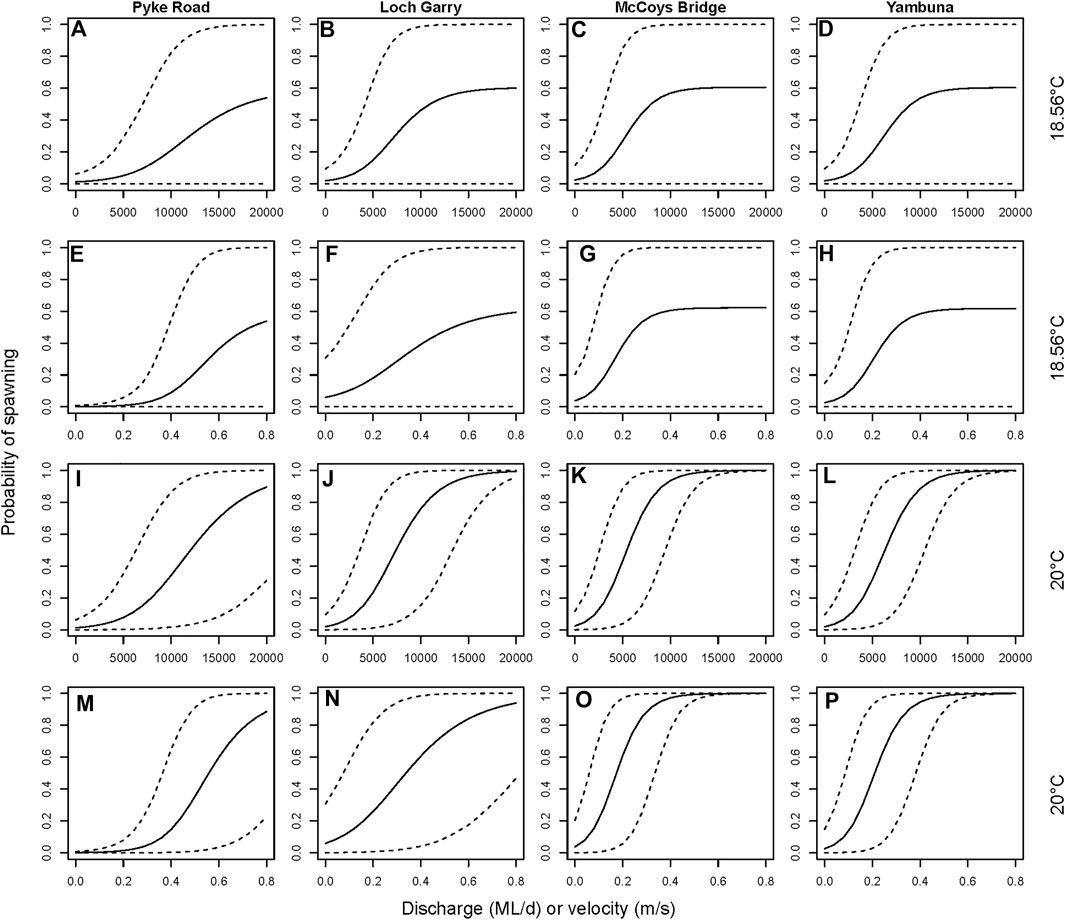
FIGURE 8. Graphs show the estimated probabilities of spawning at different combinations of flow and temperature. Predictions are based on the two models that incorporated antecedent flows (Q5wk) and are predicted for the mean Q5wk across the data set. Y axes are the estimated probabilities and x-axes are the discharge (ML∙d−1, panels (A–D), (I–L) and Velocity (m∙s−1, panels (E–H), (M–P). The x-axis limits are approximately equivalent at the mid-points (i.e. 10,000 ML d−1 ≈ 0.40 m s−1) for the hydraulic models for Loch Garry and McCoys Bridge (Figure 2). Solid line is the median estimate of probability with the dotted lines encompassing the 95% credible interval of the estimate. Columns show the different sites, with the first two rows being predictions at the approximate median estimate for Te. crit of 18.56°C and rows three and four being predictions at 20.0°C. Within each temperature, the pair of graphs for each site compares the predictions for the discharge-based model (Q; panels (A–D), (I–L) and reach-averaged velocity-based model (V; panels (E–H), (M–P).
Discussion
For the results presented above, the hydraulics-based approach to modelling ecological responses to changing flows, using reach-averaged velocity as one example of a hydraulic parameter, resulted in similar precision of predictions of spawning of golden perch as did the hydrology-based models. Thus, in this case, a hydraulics-based approach to evaluating and predicting the benefits of environmental flows would not give managers greater confidence to use limited environmental water to promote spawning in golden perch. However, we assessed only a single hydraulic metric, and one that is averaged across the reach and may not recognize the diversity and complexity of habitat usage by the biota. Hydraulics-based investigations of ecological responses to changing flows may require greater specificity of the hydraulic conditions that are related to biotic response.
Why do we not see improved performance?
Our starting hypothesis was that hydraulics-based models would better predict spawning than hydrology-based models. As argued in the introduction, a hydraulics-based approach to flow-response relationships is arguably more realistic in terms of the characterization of the physical effects that we would expect ‘matter’ to biota (i.e. conditions felt or perceived), that it has greater potential to identify cause-effect relationships, and that the non-linear relationship between discharge and velocity ought to have resulted in different performance of the two models. We can advance several hypotheses as to why our results indicated no substantial differences in the two modelling approaches. First, we acknowledge that the velocity data used here are the outputs from a model, rather than direct measurements from the river as is the case with the discharge data. Any intermediate modelling introduces new uncertainties into the resulting outputs. River2D does not provide uncertainty estimates on its outputs. However, it is acknowledged that 2D models have uncertainties in the fine details of flow structure compared to high-quality field data (Lane et al., 1999). It is plausible that unreported uncertainties in the velocity data introduce extra variability into the modelled relationship between velocity and spawning, enough to cancel out any benefit of the stronger mechanistic relationship. Secondly, we used reach-averaged velocities as the hydraulic metric in these analyses. It is more likely that golden perch in the Goulburn River are responding to hydraulic forces at particular points in the river—perhaps near hydraulic controls where the channel is narrowest and point-scale velocities are therefore the highest (e.g. the red areas in Figure 2). This simplification could also have added extra variability into the modelled relationship between velocity and spawning. We must also recognize that we modelled only short sections of the river for hydraulics (<1 km), yet fish move through much longer reaches of the lower Goulburn River during spawning season (Webb et al., 2019a), and we may have missed critical sections of habitat. Moreover, although we detected eggs and larvae at these sites, the pelagic spawning of golden perch and the fact that eggs and larvae drift downstream after spawning (Koster et al., 2017; Stuart & Sharpe, 2020), means that the actual spawning by fish is likely to have taken place a short distance upstream rather than directly at the sites where spawning was detected. Finally, although the non-linear relationship between discharge and velocity seemingly ought to result in different performances of the model, we note that we are looking at something of a threshold response (spawning or no spawning) and that in the fitted models these probabilities change quite rapidly near the middle of the discharge-velocity rating curves shown in Figure 3 (see Figure 8I–P). At that point, the relationship between discharge and velocity is close to linear.
Related to this last point, the relationship between discharge and reach-averaged velocity presented here is quite simple. Like other rivers subjected to extensive regulation of flows over a prolonged period (sensu Graf, 2006), the lower Goulburn River has a simplified rectangular channel with few complex geomorphic features. Figure 3 thus shows a reasonably simple relationship between discharge and reach-averaged velocity for the three models used in this analysis. This means that for the most part discharge is an effective and easily measured surrogate for velocity in this system. We also recognize that hydrology may not characterize the complex interactions of habitat, such as where both velocity and depth are important to biota, and where channel width acts to cause increases in both. However, the simple channel of the lower Goulburn River means that depth and velocity will be highly correlated with increases in discharge, and it would not be possible to tease out their individual effects. A future analysis that incorporates spawning data from a variety of river channels of different sizes would be better placed to elucidate the relative influences of velocity versus depth.
Other channels with more complex geomorphology may show more complex relationships, and hence have more extreme differences in outcomes from discharge versus velocity-based analyses. For such channels, we would expect to see a greater improvement in our analyses of environmental response by taking a hydraulics-based approach. Other hydraulics-based descriptors of the stream environment may be even more complex, presenting non-monotonic relationships with discharge. For example, shallow slow-flowing areas are believed to be important locations for primary and secondary production in flowing river systems. They are areas of increased hydraulic retention of organic matter (Vietz et al., 2013), and provide habitat for instream vegetation, zooplankton and small bodied fish (Humphries et al., 2006). At the sites used in these analyses, total area of such habitat decreases with increasing discharge, but only up until the point where low-level benches described above are inundated. After this, the area of shallow slow-water habitat increases again until those benches are fully inundated (Webb et al., 2019a). Any analysis of ecological response that rests upon an assumption of the importance of such habitat would perform much better using the area (or number of patches) of shallow-slow water habitat as its main predictor variable rather than total discharge.
The ecological data used in this study are also of course subject to uncertainty. The estimated abundances of golden perch eggs and larvae that are calculated from the survey data have been converted to binary (spawning detected/not detected) data for the analysis presented here, which would reduce the impacts of measurement uncertainty. However, the three drift nets at a site are sampling only a tiny proportion of the cross-sectional area of the river channel, and it is likely that if there is a small number of eggs drifting downstream, then sometimes we will not detect them. Such measurement uncertainty is carried through to the statistical analysis of data (although this is seldom explicitly recognized in analyses of ecological data; Webb and King, 2009), introducing greater uncertainty into the flow-spawning relationships described by the Bayesian analysis. This uncertainty may also have acted to prevent us from detecting a difference in the predictive power of velocity-based versus discharged-based analyses.
Ecological interpretation
For three out of the four sites in the analyses (Pyke Road being the exception), spawning of golden perch was much more likely once reach-averaged flow velocity exceeded ∼0.3 m s−1/∼7000 ML d−1, but only when water temperatures were above ∼18.6°C. These findings have an intuitive evolutionary interpretation. At increased temperatures, eggs and larvae will develop more quickly (Todd et al., 2005). As described above, golden perch is a pelagophilic species, where eggs are shed in the upper water column and drift long distances downstream on flowing waters (Koster et al., 2017; Stuart & Sharpe, 2020); at lower discharges (and hence velocities and turbulences) eggs are more likely to settle on the riverbed and die. Velocity, and related turbulence, will be more important for keeping eggs suspended in the water column than discharge per se because in a different, larger river channel, the same discharge may not generate sufficient velocities to keep eggs entrained within the water column. Moreover, golden perch possess a lateral line system that allows them to detect water flow or movement (Bleckmann & Zelick, 2009). Fish will thus be able to detect changes in velocity in the water column, whereas they cannot directly detect changes in discharge. Together, increased flows and temperatures increase the likelihood of eggs and larvae surviving through these most vulnerable of life history stages. In the lower Goulburn River, with most of the spawning taking place at the most downstream sites, higher velocities will mean that most eggs are exported from the system into the River Murray. This supposition is backed up by our fish assemblage monitoring data (e.g. Webb et al., 2019a), where we have only occasionally detected young-of-year golden perch in the Goulburn River, and then most of these fish have been shown to be hatchery reared.
Our analysis found weak evidence that flows in the 5 weeks prior to the spawning survey affect the probability of spawning. However, smaller flow increases in the weeks prior to spawning have been linked to improved spawning success (Cockayne et al., 2013). Future analyses of the data could consider flows over longer periods prior to spawning, or potentially separated from spawning by some duration (e.g. flows between 2 months and 1 month prior to spawning). They could also consider other descriptors of the prior flows than the average discharge we used here. However, it must be remembered that the data set upon which our analyses are based is still quite small (one river for 8 years) and so it is difficult to discern more subtle drivers of spawning, such as may be the case for antecedent flows.
Implications for ecohydraulics research
The velocity-based analysis of spawning probability presented here is based on a 1-dimensional hydraulic interpretation of the river (our models are 2-dimensional, but we used the reach-averaged velocity). Two-dimensional and even 3-dimensional hydraulic models of river flows and other aquatic environments are becoming much more common as computational power and bathymetric data improve with advances in computers and remote sensing. Our focus here, however, was on relative simplicity of modelling for management purposes. Greater complexity in hydraulic modelling may increase the precision of flow-ecology relationships, but this complexity may act to reduce the use of modelling in management.
As noted above, it is likely that golden perch are responding to hydraulic forces at particular points in the river. However, given that we do not physically observe the occurrence of spawning, and indeed at least some of the spawning will take place upstream of the modelled reaches where the eggs/larvae are collected, we’re unable to tie the ecological responses to such fine-scale hydraulic characteristics of the river. This raises a conundrum for the future of ecohydraulics research such as presented here. If the ecological data cannot be collected at a scale commensurate to that of the hydraulic models available, is it worth the effort to develop anything other than 1-dimensional models for describing hydraulics-ecology relationships (Nestler et al., 2016)?
Other ecological data may be more suited to 2D and 3D hydraulic models. Instream and littoral vegetation, for example, is often sampled at specific points in the channel. The hydraulic environment for these organisms could be well characterized using higher-dimensional models. Drone-based remote sensing is now also offering the opportunity to do complete censuses of bank vegetation and geomorphic changes, rather than the limited number of points that can be collected using traditional ground-based sampling (Vietz et al., 2021). The challenge for the discipline in this case is how to analyze such a huge number of data points using statistical methods that were originally developed for limited samples.
Using hydraulic models for environmental water management?
The results of this study did not show any improvement in our ability to predict golden perch spawning using a hydraulics-based view of flow. However, we have argued that hydraulics offer a more mechanistic interpretation of flow-ecology responses and also that in geomorphically complex rivers, the advantages of a hydraulics-based approach should be stronger. We seldom see hydraulic interpretations of ecological responses to environmental flows. The most obvious reason for this is the difficulty (and hence expense) of taking a hydraulics-based approach, and the difficulty of appropriately characterizing all the diversity in hydraulics that will matter to aquatic flora and fauna. In this project, we assessed only one hydraulic characteristic—reach-averaged velocity—of the many potential descriptors of hydraulics.
In the LTIM Project, we had the relative luxury of being able to establish hydraulic models with the assessment of multiple ecological responses in mind (Webb et al., 2018b). In addition to fish spawning, the models have been used to assess impacts of changing flows on bank condition and vegetation (Webb et al., 2019a). If one were developing hydraulic models for the analysis of a single ecological response, it would be harder to argue their value to funders. The models used in this study were developed in 2014 but have recently been re-assessed against field data for their ability to predict shallow, slow-flowing areas (W.M. Bovill, University of Melbourne, unpubl. Data). They are still performing well in this regard, but new models will be needed at some point in the future. By that stage, we hope to have been able to perform more, and more detailed, hydraulics-based analyses for multiple ecological responses, demonstrating the value of hydraulic models.
Environmental water management is also entering a new era, with finer-scale decisions required at greater frequency to gain more ecological benefit from less water. This active management of environmental water (sensu Horne et al., 2018a; Horne et al., 2018b) puts the onus on local-scale decisions to gain the most benefit possible from environmental water allocations. Improving flow outcomes through adaptive management (Webb et al., 2017b; Webb et al., 2018a; Watts et al., 2020) will rely upon an improved ability to predict ecological responses to environmental flow events. In this new management landscape, hydraulic models would offer managers a greater ability to optimize environmental water outcomes if they can out-perform traditional hydrology-based models.
Hydraulic analyses will also offer an increased ability to combine the results from multiple monitoring locations to improve our ability to predict responses at larger scales and in areas that are not monitored. Limited resources for monitoring mean that it is only ever possible to monitor a few sites out of many on a river, or a few rivers out of many in a basin. The LTIM Project and MER Program are monitoring just seven selected areas across the one million square kilometers of the Murray-Darling Basin. From these results researchers must reach conclusions about the benefits of environmental flows at the whole of basin scale (Gawne et al., 2020). Within these projects, golden perch are being monitored (with greater and lesser levels of intensity) in the lower Murray River, the lower Goulburn River, the Edward-Wakool system, the Lachlan River, and the Murrumbidgee River. An ability to combine results from these different areas to create a more general predictive model would greatly enhance our ability to predict responses for those many areas of the basin where golden perch are found, but which are not being monitored. It could also potentially help us to tease apart the individual influences of different hydraulic parameters that are highly correlated in the Goulburn River (e.g. velocity and depth). Such a multi-river view would also help to improve our understanding of this species’ populations in different rivers and regions as being parts of a broader meta-population throughout the southern Murray-Darling Basin (Beheregaray et al., 2017). However, hydrology-based analyses will probably never be able to be effectively combined across locations. The systems listed above all have very different channel sizes, with the lower Murray carrying two orders of magnitude more water than the Edward-Wakool. By translating the very different hydrologies of these rivers into hydraulics-based characterisations of flow, we have a better chance of being able to synthesize ecological response data across rivers of different size (Webb et al., 2017a). Tantalizingly, monitoring from the lower Murray River, suggests that reach-averaged discharge velocities of ∼0.3 m s−1, similar to those seen in this study, are associated with golden perch spawning (Q. Ye, SARDI Aquatic Sciences, pers. comm.). Future collaborative work among the different management agencies could focus on achieving similar flow velocities in the Edward-Wakool, Lachlan, and Murrumbidgee rivers to test the generality of this finding.
Beyond this study, hydraulic models have shown good performance to analyze other ecological responses to environmental flows in the Goulburn River. We have recently used the hydraulic models in the Goulburn River to identify discharge rates that maximize the extent of shallow inundation of the low-level benches (Webb et al., 2019a). We are hoping to be able to achieve this type of discharge during the summer release of water being traded downstream for agriculture as inter-valley transfers. Inter-valley transfers lead to higher than normal summer flows, and cause severe impacts to banks and vegetation (Vietz et al., 2019; Webb et al., 2019a). Our ambition is to use these flows to inundate benches and thereby create opportunities for vegetation recruitment and provide habitat for small-bodied fish, partially ameliorating the negative effects of these important downstream transfers of water.
This final example provides further evidence of how hydraulic models may be able to be used to inform more ecologically realistic and relevant management decisions for flow management. With environmental water being a scarce commodity, it is incumbent upon water managers to use it as effectively as possible. Hydraulic models provide an opportunity to do this, improving environmental, economic, and social outcomes for all stakeholders.
Data availability statement
The raw data supporting the conclusion of this article will be made available by the authors, without undue reservation.
Author contributions
JAW leads the overall monitoring program and led the writing of the manuscript. DG led the data analysis, WK leads the fish monitoring, CL-A and GV developed and implemented the hydraulic models. All authors contributed to the final manuscript.
Funding
The Goulburn River STIM, LTIM and MER projects were funded by the Commonwealth Environmental Water Office, with co-contributions from Victorian Environmental Water Holder, and Victorian Department of Environment, Land, Water and Planning.
Acknowledgments
We acknowledge the contributions of other members of the Goulburn monitoring teams, as well as all stakeholders in the lower Goulburn River Selected Area. David Dawson (Arthur Rylah Institute) collected many of the spawning data analyzed in this paper, Dion Iervasi (Austral Research and Consulting) collected bathymetry data for the development of the 2D models, Kathryn Russell (Streamology) provided hydraulic model development and outputs, and Chandra Jayasuriya (University of Melbourne) produced the original map from which Figure 1 was developed.
Conflict of interest
Authors CL-A and GV were employed by the Streamology Pty. Ltd.
The remaining authors declare that the research was conducted in the absence of any commercial or financial relationships that could be construed as a potential conflict of interest.
Publisher’s note
All claims expressed in this article are solely those of the authors and do not necessarily represent those of their affiliated organizations, or those of the publisher, the editors and the reviewers. Any product that may be evaluated in this article, or claim that may be made by its manufacturer, is not guaranteed or endorsed by the publisher.
Supplementary Material
The Supplementary Material for this article can be found online at: https://www.frontiersin.org/articles/10.3389/fenvs.2022.882495/full#supplementary-material
References
Arthington, A. H., Mackay, S. J., James, C. S., Rolls, R. J., Sternberg, D., Barnes, A., et al. (2012). Ecological-limits-of-hydrologic-alteration: A test of the ELOHA framework in south-east queensland. Canberra Waterlines Report Series, National Water Commission.
Arthington, A. H., Bhaduri, A., Bunn, S. E., Jackson, S. E., Tharme, R. E., Tickner, D., et al. (2018). The Brisbane declaration and global action agenda on environmental flows (2018). Front. Environ. Sci. 6, 45. doi:10.3389/fenvs.2018.00045
Beheregaray, L. B., Pfeiffer, L. V., Attard, C. R., Sandoval-Castillo, J., Domingos, F. M., Faulks, L. K., et al. (2017). Genome-wide data delimits multiple climate-determined species ranges in a widespread Australian fish, the golden perch (Macquaria ambigua). Mol. Phylogenetics Evol. 111, 65–75. doi:10.1016/j.ympev.2017.03.021
Bice, C. M., Gibbs, M. S., Kilsby, N. N., Mallen-Cooper, M., and Zampatti, B. P. (2017). Putting the “river” back into the lower River Murray: Quantifying the hydraulic impact of river regulation to guide ecological restoration. Trans. R. Soc. S. Aust. 141 (2), 108–131. doi:10.1080/03721426.2017.1374909
Bleckmann, H., and Zelick, R. (2009). Lateral line system of fish. Integr. Zool. 4 (1), 13–25. doi:10.1111/j.1749-4877.2008.00131.x
Carpenter, B., Gelman, A., Hoffman, M. D., Lee, D., Goodrich, B., Betancourt, M., et al. (2017). Stan: A probabilistic programming language. J. Stat. Softw. 76 (1). doi:10.18637/jss.v076.i01
Cockayne, B., McDougall, A., Espinoza, T., Burndred, K., Thrupp, C., Broadfoot, C., et al. (2013). Riverine flow and spawning requirements of Macquaria ambigua oriens: Implications for conservation and management. Mar. Freshw. Res. 64 (1), 42–53. doi:10.1071/mf12047
EarthTech (2003). Thomson River environmental flow requirements & options to manage flow stress: report to west gippsland catchment management authority, Dept. of Sustainability and Environment, Melbourne Water Corporation and Southern Rural Water. Earth Tech Engineering, Melbourne, Australia.
Gawne, B., Hale, J., Stewardson, M. J., Webb, J. A., Ryder, D. S., Brooks, S. S., et al. (2020). Monitoring of environmental flow outcomes in a large river basin: The Commonwealth Environmental Water Holder's long-term intervention in the Murray–Darling Basin, Australia. River Res. Appl. 36 (4), 630–644. doi:10.1002/rra.3504
Gelman, A., Carlin, J. B., Stern, H. S., Dunson, D. B., Vehtari, A., and Rubin, D. B. (2013). Bayesian data analysis. Third Edition, CRC Press. Boca Raton, FA, USA.
Gelman, A., and Hill, J. (2007). Data analysis using regression and multilevel/hierarchical models. Cambridge University Press. Cambridge, England.
Graf, W. L. (2006). Downstream hydrologic and geomorphic effects of large dams on American rivers. Geomorphology, 79 (3), 336–360. doi:10.1016/j.geomorph.2006.06.022
Harby, A., Martinez-Capel, F., and Lamouroux, N. (2017). From microhabitat ecohydraulics to an improved management of river catchments: Bridging the gap between scales. River Res. Appl. 33, 189–191. doi:10.1002/rra.3114
Hart, B. T. (2016). The Australian Murray-Darling Basin plan: Factors leading to its successful development. Ecohydrol. Hydrobiology 16 (4), 229–241. doi:10.1016/j.ecohyd.2016.09.002
A. C. Horne, J. A. Webb, M. J. Stewardson, B. Richter, and M. Acreman (2017). Water for the environment: From policy and science to implementation and management. Elsevier. Amsterdam, Netherlands.
Horne, A. C., Kaur, S., Szemis, J. M., Costa, A. M., Nathan, R., Webb, J. A., et al. (2018a). Active management of environmental water to improve ecological outcomes. J. Water Resour. Plan. Manag. 144 (12), 04018079. doi:10.1061/(asce)wr.1943-5452.0000991
Horne, A. C., Szemis, J. M., Webb, J. A., Kaur, S., Stewardson, M. J., Bond, N., et al. (2018b). Informing environmental water management decisions: Using conditional probability networks to address the information needs of planning and implementation cycles. Environ. Manag. 61 (3), 347–357. doi:10.1007/s00267-017-0874-8
Horne, A., Webb, A., Rumpff, L., Mussehl, M., Fowler, K., and John, A. (2020). Kaiela (lower Goulburn River) environmental flows study. Melbourne: The Univeristy of Melbourn
Humphries, P., Cook, R. A., Richardson, A. J., and Serafini, L. G. (2006). Creating a disturbance: Manipulating slackwaters in a lowland river. River Res. Appl., 22(5), 525–542. doi:10.1002/Rra.920
Humphries, P., and Walker, K. (2013). Ecology of Australian freshwater fishes. CSIRO Publishing. Clayton, Australia.
Jorda-Capdevila, D., and Rodríguez-Labajos, B. (2017). Socioeconomic value(s) of restoring environmental flows: Systematic review and guidance for assessment. River Res. Appl. 33 (3), 305–320. doi:10.1002/rra.3074
King, A. J., Tonkin, Z., and Mahoney, J. (2009). Environmental flow enhances native fish spawning and recruitment in the Murray River, Australia. River Res. Appl. 25 (10), 1205–1218. doi:10.1002/rra.1209
King, A. J., Gwinn, D. C., Tonkin, Z., Mahoney, J., Raymond, S., and Beesley, L. (2016). Using abiotic drivers of fish spawning to inform environmental flow management. J. Appl. Ecol. 53 (1), 34–43. doi:10.1111/1365-2664.12542
Koster, W. M., Dawson, D. R., O'Mahony, D. J., Moloney, P. D., and Crook, D. A. (2014). Timing, frequency and environmental conditions associated with mainstem–tributary movement by a lowland river fish, golden perch (Macquaria ambigua). PLoS One 9 (512), e96044. doi:10.1371/journal.pone.0096044
Koster, W., Dawson, D., Liu, C., Moloney, P., Crook, D., and Thomson, J. (2017). Influence of streamflow on spawning-related movements of golden perch Macquaria ambigua in south-eastern Australia. J. Fish. Biol. 90 (1), 93–108. doi:10.1111/jfb.13160
Lane, S., Bradbrook, K., Richards, K., Biron, P., and Roy, A. (1999). The application of computational fluid dynamics to natural river channels: Three-dimensional versus two-dimensional approaches. Geomorphology 29 (1-2), 1–20. doi:10.1016/s0169-555x(99)00003-3
Mallen-Cooper, M., and Zampatti, B. P. (2018). History, hydrology and hydraulics: Rethinking the ecological management of large rivers. Ecohydrology 11 (5), e1965. doi:10.1002/eco.1965
McManamay, R. A., Orth, D. J., Dolloff, C. A., and Mathews, D. C. (2013). Application of the ELOHA framework to regulated rivers in the upper Tennessee river basin: A case study. Environ. Manag. 51 (6), 1210–1235. doi:10.1007/s00267-013-0055-3
Nestler, J. M., Stewardson, M. J., Gilvear, D., Webb, J. A., and Smith, D. L. (2016). Ecohydraulics exemplifies the emerging "paradigm of the interdisciplines. J. Ecohydraulics 1 (1-2), 5–15. doi:10.1080/24705357.2016.1229142
Parasiewicz, P. (2007). The MesoHABSIM model revisited. River Res. Appl. 23 (8), 893–903. doi:10.1002/rra.1045
Poff, N. L., Allan, J. D., Bain, M. B., Karr, J. R., Prestegaard, K. L., Richter, B. D., et al. (1997). The natural flow regime. Bioscience 47 (11), 769–784. doi:10.2307/1313099
Poff, N. L., Allan, J. D., Palmer, M. A., Hart, D. D., Richter, B. D., Arthington, A. H., et al. (2003). river flows and water wars: Emerging science for environmental decision making. Front. Ecol. Environ. 1 (6), 298–306. doi:10.1890/1540-9295(2003)001[0298:rfawwe]2.0.co;2
Poff, N. L., Brown, C. M., Grantham, T. E., Matthews, J. H., Palmer, M. A., Spence, C. M., et al. (2016). Sustainable water management under future uncertainty with eco-engineering decision scaling. Nat. Clim. Chang. 6 (1), 25–34. doi:10.1038/nclimate2765
Poff, N. L., Tharme, R. E., and Arthington, A. H. (2017). “Evolution of environmental flows assessment science, principles, and methodologies,” in Water for the environment: From policy and science to implementation and management. Editors A. C. Horne, J. A. Webb, M. J. Stewardson, B. D. Richter, and M. Acreman (Elsevier), Amsterdam, Netherlands, 207–241.
Stan Development Team (2018). RStan: the R interface to Stan. Available at: http://mc-stan.org.
Stuart, I. G., and Sharpe, C. P. (2020). Riverine spawning, long distance larval drift, and floodplain recruitment of a pelagophilic fish: A case study of golden perch (Macquaria ambigua) in the arid darling river, Australia. Aquat. Conserv. 30 (4), 675–690. doi:10.1002/aqc.3311
Stuart, I. G., and Sharpe, C. P. (2021). Ecohydraulic model for designing environmental flows supports recovery of imperilled Murray cod (Maccullochella peelii) in the Lower Darling–Baaka River following catastrophic fish kills. Mar. Freshw. Res. 73, 247–258. doi:10.1071/mf20377
Todd, C. R., Ryan, T., Nicol, S. J., and Bearlin, A. R. (2005). The impact of cold water releases on the critical period of post-spawning survival and its implications for Murray cod (Maccullochella peelii peelii): A case study of the mitta mitta river, southeastern Australia. River Res. Appl. 21 (9), 1035–1052. doi:10.1002/rra.873
Turner, M., and Stewardson, M. (2014). Hydrologic indicators of hydraulic conditions that drive flow–biota relationships. Hydrological Sci. J. 59 (3-4), 659–672. doi:10.1080/02626667.2014.896997
Vietz, G. J., Sammonds, M. J., and Stewardson, M. J. (2013). Impacts of flow regulation on slackwaters in river channels. Water Resour. Res. 49 (4), 1797–1811. doi:10.1002/wrcr.20094
Vietz, G., Donges, M., Houghton, J., Mole, B., Morris, K., and Clarke, S. (2019). Goulburn riverbanks and bank vegetation: Influence of intervalley transfers (IVT). Report by Streamology for the Goulburn broken catchment management authority and victorian environmental water holder. Streamology Pty. Ltd. Bright, Australia.
Vietz, G., Sutton, N., Houghton, J., Gower, T., and Lauchlan-Arrowsmith, C. (2021). Assessing waterways from the sky: A new era in monitoring using drones. Proceedings of the 10th Australian Stream Management Conference 2020. Kingscliff, Australia, August 2021.
Vorosmarty, C. J., McIntyre, P. B., Gessner, M. O., Dudgeon, D., Prusevich, A., Green, P., et al. (2010). Global threats to human water security and river biodiversity. Nature 467 (7315), 555–561. doi:10.1038/nature09440
Watts, R. J., Dyer, F., Frazier, P., Gawne, B., Marsh, P., Ryder, D. S., et al. (2020). Learning from concurrent adaptive management in multiple catchments within a large environmental flows program in Australia. River Res. Appl. 36 (4), 668–680. doi:10.1002/rra.3620
Webb, J. A., and King, E. L. (2009). A Bayesian hierarchical trend analysis finds strong evidence for large-scale temporal declines in stream ecological condition around Melbourne, Australia. Ecography 32, 215–225. doi:10.1111/j.1600-0587.2008.05686.x
Webb, J. A., Stewardson, M. J., and Koster, W. M. (2010). Detecting ecological responses to flow variation using Bayesian hierarchical models. Freshw. Biol. 55, 108–126. doi:10.1111/j.1365-2427.2009.02205.x
Webb, A., Vietz, G., Windecker, S., Hladyz, S., Thompson, R., Koster, W., et al. (2015). Monitoring and reporting on the ecological outcomes of commonwealth environmental water delivered in the lower Goulburn River and Broken Creek in 2013/14. Melbourne: Report prepared for the Commonwealth Environmental Water Office, University of Melbourne.
Webb, A., Casanelia, S., Earl, G., Grace, M., King, E., Koster, W., et al. (2016). Commonwealth environmental water Office long term intervention monitoring project: Goulburn River Selected area evaluation report 2014-15. Available at: http://www.environment.gov.au/water/cewo/publications/goulburn-ltim-report-2015-16.
Webb, J. A., Arthington, A. H., and Olden, J. D. (2017a). “Models of ecological responses to flow regime change to inform environmental flow assessments,” in Water for the environment: From policy and science to implementation and management. Editors A. C. Horne, J. A. Webb, M. J. Stewardson, B. Richter, and M. Acreman (Elsevier), Amsterdam, Netherlands 287–316.
Webb, J. A., Watts, R. J., Allan, C., and Warner, A. T. (2017b). “Principles for monitoring, evaluation and adaptive management of environmental flows,” in Water for the environment: From policy and science to implementation and management. Editors A. C. Horne, J. A. Webb, M. J. Stewardson, B. D. Richter, and M. Acreman (Elsevier), Amsterdam, Netherlands, 599–623.
Webb, A., Sharpe, A., Koster, W., Pettigrove, V., Grace, M., Vietz, G., et al. (2018a). Long-term intervention monitoring program for the lower Goulburn River: Final monitoring and evaluation plan. Melbourne: Report prepared forthe Commonwealth Environmental Water Office, University of Melbourne.
Webb, J. A., Watts, R. J., Allan, C., and Conallin, J. C. (2018b). Adaptive management of environmental flows. Environ. Manag. 61 (3), 339–346. doi:10.1007/s00267-017-0981-6
Webb, A., Guo, D., King, E., Treadwell, S., Baker, B., Casanelia, S., et al. (2019a). Commonwealth environmental water Office long term intervention monitoring project Goulburn River selected area scientific report 2017–18. Melbourne: University of Melbourne.
Webb, A., Treadwell, S., Lakhanpal, G., Baker, B., Casanelia, S., Grace, M., et al. (2019b). Lower Goulburn River selected area monitoring, evaluation and research plan (2019-2022). Prepared for the Commonwealth Environmental Water Office. Melbourne: University of Melbourne. Available at: https://www.awe.gov.au/water/cewo/publications/mer-plan-goulburn-2019.
Wheaton, J. M., Bouwes, N., Mchugh, P., Saunders, C., Bangen, S., Bailey, P., et al. (2018). Upscaling site-scale ecohydraulic models to inform salmonid population-level life cycle modeling and restoration actions–Lessons from the Columbia River Basin. Earth Surf. Process. Landf. 43 (1), 21–44. doi:10.1002/esp.4137
Keywords: hydraulics, flow-ecology relationship, environmental flows, golden perch, murray-darling basin. (Min.5-Max. 8)
Citation: Webb JA, Guo D, Koster WM, Lauchlan-Arrowsmith C and Vietz GJ (2022) Can hydraulic measures of river conditions improve our ability to predict ecological responses to changing flows? Flow velocity and spawning of an iconic native Australian fish. Front. Environ. Sci. 10:882495. doi: 10.3389/fenvs.2022.882495
Received: 23 February 2022; Accepted: 31 October 2022;
Published: 30 November 2022.
Edited by:
Rui Pedro Rivaes, University of Lisbon, PortugalReviewed by:
Nitin Kaushal, World Wide Fund for Nature, IndiaAbul Baki, Clarkson University, United States
Copyright © 2022 Webb, Guo, Koster, Lauchlan-Arrowsmith and Vietz. This is an open-access article distributed under the terms of the Creative Commons Attribution License (CC BY). The use, distribution or reproduction in other forums is permitted, provided the original author(s) and the copyright owner(s) are credited and that the original publication in this journal is cited, in accordance with accepted academic practice. No use, distribution or reproduction is permitted which does not comply with these terms.
*Correspondence: J. Angus Webb, YW5ndXMud2ViYkB1bmltZWxiLmVkdS5hdQ==