- 1Economics and Management School, Wuhan University, Wuhan, China
- 2School of Finance, Shandong University of Finance and Economics, Jinan, China
In order to identify the impact mechanism between income inequality and carbon emissions and clarify the nonlinear relationship between income inequality and carbon emissions in different degrees, so as to provide theoretical support for government departments to formulate policies of reducing carbon emission and optimizing resource allocation efficiency, we investigated the relationship between carbon emissions and domestic income inequality in the United States and France from 1915 to 2019 using wavelet decomposition and Quantile-on-Quantile regression. The results imply that 1) For France, the impact of income inequality on carbon emissions is negative when the income inequality is low. With the increase of income inequality, the impact of income inequality on carbon emission changes from negative to positive, and the increase of carbon emissions will amplify the effect. For United States, with the deepening of income inequality, its emission enhancing effect has been gradually reversed. In addition, the impacts of carbon emissions on income inequality in the two countries are quite similar. 2) In the short term, the relationship between income inequality and carbon emissions in the two countries has obvious random volatility characteristics. 3) In the medium term, there is a three-dimensional inverted “V” shaped relationship between income inequality and carbon emissions across quantiles in the United States. As mentioned for France, on the contrary, a three-dimensional “V” shaped relationship across quantiles exists. 4) In the long run, the relationship between income inequality and carbon emissions in the United States is “V” shaped across quantiles. Carbon emissions in France are mainly inhibited by domestic income inequality. According to the empirical results, we recommend that the governments should make greater efforts to ensure the synergy between income distribution and environmental governance to ensure a sustainable and prudent development of economy.
Introduction
Income inequality and environmental degradation have become a top priority in achieving the Sustainable Development Goals. Over the past three decades, the global economy has boomed. The GDP of the world increased as a whole as a result of increased production technology, investment and labor productivity. The scale of national income keeps growing, in the meantime, the income gap caused by the uneven distribution of national income also presents a growing trend. Income inequality represents the difference existing in economic, income or welfare among individuals within a group, groups in a population, or countries. Income inequality is more pronounced in some developed countries, for example, America. Certainly, it is also a large problem in developing countries. Income inequality has a detrimental impact on social stability, resulting in a rise in crime rates and huge social pressure, even leading to the breakdown of social order (Feldstein and Wrobel, 1998). At the same time, income inequality weakens aggregate demand, which negatively affects employment levels and economic growth. (Fitoussi and Saraceno, 2010). In the stage of industrialization, it is inevitable to consume a large amount of fossil fuels. High energy consumption that emits greenhouse gases such as CO2 can cause irrecoverable damage to the environment. The massive emission of greenhouse gases leads to the rising environmental degradation, which threatens the living environment of the future generations. As Zhu (2002) said, fossil fuel consumption, forest loss and many other factors has increased the emission of CO2 and other pollutants, leading to environmental degradation, climate change, global warming, and water and air pollution. The most obvious example is Indonesia. Indonesia is the largest contributor in CO2 emissions in Southeast Asia with its emissions increasing every year. Following the current trend, this country with numerous islands may lose nearly 2000 islands because of the global warming and climate change caused by the concentrated increase in CO2 emissions (Kusumawardani and Dewi, 2020).
Overall, there is a close relationship between income inequality and carbon emissions: income inequality has impact on carbon emissions in two aspects. On the one hand, the decline of income inequality will increase the middle and high income groups, reduce the poor, and expand the scale of the middle class. Therefore, consumer demand for energy and other carbon-intensive products has increased, and carbon emissions will increase. On the other hand, the widening income gap will make the distribution of political rights and wealth more conducive to the rich. With economic growth, the demand structure of high-income groups for materials will gradually change, for example, the demand of resource products will decline, thus, carbon emissions will also continue to decline. In addition, it is worth noting that carbon emissions also play a two-way role in income inequality. First, with the rapid increase of carbon emissions in a country, the level of domestic production and economic development also continue to improve, and wealth is continuously accumulated. A large share of the wealth cake will be obtained by the producers and investors with more resource endowments. Accordingly, the domestic Matthew effect may be intensified and the degree of income imbalance will also increase. Second, the increase of carbon emissions will cause national concerns about climate, environment and economic sustainability. Therefore, with the increase of government’s administrative intervention on carbon emissions and environmental regulation, the economic development modes of countries will change from resource-driven to technological innovation-driven, and the status of the domestic industry will be washed up, and wealth will be redistributed, so the income inequality of a country will decrease. Income inequality and carbon pollution not only exacerbate economic vulnerability, but also trigger social problems. Therefore, it is very important to explore the causal relationship between income inequality and carbon emissions, and strive to find the equilibrium point between income inequality and carbon emissions, so as to realize Pareto optimality for economic stability and sustainable development of countries. Based on previous research, we can know that the results obtained by different research objects, research methods or research scopes are not similar. Coupled with the major role of globalization, the adverse social effects of income inequality and CO2 emissions will be more complex. Therefore, it can be said that the cognition of the relationship between the two has not reached a consensus in theory and experience. Moreover, the time series estimates used in previous studies cannot show the real dependence between income inequality and emissions at the lower and higher quantiles of the time series. The government’s decision can be misled by the biased result due to the above reasons. Hence, this study is an attempt to examine what we can get about the relationship between inequality and emissions if we focus on dependence between different quantiles of time series data.
It is worth noting that the level of economic development is the key factor in this study. Economic growth has an impact on carbon emissions and income inequality, and it also plays an important role in regulating the relationship between carbon emissions and income inequality (Ravallion et al., 2000), for example, Kuznet (1955) put forward the inverted “U” hypothesis of economic growth and income inequality, and Grossman and Krueger (1992) proposed the Environmental Kuznets Curve (EKC), indicating the nonlinear relationship between environmental pollution and economic growth. Thus, considering the heterogeneity brought by economic development, this paper integrates the level of economic development into the research framework as an important basis for selecting sample countries. Furthermore, the existing research conclusions on the relationship between income inequality and carbon emissions have strong heterogeneity, which may be affected by the different sample period span, and long enough sample span is an important basis for the rationality and stability of the research conclusion. Therefore, based on the above considerations, the United States and France are selected as the research samples, which provide Gini coefficient and carbon emission data with a sample period longer than a century and are at different stages of environmental Kuznets curve, with highly representative. The comparison of the research results of the two countries can provide important theoretical basis for the introduction and formulation of relevant policies. Meanwhile, we use the Quantile-on-Quantile regression (QQR) approach proposed by Sim and Zhou (2015). The approach combines quantile regression (QR) and non-parametric estimation techniques, which is an improvement of the traditional QR model and is robust to outliers and could account for slope heterogeneity, allowing researchers to investigate how an indicator’s (variable) quantiles influence the quantiles of another indicator. In addition, the QQR method considers the nonlinear relevance between income inequality and CO2 emissions. It can extract complex phenomena and relationship among research objects, which is difficult to achieve through traditional measurement methods. This should be the first article using the QQR method to study the nexus between income inequality and CO2 emissions.
The research shows that: First, For France, the impact of income inequality on carbon emissions is negative when the income inequality is low. With the increase of income inequality, the impact of income inequality on carbon emission changes from negative to positive, and the increase of carbon emissions will amplify the effect. For United States, with the deepening of income inequality, its emission enhancing effect has been gradually reversed. In addition, the impacts of carbon emissions on income inequality in the two countries are quite similar. Second, based on short-term perspective, the relationship between income inequality and carbon emissions in the two countries has obvious random volatility characteristics. Third, based on the medium-term perspective, there is a three-dimensional inverted “V” shaped relationship between income inequality and carbon emissions across quantiles in the United States. As mentioned for France, on the contrary, a three-dimensional “V” shaped relationship across quantiles exists. Fourth, based on the long-term perspective, the relationship between income inequality and carbon emissions in the United States is “V” shaped across quantiles. Carbon emissions in France are mainly inhibited by domestic income inequality. The results will help countries to formulate sustainable economic development policies.
The remaining section of this paper is outlined as follows: Literature review is presented in Literature Review Section. Methodology and Data Section presents methodology and data, while Conclusion Section presents empirical results. Conclusion is presented in Limitation Section.
Literature Review
In recent years, with the rapid development of the global economy, the environmental quality of various countries has been declining, and the income imbalance has intensified. Therefore, the problems of environmental deterioration and income inequality have become the focus of attention of all countries in the world. A large number of studies have been carried out on the two parameters of income inequality and carbon emissions, among which the United States, as the global economic and financial center, is the key research country for scholars. For example, Baek and Gweisah (2013) re-examined the growth-inequality-environment nexus in the US by using ARDL model, finding that a more equitable income distribution in the US can help improving environmental quality, which is same with the results of Torras and Boyce, (1998). Jorgenson et al. (2017) investigated the relationship between U.S. state-level CO2 emissions and two measures of income inequality during the period of 1997–2012 and concluded that there is no substantial relationship between the Gini coefficient and CO2 emissions, and this conclusion was later proved by Mader (2018). Barnhart et al. (2017) studied related content in the US and came to a conclusion that the impact of income inequality on per capita emissions in the US differs due to different income levels. In detail, the increase on income inequality will reduce emissions in low-and middle-income areas, while for high- and middle-income areas, the conclusion is opposite. In addition, research findings on income inequality and carbon emissions vary from time dimension, for example, Liu C. et al. (2019) argued that income inequality on the CO2 emissions of the US is significantly positive in the short term while negative in the long run using the panel ARDL model analysis.
With the rapid development of China’s economy, China has gradually become the second largest economy in the world, and the international status of China has been rising. Therefore, in addition to the United States, the income distribution and environmental quality in China are also another concern of scholars. For example, Liu et al. (2018) found that the effect of income inequality on carbon emissions is significantly negative by analyzing the data of Chinese cities during the period of 1996–2015. In a meanwhile, Jun et al. (2011) concluded the same conclusion by taking industrial wastewater and gas emissions as the dependent variable, whose data period spanned from 1996 to 2008. While on the contrary, Golley and Meng (2012) found the rich have higher emissions than the poor, thus proving that there is a positive nexus between income inequality and emissions. Conducting a panel regression analysis on income distribution and carbon emission data in China during the period of 1995–2010, Zhang and Zhao (2014) argued that the reduction in income inequality limited CO2 emissions. Based on the EKC hypothesis, Hao et al. (2016) analyzed the relationship between Gini and CO2 emissions in 23 regions of China in the period 1995-2012, and suggested that the deterioration of income distribution has increased the areas’ CO2 emissions.
With the deepening of academic research on the relationship between income inequality and carbon emissions, scholars began to expand the number of sample countries and strive to make conclusions universal, in order to provide theoretical reference for solving the problems of global carbon emissions and income inequality. In the literature, it is well documented that the nexus between income inequality and emissions is significantly negative. For example, the research of Ravallion et al. (2000) used the data of 138countries from 1960 to 2008 to study the relationship between distribution of income and carbon contamination, and mainly found that reducing poverty through income redistribution will change the consumption of the rich from low-polluting products to high-polluting. In addition, production and electricity-related factors are also important mechanisms for income inequality affecting carbon emissions (Coondoo and Dinda, 2008). Subsequently, Gassebner et al. (2011) tested the economic, political and demographic determinants of different environmental indicators by using the data of 120 countries from 1960 to 2001 and demonstrated that increasing income inequality reduces pollution. Moreover, the relationship among environmental performance, income, and income inequality from 1995 to 2014 was investigated by Morse (2018), he found that an increase in income level and a decrease in income inequality would increase environmental performance, taking 180 countries as the research sample. However, a large number of studies still use multi-country data to prove that there is a positive relationship between income inequality and carbon emissions, for example, Knight et al. (2017) investigated and found that increased income inequality led to inequality of political and economic rights, thus exacerbating environmental degradation, utilizing the data of 26 high-income countries during the period of 2000–2010. Ridzuan (2019) concluded that income inequality has delayed the EKC turning point by using a large research sample (N = 170–174), indicating that increased income inequality may cause environmental pollution. At the same time, Padhan et al. (2019) used panel data for the period 1971–2013 to explore the implications of growth, wealth disparities and per capita energy consumption on carbon emissions in a sample of Next Eleven (N-11) countries, and found that income inequality will exacerbate carbon emissions. Additionally, Baloch et al. (2020) reached the same conclusion for 40 sub-Saharan African countries.
Furthermore, some studies have shown that there is no substantive relationship between income inequality and carbon emission. For example, Magnani (2000) took OECD countries as research samples to study the relationship between income inequality and carbon emissions, and found that there is no significant relationship between the Gini coefficient and research and development expenditure on environmental protection. Wolde-Rufael and Idowu (2017) crunched the Gini Coefficient data set for China and India and found that income inequality had little impact on carbon emissions. Barra and Zotti (2018) conducted a study among 120 countries which indicated that the EKC hypothesis is invalid, meaning that there is a negligible impact on environmental quality for income.
Notably, some scholars have found significant heterogeneity in the relationship between income inequality and carbon emissions, and the level of economic development is an important factor affecting the relationship (Coondoo and Dinda, 2008; Mahalik et al., 2018). For example, Hübler (2017) found that the impact of income inequality on carbon emissions varied in countries with different income levels. The impact may be negative in low- and middle-income countries but positive in high- and middle-income countries. Rojas-Vallejos and Lastuka (2020) used a panel data consisting of 68countries over a 50-years period from 1961 to 2010 to confirm that income inequality has a negative impact on CO2 emissions in low- and middle-income countries, but it is slightly positive if specific income levels can be improved. Chen et al. (2020) found that more equal income distribution is conducive to reducing per capita CO2 emissions for developing countries, while income inequality in most developed countries hardly affects CO2 emissions. While, Some studies also show that the above nexus have different correlations at different time periods, for instance, Uddin et al. (2020) stated that the impact of income inequality on CO2 emissions in G7 countries showed a positive correlation during the period 1870-1880 and negative during 1950-2000, while there is no significant nexus during 2000-2014.
The requirements for the time span of sample data of the existing research are relatively loose. The sample period span selected by most studies is not more than 50 years, and the sample period span of few literatures is more than a century. The current research conclusions fail to reach an agreement may be related to the difference in the period span of the research sample, and lengthening the sample period span can ensure a stable relationship between income inequality and carbon emissions to a certain extent. Moreover, the causal relationship between different levels of income imbalance and carbon emissions is explored by few literatures. Thus, in order to satisfy the comparability of the research conclusions of the sample countries and the long-term nature of the sample period span, this paper uses the data of the United States and France from 1915 to 2019, and utilizes the wavelet decomposition and QQR model to conduct in-depth research on the causal relationship between income inequality and carbon emissions, for providing a theoretical basis for government departments to formulate policies to regulate income distribution and effectively control carbon emission reduction.
Methodology and Data
Methodology
Wavelet Decomposition
In this paper, the maximum overlap discrete wavelet transform (MODWT) proposed by Percival and Mofjeld (1997) is introduced to decompose the original time series into three components, indicating the fluctuation and trend in the short, medium and long term respectively. The MODWT approach is an extension of DWT method and overcomes some technical shortcomings of DWT. In addition, the wavelet filter we choose is LA8, that is, the wavelet filter with length of 8 corresponding to the Daubechies least asymmetric scale filter. Its biggest feature is that it has the approximate linear phase, and it is easy to obtain zero-phase wavelet transform.
The wavelet transform decomposes the time series into different frequency bands by performing successive low-pass filtering and high-pass filtering on the signal. More specifically, the original sequence is decomposed into a set of wavelet coefficients
As we incorporate the MODWT, we utilize the rescaled scaling
where
The Quantile-on-Quantile Approach
In the framework of the study, the QQ approach is proposed to analyze the effect of the quantiles of income inequality on the quantiles of carbon emissions of sample countries. This approach takes the following nonparametric quantile regression model as the starting point:
Where
We inspect Eq. 3 in the neighborhood of
Where
After substituting Eq. 5 into Eq. 3 and include the vector of control variables
The underlined part of Eq. 6 is the
At last, we could replace
to obtain the estimates
Data
In this paper,
This paper selects the United States and France as the research samples, mainly because the income distribution data of the two countries can be traced back to a century. In addition, the level of economic development is closely related to income distribution and environmental pollution, and there are obvious differences in the level of economic development between the two countries, meanwhile, the dynamic relationship between the level of economic development and the Gini coefficient also shows different characteristics. Thus, it is feasible and reasonable to take the United States and France as research samples.
Considering the availability of the sample data and the data after the first-order logarithmic difference processing, the sample span selected in this paper covers the period of 1915–2019, with a total of 630 observations.
Descriptive statistics of the major variables are shown in Table 1.
Empirical Results
In this section, we use the wavelet decomposition method following Kassouri et al. (2022) and Bilgili et al. (2021) to extract the short, medium- and long-term factors from the original time series of income inequality and carbon emissions. It enables us to investigate the emission-inequality nexus at different periodic scales. The wavelet decomposed and the original time series are plotted in Figures 1, 2, which reflect the varied periodic fluctuation characteristics of these series.
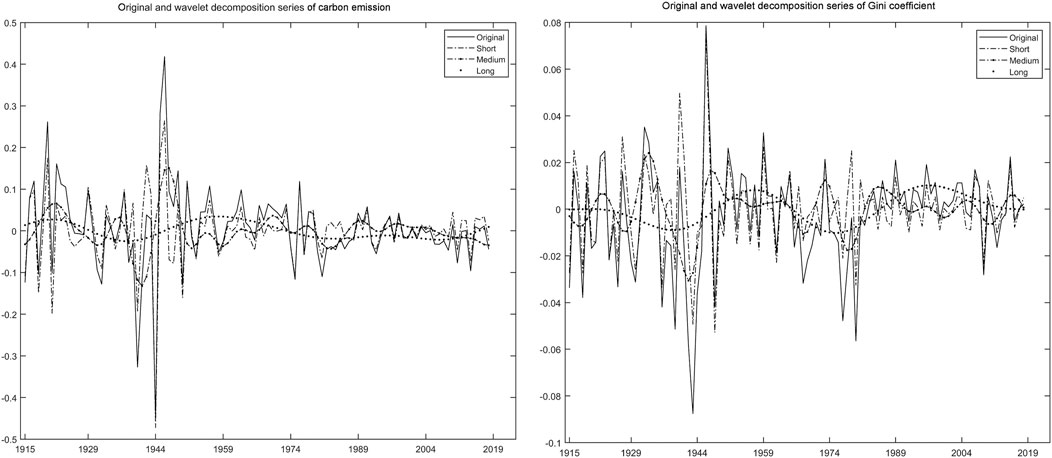
FIGURE 1. Original and wavelet decomposition series of carbon emission and Gini coefficient for France.
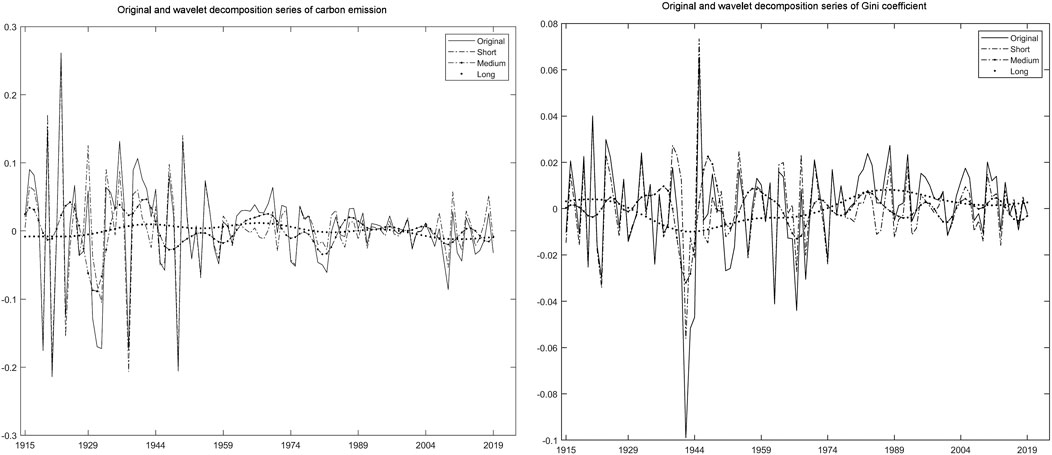
FIGURE 2. Original and wavelet decomposition series of carbon emission and Gini coefficient for the U.S.
Subsequently, the quantile-on-quantile regressions are adopted to empirically investigate the interaction between income inequality and carbon emission of sample countries. This method has been widely used in various fields of finance and economics, such as energy consumption (Shahbaz et al., 2018), tourism economics and stock volatility (Gupta et al., 2018; Mishra et al., 2019). The main advantage of this technique is to capture the association at different quantiles of the income inequality and emissions. In this way, we can know how the upper, lower, and middle quantiles of income inequality affect the upper, lower, and middle quantiles of the emission differently. In addition, we can divide the segments that require variables among different numbers of quantiles according to our own requirements.
This section presents the primary empirical results of the QQR analysis both using the original series and the series after wavelet decomposition. All regressions take GDP as a control variable.
Considering that the basic data used in this study are time series, it is necessary to test the stability of each sequence. In this paper, we decompose original time series into short, medium and long term series, while the conventional ADF test is not reliable when the series display different co-movements over time. Thus, in order to deal with non-linearity and time-varying structure of the data generating process, referring for data stability test method of Xiang et al. (2021), we use the Fourier unit root test developed by Enders and Lee (2012) to test the stability of the time series data, controlling the possible sharp and smooth structural breaks in the time series data. The Fourier unit root test results of the carbon emission, income inequality and GDP are shown in the Table 2. The F statistic shows that the data sequences have significant structural changes, and the tau statistic shows that some of the original data cannot reject the unit root hypothesis after controlling the structural changes, while the tau statistics of the data sequences after wavelet decomposition reject the unit root hypothesis, which means that if only the original data sequence is empirically analyzed, the rationality of the research results will not be guaranteed. However, the estimation of the causal relationship between income inequality and carbon emissions by using the short-term sequence, medium-term sequence and long-term sequence obtained by wavelet decomposition of the original sequence can not only broaden the research perspective, but also ensure the accuracy of the research conclusion, which has important theoretical significance.
The estimated results in this paper are shown in Figures 3–10. Figures 3–6 display the impact of income inequality on carbon emissions in the U.S. and France. Figures 7–10 display the effect of carbon emission on income inequality in France and the U.S. Throughout the graphs, the z-axis indicates the effect of the
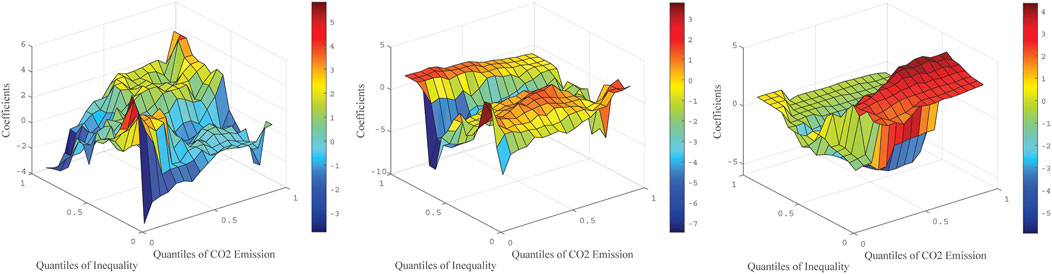
FIGURE 4. The impact of income inequality on carbon emissions in the short, medium and long term (France).
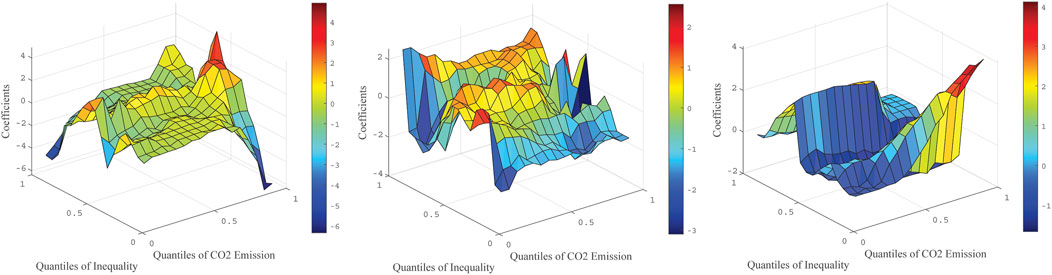
FIGURE 6. The impact of income inequality on carbon emissions in the short, medium and long term (U.S.).
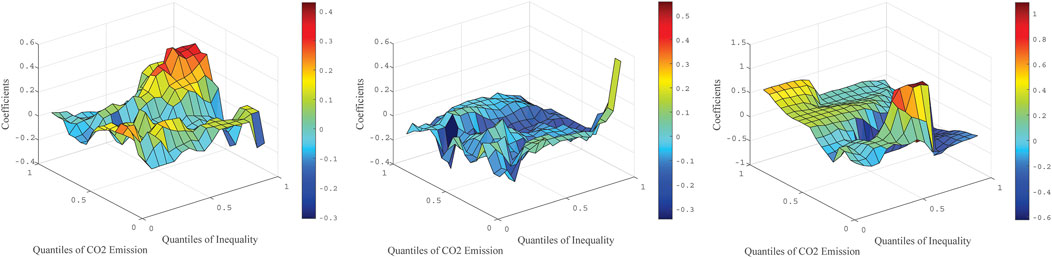
FIGURE 8. The impact of carbon emission on income inequality in the short, medium and long term (France).
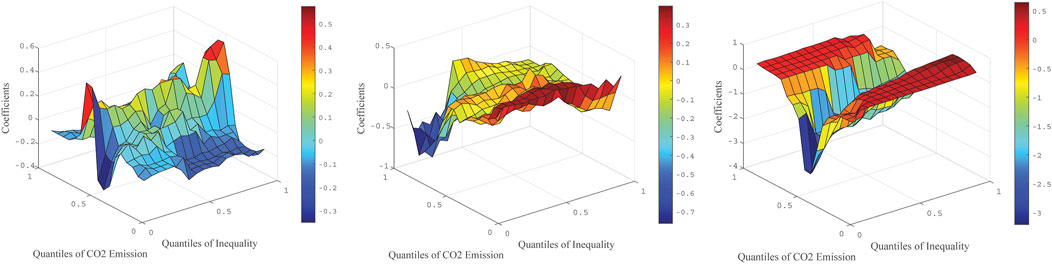
FIGURE 10. The impact of carbon emission on income inequality in the short, medium and long term (U.S.).
The Impact of Inequality on Emission
Some noteworthy results of the original series emerge in Figure 3. It is shown that the impacts of the income inequality on carbon emission in France vary across quantiles.
Carbon emissions will be exacerbated by income inequality when the Gini coefficient and carbon emissions are at a low quantile, while the impact of income inequality on emissions is negative when the Gini coefficient is at a low quantile and carbon emissions are at a high quantile. This expresses that when the income gap is narrow, the income inequality will reduce carbon emissions especially when the emissions are at a high level. Yet in the second phase, a high-quantile Gini coefficient will promote the increase of carbon emissions. This positive effect is strongest when the Gini coefficient is in the middle and high quantile and the carbon emission is in low quantile, while this promotion will gradually stabilize as the carbon emission quantile increase. This indicates that when income distribution inequality is high, income disparity will promote the increase of carbon emission. And the effect increases as emissions lower.
The positive effect result may be explained as follows. Boyce (1994) had said that when the income gap between the rich and the poor widens and the quality of economic development is not good, the poor are more likely to over-explore natural resources and do rude production to increase income, thus damaging the environment, whereas the rich may not necessarily increase investment to improve the environment. This positive impact is particularly pronounced at low levels of carbon emissions, and the maximum (4.79) located at the 0.5 quantile of Gini coefficient and the 0.11 quantile of carbon emission (Figure 3). From the perspective of the distribution of social rights, Boyce, (1995) believes that income inequality leads to unequal distribution of rights, which attributes to the concentration of political rights. Some of the rich have the privilege to evade carbon emission management. Grunewald et al. (2012) found that the higher household wealth, the more disproportionate the increase is in the demand for emissions-intensive products and services, which also proves that income inequality will increase the carbon emissions.
As the income gap is narrow, the society as a whole has higher requirement for environmental quality. In other words, a relatively equal income distribution provides citizens with more political rights and enables them to express their requirements for higher environmental quality (Heerink et al., 2001). As illustrated by Figure 3, income inequality has the greatest negative impact (−6.9) on the environment pollution at the 0.27 quantile of Gini index and the 0.94 quantile of carbon emission. Due to poor environmental conditions when carbon emissions are extremely large, people are desperate to improve the environment, thus the corresponding reduction in emissions is more obvious. Ravallion et al. (2000) explored the alternative relationship between income equality and pollutant emissions, and concluded that the increase in income will shift the consumption of high-pollutant products to the low-pollutant ones. Recently, France has enhanced its environmental protection efforts, invested more founds to encourage people to buy clean energy vehicles and promoted the development and innovation of renewable energy.
However, the regression based on the original series has revealed valuable information. It can be seen from Figure 3 that the influence of inequality still shows great random fluctuation at different quantiles. Especially with the increase of Gini coefficient, the influence of income inequality does not seem to show obvious regularity. There may still be some short-term random shocks affecting the estimation results albeit GDP have been controlled to lag for one period. Thus, we would like to know whether the impact of income inequality on carbon emissions has a long-term and far-reaching impact, or only a transitory impact. For this purpose, we use the wavelet transform to decompose the time series of variables into three different frequencies, namely short-, medium- and long-run.
In the short-run (Figure 4, column 1), we found that, ignoring the extreme performance of marginal data, the impact of income inequality on carbon emissions is still random. When the Gini coefficient falls in the range of 0.3–0.5 quantile, regardless of where carbon emissions are in the quantile, income inequality has a strong positive impact on carbon emissions. It can be understood that income inequality affects consumption and production in the short term. Following Golley and Meng (2012), they deemed that wealthy households have paid more on energy consumption (Ridzuan, 2019).
Looking at the medium-run (Figure 4, column 2), when the Gini coefficient is in the high quantile (0.5–0.8), the impact of income inequality on carbon emissions is significantly negative, and in the Gini coefficient quantile interval, the lower the quantile of carbon emissions, the more obvious the negative effect of income inequality on carbon emissions. When the Gini coefficient is in the low quantile, the impact of income inequality on carbon emissions is relatively stable, most of which are at 0.4–1.3, only when the carbon emissions quantile is in the middle of 0.4–0.6, which is relatively more vulnerable.
In the long-run (Figure 4, column 3), the overall result is roughly similar to that in the medium term, but the impact of income inequality on carbon emissions is more significant. As illustrated by Figure 4, when the Gini coefficient is at 0.5 quantile and above, regardless of whether carbon emissions are in high or low quantiles, income inequality will promote the reduction of carbon emissions, but when the level of income inequality is low, carbon emissions will increase due to its influence. This further confirms the medium-run conclusion, and when supporting the environment-friendly enterprise, the government’s policies are also functioning normally. At the same time, investment in clean technology is increased and the degree of environmental pollution is reduced through technological reforms. When the Gini coefficient is less than 0.5, the level of income inequality in society is not high, but as income inequality increases, it will weaken aggregate demand, thus putting a negative impact on employment and economic growth (Ridzuan, 2019). Moreover, as far as France is concerned, the GDP growth rate in the early period of the sample is low, while the Gini coefficient was relatively high. The opposite is true in the mid-to late period of the sample that the Gini coefficient decreased with the growth of GDP. This is in line with the Kuznets curve. That is, in the early stage of development, higher inequality will reduce emissions. With the economic developing, the level of income inequality will decrease, and income inequality will promote the reduction of carbon emissions.
The results for the original series (Figure 5) show that carbon emissions increase with the increasing inequality when the Gini coefficient is at the low-to-middle quantile, that is, when the degree of income inequality is low, unequal income distribution will promote the increase of carbon emissions. And this positive impact will become more obvious as the quantile of carbon emissions increases. While at the high-quantile Gini coefficient, income inequality will reduce carbon emissions, which means that in the high-quantile Gini coefficient level, income inequality mitigates environmental pollution and carbon emissions. It is worth noting that when the Gini coefficient is at a high quantile and carbon emissions are at a low quantile, that is, when income inequality is high, but there is no carbon pollution problem, the further increase in income inequality has a further inhibitory effect on carbon emissions. High income levels are closely related to better environmental protection and high-efficiency technologies ranging from improving the use efficiency of natural resources, weakening the impact of production on nature and continuously developing clean technologies to reduce pollution per unit of output and the total carbon emissions. In the post-industrial society like the United States, the income gap and economic growth are increasing in the same direction, which has a positive impact on the development of clean technology. And as the income level of residents continues to increase, the demand for high environmental quality growing. This has contributed to structural changes in the economy, thereby slowing down the deterioration of the environment.
The short-term results (Figure 6, column 1) show some random volatility after removing some edge extreme data, but the results are roughly similar to the original data results and the overall impact is relatively moderate. That is, when the Gini coefficient is in the high quantile, the increase in income inequality will reduce carbon emissions, and at the low quantile level of the Gini coefficient, income inequality will promote the increase of carbon emissions. It should be noted that when the Gini coefficient is around 0.5–0.6 and carbon emissions are at high quantiles, the positive impact will be stronger. This indicates that impact of production and consumption on carbon emissions is the most obvious in the short term. As mentioned above, economic growth brings about environmental pollution, whereas the technical reforms and policy measures adopted take a long period to affect carbon emissions. Therefore, in the short term, the positive impact of income inequality on carbon emissions is more obvious. This is similar to French short-run result.
Compared with France, the US mid-run result (Figure 6, column 2) has more volatility, as shown in Figure 6 (column 2). Ignoring the extreme data, when the Gini coefficient is in the low quantile (0.1–0.3), the increase of the income inequality reduces carbon emissions, especially when carbon emissions are in a high quantile. In the case of the middle and high quantiles of the Gini coefficient, income inequality leads to increases in emissions. In high-income countries, if economic growth is accompanied with a reduction of inequality, then the public’s preference for consumption of private goods will shift to public goods. If and only if income growth does not produce “large” income inequality, the downward phase of the environmental Kuznets curve can be realized (Magnani, 2000). In a circumstance of higher inequality, economic growth is accompanied by increased inequality, thus the demand for environmental goods is not high, and the conditions for environmental improvement are not in place, so income inequality here is positively related to emissions (Ravallion et al., 2000).
The long-run results in the United States (Figure 6, column 3) reflect that when the Gini coefficient is in the 0.3–0.7 quantile, as income inequality increases, carbon emissions decrease. As the consumption habits of residents and the survival of manufacturers are affected by income inequality, it may take some time before influencing the energy structure and pollution emissions (Liu Q. et al., 2019). Moreover, the development and application of technology also takes time, so the long-run results are different from the short-term and medium-term results. Although economic growth has brought about a rise in inequality, when the economy is highly developed, it will also promote technology upgrade and residents’ demands, so as to obtain more long-term and prosperous economic development. So, it shows that the impact of income inequality reduces carbon emissions. The United States and France have different levels of economic development in the later period, so the results are also different.
The Impact of Emission on Income Inequality
The results of the original series of France (Figure 7) show that the correlation coefficient between carbon emissions and the Gini is not large, which indicates that the impact of carbon emissions on income inequality is relatively small. When carbon emissions are in the middle and high quantile level, along with the increase of carbon emissions, income inequality will decrease, and the inhibitory effect is strongest when the Gini coefficient is in the low quantile level; when carbon emissions are at a low quantile level, carbon emissions promote income inequality, and whether the Gini coefficient is at a high quantile or a low quantile, the positive effect intensity is almost equal. Comparing the results of the United States (Figure 9), they are very similar. There is no significant fluctuation, and the overall situation is relatively stable.
Environmental pollution widens the income gap between skilled and unskilled workers, and the brain drain caused by the weakening of ecosystem vitality and the impact of air quality on human health will widen this income inequality (Li et al., 2020). In view of the harm caused by environmental pollution to human health, high-skilled and high-income workers may be transferred from heavily polluted areas to areas with better air quality (Zhang et al., 2018). Moreover, low-income groups are exposed to more pollutants than high-income groups, thus environmental pollution will increase the impact of income inequality on health inequality, fall into a poverty trap, and further widen the income gap (Yang and Liu. 2018). Furthermore, inequality has influenced immigration decisions. As the return on human capital increases, well-educated people are more likely to migrate (Zhu, 2002). In conclusion, carbon emissions can promote income inequality. In the case of high-quantile carbon emissions, the income inequality slightly weakens as emissions increase. This can be considered as high production requires high energy consumption, thus increasing jobs required, which can slightly weaken the income gap.
It can be seen that the short-term results of the two countries (Figure 8, column 1; Figure 10, column 1) have greater fluctuations. On the one hand, the randomness of the data may be obvious. On the other hand, it may be concluded that the impact of air pollution on people is not easy to change in the short term, meanwhile, the macro measures take time to take effect. During the sample period, France’s economic growth was accompanied by a decrease in the Gini coefficient. High economic output would usually result in an increase in carbon emissions due to high energy consumption, which can roughly explain the fact that carbon emissions in France’s medium-run results have reduced inequality. In the US, when carbon emissions are in the middle and low quantiles, it has a positive role in promoting income inequality. With a higher level of economic development, people have higher requirements for environmental quality, which will promote the migration of residents, especially to high-skilled and high-income workers.
The long-run results of France (Figure 8, column 3) show that carbon emissions will mainly reduce income inequality. The results of the United States (Figure 10, column 3) indicate that when carbon emissions are at the medium quantiles (0.3–0.6), the increase in carbon emissions has a significantly negative impact on inequality. When the carbon emission quantile is 0.5 and the Gini coefficient is 0.11, the coefficient reaches the maximum (−3.2). While in the case of low and high levels of income inequality, the exacerbating effect is strong.
Conclusion
This paper investigates relationship between income inequality and carbon emissions by utilizing wavelet decomposition and QQ approach. On the basis of existing literature, relevant research conclusions have been enriched and expanded. The main conclusions of this paper are as follows:
First, based on the original sequence, income inequality in France has an inhibitory effect on domestic carbon emissions when the degree of income inequality is low. While with the gradual increase of income inequality, the inhibitory effect gradually reverses and even aggravates carbon emissions, and with the gradual increase of carbon emissions in France, the impact of income inequality on carbon emissions will be amplified in the same direction. In the case of the United States, the impact of income inequality on carbon emissions is diminishing as income inequality increases. The impact of carbon emissions on income inequality in both countries is similar, with negative effects at 0.6 quantiles. Second, based on the short-term perspective, the relationship between income inequality and carbon emissions in both countries has strong random volatility characteristics, which is mainly due to the short-term technical reform has not been completed, policy measures have not yet entered into force, and the relationship between income inequality and carbon emissions has not yet formed a trend. Third, based on the medium-term perspective, the impact of income inequality on carbon emissions in the two countries is on the contrary, which is mainly related to the level and characteristics of their economic development. With the intensification of income inequality under the sustained economic development, the impact of income inequality on carbon emissions in the United States presents a three-dimensional inverted “V” shape, while that in France is “V” shape. The impact of carbon emissions on income inequality in the two countries is roughly the same, and the impact is gradually weakened with the increase of carbon emissions, but the difference is that the increase of carbon emissions in France will inhibit income inequality, while carbon emissions in the United States have an aggravating impact on income inequality. Fourth, based on the long-term perspective, considering technological reform and policy factors, the relationship between income inequality and carbon emissions tends to be stable. The impact of income inequality in France on domestic carbon emissions is still similar to that in the medium term, while income inequality in the United States has a significant inhibitory impact on carbon emissions. In addition, the impact of domestic carbon emissions on income inequality in the United States shows a “V” shaped structure across quantiles with the increase of carbon emissions, while the impact of carbon emissions on income inequality in France is mainly negative.
Some policy implications can be drawn from the above conclusions. First, the government should establish a dynamic coordination mechanism between income inequality and carbon emissions to ensure that the matching and synergy of domestic resource allocation efficiency, income distribution rationality and environmental governance can be considered while promoting domestic economic development, and effectively prevent the amplification of income distribution imbalance. Second, based on the short-term perspective, the government should strengthen the dynamic adjustment of income imbalances and improve the intervention of environmental governance to prevent the random changes in the relationship between income inequality and carbon emissions from having a negative impact on the economy. Third, the administrative interventions of governments on income distribution and emission reduction should fully consider the level and characteristics of national economic development, so as to ensure the maximization of policy effectiveness. Fourthly, the government should increase investment in research and development of emission reduction technologies and training of professional talents, enhance the control of carbon emissions, and weaken the aggravating effect of carbon emissions on income inequality. At the same time, effectively reducing carbon emissions can still slow down the environmental governance pressure caused by domestic income inequality, and ensure sustainable economic development.
Limitation
The selection of sample countries in this paper focuses on the period span of data and the heterogeneity caused by economic development, and ignores the problem of a small number of sample countries. Therefore, the representativeness of the conclusions of this paper is somewhat lacking. Future longitudinal studies are needed to expand the number of sample countries to enrich research conclusions and enhance the representativeness and universality of research conclusions.
Data Availability Statement
The original contributions presented in the study are included in the article/Supplementary material, further inquiries can be directed to the corresponding author.
Author Contributions
ZY: conceptualization, validation, resources, and writing original draft. JR: software, writing—review and editing, and visualization. SM: formal analysis, data curation, and investigation. XC: methodology, writing—review and editing, supervision, and resources. SC: software, visualization, investigation and funding acquisition. LX: supervision, methodology, and funding acquisition. All authors: contributed to the article and approved the submitted version.
Conflict of Interest
The authors declare that the research was conducted in the absence of any commercial or financial relationships that could be construed as a potential conflict of interest.
Publisher’s Note
All claims expressed in this article are solely those of the authors and do not necessarily represent those of their affiliated organizations, or those of the publisher, the editors and the reviewers. Any product that may be evaluated in this article, or claim that may be made by its manufacturer, is not guaranteed or endorsed by the publisher.
Acknowledgments
We acknowledge the financial support from Shandong Provincial Natural Science Foundation (Grant Number: ZR2020QG032), Shandong Provincial Social Science Planning Office (Grant Numbers: 21DGLJ12 and 21DJJJ02), Taishan Scholars Program of Shandong Province, China (Grant Numbers: ts201712059 and tsqn201909135) and Youth Innovative Talent Technology Program of Shandong Province, China (Grant Number: 2019RWE004). All errors remain our own.
Footnotes
1The dataset is available at: https://www.globalcarbonproject.org.
2The dataset is available at: http://wid.world.
3The dataset is available at: https://www.rug.nl/ggdc/historicaldevelopment/maddison/releases/maddison-project-database-2020?lang=en.
References
Baek, J., and Gweisah, G. (2013). Does Income Inequality Harm the Environment? Empirical Evidence from the United States. Energy Policy 62, 1434–1437. doi:10.1016/j.enpol.2013.07.097
Baloch, M. A., Danish, S. U. D., Khan, S. U.-D., Ulucak, Z. Ş., and Ahmad, A. (2020). Analyzing the Relationship between Poverty, Income Inequality, and CO2 Emission in Sub-saharan African Countries. Sci. Total Environ. 740, 139867. doi:10.1016/j.scitotenv.2020.139867
Barnhart, N. M. (2017). Gender Wage Inequality: Is More Legislation the Answer? Seattle University Law Review 40 (4), 1435. Available at: http://digitalcommons.law.seattleu.edu/sulr/vol40/iss4/12
Barra, C., and Zotti, R. (2018). Investigating the Non-linearity between National Income and Environmental Pollution: International Evidence of Kuznets Curve. Environ. Econ. Pol. Stud 20 (1), 179–210. doi:10.1007/s10018-017-0189-2
Bilgili, F., Kuşkaya, S., Gençoğlu, P., Kassouri, Y., and Garang, A. P. M. (2021). The Co-movements between Geothermal Energy Usage and CO2 Emissions through High and Low Frequency Cycles. Environ. Sci. Pollut. Res. 28 (45), 63723–63738. doi:10.1007/s11356-020-11000-x
Boyce, J. K. (1995). Equity and the Environment: Social justice Today as a Prerequisite for Sustainability in the Future[J]. Alternatives Perspect. Soc. Environ. 21, 12–17. https://www.researchgate.net/publication/293124664_Equity_and_the_environment_social_justice_today_as_a_prerequiste_for_sustainability_in_the_future.
Boyce, J. K. (1994). Inequality as a Cause of Environmental Degradation. Ecol. Econ. 11 (3), 169–178. doi:10.1016/0921-8009(94)90198-8
Chen, J., Xian, Q., Zhou, J., and Li, D. (2020). Impact of Income Inequality on CO2 Emissions in G20 Countries. J. Environ. Manage. 271, 110987. doi:10.1016/j.jenvman.2020.110987
Coondoo, D., and Dinda, S. (2008). Carbon Dioxide Emission and Income: A Temporal Analysis of Cross-Country Distributional Patterns. Ecol. Econ. 65 (2), 375–385. doi:10.1016/j.ecolecon.2007.07.001
Enders, W., and Lee, J. (2012). A Unit Root Test Using a Fourier Series to Approximate Smooth Breaks*. Oxford Bull. Econ. Stat. 74 (4), 574–599. doi:10.1111/j.1468-0084.2011.00662.x
Feldstein, M., and Wrobel, M. V. (1998). Can State Taxes Redistribute Income? J. Public Econ. 68 (3), 369–396. doi:10.1016/S0047-2727(98)00015-2
Fitoussi, J. P., and Saraceno, F. (2010). Europe: How Deep Is a Crisis? Policy Responses and Structural Factors behind Diverging Performances. J. Globalization Dev. 1 (1), 17. doi:10.2202/1948-1837.1053
Gassebner, M., Lamla, M. J., and Sturm, J.-E. (2011). Determinants of Pollution: what Do We Really Know. Oxford Econ. Pap. 63 (3), 568–595. doi:10.1093/oep/gpq029
Golley, J., and Meng, X. (2012). Income Inequality and Carbon Dioxide Emissions: The Case of Chinese Urban Households. Energ. Econ. 34 (6), 1864–1872. doi:10.1016/j.eneco.2012.07.025
Grossman, G. M., and Krueger, A. B. (1992). Environmental Impacts of a North American Free Trade Agreement[J]. CEPR Discussion Papers. 8, 223–250. doi:10.3386/w3914
Grunewald, N., Harteisen, M., Lay, J., Minx, J., and Renner, S. (2012). “The Carbon Footprint of Indian Households,” in 32nd General Conference of The International Association for Research in Income and Wealth, 5–11. http://www.iariw.org.
Gupta, R., Pierdzioch, C., Selmi, R., and Wohar, M. E. (2018). Does Partisan Conflict Predict a Reduction in US Stock Market (Realized) Volatility? Evidence from a Quantile-on-Quantile Regression Model. Working Papers 43. doi:10.1016/j.najef.2017.10.006
Hao, Y., Chen, H., and Zhang, Q. (2016). Will Income Inequality Affect Environmental Quality? Analysis Based on China's Provincial Panel Data. Ecol. Indicators 67 (aug), 533–542. doi:10.1016/j.ecolind.2016.03.025
Heerink, N., Mulatu, A., and Bulte, E. (2001). Income Inequality and the Environment: Aggregation Bias in Environmental Kuznets Curves. Ecol. Econ. 38 (3), 359–367. doi:10.1016/S0921-8009(01)00171-9
Hübler, M. (2017). The Inequality-Emissions Nexus in the Context of Trade and Development: A Quantile Regression Approach. Ecol. Econ. 134, 174–185. doi:10.1016/j.ecolecon.2016.12.015
Jorgenson, A., Schor, J., and Huang, X. (2017). Income Inequality and Carbon Emissions in the United States: A State-Level Analysis, 1997-2012. Ecol. Econ. 134, 40–48. doi:10.1016/j.ecolecon.2016.12.016
Jun, Y., Zhong-kui, Y., and Peng-fei, S. (2011). Income Distribution, Human Capital and Environmental Quality: Empirical Study in China. Energ. Proced. 5, 1689–1696. doi:10.1016/j.egypro.2011.03.288
Kassouri, Y., Bilgili, F., and Kuşkaya, S. (2022). A Wavelet-Based Model of World Oil Shocks Interaction with CO2 Emissions in the US. Environ. Sci. Pol. 127, 280–292. doi:10.1016/j.envsci.2021.10.020
Knight, K. W., Schor, J. B., and Jorgenson, A. K. (2017). Wealth Inequality and Carbon Emissions in High-Income Countries. Social Currents 4 (5), 403–412. doi:10.1177/2329496517704872
Kusumawardani, D., and Dewi, A. K. (2020). The Effect of Income Inequality on Carbon Dioxide Emissions: A Case Study of Indonesia. Heliyon 6 (8), e04772. doi:10.1016/j.heliyon.2020.e04772
Kuznets, S. (1955). Economic Development and Income Inequality[J]. Am. Econ. Rev. 45 (1), 1–28. https://ci.nii.ac.jp/naid/10029870432/.
Li, B., Cheng, S., and Xiao, D. (2020). The Impacts of Environmental Pollution and Brain drain on Income Inequality. China Econ. Rev. 62, 101481. doi:10.1016/j.chieco.2020.101481
Liu, C., Jiang, Y., and Xie, R. (2019). Does Income Inequality Facilitate Carbon Emission Reduction in the US? J. Clean. Prod. 217, 380–387. doi:10.1016/j.jclepro.2019.01.242
Liu, Q., Wang, S., Zhang, W., and Li, J. (2018). Income Distribution and Environmental Quality in China: A Spatial Econometric Perspective. J. Clean. Prod. 205, 14–26. doi:10.1016/j.jclepro.2018.09.090
Liu, Q., Wang, S., Zhang, W., Li, J., and Kong, Y. (2019). Examining the Effects of Income Inequality on CO2 Emissions: Evidence from Non-spatial and Spatial Perspectives. Appl. Energ. 236, 163–171. doi:10.1016/j.apenergy.2018.11.082
Mader, S. (2018). The Nexus between Social Inequality and CO2 Emissions Revisited: Challenging its Empirical Validity. Environ. Sci. Pol. 89, 322–329. doi:10.1016/j.envsci.2018.08.009
Magnani, E. (2000). The Environmental Kuznets Curve, Environmental protection Policy and Income Distribution. Ecol. Econ. 32 (3), 431–443. doi:10.1016/S0921-8009(99)00115-9
Mahalik, M. K., Mallick, H., Padhan, H., and Sahoo, B. (2018). Is Skewed Income Distribution Good for Environmental Quality? A Comparative Analysis Among Selected BRICS Countries. Environ. Sci. Pollut. Res. 25 (23), 23170–23194. doi:10.1007/s11356-018-2401-8
Mallat, S. G. (1989). A Theory for Multiresolution Signal Decomposition: the Wavelet Representation. IEEE Trans. Pattern Anal. Machine Intell. 11 (7), 674–693. doi:10.1109/34.192463
Mishra, S., Sharif, A., Khuntia, S., Meo, S. A., and Khan, S. A. R. (2019). Does Oil Prices Impede Islamic Stock Indices? Fresh Insights from Wavelet- Based Quantile-on-Quantile Approach. Resources Policy 62, 292–304. doi:10.1016/j.resourpol.2019.04.005
Morse, S. (2018). Relating Environmental Performance of Nation States to Income and Income Inequality. Sust. Dev. 26 (1), 99–115. doi:10.1002/sd.1693
Padhan, H., Haouas, I., Sahoo, B., and Heshmati, A. (2019). What Matters for Environmental Quality in the Next Eleven Countries: Economic Growth or Income Inequality? Environ. Sci. Pollut. Res. 26 (22), 23129–23148. doi:10.1007/s11356-019-05568-2
Percival, D. B., and Mofjeld, H. O. (1997). Analysis of Subtidal Coastal Sea Level Fluctuations Using Wavelets. J. Am. Stat. Assoc. 92 (439), 868–880. doi:10.1080/01621459.1997.10474042
Ravallion, M., Heil, M., and Jalan, J. (2000). Carbon Emissions and Income Inequality. Oxford Econ. Pap. 52 (4), 651–669. doi:10.1093/oep/52.4.651
Ridzuan, S. (2019). Inequality and the Environmental Kuznets Curve. J. Clean. Prod. 228, 1472–1481. doi:10.1016/j.jclepro.2019.04.284
Rojas-Vallejos, J., and Lastuka, A. (2020). The Income Inequality and Carbon Emissions Trade-Off Revisited. Energy Policy 139, 111302. doi:10.1016/j.enpol.2020.111302
Shahbaz, M., Lahiani, A., Abosedra, S., and Hammoudeh, S. (2018). The Role of Globalization in Energy Consumption: A Quantile Cointegrating Regression Approach. Energ. Econ. 71, 161–170. doi:10.1016/j.eneco.2018.02.009
Sim, N., and Zhou, H. (2015). Oil Prices, US Stock Return, and the Dependence between Their Quantiles. J. Banking Finance 55, 1–8. doi:10.1016/j.jbankfin.2015.01.013
Torras, M., and Boyce, J. K. (1998). Income, Inequality, and Pollution: a Reassessment of the Environmental Kuznets Curve. Ecol. Econ. 25 (2), 147–160. doi:10.1016/S0921-8009(97)00177-8
Uddin, M. M., Mishra, V., and Smyth, R. (2020). Income Inequality and CO2 Emissions in the G7, 1870-2014: Evidence from Non-parametric Modelling. Energ. Econ. 88, 104780. doi:10.1016/j.eneco.2020.104780
Wolde-Rufael, Y., and Idowu, S. (2017). Income Distribution and CO2 Emission: A Comparative Analysis for China and India. Renew. Sustain. Energ. Rev. 74, 1336–1345. doi:10.1016/j.rser.2016.11.149
Xiang, L., Zhang, H., Gao, K., and Xiao, Z. (2021). Oil Volatility-Inflation Pass through in China: Evidence from Wavelet Analysis. Energ. Rep. 7, 2165–2177. doi:10.1016/j.egyr.2021.04.021
Yang, T., and Liu, W. (2018). Does Air Pollution Affect Public Health and Health Inequality? Empirical Evidence from China. J. Clean. Prod. 203, 43–52. doi:10.1016/j.jclepro.2018.08.242
Zhang, C., and Zhao, W. (2014). Panel Estimation for Income Inequality and CO2 Emissions: A Regional Analysis in China. Appl. Energ. 136, 382–392. doi:10.1016/j.apenergy.2014.09.048
Zhang, Z., Hao, Y., and Lu, Z.-N. (2018). Does Environmental Pollution Affect Labor Supply? an Empirical Analysis Based on 112 Cities in China. J. Clean. Prod. 190, 378–387. doi:10.1016/j.jclepro.2018.04.093
Keywords: income inequality, CO2 emissions, Quantile-on-quantile regression, nonparametric estimation, wavelet decomposition
Citation: Yang Z, Ren J, Ma S, Chen X, Cui S and Xiang L (2022) The Emission-Inequality Nexus: Empirical Evidence From a Wavelet-Based Quantile-on-Quantile Regression Approach. Front. Environ. Sci. 10:871846. doi: 10.3389/fenvs.2022.871846
Received: 08 February 2022; Accepted: 11 March 2022;
Published: 31 March 2022.
Edited by:
Ehsan Elahi, Shandong University of Technology, ChinaReviewed by:
Yacouba Kassouri, Leipzig University, GermanySeyi Akadiri, Eastern Mediterranean University, Turkey
Copyright © 2022 Yang, Ren, Ma, Chen, Cui and Xiang. This is an open-access article distributed under the terms of the Creative Commons Attribution License (CC BY). The use, distribution or reproduction in other forums is permitted, provided the original author(s) and the copyright owner(s) are credited and that the original publication in this journal is cited, in accordance with accepted academic practice. No use, distribution or reproduction is permitted which does not comply with these terms.
*Correspondence: Shihao Cui, Y3Vpc2hpaGFvZWR1QHFxLmNvbQ==