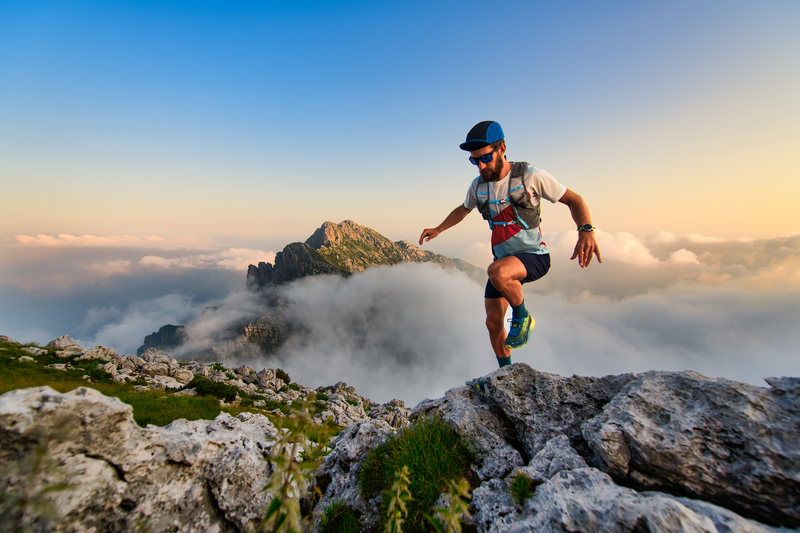
94% of researchers rate our articles as excellent or good
Learn more about the work of our research integrity team to safeguard the quality of each article we publish.
Find out more
ORIGINAL RESEARCH article
Front. Environ. Sci. , 29 March 2022
Sec. Atmosphere and Climate
Volume 10 - 2022 | https://doi.org/10.3389/fenvs.2022.867908
This article is part of the Research Topic Air Pollution and Climate Change: Interactions and Co-mitigation View all 18 articles
In addition to the well-constrained inorganic acid-base chemistry of ammonia resulting in fine particulate matter (PM2.5) formation, ammonia also reacts with certain organic compounds in secondary organic aerosol (SOA) to produce less basic nitrogen-containing organic compounds. In this study, the potential meteorology and air quality impacts of the heterogeneous uptake of NH3 by SOA are investigated using the WRF-CMAQ two-way coupled model, which calculates the two-way radiative forcing feedback caused by aerosol between meteorology and chemistry in a single simulation. Simulations with and without the NH3-SOA uptake are performed over the contiguous US for July 2014 and July 2050 under the RCP 8.5 IPCC scenario to study the potential impact of climate change. A comparison with multiple observation network data shows that the NH3-SOA uptake improves the model performance for PM2.5 prediction (bias reduced from −22% to −17%), especially the underestimation of organic carbon over the Southeastern US (bias reduced from −17% to −7%). Secondly, the addition of the NH3-SOA chemistry significantly impacts the concentration of NH3 and NH4+, thus affecting the modeled particle acidity. Including the NH3-SOA uptake also impacts the meteorological conditions through the WRF-CMAQ two-way feedback. Moreover, the impact on meteorological conditions results in different windspeed or dispersion conditions, thus affecting air quality predictions. Finally, simulations including the NH3-SOA uptake under the warmer climate conditions of 2050 show a smaller impact on air quality predictions than it does under current climate conditions. This study confirms the importance and necessity of including NH3-SOA chemistry in air quality predictions.
As an important atmospheric trace gas, ammonia (NH3) is found in various sources of natural and anthropogenic emissions (Bouwman et al., 1997). Globally, roughly half of the NH3 emissions originates from agricultural activities, for example, satellite observations show ammonia hot spots in major agricultural areas (Clarisse et al., 2009). As a highly water-soluble molecule, the majority of atmospheric ammonia is lost by deposition on wet particles and aquatic surfaces (Dentener et al., 2006) as part of the global nitrogen cycle. Ammonia has long been considered an important precursor to the inorganic component of fine particulate matter (PM2.5, particles with sizes below 2.5 μm that penetrate easily the respiratory tract). Ammonia is converted into inorganic ammonium salts with low volatility by neutralizing inorganic acids commonly found in polluted air, such as sulfuric acid and nitric acid. Such salts accumulate as solids in PM2.5 (Seinfeld and Pandis 2016). Ammonium nitrate and ammonium sulfate constitute an important fraction of the total PM2.5 in many geographical areas (Behera and Sharma 2010).
In addition to the well-constrained inorganic acid-base chemistry of ammonia leading to PM2.5 formation, there is experimental evidence that ammonia reacts with certain organic compounds in secondary organic aerosol (SOA) leading to the formation of nitrogen-containing organic compounds (NOC) (Bones et al., 2010). SOA produced by photooxidation of biogenic and anthropogenic volatile organic compounds (VOCs) represents a large fraction of PM2.5. Although considerable uncertainty still exists over chemical reactions between NH3 and SOA, the general mechanism involves the reaction of ammonia with carbonyls within the organic particles to produce relatively unstable imines and amines, which may be stabilized by intramolecular cyclization into heteroaromatic NOC based on imidazole, pyrrole, indole, etc., (Montoya-Aguilera et al., 2018). These reactions change the chemical composition of organic particles and their physical properties, such as color, viscosity, and condensed-phase diffusivity (Laskin, Laskin, and Nizkorodov 2015; Li et al., 2015; Bell et al., 2017; Liu et al., 2018).
Until recently, interactions between NH3 and SOA (SOA-NH3 uptake) were not considered in air quality models. In our previous work to incorporate such interactions into air quality models, a significant decrease was found in predicted NH3 and inorganic PM2.5 concentration in a regional study (Horne et al., 2018) on the South Coast Air Basin of California (SoCAB) using the UCI-CIT model (Nguyen and Dabdub 2002). Furthermore, we found a significant SOA concentration increase in the southeast of the US during the summertime in a national-scale study (Zhu et al., 2018) resulting from enhanced acid-catalyzed reactions (Pye et al., 2013) when the SOA-NH3 uptake was incorporated into the Community Multiscale Air Quality (CMAQ v5.2) model (Byun and Schere 2006). In general, the ammonia loss to the SOA uptake is smaller than the traditional inorganic acidic uptake pathway. However, there are situations in which the SOA-NH3 uptake becomes competitive and has a modest impact on organic aerosol and PM2.5 concentrations.
An air quality model can either be an offline model where the meteorology is specified from a foreign source or an online model where the meteorology is adjusted based on the chemical constituents within the model. Our previous SOA-NH3 uptake sensitivity studies (Horne et al., 2018; Zhu et al., 2018) were based on offline air quality models, which means no feedback was considered between pollutant concentration and meteorological conditions (Grell and Baklanov 2011). However, field experiments and satellite observation have shown that chemistry-atmosphere feedbacks play an essential role in the Earth system (Kaufman and Fraser 1997; Rosenfeld et al., 2007, 2008; Pausata et al., 2015). On the one hand, meteorological conditions dictate both the formation and transport of air pollutants. On the other hand, chemical species can also influence the meteorological parameters by changing the atmospheric radiation budget and through cloud formation. In this study, a more comprehensive air quality impact study of the effects of SOA-NH3 uptake is conducted by including the chemistry-atmosphere feedbacks using the two-way coupled Weather Research and Forecasting—Community Multi-scale Air Quality (WRF-CMAQ) model (Wong et al., 2012). Developed by the US Environmental Protection Agency (EPA), the CMAQ model is an offline model historically and is widely used for air quality simulations worldwide (X. H. Liu et al., 2010; Canty et al., 2015; Sharma et al., 2016; Matthias 2008; de Almeida Albuquerque et al., 2018). In recent years, it was further developed and coupled with the Weather Research and Forecasting (WRF) model to simulate the two-way radiative forcing feedbacks caused by the aerosol direct effect between chemistry and meteorology (Wong et al., 2012). This new WRF-CMAQ coupled model has been evaluated in several applications and used to investigate aerosol direct effects on meteorology (Wang et al., 2014; Gan et al., 2015; Hogrefe et al., 2015; Xing et al., 2016; Hong et al., 2017).
Many studies (Brasseur et al., 2006; Fiore et al., 2012; Lin, Penner, and Zhou 2016; Campbell et al., 2018; Zhu et al., 2019) have demonstrated that climate change could impact significantly the physical and chemical processes that govern the formation of atmospheric pollutants, including ammonia and SOA. Furthermore, both emissions of ammonia (Skjøth and Geels 2013) and VOC precursors (Constable et al., 1999) are expected to increase in the future due to the rising temperature as a result of climate change. Combining these two factors is likely to lead to significant changes in the mass concentration and chemical composition of SOA. As a preliminary study, the potential impact of the physical and chemical processes alone due to the implementation of the SOA-NH3 uptake mechanism is investigated under the changing climate using the WRF-CMAQ model. The Representative Concentration Pathway (RCP) 8.5 (business-as-usual) dataset for the year 2050 (Bruyère et al., 2013; Monaghan et al., 2014) is used here to provide a midterm outlook and the worst scenario estimation. Our results show that the inclusion of the NH3-SOA uptake under the warmer climate conditions of 2050 produces a smaller impact on air quality predictions than under current climate conditions. But overall, this study highlights the importance and necessity of including NH3-SOA chemistry in air quality predictions.
This study uses the two-way coupled WRF-CMAQ (WRF v3.8 and CMAQ v5.2) as the base platform to integrate the SOA-NH3 uptake process to conduct regional meteorology and air quality simulations. WRF is the meteorology driver of the model, while CMAQ handles the air quality dynamics. A detailed description of the two-way coupled WRF-CMAQ model is presented in Wong et al. (2012). The publicly available version used in this study supports the Rapid and accurate Radiative Transfer Model for General Circulation Models (RRTMG) radiation scheme (Clough et al., 2005) for shortwave aerosol direct effects. It uses a core-shell model to perform the aerosol optics calculation. The aerosol indirect effects that result from interactions between aerosols and cloud formation are not considered in this version.
For WRF configuration, the Asymmetric Convective model version 2 (ACM2) (Pleim 2007) is used as the planetary boundary layer scheme with the Pleim-Xiu land surface model (Xiu and Pleim 2001). The Morrison double-moment scheme (Morrison, Thompson, and Tatarskii 2009) is used for the microphysics option of WRF, and version 2 of Kain-Fritsch convective parametrization (Kain 2004) is used for cumulus physics. Both longwave and shortwave radiations are solved by the RRTMG scheme (Iacono et al., 2008). For the current scenarios, WRF input is derived from the National Centers for Environmental Prediction (NCEP) North American Mesoscale Forecast System (NAM) 12 km analysis data (NCEP 2015). For the future (year 2050) climate scenarios, the RCP 8.5 National Center for Atmospheric Research (NCAR) Community Earth System Model (CESM) global bias-corrected CMIP5 dataset (Monaghan et al., 2014) is used to provide a midterm outlook and the worst scenario estimation, where CAM-Chem (Lamarque et al., 2012) is used for chemistry-climate modeling and MOZART as the chemical mechanism (Emmons et al., 2010).
The CMAQ model is configured using the Carbon Bond 2006 (CB06) chemical mechanism for gas-phase chemistry (Yarwood et al., 2010), including 127 species as detailed on the model’s website (Adams 2017). The aerosol dynamics are solved by the sixth-generation CMAQ aerosol module (AERO6) (Appel et al., 2013), which includes 21 inorganic species and 34 organic species (28 SOA and 6 primary organic species) as detailed on the CMASWIKI website (Pye 2016). The modeling domain is the same as Zhu et al. (2018), which covers the contiguous US (CONUS) with a 12 km × 12 km horizontal-grid resolution and a 29-layers logarithmic vertical structure. The initial and boundary conditions are downscaled from the Model for Ozone and Related Chemical Tracers (MOZART v4.0) (Emmons et al., 2010). Emissions are generated based on the 2014 National Emissions Inventory (NEI) version 2 (US EPA 2018) and spatially/temporally resolved using the Sparse Matrix Operator Kernel Emission (SMOKE, version 4.5) processor (US EPA 2017). Biogenic emissions are calculated using the Biogenic Emission Inventory System (BEIS) (Schwede et al., 2005). As a preliminary study and only accounting for the change in climate alone, the same emissions are used for both 2014 and 2050 cases.
The SOA-NH3 uptake mechanism is incorporated into the AERO6 module using the same method as our previous study (Zhu et al., 2018). In AERO6, all particles are considered spherical and internally mixed, which means a homogeneous distribution of all chemical substances within the particle. However, as the uptake coefficient used in this study is measured from pure SOA particles, how the uptake coefficient could be changed with SOA mass ratios within the particle is unknown. In the absence of better information, we assume that the uptake coefficient is proportional to the SOA mass fraction within the particle. In general, the uptake of NH3 by SOA is calculated based on the representative wet surface area concentration of SOA (SSOA) and the reactive uptake coefficient
where
In total, four simulations with two-way feedback are performed in this study, two for 2014 and two for 2050. For each year, there is one case considering the SOA-NH3 uptake and one case without the uptake. Table 1 summarizes the naming and definition of these six simulation cases. July is selected as the simulation period, with the first week discarded as a spin-up. Our previous study (Zhu et al., 2018) showed that SOA-NH3 uptake produced an additional impact on acid-catalyzed SOA formation during the summer (Pye et al., 2013), suggesting that July is a good choice for this simulation.
Model validation and performance analysis are quantified using the Atmospheric Model Evaluation Tool (AMET) (Appel et al., 2011) developed by the US EPA. AMET organizes, provides consistency, and speeds up the evaluation process for operational meteorological and air quality model simulations. It can match the model output for particular locations to the corresponding observed values from one or more monitor networks. For meteorological model evaluation, observations are obtained from NCEP Meteorological Assimilation Data Ingest System (MADIS) (NCEP 2019) and Baseline Surface Radiation Network (BSRN) (AWI 2019). For air quality model evaluation, measurement network data provided by AMET includes AErosol RObotic NETwork (AERONET) (NASA 2019), Ammonia Monitoring Network (AMoN) (NADP 2019), Air Quality System (AQS) (US EPA 2019a), Clean Air Status and Trends Network (CASTNET) (US EPA 2019c), Chemical Speciation Network (CSN) (US EPA 2019b), Interagency Monitoring of Protected Visual Environments (IMPROVE) (USGS 2019a), National Atmospheric Deposition Program (NADP), National Air Pollution Surveillance (NAPS) (Canada 2019), South-Eastern Aerosol Research and Characterization (SEARCH) (USGS 2019b), Tropospheric Ozone Assessment Report (TOAR) (Schultz et al., 2017). The model performance is evaluated and compared for the two 2014 cases in section 3.1.
In this section, the impact on model performance by including the NH3-SOA chemistry is first presented. Then, the chemical and meteorological impacts of the new chemistry are further investigated for the two 2014 cases. Finally, the sensitivity variation resulting from climate change is discussed by comparing the 2014 and 2050 cases.
The model performance of the 2014 simulations with and without ammonia uptake is evaluated for PM2.5, NH4+, and Organic Carbon (OC) against multiple observation networks detailed in section 2.2 (Table 2). The PM2.5 model performance of both Base_14 and UpTk_14 satisfies the recommended performance criteria proposed by Emery et al. (2017), with normalized mean bias (NMB) <
TABLE 2. Model performance statistics for PM2.5, NH4+, and Organic Carbon (OC) under different scenarios. PM2.5 and OC observation (Obs.) data are from three networks (IMPROVE, CSN and AQS); NH4+ observation data are from four networks (IMPROVE, CSN, CASTNET, and AQS). (Average—Avg.; Simulation—Sim.; Normalized Mean Bias—NMB; Normalized Mean Error—NME).
FIGURE 1. Changes in model errors between Base_14 and UpTk_14 for (A) PM2.5 and (B) OC at each observation site. Negative values indicate reduced model errors in UpTk_14 compares to Base-14. For (A), the SQUARE (AQS sites) only records daily mean value, the DIAMOND (AQS sites) records hourly data.
By comparing simulations UpTk_14 and Base_14, more details can be revealed on the impact on NH3 and PM2.5. Figure 2A shows the time-averaged NH3 concentration over the simulation domain for Base_14. Hot spots can be identified in regions with high agricultural NH3 emissions (e.g., California, North Carolina, Iowa, and Idaho, Supplementary Figure S2 in the SI) or regions with intense wildfire activities (e.g., Washington, Oregon, and Texas, Supplementary Figure S3 in the SI). Figure 2B shows the NH3 concentration difference between UpTk_14 and Base_14. The inclusion of the NH3-SOA uptake process results in an overall decrease in NH3 concentration throughout the domain, with the most significant decrease of almost 30% in California. The enhanced NH3 reduction for California is most likely due to the unique spatial overlap between domains of high NH3 and SOA concentrations (Supplementary Figure S4 in the SI). Among the states with top NH3 emissions, Iowa is the only one increasing NH3 concentration after including the NH3-SOA uptake. A more detailed investigation shows this is most likely due to the meteorological feedback resulting from the changing air quality conditions. Figure 2D shows the changes of 10-m wind speed for Iowa after the addition of NH3-SOA uptake. The overall reduction in wind speed indicates a loss in dispersion capability, especially over the northwest part of the state, where most of the NH3 emissions are located (Figure 2C). As a result, more NH3 is accumulated over those regions, resulting in the unusual increase of NH3 concentrations (Figure 2E). This phenomenon also implies that the most effective meteorological factor that could impact the atmospheric chemical concentrations from the chemistry-atmosphere feedbacks would be the change in atmospheric dispersion capability.
FIGURE 2. Spatial distribution of time-averaged NH3 concentration for Base_14 (A) CONUS and (C) State of Iowa. Spatial distribution of differences between UpTk_14 and Base_14 uptake case: (B) NH3 concentration for CONUS, (D) 10-m wind speed for Iowa and (E) NH3 concentration for Iowa.
The inclusion of NH3-SOA uptake also resulted in significant changes on modeled PM2.5. Figure 3A shows the changes of PM2.5 and its components (e.g., NH4+ and SOA) for the entire CONUS and four states with the highest NH3 emissions. In general, PM2.5 and SOA increased after the inclusion of NH3-SOA uptake, while NH4+ decreased. Among them, North Carolina shows the most significant decrease in NH4+ concentration and the largest increase in SOA. However, western states like California and Idaho exhibit both NH4+ and SOA decreases after implementing the NH3-SOA uptake. Figure 4A shows the mean PM2.5 concentration for Base_14. The high concentration along the southeast boundary of the domain is caused by foreign PM2.5 that originated from the western Sahara Desert in Africa.
FIGURE 3. Normalized averaged concentration changes due to the NH3- SOA uptake for the United States and four states with top anthropogenic NH3 emissions for the (A) 2014 case and (B) 2050 case.
FIGURE 4. Spatial distribution of time-averaged concentration for Base_14 (A) PM2.5 and (B) SOA. Spatial distribution of differences between UpTk_14 and Base_14 uptake case: (D) PM2.5, (E) SOA, (C) pH, and (F) 10-metter wind speed.
At the same time, some hot spots with high PM2.5 concentrations are caused by wildfires, like those in Washington, Oregon, and Texas. The result of the PM2.5 distribution shows a clear pattern with the majority of the pollution happening over the eastern part of the CONUS, except for California. Figure 4D presents the change in PM2.5 concentration after introducing the NH3-SOA uptake. The overall impact on PM2.5 is smaller than the impact on NH3. The 4% increase of domain-wide PM2.5 concentrations is still significant, with peak changes as large as 160 μg/m3. Most of the increases occur over the eastern part of the CONUS, overlapped mainly with the region with high PM2.5 concentration, except California. Such a distribution pattern is consistent with previous studies (Horne et al., 2018; Zhu et al., 2018). More detailed investigation shows that almost half of the PM2.5 changes result from the increase of SOA concentrations (Figure 4E). The most impacted region states close to the lower Mississippi River (e.g., Louisiana, Alabama, Mississippi, and Georgia), which also have elevated SOA background concentrations (Figure 4B). The drive behind the increase of SOA concentration is a change in particle acidity as more NH3 is consumed by the NH3-SOA uptake process (Figure 4C). The acid-catalyzed SOA formation pathway of isoprene epoxydiols is enhanced by increasing particle acidity (Pye et al., 2013), which is also observed in our previous works (Zhu et al., 2018; Wu et al., 2021).
Interestingly, the situation is different in Florida, where a decrease in PM2.5 and SOA concentration is predicted. Like the anomaly of increasing NH3 in Iowa, this particular case also results from the meteorological feedback effects. Figure 4F shows the changes of 10-m wind speed after adding the NH3-SOA uptake process, where a substantial increase in atmospheric dispersion is observed over Florida. Such an increase in wind speed clears out PM2.5 and decreases its overall concentration. The exact magnitude correlations between the change in wind and air pollutant concentrations are likely to be affected by multiple factors (e.g., boundary lay high, topographic, etc.) and would be an interesting subject for future investigations.
Changes in background meteorological conditions under RCP 8.5 climate scenarios largely altered the atmospheric chemistry conditions in the model. From the SI, Supplementary Figure S5 shows the meteorological and air quality changes between Base_50 and Base_14. First, there is a general increase in the surface temperature and a decrease in 10-m wind speed over the continent. The particle acidity also experiences dramatic changes. Furthermore, there is some substantial increase in pH values over the southeastern of the US, largely overlapping with the high SOA region in Figure 4B. For air pollutants, the overall NH3 concentration is decreased by 2.1%, with significant decreases in Washington, Oregon, and Texas. Decreases in those states have a direct connection with wildfire emissions. The plume rise or vertical distribution of wildfire emissions are calculated inline with the CMAQ model based on a Briggs plume rise formulation (Briggs 1982), which means the change in meteorological conditions (e.g., temperature, wind speed, and mixing height) could broadly impact the distribution of related emissions. In addition, the change in dispersion directions alters the distribution of NH3 concentrations in California and North Carolina. Also, some significant increase in PM2.5 concentration are observed in the southeast corner of the simulation domain, most likely due to the spread of the west Sahara original sandstorm from the boundary conditions under the new meteorology. Interestingly, SOA concentration decreases significantly over the southeastern CONUS, leading to a 15% decrease domain wide.
Figure 3B shows the impacts on air pollutants after including the NH3-SOA uptake in the 2050 climate scenario. Compared to the impacts for the 2014 case (Figure 3A), the domain-wide NH3 reduction is 30% smaller under the future climate scenario. One reason is that the lower background NH3 concentration produced less NH3 available for uptake. Another more important reason is the meteorological feedback due to the NH3-SOA uptake changed the wildfire emission, and the gains in wildfire emissions counterbalanced the reduction caused by the NH3-SOA uptake (Figure 5A). The significant increase in pollutants concentrations in Idaho is also caused by wildfire emissions (Figures 5A–D). For Iowa, the NH3 anomaly is largely reduced as the overall wind speed increased by 1.7% (Figure 5F). Due to the decrease in background SOA concentration, the impact of SOA (Figure 5C) and particle acidity (Figure 5E) over the southeastern CONUS due to NH3-SOA uptake is largely reduced compares to the 2014 case (Figure 4). Even with the additional gain from altered wildfire emissions, the increase of SOA is only about half of the 2014 case, and the decrease in pH is only 47%. Even for California, the impact caused by the NH3-SOA uptake is also largely reduced. Only North Carolina shows similar impacts to the 2014 cases. Although, climate change could also impact the upstream emissions that have not been considered in this study. These preliminary results imply that under a warmer climate, the impact of the NH3-SOA uptake might be reduced compared to current climate conditions. Also, the impact on smoke plume rise characters could be a significant factor during the meteorology and air quality two-way feedback, as observed in this study.
FIGURE 5. Spatial distribution of differences between UpTk_50 and Base_50 uptake case: (A) NH3, (B) PM2.5, (C) SOA, (D) NH4+, (E) pH, and (F) 10-metter wind speed.
In this study, the potential meteorology and air quality impacts of the chemical uptake of NH3 by SOA, resulting in nitrogen-containing organics, are investigated with the WRF-CMAQ two-way coupled model. Simulations over the continental US are performed for July 2014 and July 2050 under the RCP 8.5 IPCC scenario to study the potential joint impact due to climate change. First, results show that the NH3-SOA uptake process could reduce the model underestimation of PM2.5 (23% bias reduction), especially the underestimation of SOA over the southeastern CONUS (61% error reduction). Such an improvement in model performance indicates the importance of including NH3-SOA chemistry in the air quality model and its potential benefits. Secondly, a significant reduction in the concentration of NH3 was observed with the NH3-SOA uptake turned on, which also increased the model predicted particle acidity. In general, the inclusion of the NH3-SOA uptake would lead to lower ammonium concentrations but higher SOA concentrations, with some exceptions. Western U.S. states usually have decreased PM2.5 concentrations with the uptake included, whereas Eastern U.S. states usually exhibit increased PM2.5 concentrations. Most of the impact on pollution concentrations caused by the air quality and meteorology two-way feedback results from changes in wind speed and resulting dispersion conditions. As illustrated by the 2050 scenario, the impact on wildfire plume calculation due to the atmospheric chemistry and meteorology interaction through the two-way feedback could also significantly impact the resulting pollution distribution. Finally, as a preliminary study, we observe that under a warmer climate, including the NH3-SOA uptake produces a lower impact on air quality predictions than it does in the current climate.
Results show that the inclusion of the two-way feedback process has significant impacts on model behaviors when the new mechanism is implemented. It is noted that results from this study only consider the shortwave feedback. There are other feedback processes between the air pollutant and meteorology, including longwave feedbacks, the oceanic response and other indirect effects (Seinfeld and Pandis 2016) that are not included in this study and could be explored in future research. Indirect effects like changes in cloud formation and cloud albedo due to changes in aerosol concentration could enhance the meteorological feedback and further affect aerosol predations (Menon et al., 2002). It is likely the indirect effects could enhance the feedback impact over the southeast of the U.S. The oceanic response due to changes in aerosol estimation could also affect large-scale circulations (Cai et al., 2006), which should be considered for long-term simulations but most likely to be insignificant in our short-term study. Moreover, it is also noted that the NH3 uptake coefficient used in this study is on the higher end of current laboratory measurement (
The raw data supporting the conclusion of this article will be made available by the authors, without undue reservation.
SZ designed and conducted WRF-CMAQ simulations and SZ wrote the manuscript. SZ, KW, SN and DD reviewed and modified the manuscript. KW contributes analysis on meteorological results. SN provides the chemical mechanism of ammonia uptake by SOA. DD administered the project. All authors contributed to manuscript revision and read and approved the submitted version.
The authors declare that the research was conducted in the absence of any commercial or financial relationships that could be construed as a potential conflict of interest.
All claims expressed in this article are solely those of the authors and do not necessarily represent those of their affiliated organizations, or those of the publisher, the editors and the reviewers. Any product that may be evaluated in this article, or claim that may be made by its manufacturer, is not guaranteed or endorsed by the publisher.
This publication was developed under Assistance Agreement No. EPA 83588101 was awarded by the US Environmental Protection Agency to the Regents of the University of California. It has not been formally reviewed by the EPA. The views expressed in this document are solely those of the authors and do not necessarily reflect those of the agency. The EPA does not endorse any products or commercial services mentioned in this publication.
The Supplementary Material for this article can be found online at: https://www.frontiersin.org/articles/10.3389/fenvs.2022.867908/full#supplementary-material
Adams, L. (2017). Mechanism for Cb6r3_ae6_aq Uses the Following Species. Available at: https://github.com/USEPA/CMAQ/blob/5.2/DOCS/User_Manual/Appendix_A/cb6r3_ae6_aq/CB6_species_table. (Accessed September 2, 2020).
Appel, K. W., Gilliam, R. C., Davis, N., Zubrow, A., and Howard, S. C. (2011). Overview of the Atmospheric Model Evaluation Tool (AMET) v1.1 for Evaluating Meteorological and Air Quality Models. Environ. Model. Softw. 26 (4), 434–443. doi:10.1016/j.envsoft.2010.09.007
Appel, K. W., Pouliot, G. A., Simon, H., Sarwar, G., Pye, H. O. T., Napelenok, S. L., et al. (2013). Evaluation of Dust and Trace Metal Estimates from the Community Multiscale Air Quality (CMAQ) Model Version 5.0. Geosci. Model. Dev. 6 (4), 883–899. doi:10.5194/gmd-6-883-2013
AWI (2019). Baseline Surface Radiation Network: Baseline Surface Radiation Network. Available at: https://bsrn.awi.de/. (Accessed September 2, 2020).
Behera, S. N., and Sharma., M. (2010). Investigating the Potential Role of Ammonia in Ion Chemistry of Fine Particulate Matter Formation for an Urban Environment. Sci. Total Environ. 408 (17), 3569–3575. doi:10.1016/j.scitotenv.2010.04.017
Bell, D. M., Imre, D., T. Martin, S., and Zelenyuk, A. (2017). The Properties and Behavior of α-pinene Secondary Organic Aerosol Particles Exposed to Ammonia under Dry Conditions. Phys. Chem. Chem. Phys. 19 (9), 6497–6507. doi:10.1039/c6cp08839b
Bones, D. L., Henricksen, D. K., Man, S. A., Gonsior, M., Bateman, A. P., Nguyen, T. B., et al. (2010). Appearance of strong Absorbers and Fluorophores in Limonene-O3secondary Organic Aerosol Due to NH4+-mediated Chemical Aging over Long Time Scales. J. Geophys. Res. 115 (D5), D05203. doi:10.1029/2009JD012864
Bouwman, A. F., Lee, D. S., Asman, W. A. H., Dentener, F. J., Van Der Hoek, K. W., and Olivier, J. G. J. (1997). A Global High-Resolution Emission Inventory for Ammonia. Glob. Biogeochem. Cycles 11 (4), 561–587. doi:10.1029/97GB02266
Brasseur, G. P., Schultz, M., Granier, C., Saunois, M., Diehl, T., Botzet, M., et al. (2006). Impact of Climate Change on the Future Chemical Composition of the Global Troposphere. J. Clim. 19 (16), 3932–3951. doi:10.1175/JCLI3832.1
Briggs, G. A. (1982). “Plume Rise Predictions,” in Lectures on Air Pollution and Environmental Impact Analyses (Boston, MA: American Meteorological Society), 59–111, 59–111. doi:10.1007/978-1-935704-23-2_3
Bruyère, C. L., DoneDoneHolland, J. M. Greg. J., Holland, G. J., and Fredrick, S. (2013). Bias Corrections of Global Models for Regional Climate Simulations of High-Impact Weather. Clim. Dyn. 43 (7–8), 1847–1856. doi:10.1007/s00382-013-2011-6
Byun, D., and Schere, K. L. (2006). Review of the Governing Equations, Computational Algorithms, and Other Components of the Models-3 Community Multiscale Air Quality (CMAQ) Modeling System. Appl. Mech. Rev. 59, 51. doi:10.1115/1.2128636
Cai, W., Bi, D., Church, J., Cowan, T., Dix, M., and Rotstayn, L. (2006). Pan-Oceanic Response to Increasing Anthropogenic Aerosols: Impacts on the Southern Hemisphere Oceanic Circulation. Geophys. Res. Lett. 33 (21), 1–5. doi:10.1029/2006GL027513
Campbell, P., Zhang, Y., Yan, F., Lu, Z., and Streets, D. (2018). Impacts of Transportation Sector Emissions on Future U.S. Air Quality in a Changing Climate. Part II: Air Quality Projections and the Interplay between Emissions and Climate Change. Environ. Pollut. 238 (July), 918–930. doi:10.1016/j.envpol.2018.03.016
Canada (2019). National Air Pollution Surveillance (NAPS) Program - Open Government Portal. Available at: https://open.canada.ca/data/en/dataset/1b36a356-defd-4813-acea-47bc3abd859b. (Accessed September 2, 2020).
Canty, T. P., Hembeck, L., Vinciguerra, T. P., Anderson, D. C., Goldberg, D. L., Carpenter, S. F., et al. (2015). Ozone and NOx Chemistry in the Eastern US: Evaluation of CMAQ/CB05 with Satellite (OMI) Data. Atmos. Chem. Phys. Discuss. 15 (4), 4427–4461. doi:10.5194/acpd-15-4427-2015
Clarisse, L., Clerbaux, C., Dentener, F., Hurtmans, D., and Coheur, P.-F. (2009). Global Ammonia Distribution Derived from Infrared Satellite Observations. Nat. Geosci 2, 479–483. doi:10.1038/ngeo551
Clough, S. A., Shephard, M. W., Mlawer, E. J., Delamere, J. S., Iacono, M. J., Cady-Pereira, K., et al. (2005). Atmospheric Radiative Transfer Modeling: A Summary of the AER Codes. J. Quantitative Spectrosc. Radiative Transfer 91 (2), 233–244. doi:10.1016/j.jqsrt.2004.05.058
Constable, J. V. H., Guenther, A. B., Schimel, D. S., and Monson, R. K. (1999). Modelling Changes in VOC Emission in Response to Climate Change in the Continental United States. Glob. Change Biol. 5 (7), 791–806. doi:10.1046/j.1365-2486.1999.00273.x
de Almeida Albuquerque, T. T., de Fátima Andrade, M., Ynou, R. Y., Moreira, D. M., Andreão, W. L., dos Santos, F. S., et al. (2018). WRF-SMOKE-CMAQ Modeling System for Air Quality Evaluation in São Paulo Megacity with a 2008 Experimental Campaign Data. Environ. Sci. Pollut. Res. 25 (36), 36555–36569. doi:10.1007/s11356-018-3583-9
Dentener, F., Drevet, J., Lamarque, J. F., Bey, I., Eickhout, B., Fiore, A. M., et al. (2006). Nitrogen and Sulfur Deposition on Regional and Global Scales: A Multimodel Evaluation. Glob. Biogeochem. Cycles 20 (4), a–n. doi:10.1029/2005GB002672
Emery, C., Liu, Z., Russell, A. G., Odman, M. T., Yarwood, G., and Kumar, N. (2017). Recommendations on Statistics and Benchmarks to Assess Photochemical Model Performance. J. Air Waste Manage. Assoc. 67 (5), 582–598. doi:10.1080/10962247.2016.1265027
Emmons, L. K., Walters, S., Hess, P. G., Lamarque, J.-F., Pfister, G. G., Fillmore, D., et al. (2010). Description and Evaluation of the Model for Ozone and Related Chemical Tracers, Version 4 (MOZART-4). Geosci. Model. Dev. 3 (1), 43–67. doi:10.5194/gmd-3-43-2010
Fiore, A. M., Naik, V., Spracklen, D. V., Steiner, A., Unger, N., Prather, M., et al. (2012). Global Air Quality and Climate. Chem. Soc. Rev. 41 (19), 6663. doi:10.1039/c2cs35095e
Gan, C. M., Binkowski, F., Pleim, J., Xing, J., Wong, D., Mathur, R., et al. (2015). Assessment of the Aerosol Optics Component of the Coupled WRF-CMAQ Model Using CARES Field Campaign Data and a Single Column Model. Atmos. Environ. 115 (August), 670–682. doi:10.1016/j.atmosenv.2014.11.028
Grell, G., and Baklanov, A. (2011). Integrated Modeling for Forecasting Weather and Air Quality: A Call for Fully Coupled Approaches. Atmos. Environ. 45 (38), 6845–6851. doi:10.1016/j.atmosenv.2011.01.017
Hogrefe, C., Pouliot, G., Wong, D., Torian, A., Roselle, S., Pleim, J., et al. (2015). Annual Application and Evaluation of the Online Coupled WRF-CMAQ System over North America under AQMEII Phase 2. Atmos. Environ. 115 (August), 683–694. doi:10.1016/j.atmosenv.2014.12.034
Hong, C., Zhang, Q., Zhang, Y., Tang, Y., Tong, D., and He, K. (2017). Multi-Year Downscaling Application of Two-Way Coupled WRF v3.4 and CMAQ v5.0.2 over East Asia for Regional Climate and Air Quality Modeling: Model Evaluation and Aerosol Direct Effects. Geosci. Model. Dev. 10 (6), 2447–2470. doi:10.5194/gmd-10-2447-2017
Horne, J. R., Zhu, S., Montoya-Aguilera, J., Hinks, M. L., Wingen, L. M., Nizkorodov, S. A., et al. (2018). Reactive Uptake of Ammonia by Secondary Organic Aerosols: Implications for Air Quality. Atmos. Environ. 189, 1–8. doi:10.1016/j.atmosenv.2018.06.021
Iacono, M. J., Delamere, J. S., Mlawer, E. J., Shephard, M. W., Clough, S. A., and Collins, W. D. (2008). Radiative Forcing by Long-Lived Greenhouse Gases: Calculations with the AER Radiative Transfer Models. J. Geophys. Res. 113 (13). doi:10.1029/2008JD009944
Kain, J. S. (2004). The Kain-Fritsch Convective Parameterization: An Update, J. Appl. Meteorol. 43, 1702–2181. doi:10.1175/1520-0450(2004)043<0170
Kaufman, Y. J., and Fraser., R. S. (1997). The Effect of Smoke Particles on Clouds and Climate Forcing. Science 277 (5332), 1636–1639. doi:10.1126/science.277.5332.1636
Lamarque, J.-F., Emmons, L. K., Hess, P. G., Kinnison, D. E., Tilmes, S., Vitt, F., et al. (2012). CAM-chem: Description and Evaluation of Interactive Atmospheric Chemistry in the Community Earth System Model. Geosci. Model. Dev. 5 (2), 369–411. doi:10.5194/gmd-5-369-2012
Laskin, A., Laskin, J., and Nizkorodov, S. A. (2015). Chemistry of Atmospheric Brown Carbon. Chem. Rev., 115, 4335–4382. doi:10.1021/cr5006167
Li, J., Zhang, M., Wu, F., Sun, Y., and Tang, G. (2017). Assessment of the Impacts of Aromatic VOC Emissions and Yields of SOA on SOA Concentrations with the Air Quality Model RAMS-CMAQ. Atmos. Environ. 158, 105–115. doi:10.1016/j.atmosenv.2017.03.035
Li, Y. J., Liu, P., Gong, Z., Wang, Y., Bateman, A. P., Bergoend, C., et al. (2015). Chemical Reactivity and Liquid/Nonliquid States of Secondary Organic Material. Environ. Sci. Technol. 49 (22), 13264–13274. doi:10.1021/acs.est.5b03392
Lin, G., PennerPenner, J. E., and Zhou, C. (2016). How Will SOA Change in the Future? Geophys. Res. Lett. 43 (4), 1718–1726. doi:10.1002/2015GL067137
Liu, P., Li, Y. J., Wang, Y., Bateman, A. P., Zhang, Y., Gong, Z., et al. (2018). Highly Viscous States Affect the Browning of Atmospheric Organic Particulate Matter. ACS Cent. Sci. 4 (2), 207–215. doi:10.1021/acscentsci.7b00452
Liu, X.-H., Zhang, Y., Cheng, S.-H., Xing, J., Zhang, Q., Streets, D. G., et al. (2010). Understanding of Regional Air Pollution over China Using CMAQ, Part I Performance Evaluation and Seasonal Variation. Atmos. Environ. 44 (20), 2415–2426. doi:10.1016/j.atmosenv.2010.03.035
Matthias, V. (2008). The Aerosol Distribution in Europe Derived with the Community Multiscale Air Quality (CMAQ) Model: Comparison to Near Surface In Situ and Sunphotometer Measurements. Atmos. Chem. Phys. 8 (17), 5077–5097. doi:10.5194/acp-8-5077-2008
Menon, S., AnthonyDel Genio, D., Koch, D., and George, T. (2002). GCM Simulations of the Aerosol Indirect Effect: Sensitivity to Cloud Parameterization and Aerosol Burden. J. Atmos. Sci. 59 (3 PT 2), 6922–7713. doi:10.1175/1520-0469(2002)059<0692:gsotai>2.0.co;2
Monaghan, A. J., Steinhoff, D. F., Bruyere, C. L., and Yates, D. (2014). NCAR CESM Global Bias-Corrected CMIP5 Output to Support WRF/MPAS Research. Available at: https://rda.ucar.edu/datasets/ds316.1/ (Accessed September 2, 2020). doi:10.5065/D6DJ5CN4
Montoya-Aguilera, J., Hinks, M. L., Aiona, P. K., Wingen, L. M., Horne, J. R., Zhu, S., et al. (2018). Reactive Uptake of Ammonia by Biogenic and Anthropogenic Organic Aerosols. ACS Symp. Ser. 1299, 127–147. doi:10.1021/bk-2018-1299.ch007
Morrison, H., Thompson, G., and Tatarskii, V. (2009). Impact of Cloud Microphysics on the Development of Trailing Stratiform Precipitation in a Simulated Squall Line: Comparison of One- and Two-Moment Schemes. Monthly Weather Rev. 137 (3), 991–1007. doi:10.1175/2008MWR2556.1
Nadp, (2019). Atmospheric Ammonia Network. Available at: http://nadp.slh.wisc.edu/amon/ (Accessed September 2, 2020).
NASA (2019). Aerosol Robotic Network (AERONET) Homepage. Available at: https://aeronet.gsfc.nasa.gov/ (Accessed September 2, 2020).
Ncep, (2019). NCEP Meteorological Assimilation Data Ingest System (MADIS). Available at: https://madis.ncep.noaa.gov/ (Accessed September 2, 2020).
Ncep, (2015). NCEP North American Mesoscale (NAM) 12 Km Analysis. Boulder, Colo: Research Data Archive at the National Center for Atmospheric Research, Computational and Information Systems LaboratoryUpdated Daily. doi:10.5065/G4RC-1N91
Nguyen, K., and Dabdub, D. (2002). NO X and VOC Control and its Effects on the Formation of Aerosols. Aerosol Sci. Tech. 36 (5), 560–572. doi:10.1080/02786820252883801
Pausata, F. S. R., Gaetani, M., Messori, G., Kloster, S., and Dentener, F. J. (2015). The Role of Aerosol in Altering North Atlantic Atmospheric Circulation in Winter and its Impact on Air Quality. Atmos. Chem. Phys. 15 (4), 1725–1743. doi:10.5194/acp-15-1725-2015
Pleim, J. E. (2007). A Combined Local and Nonlocal Closure Model for the Atmospheric Boundary Layer. Part I: Model Description and Testing. J. Appl. Meteorology Climatology 46 (9), 1383–1395. doi:10.1175/JAM2539.1
Pye, H. O. T. (2016). CMAQv5.1 SOA Update - CMASWIKI. Available at: https://www.airqualitymodeling.org/index.php/CMAQv5.1_SOA_Update (Accessed May 15, 2020).
Pye, H. O. T., Pinder, R. W., Piletic, I. R. Ying. Xie., Xie, Y., Capps, S. L., Lin, Y.-H., et al. (2013). Epoxide Pathways Improve Model Predictions of Isoprene Markers and Reveal Key Role of Acidity in Aerosol Formation. Environ. Sci. Technol. 47 (19), 11056–11064. doi:10.1021/es402106h
Rosenfeld, D., Dai, J., Yu, X., Yao, Z., Xu, X., Yang, X., et al. (2007). Inverse Relations between Amounts of Air Pollution and Orographic Precipitation. Science 315 (5817), 1396–1398. doi:10.1126/science.1137949
Rosenfeld, D., Woodley, W. L., Axisa, D., Freud, E., Hudson, J. G., and Givati, A. (2008). Aircraft Measurements of the Impacts of Pollution Aerosols on Clouds and Precipitation over the Sierra Nevada. J. Geophys. Res. 113 (D15), D15203. doi:10.1029/2007JD009544
Schultz, M. G., Sabine, S., Lyapina, O., Cooper, O. R., Galbally, I., Petropavlovskikh, I., et al. (2017). Tropospheric Ozone Assessment Report, Links to Global Surface Ozone Datasets. (Elementa: Science of the Anthropocene), 5 (58), 1–26. doi:10.1525/elementa.244
Schwede, D. B., Schwede, D., George, P., and Pierce, T. (2005). Changes to the Biogenic Emissions Inventory System Version 3 (BEIS3).” in 4th Annual CMAS-3 Users’ Conference, 26. Chapel hill, NC, 28.
Seinfeld, J. H., and Pandis, S. N. (2016). Atmospheric Chemistry and Physics: From Air Pollution to Climate Change. New York: John Wiley & Sons,. https://books.google.com/books?hl=en&lr=&id=n_RmCgAAQBAJ&oi=fnd&pg=PR5&dq=Atmospheric+chemistry+and+physics+Ltd+from+air+pollution+to+climate+change&ots=gRO7FJnjfQ&sig=RcpA08Z72j9hxQ6IbbAdtJxJKPA.
Sharma, S., Chatani, S., Mahtta, R., Goel, A., and Kumar, A. (2016). Sensitivity Analysis of Ground Level Ozone in India Using WRF-CMAQ Models. Atmos. Environ. 131 (April), 29–40. doi:10.1016/j.atmosenv.2016.01.036
Skjøth, C. A., and Geels, C. (2013). The Effect of Climate and Climate Change on Ammonia Emissions in Europe. Atmos. Chem. Phys. 13 (1), 117–128. doi:10.5194/acp-13-117-2013
US EPA (2018). 2014 National Emissions Inventory, Version 2 Technical Support Document. Available at: https://www.epa.gov/sites/production/files/2018-07/documents/nei2014v2_tsd_05jul2018.pdf (Accessed July 1, 2020).
US EPA (2019a). Air Quality System (AQS). Available at: https://www.epa.gov/aqs/obtaining-aqs-data (Accessed July 1, 2020).
US EPA (2019b). Chemical Speciation Network. CSN) - General Information. Available at: https://www.epa.gov/amtic/chemical-speciation-network-csn (Accessed September 2, 2020).
US EPA (2019c). Clean Air Status and Trends Network (CASTNET). Available at: https://www.epa.gov/castnet (Accessed July 1, 2020).
US EPA (2017). SMOKE v4.5 User’s Manual. Available at: https://www.cmascenter.org/smoke/documentation/4.5/manual_smokev45.pdf (Accessed September 2, 2020).
USGS (2019a). Interagency Monitoring of Protected Visual Environments. Available at: https://my.usgs.gov/gcmp/program/show/941778 (Accessed September 2, 2020).
USGS (2019b). SouthEastern Aerosol Research and Characterization Network. Available at: https://my.usgs.gov/gcmp/program/show/943855 (Accessed September 2, 2020).
Wang, J., Wang, S., Jiang, J., Ding, A., Zheng, M., Zhao, B., et al. (2014). Impact of Aerosol-Meteorology Interactions on fine Particle Pollution during China's Severe Haze Episode in January 2013. Environ. Res. Lett. 9 (9), 094002. doi:10.1088/1748-9326/9/9/094002
Wong, D. C., Pleim, J., Mathur, R., Binkowski, F., Otte, T., Gilliam, R., et al. (2012). WRF-CMAQ Two-Way Coupled System with Aerosol Feedback: Software Development and Preliminary Results. Geosci. Model. Dev. 5 (2), 299–312. doi:10.5194/gmd-5-299-2012
Wu, K., Zhu, S., Liu, Y., Wang, H., Yang, X., Liu, L., et al. (2021). Modeling Ammonia and its Uptake by Secondary Organic Aerosol over China. Geophys. Res. Atmos. 126 (7), 1–20. doi:10.1029/2020JD034109
Xing, J., Wang, J., Mathur, R., Pleim, J., Wang, S., Hogrefe, C., et al. (2016). Unexpected Benefits of Reducing Aerosol Cooling Effects. Environ. Sci. Technol. 50 (14), 7527–7534. doi:10.1021/acs.est.6b00767
Xiu, A., and Pleim, J. E. (2001). Development of a Land Surface Model. Part I: Application in a Mesoscale Meteorological Model. J. Appl. Meteorol. 40, 192–209. doi:10.1175/1520-0450(2001)040<0192:doalsm>2.0.co;2
Yarwood, G., Whitten, G. Z., Jung, J., Heo, G., and Allen, D. (2010). Development, Evaluation and Testing of Version 6 of the Carbon Bond Chemical Mechanism (CB6). Austin, TX: Final Report Prepared for Texas Commission on Environmental Quality.
Zhu, S., Horne, J. R., Mac Kinnon, M., Samuelsen, G. S., and Dabdub, D. (2019). Comprehensively Assessing the Drivers of Future Air Quality in California. Environ. Int. 125 (April), 386–398. doi:10.1016/j.envint.2019.02.007
Keywords: ammonia, secondary organic aerosol (SOA), particulate matter, ammonium nitrate (AN), climate change, coupled meteorological and air quality modeling
Citation: Zhu S, Wu K, Nizkorodov SA and Dabdub D (2022) Modeling Reactive Ammonia Uptake by Secondary Organic Aerosol in a Changing Climate: A WRF-CMAQ Evaluation. Front. Environ. Sci. 10:867908. doi: 10.3389/fenvs.2022.867908
Received: 01 February 2022; Accepted: 11 March 2022;
Published: 29 March 2022.
Edited by:
Yang Gao, Ocean University of China, ChinaReviewed by:
Yang Yang, Nanjing University of Information Science and Technology, ChinaCopyright © 2022 Zhu, Wu, Nizkorodov and Dabdub. This is an open-access article distributed under the terms of the Creative Commons Attribution License (CC BY). The use, distribution or reproduction in other forums is permitted, provided the original author(s) and the copyright owner(s) are credited and that the original publication in this journal is cited, in accordance with accepted academic practice. No use, distribution or reproduction is permitted which does not comply with these terms.
*Correspondence: Shupeng Zhu, c3pAYXBlcC51Y2kuZWR1; Donald Dabdub, ZGRhYnViQHVjaS5lZHU=
Disclaimer: All claims expressed in this article are solely those of the authors and do not necessarily represent those of their affiliated organizations, or those of the publisher, the editors and the reviewers. Any product that may be evaluated in this article or claim that may be made by its manufacturer is not guaranteed or endorsed by the publisher.
Research integrity at Frontiers
Learn more about the work of our research integrity team to safeguard the quality of each article we publish.