- 1College of Resource and Environment, Shanxi Agricultural University, Jinzhong, China
- 2School of Geography Science, Taiyuan Normal University, Jinzhong, China
- 3Big Data Analysis Technology and Application Institute, Taiyuan Normal University, Jinzhong, China
Ambient PM2.5 (fine particulate matter with aerodynamic diameters ≤2.5 μm) is a major threat to human health. Environmental fates and human exposure to PM2.5 can be affected by various factors, and environmental greenness have been documented to be significantly associated with the exposure disparities; however, the relationship between the greenness and ambient PM2.5 on the region and city levels, and variations across different land cover types remain unclear. In this study, PM2.5 changes from 2001 to 2020 varying over different land cover types and cities were analyzed, and discussed for the relationships with environmental greenness, by taking Shanxi province as an example. The results showed in the past 2 decades, the mean annual NDVI (normalized difference vegetation index) of the study area showed a significant increasing trend (p < 0.01), and the PM2.5 concentration decreased as environmental greenness get better. The same trends were observed across different land cover types and cities. The negative correlation was stronger in the construction land with more frequent human activities, especially in the built-up areas with low vegetation coverage; but limited in the high green space coverage areas. These results provide quantitative decision-making references for the rational development, utilization and management of land resources, but also achieving regional coordinated controls of PM2.5 pollution by optimizing land use.
1 Introduction
Along with the fast development of urbanization and industrialization, environmental quality changes rapidly in most areas. Emissions from sources like industry sector, diesel or gasoline vehicles, coal and biomass burning in power plants and residential stoves lead to serious air pollution (Shi Y et al., 2020). In China, air pollution has been recognized as the 4th largest risk factor, following dietary risks, tobacco, and high blood pressure, and causing millions of premature deaths every year. Although efforts have been taken to fight against the serious air pollution issue and many countermeasures effectively reduced pollution levels, fine particulate matter (PM2.5) remains a significant contributor to high burdens of disease (Liu et al., 2017). Several large-scale epidemiological studies highlighted significant correlations between exposure to air pollutants and premature mortality (Lu et al., 2015; Fang et al., 2016; Cohen et al., 2017; Huang et al., 2017). The Chinese Longitudinal Healthy Longevity Survey (CLHLS) showed that each 10 μg/m3 increase in the past 3-years average PM2.5 was associated with 8% higher mortality in adults aged 65 years or older, and extrapolated that more than 1.7 million premature deaths among Chinese older adults was associated with exposure to ambient air pollution (Li et al., 2018).
Characteristics, fates and influencing factors of PM2.5 that can be affected by many factors have been widely discussed in literature studies. Natural meteorological conditions such as wind speed, temperature, humidity, etc., can affect transport and deposition of airborne particles, and meanwhile, factors associated with human activities, like combustion emissions and land use/cover change (LUCC), especially the deterioration of natural ecological environment such as grassland and woodland being caused by urban expansion and increase of cultivated land, influence PM2.5 fates notably (Lin et al., 2014; Shi K et al., 2020). It has been realized that it is necessary to include the LUCC into researches of PM2.5 influencing factors from the perspective of environmental geography (Fan et al., 2019). Usually, resident, road and industry lands in urban are associated with high PM2.5 intensities as activities like biomass burning and coal combustions in these areas contribute obviously to increased PM2.5 concentration (Huang et al., 2014; Zhang et al., 2016), while forest usually acts as the adsorption sink reducing ambient PM2.5 concentration significantly (Dzierżanowski et al., 2011). She et al. found that PM concentration was proportional to patch area and patch number of LUCC (She et al., 2017). Different landscape patterns cam also affects the interaction between woodland, water and atmospheric PMs (Wu et al., 2015).
The relationship between land cover patterns and air pollution is complex and usually pattern-process relationships (Lam and Niemeier, 2005; Bandeira et al., 2011; Chen et al., 2013; Zhang et al., 2013). Results primarily focusing on urban land cover types (e.g., urban forests, built-up land) may be not generalized to settings where greenspace largely represents regional woodland, grassland, farmland, and open areas (Taylor and Hochuli, 2017). Vegetation was found to have potentially offsetting effects in increasing PM2.5 levels driven by industrial structure and energy-related emissions (Wang et al., 2018). It was suggested that the response of PM pollution to LUCC had obvious differences across different regions, and the correlation between PM pollution and LUCC was weak in coastal areas but strong in inland areas (Sun et al., 2016). According to an investigation of spatial scale effect by Chen et al., the capability for a neighborhood green space to attenuate PM2.5 pollution would be vanished when its size smaller than 200 m, and would be maximized when its size within 400–500 m (Chen et al., 2019). Impacts of land cover pattern changes on the spatial distribution of PM2.5 from the view of different scales, i.e., regional, city and district levels, is still limited.
In China, Shanxi Province, as the country’s main energy base, has vigorously developed the coal industry. Its industrial development, population growth, and urban expansion have caused significant changes in land use patterns and increasingly serious air pollution problems in the past several decades, which directly threaten the physical and mental health of local people and severely hinder its regional sustainability (Bandeira et al., 2011). Development and in-depth study of the relationship between land cover pattern and typical air pollutant PM2.5 can enrich relevant research on the impact of land cover pattern caused by human activities on the ecological environment. Taking Shanxi Province as the research area, this study aims at analyzing 1) the land cover pattern and environment greenness characteristics, which has rapidly developed urbanization in the past 20 years; 2) characteristics of the spatiotemporal changes of PM2.5 pollution in these 20 years under the background of air pollution prevention and control; 3) how does the environment greenness change affect PM2.5 pollution. Carrying out researches on the impact of changes in land cover patterns on PM2.5 can not only provide quantitative decision-making references for the rational development, utilization and management of land resources, but also achieve regional coordinated controls of PM2.5 pollution by optimizing land use methods.
2 Materials and Methods
2.1 Study Area
Shanxi Province (ranging from 110°14′-114° 33′E, 34°34′ -40° 43′N) is in the hinterland of China, with an area of about 156,300 km2 (Figure 1), relying on Taihang Mountain and neighboring Hebei in the east, acing Shaanxi across the Yellow River in the west, and adjoining the Inner Mongolia to the north and Henan Province to the south. There are 11 prefecture-level cities, namely Taiyuan, Datong, Yangquan, Changzhi, Jincheng, Shuozhou, Jinzhong, Xinzhou, Linfen, Yuncheng and Lvliang. Shanxi Province has a very complex topography, with mountains, hills, plateaus and basins widely distributed. With the acceleration of urbanization and industrial development, the emission of particulate pollutants is increasing, and the air pollution pressure is becoming more and more urgent, which has become an important constraint factor for the opening-up and sustainable economic development of Shanxi Province.
2.2 Data Source
The land cover data in this study (including 2000 and 2020) were obtained from the 30-m spatial resolution global land cover data (GlobeLand30, http://www.globallandcover.com/). The images used for classification in this dataset are mainly multi-spectral images of 30-m spatial resolution, including TM5 (Thematic Mapper), ETM+ (Enhanced Thematic Mapper), OLI (Operational Land Imager) multi-spectral images of Landsat and HJ-1 multi-spectral images. The selection principle of these images includes the multispectral image of vegetation growth season within ±2 years of the data production base year or the update year, under the premise of ensuring that the image is cloudless (less cloud). The confusion matrix was used to verify the accuracy of GlobeLand30 data, and its overall accuracy reached over 80%. Based on the existing GlobeLand30 classification system, this study divides land cover types into seven categories: cropland, woodland, grassland, wetland, water body, built-up land, and barren land.
In this study, NDVI (normalized differential vegetation index), a symbolic index representing vegetation growth status and coverage, was selected to reflect environment greenness. NDVI is the ratio of the difference between the near-infrared region and red visible reflectance to the sum of these two measures, ranging from −1.0 to 1.0. Negative NDVI values are often thought of as blue space or water, whereas larger values indicate denser green vegetation (Tucker et al., 2020). We measured NDVI values from the Moderate-Resolution Imaging Spectro-Radiometer (MODIS) in the National Aeronautics and Space Administration’s Terra Satellite (http://wist.echo.nasa.gov) from 1 January 2001 to 31 December 2020. MODIS has a temporal resolution of 16 days and varying spatial resolution up to 250 m. After the projection transformation, format conversion and splicing processing of the original data set, the annual average of NDVI was calculated for analysis. This calculation process was conducted using Google Earth Engine.
Estimates of ground-level concentrations of PM2.5 were obtained from the ChinaHighPM2.5. It is generated from MODIS/Terra + Aqua MAIAC AOD products together with other auxiliary data (e.g., ground-based measurements, satellite remote sensing products, atmospheric reanalysis, and model simulations) using artificial intelligence by considering the spatiotemporal heterogeneity of air pollution. Hourly PM2.5 were obtained from the China National Environmental Monitoring Center. Daily PM2.5 values were then averaged from valid hourly observations at each monitoring station. Auxiliary data, including meteorological variables, surface conditions, pollutant emissions, and population distributions, that may potentially affect PM2.5 concentrations, were collected to improve PM2.5-AOD relationships in China. In this study, meteorological variables considered included temperature, relative humidity, precipitation, evaporation, surface pressure, wind speed, and wind direction, as described in detail elsewhere (Wei et al., 2021). Annual PM2.5 estimates were calculated from 2000 to 2020, at 1 × 1 km spatial resolution, which was averaged from the Level 2 daily products. Since the data for the year 2000 is averaged from March 2000 to December 2000, we extract the annual PM2.5 data from 2001 to 2020 for analysis. The annual PM2.5 estimates are highly related to ground-based measurements (R2 = 0.94) with an average root-mean-square error (RMSE) of 5.07 μg/m3, as described in detail elsewhere.
2.3 Data Analysis
2.3.1 Land Cover Change Rate
To analyze and assess the dynamic degree of land cover types objectively, the land change rate (LCR) of each land cover type was calculated, which is expressed as follows:
where Ua and Ub represent the area of each land cover type at the beginning year and ending year of the study period, respectively.
2.3.2 Correlation Analysis Between Vegetation Dynamics and PM2.5 Change
The Pearson correlation analysis model is used to calculate the correlation coefficient between NDVI and PM2.5 from 2001 to 2020, and to study the relationships between environmental greenness and air pollution on the spatial scales and pixel scales. The equation is as follows:
where n is the number of years, xi represents the value of variable x in the year i, and yi represents the value of variable y in the year i. x and y represent means of the two variables, respectively. rxy represents the correlation coefficient between x and y ranging from -1 to 1. If the rxy > 0, it indicates variables x and y have a positive correlation. On the contrary, if the rxy < 0, it indicates variables x and y have a negative correlation. In addition, if the absolute value of rxy is closer to 1, the correlation between variable x and variable y is stronger. In this study, x and y refer to NDVI, which represents environmental greenness, and PM, which represents air pollution, respectively.
2.3.3 Trend Analysis of Normalized Difference Vegetation Index and PM2.5
In this study, we applied a simple linear regression analysis method based on ordinary least squares (OLS) (Jiang et al., 2017) to detect the trend of mean annual NDVI, and PM2.5 at the regional or pixel scale from 2001 to 2020. The expression of the slope is:
where Ni is the value of parameter (NDVI or PM2.5) in the year i, and n represents the number of years. If the Slope >0, it means the parameter exhibits an upward trend. Otherwise, if the Slope <
Zero, it means the parameter exhibits a downward trend. In addition, the T-test method was operated to examine whether the trend of the parameter was significant at the basin or pixel scale.
3 Results
3.1 Land Cover Change Between 2000 and 2020
The distribution of land cover types showed significant spatial and temporal differences (Figure 2). Cropland is the most widely distributed type, accounting for more than 40% of the total area, mainly distributed in basin located in the central, northeast and southeast, and southwest in Shanxi Province. Woodland and grassland accounted for 28.28 and 23.78% of the total area in 2020, respectively, comprising two dominant natural land cover types. Woodland is distributed mainly along mountain ranges, such as Taihang Mountain, Lvliang Mountain and other mountains. Grassland is mainly distributed in the west and center part. Built-up land is mainly distributed in the central and southeastern basin, accounting for 5.44% of land cover types. The proportions of wetland, water body and barren land in the study area are few, accounting for ∼0.15, 0.38, and 0.06%, respectively. The area statistics of different land cover types are shown in Table 1.
The statistical area change of land cover types results are shown that areas of cropland and grassland had a decrease rate of 7.17 and 4.60%, respectively. Our estimates showed a 5070.5 km2 and 1796.4 km2 net loss areas of cropland and grassland, respectively (Table 1). Grassland loss occurred mainly in the forest-grass ecotone of the northern and central parts, with woodland (contribution to 74.13%) and built-up land (contribution to 23.25%) expansion being the main proximate driver. While cropland decrease was also extensive in these regions, decreased croplands occurred mainly in the central and southwestern basins. As can be seen from Figure 3, the main proximate driver of cropland reduction is built-up extension (contribution to 77.94%), followed by conversion of farmland to woodland and grassland (contribution to 16.73%). The area of built-up land increased the most (4362.7 km2) and the increase rate was the highest (105.04%), followed by the area of woodland, the increase area was 1548.0 km2. The increase rates of wetland, water body and barren land were high, but the increase areas were very small due to the low distribution area. Expanded built-up lands were mainly due to the occupation of cropland (contribution to 86.34%) and grassland (contribution to 11.5%), with Taiyuan, Datong and Yuncheng as the center, and other cities also expanded. Woodland expansions were mainly distributed in the central part, and returning grassland (contribution to 84.89%) or cropland (contribution to 15.11%) to forest were the main proximate driver.
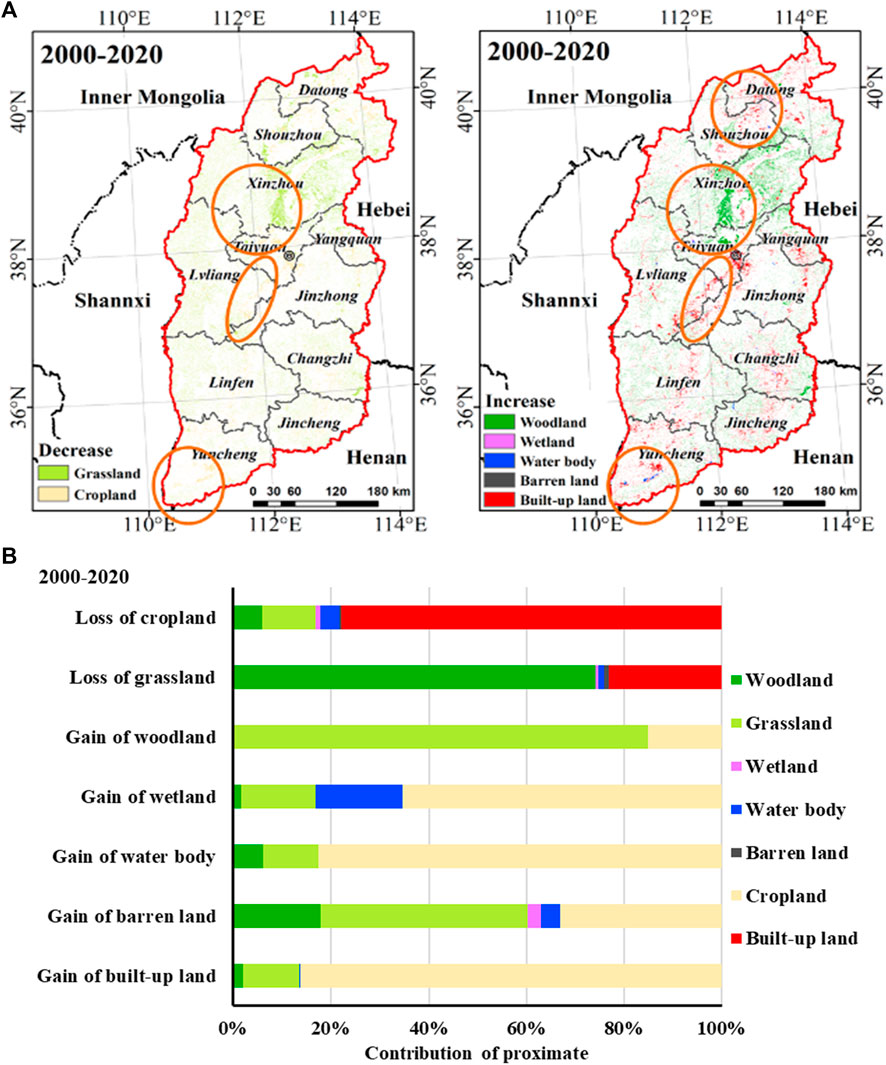
FIGURE 3. The spatial location (A) and contribution of proximate driver (B) of decreasing and increasing land cover type.
3.2 Spatiotemporal Patterns of Normalized Difference Vegetation Index and PM2.5
At the regional scale, the mean annual NDVI of the study area is 0.36 from 2001 to 2020, which showed significant increasing trends, and the increased rate is 0.0058/year (p < 0.01, Figure 4A). Of the total pixels, 93.67% of the entire area had significant increase detected, while only 0.97% experienced significant NDVI decreases in the study area, which were detected mainly in the central construction area (Figure 5). It indicates that the environmental greenness of Shanxi Province has been improved in the last 20 years. We analyzed the mean annual NDVI changes of four main land cover types (Table 2) and found that the mean annual NDVI of Woodland is 0.49, which is the highest value of four main land cover types. The mean annual NDVI of Built-up land had lowest value. Significant increase in mean annual NDVI was found in woodland (0.0064/year, p < 0.01), grassland (0.0065/year, p < 0.01), cropland (0.0055/year, p < 0.01), and built-up land (0.0029/year, p < 0.01). The analysis of the mean annual NDVI in different cities shows that the mean annual NDVI of different cities in Shanxi Province is significantly increased, but the change trends are different (Table 2). The mean annual NDVI value of Jincheng from 2001 to 2020 is the highest among different cities in Shanxi Province, with an increased trend of 0.005/year. Shuozhou has the lowest mean annual NDVI from 2001 to 2020, which is 0.27, lower than the mean annual NDVI of the entire region. The city with the slowest increase of the mean annual NDVI is Jincheng, with an increase rate of 0.005/year, and the city with the fastest increase of the mean annual NDVI is Lvliang (0.0073/year).
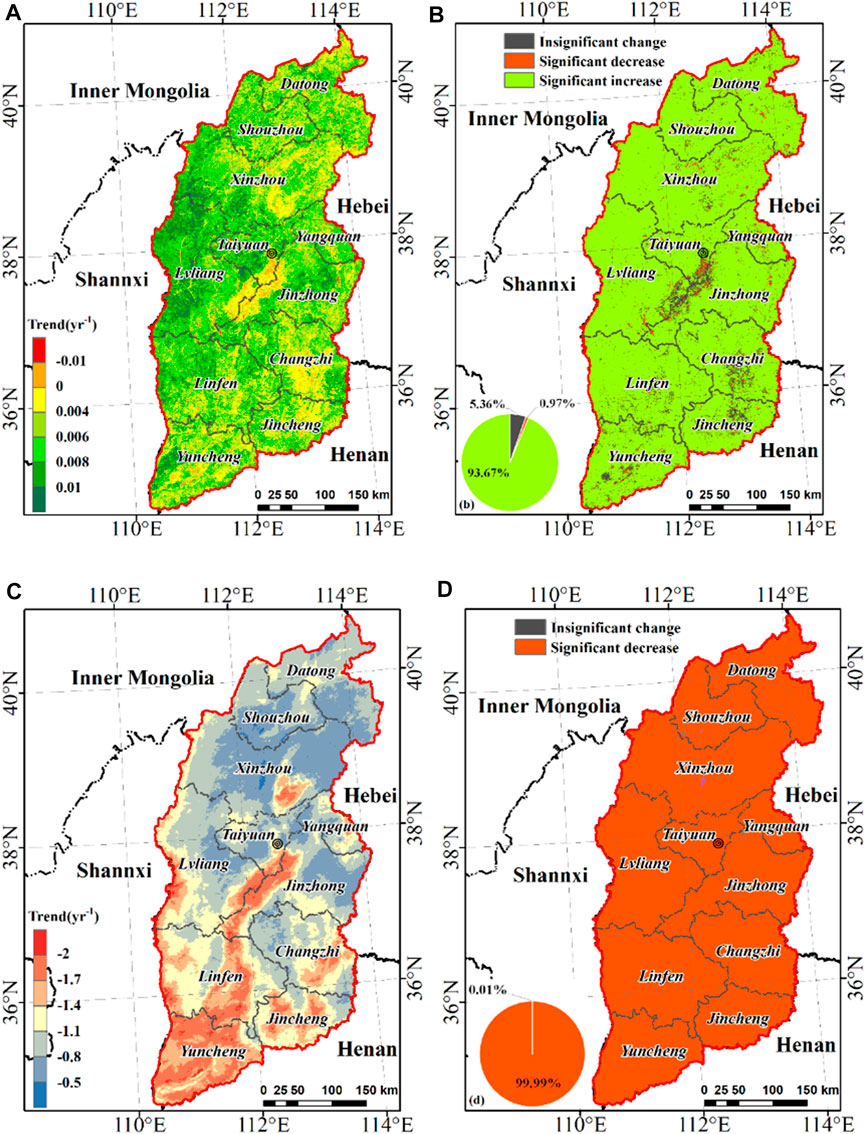
FIGURE 5. Difference in the NDVI change trend (A,B) and PM2.5 trend (C,D) in Shanxi Province from 2001 to 2020.
The annual average of PM2.5 concentration in Shanxi Province was 50.77 μg/m3 from 2001 to 2020, showing a significant decrease trend (over 99% of the entire area), especially in the central and southern regions, whose decrease trend was faster than that of other regions, indicating that the atmospheric environment in Shanxi Province was getting better during these 2 decades (Figure 4B, and Figure 5). Different decrease trends were observed in four main land cover types (Table 2). The annual average of PM2.5 concentration of Woodland is 46.59 μg/m3, which is the lowest value of four main land cover types, and the annual average of PM2.5 concentration of the Built-up land had highest value (62.10 μg/m3). The most significant decrease was found in the built-up land (1.388 μg/m3year−1) from 2001 to 2020, followed by the cropland and grassland, and the lowest in the woodland. According to the regional statistics of the annual average of PM2.5 concentration in different cities, the annual average of PM2.5 concentration of different cities in Shanxi Province showed a significant decrease trend from 2001 to 2020 (Table 2). More than half of the cities have higher annual average of PM2.5 concentration than the average value in Shanxi Province, with Yuncheng having the highest annual average of PM2.5 concentration at 69.33 μg/m3. The city with the slowest decrease of the annual average of PM2.5 concentration is Datong, with a decrease rate of 0.882 μg/m3year−1, and the city with the fastest decrease of the annual average of PM2.5 concentration is Yuncheng (1.680 μg/m3year−1).
3.3 Spatially Different Correlation Between Normalized Difference Vegetation Index Dynamics and PM2.5 Variations
The relationship between the NDVI dynamics and PM2.5 variations over the period 2001–2020 was analyzed by using the Pearson correlation method in the overall region at Shanxi Province (Figure 6). The annual average of PM2.5 concentration had significant negative correlation with the mean annual NDVI (R = −0.723, p < 0.01). Thus, environmental greenness was influential in the decrease of PM2.5 concentration in this region. Of the total pixels, PM2.5 concentration showed significant negative correlation with NDVI in most areas of Shanxi Province, with proportion of 83.77%, which were mainly distributed in vegetated areas. While 15.56% of the entire area showed insignificant correlation between PM2.5 concentration and NDVI, and only 0.67% showed significant positive correlation, which mainly occurred in the areas with expansion of built-up land.
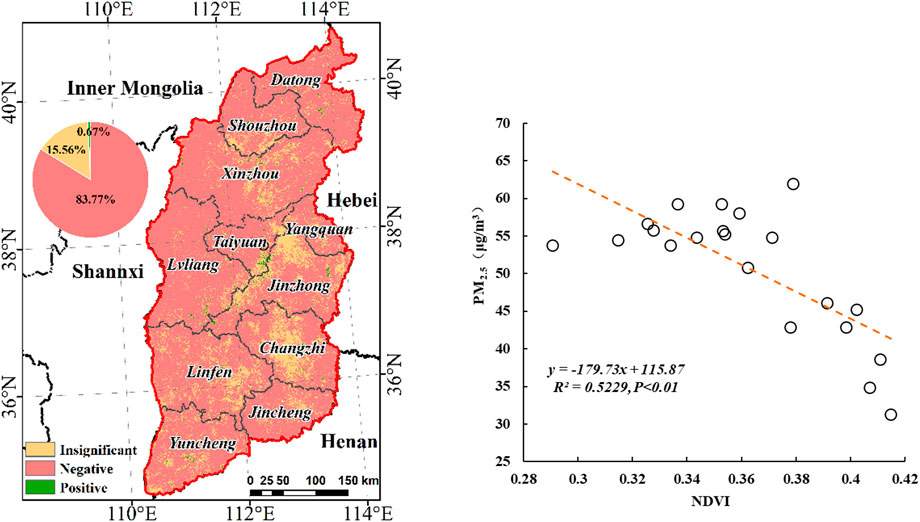
FIGURE 6. The correlation between NDVI and PM2.5. The pie charts illustrate the area percentage of different spatial patterns of the correlations.
The correlations of PM2.5 concentration with NDVI by the main land cover types and different cities were also calculated, and listed in Table 3. PM2.5 concentration of four main land cover types was significantly negative correlated with NDVI. It is important to note that PM2.5 concentration had the strongest negative correlation with NDVI (R = −0.827, p < 0.01) in the built-up land, followed by the cropland (R = -0.726, p < 0.01) and then the woodland (R = −0.710, p < 0.01), and grassland has the weakest negative correlation with NDVI. The relationship between PM2.5 concentrations with NDVI in different cities showed similar results (Table 3), and the trend of correlation coefficient was different among different cities. PM2.5 concentrations were negatively correlated with NDVI for all cities, and the correlation coefficient ranged from −0.626 to −0.817, with Datong showing the strongest significant correlation (R = −0.817, p < 0.01), following by Yuncheng (R = −0.772, p < 0.01) and then Lvliang (R = −0.743, p < 0.01). The weakest negative correlation between PM2.5 concentration and NDVI was occurred in Jinzhong.
At the pixel scale, the correlation analysis between PM2.5 concentrations and NDVI in the main land cover types and different cities revealed that PM2.5 concentrations had significantly negative correlations with NDVI in most areas of woodland, grassland, cropland and built-up land, with proportion of 91.12, 87.15, 79.57 and 72.58%, respectively (see Table 3). The insignificant correlation areas are relatively few, mainly in the built-up land (26.28%), followed by cropland (19.91%). Among different cities, Lvliang has the highest proportion of significant negative correlation between PM2.5 concentrations and NDVI (92.59%), and almost the same result was showed in Datong (92.58%). The areas that PM2.5 concentrations showed insignificant correlation with NDVI mainly occurred in Changzhi and Jinzhong, with proportion of 29.42 and 26.95%, respectively. There are few areas with significant positive correlation between PM2.5 concentrations and NDVI in all cities, and the highest proportion occurs in Taiyuan, accounting for only 1.75%. The trends of the correlation coefficient between PM2.5 concentration and NDVI were different with the change of NDVI, PM2.5 concentration, and area of land cover type (Figure 7). The correlation coefficient becomes stronger with the decrease of NDVI. At the same time, the smaller ratios of woodland and grassland area were, the higher the negative correlation coefficient between PM2.5 concentration and NDVI is, while the larger the built-up land area is, the higher the negative correlation coefficient between PM2.5 concentration and NDVI is. These indicate that in the region with lower vegetation coverage, the increase of environmental greenness has a stronger reduction effect on PM2.5 concentration. Thus, increasing environmental greenness has a stronger effect on PM2.5 concentration reduction in low-vegetation areas than that in high-vegetation areas.
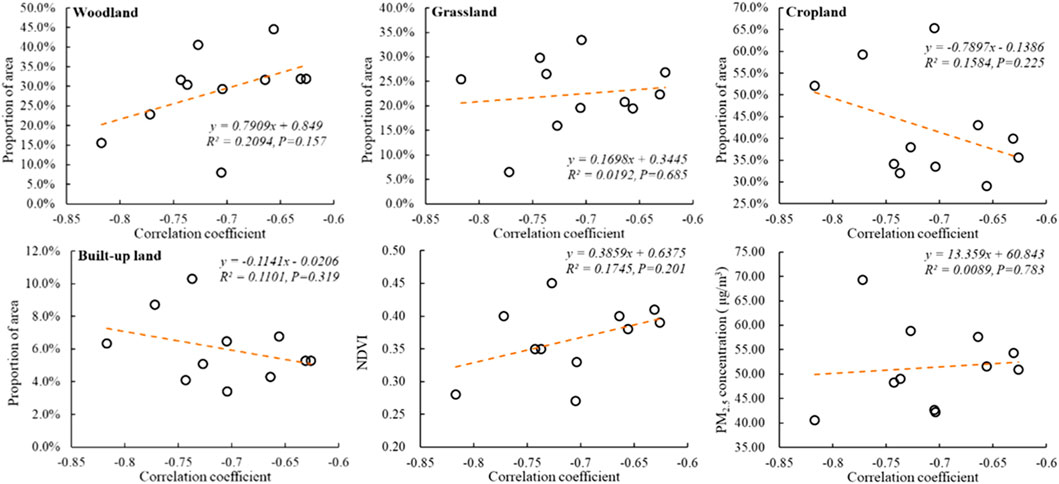
FIGURE 7. The trends of the correlation coefficient between PM2.5 concentration and NDVI with the change of NDVI, PM2.5 concentration, and area of land cover types.
4 Discussion
Results here are expected to be informative for regional land use planning and ecological environment construction to improve air quality, especially to control PM2.5 pollution. With the construction of the Beautiful China in recent years, China’s ecological environment has improved and air pollution has been effectively controlled. With the implementation of the Air Pollution Prevention and Control Action Plan (APPCAP) since 2013, significant declines in pollutants concentrations have achieved in nationwide of China (Bai et al., 2021); however, there are significant differences in PM2.5 concentrations across different regions (Huang et al., 2018). Previous studies revealed that more than 70% of Chinese cities were found to exceed Grade II of the Chinese National Ambient Air Quality Standard, with the highest levels in the North China (Wang et al., 2018). This study found that PM2.5 pollution in Shanxi province has decreased significantly since 2013, which is consistent with the overall pollution trend in China.
As an important energy base in China, the economic development of Shanxi Province has been dominated by the energy consumption, which produces large amounts of harmful emissions (Wei et al., 2018). Different from previous studies that mainly discussed the relationship between urban green space and PM2.5 concentration, this study explored the impact of environmental greenness on PM2.5 concentration from multiple perspectives of different land cover types and different cities at the regional scale (Feng et al., 2017; Chen et al., 2019). The results showed that less vegetation cover has limited ability to deal with high PM2.5 concentration, which was consistent with a previous observation showing that higher green space coverage the site had, the lower the PM2.5 concentration were there (Yang and Jiang, 2021). It has been recognized that vegetation may play an important role in reducing air pollution and improving air quality. For example, Sun et al. found that the concentrations of pollutants including SO2, NO2, CO, PM2.5, and PM10 all have a negative correlation with the NDVI value (Sun et al., 2019). Some studies pointed out that the vegetation cover and PM2.5 concentration correlated negatively (Jin et al., 2020; Kulsum and Moniruzzaman, 2021). The results of this study further confirmed the importance of environmental greenness in mitigating air pollution.
Some scholars studied the mechanism of green vegetation reducing air particulate pollution, and found that plants absorb pollutants through root absorption and leaf absorption pathways, as well as three mitigation mechanisms of green space on particulate matter: deposition, dispersion and modification (Diener and Mudu, 2021). Greenness, on the contrary, can effectively reduce the amount of fine particulate matter in the air. However, in the present study, we found that although environmental greenness has a significant reduction effect on PM2.5 concentration, the reduction effect of NDVI on PM2.5 is affected by green space coverage and PM2.5 concentration level. With the increase of woodland and grassland area, the reduction effect of NDVI on PM2.5 is weakened, and with the expansion of built-up area (that is, the area of green space decreases), the reduction effect of NDVI on PM2.5 is enhanced. Woodlands may be more effective than grasslands in removing particulate matter, but the ability of green space to reduce PM2.5 also has its limitations (Qiu et al., 2018). In high-density urban areas with low vegetation coverage, PM2.5 concentration is high and pollution is serious. Improving environment greenness can more effectively control particulate pollution, while in high-density vegetation areas, air particulate concentration is relatively low, and continuously increases of environment greenness will reduce PM2.5 reduction efficiency (Chen et al., 2019). In addition, as the concentration of PM2.5 in the environment continues to increase, the reduction effect of NDVI on PM2.5 is weakened. In the environment with high concentration of PM2.5, the absorption of particulate matter by leaves will eventually reach saturation, and then the protection efficiency of particulate pollution is reduced (Hui et al., 2020). This suggests that, in densely populated residential or commercial areas, increasing environmental greenness may offer greater opportunities to improve air quality.
However, the mechanism, process, and outcome of PM mitigation by green space are complex and subjected to various influencing factors. Because of limited sample sites, evidence to quantitatively define the level of influence of green space on PM reduction is insufficient in this study. In addition to the land cover types and environmental greenness, researchers found that the landscape pattern (e.g., Patch area, degree of urban cluster, etc.), can also strongly affect PM2.5 concentration (Wu et al., 2015), which was not addressed here due to limited information available. It is also necessary to note that the study did not consider possible time-lag effect, as it was found that the LUCC caused by natural disasters or human activities may have smaller impacts on air pollution in a short period of time, but stronger impacts over a few years (Sun et al., 2016). The time-lag effect is an interesting issue to be investigated in future.
5 Conclusion
Based on GlobeLand30, MODIS NDVI and ChinaHighPM2.5 data, this study investigated the spatiotemporal patterns of land cover types and environmental greenness in Shanxi province, and their relationships with ambient PM2.5 over a period from 2001 to. This study found that although the vegetation area in Shanxi Province decreased since 2000, the environment greenness did show an upward trend. The PM2.5 concentration fluctuated before 2013, and then started to decline continuously. Through the multi-scale analysis, it is found that there is a significant negative correlation between the PM2.5 concentration and environment greenness, confirming the important role of regional greenness on PM2.5 reduction. The study further demonstrates the multiscale effects of the relationship between PM2.5 concentration and environment greenness, that is, PM2.5 concentration is negatively correlated with environmental greenness, and the reduction effect of greenness on PM2.5 was stronger with the low green space coverage areas than in high green space coverage areas, and higher in the low PM2.5 concentration area than in high concentration area. This indicates that the reduction effect of environmental greenness on air particulate pollution is limited, but in construction land with frequent human activities, especially in built-up areas with low vegetation coverage, improving environmental greenness can effectively reduce PM pollution. The results of this study provide a theoretical basis for regional environmental planning and prevention and control of regional PM2.5 pollution.
Data Availability Statement
The original contributions presented in the study are included in the article/Supplementary Material, further inquiries can be directed to the corresponding author.
Author Contributions
GG and YD designed the study. GG and LL analyzed and interpreted the results of the data. GG drafted the manuscript. YD revised the manuscript.
Conflict of Interest
The authors declare that the research was conducted in the absence of any commercial or financial relationships that could be construed as a potential conflict of interest.
Publisher’s Note
All claims expressed in this article are solely those of the authors and do not necessarily represent those of their affiliated organizations, or those of the publisher, the editors and the reviewers. Any product that may be evaluated in this article, or claim that may be made by its manufacturer, is not guaranteed or endorsed by the publisher.
References
Bai, X., Tian, H., Liu, X., Wu, B., Liu, S., Hao, Y., et al. (2021). Spatial-temporal Variation Characteristics of Air Pollution and Apportionment of Contributions by Different Sources in Shanxi Province of China. Atmos. Environ. 244, 117926. doi:10.1016/j.atmosenv.2020.117926
Bandeira, J. M., Coelho, M. C., Sá, M. E., Tavares, R., and Borrego, C. (2011). Impact of Land Use on Urban Mobility Patterns, Emissions and Air Quality in a Portuguese Medium-Sized City. Sci. Total Environ. 409 (6), 1154–1163. doi:10.1016/j.scitotenv.2010.12.008
Chen, L., Sun, R., and Liu, H. (2013). Eco-environmental Effects of Urban Landscape Pattern Changes: Progresses, Problems, and Perspectives. Acta Eco. Sin. 33 (4), 1042–1050. doi:10.5846/stxb201205070659
Chen, M., Dai, F., Yang, B., and Zhu, S. (2019). Effects of Neighborhood Green Space on PM2.5 Mitigation: Evidence from Five Megacities in China. Build. Environ. 156, 33–45. doi:10.1016/j.buildenv.2019.03.007
Cohen, A. J., Brauer, M., Burnett, R., Anderson, H. R., Frostad, J., Estep, K., et al. (2017). Estimates and 25-year Trends of the Global burden of Disease Attributable to Ambient Air Pollution: an Analysis of Data from the Global Burden of Diseases Study 2015. Lancet 389 (10082), 1907–1918. doi:10.1016/s0140-6736(17)30505-6
Diener, A., and Mudu, P. (2021). How Can Vegetation Protect Us from Air Pollution? A Critical Review on Green Spaces' Mitigation Abilities for Air-Borne Particles from a Public Health Perspective - with Implications for Urban Planning. Sci. Total Environ. 796, 148605. doi:10.1016/j.scitotenv.2021.148605
Dzierżanowski, K., Popek, R., Gawrońska, H., Sæbø, A., and Gawroński, S. W. (2011). Deposition of Particulate Matter of Different Size Fractions on Leaf Surfaces and in Waxes of Urban forest Species. Int. J. Phytoremed. 13 (10), 1037–1046. doi:10.1080/15226514.2011.552929
Fan, S., Li, X., and Dong, L. (2019). Field Assessment of the Effects of Land-Cover Type and Pattern on PM10 and PM2.5 Concentrations in a Microscale Environment. Environ. Sci. Pollut. Res. 26 (3), 2314–2327. doi:10.1007/s11356-018-3697-0
Fang, D., Wang, Q. g., Li, H., Yu, Y., Lu, Y., and Qian, X. (2016). Mortality Effects Assessment of Ambient PM2.5 Pollution in the 74 Leading Cities of China. Sci. Total Environ. 569-570, 1545–1552. doi:10.1016/j.scitotenv.2016.06.248
Feng, H., Zou, B., and Tang, Y. (2017). Scale- and Region-Dependence in Landscape-PM2.5 Correlation: Implications for Urban Planning. Remote Sensing 9 (9), 918. doi:10.3390/rs9090918
Huang, Y., Shen, H., Chen, H., Wang, R., Zhang, Y., Su, S., et al. (2014). Quantification of Global Primary Emissions of PM2.5, PM10, and TSP from Combustion and Industrial Process Sources. Environ. Sci. Technol. 48 (23), 13834–13843. doi:10.1021/es503696k
Huang, F., Pan, B., Wu, J., Chen, E., and Chen, L. (2017). Relationship between Exposure to PM2.5 and Lung Cancer Incidence and Mortality: A Meta-Analysis. Oncotarget 8 (26), 43322–43331. doi:10.18632/oncotarget.17313
Huang, Y., Yan, Q., and Zhang, C. (2018). Spatial–temporal Distribution Characteristics of PM2.5 in China in 2016. J. Geovis. Spat. Anal. 2 (2), 1–18. doi:10.1007/s41651-018-0019-5
Hui, L., Yinlong, Z., and Yongbo, W. (2020). Research Progress on Foliar Absorption Mechanism of Pollutants. Environ. Chem. 39 (12), 3371–3383. doi:10.7524/j.issn.0254-6108.2019091706
Jiang, L., Guli·Jiapaer, J., Bao, A., Guo, H., and Ndayisaba, F. (2017). Vegetation Dynamics and Responses to Climate Change and Human Activities in Central Asia. Sci. Total Environ. 599-600, 967–980. doi:10.1016/j.scitotenv.2017.05.012
Jin, N., Li, J., Jin, M., and Zhang, X. (2020). Spatiotemporal Variation and Determinants of Population's PM2.5 Exposure Risk in China, 1998-2017: a Case Study of the Beijing-Tianjin-Hebei Region. Environ. Sci. Pollut. Res. 27 (25), 31767–31777. doi:10.1007/s11356-020-09484-8
Kulsum, U., and Moniruzzaman, M. (2021). Quantifying the Relationship of Vegetation Cover and Air Pollution: A Spatiotemporal Analysis of PM2.5 and NDVI in Greater Dhaka, Bangladesh. Jagannath Univ. J. Sci. 7 (2), 54–63.
Lam, T., and Niemeier, D. (2005). An Exploratory Study of the Impact of Common Land-Use Policies on Air Quality. Transport. Res. D: Transport Environ. 10 (5), 365–383. doi:10.1016/j.trd.2005.04.011
Li, T., Zhang, Y., Wang, J., Xu, D., Yin, Z., Chen, H., et al. (2018). All-cause Mortality Risk Associated with Long-Term Exposure to Ambient PM2.5 in China: a Cohort Study. Lancet Public Health 3 (10), e470–e477. doi:10.1016/s2468-2667(18)30144-0
Lin, G., Fu, J., Jiang, D., Hu, W., Dong, D., Huang, Y., et al. (2014). Spatio-temporal Variation of PM2.5 Concentrations and Their Relationship with Geographic and Socioeconomic Factors in China. Int. J. Environ. Res. Public Health 11 (1), 173–186. doi:10.3390/ijerph110100173
Liu, M., Huang, Y., Ma, Z., Jin, Z., Liu, X., Wang, H., et al. (2017). Spatial and Temporal Trends in the Mortality burden of Air Pollution in China: 2004-2012. Environ. Int. 98, 75–81. doi:10.1016/j.envint.2016.10.003
Lu, F., Xu, D., Cheng, Y., Dong, S., Guo, C., Jiang, X., et al. (2015). Systematic Review and Meta-Analysis of the Adverse Health Effects of Ambient PM2.5 and PM10 Pollution in the Chinese Population. Environ. Res. 136, 196–204. doi:10.1016/j.envres.2014.06.029
Qiu, L., Liu, F., Zhang, X., and Gao, T. (2018). The Reducing Effect of green Spaces with Different Vegetation Structure on Atmospheric Particulate Matter Concentration in BaoJi City, China. Atmosphere 9 (9), 332. doi:10.3390/atmos9090332
She, Q., Peng, X., Xu, Q., Long, L., Wei, N., Liu, M., et al. (2017). Air Quality and its Response to Satellite-Derived Urban Form in the Yangtze River Delta, China. Ecol. Indicators 75, 297–306. doi:10.1016/j.ecolind.2016.12.045
Shi K, K., Shen, J., Wang, L., Ma, M., and Cui, Y. (2020). A Multiscale Analysis of the Effect of Urban Expansion on PM2.5 Concentrations in China: Evidence from Multisource Remote Sensing and Statistical Data. Build. Environ. 174, 106778. doi:10.1016/j.buildenv.2020.106778
Shi Y, Y., Bilal, M., Ho, H. C., and Omar, A. (2020). Urbanization and Regional Air Pollution across South Asian Developing Countries - A Nationwide Land Use Regression for Ambient PM2.5 Assessment in Pakistan. Environ. Pollut. 266 (Pt 2), 115145. doi:10.1016/j.envpol.2020.115145
Sun, L., Wei, J., Duan, D. H., Guo, Y. M., Yang, D. X., Jia, C., et al. (2016). Impact of Land-Use and Land-Cover Change on Urban Air Quality in Representative Cities of China. J. Atmos. Solar-Terrestrial Phys. 142, 43–54. doi:10.1016/j.jastp.2016.02.022
Sun, S., Li, L. J., Zhao, W. J., Qi, M. X., Tian, X., and Li, S. S. (2019). Variation in Pollutant Concentrations and Correlation Analysis with the Vegetation Index in Beijing-Tianjin-Hebei. Huan Jing Ke Xue 40 (4), 1585–1593. doi:10.13227/j.hjkx.201809178
Taylor, L., and Hochuli, D. F. (2017). Defining Greenspace: Multiple Uses across Multiple Disciplines. Landscape Urban Plann. 158, 25–38. doi:10.1016/j.landurbplan.2016.09.024
Tucker, R., Callaham, J. A., Zeidler, C., Paul, A.-L., and Ferl, R. J. (2020). NDVI Imaging within Space Exploration Plant Growth Modules - A Case Study from EDEN ISS Antarctica. Life Sci. Space Res. 26, 1–9. doi:10.1016/j.lssr.2020.03.006
Wang, S., Liu, X., Yang, X., Zou, B., and Wang, J. (2018). Spatial Variations of PM2.5 in Chinese Cities for the Joint Impacts of Human Activities and Natural Conditions: A Global and Local Regression Perspective. J. Clean. Prod. 203, 143–152. doi:10.1016/j.jclepro.2018.08.249
Wei, W., Li, P., Wang, H., and Song, M. (2018). Quantifying the Effects of Air Pollution Control Policies: A Case of Shanxi Province in China. Atmos. Pollut. Res. 9 (3), 429–438. doi:10.1016/j.apr.2017.11.010
Wei, J., Li, Z., Lyapustin, A., Sun, L., Peng, Y., Xue, W., et al. (2021). Reconstructing 1-Km-Resolution High-Quality PM2.5 Data Records from 2000 to 2018 in China: Spatiotemporal Variations and Policy Implications. Remote Sensing Environ. 252, 112136. doi:10.1016/j.rse.2020.112136
Wu, J., Xie, W., Li, W., and Li, J. (2015). Effects of Urban Landscape Pattern on PM2.5 Pollution-A Beijing Case Study. PLoS One 10 (11), e0142449. doi:10.1371/journal.pone.0142449
Yang, W., and Jiang, X. (2021). Evaluating the Influence of Land Use and Land Cover Change on fine Particulate Matter. Sci. Rep. 11 (1), 17612. doi:10.1038/s41598-021-97088-8
Zhang, R., Pu, L., and Liu, Z. (2013). Advances in Research on Atmospheric Environment Effects of Land Use and Land Cover Change. Area Res. Dev. 32, 123–128.
Keywords: land cover, NDVI, fine particulate matter, spatiotemporal patterns, environmental greenness
Citation: Guo G, Liu L and Duan Y (2022) Evaluating the Association of Regional and City-Level Environmental Greenness and Land Over Patterns With PM2.5 Pollution: Evidence From the Shanxi Province, China. Front. Environ. Sci. 10:875619. doi: 10.3389/fenvs.2022.875619
Received: 14 February 2022; Accepted: 28 March 2022;
Published: 13 May 2022.
Edited by:
Wenqiu Ma, China Agricultural University, ChinaCopyright © 2022 Guo, Liu and Duan. This is an open-access article distributed under the terms of the Creative Commons Attribution License (CC BY). The use, distribution or reproduction in other forums is permitted, provided the original author(s) and the copyright owner(s) are credited and that the original publication in this journal is cited, in accordance with accepted academic practice. No use, distribution or reproduction is permitted which does not comply with these terms.
*Correspondence: Yonghong Duan, eWhkdWFucGt1QHNpbmEuY29t