- 1Envix-Lab, Department of Biosciences and Territory, Molise University, Isernia, Italy
- 2LaCEMod, Department of Life, Health and Environmental Sciences, Environmental Sciences Sect., University of L'Aquila, L'Aquila, Italy
- 3Vegetation Ecology Laboratory, Department of Sciences, Roma Tre University, Roma, Italy
Invasive alien plants (IAPs) are increasingly threatening biodiversity worldwide; thus, early detection and monitoring tools are needed. Here, we explored the potential of unmanned aerial vehicle (UAV) images in providing intermediate reference data which are able to link IAP field occurrence and satellite information. Specifically, we used very high spatial resolution (VHR) UAV maps of A. saligna as calibration data for satellite-based predictions of its spread in the Mediterranean coastal dunes. Based on two satellite platforms (PlanetScope and Sentinel-2), we developed and tested a dedicated procedure to predict A. saligna spread organized in four steps: 1) setting of calibration data for satellite-based predictions, by aggregating UAV-based VHR IAP maps to satellite spatial resolution (3 and 10 m); 2) selection of monthly multispectral (blue, green, red, and near infra-red bands) cloud-free images for both satellite platforms; 3) calculation of monthly spectral variables depicting leaf and plant characteristics, canopy biomass, soil features, surface water and hue, intensity, and saturation values; 4) prediction of A. saligna distribution and identification of the most important spectral variables discriminating IAP occurrence using a fandom forest (RF) model. RF models calibrated for both satellite platforms showed high predictive performances (R2 > 0.6; RMSE <0.008), with accurate spatially explicit predictions of the invaded areas. While Sentinel-2 performed slightly better, the PlanetScope-based model effectively delineated invaded area edges and small patches. The summer leaf chlorophyll content followed by soil spectral variables was regarded as the most important variables discriminating A. saligna patches from native vegetation. Such variables depicted the characteristic IAP phenology and typically altered leaf litter and soil organic matter of invaded patches. Overall, we presented new evidence of the importance of VHR UAV data to fill the gap between field observation of A. saligna and satellite data, offering new tools for detecting and monitoring non-native tree spread in a cost-effective and timely manner.
1 Introduction
Biological invasions are among the major threats impinging on biodiversity across the world (Simberloff et al., 2013; Vilà and Hulme, 2017; Pyšek et al., 2020). Invasive alien species (IAS), i.e., non-native species introduced by humans into a natural system outside of their native range, are causing intense direct impact and indirect impact on invaded ecosystems, compromising their ecological functions and services (Bartz and Kowarik, 2019). IAS invasions may alter community composition and species assemblage strategies (e.g., photosynthetic rate and standing and dead biomass; Linders et al., 2019) and degrade soil properties (e.g., nutrient content and water surface; Castro-Díez al., 2019).
Among the most invasive plants impinging on coastal ecosystems worldwide, Acacia saligna (Labill.) H. Wendl (Starfinger and Schrader, 2021) is one of the most aggressive plants, and it was recently included in the European Regulation on Invasive Alien Species (EU1143/2014; hereafter, IAS Regulation). A. saligna is a small evergreen tree, native of Western Australia (Maslin, 1974), with fast-growing and intense vegetative and sexual propagation (Witkowski, 1991). Being introduced as fodder (Asefa and Tamir, 2006; George et al., 2007), windbreak and dune stabilization (Bar et al., 2004), and for ornamental purposes (Donaldson et al., 2014), it has become invasive in coastal areas across the world (e.g., South Africa, North Africa, Horn of Africa, Chile, and the Mediterranean; Thompson et al., 2015; Lozano et al., 2020). Several negative effects of A. saligna invasion on natural ecosystems were reported, for e.g., the alteration of biodiversity values, the decline of focal species, and the drastic change of vegetation structure toward dense monospecific A. saligna woodlands (Le Maitre et al., 2011; Del Vecchio et al., 2013; Tozzi et al., 2021). A. saligna also modifies soil nitrogen and organic matter content (Yelenik et al., 2004; El-Gawad and El-Amier, 2015), altering soil microbial communities (Crisóstomo et al., 2013). Furthermore, invaded areas tend to present poorer aesthetic and recreational landscape values than natural ones (Lehrer et al., 2011).
The growing pressure exerted by IAS across different ecosystems worldwide urges the scientific community and civil society to identify adequate monitoring and management strategies (Brundu et al., 2018). As the Convention on Biological Diversity (CBD; https://www.cbd.int/) claims for a global strategy against IAS by 2030, the European Regulation on Invasive Alien Species (EU1143/2014) provides clear guidelines to prevent, minimize, and mitigate the occurrence and effects of alien species on natural ecosystems (Genovesi et al., 2015; Branquart et al., 2016).
The analysis of invasion processes was traditionally based on field campaigns, often combined with visual interpretation of aerial photos; both approaches were widely recognized as expensive, time-consuming, and limited to depicting IAS occurrences on remote and inaccessible areas (Del Vecchio et al., 2013; Stanisci et al., 2014; Royimani et al., 2019). Indeed, field campaigns for IAS spread analysis require an accurate work plan to be carried out in a specific sampling period, often in limited areas (Bolch et al., 2020). In addition, the visual interpretation of aerial photos is very time-consuming, even for skilled photo-interpreters (Bolch et al., 2020). During the last decade, there has been increasing evidence of the potential of remote sensing (RS) for IAS early detection, monitoring, and mapping in a cost-effective, spatially contiguous, and timely manner (Huang and Asner, 2009; Royimani et al., 2019). Indeed, the distribution of different IAS can be detected by using expensive very high-resolution (spatial and spectral) RS images (e.g., airborne and satellite platforms with multispectral or hyperspectral sensors; Underwood et al., 2003; Paz-Kagan et al., 2017; Niphadkar et al., 2017) or combining free coarser RS images and field-collected occurrences as calibration data (Zhou et al., 2018; Kattenborn et al., 2019).
However, the combination of standard satellite RS images and field-collected occurrences for IAS detection has different shortcomings that should be overcome (Bradley 2014; Pettorelli et al., 2014) as: 1) satellite images regularly covering the overall Earth surface using standardized multispectral sensors (fixed spatial resolution and zenith angle) could weakly describe some important ecological parameters (e.g., IAS flower color and the blooming period or altered soils on invaded areas) which are essential for IAS detection (Müllerová et al., 2017); 2) the acquisition of high quality IAS occurrence data in the field may be hampered by local conditions, such as a dense canopy cover, trouble in reaching remote invaded areas (Kaartinen et al., 2015; McGaughey et al., 2017), or the complexity of the invaded ecosystem mosaic (Bradley 2009; Hawthorne et al., 2017); 3) building a reliable database of field IAS occurrences may require a great effort to collect spatially accurate records using Real-Time Kinematics Global Navigation Satellite System (RTK GNSS) devices (Piiroinen et al., 2018; Dao et al., 2021).
A valid alternative to increase the spectral information and the amount of accurate IAS occurrence data at a local scale is offered by unmanned aerial vehicles (UAVs). Indeed, the use of UAVs allows the collection of highly customized data as the operator can easily set several parameters that are useful to detect alien species (e.g., type of sensor, angle of view, spatial resolution, time, and frequency of acquisition), which are commonly standardized on most satellite platforms (Kattenborn et al., 2019; Alvarez-Vanhard et al., 2021). In addition, since UAV images register environmental complexity as a continuous surface (Kattenborn et al., 2019; Riihimäki et al., 2019) at very high spatial resolution (VHR), variations of spectral values caused by IAS can be detected in a smoothed way (Anderson 2018; Leitão et al., 2018), helping to fill the gap between field observations and satellite data. On the other side, technical constraints of UAVs (e.g., battery capability and surveying restrictions) often prevent the use of this technology to describe ecosystem patterns on a large scale. However, the ability to acquire highly customized data makes UAVs an interesting option to provide accurate maps of local IAS occurrence (e.g., Wijesingha et al., 2020; Marzialetti et al., 2021), supporting satellite detection at wider scales. The contribution of VHR images for aiding IAS satellite detection deserves to be further explored (Elkind et al., 2019; Alvarez-Vanhard et al., 2021).
The present work sets out to investigate the potential of UAV data depicting the smooth occurrence of A. saligna at a local scale to support its satellite-based detection at a wide scale in complex and dynamic environments such as Mediterranean coastal dunes. Specifically, we used VHR (0.05 m) multispectral (blue, green, red, and near infrared) UAV images collected during the A. saligna blooming period to provide occurrence data needed to predict the spread of this IAS using two satellite platforms (free for research purposes) with different spatial resolutions (e.g., PlanetScope: 3 m and Sentinel-2: 10 m). Moreover, we also compared IAS detection performance achieved by the two satellite platforms, highlighting their differences in mapping invaded coastal dune environments.
2 Materials and methods
2.1 Study area and target species
The study area includes a representative tract of recent (i.e., Holocenic) dunes along the Adriatic coast of Central Italy, characterized by a Mediterranean climate (Figure 1A; Acosta et al., 2009; Carranza et al., 2008). We selected two areas: 1) an invaded one of approximately 11 ha as the UAV flight calibration area (Figure 1B, red polygon, Marzialetti et al., 2021) and 2) a wider one at high invasion risk (Marzialetti et al., 2019) of approximately 70 ha as the prediction area (Figure 1B, green polygon). As in other coastal areas of the Adriatic coast, the analyzed dunes are low (less than 8–10 m) and occupy a narrow strip parallel to the seashore. Under natural conditions, the psammophilous vegetation mosaic follows a sea–inland gradient ranging from the annual pioneer communities on the upper beach to the Mediterranean maquis and Pinus spp. woods in the inner fore dune sectors (Acosta et al., 2003; Carranza et al., 2008; Bazzichetto et al., 2016). This zonation promotes the development of highly specialized biodiversity, which shares few species with other terrestrial communities (Drius et al., 2016; Marzialetti et al., 2020), and its integrity assures manifold ecosystem services (Drius et al., 2013). This area is impinged by several human-related disturbances as most of the Mediterranean coastal landscapes: agricultural pressure (Malavasi et al., 2013), tourism and urban expansion (Carranza et al., 2018), beach pollution (Di Febbraro et al., 2021), and alien species invasions (Del Vecchio et al., 2013; Marzialetti et al., 2019). The analyzed area is inside a special area of conservation (SAC, Habitat Directive 92/43/EEC; Foce Trigno—Marina di Petacciato IT7228221) and is a node of the Long-Term Ecological Research Network (LTER, http://www.lter-europe.net/; Stanisci et al., 2014; Drius et al., 2013), which makes it an excellent testing ground to develop methodologies which are able to evaluate and monitor invasion processes.
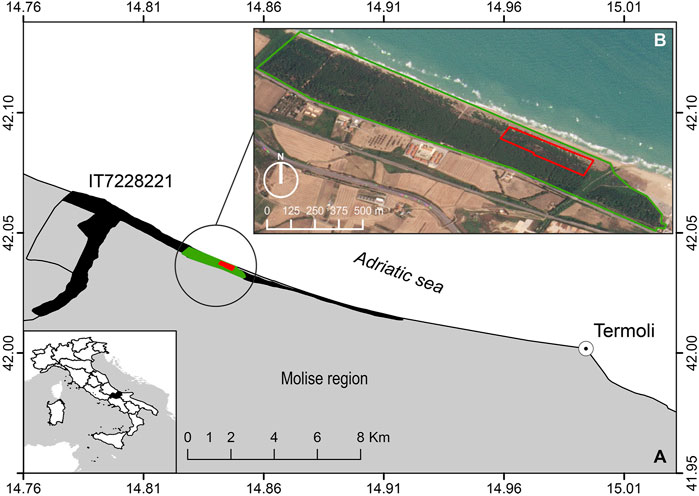
FIGURE 1. (A) Study area reporting the UAV flight calibration area in red and the prediction area in green (coordinate system in WGS 84 epsg: 4326). SAC and LTER site shape is reported in black (Foce Trigno–Marina di Petacciato—IT7228221, https://deims.org/1835cda2-b56d-400a-b413-ab5c74086dc5). (B) Enlargement of the study area projected on the PlanetScope image of 20 July 2021. Red polygon represents UAV flight coverage used for preparing calibration data, and the green polygon represents the satellite prediction area.
2.2 Data collection and analysis
The proposed framework for detecting and predicting A. saligna distribution was structured following four steps that are schematically reported in Figure 2: (A) UAV-based A. saligna maps and calibration data, (B) satellite imagery selection, (C) remote sensing variable calculation, and (D) A. saligna satellite-based predictions.
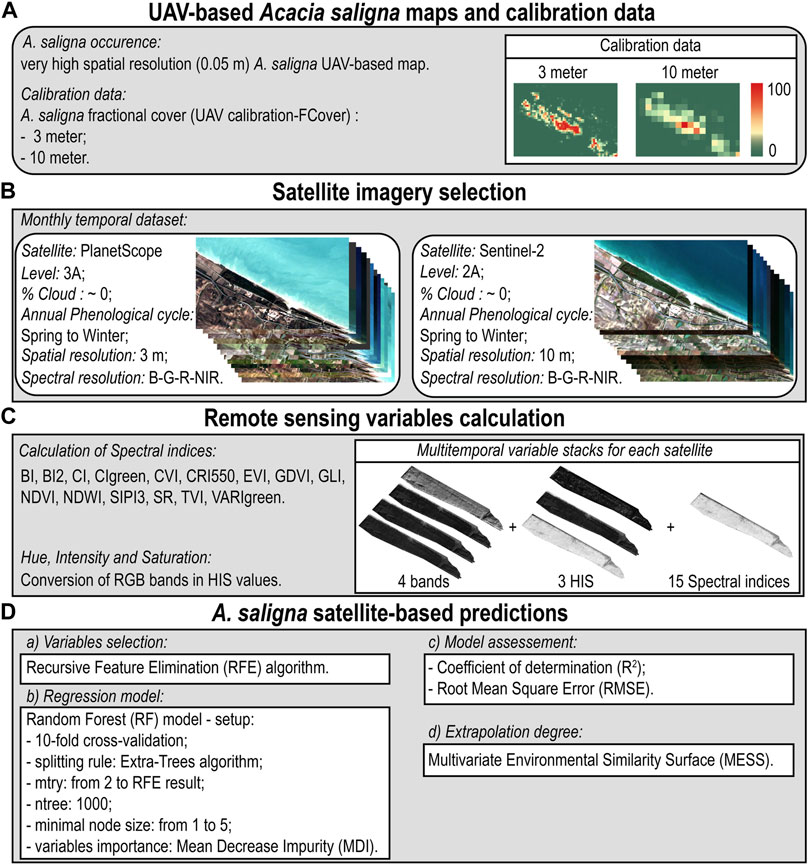
FIGURE 2. Workflow synthesizing the procedure for the satellite-based prediction of A. saligna distribution using the UAV-based IAS VHR information as calibration data. The proposal framework: (A) UAV-based A. saligna maps and calibration data, (B) Satellite imagery selection, (C) Remote sensing variables calculation, (D) A. saligna satellite-based predictions.
2.2.1 Unmanned aerial vehicle-based Acacia saligna calibration data
As calibration data for satellite-based predictions, we used a VHR (0.05 m) presence/absence map of A. saligna derived from the combination of a set of highly accurate UAV-based maps produced for the study area (on Figure 1, red polygon) and recently published by Marzialetti et al. (2021; Supplementary material 1 Supplementary Figure S1). In that research, UAV images were collected in pre-flowering and flowering periods using a multirotor quadcopter (DJI Phantom 4 Pro V2.0) equipped with two sensors: the CMOS (complementary metal oxide semiconductor) RGB (red–green–blue) camera with 20 Mpx and the Parrot Sequoia multispectral sensor G, R, REdge, and NIR bands (Green: 550 ± 40 nm; Red: 660 ± 40 nm; Red Edge: 735 ± 10 nm; and Near Infrared: 790 ± 40 nm) with 1.2 Mpx for each band. This study (Marzialetti et al., 2021) indicated a very high predictive performance (overall accuracy >95%, Kappa statistic >0.75) of four VHR maps derived from images registered during the IAP flowering period. So, in order to define robust calibration data (Figure 2A, calibration area) and reduce possible errors, we stacked the flowering period maps reporting as occurrences only those pixels in which A. saligna was predicted in at least three of the four maps. Then, based on these VHR calibration data (0.05 m), we calculated, either for PlanetScope or for Sentinel-2 platform, the fractional cover maps of A. saligna reporting the percent of VHR-invaded pixels in 3 m (FCoverPS) and 10 m (FcoverS2) grid cells, respectively.
2.2.2 Satellite imagery selection
As Mediterranean coastal areas are characterized by a highly dynamic landscape undergoing substantial seasonal changes, we relied on RS multi-temporal stacks for gathering monthly images. In order to capture the annual phenology both for A. saligna and for native coastal dune vegetation, we used monthly multispectral images recorded by two satellite platforms, which are accessible free of charge for research purposes (Figure 2B). PlanetScope (PS) satellite constellation consists of multiple DOVE CubeSat acquiring four bands: blue (BPS, 455–515 nm), green (GPS, 500–590 nm), red (RPS, 590–670 nm), and near infrared (NIRPS, 780–860 nm), with 3 m of spatial resolution (PlanetLabs Inc 2021; Cheng et al., 2020). We downloaded 12 cloud-free PlanetScope images (November 2020–October 2021) with a zenith view angle lower than 5° (i.e., nadir viewing; https://www.planet.com/explorer/). We specifically used the surface reflectance products (i.e., processing level 3B) with atmospherical correction already performed by PlanetLabs (Supplementary Material 2 Supplementary Table S1, Kotchenova et al., 2006; Kotchenova and Vermote, 2007).
The Sentinel-2 satellite (S2) is equipped with a multispectral instrument (MSI) sensor including 13 bands in the range of visible, near-infrared (10 m), and short-wave infrared (20 m). We used the 10 m bands: blue (BS2, 459–525 nm), green (GS2, 541–577 nm), red (RS2, 649–680 nm), and near infrared (NIRS2, 780–886 nm) bands (Drusch et al., 2012). We downloaded 12 cloud-free Sentinel-2 images (November 2020–October 2021; Copernicus Open Access Hub: https://scihub.copernicus.eu/). As the image of January 2021 showed high cloud coverage, we replaced it with a cloud-free image registered in January 2020 (Supplementary Material 2 Supplementary Table S2). We used atmospherically corrected images at the surface reflectance (processing level 2A; European Space Agency using a Sen2Cor processor, Louis et al., 2019).
2.2.3 Remote sensing variable calculation
As IAS spread could alter environmental features such as biomass, chlorophyll content, and soil features in invaded native ecosystems (Linders et al., 2019; Castro-Díez al., 2019), we calculated monthly RS variables related to these main biophysical parameters (Figure 2C; Table 1, Supplementary Material S3). In particular, we calculated a set of spectral variables using the R environmental ( R: https://www.r-project.org/) that could help in distinguishing A. saligna invaded areas from native vegetation, depicting leaf and plant characteristics (e.g., leaf content of chlorophyll and carotenoids) and canopy biomass, as well as soil features (e.g., bare surfaces and organic content), and the presence of surface water (Masemola et al., 2020; Shoko et al., 2020). For leaf and plant characteristics, we estimated leaf pigments using six spectral indices: the chlorophyll vegetation index (CVI) and the green leaf index (GLI) for chlorophyll content; the chlorophyll index green (CIgreen), which quantifies the photosynthetic activity; the carotenoid reflectance index 550 (CRI550) and the structure intensive pigment index 3 (SIPI3), which quantify the carotenoids and photosynthetic pigments; and the green difference vegetation index (GDVI), which estimates the content of nitrogen (Tucker et al., 1979; Peñuelas et al., 1995; Gobron et al., 2000; Gitelson et al., 2005; Vincini et al., 2008). Canopy biomass characteristics were described by computing five spectral indices: the normalized difference vegetation index (NDVI) and simple ratio (SR), which are canonical biomass indices; the enhanced vegetation index (EVI), which improves the biomass estimate compared to two previous indices, reducing the atmospheric influence; the transformed vegetation index (TVI), which is sensitive to the photosynthetically active biomass; and the visible atmospherically resistant index green (VARIgreen), able to estimate the canopy biomass using visible bands (B, G, and R, Huete et al., 2002; Tucker, 1979; Birth and McVey, 1968; Rouse et al., 1973; Gitelson et al., 2002). As for soil features, we calculated the brightness index (BI) and brightness index 2 (BI2) as proxies of the bare surface, while the coloration index (CI) was used for organic content (Escadafal et al., 1989; Mathieu et al., 1998). We also computed the normalized difference water index (NDWI) to estimate seasonal water surface fluctuations along coastal dunes (McFeeters, 1996).
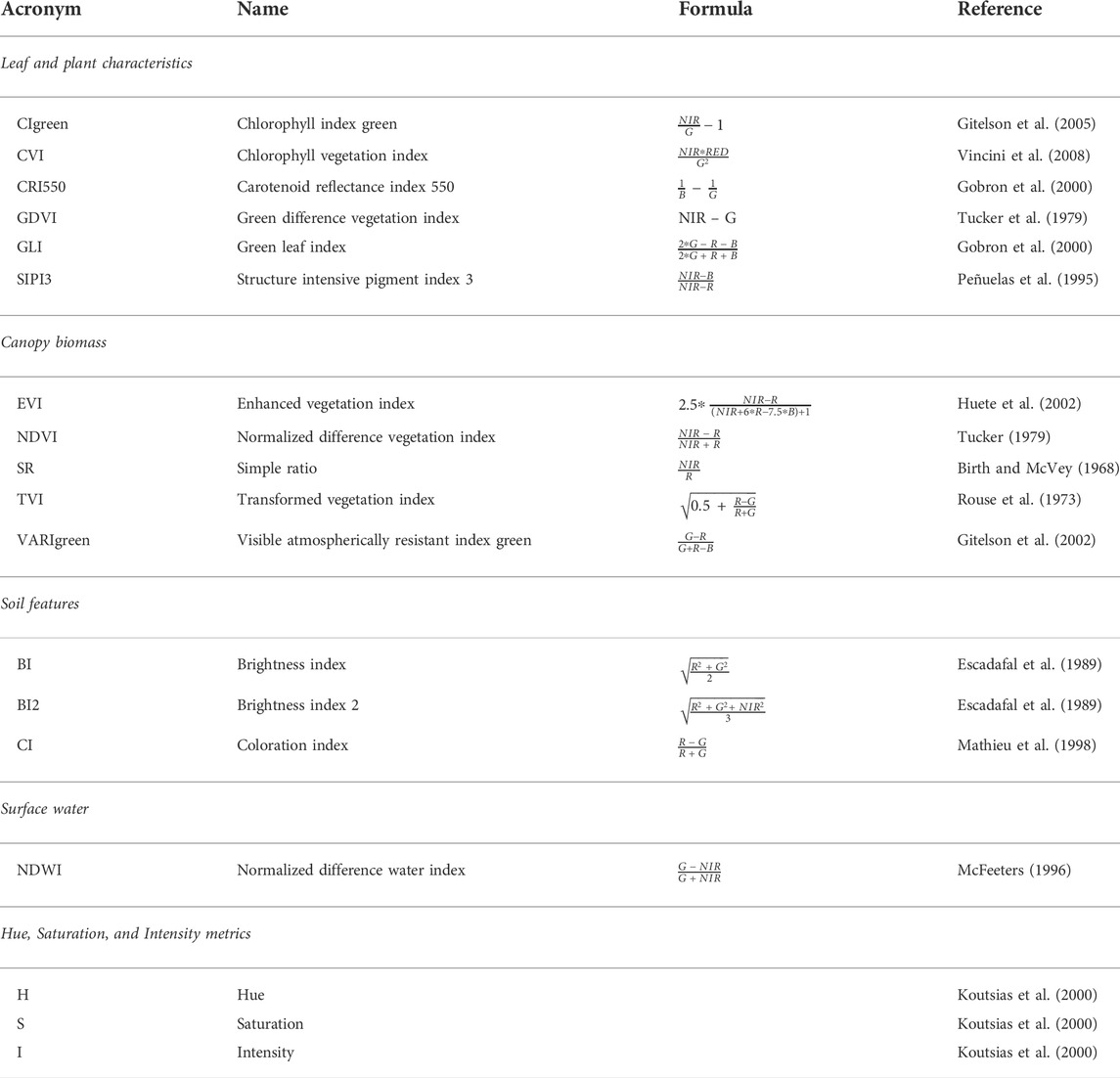
TABLE 1. Computed spectral indices and metrics: acronyms, full names, formulas, and references. Concerning the spectral indices are grouped by the main biophysical parameters related to leaf and plant characteristics, canopy biomass, soil features, and surface water (see for details Supplementary Material S2).
In addition, we transformed the surface reflectance values of RGB bands for all the images into digital numbers and converted them into hue (H), intensity (I), and saturation (S) metrics (Neteler and Mitasova, 2004; Zhang, 2004, Figure 2C; i.rgb.his tool in GRASS GIS 7.8; GRASS Development Team, 2020, Table 1), so as to catch color differences among A. saligna leaves and inflorescences and native vegetation. HIS variables are particularly effective in discriminating A. saligna from native vegetation at a local scale (Yang et al., 2020; Marzialetti et al., 2021). For each pixel, the H metric depicts the dominant wavelength, the I metric depicts the brightness of a color (e.g., the relative degree of black or white), and the S metric depicts the purity of the color, defined as the absence of mixture with other wavelengths (Koutsias et al., 2000; Tu et al., 2005).
For each satellite (e.g., PlanetScope and Sentinel-2 images) and area (e.g., calibration and prediction), we built a multi-temporal stack including monthly bands (RGB and NIR), HIS values, and spectral indices (i.e., 264 layers; Figure 2C and Table 1).
2.2.4 A. saligna satellite-based predictions
To upscale A. saligna FCover derived from UAV to PlanetScope (predicted-FCoverPS) and Sentinel-2 (predicted-FCoverS2) resolutions, we modelled this variable against the spectral indices using random forest (RF; Figure 2D, Breiman, 2001). RF is a machine learning algorithm that operates with a large combination of decision trees, reducing the error in classification and regression by using bootstrap in the considered explanatory variables (Cutler et al., 2007; Chan and Paelinckx, 2008). RF is commonly applied to spatial regression and classification of remote sensing data (Belgiu and Drăguţ, 2016; Izquierdo-Verdiguier and Zurita-Milla, 2020), also accommodating well with highly correlated explanatory variables (Meyer et al., 2017).
Given the high dimensionality of our data, we reduced the multitemporal spectral variables using the recursive feature elimination algorithm (RFE, Figure 2D, Kuhn and Johnson, 2013). RFE is a feature selection algorithm that estimates the lowest possible number of features without reducing the final performance metrics of the RF model (Demarchi et al., 2020). RFE iteratively computes RF models with all the explanatory variables in the calibration data and then drops in each cycle the lowest important variable (i.e., scoring the lowest value of the mean decrease impurity (MDI) index). At the end of all cycles, RFE identifies the best number of explanatory variables by comparing the predictive performance metrics of all the RF models produced (Meyer et al., 2017; Lou et al., 2020). We performed the RFE algorithm through the “caret” R package (function rfe, Kuhn et al., 2021) applying a 10-fold cross-validation and calculating the root mean square error (RMSE, Castillo-Riffart et al., 2017) to assess the predictive performance of each subset of variables (i.e., we set a maximum of 80 variables). Once we identified the best number of variables, we fine-tuned the RF model according to three parameters: the number of uncorrelated decision trees (Ntree),the number of variables randomly selected at each node of decision trees (Mtry), and the minimum number of observations in a terminal node (minimal node size, Belgiu and Drăguţ, 2016; Probst et al., 2018). We set a high number of uncorrelated decision trees (Ntree = 1,000), tested different Mtry values ranging from 2 to the number of variables as indicated by RFE results, and checked a range of minimal node size from 1 to 5 (Figure 2D). Furthermore, we used the Extra-Trees algorithm as a splitting procedure to apply the regression on independent tree nodes (Geurts et al., 2006).
The RF model reporting the lowest RMSE under a 10-fold cross-validation (Figure 2D, Routh et al., 2018) was selected as the optimal one and used to predict A. saligna FCover. Along with RMSE, RF predictive performance was assessed by calculating the coefficient of determination R2 between observed and predicted values under cross-validation. RMSE and R2 values by RF models obtained from PlanetScope and Sentinel-2 images were compared through the Mann–Whitney test to assess which of the two satellites performed best in predicting A. saligna FCover.
The relative importance of spectral variables in RF models was estimated through the MDI index (i.e., the Gini index with the sum of squares as an impurity measure, Nembrini et al., 2018; Figure 2D). Then, we explored the shape of the relationships between predicted-FCover of A. saligna and the most important variables in both RF models by using partial dependence (PD, Friedman, 2001) plots. PD plots measure the marginal effect of a given explanatory variable on the predicted values of RF model, f constant, and the other variables’ constant (e.g., to their median value; Elith et al., 2008).
Lastly, we evaluated the degree of extrapolation on values of spectral variables outside the UAV flight area by computing the multivariate environmental similarity surface (MESS) in both RF models (Figure 2D, Elith et al., 2010). MESS measures the similarity between values of spectral variables inside the calibration area and values in the prediction area. Negative values (MESS <0) indicate pixels with values of spectral variables dissimilar from the calibration area (Elith et al., 2010). We computed MESS using the R package “dismo” (function mess, Hijmans and Elith 2021) and reported the percentage of negative MESS values in both RF models.
3 Results
The RF model on Sentinel-2 data retained 25 spectral variables after RFE with Mtry and minimal node size equal to 24 and 1, respectively, performing slightly better (R2 = 0.707 ± 0.088) than the model on PlanetScope data, which retained 75 variables and obtained Mtry and minimal node size equal to 66 and 1, respectively (R2 = 0.628 ± 0.028, Figure 3, see also Supplementary Material S4). The RMSE of the Sentinel-2 model was significantly lower than that of PlanetScope (Mann–Whitney U = 0, p < 0.001; Supplementary Material S5).
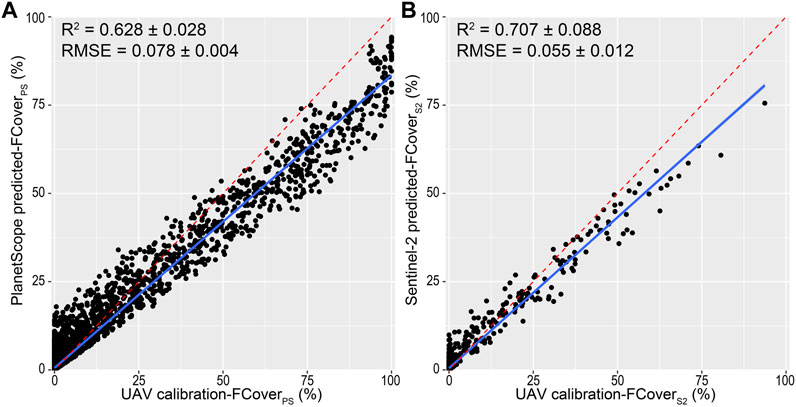
FIGURE 3. Relationship between calibration A. saligna fractional cover (e.g., UAV calibration-FCover) and predicted fractional cover obtained by (A) PlanetScope (predicted-FCoverPS) and (B) Sentinel-2 (predicted-FCoverS2) classification models. Blue depicts the linear regression between predicted FCover and calibration FCover. Red dashed lines depict ideal regressions of perfect match among predicted-FCover and calibration-FCover values. RF predictive performance (R2) and root mean square error (RMSE) for each regression are also reported.
The relationship between A. saligna observed (i.e., derived from UAV) and predicted (i.e., satellite-based) FCover values varied between the two satellite platforms, with R2 PlanetScope = 0.628 ± 0.028 and R2 Sentinel-2 = 0.707 ± 0.088 (Figure 3). We observed a moderate variability as well as a wider range (e.g., from 0 to 95%) in FCover values predicted by the PlanetScope RF model. Predicted values of the Sentinel-2 model presented a lower variability and a reduced range (from 0 to 75%). In both RF models, predicted FCover values are slightly over-estimated compared with the lowest observed values and under-estimated compared with the highest observed values (Figure 3).
Spatially explicit predictions of A. saligna FCover evidenced differences in the ability of the two satellite platforms to depict the smoothed spatial variations in A. saligna observed cover as well as the invaded patch edges, which varied markedly with the spatial resolution of the considered satellite images (Figure 4). The RF model based on PlanetScope, with a finer spatial resolution (3 m), was able to delineate invaded patches and accurately describe the smooth FCover gradient between the invaded core areas and edges (Figure 4C), predicting even small patches correctly. On the contrary, the model based on Sentinel-2 images, with a coarser spatial resolution (10 m), delineated A. saligna edges quite poorly, as well as the fuzzy variability of the observed FCover values in invaded areas. In addition, it was unable to map small invaded patches (Figure 4D).
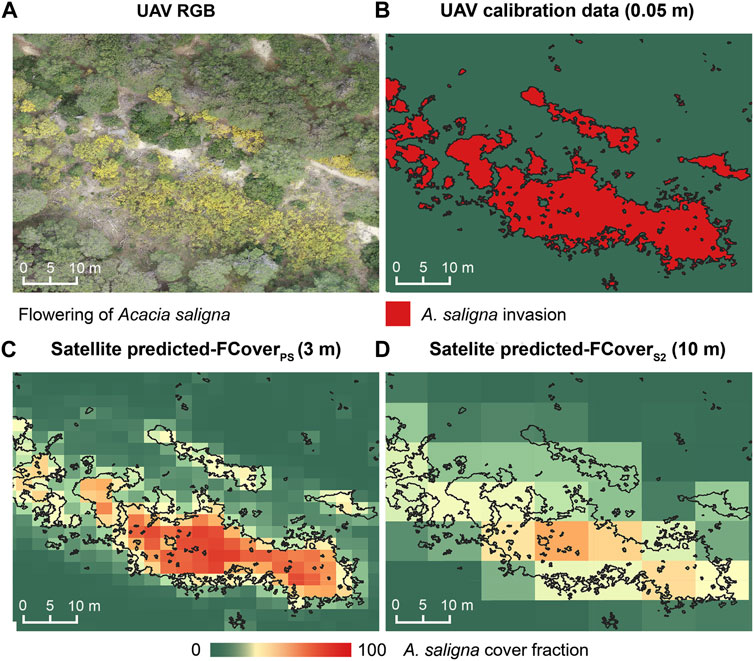
FIGURE 4. Visual example of (A) UAV orthophotographs captured during the A. saligna flowering period, (B) calibration data derived by the UAV-based VHR A. saligna map 0.05 m, (C) A. saligna fractional cover on the PlanetScope model (predicted-FCoverPS), and (D) A. saligna fractional cover on the Sentinel-2 model (predicted-FCoverS2). Black edges reported on boxes C and D depict the shape of UAV-based A. saligna distribution used as calibration data.
The relative importance of spectral variables measured by the MDI index differed between Sentinel-2 and PlanetScope models (Figure 5). In the PlanetScope model, the first 60% of the cumulated MDI percentage was achieved with 18 spectral variables (i.e., from CVI.07 to NDWI.07; Figure 5A), while in the Sentinel-2 model the same percentage was achieved with six spectral variables (from H.07 to CVI.08). In the PlanetScope RF model, the three most important variables were chlorophyll vegetation index of July (CVI.07; 7%), hue of July (H.07; 6,9%), and hue of May (H.05; 5,8%; Figure 5A). According to PD plots, high CVI.07 and the extreme values (low and high) of H.07 and H.05 were consistently associated with an increase in A. saligna FCover (Supplementary Material S6). Other important variables in the PlanetScope model were related to summer (e.g. BI2.06, BI2.07, GLI.07, CI.07, VARIgreen.07, CIgreen.07, TVI.07, NDWI.07, NIR.07, and GDVI.08; Figure 5A) and autumn (e.g. G.09, CVI.09, EVI.10, SR.10, SIPI3.10, and NDVI.10; Figure 5A).
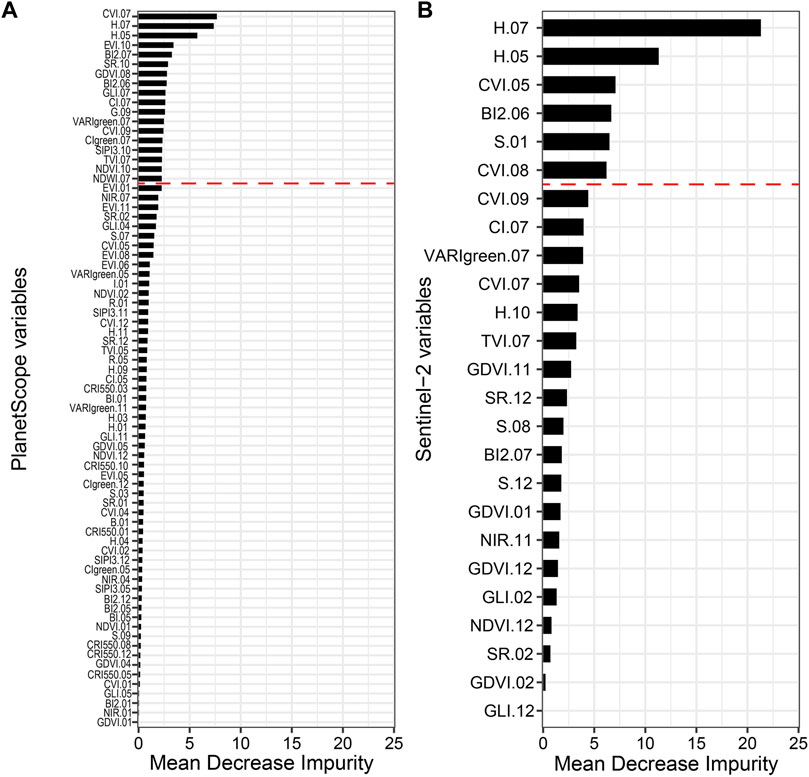
FIGURE 5. Relative importance of RS spectral variables in percentage (%) calculated using the mean decrease impurity for (A) PlanetScope (75 variables) and (B) Sentinel-2 (25 variables) RF models. Spectral variables are reported in Table 1. Red dashed lines indicate the 60% of cumulated MDI percentage.
In the Sentinel-2 RF model, the spectral variables included in the first 60% of the cumulated MDI percentage are related to summer (H.07, BI2.06, and CVI.08), spring (H.05 and CVI.05), and winter (S.01) features. Specifically, the hues of July (H.07) and May (H.05), with 21 and 11% MDI, respectively, were the two most important variables (Figure 5B). H.07, H.05, CVI.05, CVI.08, and S.01 showed a positive relationship with predicted FCover values, while BI2.06 showed a slightly decreasing relationship (Supplementary Material S6).
The degree of extrapolation in the predicted area varied among the two RF models. The PlanetScope model showed a low percentage of pixels with negative MESS values (6.043%) in areas where A. saligna occurrence is very unlikely (e.g., on the sandy beach close to the sea; Supplementary Material S7). The Sentinel-2 RF model showed a higher degree of extrapolation with 13.792% of pixels with negative MESS values (Supplementary Material S7).
4 Discussion
The present work contributed to improving monitoring tools for A. saligna detection and spread, extending the utilization of UAV data to support IAS satellite modelling on Mediterranean coastal dunes. This approach was previously tested in a temperate broadleaved forest (Kattenborn et al., 2019; Gränzig et al., 2021; Holden et al., 2021), tundra coniferous formations (Riihimäki et al., 2019), and wetlands (Zhou et al., 2018; Doughty et al., 2021). It is implemented in this study for the first time in complex coastal landscapes.
Our results evidenced a good potential of UAV-based fine-scale maps as a source of calibration data for satellite-based prediction of a woody IAP in partially invaded Mediterranean coasts.
The RF models for both satellite platforms predicted A. saligna FCover properly, although they slightly over-estimated A. saligna FCover comparedwith lower values and under-estimated compared with the higher ones. A similar overestimation of cells with low cover values was registered by Kattenborn et al. (2019) with other IAPs (e.g., Pinus radiata, invading forest and Ulex europaeus invading scrublands) in South America. These authors underlined the limits for detecting cover fractions below a ∼12% threshold. Differently, the under-estimation of pixels with high Fcover values may be related to the small number of coarse satellite grids dominated by A. saligna inside the calibration area.
Concerning the predictive accuracy, as previously observed in tundra and taiga ecosystems (Riihimäki et al., 2019; Fraser et al., 2021), and also on coastal dune landscapes, satellite images with a coarse spatial resolution supported distribution models with higher predictive accuracy than those with fine spatial resolution. According to RMSE values, the 10 m resolution Sentinel-2 model predicted the VHR UAV calibration data more accurately than the 3 m PlanetScope model. As reported in previous studies (Dark and Bram, 2007; Riihimäki et al., 2019; Fraser et al., 2021), such differences may be due to the modifiable area unit problem (MAUP) and the decreased variance of the coarser-resolution data, arising due to the aggregation of finer scale data (e.g., 0.05 m VHR IAP map) on coarser grids (e.g., 10 m resolution FcoverS2). MAUP affects the statistical analyses because with the aggregation of fine cell information in the larger ones (e.g., 3 m or 10 m), a decrease of the between-cell variability is verified and an increase of the explanatory power of derived models occurs.
On the other hand, the finer spatial resolution of PlanetScope (3 m) allowed the maintenance of a good part of the spatial information derived by UAV data, as verified by the variability of its FCover values ranging from 0 to almost 100 percent. In the Sentinel-2 FCover values, the relation among VHR UAV occurrences is limited to a smaller range.
Other scale issues that might influence the IAS model accuracy are the low number of pixels registering “pure” A. saligna patches in Sentinel-2 images and the relatively limited number of total pixels in the prediction area. In fact, the fuzzy shape of A. saligna patches as derived by Sentinel-2 models corresponds to better model performance values (Riihimäki et al., 2019; He et al., 2021). On the other hand, the finer spatial resolution of PlanetScope images allowed us to model the variability of FCover values registered by the very high UAV-based map, thus offering a good support for identifying A. saligna patch edges. Similarly, this complementarity between coarse and fine-scale images has been observed in previous studies predicting the fractional cover of tundra vegetation and lichen of taiga using UAV and satellite images with different spatial resolutions (e.g., PlanetScope, Sentinel-2, and Landsat; Riihimäki et al., 2019; He et al., 2021).
The analysis of multi-temporal spectral variables derived from visible (blue, green, and red) and NIR bands acquired by Sentinel-2 and PlanetScope platforms effectively depicted the phenological behavior of specific IAS. Our results pinpointed the summer biomass production peak (see CVI of July for PlanetScope and CVI of August for Sentinel-2) among the most important parameters for modelling the fractional cover of A. saligna along coastal dune systems. Previous research studies based on medium-resolution satellite images (RGB and NIR bands of Sentinel-2) evidenced the importance of spectral indices depicting the characteristic Acacia spp. summer productivity peak to detect invaded patches embedded in natural landscapes (e.g., montane forest and alluvial wetlands; see Masemola et al., 2020; Kattenborn et al., 2019). As observed for other Acacia species (e.g., A. dealbata, A. mearnsii, and A. longifolia; Masemola et al., 2020; Groβe-Stoltenberg et al., 2016), and also for A. saligna, the analyzed RS variables denoted high leaf chlorophyll content during the summer season. Such photosynthetic temporal patterns can be described by several monthly RS spectral indices (e.g., CIgreen, CVI, GLI, and GDVI) and, in our case, by the high July CVI values. This peak of chlorophyll content is consistent with the previous field ecophysiological studies along the Mediterranean coastal environments (Nativ et al., 1999; Morris et al., 2011).
Our results also evidenced that soil RS variables (summer BI2 and CI values) are important for modelling the fractional cover of A. saligna, which is consistent with the alterations in leaf litter content and soil organic matter in IAS patches usually observed in the field (Del Vecchio et al., 2013; Nsikani et al., 2017; Tozzi et al., 2021). In fact, Acacia species, being nitrogen-fixing plants, modify soil features, increasing the organic content and the development of soil microbiomes (Del Vecchio et al., 2013). This nitrogen-fixing action alters the nature of the unconsolidated coastal dune soil characterized by low organic content (Le Maitre et al., 2011). Both PlanetScope and Sentinel-2 models highlighted a reduced summer bare surface on A. saligna patches compared to the coastal dune native vegetation, indicating a dense canopy of the IAS as well as an enriched soil organic content. It is also noteworthy that the nitrogen content in leaves, as calculated by GDVI, was markedly higher in A. saligna patches than native vegetation ones (Yelenik et al., 2004; Hellmann et al., 2011).
Interestingly, during the summer period, the two RS models distinguished the A. saligna patches from native vegetation by different hue values of leaves (see H of July). This pattern may be due to the different coloration of leaves between A. saligna and dominant native species, particularly the gymnosperm species such as Pinus spp., Juniperus oxicedrus, and the maquis species such as Pistacia lentiscus and Phyllirea angustifolia.
Acacia saligna is characterized by an early blooming period compared to the coastal dune vegetation and conforms to pure yellow color patches in April–May (Milton and Moll, 1982; Paz-Kagan et al., 2019). The spectral variables characterizing the A. saligna blooming period are very useful for detecting the IAS using remote sensing images with very fine spatial (e.g., VHR UAV images; Marzialetti et al., 2021) or spectral (e.g., yellow wavelength bands; Paz-Kagan et al., 2019) resolution. The models developed in this study using coarse spatial and spectral information, freely available from PlanetScope and Sentinel-2 platforms, partially confirm the usefulness of RS variables registered during the blooming period, as suggested by the distinct behavior of hue and the chlorophyll content of leaves in May. Moreover, the combined use of multi-temporal RS variables and machine learning algorithms allowed to describe different RS ecological conditions over time and seasons, supporting the detection of A. saligna and the identification of the most important RS variables to distinguish the invaded patches from native vegetation ones.
Finally, the adopted approach offers economic commitments and reduces the time necessary to evaluate A. saligna invasion levels in complex environments such as coastal dunes. In this way, this approach supports the prioritization of monitoring and management actions claimed by the EU IAS Regulation 1,143/2014 (Simberloff et al., 2013; Rai and Sing, 2020; Souza-Alonso et al., 2017). In addition, this methodology could easily be applied to other IAS in complex environments. UAV images could be considered appropriate candidates to become a major tool to gather reference data. In this context, we could better outline operational workflows for the early detection and monitoring of IAS invasion status over time, which is essential to define adequate management actions and to tackle these invasive species (Holden et al., 2021).
5 Conclusion
Developing early detection and monitoring tools for IAS that are able to operate at wide scales is an urgent challenge requested by the IAS European Regulation (IAS Regulation 1,143/2014). In this study, we tested a methodological workflow, applied for the first time on coastal dune landscapes, to predict the fractional cover of the invasive alien species A. saligna by applying a combination of VHR UAV data and two satellite multi-temporal images (PlanetScope and Sentinel-2) with a different spatial resolution (3 and 10 m, respectively). We presented new evidence on the importance of VHR UAV data to fill the gap between field observation of A. saligna and satellite data in complex and dynamic environments such as the Mediterranean coastal dunes. The predictions based on PlanetScope and Sentinel-2 multi-temporal data accurately predicted A. saligna fractional cover derived by the UAV-based map with high performance metrics in both models (R2 > 0.6 and RMSE <0.08). The PlanetScope-based model was able to outline invaded area edges even for small patches. Moreover, the model accurately described the smooth FCover gradient between the invaded core areas and edges, confirming the importance of finer spatial resolutions.
The over-estimation and under-estimation of the lowest and highest fractional cover predicted values in both satellites gave evidence of a reduced capability of the satellite-based model to detect early stages of the invasion process and dense monospecific patches.
Nevertheless, from an applied perspective, our results also allow to identify the set of RS variables depicting effective ecological parameters for predicting A. saligna occurrence in coastal areas, and then contribute to improving monitoring activities. Our encouraging results support the usefulness of combining UAV and satellite images to detect and monitor A. saligna spread in the coastal areas in a timely and cost-effective manner.
Overall, we could conclude that our approach, based on satellite images available worldwide and free of charge for research purposes, could be potentially applied to a wide variety of landscapes and IAPs. This procedure could be important not only in Europe (IAS regulation 1143/2014) but also around the world (global strategy against IAS by CBC) because IAS management requires a common approach worldwide. With this in mind, further case studies could be implemented in the future to better test and extend the synergetic use of unmanned aerial vehicles and satellite images presented here. Moreover, this approach could provide comparable information for other coastal ecosystems, for other invasive alien species (e.g., Carpobrotus spp., Agave americana, and Yucca gloriosa), and for other biogeographical areas as well.
Data availability statement
The raw data supporting the conclusion of this article will be made available by the authors, without undue reservation.
Author contributions
All authors contributed substantially to the work: FM, MC, and MDF conceived and designed the study; FM, LF, and WDS collected remote sensing data; FM, MDF, and MC analyzed the data; FM, MDF, and MC led the writing of the manuscript; AA and MC supervised the research. All authors contributed critically to the drafts and gave final approval for publication. All authors have read and agreed to the published version of the manuscript.
Funding
This study was carried out with a support of the bilateral program Italy–Israel DERESEMII (Developing state-of-the-art remote sensing tools for monitoring the impact of invasive plant) and the Interreg Italia—Croazia CASCADE (CoAStal and marine waters integrated monitoring systems for ecosystems proteCtion AnD management—Project ID 10255941). The Grant of Excellence Departments, MIUR—Italy (ARTICOLO 1, COMMI 314–337 LEGGE 232/2016) is also gratefully acknowledged.
Acknowledgments
The authors are grateful to the Long-Term Ecological Research (LTER) and LTER—Italia. The authors are grateful to Planet Labs for free PlanetScope imagery for research purposes. Furthermore, the authors acknowledge Silvia Cascone and Francesco Pio Tozzi, for the help given during the acquisition of UAV images. The authors thank the editor and the reviewers for their time and comments which helped them to improve the original version of the article.
Conflict of interest
The authors declare that the research was conducted in the absence of any commercial or financial relationships that could be construed as a potential conflict of interest.
Publisher’s note
All claims expressed in this article are solely those of the authors and do not necessarily represent those of their affiliated organizations, or those of the publisher, the editors, and the reviewers. Any product that may be evaluated in this article, or claim that may be made by its manufacturer, is not guaranteed or endorsed by the publisher.
Supplementary material
The Supplementary Material for this article can be found online at: https://www.frontiersin.org/articles/10.3389/fenvs.2022.880626/full#supplementary-material
References
Acosta, A. T. R., Blasi, C., Carranza, M. L., Ricotta, C., and Stanisci, A. (2003). Sandy coastal landscape of the lazio region (Central Italy). phyto. 33 (4), 715–726. doi:10.1127/0340-269X/2003/0033-0715
Acosta, A. T. R., Carranza, M. L., and Izzi, C. F. (2009). Are there habitats that contribute best to plant species diversity in coastal dunes? Biodivers. Conserv. 18, 1087–1098. doi:10.1007/s10531-008-9454-9
Alvarez-Vanhard, E., Corpetti, T., and Houet, T. (2021). UAV & satellite synergies for optical remote sensing applications: a literature review. Sci. Remote Sens. 3, 100019. doi:10.1016/j.srs.2021.100019
Anderson, C. B. (2018). Biodiversity monitoring, earth observations and the ecology of scale. Ecol. Lett. 21, 1572–1585. doi:10.1111/ele.13106
Asefa, G., and Tamir, B. (2006). Effects of supplementing different forms of Acacia saligna leaves to grass hay on feed intake and growths of lambs. Trop. Sci. 46, 205–208. doi:10.1002/ts.178
Bar, P., Cohen, O., and Shoshany, M. (2004). Invasion rate of the alien species Acacia saligna within coastal sand dune habitats in Israel. Isr. J. Plant Sci. 52, 115–124. doi:10.1560/8BK5-GFVT-NQ9J-TLN8
Bartz, R., and Kowarik, I. (2019). Assessing the environmental impacts of invasive alien plants: a review of assessment approaches. NeoBiota 43, 69–99. doi:10.3897/neobiota.43.30122
Bazzichetto, M., Malavasi, M., Acosta, A. T. R., and Carranza, M. L. (2016). How does dune morphology shape coastal ec habitats occurence? a remote sensing approach using airborne LiDAR on the mediterranean coast. Ecol. Indic. 71, 618–626. doi:10.1016/j.ecolind.2016.07.044
Belgiu, M., and Drăguţ, L. (2016). Random forest in remote sensing: a review of applications and future directions. ISPRS J. Photogramm. Remote Sens. 114, 24–31. doi:10.1016/j.isprsjprs.2016.01.011
Birth, G. S., and McVey, G. R. (1968). Measuring the color of growing turf with a reflectance spectrophotometer 1. Agron. J. 60, 640–643. doi:10.2134/agronj1968.00021962006000060016x
Bolch, E. A., Santos, M. J., AdeKhannaBasinger, C. S. N. T., Reader, M. O., and Hestir, E. L. (2020). Remote detection of invasive alien species” in Remote Sensing of plant biodiversity. Cham, Switzerland): Springer Open, 267–308.
Bradley, B. A. (2009). Accuracy assessment of mixed land cover using a GIS-designed sampling scheme. Int. J. Remote Sens. 30 (13), 3515–3529. doi:10.1080/01431160802562263
Bradley, B. A. (2014). Remote detection of invasive plants: a review of spectral, textural and phenological approaches. Biol. Invasions 16, 1411–1425. doi:10.1007/s10530-013-0578-9
Branquart, E., Brundu, G., Buholzer, S., Chapman, D., Ehret, P., Fried, G., et al. (2016). A prioritization process for invasive alien plant species incorporating the requirements of EU regulation no. 1143/2014. EPPO Bull. 46, 603–617. doi:10.1111/epp.12336
Brundu, G., Lozano, V., and Branquart, E. (2018). Information on measures and related costs in relation to species considered for inclusion on the union list: Acacia saligna. Technical note prepared by IUCN for the European Commission.
Carranza, M. L., Acosta, A. T. R., Stanisci, A., Pirone, G., and Ciaschetti, G. (2008). Ecosystem classification for EU habitat distribution assessment in sandy coastal environments: an application in central Italy. Environ. Monit. Assess. 140, 99–107. doi:10.1007/s10661-007-9851-7
Carranza, M. L., Drius, M., Malavasi, M., Frate, L., Stanisci, A., and Acosta, A. T. R. (2018). Assessing land take and its effects on dune carbon pools. an insight into the mediterranean coastline. Ecol. Indic. 85, 951–955. doi:10.1016/j.ecolind.2017.10.052
Castillo-Riffart, I., Galleguillos, M., Lopatin, J., and Perez-Quezafa, J. F. (2017). Predicting vascular plant diversity in anthropogenic peatlands: comparison of modelling methods with free satellite data. Remote Sen. 9, 681. doi:10.3390/rs9070681
Castro-Díez, P., Vaz, A. S., Silva, J. S., van Loo, M., Alonso, Á., Aponte, C., et al. (2019). Global effects of non-native tree species on multiple ecosystem services. Biol. Rev. 94, 1477–1501. doi:10.1111/brv.12511
Chan, J. C.-W., and Paelinckx, D. (2008). Evaluation of random forest and adaboost tree-based ensemble classification and spectral band selection for ecotope mapping using airborne hyperspectral imagery. Remote Sens. Environ. 112, 2999–3011. doi:10.1016/j.rse.2008.02.011
Cheng, Y., Vrieling, A., Fava, F., Meroni, M., Marshall, M., and Gachoki, S. (2020). Phenology of short vegetation cycles in a kenyan rangeland from planetScope and sentinel-2. Remote Sens. Environ. 248, 112004. doi:10.1016/j.rse.2020.112004
Crisóstomo, J. A., Rodríguez-Echeverría, S., and Freitas, H. (2013). Co-introduction of exotic rhizobia to the rhizosphere of the invasive legume Acacia saligna, an intercontinental study. Appl. Soil Ecol. 64, 118–126. doi:10.1016/j.apsoil.2012.10.005
Cutler, D. R., Edwards, T. C., Beard, K. H., Cutler, A., Hess, K. T., Gibson, J., et al. (2007). Random forest for classification in ecology. Ecology 88 (11), 2783–2792. doi:10.1890/07-0539.1
Dao, P. D., Axiotis, A., and He, Y. (2021). Mapping native and invasive grassland species and characterizing topography-driven species dynamics using high spatial resolution hyperspectral imagery. Int. J. Appl. Earth Obs. Geoinf. 104, 102542. doi:10.1016/j.jag.2021.102542
Dark, S. J., and Bram, D. (2007). The modifiable areal unit problem (MAUP) in physical geography. Prog. Phys. Geogr. Earth Environ. 31, 471–479. doi:10.1177/0309133307083294
Del Vecchio, S., Acosta, A. T. R., and Stanisci, A. (2013). The impact of Acacia saligna invasion on Italian coastal dune EC habitats. C. R. Biol. 336, 364–369. doi:10.1016/j.crvi.2013.06.004
Demarchi, L., Kania, A., Ciężkowski, W., Piórkowski, H., Oświecimska-Piasko, Z., and Chormański, J. (2020). Recursive feature elimination and random forest classification of natura 2000 grasslands in lowland river valleys of poland based on airborne hyperspectral and LiDAR data fusion. Remote Sens. (Basel). 12, 1842. doi:10.3390/rs12111842
Di Febbraro, M., Frate, L., de Francesco, M. C., Stanisci, A., Pio Tozzi, F., Varricchione, M., et al. (2021). modelling beach litter accumulation on mediterranean coastal landscapes: an integrative framework using species distribution models. Land 10 (1), 54. doi:10.3390/land10010054
Donaldson, J. E., Hui, C., Richardson, D. M., Robertson, M. P., Webber, B. L., and Wilson, J. R. H. (2014). Invasion trajectory of alien trees: the role of introduction pathway and planting history. Glob. Change Biol. 20, 1527–1537. doi:10.1111/gcb.12486
Doughty, C. L., Ambrose, R. F., Okin, G. S., and Cavanaugh, K. C. (2021). Characterizing spatial variability in coastal wetland biomass across multiple scales using UAV and satellite imagery. Remote Sens. Ecol. Conserv. 7 (3), 411–429. doi:10.1002/rse2.198
Drius, M., Carranza, M. L., Stanisci, A., and Jones, L. (2016). The role of Italian coastal dunes as carbon sinks and diversity sources. a multi-service perspective. Appl. Geogr. 75, 127–136. doi:10.1016/j.apgeog.2016.08.007
Drius, M., Malavasi, M., Acosta, A. T. R., Ricotta, C., and Carranza, M. L. (2013). Boundary-based analysis for the assessment of coastal dune landscape integrity over time. Appl. Geogr. 45, 41–48. doi:10.1016/j.apgeog.2013.08.003
Drusch, M., Del Bello, U., Carlier, S., Colin, O., Fernandez, V., Gascon, F., et al. (2012). Sentinel-2: ESA’s optical high-resolution mission for GMES operational services. Remote Sens. Environ. 120, 25–36. doi:10.1016/j.rse.2011.11.026
El-Gawad, A. A. M., and El-Amier, Y. A. (2015). Allelopathy and potential impact of invasive Acacia saligna (labill.) wendl. On plant diversity in the nile delta coast of egypt. Int. J. Environ. Res. 9 (3), 923–932.
Elith, J., Kearney, M., and Philips, S. (2010). The art of modelling range-shifting species. Methods Ecol. Evol. 1, 330–342. doi:10.1111/j.2041-210X.2010.00036.x
Elith, J., Leathwick, J. R., and Hastie, T. (2008). A working guide to boosted regression trees. J. Anim. Ecol. 77 (4), 802–813. doi:10.1111/j.1365-2656.2008.01390.x
Elkind, K., Sankey, T. T., Munson, S. M., and Aslan, C. E. (2019). Invasive buffelgrass detection using high-resolution satellite and UAV imagery on google earth engine. Remote Sens. Ecol. Conserv. 5 (4), 318–331. doi:10.1002/rse2.116
Escadafal, R., Girard, M.-C., and Courault, D. (1989). Munsell soil color and soil reflectance in the visible spectral bands of landsat MSS and TM data. Remote Sens. Environ. 46, 37–46. doi:10.1016/0034-4257(89)90035-7
Fraser, R. H., Pouliot, D., and van der Sluijs, J. (2021). UAV and high resolution satellite mapping of forage lichen (Cladonia spp.) in a rocky canadian shield landscape. Can. J. Remote Sens. 48, 5–18. doi:10.1080/07038992.2021.1908118
Friedman, J. H. (2001). Greedy function approximation: a gradient boosting machine. Ann. Stat. 1189–1232. doi:10.1214/aos/1013203451
Genovesi, P., Carboneras, C., Vilà, M., and Walton, P. (2015). EU adopts innovative legislation on invasive species: a step towards a global response to biological invasions? Biol. Invasions 17, 1307–1311. doi:10.1007/s10530-014-0817-8
George, N., Henry, D., Yan, G., and Byrne, M. (2007). Variability in feed quality between populations of Acacia saligna (labill.) H. Wendl. (Mimosoideae)—implications for domestication. Anim. Feed Sci. Technol. 136, 109–127. doi:10.1016/j.anifeedsci.2006.08.026
Geurts, P., Ernst, D., and Wehenkel, L. (2006). Extremely randomized trees. Mach. Learn. 63, 3–42. doi:10.1007/s10994-006-6226-1
Gitelson, A. A., Kaufman, Y. J., Stark, R., and Rundquist, D. (2002). Novel algorithms for remote estimation of vegetation fraction. Remote Sens. Environ. 80, 76–87. doi:10.1016/S0034-4257(01)00289-9
Gitelson, A. A., Viña, A., Ciganda, V., Rundquist, D. C., and Arkebauer, T. J. (2005). Remote estimation of canopy chlorophyll content in crops. Geophys. Res. Lett. 32 (8), L08403. doi:10.1029/2005GL022688
Gobron, N., Pinty, B., Verstraete, M. M., and Widlowski, J.-L. (2000). Advanced vegetation indices optimized for up-coming sensors: design, performance, and applications. IEEE Trans. Geosci. Remote Sens. 38 (6), 2489–2505. doi:10.1109/36.885197
Gränzig, T., Fassnacht, F. E., Kleinschmit, B., and Förster, M. (2021). Mapping the fractional coverage of the invasive shrub Ulex europaeus with multi-temporal Sentinel-2 imagery utilizing UAV orthoimages and a new spatial optimization approach. Int. J. Appl. Earth Obs. Geoinf. 96, 102281. doi:10.1016/j.jag.2020.102281
Grass Development Team, (2020). Geographic resources analysis support system (GRASS) software. Open Source Geospatial Foundation. http://grass.osgeo.org (Accessed November 28, 2021).
Groβe-Stoltenberg, A., Hellmann, C., Werner, C., Oldeland, J., and Thiele, J. (2016). Evaluation of continuous VNIR-SWIR spectra versus narrowband hyperspectral indices to discriminate the invasive Acacia longifolia within a mediterranean dune ecosystem. Remote Sens. (Basel). 8, 334. doi:10.3390/rs8040334
Hawthorne, T. L., Elmore, V., Strong, A., Bennett-Martin, P., Finnie, J., Parkman, J., et al. (2017). Mapping non-native invasive species and accessibility in an urban forest: a case study of participatory mapping and citizen science in atlanta, Georgia. Appl. Geogr. 56, 187–198. doi:10.1016/j.apgeog.2014.10.005
He, L., Chen, W., Leblanc, S. G., Lovitt, J., Arsenault, A., Schmelzer, I., et al. (2021). Integration of multi-scale remote sensing data for reindeer lichen fractional cover mapping in eastern canada. Remote Sens. Environ. 267, 112731. doi:10.1016/j.rse.2021.112731
Hellmann, C., Sutter, R., Rascher, K. G., Máguas, C., Correia, O., and Werner, C. (2011). Impact of an exotic N2-fixing Acacia on composition and N status of a native mediterranean community. Acta oecol. (Montrouge). 37 (1), 43–50. doi:10.1016/j.actao.2010.11.005
Hijmans, R. J., and Elith, J. (2021). Species distribution modeling. Available at: https://rspatial.org/raster/sdm/ (Accessed October 13, 2021).
Holden, P. B., Rebelo, A. J., and New, M. G. (2021). Mapping invasive alien trees in water towers: a combined approach using satellite data fusion, drone technology and expert engagement. Remote Sens. Appl. Soc. Environ. 21, 100448. doi:10.1016/j.rsase.2020.100448
Huang, C., and Asner, G. P. (2009). Applications of remote sensing to alien invasive plant studies. Sensors 9, 4869–4889. doi:10.3390/s90604869
Huete, A., Didan, K., Miura, T., Rodriguez, E. P., Gao, X., and Ferreira, L. G. (2002). Overview of the radiometric and biophysical performance of the MODIS vegetation indices. Remote Sens. Environ. 83, 195–213. doi:10.1016/S0034-4257(02)00096-2
Izquierdo-Verdiguier, E., and Zurita-Milla, R. (2020). An evaluation of guided regularized random forest for classification and regression tasks in remote sensing. Int. J. Appl. Earth Obs. Geoinf. 88, 102051. doi:10.1016/j.jag.2020.102051
Kaartinen, H., Hyyppä, J., Vastaranta, M., Kukko, A., Jaakkola, A., Yu, X., et al. (2015). Accuracy of kinematic positioning using global satellite navigation systems under forest canopies. Forests 6, 3218–3236. doi:10.3390/f6093218
Kattenborn, T., Lopatin, J., Förster, M., Braun, A. C., and Fassnacht, F. E. (2019). UAV data as alternative to field sampling to map woody invasive species based on combined Sentinel-1 and Sentinel-2 data. Remote Sens. Environ. 227, 61–73. doi:10.1016/j.rse.2019.03.025
Kotchenova, S. Y., Vermote, E. F., Matarrese, R., and Klemm, F. J. (2006). Validation of a vector version of the 6S radiative transfer code for atmospheric correction of satellite data. Part I: path radiance. Appl. Opt. 45 (26), 6762–6774. doi:10.1364/AO.45.006762
Kotchenova, S. Y., and Vermote, E. F. (2007). Validation of a vector version of the 6S radiative transfer code for atmospheric correction of satellite data. Part II. homogeneous lambertian and anisotropic surfaces. Appl. Opt. 46 (20), 4455–4464. doi:10.1364/AO.46.004455
Koutsias, N., Karteris, M., and Chuvieco, E. (2000). The use of Intensity-Hue-Saturation transformation of landsat-5 thematic mapper data for burned land mapping. Photogramm. Eng. Rem. S. 66, 829–839.
Kuhn, M., Wing, J., Weston, S., Williams, A., Keefer, C., Engelhardt, A., et al. (2021). Classification and regression training, R package version 6.0-88. Available at: https://github.com/topepo/caret/ (Accessed on November 28, 2021).
Le Maitre, D. C., Gaertner, M., Marchante, E., Ens, E.-J., Hokmes, P. M., Pauchard, A., et al. (2011). Impacts of invasive australian acacias: implications for management and restoration. Divers. Distrib. 17, 1015–1029. doi:10.1111/j.1472-4642.2011.00816.x
Lehrer, D., Becker, N., and Bar Kutiel, P. (2011). The economic impact of the invasion of Acacia saligna in Israel. Int. J. Sustain. Dev. World Ecol. 18 (2), 118–127. doi:10.1080/13504509.2011.554072
Leitão, P. J., Schwieder, M., Pötzschner, F., Pinto, J. R. R., Teixeira, A. M. C., Pedroni, F., et al. (2018). From sample to pixel: multi-scale remote sensing data for upscaling aboveground carbon data in heterogeneous landscapes. Ecosphere 9 (8), e02298. doi:10.1002/ecs2.2298
Linders, T. E. W., Schaffner, U., Eschen, R., Abebe, A., Choge, S. K., Nigatu, L., et al. (2019). Direct and indirect effects of invasive species: biodiversity loss is a major mechanism by which an invasive tree affects ecosystem functioning. J. Ecol. 107, 2660–2672. doi:10.1111/1365-2745.13268
Lou, P., Fu, B., He, H., Li, Y., Tang, T., Lin, X., et al. (2020). An optimized object-based random forest algorithm for marsh vegetation mapping using high-spatial-resolution GF-1 and ZY-3 data. Remote Sens. (Basel). 12, 1270. doi:10.3390/rs12081270
Louis, J., Pflug, B., Main-Knorn, M., Debaecker, V., Mueller-Wilm, U., Iannone, R. Q., et al. (2019). Sentinel-2 global surface reflectance level-2A product generated with Sen2cor. IEEE Int. Geosci. Remote Se, 8522–8525. doi:10.1109/IGARSS.2019.8898540
Lozano, V., Marzialetti, F., Carranza, M. L., Chapman, D., Branquart, E., Dološ, K., et al. (2020). Modelling Acacia saligna invasion in a large mediterranean island using PAB factors: a tool for implementing the European legislation on invasive species. Ecol. Indic. 116, 106516. doi:10.1016/j.ecolind.2020.106516
Malavasi, M., Santoro, R., Cutini, M., Acosta, A. T. R., and Carranza, M. L. (2013). What has happened to coastal dunes in the last half century? a multitemporal coastal landscape analysis in central Italy. Landsc. Urban Plan. 119, 54–63. doi:10.1016/j.landurbplan.2013.06.012
Marzialetti, F., Bazzichetto, M., Giulio, S., Acosta, A. T. R., Stanisci, A., Malavasi, M., et al. (2019). Modelling Acacia saligna invasion on the adriatic coastal landscape: an integrative approach using LTER data. Nat. Conserv. 34, 127–144. doi:10.3897/natureconservation.34.29575
Marzialetti, F., Di Febbraro, M., Malavasi, M., Giulio, S., Acosta, A. T. R., and Carranza, M. L. (2020). Mapping coastal dune landscape through spectral Rao’s Q temporal diversity. Remote Sens. (Basel). 12, 2315. doi:10.3390/rs12142315
Marzialetti, F., Frate, L., De Simone, W., Frattaroli, A. R., Acosta, A. T. R., and Carranza, M. L. (2021). Unmanned aerial vehicle (UAV)-based mapping of Acacia saligna invasion in the mediterranean coast. Remote Sens. (Basel). 13, 3361. doi:10.3390/rs13173361
Masemola, C., Cho, M. A., and Ramoelo, A. (2020). Sentinel-2 time series based optimal features and time window for mapping invasive australian native Acacia species in kwaZulu Natal, south africa. Int. J. Appl. Earth Obs. Geoinf. 93, 102207. doi:10.1016/j.jag.2020.102207
Maslin, B. R. (1974). Studies in the genus Acacia-3. The taxonomy of. A. saligna (Labill.) H. Wendl. Nuytsia 1 (4), 332–340.
Mathieu, R., Pouget, M., Cervelle, B., and Escadafal, R. (1998). Relationships between satellite-based radiometric indices simulated using laboratory reflectance data and typic soil color of an arid environment. Remote Sens. Environ. 66, 17–28. doi:10.1016/S0034-4257(98)00030-3
McFeeters, S. K. (1996). The use of the normalized difference water index (NDWI) in the delineation of open water features. Int. J. Remote Sens. 17 (7), 1425–1432. doi:10.1080/01431169608948714
McGaughey, R. J., Ahmed, K., Andersen, H.-E., and Reutebuch, S. E. (2017). Effect of occupation time on the horizontal accuracy of a mapping-grade GNSS receiver under dense forest canopy. Photogramm. Eng. remote Sens. 83 (12), 861–868. doi:10.14358/PERS.83.12.861
Meyer, H., Lehnert, L. W., Wang, Y., Reudenbach, C., Nauss, T., and Bendix, J. (2017). From local spectral measurement to maps of vegetation cover and biomass on the qinghai-tibet-plateau: do we need hyperspectral information? Int. J. Appl. Earth Obs. Geoinf. 55, 21–31. doi:10.1016/j.jag.2016.10.001
Milton, S. J., and Moll, E. (1982). Phenology of australian acacias in the S.W. cape, south africa, and its implications for management. Bot. J. Linn. Soc. 84, 295–327. doi:10.1111/j.1095-8339.1982.tb00367.x
Morris, T. L., Esler, K. J., Barger, N. N., Jacobs, S. M., and Cramer, M. D. (2011). Ecophysiological traits associated with the competitive ability of invasive australian acacias. Divers. Distrib. 17, 898–910. doi:10.1111/j.1472-4642.2011.00802.x
Müllerová, J., Brůna, J., Bartaloš, T., Dvořák, P., Vítková, M., and Pyšek, P. (2017). Timing is important: unmanned aircraft vs. satellite imagery in plant invasion monitoring. Front. Plant Sci. 8, 887. doi:10.3389/fpls.2017.00887
Nativ, R., Ephrath, J. E., Berliner, P. R., and Saranga, Y. (1999). Drought resistance and water use efficiency in Acacia saligna. Aust. J. Bot. 47, 577–586. doi:10.1071/BT98022
Nembrini, S., König, I. R., and Wright, M. N. (2018). The revival of the gini importance? Bionformatics 34 (21), 3711–3718. doi:10.1093/bioinformatics/bty373
Niphadkar, M., Nagendra, H., Tarantino, C., Adamo, M., and Blonda, P. (2017). Comparing pixel and object-based approaches to map an understorey invasive shrub in tropical mixed forests. Front. Plant Sci. 8, 892. doi:10.3389/fpls.2017.00892
Nsikani, M. M., Novoa, A., Van Wilgen, B. W., Keet, J.-H., and Gaertner, M. (2017). Acacia saligna’s soil legacy effects persist up to 10 years after clearing: implications for ecological restoration. Austral Ecol. 42 (8), 880–889. doi:10.1111/aec.12515
Paz-Kagan, T., Caras, T., Heermann, I., Shachak, M., and Karnieli, A. (2017). Multiscale mapping of species diversity under changed land use using imaging spectroscopy. Ecol. Appl. 27 (5), 1466–1484. doi:10.1002/eap.1540
Paz-Kagan, T., Silver, M., Panov, N., and Karnieli, A. (2019). Multispectral approach for identifying invasive plant species based on flowering phenology characteristics. Remote Sens. (Basel). 11, 953. doi:10.3390/rs11080953
Peñuelas, J., Baret, F., and Filella, I. (1995). Semi-empirical indices to assess carotenoids/chlorophyll a ratio from leaf spectral reflectance. Photosynthetica 31 (2), 221–230.
Pettorelli, N., Laurence, W. F., O’Brien, T. G., Wegmann, M., Nagendra, H., and Turner, W. (2014). Satellite remote sensing for applied ecologists: opportunities and challenges. J. Appl. Ecol. 51, 839–848. doi:10.1111/1365-2664.12261
Piiroinen, R., Fassnacht, F. E., Heiskanen, J., Maeda, E., Mack, B., and Pellikka, P. (2018). Invasive tree species detection in the eastern arc mountains biodiversity hotspot using one class classification. Remote Sens. Environ. 218, 119–131. doi:10.1016/j.rse.2018.09.018
Probst, P., Wright, M. N., and Boulesteix, A. L. (2018). Hyperparameters and tuning strategies for random forest. WIREs Data Min. Knowl. Discov. 9, e1301. doi:10.1002/widm.1301
Pyšek, P., Hulme, P. E., Simberloff, D., Bacher, S., Balckburn, T. M., Carlton, J. T., et al. (2020). Scientists’ warning on invasive alien species. Biol. Rev. 95, 1511–1534. doi:10.1111/brv.12627
Riihimäki, H., Luoto, M., and Heiskanen, J. (2019). Estimating fractional cover of tundra vegetation at multiple scales using unmanned aerial systems and optical satellite data. Remote Sens. Environ. 224, 119–132. doi:10.1016/j.rse.2019.01.030
Rouse, J. W., Haas, R. H., Schell, J. A., and Deering, D. W. (1973). Monitoring vegetation systems in the great plains with ERTS. NASA, Third ERTS Symp. 1, 309
Routh, D., Seegmiller, L., Bettigole, C., Khun, C., Oliver, C. D., and Glick, H. B. (2018). Improving the reliability of mixture tuned matched filtering remote sensing classification results using supervised learning algorithms and cross-validation. Remote Sens. (Basel). 10, 1675. doi:10.3390/rs10111675
Royimani, L., Mutanga, O., Odindi, J., Dube, T., and Matongera, T. N. (2019). Advancements in satellite remote sensing for mapping and monitoring of alien invasive plant species (AIPs). Phys. Chem. Earth Parts A/B/C 112, 237–245. doi:10.1016/j.pce.2018.12.004
Shoko, C., Mutanga, O., and Dube, T. (2020). Remotely sensed characterization of Acacia longifolia invasive plants in the Cape floristic region of the western cape, South Africa. J. Appl. Remote Sens. 14 (4), 044511. doi:10.1117/1.JRS.14.044511
Simberloff, D., Martin, J.-L., Genovesi, P., Maris, V., Wardle, D. A., Aronson, J., et al. (2013). Impacts of biological invasions: what’s what and the way forward. Trends Ecol. Evol. 28 (1), 58–66. doi:10.1016/j.tree.2012.07.013
Souza-Alonso, P., Rodríguez, J., González, L., and Lorenzo, P. (2017). Here to stay. recent advances and perspectives about Acacia invasion in mediterranean areas. Ann. For. Sci. 74 (55), 55–20. doi:10.1007/s13595-017-0651-0
Stanisci, A., Acosta, A. T. R., Carranza, M. L., de Chiro, M., Del Vecchio, S., Di Martino, L., et al. (2014). EU habitats monitoring along the coastal dunes of the LTER sites of abruzzo and molise (Italy). Plant Sociol. 51, 51–56. doi:10.7338/pls2014512S1/07
Starfinger, U., and Schrader, G. (2021). Invasive alien plants in plant health revisited: another 10 years. EPPO Bull. 00, 632–638. doi:10.1111/epp.12787
Thompson, G. D., Bellstedt, D. U., Richardson, D. M., Wilson, J. R. U., and Le Roux, J. J. (2015). A tree well travelled: global genetic structure of the invasive tree Acacia saligna. J. Biogeogr. 42, 305–314. doi:10.1111/jbi.12436
Tozzi, F. P., Carranza, M. L., Frate, L., and Stanisci, A. (2021). The impact of Acacia saligna on the composition and structure of the mediterranean maquis. Biodiversity 22 (1-2), 53–66. doi:10.1080/14888386.2021.1936640
Tu, T.-M., Lee, Y.-C., Chang, C.-P., and Huang, P. S. (2005). Adjustable intensity-hue-saturation and brovey transform fusion technique for IKONOS/ QuickBird imagery. Opt. Eng. 44, 116201. doi:10.1117/1.2124871
Tucker, C. J., Elgin, J. H., McMurtrey, J. E., and Fan, C. J. (1979). Monitoring corn and soybean crop development with hand-held radiometer spectral data. Remote Sens. Environ. 8, 237–248. doi:10.1016/0034-4257(79)90004-X
Tucker, C. (1979). Red and Photographic infrared linear combinations for monitoring vegetation. Remote Sens. Environ. 8, 127–150. doi:10.1016/0034-4257(79)90013-0
Underwood, E., Ustin, S., and Di Pietro, D. (2003). Mapping nonnative plants using hyperspectral imagery. Remote Sens. Environ. 86, 150–161. doi:10.1016/S0034-4257(03)00096-8
Vilà, M., and Hulme, P. E. (2017). Impact of biological invasions on ecosystem services. Cham, Switzerland: Springer Nature.
Vincini, M., Frazzi, E., and D’Alessio, P. (2008). A broad-band leaf chlorophyll vegetation index at the canopy scale. Precis. Agric. 9, 303–319. doi:10.1007/s11119-008-9075-z
Wijesingha, J., Astor, T., Schulze-Brüninghoff, D., and Wachendorf, M. (2020). Mapping invasive Lupinus polyphyllus Lindl. in semi-natural grasslands using object-based image analysis of UAV-borne images. PFG 88, 391–406. doi:10.1007/s41064-020-00121-0
Yang, X., Smith, A. M., Bourchier, R. S., Hodge, K., and Ostrander, D. (2020). Flowering leafy spurge (Euphorbia esula) detection using unmanned aerial vehicle imagery in biological control sites: impacts of fligh heigh, flight time and detection method. Weed Technol. 34 (4), 575–588. doi:10.1017/wet.2020.8
Yelenik, S. G., Stock, W. D., and Richardson, D. M. (2004). Ecosystem level impacts of invasive Acacia saligna in the south african fynbos. Restor. Ecol. 12 (1), 44–51. doi:10.1111/j.1061-2971.2004.00289.x
Zhang, Y., Li, C., Jiang, X., Zhang, S., Wu, Y., Liu, B., et al. (2004). Human placenta-derived mesenchymal progenitor cells support culture expansion of long-term culture-initiating cells from cord blood CD34+ cells. Exp. Hematol. 70 (6), 657–664. doi:10.1016/j.exphem.2004.04.001
Keywords: upscaling, invasive alien plants, Sentinel-2, PlanetScope, environmental monitoring, random forest model, coastal dune landscapes
Citation: Marzialetti F, Di Febbraro M, Frate L, De Simone W, Acosta ATR and Carranza ML (2022) Synergetic use of unmanned aerial vehicle and satellite images for detecting non-native tree species: An insight into Acacia saligna invasion in the Mediterranean coast. Front. Environ. Sci. 10:880626. doi: 10.3389/fenvs.2022.880626
Received: 21 February 2022; Accepted: 07 July 2022;
Published: 08 August 2022.
Edited by:
Thomas Campagnaro, University of Padua, ItalyReviewed by:
M Arasumani, University of Greifswald, GermanyFrancesco Chianucci, Council for Agricultural and Economics Research (CREA), Italy
Joseph J Erinjery, Kannur University, India
Vahid Nasiri, University of Tehran, Iran
Copyright © 2022 Marzialetti, Di Febbraro, Frate, De Simone, Acosta and Carranza. This is an open-access article distributed under the terms of the Creative Commons Attribution License (CC BY). The use, distribution or reproduction in other forums is permitted, provided the original author(s) and the copyright owner(s) are credited and that the original publication in this journal is cited, in accordance with accepted academic practice. No use, distribution or reproduction is permitted which does not comply with these terms.
*Correspondence: Mirko Di Febbraro, bWlya28uZGlmZWJicmFyb0B1bmltb2wuaXQ=; Alicia Teresa Rosario Acosta, YWxpY2lhdGVyZXNhcm9zYXJpby5hY29zdGFAdW5pcm9tYTMuaXQ=