- 1School of Forestry and Biotechnology, Zhejiang A&F University, Hangzhou, China
- 2School of Humanities and Law, Zhejiang A&F University, Hangzhou, China
Predicting the spatial distribution of species in relation to suitable areas under global climate change could provide some references for conservation and long-term management strategies for the species. In this study, the MaxEnt was optimized by adjusting the feature combination and regulation magnification parameters with the ENMeval data package. Based on 127 Cremastra appendiculata spatial distribution locations and 14 environmental factors, the potential distribution areas of C. appendiculata under the present and future climate conditions (2050s, 2070s) were simulated, and the dominant environmental factors influencing the spatial distribution of C. appendiculata were analyzed. The feature combination (FC) and the regularization multiplier (RM) were selected as per the Akaike information criterion (AIC). The model showed complexity and degree of over-fitting (delta AICc = 0, omission rate = 0.106, the difference in the curve values between the training and testing areas was 0.021) after establishing the optimal model (FC = LQH and RM = 2.5), and the results indicated that the optimal model performed well in simulating the potential spatial distribution of C. appendiculata (the area under the receiver operating characteristic curve = 0.933). The results showed that the suitable habitat of C. appendiculata currently in China is 187.60 × 104 km2, while the highly suitable habitat is 118.47 × 104 km2, the moderately suitable habitat is 53.25 × 10 4 km2, and the poorly suitable habitat is 15.88 × 104 km2. There is an increasing trend in the suitable habitat of C. appendiculata under six climate scenarios, including SSP1-2.6, SSP2-4.5, and SSP5-8.5 in the 2050s and the 2070s, and that habitat will extend to the northwest as a whole. The highly suitable habitat of C. appendiculata in nature reserves is 0.47 × 104 km2; consequently, there is a large gap in the protection of C. appendiculata. The distribution of C. appendiculata was influenced by the temperature, precipitation, and normalized vegetation index.
Introduction
Currently, global warming is one of the major environmental problems the world is facing (Akbar et al., 2021; Dai et al., 2021). It is expected that by 2100, global temperatures will rise by 1.4 to 5.8°C compared with 1900, two- to ten- fold higher than that in the twentieth century, and the average temperature will increase 2.2°C by 2050 in China; global average temperature might rise 3.7°C by the end of twenty-first century under the scenarios of Representative Concentration Paths (RCPs), according to the fifth assessment report of the Intergovernmental Panel on Climate Change (Yan et al., 2021). The growth and development, spatial distribution, and population size of plants will be influenced by a globally changing climate (Ouyang et al., 2021). Increasingly, studies have demonstrated that the range of suitable habitats for plants will expand, contract, or migrate under the effect of global climate change. For example, a warming climate may decrease the range of suitable habitats for Semiliquidambar cathayensis (Hamamelidaceae), making them migrate to higher altitude and higher latitude areas (Ye et al., 2020); the highly suitable habitat of the deciduous shrub Hydrangea macrophylla showed an increasing trend, and the total suitable habitat expands to the north with climate warming (Yan et al., 2021). Therefore, exploring the relationship between species distribution and climate factors and predicting the changes in species distribution patterns in different climate scenarios at present and in the future have become a hot topic in biogeography and ecology (Chakraborty et al., 2016; Wang et al., 2016; Dyderski., 2018).
With the deeper study of the development of geographic information science, species distribution models (SDMs) have provided the most commonly used approach to examining the influence of a changing climate on the appropriate habitats for species (Descombes et al., 2015). According to the ecological principle called the niche theory along with its specific algorithm, the niche characteristics of the plant are indicated by spatial distribution and environmental variables, to forecast the appropriate area of the plant (Guisan and Zimmermann, 2000; Phillips et al., 2006). The development of the species distribution model provides effective methods for the research of environmental science (Du et al., 2017), the protection of biodiversity (Remya et al., 2015), and the defense of invasive species (Luizza et al., 2016) among other benefits. There is a variety of species distribution models based on different algorithms, such as CLIMEX (Sutherst and Maywald, 1985), GARP (Stockwell et al., 2006), ENFA (Hirzel et al., 2002), DOMAIN (Carpenter et al., 1993), and MaxEnt (Philips et al., 2006), among the existing models. The MaxEnt model has provided accurate predictions, even when there are few species distribution records to work with (Kumar and Stohlgren, 2009). The MaxEnt model has been widely used in the field of species distribution research and to study the suitable distribution of Chinese Ziziphus jujube (Zhao et al., 2021a), Semiliquidambar cathayensis (Ye et al., 2020), and Panax notoginseng (Zhan et al., 2022). Recent studies have demonstrated the utility of the MaxEnt model for simulating the possible distribution of species, and the complexity is often high, which is not conducive to model transfer. However, the ENMeval data package was used to adjust the MaxEnt model parameters, which can better forecast the potential suitable area of the species (Zhao et al., 2021b).
Cremastra appendiculata (Orchidaceae), contained in the Chinese pharmacopeia, is an endangered and rare plant species in China (Mao et al., 2018). C. appendiculata (“山慈菇” in Chinese) is a herbal traditional Chinese medicine with a spatial distribution encompassing the Yellow River Basin and most of southern China; the herb commonly flourishes in gully wetlands or forest wetlands at an altitude ranging from 500 to 2,900 m (Liu et al., 2021a). It is sweet, slightly pungent, and cool with the effects of clearing heat and detoxification, resolving phlegm, and dispersing snot; it is mainly used to treat carbuncles, swelling, toxins, phlegm nucleus and lymph node cancer, and snake and insect bites (Chinese Pharmacopoeia Commission, 2010; Liu et al., 2021b). Due to the increasing demand for C. appendiculata, its wild resources can no longer meet people’s needs, and the species is in a threatened or endangered condition (Zhang et al., 2006). It has been listed in publications of the Convention on International Trade in Endangered Species (CITES) (Hinsley et al., 2018). Therefore, it is important to protect C. appendiculata resources. Recently, because of the unique physiological and pharmacological characteristics, more researchers have been paying attention to C. appendiculata increasingly in the world. Up to now, there are some studies on its rapid propagation (Zhang et al., 2006), population quantity and genetic drift (Chung et al., 2004), chemical compositions (Ikeda et al., 2005), and pharmacological action (Yang et al., 2010). However, there is no research on the potential suitable distribution of the endangered C. appendiculata in China under global climate change. The MaxEnt model was utilized to simulate the potential spatial distribution of C. appendiculata according to climatic scenarios (SSP1-2.6, SSP2-4.5, and SSP5-8.5) for both the current period (1970–2000) and the future: the 2050s (2041–2060) and the 2070s (2061–2080). The current study aimed to improve the understanding of the distributional property and ecological suitability of C. appendiculata and act as a theoretical reference for the conservation and use of wild C. appendiculata. The objectives of the study were to (a) identify the patterns of spatial distribution and future changes in C. appendiculata in China; (b) identify the relevant ecological factors inhibiting C. appendiculata distribution; (c) predict the suitable distribution of C. appendiculata and the trajectory of its centroid distribution under present and future scenarios of climate change; and (d) provide rational evidence to facilitate the conservation and introduction of C. appendiculata resources.
Materials and Methods
Occurrence Data for Cremastra appendiculata
Data for the geographic distribution of C. appendiculata were obtained from databases such as the Global Biodiversity Information Platform (https://www.gbif.org/) and the China National Specimen Information Infrastructure (www.nsii.org.cn/) and the distribution location of C. appendiculata from consulting-related documents. After excluding duplicate distribution points and sample records with unclear geographical locations and times, we obtained the corresponding longitude and latitude coordinates through Google Maps and deleted the data less than 0.4 from the obtained longitude and latitude. Finally, 127 distribution points in China were determined.
Environmental Data
The WorldClim dataset provided bioclimatic data (Version 2.0, www.worldclim.org/) for the period 1970–2000 and for future climate data (2050s: 2041–2060; 2070s: 2061–2080) with 2.5’ spatial resolution. We used general circulation model (GCM) predictions under shared social-economic paths (SSPs) proposed by the Coupled Model Intercomparison Project Phase 6 (CMIP6) of IPCC to predict the future climate change (Popp et al., 2017). We selected the Beijing Climate Center Climate System Model (BCC-CSM2-MR) as the climate system model, which was developed at the National Climate Center because it demonstrates the best performance in East Asia (Xin et al., 2013; Wu et al., 2019). BCC-CSM2-MR alone was usually used in China to predict species distributions (Zhang et al., 2021). SSP1-2.6, SSP2-4.5, SSP3-7.0, and SSP5-8.5 represent a low-forcing, medium-forcing, medium-to-high-forcing, and high-forcing scenario, respectively (Fischer et al., 2005). We chose SSP1–2.6, SSP2–4.5, and SSP5–8.5 representative of the minimum, moderate, and maximum greenhouse emission scenarios, respectively, to predict the potential spatial distribution of C. appendiculata. LUCC data were obtained from the Chinese Academy of Sciences Resource and Environmental Science Data Center (http://www.resdc.cn). Topographic data were obtained from the United Nations Food and Agriculture Organization (FAO) Soil Map and Database v1.2 World Soil Database (http://www.fao.org/soils-portal/soil-survey/soil-maps-and-databases/harmonized-world-soil-database-v12-en).
The MaxEnt model will produce over-fitted simulations because various ecological factors show collinearity relationships (Graham, 2003). The Spearman coefficient method was used to minimize the overfitting of model predictions by identifying correlations between 28 ecological factors. If factors with a correlation coefficient were greater than or equal to 0.8, the most ecologically significant factors were retained (Liu and Shi, 2020). Finally, 14 environmental variables were selected from 28 environmental variables to identify the potential spatial distributions of C. appendiculata and to determine key environmental variables (Table 1).
Model Parameter Optimization and Model Building
The potential distribution of C. appendiculata over different periods was predicted using the MaxEnt; the two parameters of feature combination (FC) and regulation magnification (RM) were adjusted by the ENMeval package. There are five feature combinations in the MaxEnt model, namely, linear features, quadratic features, product features, threshold features, and hinge features. The default parameters of MaxEnt are RM = 1 and FC = LQHPT. For the future optimization model, we set RM to 0.5–4, increased 0.5 each time, and adopted six feature combinations, namely, L, LQ, H, LQH, LQHP, and LQHPT (Muscarella et al., 2014). The distribution position of C. appendiculata was divided into four parts by the ENMeval data package, of which three parts were used for training and one was used for testing. The 48 parameter combinations mentioned earlier were assessed using the ENMeval data package, as per the change of the Akaike information criterion (△AICC). The differences between the training AUC and the test AUC (AUC. DIFF) and the 10% training omission rate (OR10) were utilized to assess the performance of the model (Phillips et al., 2017).
The 127 distribution points of C. appendiculata were imported into MaxEnt model software; three quarters of the samples were chosen for model training, with the remaining samples being used for verification (Zhang et al., 2020). The maximum iteration number was set to 10,000, and the operation was repeated 10 times (Zhan et al., 2022). The area under the receiver operating characteristic curve (AUC) was utilized to assess the model prediction accuracy. The value of AUC was 0–1, depending on how close the AUC value was to 1, and the prediction result of the model was more accurate (Hanley and McNeil, 1982; Swets, 1988; Dai et al., 2019).
Classification of Suitable Habitat
We used ArcGIS 10.5 (Esri, Redlands, California, United States) to process the suitability classification and visualization of C. appendiculata. We quantified the potential distribution probability of C. appendiculata from 0 to 1, and four groups were reclassified, following the method used by Yang et al. (2013); the habitat suitability level of C. appendiculata was divided into four categories: unsuitable (0–0.2), poorly suitable (0.2–0.4), moderately suitable (0.4–0.6), and highly suitable (0.6–1) habitats. The differences in the suitable areas of C. appendiculata were compared in different periods to obtain a map of the distribution patterns of C. appendiculata under different periods of the scenarios of climate change.
Analysis of the Multivariate Environmental Similarity Surface
The Multivariate Environmental Similarity Surface (MESS) is an indicator used to express how similar a site in space is to a set of reference points relative to a set of predictors. As the S value is positive, the smaller the S values, the more significant is the climate difference at the point. If the maximum value of the multi-environmental similarity is 100, no difference exists. A negative value indicates that the value of at least one climate variable at the site is beyond the range of the corresponding value of the reference layer. The environmental change at the reference point is good (Elith et al., 2010). The operation was performed by running the “density. Tools. Novel” tool in the MaxEn Jar file in the command window.
Results
Generation of the Optimal Model and Assessment of the Model Accuracy
The potential spatial distribution of C. appendiculata in China was simulated using the MaxEnt model. The delta.AICc of 109.59 was obtained with the model set, according to default parameters. However, a delta.AICc of 0 was obtained when the model was established with the optimized parameters (FC = LQH and RM = 2.5) (Figure 1; Table 2). The AUC.DIFF and omission rate decreased by 67.19% and 63.32%, respectively, indicating lower over-fitting of the optimized parameters than the default parameters. The model was reconstructed using the optimized parameters (FC = LQH and RM = 2.5), after which the model was used to simulate the suitable spatial distribution of C. appendiculata in China. Under this model, the average AUC under training reached 0.933 (Supplementary Figure S1). The software simulations confirmed the high accuracy of the MaxEnt model for predicting the spatial distribution of C. appendiculata in China.
Current Potential Distribution
The main distribution areas of C. appendiculata are in southern China, which cover parts of Yunnan, Tibet, Sichuan, Chongqing, Guangxi, Guangdong, Gansu, Shaanxi, Zhejiang, Jiangsu, Anhui, Hubei, Henan, Shanxi, Hebei, and Jiangsu (Figure 2). Most of the screened sample points showed a scattered distribution in areas of suitable C. appendiculata habitat. This result confirmed that the model can predict the potential spatial distribution of C. appendiculata. The area of current potential suitable habitats for C. appendiculata in China is 187.60 × 104 km2 (Supplementary Figure S2). For C. appendiculata in China, the highly suitable habitat is 118.47 × 104 km2, particularly distributed in east-central Sichuan, central Guizhou, southern Hunan, southern Jiangxi, and northern Zhejiang; the moderately suitable habitat is 53.25× 104 km2 (Supplementary Figure S2), which is mainly located in southern Hunan, northern and central Guizhou, southern Jiangxi, eastern Sichuan, northern Guizhou, northern Yunnan, eastern Hubei, and central Shaanxi; and the lowly suitable habitat is 15.88 × 104 km2 (Supplementary Figure S2), especially located in southeastern Tibet, central and southern Yunnan, northern Guangxi, northern and eastern Guangdong, southern Zhejiang, southern Jiangxi, southern Hunan, eastern Sichuan, northern Hubei, and eastern Shandong.
Future Potential Distribution
The distribution map of potentially suitable habitats for C. appendiculata in China under six different scenarios of future climate change appears in Figure 3. As shown in Supplementary Figure S2, by the 2050s, for SSP1-2.6, the highly suitable habitat for C. appendiculata will represent 13.63% of the total land area of China, and it will be increased by 10.47% than that under modern climate conditions. By the 2050s, for SSP2-4.5, the highly suitable habitat for C. appendiculata will represent 16.31% of the total land area of China, and it will be increased by 32.15% than that under modern climate conditions. By the 2050s, for SSP5-8.5, the highly suitable habitat for C. appendiculata will represent 15.74% of the total land area of China, and it will be increased by 27.57% than that under modern climate conditions. By the 2050s, for SSP1-2.6, the moderately suitable habitat for C. appendiculata will represent 5.27% of the total land area of China, and the moderately appropriate habitat of C. appendiculata will be increased by 0.09% than that under modern climate conditions. By the 2050s, for SSP2-4.5, the moderately suitable habitat for C. appendiculata will represent 5.82% of the total land area of China, and it will be increased by 4.94% than that under modern climate conditions. By the 2050s, for SSP5-8.5, the C. appendiculata moderately suitable habitat will represent 5.4% of the total land area of China, and it will be decreased by 2.65% than that under modern climate conditions. By the 2050s, for SSP1-2.6, the total suitable C. appendiculata habitat will represent 20.44% of the total land area of China, and it will be increased by 4.39% than that under modern climate conditions. By the 2050s, for SSP2-4.5, the total suitable habitat for C. appendiculata will represent 23.85% of the total land area of China, and the most appropriate habitat of C. appendiculata will be increased by 18.06% than that under modern climate conditions. By the 2050s, for SSP5-8.5, the total suitable habitat for C. appendiculata will represent 22.74% of the total land area of China, and it will be increased by 14.05% than that under modern climate conditions. In a word, the potential distribution for C. appendiculata under different scenarios of climate change in the 2050s showed an increasing trend (Figure 3).
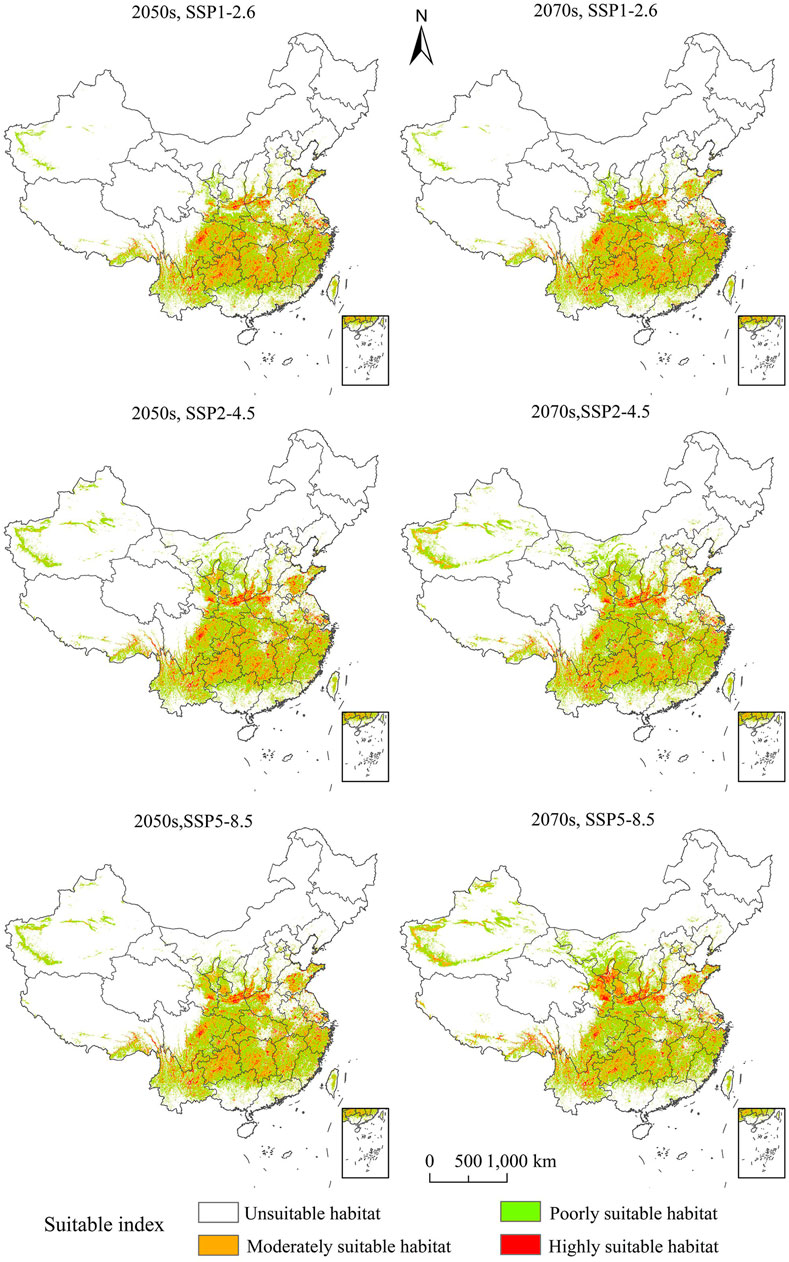
FIGURE 3. Potential spatial distribution of Cremastra appendiculata under future scenarios of climate change.
By the 2070s, for SSP1-2.6, the most suitable habitat area for C. appendiculata will represent 13.71% of the total land area of China, and it will be increased by 11.11% than that under modern climate conditions. By the 2070s, for SSP2-4.5, the most suitable habitat for C. appendiculata will represent 17.83% of the total land area of China, and it will be increased by 44.47% than that under modern climate conditions. By the 2070s, for SSP5-8.5, the most suitable habitat for C. appendiculata will represent 18.22% of the total land area of China, and it will be increased by 47.64% than that under modern climate conditions. By the 2070s, for SSP1-2.6, the moderately suitable habitat for C. appendiculata will represent 5.55% of the total land area of China, and it will be increased by 0.09% than that under modern climate conditions. By the 2070s, for SSP2-4.5, the moderately suitable habitat for C. appendiculata will represent 5.79% of the total land area of China, and it will be increased by 4.38% than that under modern climate conditions. By the 2070s, for SSP5-8.5, the moderately suitable habitat for C. appendiculata will represent 5.97% of the total land area of China, and it will be increased by 7.61% than that under modern climate conditions. By the 2070s, for SSP1-2.6, the total suitable habitat for C. appendiculata will represent 20.89% of the total land area of China, and it will be increased by 5.74% than that under modern climate conditions. By the 2070s, for SSP2-4.5, the total suitable habitat for C. appendiculata will represent 26.02% of the total land area of China, and the most suitable habitat of C. appendiculata will increase by 18.06% than that under modern climate conditions. By the 2070s, for SSP5-8.5, the suitable habitat for C. appendiculata will represent 22.74% of the total land area of China, and it will be increased by 24.91% than that under modern climate conditions. Generally speaking, the potential spatial distribution for C. appendiculata under different climate scenarios of climate change in the 2070s will show an increasing trend (Figure 3).
It can be seen in Supplementary Figure S3 that in the 2070s, for SSP1-2.6, the area of lost suitable habitat for C. appendiculata will be the smallest, at 8.36 × 104 km2. In the 2050s, for SSP1-2.6, the area in which the suitable habitat of C. appendiculata increases is the smallest, which will be 18.73 × 104 km2. For the SSP5-8.5 scenario during the 2070s, the gained area and lost area of C. appendiculata will be the largest; the area of the gained area will be 88.18 × 104 km2, and the area of the lost area will be 25.19 × 104 km2, respectively. The gained area of C. appendiculata under climate change will be greater than the lost area, indicating that the potential area of spatial distribution of C. appendiculata will also increase (Figure 4).
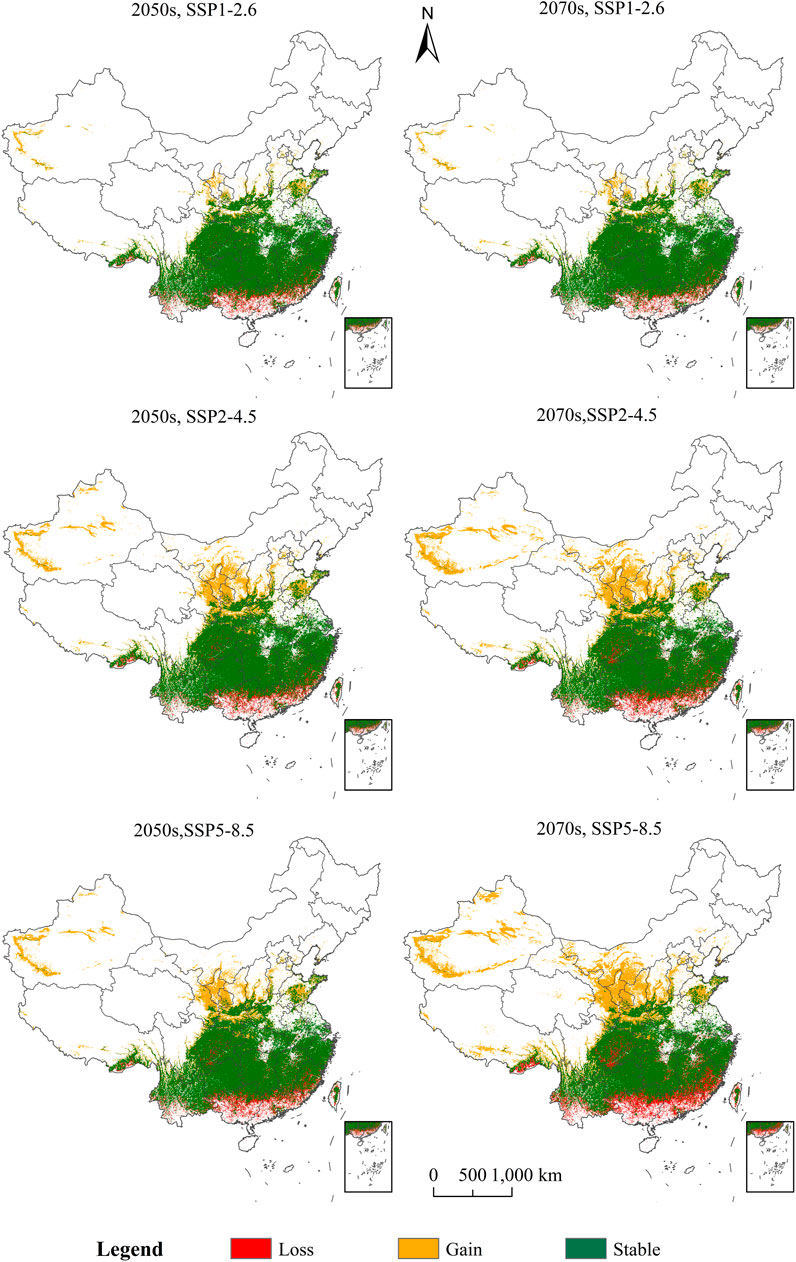
FIGURE 4. Changes in the potential spatial distribution of Cremastra appendiculata under scenarios of climate change.
Change Trend of Shifts in the Centroid Area Distribution of the Highly Suitable Area for Cremastra appendiculata
The highly suitable habitat of C. appendiculata in different periods was extracted by ArcGIS software, and the centroid position of the most suitable habitat was calculated by ArcGIS. The method of Yue et al. (2011) was used to calculate the centroid direction, displacement, and distance of the most suitable habitat. The C. appendiculata current habitat centroid appears in northwest Hunan at 110°10′E and 28°50′N (Figure 5). There is a shift in the centroid of the suitable habitat of 109° 58′E and 29°42′N in the 2050s and 109°50′E and 29°38′N in the 2070s under SSP1-2.6. There is a shift in the centroid of the suitable habitat of 108°39′E and 30°63′N in the 2050s and 109°50′E and 29°38′N in the 2070s under SSP2-4.5. Under SSP5-8.5, the centroid of the suitable habitat will shift to 108°48′E and 30°39′N in the 2050s and 106° 83′E and 31°87′N in the 2070s. In summary, we observed that the core distribution of C. appendiculata will shift to the northwest under future emission trajectories (SSP1-2.6, SSP2-4.5, and SSP5-8.5).
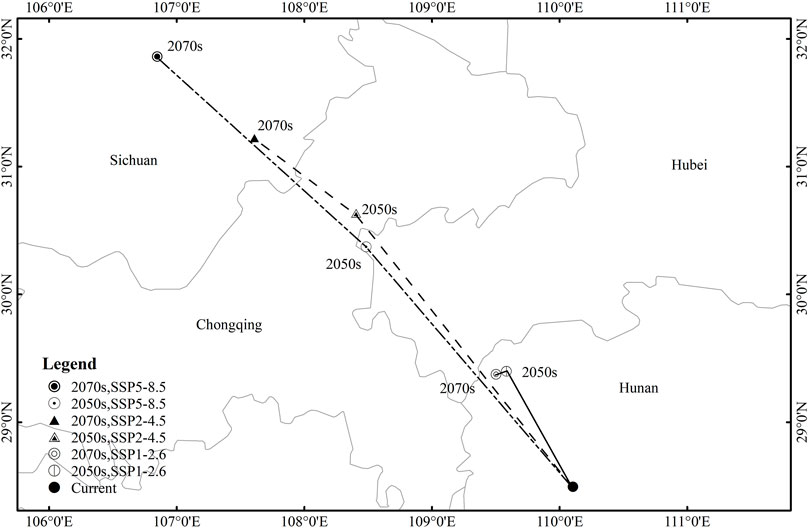
FIGURE 5. Shifts in the centroid area distributions for Cremastra appendiculata highly suitable area under different scenarios of climate change.
Analysis of the Multivariate Environmental Similarity Surface of Cremastra appendiculata Potential Distribution Areas Under Future Climate Changes
The area of potential distribution of the climate anomaly area (S < 0) is small in the whole potential distribution area under the future climate scenario (Figure 6). No suitable habitat area for C. appendiculata was predicted in the abnormal climate zone compared with the potential distribution area under the same climate scenario during the same period. The average similarities of the 127 valid distribution record points of C. appendiculata were 8.01, 7.58, and 6.99 in the 2050s under SSP1-2.6, SSP2-4.5, and SSP5-8.5, respectively, and 6.21, 5.85, and 4.14 in the 2070s under SSP1-2.6, SSP2-4.5, and SSP5-8.5, respectively. It shows that the degree of anomaly in the 2070s under SSP5-8.5 is the highest.
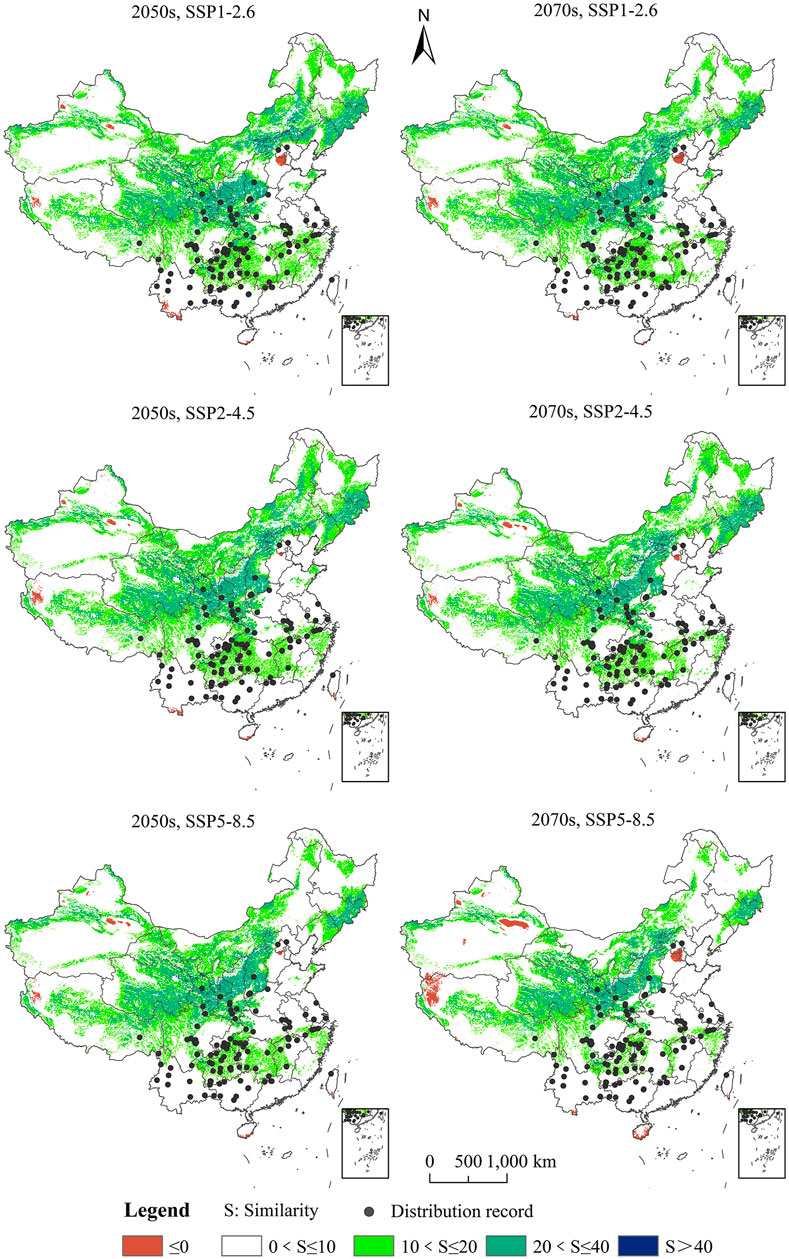
FIGURE 6. Analysis of the Multivariate Environmental Similarity Surface (MESS) of the potential distribution area for Cremastra appendiculata under future climate changes.
Gap Analysis
In ArcGIS 10 2, the systematic conservation planning map of C. appendiculata is superimposed and analyzed on the vector boundary of China’s nature reserve to obtain its conservation vacancy map. Gap analysis is carried out to identify its conservation vacancy area (Figure 7), thus providing a theoretical reference for its scientific protection and management. According to the gap analysis, there are almost no nature reserves. The area of the most appropriate habitat of C. appendiculata in the reserve was 0.47 × 104 km2, there is a large gap in the protection of C. appendiculata, and there are few areas appropriate for growth in the protection area, which need to be protected urgently.
Environmental Variable Analysis
Among the 14 environmental variables predicted by MaxEnt (Table 3), the top three variables and their rates of contribution are as follows: the lowest temperature of the coldest month (bio6, 63.9% contribution rate), the wettest seasonal rainfall (bio16, 13.8%), and the normalized vegetation index (NDVI, 11.8%). These variables represented almost 89.5 of the protections (Table 3). The jackknife test also confirmed that the lowest temperature of the coldest month (bio6) is the most influential environmental factor, followed by the wettest seasonal rainfall (bio16) and precipitation seasonality (coefficient of variation) (bio15) when using only a single environmental factor (Supplementary Figure S4), suggesting that these environmental factors are important for the growth suitability of C. appendiculata under modern climatic conditions. The response curves of the dominant environmental variables (Supplementary Figure S5) indicated the most suitable habitat for C. appendiculata as follows: the lowest temperature of the coldest month (bio6) was −3–6°C, the wettest seasonal rainfall (bio16) was 480–1300 mm, precipitation seasonality (coefficient of variation) (bio15) was 45–75, and the normalized vegetation index was 0.35–0.8. Climates suitable for the distribution of C. appendiculata are characterized by cold and humid climate conditions. However, C. appendiculata still showed a low tolerance to temperature.
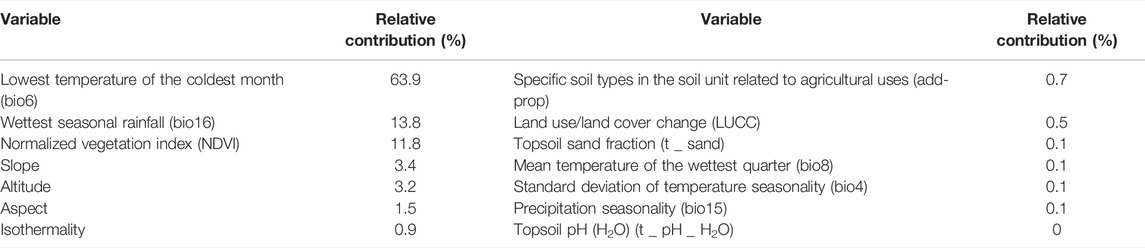
TABLE 3. Proportional contributions of environmental predictors on the possible spatial distribution of C. appendiculata in China.
Discussion
Climate, soil, terrain, and human factors will affect the spatial distribution of species. In order to consider the environmental factors affecting species comprehensively, distribution, climate, terrain, and soil factors were selected to simulate the potential distribution of C. appendiculata. When the MaxEnt model is used to predict the potential distribution of species, the model is overfitted, resulting in the decline of the model’s accuracy. This study optimized the model by setting two parameters: characteristic parameter (FC) and regulation magnification (RM) in the MaxEnt model. In this study, 48 combinations were set, and the accuracy of the model was evaluated by △AICC and AUC. The results showed that when FC was LQPH and RM = 2.5, the overfitting and complexity of the model were lower than the default parameters. The appropriate growth area of C. appendiculata in different periods was predicted by optimized parameter modeling. The average AUC of the model test was 0.933, indicating a good model prediction accuracy. The response curve obviously became smooth and nearby the positive distribution curve, which conformed to Shelford’s tolerance law (Zhao et al., 2021b; Song et al., 2021).
The results of the assessment of important climatic variables indicated that the most crucial factors restricting the spatial distribution of the current potential suitable habitat for C. appendiculata are temperature, precipitation, and normalized vegetation index. This was consistent with the habitat of C. appendiculata, which preferred a cold, cool, and wet environment (Averyanov et al., 2003). C. appendiculata is born in the wetlands under the forest or on wetlands beside ditches, and it mostly grows in places with shade, scattered light, high air humidity, and clear air with high moisture (Wei et al., 2017). The main areas of distribution of C. appendiculata are in the Yellow River Basin and most areas to the south. C. appendiculata usually grows in gully wetlands or forest wetlands at an altitude of 500–900 m (Liu et al., 2021b). The results of the present study were consistent with the climatic factors (Gao et al., 2016) and spatial distribution (Liu et al., 2021a) of C. appendiculata determined in previous research, suggesting that the area of suitable habitat predicted by the MaxEnt model was consistent with measurements.
C. appendiculata is found in the Yellow River Basin and across most areas in southern China (Liu et al., 2021b). The results of the optimized maximum entropy model indicated that the current appropriate habitat of C. appendiculata was largely consistent with the actual distribution, indicating that the MaxEnt model is more reliable and more accurate for its distribution. Climate change results in changes in temperature in different regions and changes in the spatial distribution patterns of rainfall. Changes in these climate factors that approach or exceed the current threshold of plant growth can result in the rise of plants’ lowest altitude line or migration to high altitude (Sekercioglu et al., 2008). The habitat of C. appendiculata showed a northwest migration under future climate change (Figure 5). Compared with the current situation, the average suitable habitat showed an increasing trend. At present, many studies and observations have shown that climate change has affected and changed the distribution pattern of the species, and many species have migrated to high latitude or high altitude areas to find more suitable habitats (Parmesan and Yohe, 2003; Walther et al., 2005). As with our results, Zhan et al. (2022) studied the effect of climate change on the distribution pattern of Panax Notoginseng in China and found that its suitable habitat would increase to high altitude. Guo et al. (2021) found that moderate warming would expand the suitable habitat of Ophiocordyceps sinensis and expand the area of O. sinensis with high adenosine content. The study showed that there is a large gap in the protection of C. appendiculata, and there are few areas suitable for its growth in the protection area, which needs to be protected urgently. This study regards the rare and endangered plant C. appendiculata as the protection goal and has determined its systematic protection planning area. The conservation of plant species in situ is an effective measure to protect endangered plants according to their living habitats, and this protection strategy can not only protect the plants themselves but can also protect the surrounding ecological environment to avoid further deterioration of plants in the future (Heywood, 2015). Relevant researchers can also establish a certain range of nature reserves to strengthen protection according to the actual development stage of endangered plants. The results could provide a certain theoretical reference for the optimization of the results of China’s nature reserves. In the process of practical application, the results of this study need to be combined with the actual local situation. The MaxEnt model only predicts the probability of occurrence of certain sites, but it is not known whether it is suitable for growth. Suitable sites for both occurrence and growth are the real suitable areas for species. The growth suitability will be studied in the future.
Conclusion
The potential C. appendiculata suitable habitat in China was simulated in the study using the MaxEnt model with model parameters optimized using the ENMeval package. The results of the study indicated under the current climatic conditions that the suitable habitat of C. appendiculata mainly occurs in parts of Yunnan, Tibet, Sichuan, Chongqing, Guangxi, Guangdong, Gansu, Shaanxi, Zhejiang, Jiangsu, Anhui, Hubei, Henan, Shanxi, Hebei, and Jiangsu. An increasing trend has been noticed in the suitable regional habitat of C. appendiculata under three scenarios of climate change (SSP 1–2.6, SSP 2–4.5, and SSP 5–8.5) during the 2050s and 2070s, and its suitable area of habitat extended northwest. Temperature, precipitation, and normalized vegetation index all have a considerable effect on the distribution of the suitable habitat of C. appendiculata under different emission scenarios at present and in the future. The results provide valuable references for formulating the adaptive ability of C. appendiculata to deal with climate change and can provide theoretical support for its further introduction and cultivation.
Data Availability Statement
The original contributions presented in the study are included in the article/Supplementary Material; further inquiries can be directed to the corresponding author.
Author Contributions
XO: conceptualization, software, writing draft, resources, methodology, visualization, validation, and data curation. AC: funding acquisition, project administration, revising draft, and supervision. GB: editing. YM: methodology. LZ: methodology. HL: funding acquisition, project administration, revising draft, and supervision. All authors have read and agreed to the published version of the manuscript.
Funding
This project was funded by the Key Research and Development Program of Zhejiang Province (No. 2019C02024).
Conflict of Interest
The authors declare that the research was conducted in the absence of any commercial or financial relationships that could be construed as a potential conflict of interest.
Publisher’s Note
All claims expressed in this article are solely those of the authors and do not necessarily represent those of their affiliated organizations, or those of the publisher, the editors, and the reviewers. Any product that may be evaluated in this article, or claim that may be made by its manufacturer, is not guaranteed or endorsed by the publisher.
Supplementary Material
The Supplementary Material for this article can be found online at: https://www.frontiersin.org/articles/10.3389/fenvs.2022.878115/full#supplementary-material
Supplementary Figure S1 | Reliability test of the distribution model created for Cremastra appendiculata.
Supplementary Figure S2 | Suitable habitat area for Cremastra appendiculata under different scenarios of climate change.
Supplementary Figure S3 | Changes in suitable habitat areas for Cremastra appendiculata in the future.
Supplementary Figure S4 | Jackknife test of variable importance for Cremastra appendiculata.
Supplementary Figure S5 | Response curves of probability of presence for Cremastra appendiculata.
References
Akbar, M. W., Yuelan, P., Maqbool, A., Zia, Z., and Saeed, M. (2021). The Nexus of Sectoral-Based CO2 Emissions and Fiscal Policy Instruments in the Light of Belt and Road Initiative. Environ. Sci. Pollut. R. 28 (25), 1–15. doi:10.1007/s11356-021-13040-3
Averyanov, L., Loc, P. K., Hiep, N. T., and Harder, D. (2003). Phytogeographic Review of Vietnam and Adjacent Areas of Eastern Indochina. Komarovia 3, 1–83.
Carpenter, G., Gillison, A. N., and Winter, J. (1993). DOMAIN: a Flexible Modelling Procedure for Mapping Potential Distributions of Plants and Animals. Biodivers. Conserv. 2 (6), 667–680. doi:10.1007/bf00051966
Chakraborty, A., Joshi, P. K., and Sachdeva, K. (2016). Predicting Distribution of Major Forest Tree Species to Potential Impacts of Climate Change in the Central Himalayan Region. Ecol. Eng. 97, 593–609. doi:10.1016/j.ecoleng.2016.10.006
Chung, M. Y., Nason, J. D., and Chung, M. G. (2004). Implications of Clonal Structure for Effective Population Size and Genetic Drift in a Rare Terrestrial Orchid,Cremastra Appendiculata. Conserv. Biol. 18 (6), 1515–1524. doi:10.1111/j.1523-1739.2004.00291.x
Chinese Pharmacopoeia Commission (2010). Pharmacopoeia of the People’s Republic of China, 1. Beijing, China: Chinese Medical Science and Technology Press, 292.
Dai, Y., Hacker, C. E., Zhang, Y., Li, W., Li, J., Zhang, Y., et al. (2019). Identifying the Risk Regions of House Break‐ins Caused by Tibetan Brown Bears ( Ursus arctos Pruinosus ) in the Sanjiangyuan Region, China. Ecol. Evol. 9 (24), 13979–13990. doi:10.1002/ece3.5835
Dai, Y., Peng, G., Wen, C., Zahoor, B., Ma, X., Hacker, C. E., et al. (2021). Climate and Land Use Changes Shift the Distribution and Dispersal of Two Umbrella Species in the Hindu Kush Himalayan Region. Sci. Total Environ. 777, 146207. doi:10.1016/j.scitotenv.2021.146207
Descombes, P., Wisz, M. S., Leprieur, F., Parravicini, V., Heine, C., Olsen, S. M., et al. (2015). Forecasted Coral Reef Decline in Marine Biodiversity Hotspots under Climate Change. Glob. Change Biol. 21 (7), 2479–2487. doi:10.1111/gcb.12868
Du, F. K., Hou, M., Wang, W., Mao, K., and Hampe, A. (2017). Phylogeography of Quercus Aquifolioides Provides Novel Insights into the Neogene History of a Major Global Hotspot of Plant Diversity in South-West China. J. Biogeogr. 44 (2), 294–307. doi:10.1111/jbi.12836
Dyderski, M. K., Paź, S., Frelich, L. E., and Jagodziński, A. M. (2018). How Much Does Climate Change Threaten European Forest Tree Species Distributions? Glob. Change Biol. 24 (3), 1150–1163. doi:10.1111/gcb.13925
Elith, J., Kearney, M., and Phillips, S. (2010). The Art of Modelling Range-Shifting Species. Methods. Ecol. Evol. 1 (4), 330–342. doi:10.1111/j.2041-210x.2010.00036.x
Fischer, G., Shah, M., N. Tubiello, F., and van Velhuizen, H. (2005). Socio-economic and Climate Change Impacts on Agriculture: an Integrated Assessment, 1990-2080. Phil. Trans. R. Soc. B 360 (1463), 2067–2083. doi:10.1098/rstb.2005.1744
Gao, X. F., Lv, X., Li, X. L., Zhang, M. S., Wu, Y. Q., and Wang, W. Z. (2016). The Correlation between Pseudobulb Morphogenesis and Main Biochemical Components of Cremastra Appendiculata (D. Don) Makino. Afr. J. Plant. Sci. 10 (5), 89–96. doi:10.5897/AJPS2016.1411
Graham, M. H. (2003). Confronting Multicollinearity in Ecological Multiple Regression. Ecology 84 (11), 2809–2815. doi:10.1890/02-3114
Guisan, A., and Zimmermann, N. E. (2000). Predictive Habitat Distribution Models in Ecology. Ecol. Model. 135, 147–186. doi:10.1016/S0304-3800(00)00354-9
Guo, Y., Zhao, Z., and Li, X. (2021). Moderate Warming Will Expand the Suitable Habitat of Ophiocordyceps Sinensis and Expand the Area of O. Sinensis with High Adenosine Content. Sci. Total Environ. 787, 147605. doi:10.1016/j.scitotenv.2021.147605
Hanley, J. A., and McNeil, B. J. (1982). The Meaning and Use of the Area under a Receiver Operating Characteristic (ROC) Curve. Radiology 143 (1), 29–36. doi:10.1148/radiology.143.1.7063747
Heywood, V. H. (2015). In Situ conservation of Plant Species - an Unattainable Goal? Isr. J. Plant. Sci. 63 (4), 211–231. doi:10.1080/07929978.2015.1035605
Hinsley, A., De Boer, H. J., Fay, M. F., Gale, S. W., Gardiner, L. M., Gunasekara, R. S., et al. (2018). A Review of the Trade in Orchids and its Implications for Conservation. Bot. J. Linn. Soc. 186 (4), 435–455. doi:10.1093/botlinnean/box083
Hirzel, A. H., Hausser, J., Chessel, D., and Perrin, N. (2002). Ecological-niche Factor Analysis: How to Compute Habitat-Suitability Maps without Absence Data? Ecology 83 (7), 2027–2036. doi:10.1890/0012-9658(2002)083[2027:enfaht]2.0.co;2
Ikeda, Y., Nonaka, H., Furumai, T., and Igarashi, Y. (2005). Cremastrine, a Pyrrolizidine Alkaloid from Cremastra Appendiculata. J. Nat. Prod. 68 (4), 572–573. doi:10.1021/np049650x
Kumar, S., and Stohlgren, T. J. (2009). Maxent Modeling for Predicting Suitable Habitat for Threatened and Endangered Tree Canacomyrica Monticola in New Caledonia. J. Ecol. Nat. Environ. 1 (4), 94–98. doi:10.5897/JENE.9000071
Liu, J., He, C., Tang, Y., Liu, W., Xu, Y., Li, Z., et al. (2021a). A Review of Cremastra Appendiculata (D.Don) Makino as a Traditional Herbal Medicine and its Main Components. J. Ethnopharmacol. 279, 114357. doi:10.1016/j.jep.2021.114357
Liu, J., He, C., Tang, Y., Liu, W., Xu, Y., Li, Z., et al. (2021b). Cremastra Appendiculata (D. Don) Makino, A Potential Anti-Tumor Traditional Chinese Medicine. J. Ethnopharmacol. 279, 114357.
Liu, Y., and Shi, J. (2020). Predicting the Potential Global Geographical Distribution of Two Icerya Species under Climate Change. Forests 11 (6), 684. doi:10.3390/f11060684
Luizza, M. W., Wakie, T., Evangelista, P. H., and Jarnevich, C. S. (2016). Integrating Local Pastoral Knowledge, Participatory Mapping, and Species Distribution Modeling for Risk Assessment of Invasive Rubber Vine (Cryptostegia grandiflora) in Ethiopia’s Afar Region. Ecol. Soc. 21 (1), 22. doi:10.5751/es-07988-210122
Mao, S. L., Zhou, Y., Yuan, H., Lu, C., and Chang, H. (2018). The Complete Chloroplast Genome Sequence of Cremastra Appendiculata (Orchidaceae) Revealed by Next-Generation Sequencing and Phylogenetic Implication. Mitochondrial DNA Part B 3 (2), 1108–1109. doi:10.1080/23802359.2018.1516122
Muscarella, R., Galante, P. J., Soley-Guardia, M., Boria, R. A., Kass, J. M., Uriarte, M., et al. (2014). ENMeval: An R Package for Conducting Spatially Independent Evaluations and Estimating Optimal Model Complexity forMaxentecological Niche Models. Methods. Ecol. Evol. 5 (11), 1198–1205. doi:10.1111/2041-210x.12261
Ouyang, L., Arnold, R. J., Chen, S., Xie, Y., He, S., Liu, X., et al. (2021). Prediction of the Suitable Distribution of Eucalyptus Grandis in China and its Responses to Climate Change. New. For. 53 (1), 81–99. doi:10.1007/s11056-021-09845-2
Parmesan, C., and Yohe, G. (2003). A Globally Coherent Fingerprint of Climate Change Impacts across Natural Systems. Nature 421 (6918), 37–42. doi:10.1038/nature01286
Phillips, S. J., Anderson, R. P., Dudík, M., Schapire, R. E., and Blair, M. E. (2017). Opening the Black Box: an Open-Source Release of Maxent. Ecography 40, 887–893. doi:10.1111/ecog.03049
Phillips, S. J., Anderson, R. P., and Schapire, R. E. (2006). Maximum Entropy Modeling of Species Geographic Distributions. Ecol. Model. 190 (3-4), 231–259. doi:10.1016/j.ecolmodel.2005.03.026
Popp, A., Calvin, K., Fujimori, S., Havlik, P., Humpenöder, F., Stehfest, E., et al. (2017). Land-use Futures in the Shared Socio-Economic Pathways. Glob. Environ. Change 42, 331–345. doi:10.1016/j.gloenvcha.2016.10.002
Remya, K., Ramachandran, A., and Jayakumar, S. (2015). Predicting the Current and Future Suitable Habitat Distribution of Myristica Dactyloides Gaertn. Using MaxEnt Model in the Eastern Ghats, India. Ecol. Eng. 82, 184–188. doi:10.1016/j.ecoleng.2015.04.053
Sekercioglu, C. H., Schneider, S. H., Fay, J. P., and Loarie, S. R. (2008). Climate Change, Elevational Range Shifts, and Bird Extinctions. Conserv. Biol. 22 (1), 140–150. doi:10.1111/j.1523-1739.2007.00852.x
Song, J., Zhang, H., Li, M., Han, W., Yin, Y., and Lei, J. (2021). Prediction of Spatiotemporal Invasive Risk of the Red Import Fire Ant, Solenopsis invicta (Hymenoptera: Formicidae), in China. Insects 12 (10), 874. doi:10.3390/insects12100874
Stockwell, D. R. B., Beach, J. H., Stewart, A., Vorontsov, G., Vieglais, D., and Pereira, R. S. (2006). The Use of the GARP Genetic Algorithm and Internet Grid Computing in the Lifemapper World Atlas of Species Biodiversity. Ecol. Model. 195 (1-2), 139–145. doi:10.1016/j.ecolmodel.2005.11.016
Sutherst, R. W., and Maywald, G. F. (1985). A Computerised System for Matching Climates in Ecology. Agr. Ecosyst. Environ. 13 (3), 281–299. doi:10.1016/0167-8809(85)90016-7
Swets, J. A. (1988). Measuring the Accuracy of Diagnostic Systems. Science 240 (4857), 1285–1293. doi:10.1126/science.3287615
Walther, G. R., Beißner, S., and Burga, C. A. (2005). Trends in the Upward Shift of Alpine Plants. J. Veg. Sci. 16 (5), 541–548. doi:10.1111/j.1654-1103.2005.tb02394.x
Wang, T., Wang, G., Innes, J., Nitschke, C., and Kang, H. (2016). Climatic Niche Models and Their Consensus Projections for Future Climates for Four Major Forest Tree Species in the Asia-Pacific Region. For. Ecol. Manag. 360, 357–366. doi:10.1016/j.foreco.2015.08.004
Wei, B., Lv, X., Gao, X., and Zhang, M. (2017). Advances in Orchidacea Medicinal Plant Cremastra Appendiculata. Guizhou. Agric. Sci. 45 (7), 82–92.
Wu, T., Lu, Y., Fang, Y., Xin, X., Li, L., Li, W., et al. (2019). The Beijing Climate Center Climate System Model (BCC-CSM): the Main Progress from CMIP5 to CMIP6. Geosci. Model Dev. 12 (4), 1573–1600. doi:10.5194/gmd-12-1573-2019
Xin, X., Wu, T., Li, J., Wang, Z., Li, W., and Wu, F. (2013). How Well Does BCC_CSM1.1 Reproduce the 20th Century Climate Change over China? Atmos. Ocean. Sci. Lett. 6 (1), 21–26.
Yan, X., Wang, S., Duan, Y., Han, J., Huang, D., and Zhou, J. (2021). Current and Future Distribution of the Deciduous Shrub Hydrangea Macrophylla in China Estimated by MaxEnt. Ecol. Evol. 11 (22), 16099–16112. doi:10.1002/ece3.8288
Yang, M., Cai, L., Tai, Z., Zeng, X., and Ding, Z. (2010). Four New Phenanthrenes from Monomeria Barbata Lindl. Fitoterapia 81 (8), 992–997. doi:10.1016/j.fitote.2010.06.019
Yang, X.-Q., Kushwaha, S. P. S., Saran, S., Xu, J., and Roy, P. S. (2013). Maxent Modeling for Predicting the Potential Distribution of Medicinal Plant, Justicia Adhatoda L. In Lesser Himalayan Foothills. Ecol. Eng. 51, 83–87. doi:10.1016/j.ecoleng.2012.12.004
Ye, X.-z., Zhao, G.-h., Zhang, M.-z., Cui, X.-y., Fan, H.-h., and Liu, B. (2020). Distribution Pattern of Endangered Plant Semiliquidambar Cathayensis (Hamamelidaceae) in Response to Climate Change after the Last Interglacial Period. Forests 11 (4), 434. doi:10.3390/f11040434
Yue, T.-X., Fan, Z.-M., Chen, C.-F., Sun, X.-F., and Li, B.-L. (2011). Surface Modelling of Global Terrestrial Ecosystems under Three Climate Change Scenarios. Ecol. Model. 222 (14), 2342–2361. doi:10.1016/j.ecolmodel.2010.11.026
Zhan, P., Wang, F., Xia, P., Zhao, G., Wei, M., Wei, F., et al. (2022). Assessment of Suitable Cultivation Region for Panax Notoginseng under Different Climatic Conditions Using MaxEnt Model and High-Performance Liquid Chromatography in China. Industrial Crops Prod. 176, 114416. doi:10.1016/j.indcrop.2021.114416
Zhang, K., Sun, L., and Tao, J. (2020). Impact of Climate Change on the Distribution of Euscaphis Japonica (Staphyleaceae) Trees. Forests 11 (5), 525. doi:10.3390/f11050525
Zhang, M. S., Wu, S. J., Jie, X. J., Zhang, L. X., Jiang, X. H., Du, J. C., et al. (2006). Effect of Endophyte Extract on Micropropagation of Cremastra Appendiculata (D. Don.) Makino (Orchidaceae). Propag. Ornam. Plants 6 (2), 83–89.
Zhang, S., Liu, X., Li, R., Wang, X., Cheng, J., Yang, Q., et al. (2021). AHP-GIS and MaxEnt for Delineation of Potential Distribution of Arabica Coffee Plantation under Future Climate in Yunnan, China. Ecol. Indic. 132, 108339. doi:10.1016/j.ecolind.2021.108339
Zhao, G., Cui, X., Sun, J., Li, T., Wang, Q., Ye, X., et al. (2021a). Analysis of the Distribution Pattern of Chinese Ziziphus Jujuba under Climate Change Based on Optimized Biomod2 and MaxEnt Models. Ecol. Indic. 132, 108256. doi:10.1016/j.ecolind.2021.108256
Keywords: climate change, Cremastra appendiculata, ENMeval, MaxEnt, spatial distribution
Citation: Ouyang X, Chen A, Brien Strachan G, Mao Y, Zuo L and Lin H (2022) Simulation of the Potential Suitable Distribution of the Endangered Cremastra appendiculata in China Under Global Climate Change. Front. Environ. Sci. 10:878115. doi: 10.3389/fenvs.2022.878115
Received: 24 February 2022; Accepted: 09 May 2022;
Published: 04 July 2022.
Edited by:
Yong Wang, Guizhou University, ChinaReviewed by:
Guanghua Zhao, Shanxi Normal University, ChinaYunchuan Dai, Chongqing Academy of Social Sciences, China
Copyright © 2022 Ouyang, Chen, Brien Strachan, Mao, Zuo and Lin. This is an open-access article distributed under the terms of the Creative Commons Attribution License (CC BY). The use, distribution or reproduction in other forums is permitted, provided the original author(s) and the copyright owner(s) are credited and that the original publication in this journal is cited, in accordance with accepted academic practice. No use, distribution or reproduction is permitted which does not comply with these terms.
*Correspondence: Haiping Lin, MTMzOTY1NTc4MDVAMTYzLmNvbQ==