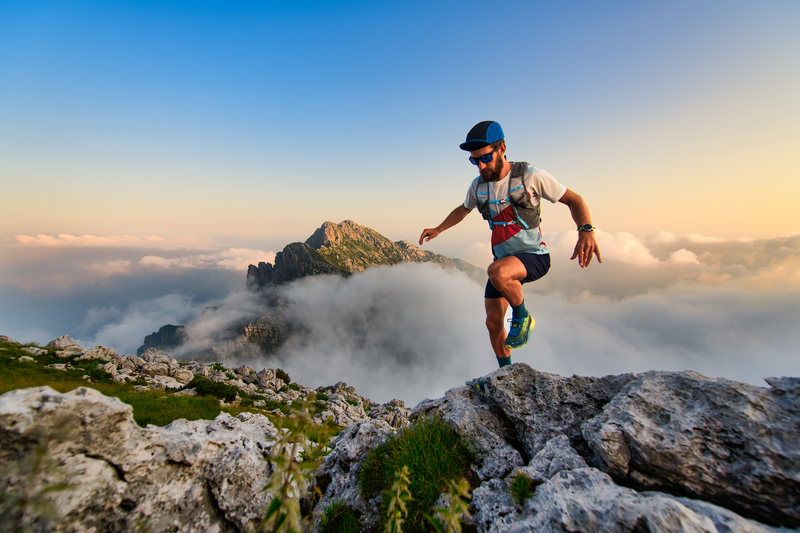
94% of researchers rate our articles as excellent or good
Learn more about the work of our research integrity team to safeguard the quality of each article we publish.
Find out more
ORIGINAL RESEARCH article
Front. Environ. Sci. , 21 March 2022
Sec. Environmental Economics and Management
Volume 10 - 2022 | https://doi.org/10.3389/fenvs.2022.874367
This article is part of the Research Topic Carbon Neutrality Approaches in Buildings and Agriculture Sectors View all 12 articles
Green building projects (GBPs) involve multiple interdependent stakeholders, whose individual and separate concerns have different degrees of impact on sustainability management. These concerns are highly complex, subject to many uncertainties, and pose significant challenges to decision-makers during sustainability assessments, especially with regard to the social aspects of the project. As such, addressing the complexity of stakeholder concerns and optimizing the decision-making process in green building projects from the stakeholder perspective are crucial to improving practices in social sustainability management. However, to date, there is a lack of relevant empirical studies on this subject. This study proposes a decision-making model based on Bayesian networks (BN); a project network decision model is also constructed from a social sustainability perspective. A diagnostic analysis and sensitivity analysis of the constructed model identify the key stakeholder concerns that affect the social sustainability of the project. To verify its feasibility, the BN model is applied to a green building project, specifically, the Wuhan International Commerce Center, China. The results identify green design and construction, an abundance and stability of project funds, and conveniently-situated service facilities as the primary, sensitive stakeholder concerns that significantly impact social sustainability. The findings show that the BN model can be used as a long-term management decision-making tool for this project. The uncertainty problem associated with changes in sustainability levels induced by the multiplicity of stakeholders is addressed in this study. Furthermore, the findings expand the topic of social sustainability in green construction projects. These findings aid project decision-makers in managing stakeholders individually based on their various concerns, as well as improving the social sustainability of green building projects.
The public has become aware of the importance of sustainable building due to the enormous energy consumption and severe environmental impact of the global construction industry. Therefore, the development and construction of green buildings has become a standard solution and practice in many nations (Hwang and Tan 2012). The aim of green building practices is to improve the efficiency of the resource utilization of building projects throughout their whole life cycle. Green buildings also decrease environmental pollution, improve the human living environment, and ultimately promote the sustainable development of society (Darko et al., 2019). The complexity of green building projects (GBPs) is often manifested in rigorous technical evaluation indicators, the engagement of numerous stakeholders, many uncertain risks, and high-level sustainability goals (Zhao et al., 2016; Mok et al., 2018; Bohari et al., 2020). Although many studies have focused on the interrelationship between GBP stakeholders (Doloi 2013; Seuring and Gold 2013; Yang and Shen 2015; Li et al., 2018a), discovering how to face the challenges posed by the complex interrelationships among stakeholders in construction projects remains difficult (Lin et al., 2019; Yang et al., 2020). This is because these previous studies have mostly separated social sustainability from stakeholders. Adopting such a fragmented strategy undermines the synergistic effect of the complexity of interacting stakeholder concerns (drivers), as well as having a negative impact on sustainability.
In fact, achieving the social sustainability objectives related to green building requires the consideration of more human activities; project success also depends more on the participation of stakeholders (Mok et al., 2017a). Moreover, studies have shown that different stakeholder concerns (and the complex interactions) create many obstacles to a project’s success, which in turn increases the difficulty of managing stakeholders (Control et al., 2008; Hwang and Ng 2013; Li et al., 2016; Luo et al., 2017). Additionally, the increasing demand for sustainable knowledge and technology renders the managing of stakeholders even more challenging (Schröpfer et al., 2017). These challenges affect the realization of social sustainability objectives. Project decision-makers, therefore, need to coordinate this interrelationship according to different stakeholder concerns and appropriately arrange stakeholder participation that follows the whole project’s life cycle. Although stakeholder management is generally adopted in existing GBP management literature (Qiang et al., 2021), as it presents a systematic approach to sustainability assessments, the interdependency between social sustainability and stakeholder concern complexity has not been reflected in the existing framework.
Although extensive research has focused on sustainability assessment indicators, so far, no general consensus has been reached on social sustainability indicators for GBPs (Chen et al., 2015; Al-Jebouri et al., 2017; Goel et al., 2020). These studies have addressed various topics, such as megaproject social responsibility (Lin H. et al., 2017), stakeholder engagement with regard to achieving sustainability (Bal et al., 2013), a lifecycle-based sustainability indicator framework (Chong et al., 2016), and successful delivery of GBPs (Olanipekun et al., 2017). Social sustainability evaluation indicators can effectively identify the social sustainability level of GBPs; these indicators are powerful decision-supporting tools that foster sustainable development (Zhong and Wu 2015; Chong et al., 2016; Olanipekun et al., 2017; Yadegaridehkordi et al., 2020). For green buildings to be socially sustainable, they must abide by relatively strict evaluation standards. The complexity of the project also often results in differences between the practiced decision-making and the ultimate goal (San Cristóbal et al., 2018). Multitudinous sustainable evaluation indicators and stakeholder concerns are available. The connections between them are sophisticated and often change when they are integrated, thus further aggravating the decision-making problems. However, the existing stakeholder management body of knowledge in terms of traditional assessment methodologies and network analysis, on the other hand, is insufficient and cannot provide effective solutions to the aforementioned problems. To address this issue, this research proposes a new method for answering the following research questions (RQ):
RQ1: In existing literature, how is the interdependency between stakeholder concern complexity and social sustainability, in particular, treated?
RQ2: How can a decision-making model be developed that prioritizes stakeholder concerns while also facilitating GBPs sustainability management practices?
RQ3: In GBPs, how are stakeholder concern complexity and social sustainability interdependent?
The Bayesian network (BN) is widely used in decision-making research, given BN’s superiority when dealing with complex uncertain relations and with capturing interdependency between distinct concepts (represented by stakeholders and sustainability in this paper) (Castillo et al., 2016; Bakshan et al., 2017; Chen et al., 2019). In addition, the use of BN statistics data for sustainable decision-making offers confirmed advantages over traditional optimization approaches (Mkrtchyan et al., 2016; Sierra et al., 2018). Within the theoretically-grounded BN framework, this paper aims to propose a new process, namely “stakeholder concern and sustainability management (SCSM)”. This approach integrates all stages of the decision-making process and the consequences affecting the GBP’s sustainability objectives. A BN model that represents the relationships among stakeholder concerns and social sustainability objectives is constructed in this study. In this model, the stakeholder concern complexity attributes are represented as deterministic nodes; social sustainability indicators are represented as chance nodes. Through a diagnostic analysis and a sensitivity analysis of this constructed model, key stakeholder concerns that affect the social sustainability of the project can be identified. To verify its feasibility, the BN model is applied to a specific GBP, namely the Wuhan International Commerce Center, China. The decision-making model is used to individually manage stakeholders, according to their different concerns. By optimizing sustainable decisions through the developed model, decision-makers can improve the social sustainability of GBPs. Using a systematic review method, this paper identifies current research gaps and sustainability needs in the construction industry and presents findings from 13 semi-structured interviews conducted with green building sector experts from China. New insights into future developments are also provided, as well as the potential for future research in the field.
This study is structured as follows: In Section 2, the theoretical foundation of stakeholder concern and Bayesian networks are introduced. In Section 3, a Bayesian network analysis method is developed. In Section 4, the SCSM model is established and verified through a case study. In Section 5, the obtained results are discussed. Finally, the conclusion, including the main contributions, potential future work, and limitations are presented in Section 6.
The concept of “stakeholder concern complexity” is grounded in stakeholder theory. Quite a few papers have, in fact, investigated GBP complexity from the perspective of stakeholders by analyzing the stakeholders’ roles, interactions, and impacts on project success. Project stakeholders can be both individuals and organizations that are involved in a project, or who have influenced the project as a result of project implementation (Institute 2009). In a GBP, the relationships between stakeholders are more complex than in typical projects, due to the fact that increased project complexity creates various interests and conflicts (Yang and Zou 2014; Yang et al., 2016; He et al., 2020). Considering the complexity of the various interactions from a network perspective is a reasonable choice and has been widely applied in existing research. Topics that have been addressed from this perspective include stakeholder engagement networks (Mok et al., 2015; Mok and Shen 2016; Burga and Rezania 2017), stakeholder collaboration networks (Cao et al., 2016; Tang et al., 2018; Li et al., 2019), stakeholder-related risk networks (Yang and Zou 2014; Castillo et al., 2016; Yang et al., 2016), stakeholder-related indicator networks (Wu et al., 2018), and stakeholder concern networks (Mok et al., 2017b, 2018). Journeault et al. (2021) pointed out that five critical roles of stakeholders, namely those of the trainer, analyst, coordinator, specialist, and financial provider, should be focused upon by the government, as these roles contribute significantly to overcoming different barriers to the integration of sustainability practices. These studies particularly emphasize the significance of stakeholder engagement for green practices; they also clarify the undeniable responsibility of stakeholders to foster a more sustainable construction environment. Decision-makers face the challenge of identifying and filtering the key stakeholders from all the project participants. Thus, stakeholder analysis is a vital process for the decision-makers when addressing this issue (Li et al., 2012).
According to the definition and characteristics of the stakeholder as presented above, stakeholder concerns in this study refer to interests that are closely related to stakeholders, and which have a certain probability to change during the implementation of the project (Olander and Landin 2005). In previous studies, different classifications of stakeholder concerns were applied, such as social, cost, legal, organizational, technological, environmental, procurement, and ethical (Mok et al., 2017b); economic, environmental, social, and ethical (Mok et al., 2018), and social, economic, safety, equity, responsibility, and ethical (Lin X. et al., 2017; Sperry and Jetter 2019). Project failure is generally the result of decision-makers not being able to address the diversity of stakeholder concerns, which in turn leads to an inability among project stakeholders to achieve a unified sustainable goal (He et al., 2020). Therefore, conflicts of interest must necessarily be reduced. This can be done by optimizing decision-making and coordinating the complex interactions between stakeholder concerns (Toor and Ogunlana 2010).
The complexity of stakeholder concerns further aggravates the complexity of GBPs and imposes challenges on project sustainability decision-making. Although some sustainability studies have used a stakeholder analysis perspective, it appears that the analysis is mostly related to stakeholder relationships, rather than a comprehensive assessment of the stakeholder concerns related to social sustainability objectives. In addition, only a limited number of studies have been conducted in the context of GBPs. Current social sustainability studies lack the integration of empirical and rationalistic angles that could be used to assess causality between stakeholder concern and social sustainability in GBPs. Bridging this gap would enhance the understanding of stakeholder management and improve the sustainability outcomes of GBPs.
In this study, the major stakeholder concerns were identified by integrating previous research with GBP implementation practices. The initial stakeholder concerns are summarized in Table 1. This list of concerns does not include all concerns related to GBPs. Instead, the included concerns only relate to social sustainability, and the concerns are developed to build relationships with sustainability indicators, in order to optimize project decisions.
Consideration of social sustainability during the construction project life cycle has been strongly encouraged, ever since the Brundtland Report (WCED 1987). Although numerous scholars have attempted to precisely define social sustainability, it is a concept in chaos (Zhang and Mohandes 2020). To achieve consensus, context-specific conception should be adopted in GBPs. The concept of social sustainability refers to the process of constructing a structure that meets the GBP stakeholders’ needs throughout the building’s life (Almahmoud and Doloi 2015). Landorf (2011) proposed that stakeholders, such as users and neighborhood communities, are centric in terms of social sustainability management, particularly because their diverse needs are the foundation of social sustainability. According to Landorf (2011), user comfort, health and safety, and access to services are common concerns of pertinent stakeholders, including the client, end-users, and local authorities. Therefore, in GBPs, social sustainability is closely related to the involvement of stakeholders and largely depends on the stakeholders’ concerns.
To assess the social sustainability efficacy, several evaluations, frameworks and models have been developed by scholars over the past two decades (Zuo et al., 2012; Valdes-Vasquez and Klotz 2013; Almahmoud and Doloi 2015). Fernández-Sánchez and Rodríguez-López (2010) selected the ISO-21929 standard as the indicator framework. That study created a methodology identifying sustainability indicators with the technology used in risk management, and categorized social sustainability indicators into six subcategories: culture, accessibility, participation, security, public utility, and social integration. Heravi et al. (2015) compared the importance of seven categories of social indicators in the three phases of construction, operation and maintenance, and demolition. The study proposed that infrastructure improvement and health and safety are the most important social sustainability indicators for the construction phase and operation phase, respectively. Olakitan Atanda (2019) developed a social sustainability assessment framework by adopting Delphi techniques and interviews. The study found that participation and control, environmental education and social equity were the three highest weights in the 35 sustainability indicators of eight categories. Akhanova et al. (2020) employed the stepwise weight assessment ratio analysis (SWARA) to calculate the weights of sustainability indicators in Kazakhstan. The study showed that indoor environmental quality and the quality of building planning solutions are the highest priorities for sustainable construction.
Although social sustainability has also been reported as an important aspect of GBPs, it is typically found to be paid less attention, due to a lack of analytical and theoretical underpinning (Siew et al., 2019; Zang et al., 2022). There appears to be a lack of guidelines that can be used when measuring uncertainties arising from changing stakeholder concerns. Akhanova et al. (2020) showed that the interaction of stakeholder involvement necessarily changes throughout a project life cycle, in order to adapt to the complexities and uncertainties imposed by multifaceted sustainability outcomes. Nevertheless, there is a lack of research into the interactions between social sustainability and stakeholder concern complexity. To visualize the casual relationship between the two, and to develop a decision-making model for sustainability-related objectives, a BN graph is used in this study.
Researchers have been using different techniques for capturing interdependency between stakeholder engagement and project sustainability, including Analytical Network Process (ANP) (Kiani Mavi and Standing 2018); social network analysis (SNA) (Wu et al., 2021b; Wang et al., 2021)and structural equation modelling (SEM) (Teng et al., 2019; González-Rodríguez and Tussyadiah 2021). Although the ANP can take the complex interdependence at different levels into account, there is still limitations in exploring the behavioral causality of interrelated non-human objects (Zhao et al., 2017). The main criticism of SNA is its inability to address more uncertainty issues and update beliefs upon receiving new interaction relationships (Lee et al., 2018). SEM has its limitation in guaranteeing complex cause-effect relationship when necessary causal conditions cannot be met (Khan et al., 2019).
Existing research on GBPs have mainly focused on environmental and technological aspects like design optimization, GB techniques and energy efficient measures whereas to the best of the authors’ knowledge, an integrated stakeholder concern and social sustainability model has not been presented (Chen et al., 2021a; Wu et al., 2021a; Wu et al., 2021b). Green building projects involve complex and intertwined concerns among stakeholders (Wang et al., 2021), the mentioned techniques fail to assess social sustainability within a probabilistic setting of interacting concerns. Furthermore, optimal sustainability strategies cannot be made when adding new concerns to the interdependency models during the life cycle of the GBPs.
To fill this gap, a SCSM model is proposed in this study, which grounded in the theoretical framework of BN. As BN has been one of the most efficient methods to manifest the causal map of complex relationship between interconnected variables (Koseoglu Balta et al., 2021). In addition, there are a number of uncertainties between stakeholders in GBPs, BN presents a well-established tool to cope with these uncertainties (Kumar and Banerji 2022).
A BN is a directed acyclic graph composed of nodes and connections (Figure 1), the BN’s formation combines graph theory and probability theory. Rooted in these theories, BN interprets project sustainability by analyzing stakeholder concern complexity, diagnosing how various stakeholder behaviors can affect social sustainability, recognizing key stakeholder concerns, and identifying opportunities to improve stakeholder management. The nodes of the network represent uncertain variables, their edges represent influential links between variables (Neil et al., 2000). A BN offers advantages in terms of the decision-making related to complex and uncertain problems. As such, BNs have been widely employed in construction projects and the environmental domain. Specifically, Bakshan et al. (2017) used a BN to establish behavioral causality models that improve waste management in buildings. Zhang et al. (2020) used the BN method to analyze the load data of the pile foundation, in order to calculate the design resistance coefficient. Many studies have also addressed dynamic Bayesian networks (DBN). For example, Wu et al. (2015) used a DBN model to provide security solutions based on the dynamics of tunnel road surface damage. Špačková and Straub (2013) established a DBN model to evaluate tunnel construction performance, and Kosgodagan-Dalla Torre et al. (2017) applied a DBN to overcome the obstacles of asset network degradation modeling under conditions of limited data availability.
In addition, BNs are widely used for risk prediction. Nepal and Yadav (2015) combined a BN with specific characteristics of reliability engineering to quantify the risk factors that cause related failures. Mkrtchyan et al. (2016) analyzed human reliability risks by establishing a Bayesian trust network. Chen et al. (2019) showed that a combination of the Cloud model and BN can better reflect a real risk situation than classical fuzzy set theory. Through BN, Sanchez et al. (2020) established a project management maturity model that can effectively prevent project cost overruns. Wei et al. (2020) considered the sequence of risks in a smart city and used BNs to model flow risks. Koseoglu Balta et al. (2021) predicted and mitigated delay risk in TBM tunnel projects.
Due to the dynamic changes of the social environment, the interactions between actors have become more complex. To resolve the associated problems, “building blocks” need to be combined, in order to form larger BNs (Neil et al., 2000). Xing et al. (2010) developed dynamic tomography methods to analyze time-evolving networks. Fang et al. (2020) simulated a Zachary network to compare two Bayesian learning strategies. Chen et al. (2021b) developed Bayesian Monte Carlo simulation–driven risk inference method to address schedule issues in infrastructure projects. The study found that Bayesian social learning is more likely to cause asymptotic learning. These researches show the methodological viability of BN analysis in exploring the behavioral causality of interrelated non-human objects. They also provide insights into the network analyzing process in construction projects. It can be concluded that sustainable development is valued. Therefore, it is necessary to identify the factors that affect the social sustainability of GBPs through BN and then make optimal decisions (Sierra et al., 2018). Despite the achievements of previous studies of BNs, factors such as project complexity and stakeholder influence have rarely been addressed (Qazi et al., 2016; Yu et al., 2019), thereby presenting a need to conduct relevant research.
This study proposes a SCSM model approach to analyze the social sustainability changes of the project, which in turn have been caused by various complex stakeholder concerns. To achieve this goal, a literature review, questionnaire survey, BN analysis and semi-structured interviews are applied. Below, Figure 2 shows the SCSM implementing framework of the present study. The framework is structured in four stages: 1) stakeholder concern and sustainability indicator identification, 2) BN parameter learning, 3) Bayesian network-based indicator analysis, and 4) decision making through the optimization of GBPs from the social sustainability viewpoint.
Social sustainability has been widely explored in existing literature. However, no consensus has been reached with regard to the social sustainability indicators of green buildings (Goel et al., 2020). Therefore, it is necessary to identify key sustainability indicators that can be used to assess the social sustainability level of green buildings during the buildings’ life cycle. In practice, GBPs are complex where sustainable technology is concerned. Also, identifying all stakeholder concerns during the project life cycle is very difficult, due to numerous stakeholder concerns. Thus, this paper mainly focuses on the identification of key stakeholder concerns from the aspect of society, in order to facilitate the establishment of links with social sustainability indicators.
To identify social sustainability indicators, a literature review, questionnaires, and semi-structured interviews were used in this study. In line with the classification of sustainability indicators in existing literature, the social sustainability indicators were generalized, and initial indicator lists were formed. Next, experts who have participated in or are currently participating in GBPs and who are well-known scholars in the field were selected. The experts were selected from representative stakeholder groups, including the government, main contractors, designers, developers, consultants, and research institutions. They were invited to semi-structured interviews, to complete the social sustainability indicator frame. At the same time, experts were invited to rate the social sustainability indicators according to a 5-point Likert scale. These methods were also used to identify stakeholder concerns.
After reaching a consensus on social sustainability indicators and identifying stakeholder concerns, this paper has established causal relationships between the nodes of the BN through the following steps:
Step 1:. According to the social sustainability of green buildings, the respective importance of each social sustainability indicator can be assessed.
Step 2:. According to each social sustainability indicator, the importance and applicability of the GBP stakeholders’ concerns can be assessed.
Step 3:. According to each social sustainability indicator and stakeholder concern, the interdependency between them can be assessed.
After identifying nodes and determining the causal relationship between them via expert interviews, the network structure was constructed. Then, the network parameters could be identified from the training data set, in order to obtain the conditional probability distribution (CPD) of each node. If the network structure is known and the data is complete, maximum likelihood estimation (MLE) and Bayesian maximum a posteriori probability (MAP) are the most common methods used for parameter estimation.
The MLE assesses the degree of fit between the sample and the model based on the likelihood of the sample and the parameter. The form of the likelihood function is shown as Eq. 1:
where
If the distribution function of the variable is known, the maximum likelihood value can be obtained by using the Lagrange multiplier method of the above formula, thereby obtaining an estimate of the parameter. This paper used MLE, due to the advantages that method offers, such as consistency, asymptotic efficiency, and representative invariant.
However, in actual investigations, missing data is inevitable. Different situations cause separate mechanisms of data to be missing. The expectation-maximization (EM) method is a common iterative method (based on MLE) that can be applied in cases of missing data. Compared with the traditional missing data repair method, the EM method is based on a rigorous theoretical basis and proof of convergence. The entire EM algorithm is a repeated iteration of the step expectation (E) and step maximization (M). The iteration process can be described as follows:
Assuming that the missing data satisfy the missing at random (MAR) hypothesis, the likelihood function of parameter
where
Because this integral exists, maximizing
1) Replace missing values with estimated (or expected) values (step E):
2) Identify the parameter
3) Use these new parameters to re-estimate missing values (step E).
4) Re-estimate the parameters (step M), and iterate until convergence. Steps E and M are repeated until the preset parameter convergence condition
In BN parameter learning, parameter
1) Diagnostic analysis. The forms of inference of conditional probability in BNs mainly include causal inference, diagnostic inference, and supporting inference. The main application of this paper uses diagnostic inference, which is also referred to as “reverse inference”. The bottom-up reverse inference is an application of inference from the result of the reason. The posterior probability distribution of each indicator is calculated via diagnostic analysis, when the sustainability criterion is reached. Then, the factors that affect the achievement of the project’s sustainable goals can be identified in time. Project decision-makers can also identify relevant stakeholder concerns, thus optimizing their decision-making. If the stakeholder concern is
where s represents the state of event S, reached by the sustainability index with
2) Sensitivity analysis. A sensitivity analysis can calculate the degree of influence each variable exerts on other variables, i.e., the procedure can be used to analyze stakeholder concerns that are sensitive to changes in social sustainability indicators. A sensitivity analysis can accurately determine the contributions of different stakeholder concerns to the occurrence of social sustainability indicators. In general, a sensitivity analysis can identify changes in the probability of network node status by changing the configuration parameters of the node. This paper uses the sensitivity performance measure (SPM) to measure the contribution of each stakeholder concern
where s represents the state of event S, reached by the sustainability index with
After the completion of the third stage, the results of BN analysis can be applied to decision-making, in order to address social sustainability issues. Stakeholder concerns that are sensitive to (and have a direct impact on) social sustainability can be identified. Then, relevant stakeholder concerns can be implemented, according to the specific factors that affect the realization of the social sustainability goals of the GBP, effectively enabling reasonable decision-making. In addition, according to the stakeholder concern, the model can be reversed to relevant stakeholders, and the sustainable goals of the project can be implemented in a targeted manner. Similarly, these sustainable management measures provide feedback and thus enable optimization of a BN model that has previously been established.
This research has adopted a case study approach to explore the interactions between stakeholder concerns and the social sustainability of a unique GBP. The emphasis here is more on “how” and “why”, rather than “what”. The case study is considered applicable when the study contains various relationships/factors whose interactions are the research focus, and when “how” and “why” questions are considered (Phelan 2011). Furthermore, the collection of the data that are required to identify stakeholder concerns requires several interactions with project stakeholders, in order to generate context-dependent knowledge and minimum intervention on the part of the investigator. As such, using case study methods would be suitable.
Case selection is a rigorous process and should fulfill three criteria. Firstly, a wide range of stakeholders should be involved, as these are the sources of stakeholder concerns. Secondly, major GBPs should be considered, as they usually involve many stakeholders, thereby making the stakeholder concern analysis more meaningful. Lastly, ongoing GBPs should be chosen, as comprehensive information can then be collected. The selected case meets these criteria, and more details are described in the following section.
As the first green building research pilot city in China, Wuhan occupies a pivotal position in the development of green buildings. Wuhan has made exploratory efforts in green building design, construction, and promotion. The number of GBPs that have been approved by the national three-star certification department is among the highest in China. Wuhan’s green building development is representative of Central China. Thus, this paper selected GBPs in Wuhan as a database.
The Wuhan International Commerce Center (WHICC) is a landmark building on Yangtze River Avenue in Wuhan. The front drawing of the WHICC is illustrated in Figure 3. At the project design stage, the concept of “green development, circular development, and low-carbon development” was fully implemented, some state-of-the-art sustainable technologies were also adopted. The total project investment is $257.7 million, including two 230-m-high twin towers that integrate business, life, commerce, culture, ecology, and leisure. These two buildings have a construction area of approximately 209,000 m2 and have obtained LEED gold certification from the United States, as well as three-star certification for green buildings in China. Therefore, this project was selected as a representative case study.
Preparation of the BN model requires four elements, namely: 1) key stakeholder concerns and social sustainability indicators, 2) the decision variables and the possible states that characterize a GBP, 3) the causality of the decision variables, and 4) CPT for each relation in the network. To obtain these sorts of data, a literature review, semi-structured interviews, and a questionnaire survey were conducted in this study. Firstly, a literature review was adopted to construct the preliminary list of 26 stakeholder concerns and 20 sustainability indicators. Secondly, semi-structured interviews were conducted, in order to identify key stakeholder concerns, social sustainability indicators, decision variables, and causality presented in the form of a matrix. After experts had identified the preliminary list, according to their experience and the practical conditions of the WHICC project, several of the concerns and indicators were removed, since they were duplicated with other indicators and imposed little impact on the social sustainability of the project. For example, stakeholder concern “Reduction of environmental pollution” is more related to environmental sustainability. Ultimately, 12 key stakeholder concerns and 15 social sustainability indicators (selected by at least seven respondents) were identified (Qazi et al., 2016). Although the responses varied in relation to past experiences and general understanding of respondents, we could find some common themes emerging from the matrices. Lastly, a questionnaire survey was used as training samples to obtain the BN parameters using the MLE algorithm, in order to construct a CPT.
The semi-structured interview was conducted between July and October 2019. Due to the inherent difficulty of interviewing all stakeholders, this study selected and interviewed representative stakeholder groups, including the government, main contractors, designers, developers, consultants, and research institutions (Yu et al., 2019). To assure data representativeness, all interviewed and surveyed stakeholders were at senior management level or had 10+ years’ work experience in sustainable or green construction-related industries or in relevant disciplines (Li et al., 2018b). In addition, all experts were directly involved in the development, with in-depth knowledge of stakeholder issues throughout the project. According to the guidelines of Mok et al. (2018), the number of experts should between eight and 20 when applying semi-structured interviews. Based on work experience and expertise in the WHICC project, 13 experts were identified, this is the same number as in the research of Qazi et al. (2016). Table 2 shows the interviewees’ detailed information. Furthermore, two main principles were applied when selecting these experts. First, they are all stakeholders involved in the WHICC project. Second, they have rich experience in green building management and decision-making (Zhuang et al., 2019). To ensure the reliability and objectiveness of the collected data, a neutral relationship was maintained with all interviewees. The interviews were audiotaped with the permission of the respondents, in order to obviate any misrepresentation. After the completion of the interviews, data were internally validated, and the results were reported back to the interviewees, to facilitate the identification of fuzzy areas. Subsequently, the consensus was shared with the interviewees, for validation purposes.
The questionnaire survey was designed to classify the importance of the stakeholder concerns and social sustainability indicators identified above. Then, the survey was used as training samples, to obtain the BN parameters. This study used a chain referral sampling method, where all the respondents were selected based on their rich experience in GBPs. The questionnaire respondents included project managers, project engineers, professional designers, etc. This is due to their in-depth knowledge of green building and the fact that their concerns had a substantial impact on project sustainability level (Wu et al., 2017). Table 3 shows the demographic information of respondents. The data were measured by a 5-point Likert scale, where one represents very less important, and five represents very important. A total of 205 questionnaires were distributed, from November to December 2019, and 147 valid questionnaires were collected in total. Then, STATA15.1 software was used to perform a statistical analysis on the questionnaire, in order to ensure internal consistency. The Cronbach’s α coefficient is 0.899, which exceeds 0.80. This finding indicates that the inherent reliability of the questionnaire is reasonable. The KMO value is 0.871, which exceeds 0.8, indicating that the validity of the factor analysis is also very good. The Bartlett test result is p < 0.001, which is significant. This finding indicates that the validity is good, and factor analysis can be performed. Therefore, this questionnaire is credible and meets the requirements of statistical analysis.
The establishment of a BN model generally follows two steps. The first step obtains the structure of the BN, based on expert experience and the results of the structure learning algorithm. The second step obtains the parameters of each node of the BN through expert formulation and a parameter learning algorithm. A semi-structured interview was used to determine the causal relationship between stakeholder concerns and social sustainability indicators, in order to construct the BN. Then, the training data set was obtained via the questionnaire survey, in order to achieve the parameter learning of the BN, the probabilities of various node states were obtained accordingly. When the BN structure was known and the data were complete, MLE was applied, in order to estimate parameters through Eq. 1. The EM method was used to analyze missing data, obtain effective CPT parameters, and complete BN parameter learning. The steps in EM followed Eqs 2–4.
Based on previous stakeholder concerns and the identification of social sustainability indicators for the WHICC project, the potential causal relationships between them are identified. This study used the MLE algorithm to obtain the BN parameters from the training samples obtained in the questionnaire survey. Each node has three states, namely State 0, State 1, and State 2, which in turn correspond to high, medium, and low sustainability levels, respectively. If the probability of State 0 for this node is higher, this indicates this type of stakeholder concern or social sustainability indicator can highly improve the social sustainability level of the project, and vice versa. The software GeNIe 2.3 was applied to analyze the training samples and run the BN model, as shown in Figure 4. The model is shown to consist of 27 nodes, including 12 stakeholder concerns and 15 social sustainability indicators that could promote the project sustainability of the WHICC. In Figure 4, numbers C1-C12 represent stakeholder concerns, while S1-S15 represent social sustainability indicators, the arrows indicate causal relationships among different nodes. The BN node number and explanation are shown in Table 4.
The conditional probability distribution of each node is obtained through parameter learning, the social sustainability indicators are classified into three levels: low, medium, and high. In Figure 4, State 0 represents high, State 1 represents medium, and State 2 represents low. Then, BN parameter learning is divided into two stages: the initialization of the parameter and the matching of the access data with the BN. The initialization parameter is set to the average value 1/3 to realize the uniform distribution of the probability value of each node. Before importing the data into GeNIe software, the data must be standardized via Access software, all of the software standardization results were then matched. The MLE algorithm does not require a priori probability values and can be executed by importing data from Access. Therefore, this algorithm was selected for this study. Figure 4 also shows the parameter learning results of this case.
In addition, this paper calculates the strength of the influence between parent nodes (i.e., stakeholder concerns) and child nodes (i.e., social sustainability indicators), according to previous parameter learning results. The strength of influence in this study indicates the strength of the influence a parent has on a child. Here, “influence” essentially expresses a specific form of distance between various conditional probability distributions on child nodes, conditioned on the states of the parent node. Table 5 shows the 10 strongest influences. As a result, green design and construction (C4), convenient service facilities surrounding the GBP (C3), and abundance and stability of project funds (C9) all exert a significant impact on wages and welfare (S11). Therefore, the project team should focus on these stakeholder concerns and increase wages and welfare accordingly, in order to improve the social sustainability level of the project.
Diagnostic analysis via Eq. 5 identifies the posterior probability distribution of each indicator once the sustainability criterion is reached. According to the results of the strength of their influence, the State 0 of node S11 was set to 100%, i.e., the high level of wages and benefits that meet the socially sustainable evaluation criteria are certain. The results are depicted in Figure 5. As a result, nodes C3 (convenient service facilities surrounding the GBP), C4 (green design and construction), and C9 (abundance and stability of project funds) are influenced by node S11 (wages and welfare). Specifically, the probability of State 0 for node C3 changes from 24 to 15%, the probability of State 1 for node C4 changes from 27 to 22%, and the probability of State 1 for node C9 changes from 31 to 18%. These findings indicate that the sustainable performance of the project can be more effectively increased by changing these factors.
The sensitivity analysis analyzes and calculates the change of the parent node when the child node of the network changes, the analysis also identifies the parent node that has a greater impact on the child node. Figure 6 shows the sensitivity analysis result of the BN-based model, which used S9 as the target node. The dark-colored box indicates a sensitive factor. Nodes C2, C3, C4, C5, C9, C10, and C11 were identified as sensitive stakeholder concerns. This indicates that these concerns impose significant effects on S9 (social benefits). Therefore, the sustainability level of social welfare can be improved by changing these stakeholder concerns.
However, since S9 can only represent one aspect of the social sustainability level, other sustainability indicators must necessarily be assessed. To increase the comprehensiveness of the results of the sensitivity analysis, all stakeholder concerns introduced in this paper were analyzed. That is, all indicators were analyzed step-by-step, the union value was taken, and Eq. 6 was used to calculate the sensitive performance. In Figure 7, C4 (green design and construction), C9 (abundance and stability of project funds), and C3 (convenient service facilities surrounding the GBP) were identified as the three most sensitive stakeholder concerns. Accordingly, these three sensitive stakeholder concerns should receive major attention, given their significant effect on the overall social sustainability level of the GBP.
This study adopted the validation framework proposed by Pollino et al. (2007), who refers to the concept of “sensitivity to findings”. This method can test the predictive validity of expert-elicited networks by analyzing the degree of consistency between critical factors and sensitive factors. This method has been widely used in various BN-based studies (Pitchforth and Mengersen 2013; Yu et al., 2019). Therefore, this technique is used in this study to validate the SCSM model based on BN. The case study showed that C4 (green design and construction), C3 (convenient service facilities surrounding the GBP), and C9 (abundance and stability of project funds) were the key stakeholder concerns that significantly influence social sustainability. In the diagnostic analysis of the BN, C3, C4, and C9 were additional important concerns, affecting the social sustainability performance of the WHICC project. Concerning the sensitivity analysis of S9 (i.e., social benefits), the results show that C3, C4, and C9 are included in the sensitive concerns. In addition, S11 (i.e., wages and welfare), S2 (i.e., minimizing neighborhood disturbance), S13 (i.e., obeying laws and regulations), and S5 (i.e., market supply and demand) are identified as influential social sustainability indicators (Table 5). Figure 4 shows that C3, C4, C9, C8, C10, and C12 are linked with these nodes. This implies that these sensitive stakeholder concerns are closely related to the key social sustainability indicators, which in turn further supports the results presented above. In summary, the strengths of the influence analysis, diagnostic analysis, and sensitivity analysis were highly consistent, thus strongly verifying the reliability of the data analysis. Following this verification, the results were fed back to the 13 previously-interviewed experts, to evaluate the credibility of the results. As a result, most of the experts (at least 7) supported the key stakeholder concerns identified in this case. Furthermore, to ensure the validity of the BN model, this study asked experts for the reasons why they agreed or disagreed with the results (Yu et al., 2019).
Existing stakeholder management frameworks have primarily focused on representing various aspects of project complexity, risk, and sustainability (Zhao et al., 2016; Mok et al., 2018; Bohari et al., 2020). Despite the fact that a few studies have focused on the complexity of stakeholders and their concerns (Mok et al., 2017b, 2018; Tang et al., 2018), until now, no attempt has been made to adequately capture the interdependency between stakeholder concern and social sustainability (Chen et al., 2021a; Wu et al., 2021a; Wu et al., 2021b). As a result, there is a need to develop a decision-making model that recognizes the importance of interdependency within complex stakeholder concerns in order to achieve social sustainability. SCSM makes an effort to contribute to this new approach. With regard to social sustainability management in construction projects, the importance of decreasing travel time and the availability of amenities have been highlighted before Sierra et al. (2018). This study also identifies C3 (i.e., convenient service facilities surrounding the GBP) as a key stakeholder concern. One can see that both traditional construction projects and green construction projects must increase investment in convenience facilities and services if they are to increase their level of social sustainability. A project manager in this case study reported that, “Convenient transportation and basic service facilities are very important. This is mainly because the procurement and transportation of building materials will take a long time. If there is no developed transportation network in place, transportation will become an enormous problem.” Next, basic service facilities should be improved so that they meet the basic living needs of managers and workers on site. For example, due to site restrictions, there is no employee restaurant, and thus, employees need to go out to buy their food. However, there is no supporting restaurant nearby, so their work is greatly hindered.
The abundance and stability of project funds are also significant factors for sustainability decision-making. This result is consistent with Qazi et al. (2016), who addressed stakeholder complexity in order to identify major pitfalls in large cultural building projects. To facilitate sustainable design and construction, adequate financial support is an essential prerequisite. Construction projects commonly face problems of financial instability, this issue was also identified in this case study. The design and construction stage involves many wearisome processes, such as design changes, claims, and acceptance, all of which induce many uncertain factors that can affect the stable payment of funds. In this case study, many unfavorable consequences were caused by insufficient funds. For example, project team members became frustrated, workers could not be recruited, and there was even disruption at the construction site. Therefore, adequate and stable financial support forms the basic guarantee for increasing the social sustainability level of GBPs.
In this case study, green design and construction play an important role in improving the social sustainability level of the project. This result is inconsistent with those of Li et al. (2012), who reported that most of the rankings for this stakeholder concern are low. The main reason for this conflict in findings is that the research background of both studies differs. The research of Li et al. (2012) focused on large public infrastructure projects, while the present study focuses on GBPs. Firstly, the stakeholders involved in the project have different concerns. Public infrastructure projects are usually organized and implemented by governmental departments. These pay more attention to the employment opportunities, economic benefits, and regional economic development induced by the project. Therefore, projects of this nature often ignore sustainability issues. Secondly, in the case of the Wuhan GBP, the concept of green and sustainable development was determined at the initial stage. To meet the evaluation standards of green buildings, many advanced technologies and materials have been applied. These evaluations involve every stage of the project, thus, the project leaders have to thoroughly evaluate each acceptance standard and refine any relevant processes that do not meet these criteria. As such, this project ultimately reached the three-star standard of green building by implementing green and sustainable construction into the whole life cycle.
In existing empirical studies BN has been widely employed in project risk management (Chen et al., 2021b; Koseoglu Balta et al., 2021). However, very few studies have used the technique to manage project sustainability. The frequently-used methods of social network analysis (SNA), analytical network process (ANP), and structural equation modelling (SEM) (all of which are used to model stakeholder involvement and project complexity) can explain the interrelationships between stakeholders. However, these methods are stretched when faced with how two related factors behave according to each other’s changes. This paper refers to other experts’ experiences and implements Bayesian inference to address this problem (Qazi et al., 2016; Bakshan et al., 2017; Yu et al., 2019). Furthermore, few studies on social sustainability are available, the level of social sustainability is also difficult to quantify. Compared with previous studies on stakeholder concerns and social sustainability indicators (Mok et al., 2018; Goel et al., 2020; Yadegaridehkordi et al., 2020; Zhang and Mohandes 2020), this paper integrates both and analyzes the uncertain relationship between them. By using BN to model this uncertain relationship, the most important stakeholder concerns—i.e., those that affect the social sustainability of the project—are identified. The accuracy of project decision-making is also improved by employing optimal sustainability improvement strategies. This paper proposes an exploratory effort to address the uncertainty problem of GBPs’ social sustainability, as well as a SCSM analysis approach for a real-world case. In addition, a solid reference for sustainable management decisions of similar projects is also provided. Managers can visualize the interaction between stakeholder concerns and social sustainability, they can also appreciate propagation patterns through sustainability paths and locate key concerns, thus promoting the success of a project’s sustainability management.
The complexity of stakeholder concerns can hinder decision-making, thereby further affecting the sustainability level of GBPs. Through a review of the literature on stakeholder concerns and interdependency modeling of social sustainability in GBPs, this study establishes a major research gap, namely establishing an SCSM process, exploring the interdependency modeling of stakeholder concerns-driven social sustainability. The illustrative application of this approach gives an insight into understanding the dynamics across the entire spectrum of sustainability management. Specifically, this paper uses literature reviews, semi-structured interviews, questionnaires and obtained data to analyze stakeholder concerns and social sustainability indicators. The probability and causality of the network nodes are identified according to the results of interviews. Then, a BN model is established, and a BN diagnostic analysis and sensitivity analysis are used to identify the stakeholder concerns that have the greatest influence on the social sustainability goals. A project case in Wuhan is used to test the SCSM model for decision-making. The results identify green design and construction, convenient service facilities surrounding the project, and the abundance and stability of project funds as the three main stakeholder concerns that exert a significant impact on the social sustainability level of the GBP. These findings confirm that project managers should consider the complex interaction between project sustainability objectives and stakeholder concerns, rather than relying solely on past experiences with GBPs. Through the use of BN decision-making analysis, the findings of this work contribute to the social sustainability of the green building industry and open up new research avenues. This study also establishes an SCSM framework for a real-world case, which can be used as a guide for making sustainable management decisions for comparable GBPs.
In theory, according to previous research on GBPs (Qazi et al., 2016; Mok et al., 2018), interactions between different stakeholders and their individual concerns are characterized by uncertainties. The SNA, ANP, and SEM methods are widely used in relevant research to analyze the interrelationships between stakeholders, as well as to determine their importance. This is achieved by analyzing their position within the network. However, these types of research do not and cannot reflect the impact of uncertainty. The present study uses a BN-based model to simulate the realization of project uncertainty sustainability evaluation indicators. Using this model, the influence of stakeholder concerns is investigated, based on relevant changes. This study also bridges the areas of stakeholder concerns and social sustainability. As previously stated, there is a scarcity of study on the social sustainability of GBPs, and one of the reasons for this scarcity is the limits imposed on data acquisition. Collecting data related to the complex dynamic relationship between stakeholders is complicated, and this, coupled with non-quantifiable nature of social sustainability, makes this a particularly difficult study challenge. Based on the successful application of the BN in construction project risk assessment, this paper extends this assessment to a sustainability assessment of GBPs. This constitutes exploratory efforts to solve the uncertainty problem of social sustainability.
In practice, the research results of this article provide the project leader with more precise control of the project’s sustainability level. First, this paper identifies the indicators and stakeholders that may affect the social sustainability of the project. Project managers can use these indicators and concerns to control project quality. These indicators can be collated into a checklist, which project implementers can use to identify and specifically focus on the identified issues during the construction process, thereby reducing the need for revision. Second, this paper uses the BN model to identify key stakeholder concerns, specifically, those that exert a significant impact on social sustainability. Project leaders can focus on these concerns, which will help them to better understand the different concerns of project participants and to make more effective decisions. For example, project decision-makers can provide convenient public service facilities and effective services for project participants, which will help to improve the participants’ work performance. Third, the SCSM framework proposed in this paper can be used to assess the stakeholder concerns when faced with project social sustainability issues. Based on these concerns, project decision-makers can better understand the needs of project participants and adopt reasonable strategies to increase their awareness of social sustainability. This understanding will ultimately improve project sustainability performance. Fourth, this decision-making method can be applied to the environmental and economic sustainability assessment of the project. When confronted with a specific case, the same process can be used to identify variables and create causal links, resulting in a sustainable strategy that is unique to and appropriate for the project.
This study has some limitations. First, in the process of the identification of stakeholder concerns and social sustainability indicators, this study relied on questionnaires and interviews when determining their causality. This process is very laborious and increases the subjectivity of the results. In future studies, large samples of data can be collected instead and entered into the model to produce more objective results. Second, the SCSM approach is applied in a GBP located in Wuhan, the results may not be suitable for other types of industries because more concerns may emerge with the complexity of construction projects. Future work will validate the approach in the context of different industries and take more project-based stakeholder concerns into consideration through case studies to get a deeper insight into how the social sustainability can be improved in GBPs.
The original contributions presented in the study are included in the article/Supplementary Material, further inquiries can be directed to the corresponding author.
SW wrote the first draft of the manuscript. GQ contributed to the conception and design of the study. All authors discussed the results and contributed specific knowledge of the relevant literature.
This study is supported by the National Natural Science Foundation of China (Grant Nos 71972018 and 71561009).
The authors declare that the research was conducted in the absence of any commercial or financial relationships that could be construed as a potential conflict of interest.
All claims expressed in this article are solely those of the authors and do not necessarily represent those of their affiliated organizations, or those of the publisher, the editors and the reviewers. Any product that may be evaluated in this article, or claim that may be made by its manufacturer, is not guaranteed or endorsed by the publisher.
The Supplementary Material for this article can be found online at: https://www.frontiersin.org/articles/10.3389/fenvs.2022.874367/full#supplementary-material
Akhanova, G., Nadeem, A., Kim, J. R., and Azhar, S. (2020). A Multi-Criteria Decision-Making Framework for Building Sustainability Assessment in Kazakhstan. Sust. Cities Soc. 52 (1), 101842. doi:10.1016/j.scs.2019.101842
Al-Jebouri, M. F. A., Saleh, M. S., Raman, S. N., Rahmat, R. A. A. B. O. K., and Shaaban, A. K. (2017). Toward a National Sustainable Building Assessment System in Oman: Assessment Categories and Their Performance Indicators. Sust. Cities Soc. 31 (5), 122–135. doi:10.1016/j.scs.2017.02.014
Almahmoud, E., and Doloi, H. K. (2015). Assessment of Social Sustainability in Construction Projects Using Social Network Analysis. Facilities 33 (3–4), 152–176. doi:10.1108/f-05-2013-0042
Bakshan, A., Srour, I., Chehab, G., El-Fadel, M., and Karaziwan, J. (2017). Behavioral Determinants towards Enhancing Construction Waste Management: A Bayesian Network Analysis. Resour. Conservation Recycling 117 (2), 274–284. doi:10.1016/j.resconrec.2016.10.006
Bal, M., Bryde, D., Fearon, D., and Ochieng, E. (2013). Stakeholder Engagement: Achieving Sustainability in the Construction Sector. Sustainability 5 (2), 695–710. doi:10.3390/su5020695
Bohari, A. A. M., Skitmore, M., Xia, B., Teo, M., and Khalil, N. (2020). Key Stakeholder Values in Encouraging green Orientation of Construction Procurement. J. Clean. Prod. 270 (10), 122246. doi:10.1016/j.jclepro.2020.122246
Burga, R., and Rezania, D. (2017). Project Accountability: An Exploratory Case Study Using Actor-Network Theory. Int. J. Project Manag. 35 (6), 1024–1036. doi:10.1016/j.ijproman.2017.05.001
Cao, D., Li, H., Wang, G., Luo, X., Yang, X., and Tan, D. (2016). Dynamics of Project-Based Collaborative Networks for BIM Implementation: Analysis Based on Stochastic Actor-Oriented Models. J. Manag. Eng. 33 (3), 04016055.
Castillo, E., Calviño, A., Grande, Z., Sánchez-Cambronero, S., Gallego, I., Rivas, A., et al. (2016). A Markovian-Bayesian Network for Risk Analysis of High Speed and Conventional Railway Lines Integrating Human Errors. Computer-Aided Civil Infrastructure Eng. 31 (3), 193–218. doi:10.1111/mice.12153
Chen, C., Zhang, L., and Tiong, R. L. K. (2019). A Novel Learning Cloud Bayesian Network for Risk Measurement. Appl. Soft Comput. J. 87 (11), 105947.
Chen, L., Gao, X., Hua, C., Gong, S., and Yue, A. (2021a). Evolutionary Process of Promoting green Building Technologies Adoption in China: A Perspective of Government. J. Clean. Prod. 279 (1), 123607. doi:10.1016/j.jclepro.2020.123607
Chen, L., Lu, Q., Li, S., He, W., and Yang, J. (2021b). Bayesian Monte Carlo Simulation-Driven Approach for Construction Schedule Risk Inference. J. Manage. Eng. 37 (2), 04020115. doi:10.1061/(asce)me.1943-5479.0000884
Chen, R.-H., Lin, Y., and Tseng, M.-L. (2015). Multicriteria Analysis of Sustainable Development Indicators in the Construction Minerals Industry in China. Resour. Pol. 46 (12), 123–133. doi:10.1016/j.resourpol.2014.10.012
Chong, Y. T., Teo, K. M., and Tang, L. C. (2016). A Lifecycle-Based Sustainability Indicator Framework for Waste-To-Energy Systems and a Proposed Metric of Sustainability. Renew. Sust. Energ. Rev. 56 (4), 797–809. doi:10.1016/j.rser.2015.11.036
Control, P. P., Performance, P. M., and Contexts, D. (2008). Stakeholders’ Attributes, Behaviors, and Decision-Making Strategies in Construction Projects: Importance and Correlations in Practice. Project Manag. J. 39 (3), 28–42.
Dansoh, A., Frimpong, S., and Oppong, G. D. (2020). Exploring the Dimensions of Traditional Authority Influencing Stakeholder Management at the Pre-construction Stage of Infrastructure Projects. Construction Manag. Econ. 38 (2), 189–206. doi:10.1080/01446193.2019.1589647
Darko, A., Chan, A. P. C., Huo, X., and Owusu-Manu, D.-G. (2019). A Scientometric Analysis and Visualization of Global green Building Research. Building Environ. 149 (12), 501–511. doi:10.1016/j.buildenv.2018.12.059
Doloi, H. (2013). Cost Overruns and Failure in Project Management: Understanding the Roles of Key Stakeholders in Construction Projects. J. Constr. Eng. Manage. 139 (3), 267–279. doi:10.1061/(asce)co.1943-7862.0000621
Fang, A., Yuan, K., Geng, J., and Wei, X. (2020). Opinion Dynamics with Bayesian Learning. Complexity 2020 (2), 8261392. doi:10.1155/2020/8261392
Fernández-Sánchez, G., and Rodríguez-López, F. (2010). A Methodology to Identify Sustainability Indicators in Construction Project Management - Application to Infrastructure Projects in Spain. Ecol. Indicators 10 (6), 1193–1201.
Francisco de Oliveira, G., and Rabechini Jr, R. (2019). Stakeholder Management Influence on Trust in a Project: A Quantitative Study. Int. J. Project Manag. 37 (1), 131–144. doi:10.1016/j.ijproman.2018.11.001
Goel, A., Ganesh, L. S., and Kaur, A. (2020). Social Sustainability Considerations in Construction Project Feasibility Study: a Stakeholder Salience Perspective. Ecam 27 (7), 1429–1459. doi:10.1108/ecam-06-2019-0319
González-Rodríguez, M. R., and Tussyadiah, I. (2021). Sustainable Development in Nature-Based Destinations. The Social Dilemma of an Environmental Policy. Sust. Dev. 10 (9), 1–15. doi:10.1002/sd.2250
He, Z., Huang, D., Fang, J., and Wang, B. (2020). Stakeholder Conflict Amplification of Large-Scale Engineering Projects in China: An Evolutionary Game Model on Complex Networks. Complexity 2020 (8), 9243427. doi:10.1155/2020/9243427
Heravi, G., Fathi, M., and Faeghi, S. (2015). Evaluation of Sustainability Indicators of Industrial Buildings Focused on Petrochemical Projects. J. Clean. Prod. 109 (12), 92–107. doi:10.1016/j.jclepro.2015.06.133
Hwang, B.-G., and Ng, W. J. (2013). Project Management Knowledge and Skills for green Construction: Overcoming Challenges. Int. J. Project Manag. 31 (2), 272–284. doi:10.1016/j.ijproman.2012.05.004
Hwang, B.-G., and Tan, J. S. (2012). Green Building Project Management: Obstacles and Solutions for Sustainable Development. Sust. Dev. 20 (5), 335–349. doi:10.1002/sd.492
Institute, P. M. (2009). A Guide to the Project Management Body of Knowledge. Project Manag. J. 40 (2), 104.
Journeault, M., Perron, A., and Vallières, L. (2021). The Collaborative Roles of Stakeholders in Supporting the Adoption of Sustainability in SMEs. J. Environ. Manage. 287 (6), 112349. doi:10.1016/j.jenvman.2021.112349
Keeys, L. A., and Huemann, M. (2017). Project Benefits Co-creation: Shaping Sustainable Development Benefits. Int. J. Project Manag. 35 (6), 1196–1212. doi:10.1016/j.ijproman.2017.02.008
Khan, G. F., Sarstedt, M., Shiau, W.-L., Hair, J. F., Ringle, C. M., and Fritze, M. P. (2019). Methodological Research on Partial Least Squares Structural Equation Modeling (PLS-SEM). Intr 29 (3), 407–429. doi:10.1108/intr-12-2017-0509
Kiani Mavi, R., and Standing, C. (2018). Critical success Factors of Sustainable Project Management in Construction: A Fuzzy DEMATEL-ANP Approach. J. Clean. Prod. 194 (9), 751–765. doi:10.1016/j.jclepro.2018.05.120
Koseoglu Balta, G. C., Dikmen, I., and Birgonul, M. T. (2021). Bayesian Network Based Decision Support for Predicting and Mitigating Delay Risk in TBM Tunnel Projects. Automation in Construction 129 (6), 103819. doi:10.1016/j.autcon.2021.103819
Kosgodagan-Dalla Torre, A., Yeung, T. G., Morales-Nápoles, O., Castanier, B., Maljaars, J., and Courage, W. (2017). A Two-Dimension Dynamic Bayesian Network for Large-Scale Degradation Modeling with an Application to a Bridges Network. Computer-Aided Civil Infrastructure Eng. 32 (8), 641–656. doi:10.1111/mice.12286
Kumar, S., and Banerji, H. (2022). Bayesian Network Modeling for Economic-Socio-Cultural Sustainability of Neighborhood-Level Urban Communities: Reflections from Kolkata, an Indian Megacity. Sust. Cities Soc. 78 (3), 103632. doi:10.1016/j.scs.2021.103632
Lam, J. C. K., Cheung, L. Y. L., Wang, S., and Li, V. O. K. (2019). Stakeholder Concerns of Air Pollution in Hong Kong and Policy Implications: A Big-Data Computational Text Analysis Approach. Environ. Sci. Pol. 101 (7), 374–382. doi:10.1016/j.envsci.2019.07.007
Landorf, C. (2011). Evaluating Social Sustainability in Historic Urban Environments. Int. J. Heritage Stud. 17 (5), 463–477. doi:10.1080/13527258.2011.563788
Lee, C.-Y., Chong, H.-Y., Liao, P.-C., and Wang, X. (2018). Critical Review of Social Network Analysis Applications in Complex Project Management. J. Manag. Eng. 34 (2), 1–15. doi:10.1061/(asce)me.1943-5479.0000579
Li, H., Ng, S. T., and Skitmore, M. (2018a). Stakeholder Impact Analysis during post-occupancy Evaluation of green Buildings - A Chinese Context. Building Environ. 128 (1), 89–95. doi:10.1016/j.buildenv.2017.11.014
Li, H., Zhang, X., Ng, S. T., and Skitmore, M. (2018b). Quantifying Stakeholder Influence in Decision/evaluations Relating to Sustainable Construction in China - A Delphi Approach. J. Clean. Prod. 173 (2), 160–170. doi:10.1016/j.jclepro.2017.04.151
Li, T. H. Y., Ng, S. T., and Skitmore, M. (2012). Conflict or Consensus: An Investigation of Stakeholder Concerns during the Participation Process of Major Infrastructure and Construction Projects in Hong Kong. Habitat Int. 36 (2), 333–342. doi:10.1016/j.habitatint.2011.10.012
Li, T. H. Y., Ng, S. T. T., Skitmore, M., and Li, N. (2016). Investigating Stakeholder Concerns during Public Participation. Proc. Inst. Civil Eng. - Municipal Engineer 169 (4), 199–219. doi:10.1680/jmuen.15.00018
Li, X., Li, H., Cao, D., Tang, Y., Luo, X., and Wang, G. (2019). Modeling Dynamics of Project-Based Collaborative Networks for BIM Implementation in the Construction Industry: Empirical Study in Hong Kong. J. Construction Eng. Manag. 145 (12), 5019013. doi:10.1061/(asce)co.1943-7862.0001726
Lin, H., Zeng, S., Ma, H., Zeng, R., and Tam, V. W. Y. (2017a). An Indicator System for Evaluating Megaproject Social Responsibility. Int. J. Project Manag. 35 (7), 1415–1426. doi:10.1016/j.ijproman.2017.04.009
Lin, X., Ho, C. M. F., and Shen, G. Q. P. (2017b). Who Should Take the Responsibility? Stakeholders' Power over Social Responsibility Issues in Construction Projects. J. Clean. Prod. 154 (6), 318–329. doi:10.1016/j.jclepro.2017.04.007
Lin, X., McKenna, B., Ho, C. M. F., and Shen, G. Q. P. (2019). Stakeholders' Influence Strategies on Social Responsibility Implementation in Construction Projects. J. Clean. Prod. 235 (10), 348–358. doi:10.1016/j.jclepro.2019.06.253
Luo, L., He, Q., Xie, J., Yang, D., and Wu, G. (2017). Investigating the Relationship between Project Complexity and Success in Complex Construction Projects. J. Manage. Eng. 33 (2), 04016036. doi:10.1061/(asce)me.1943-5479.0000471
Mkrtchyan, L., Podofillini, L., and Dang, V. N. (2016). Methods for Building Conditional Probability Tables of Bayesian Belief Networks from Limited Judgment: An Evaluation for Human Reliability Application. Reliability Eng. Syst. Saf. 151 (7), 93–112. doi:10.1016/j.ress.2016.01.004
Mok, K. Y., Shen, G. Q., and Yang, J. (2015). Stakeholder Management Studies in Mega Construction Projects: A Review and Future Directions. Int. J. Project Manag. 33 (2), 446–457. doi:10.1016/j.ijproman.2014.08.007
Mok, K. Y., Shen, G. Q., and Yang, R. J. (2017a). Addressing Stakeholder Complexity and Major Pitfalls in Large Cultural Building Projects. Int. J. Project Manag. 35 (3), 463–478. doi:10.1016/j.ijproman.2016.12.009
Mok, K. Y., Shen, G. Q., Yang, R. J., and Li, C. Z. (2017b). Investigating Key Challenges in Major Public Engineering Projects by a Network-Theory Based Analysis of Stakeholder Concerns: A Case Study. Int. J. Project Manag. 35 (1), 78–94. doi:10.1016/j.ijproman.2016.10.017
Mok, K. Y., Shen, G. Q., and Yang, R. (2018). Stakeholder Complexity in Large Scale green Building Projects. Ecam 25 (11), 1454–1474. doi:10.1108/ecam-09-2016-0205
Mok, M. K. Y., and Shen, G. Q. (2016). A Network-Theory Based Model for Stakeholder Analysis in Major Construction Projects. Proced. Eng. 164 (6), 292–298. doi:10.1016/j.proeng.2016.11.622
Neil, M., Fenton, N., and Nielson, L. (2000). Building Large-Scale Bayesian Networks. Knowledge Eng. Rev. 15 (3), 257–284. doi:10.1017/s0269888900003039
Nepal, B., and Yadav, O. P. (2015). Bayesian Belief Network-Based Framework for Sourcing Risk Analysis during Supplier Selection. Int. J. Prod. Res. 53 (20), 6114–6135. doi:10.1080/00207543.2015.1027011
Olakitan Atanda, J. (2019). Developing a Social Sustainability Assessment Framework. Sust. Cities Soc. 44 (1), 237–252. doi:10.1016/j.scs.2018.09.023
Olander, S., and Landin, A. (2005). Evaluation of Stakeholder Influence in the Implementation of Construction Projects. Int. J. Project Manag. 23 (4), 321–328. doi:10.1016/j.ijproman.2005.02.002
Olanipekun, A. O., Chan, A. P. C., Xia, B., Ameyaw, E. E., and Ameyaw, E. E. (2017). Indicators of Owner Commitment for Successful Delivery of green Building Projects. Ecol. Indicators 72 (1), 268–277. doi:10.1016/j.ecolind.2016.08.017
Paper, T. (2020). Stakeholder Perceptions on the Future Application of Construction Robots for Buildings in a Dialectical System Framework. J. Manag. Eng. 36 (6), 1–13.
Phelan, S. (2011). Case Study Research: Design and Methods. Eval. Res. Edu. 24 (3), 221–222. doi:10.1080/09500790.2011.582317
Pitchforth, J., and Mengersen, K. (2013). A Proposed Validation Framework for Expert Elicited Bayesian Networks. Expert Syst. Appl. 40 (1), 162–167. doi:10.1016/j.eswa.2012.07.026
Pollino, C. A., Woodberry, O., Nicholson, A., Korb, K., and Hart, B. T. (2007). Parameterisation and Evaluation of a Bayesian Network for Use in an Ecological Risk Assessment. Environ. Model. Softw. 22 (8), 1140–1152. doi:10.1016/j.envsoft.2006.03.006
Qazi, A., Quigley, J., Dickson, A., and Kirytopoulos, K. (2016). Project Complexity and Risk Management (ProCRiM): Towards Modelling Project Complexity Driven Risk Paths in Construction Projects. Int. J. Project Manag. 34 (7), 1183–1198. doi:10.1016/j.ijproman.2016.05.008
Qiang, G., Cao, D., Wu, G., Zhao, X., and Zuo, J. (2021). Dynamics of Collaborative Networks for Green Building Projects: Case Study of Shanghai. J. Manage. Eng. 37 (3), 05021001. doi:10.1061/(asce)me.1943-5479.0000892
San Cristóbal, J. R., Carral, L., Diaz, E., Fraguela, J. A., and Iglesias, G. (2018). Complexity and Project Management: A General Overview. Complexity 154 (10), 121–132.
Sanchez, F., Bonjour, E., Micaelli, J.-P., Monticolo, D., and Monticolo, D. (2020). An Approach Based on Bayesian Network for Improving Project Management Maturity: An Application to Reduce Cost Overrun Risks in Engineering Projects. Comput. Industry 119 (8), 103227. doi:10.1016/j.compind.2020.103227
Schröpfer, V. L. M., Tah, J., and Kurul, E. (2017). Mapping the Knowledge Flow in Sustainable Construction Project Teams Using Social Network Analysis. Eng. Construction Architectural Manag. 24 (2), 229–259.
Seuring, S., and Gold, S. (2013). Sustainability Management beyond Corporate Boundaries: From Stakeholders to Performance. J. Clean. Prod. 56 (10), 1–6. doi:10.1016/j.jclepro.2012.11.033
Sierra, L. A., Yepes, V., García-Segura, T., and Pellicer, E. (2018). Bayesian Network Method for Decision-Making about the Social Sustainability of Infrastructure Projects. J. Clean. Prod. 176 (3), 521–534. doi:10.1016/j.jclepro.2017.12.140
Siew, C., Chong, H., Jack, L., Fuad, A., and Faris, M. (2019). Revisiting Triple Bottom Line within the Context of Sustainable Construction : A Systematic Review. J. Clean. Prod. 252 (12), 119884.
Špačková, O., and Straub, D. (2013). Dynamic Bayesian Network for Probabilistic Modeling of Tunnel Excavation Processes. Computer-Aided Civil Infrastructure Eng. 28 (1), 1–21.
Sperry, R. C., and Jetter, A. J. (2019). A Systems Approach to Project Stakeholder Management: Fuzzy Cognitive Map Modeling. Project Manag. J. 50 (6), 699–715. doi:10.1177/8756972819847870
Su, Y., Si, H., Chen, J., and Wu, G. (2020). Promoting the Sustainable Development of the Recycling Market of Construction and Demolition Waste: A Stakeholder Game Perspective. J. Clean. Prod. 277 (12), 122281. doi:10.1016/j.jclepro.2020.122281
Tang, Y., Wang, G., Li, H., and Cao, D. (2018). Dynamics of Collaborative Networks between Contractors and Subcontractors in the Construction Industry: Evidence from National Quality Award Projects in China. J. Constr. Eng. Manage. 144 (9), 05018009. doi:10.1061/(asce)co.1943-7862.0001555
Teng, J., Mu, X., Wang, W., Xu, C., and Liu, W. (2019). Strategies for Sustainable Development of green Buildings. Sust. Cities Soc. 44 (1), 215–226. doi:10.1016/j.scs.2018.09.038
Toor, S.-u. -R., and Ogunlana, S. O. (2010). Beyond the 'iron triangle': Stakeholder Perception of Key Performance Indicators (KPIs) for Large-Scale Public Sector Development Projects. Int. J. Project Manag. 28 (3), 228–236. doi:10.1016/j.ijproman.2009.05.005
Valdes-Vasquez, R., and Klotz, L. E. (2013). Social Sustainability Considerations during Planning and Design: Framework of Processes for Construction Projects. J. Constr. Eng. Manage. 139 (1), 80–89. doi:10.1061/(asce)co.1943-7862.0000566
Vuorinen, L., and Martinsuo, M. (2019). Value-oriented Stakeholder Influence on Infrastructure Projects. Int. J. Project Manag. 37 (5), 750–766. doi:10.1016/j.ijproman.2018.10.003
Wang, G., Li, Y., Zuo, J., Hu, W., Nie, Q., and Lei, H. (2021). Who Drives green Innovations? Characteristics and Policy Implications for green Building Collaborative Innovation Networks in China. Renew. Sust. Energ. Rev. 143 (3), 110875. doi:10.1016/j.rser.2021.110875
Wced, S. W. S. (1987). World Commission on Environment and Development. Our common future 17 (1), 1–91.
Weerasinghe, A. S., and Ramachandra, T. (2020). Implications of Sustainable Features Onlife‐cyclecosts of green Buildings. Sust. Dev. 28 (5), 1136–1147. doi:10.1002/sd.2064
Wei, Z., Zhang, L., Yue, Q., and Zhong, M. (2020). A Bayesian Network under Strict Chain Model for Computing Flow Risks in Smart City. Complexity 2020 (6), 1–8. doi:10.1155/2020/5920827
Winston, N. (2021). Sustainable Community Development: Integrating Social and Environmental Sustainability for Sustainable Housing and Communities. Sust. Dev. 30 (4), 1–12. doi:10.1002/sd.2238
Wu, G., Qiang, G., Zuo, J., Zhao, X., and Chang, R. (2018). What Are the Key Indicators of Mega Sustainable Construction Projects? -A Stakeholder-Network Perspective. Sustainability 10 (8), 2939. doi:10.3390/su10082939
Wu, G., Zhao, X., Zuo, J., and Zillante, G. (2017). Effects of Contractual Flexibility on Conflict and Project success in Megaprojects. Ijcma 29 (2), 253–278. doi:10.1108/ijcma-06-2017-0051
Wu, S. R., Fan, P., and Chen, J. (2016). Incorporating Culture into Sustainable Development: A Cultural Sustainability Index Framework for Green Buildings. Sust. Dev. 24 (1), 64–76. doi:10.1002/sd.1608
Wu, X., Liu, H., Zhang, L., Skibniewski, M. J., Deng, Q., and Teng, J. (2015). A Dynamic Bayesian Network Based Approach to Safety Decision Support in Tunnel Construction. Reliability Eng. Syst. Saf. 134 (2), 157–168. doi:10.1016/j.ress.2014.10.021
Wu, Z., He, Q., Chen, Q., Xue, H., and Li, S. (2021a). A Topical Network Based Analysis and Visualization of Global Research Trends on green Building from 1990 to 2020. J. Clean. Prod. 320 (3), 128818. doi:10.1016/j.jclepro.2021.128818
Wu, Z., He, Q., Yang, K., Zhang, J., and Xu, K. (2021b). Investigating the Dynamics of china’s green Building Policy Development from 1986 to 2019. Int. J. Environ. Res. Public Health 18 (1), 1–19.
Xing, E. P., Fu, W., and Song, L. (2010). A State-Space Mixed Membership Blockmodel for Dynamic Network Tomography. Ann. Appl. Stat. 4 (2), 535–566. doi:10.1214/09-aoas311
Xue, J., Shen, G. Q., Yang, R. J., Zafar, I., and Ekanayake, E. M. A. C. (2020). Dynamic Network Analysis of Stakeholder Conflicts in Megaprojects: Sixteen-Year Case of Hong Kong-Zhuhai-Macao Bridge. J. Constr. Eng. Manage. 146 (9), 04020103. doi:10.1061/(asce)co.1943-7862.0001895
Yadegaridehkordi, E., Hourmand, M., Nilashi, M., Alsolami, E., Samad, S., Mahmoud, M., et al. (2020). Assessment of Sustainability Indicators for Green Building Manufacturing Using Fuzzy Multi-Criteria Decision Making Approach. J. Clean. Prod. 277 (12), 122905. doi:10.1016/j.jclepro.2020.122905
Yang, F., Chi, G., Wang, G., Tang, S., Li, Y., and Ju, C. (2020). Untangle the Complex Stakeholder Relationships in Rural Settlement Consolidation in China: A Social Network Approach. Land 9 (7), 1–19. doi:10.3390/land9070210
Yang, R. J., and Shen, G. Q. P. (2015). Framework for Stakeholder Management in Construction Projects. J. Manage. Eng. 31 (4), 04014064. doi:10.1061/(asce)me.1943-5479.0000285
Yang, R. J., and Zou, P. X. W. (2014). Stakeholder-associated Risks and Their Interactions in Complex green Building Projects: A Social Network Model. Building Environ. 73 (3), 208–222. doi:10.1016/j.buildenv.2013.12.014
Yang, R. J., Zou, P. X. W., and Wang, J. (2016). Modelling Stakeholder-Associated Risk Networks in green Building Projects. Int. J. Project Manag. 34 (1), 66–81. doi:10.1016/j.ijproman.2015.09.010
Yu, T., Man, Q., Wang, Y., Shen, G. Q., Hong, J., Zhang, J., et al. (2019). Evaluating Different Stakeholder Impacts on the Occurrence of Quality Defects in Offsite Construction Projects: A Bayesian-Network-Based Model. J. Clean. Prod. 241 (12), 118390. doi:10.1016/j.jclepro.2019.118390
Zang, J., Royapoor, M., Acharya, K., Jonczyk, J., and Werner, D. (2022). Performance Gaps of Sustainability Features in green Award-Winning university Buildings. Building Environ. 207 (1), 108417. doi:10.1016/j.buildenv.2021.108417
Zhang, J., Hu, J., Li, X., and Li, J. (2020). Bayesian Network Based Machine Learning for Design of Pile Foundations. Automation in Construction 118 (10), 103295. doi:10.1016/j.autcon.2020.103295
Zhang, X., and Mohandes, S. R. (2020). Occupational Health and Safety in green Building Construction Projects: A Holistic Z-Numbers-Based Risk Management Framework. J. Clean. Prod. 275 (12), 122788. doi:10.1016/j.jclepro.2020.122788
Zhao, X., Chen, L., Pan, W., and Lu, Q. (2017). AHP-ANP-Fuzzy Integral Integrated Network for Evaluating Performance of Innovative Business Models for Sustainable Building. J. Constr. Eng. Manage. 143 (8), 04017054. doi:10.1061/(asce)co.1943-7862.0001348
Zhao, X., Hwang, B.-G., and Gao, Y. (2016). A Fuzzy Synthetic Evaluation Approach for Risk Assessment: a Case of Singapore's green Projects. J. Clean. Prod. 115 (3), 203–213. doi:10.1016/j.jclepro.2015.11.042
Zhong, Y., and Wu, P. (2015). Economic Sustainability, Environmental Sustainability and Constructability Indicators Related to concrete- and Steel-Projects. J. Clean. Prod. 108 (8), 748–756. doi:10.1016/j.jclepro.2015.05.095
Zhuang, T., Qian, Q. K., Visscher, H. J., Elsinga, M. G., and Wu, W. (2019). The Role of Stakeholders and Their Participation Network in Decision-Making of Urban Renewal in China: The Case of Chongqing. Cities 92 (9), 47–58. doi:10.1016/j.cities.2019.03.014
Keywords: green building projects, stakeholder concerns, social sustainability, Bayesian networks, decision-making process
Citation: Wen S and Qiang G (2022) Managing Stakeholder Concerns in Green Building Projects With a View Towards Achieving Social Sustainability: A Bayesian-Network Model. Front. Environ. Sci. 10:874367. doi: 10.3389/fenvs.2022.874367
Received: 12 February 2022; Accepted: 07 March 2022;
Published: 21 March 2022.
Edited by:
Ge Wang, Huazhong Agricultural University, ChinaReviewed by:
Hongyang Li, Hohai University, ChinaCopyright © 2022 Wen and Qiang. This is an open-access article distributed under the terms of the Creative Commons Attribution License (CC BY). The use, distribution or reproduction in other forums is permitted, provided the original author(s) and the copyright owner(s) are credited and that the original publication in this journal is cited, in accordance with accepted academic practice. No use, distribution or reproduction is permitted which does not comply with these terms.
*Correspondence: Guofeng Qiang, Z2YxOTk0MDNAMTI2LmNvbQ==
Disclaimer: All claims expressed in this article are solely those of the authors and do not necessarily represent those of their affiliated organizations, or those of the publisher, the editors and the reviewers. Any product that may be evaluated in this article or claim that may be made by its manufacturer is not guaranteed or endorsed by the publisher.
Research integrity at Frontiers
Learn more about the work of our research integrity team to safeguard the quality of each article we publish.