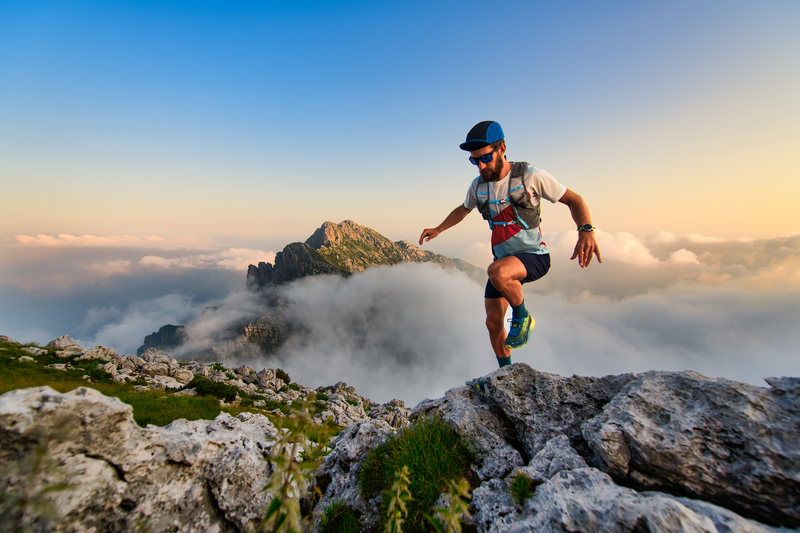
95% of researchers rate our articles as excellent or good
Learn more about the work of our research integrity team to safeguard the quality of each article we publish.
Find out more
ORIGINAL RESEARCH article
Front. Environ. Sci. , 11 May 2022
Sec. Environmental Economics and Management
Volume 10 - 2022 | https://doi.org/10.3389/fenvs.2022.864346
This article is part of the Research Topic Digital Science and Technology for Sustainable Water Futures View all 8 articles
Evaluation of gray water footprint (GWF) flow and its driving mechanism is imperative to trace the source of pollution and formulate the pollution responsibility-sharing strategies to reduce the shortage of water. We quantify the GWF of 22 sectors in Fujian Province in 2002, 2007, 2012, and 2017, using an economic input–output life cycle assessment (EIO-LCA) model, and establish a five-factor structural decomposition analysis (SDA) model to track the driving forces of the GWF. The results show that the animal husbandry sector of the primary industry is the largest outsourcing sector, outsourcing an average of 7 Bm3 GWF by other regions annually. The construction sector is an industry with more pollution outsourcing at the production end, and it should take additional pollution responsibility for its upstream sector. The changes of the GWF in Fujian Province are mainly driven by the final demand per capita and GWF outsourcing. The sector most affected by force drivers is construction, followed by machinery and equipment manufacturing, and other services. This study better reflects the sectoral pollution outsourcing situation by assessing sectoral GWF flow and net inflow GWF caused by sector trading. We aim at identifying new sectors of water pollution and sharing the pollution responsibility between upstream and downstream, providing a new perspective to analyze water quality problems in water-rich regions. Moreover, our findings imply an urgent need to develop policies related to water pollution transfer for various sectors from a whole supply chain perspective.
In parallel with climate change and environmental issues (Elahi et al., 2021; Elahi et al., 2022a; Elahi et al., 2022b), water shortage is one of the main handicaps to sustainable development globally, especially in China (Ma et al., 2020). Along with the economic globalization, the division of labor of intra-product refined, the trade links among various sectors are becoming increasingly extensive and in-depth (WTO et al., 2019). An increasing number of scholars are paying attention to the transfer of water resources by trade and its environmental impact (Mekonnen and Hoekstra, 2020; Yang et al., 2020). In addition, most of them have asserted that the primary cause for water shortage is poor water management (Dolan et al., 2021), rather than a shortfall of physical water. They have proposed trading as an effective means to mitigate the regional lack of freshwater (Zhang and Anadon, 2014). To accurately describe the impact of trade on water resources, Allan introduced the concept of virtual water in 1993 (UNFAO et al., 2021), which refers to the amount of freshwater used in the production and distribution of food and consumer goods; it is also known as “embodied water” (Han et al., 2015). Subsequently, Hoekstra and Hung (2005) proposed a concept of water footprint based on virtual water, which combines the physical water and virtual water of production and consumption. Both water footprint and virtual water have three aspects: blue water (ground and surface water), green water (soil water), and gray water (diluted water) (Hoekstra et al., 2011). According to their definitions, the gray water footprint (GWF) is defined as the amount of fresh water required to assimilate the pollutants that directly and indirectly arise from sectoral trade in the supply chain to meet particular water quality standards. The GWF includes the direct GWF (DGWF) and the indirect GWF (IGWF); the latter is also called virtual gray water or gray virtual water or embodied gray water (Cazcarro et al., 2016). The total GWF is the sum of the GWF of each sector.
The traditional view is that water-scarce regions can save water by importing water-intensive commodities from water-abundant areas and exporting water-scarce merchandise from their regions; Allan calls this the virtual water strategy (UNFAO et al., 2021). However, Zhao’s research shows that China’s virtual water trade flows are all from water-deficient regions to water-rich regions, which are further aggravating China’s water shortage (Zhao et al., 2015). Li et al. (2021) identified China’s GWF flow from water-deficient provinces in the central and northern regions to water-rich provinces in southeastern China. Zhang et al. (2019) found that the GWF exacerbates water shortages more than the blue and green footprint. In addition, Lenzen et al. (2013) indicated that the trade-driven relocation of water pollution is increasing. The sectoral trade of the GWF has been widely addressed at the international (Zhao et al., 2019), interprovincial (Li et al., 2021), and basin levels (Sun et al., 2021). However, the sectoral trade of the GWF in water-scarce regions has received more attention (Zhao et al., 2021a; Long et al., 2021), and less attention has been paid to water-rich areas. As a matter of fact, even though a region may be water-abundant, it does not mean that there is no water shortage; water-rich regions often become water-deficient areas because of water quality deterioration, which is caused by poor water management (Fu et al., 2022). Water pollution is the most prominent manifestation of water shortage in water-rich areas. Therefore, the management of GWF in water-sufficient areas is imperative. Furthermore, exploring the GWF in water-rich regions might help to identify the root causes of the GWF patterns and alleviate water shortages in China.
With the globalization of supply chains, a growing number of commodities and services are “made in the other sectors” rather than in just one. The GWF utilizing only final products (DGWF) would overlook the pollution transfers from the trade of various intermediate products (IGWF) in the supply chain. Hence, in recent years, the GWF has been extensively combined with an input–output table (Deng et al., 2021; Li et al., 2021), whereas normally, these studies focus on the flow of the GWF caused by interregional trade, especially in export and import trade. However, more than 70% GWF of most Chinese provinces came from the domestic sector (Zhang et al., 2019), and it is necessary to broaden the focus to the domestic sectoral GWF. As far as we know, except for Zhao et al. (2019) and Li et al. (2021), who quantified regional and sectoral pollution outsourcing in terms of net imports and net exports, there are few studies to date that quantify sectoral and regional GWFs outsourcing in terms of net inflows (domestic extra-provincial inflows + imports − domestic extra-provincial outflows − exports) and net outflows (domestic extra-provincial outflows + exports − domestic extra-provincial inflows − imports). Moreover, the production of the upstream sector is often consumed/outsourced by the downstream sector, which is not responsible for its pollution. Sectoral GWF flow is the primary process of water pollution consumption/outsourcing, sectors are the key link to water problem (Cazcarro et al., 2016) and water management (Zhao et al., 2021a). Therefore, it is also worth paying attention to identify key outsourcing sectors and sharing the responsibility of sectoral pollution fairly from the supply chain. In addition, the primary industry is a sector with a large amount of pollution discharge, but most existing studies have treated it as a whole sector (agriculture) (Zhao et al., 2021b; Fu et al., 2022), especially focused on the agricultural crop (Huang et al., 2021), and few have divided it further. For example, although Zhao et al. (2019) calculated the overall GWF of agriculture, forestry, animal husbandry, and fisheries, no specific data were presented for each individual sector, and most scholars have ignored the contribution of product services of agriculture, forestry, animal husbandry, and fishery sectors to the GWF. Therefore, it is necessary to take fine research of its subsectors, which is of great significance to determine the new sectors. To fill the gap, we employ an economic input–output life cycle assessment (EIO-LCA) model to recognize and analyze the detailed intermediate transmission of the domestic sectoral production and consumption processes (Okadera et al., 2015) in Fujian Province. This model can trace the redistribution of DGWF from production and consumption and assess the indirect and direct GWFs in sectoral trade with high precision. The EIO-LCA method was first used by Hendrickson and Horvath to assess the environmental impact of cross-sector business activities (Hendrickson et al., 1998). They established a nexus between economic activities and pollution discharges. Blackhurst et al. (2010) carried out a detailed assessment of indirect and direct water utilizations for United States industrial sectors using an EIO-LCA model. Egilmez and Park (2014) examined the carbon, energy and water footprint using the EIO-LCA model to evaluate the environmental performance of U.S. manufacturing. Li et al. (2017) employed EIO-LCA to estimate Beijing’s GWF. Both Egilmez and Park and Li et al. did not assess the driving forces of sectoral trade, they only quantified the water footprint without further study. Next to quantifying the GWF, it is important to identify and appraise the key forces and industry hotspots that have caused the changes in the GWF. In practice, there are two commonly used methods to analyze the driving forces in different periods: structural decomposition analysis (SDA) (Cai et al., 2020) and index decomposition analysis (IDA) [among the IDA methods, the logarithmic mean division index (LMDI) is widely used] (Zhang et al., 2019). The SDA method, which is based on an input–output table, is more convenient to obtain data, and the calculation program of SDA is more perfect. The SDA method solves the problem that zero value does not participate in the accounting, and it analyzes the influence of direct and indirect factors. Therefore, we use the SDA model to establish the driving forces model.
Just as water pollution exacerbates water shortages (Fu et al., 2022), whether GWF outsourcing will intensify the water pressure and pollution responsibility of the outsourced sectors? To answer this question, we conduct a sector-wide study on the primary industry, a water resource consumption intensity hotspot. To be specific, we quantify the indirect and direct GWFs of 22 sectors in Fujian Province in 2002, 2007, 2012, and 2017 using the EIO-LCA model. For the first time, we quantified GWF outsourcing in Fujian Province and 22 sectors using net inflows and net outflows, and investigated both the production and consumption levels of sectoral outsourcing situations. Then, the SDA model was used to analyze further sectors that contribute more to the change in the GWF and the main drivers, and precisely identify the industry’s pollution hotspot sectors and influencing factors. This study links the GWF flow with sectoral trade, integrating pollution outsourcing theory into sectoral GWF, and combining pollution responsibility of upstream with downstream departments to provide empirical research on sectoral pollution liability sharing.
The remaining of the article is organized as follows. Section 2 describes how the EIO-LCA model redistributes the direct GWF to each sector and provides an example of the SDA decomposition process. Section 3 presents the main findings. Section 4 discusses the main results. Section 5 draws the main conclusions.
Fujian Province lies on the eastern coast of China (see Figure 1) and is an important outlet to the sea for mainland China. It faces Taiwan and is adjacent to Hong Kong and Macao. It is a precipitation-rich province. The proposal of the Belt and Road Initiative in China has resulted in the economic development of Fujian Province being promoted, the trade between inside and outside the province is smoother, and the province’s new status has driven its rapid economic development. Before 2013, the Fujian’s GDP growth rate ranked in the bottom 10 of China’s 31 provinces, but, after 2013, it ranked among the top 10. However, in the process of this economic catch-up and development in Fujian, water pollution has been caused by unreasonable management and utilization, and this has led to water shortage (Yu et al., 2020). In addition to the policy, Fujian’s unique geographical location has also helped its import and export volumes to be among the top 10 in China. Therefore, the transfer of water pollution from trade is a cause for concern. Moreover, Liu et al. (2020) verified that Fujian’s GWF accounts for more than 80% of its total water footprint. According to Ma et al. (2020), Fujian is already facing a water quality shortage. Most importantly, the more abundant the water, the easier it is to fall into the trap of poor water management. Therefore, the GWF management in Fujian Province plays a vital role in its economic development and water conservation.
To specify the GWF produced by sectoral trade in Fujian Province, we used the most recent single regional input–output tables: 2002, 2007, 2012, and 2017. We first combined the sectors in the input–output table according to the industry classification standards issued by China in 2017 (GB/T 4754–2017), and finally merged them into 22 departments (see Table 1). It was assumed that the pollution could be discharged through all economic activities, such as production, consumption, and trade.
TABLE 1. Sectoral profile (according to the industrial classification for the national economic activities of China, GB/T 4754–2017).
In this study, we analyzed the pollution transfer of disparate departments in Fujian Province. This was approached from two aspects: one was through the trade originating from the links of the production and consumption process, and the other was through interregional trade (see Figure 2). We also assumed an equal discharge coefficient of the same pollutant in the same sector, regardless of the geographical origin. The input–output tables used in this study compiled by Fujian Provincial Bureau of Statistics (2002, 2007, 2012 2017a), which have all been converted into constant input–output tables with 2017 as the base year by the price index deflator method (An et al., 2018) (see Eqs 1–5 for details); here, we take only the year 2002 as an example.
We analyzed the distribution structure, transfer direction, and extent of the GWF in different departments from the perspective of production and consumption. The EIO-LCA model is as follows:
The model realizes the redistribution of the direct GWF (DGWF) consumed by pollutants discharged into Fujian’s water body, and the accounted GWF contains both the direct GWF of the sectoral final product consumption (DGWFf) and the indirect GWF of the intermediate product consumption (IGWF).
where F is the GWF matrix (22 × 22). Each element of the matrix F is expressed as fij. It is added in a vertical direction as the row vector GWFc (22 × 1), which represents the department GWF embodied in the final consumption. The horizontal addition is the column vector GWFP (1 × 22), which shows the sector GWF reflected in production. The diagonal elements of the matrix F are produced directly to satisfy its final production and consumption, we used DGWFf for it. The other element of F is the indirect GWF (IGWF) produced by the use of intermediate products of other sectors by trade (Li et al., 2017). This is divided into two aspects: one is the IGWF on the strength of consumption (IGWFc), while the other is the IGWF on account of production (IGWFp). They are both achieved by subtracting DGWFf from GWFp or GWFc. The elements of the diagonal matrix E are indicated by eij, which represents the amount of the GWF when one sector’s output 10,000 RBM (Chinese currency abbreviation), this is also called the direct discharge intensity (22 × 1). (I-A)−1 is commonly referred to as the Leontief inverse matrix (22 × 22), and I is the identity matrix. Each element of matrix A, namely, aij, is called the direct requirement coefficient, which shows the amount of goods/services purchased from industry i to produce one unit of output in industry j. xij demonstrates the amount of goods/services sold by sector i to sector j.
To calculate the DGWF of the agriculture sectors, we adopted the method of Hoekstra et al. (2011). DDW represents the amount of fresh water dilution needed to assimilate the pollutant directly discharged by the sector to the standard concentration. PL is the pollution load. Cmax and Cnat represent the standard water quality concentration of the pollutant and the natural background concentration of the receiving water, respectively.
We further adopted four formulas to calculate the pollutant load of the non-point pollution (see Eqs 11, 12, 13, and 14 for details) (Ma et al., 2012).
where Epla,i,j, Eah,i,j, Efor,j, and Eaqu,j are the pollutant intensity coefficient of agriculture, animal husbandry, forestry, and fisheries, respectively; Lpla,i,j and Li are the fertilizer loss rate of agricultural land and fecal loss rate of animals, respectively; Dah,i and Daqu are the year-end stock of livestock and the yield of freshwater fisheries, respectively; Spla,i,j is the acreage of various agricultural crops; Ri is the fecal treatment rate; Vfor,j is the forest runoff; and PLpla,i,j, PLah,i,j, PLfor,j, and PLaqu,j represent the pollution load of agriculture, animal husbandry, forestry, and fisheries, respectively. Equations 12 and 13 were also used for the production services of agriculture, forestry, animal husbandry, and fisheries.
We employed Hua’s method to calculate the DGWFsec,i,j in the secondary industry (Hua et al., 2021) (see Eqs 15, 17), and Hoekstra’s method to calculate the DGWFter,i,j in the tertiary industry (Hoekstra et al., 2011) (see Eqs 16, 17).
where PLij is the discharge load of pollutant j in industrial i, Eter,i,j is the discharge coefficient of pollution j in tertiary industry i, and fei is the discharge of wastewater in the tertiary sector i.
We can then use Eq. 18 to quantify the trade situation outside Fujian Province to make up for the deficiency of the EIO-LCA model.
where Mi represents the final consumption of the value of the national export or import commodities/services (22 × 1), and the commodities/services of the domestic outflow or inflow, respectively (22 × 1). Thus, the GWFi shows the GWF of sector i outside Fujian Province.
To more accurately represent the outsourcing of pollution by region or sector, for the first time, we used net inflows to denote outsourced regions or sectors and net outflows to denote outsourced regions or sectors. According to Li et al. (2021), GWF outsourcing is from China’s coastal regions to water-scarce regions such as the north and the central part of the country; it is thus clear that GWF outsourcing from China’s coastal provinces is mainly domestic. The integrated consideration of net inflows and net outflows is more consistent with the reality of GWF outsourcing in China than the single consideration of net imports and net exports to quantify pollution outsourcing.
SDA is a widely used method that is based on using the input–output tables to determine the drivers of changes in water resource occupancy. Specifically, to further decompose Eq. 6 into several other influencing factors. We consider five influencing factors, so we only need to decompose F into three factors, which is described as follows:
Since the SDA method can only decompose the changes of the water footprint in 2 years, we stipulated that the start year is time 0 and the end year is time 1.
If there are n drivers, there are n! decomposition methods of SDA. The five drivers in our study have 5! = 120 decomposition results. To address the non-uniqueness issue of SDA, the weighted average partition method was employed (see Liang et al. (2016) for details). The following is a list of two drivers to illustrate the specific decomposition processing.
Here, Q is the result of the structural decomposition analysis of the GWF; P is the population; E shows the intensity of the sectoral GWF; L denotes the Leontief inverse matrix; FS refers to the consumption structure, and FL is the per capita demand; △E, △L, △FS, △FL, and △P represent the GWF changes in 2 years; and QE, QL, QFS, QFL, and QP are the decomposition results of each driver. The flowchart is shown in Figure 3.
The pollution source intensity coefficient of the primary industry was from the Emission Source Statistics Survey Production and Emission Accounting Method and Coefficient Manual in China (Ministry of Ecological Environment, 2021). The discharge coefficient of pollution in the tertiary industry was from Longyan Municipal People’s Government (Longyan Environmental Protection Bureau, 2019). The discharge volume of the secondary industry was calculated based on the total data provided by the Fujian Provincial Bureau of Statistics, 2002, 2007, 2012 (2017b) following the sector discharge structure of the National Bureau of Statistics of China (2013). In the remaining years (2002, 2017), following the ratio of pollution emissions to the added value, the quantity of wastewater effluent of the secondary and tertiary sectoral levels (S6-S22) was calculated from the water utilization structure of the China Economic Census Yearbook (2008). The total pollution load at the secondary industry was disaggregated into a sector level (S6–S16) based on the waste water discharge structure. In consideration for the evaluation of water quality in the water resources bulletin of Fujian and the data available, we selected the following indicators: chemical oxygen demand (COD), total nitrogen (TN), total phosphorus (TP), Copper (Cu), zinc (Zn), ammonia nitrogen (NH4+-N), and 5-day biological oxygen demand (BOD5). These are all closely related to the GWF embodied in production, consumption and trade. The pollutants concentration in water standard uses the surface water environment quality standard (GB3838-2002) in the Ⅲ class water quality standard, including COD,
Fujian’s total GWF increased from 138 billion m3 (Bm3) to 313 Bm3 from 2002 to 2012, then decreased to 217 Bm3 in 2017; it averaged 209 Bm3 during the study period (Figure 4). The changing trend of the DGWF was the same as that of the GWF (it first increased from 138 Bm3 in 2002 to 201 Bm3 in 2012, and then dropped to 144 Bm3 in 2017). Since both IGWFP based on the production side and IGWFc based on the consumption side are redistributions of the DGWF, the sum of IGWFp is equal to the total IGWFc. Our assessment shows that, except in 2002, when DGWFf dominated by 58.2% (about 1.4 times of IGWF) in the total GWF of that year, the IGWF dominated in all other years, with an average of 73.9%. Moreover, the GWF was larger than the DGWF in other years, about 1.5–2.1 times, apart from in 2002 (DGWF was equal to GWF). The sectoral trade has caused an increase in the IGWF. It is obvious that the DGWF alone cannot accurately describe the actual pollution status of the sector and the region. About 49.7% of the DGWF in 2002 came from the primary industry, but for the next 3 years, IGWFc dominated, an average of 42.5%. It means that most of the final products of the primary industry are consumed by other sectors as intermediate products. The secondary and tertiary industries were dominated by IGWFp, accounting for an average of 52.9% in the past 4 years, which indicates that the secondary and tertiary industries produce with sizeable raw material input from other sectors. In short, the primary industry is the main source of water pollution in Fujian Province, and the secondary and tertiary industries are the sectors that generate more indirect pollution. The top five departments in the IGWFp value in 2002 were “food and tobacco” (12.1 Bm3), “textiles, clothing, and shoes” (11.3 Bm3), “chemical products” (8.1 Bm3), “machinery and equipment manufacturing” (7.3 Bm3), and “other services” (4.3 Bm3). These were followed by “food and tobacco” (26 Bm3), “machinery and equipment manufacturing” (22.8 Bm3), “textiles, clothing, and shoes” (19.1 Bm3), “chemical products” (17.5 Bm3), and “construction” (9.5 Bm3) in 2007; “construction” (52.2 Bm3), “textile, clothing, and shoes” (35.7 Bm3), “machinery and equipment manufacturing” (32.4 Bm3), “food and tobacco” (29.7 Bm3), and “chemical products” (13.7 Bm3) in 2012; and “animal husbandry” (38.3 Bm3), “construction” (31 Bm3), “textiles, clothing, and shoes” (23 Bm3), “machinery and equipment manufacturing” (22.5 Bm3), and “chemical products” (17.5 Bm3) in 2017. The top five sectors by the IGWFc value in 2002 were “animal husbandry” (44.5 Bm3), “product services of agriculture, forestry, animal husbandry, and fisheries” (5.1 Bm3), “fishery” (2 Bm3), “wholesale and retail trade” (1.3 Bm3), and “transportation, warehousing, and postal services” (0.8 Bm3); 2007 for “animal husbandry” (94.4 Bm3), “product services of agriculture, forestry, animal husbandry, and fisheries” (13.4 Bm3), “wholesale and retail trade” (4.9 Bm3), “production and supply of electric power, heat, gas, and water” (3.3 Bm3), and “fishery” (2.6 Bm3); 2012 for “animal husbandry” (82.1 Bm3), “production and supply of electric power, heat, gas, and water” (51.6 Bm3), “mining products” (22.9 Bm3), “chemical products” (12 Bm3), and “nonmetal minerals products” (10 Bm3); and 2017 for “animal husbandry” (61.2 Bm3), “production and supply of electric power, heat, gas, and water” (37.3 Bm3), “product services of agriculture, forestry, animal husbandry, and fisheries” (27.5 Bm3), “mining products” (18.4 Bm3), and “other services” (11.8 Bm3). During these 4 years, the construction sector had the largest IGWFp. In these 4 years, the construction industry had the largest IGWFp while the animal husbandry industry had the largest IGWFc, meaning that the construction industry has a substantial intermediate input sector, and the indirect pollution is relatively large, while the animal husbandry industry is dominated by direct pollution.
FIGURE 4. Structure and characteristics of sectoral GWFs. S1, S2…S22 represents 22 sectors, and the letters (A–D) represent the years 2002, 2007, 2012, and 2017, respectively. To make the diagram clearer, only the even-numbered sectors are displayed. DGWF, DGWFf, IGWFp, and IGWFc, respectively, represent direct GWF (i.e., GWF of pollutants discharged to water bodies in Fujian Province), GWF consumed by final products, indirect GWF based on production and consumption.
Figure 5 shows that the virtual gray water/IGWF transferred through Fujian’s sectoral trade significantly intensified by 2012, as the volumes involved almost quadrupled, they jumped from 57.7 Bm3 in 2002 to 226.4 Bm3 in 2012 (292.2% increase). After that, it fell to 132.1 Bm3 in 2017 (41.6% drop). On the contrary, DGWFf decreased from 80.3 Bm3 in 2002 to 37.7 Bm3 in 2007 (53.1% drop), but after that, it showed rapid growth, reaching 85.3 Bm3 in 2017 (126.7% increase) (Figures 4, 5, Supplementary Table S1). DGWFf and IGWF are in a trade-off relationship.
FIGURE 5. Sectoral GWF flow (outsourcing/outsourced) from 2002 to 2017 (unit: Bm3). S1, S2…S22 represents 22 departments, (A–D) stand for 2002, 2007, 2012, and 2017. GWF that flows to its own represent the GWF consumed by the sector’s final product (DGWFf), except for it; GWF flows generally to its outsourcing sectors (this part of the GWF is also known as virtual gray water or IGWF). The width of the outer box represents the amount of the sectoral GWFs, and the thickness of the flow represents the amount of GWFs outsourcing or being outsourced.
As we noted in Introduction, the fine research of the primary industry subsectors is of great significance to determine the new sectors. “Animal husbandry” has been the key sector with the largest GWF, which was 188 Bm3 (68.1% of the total GWF), 140 Bm3 (42.1%), 125 Bm3 (20.1%), and 99.5 Bm3 (22.9%), respectively, from 2002 to 2017, and its GWF for the final product (DGWFf) was also high, with an average of 27.2% during the 4 years. In summary, the animal husbandry sector has been a hotspot sector of pollution emissions in Fujian Province during the study period.
We observed the top five sectors in terms of the virtual gray water/IGWF flow. They were S3–S7 (10 Bm3), S3–S8 (9.7 Bm3), S3–S11 (7.2 Bm3), S3–S14 (4 Bm3), and S3–S22 (3.2 Bm3) in 2002; S3–S7 (19.9 Bm3), S3–S11 (15.8 Bm3), S3–S14 (15.5 Bm3), S3–S8 (13.5 Bm3), and S3–S19 (6.9 Bm3) in 2007; S3–S7 (19.5 Bm3), S3–S8 (17.1 Bm3), S15–S16 (14.9 Bm3), S15–S14 (9.8 Bm3), and S3–S16 (9.2 Bm3) in 2012; S15–S16 (8 Bm3), S15–S14 (6 Bm3), S3–S16 (5.2 Bm3), S3–S7 (4.9 Bm3), and S6–S16 (4.9 Bm3) in 2017. S3–S7 (“animal husbandry” to “food and tobacco”) had the largest virtual gray water/IGWF flow during these 4 years. Before 2007, the flow of virtual gray water/IGWF mainly flowed from animal husbandry to other industries. After 2007, it mainly flowed from the construction industry to other industries.
As can be seen in Figure 5, the top five sectors of the virtual gray water/IGWF trade in 2002 were “animal husbandry” (69.8%, 93.4 Bm3), “production services of agriculture, forestry, animal husbandry, and fishery” (9.3%, 12.5 Bm3), “wholesale and retail trade” (5.5%, 7.4 Bm3), “transportation, warehousing, and postal services” (2.2%, 2.9 Bm3), and “fishery” (1.9%, 2.5 Bm3). In 2007, the top five were “animal husbandry” (74.5%, 47 Bm3), “production services of agriculture, forestry, animal husbandry, and fishery” (10.3%, 6.5 Bm3), “other services” (2.9%, 1.8 Bm3), “wholesale and retail trade” (2.4%, 1.5 Bm3), and “fishery” (2.2%, 1.4 Bm3). In 2012, the top five were “animal husbandry” (27.4%, 66.1 Bm3), “production and supply of electric power, heat, gas, water” (19.2%, 46.4 Bm3), “mining products” (11.5%, 27.8 Bm3), “chemical products” (7.6%, 18.5 Bm3), and “textiles, clothing, and shoes” (6.6%, 15.8 Bm3). In 2017, the top five were “production and supply of electric power, heat, gas, and water” (18.5%, 24.9 Bm3), “production services of agriculture, forestry, animal husbandry, and fishery” (17.9%, 24 Bm3), “mining products” (17.5%, 23.5 Bm3), “animal husbandry” (12.9%, 17.2 Bm3), and “chemical products” (5.8%, 7.8 Bm3). In summary, the largest sector for virtual gray water/IGWF trade has been the animal husbandry sector over the past 4 years, followed by the production and supply of electric power, heat, gas, and water sector. We found that the total IGWF transfer through trans-regional trade accounted for 45.8%, 80.4%, 77.1%, and 61.7% of the total in the four periods. IGWF increased from 63.1 Bm3 in 2002 to 241.2 Bm3 in 2012 (282% increased); then, it decreased to 134.1 Bm3 in 2017 (44.4% decreased) (Figure 6). Exports dominated in 2002, accounting for 33.3% (21.1 Bm3) of the total trans-regional trade, followed by domestic extra-provincial inflows, which rose from 28.4% in 2007 to 37.5% in 2017. Since 2012, domestic extra-provincial inflows and outflows have accounted for more than 50%. This means that the virtual gray water trade was dominated by the domestic market from 2012. Considering the net inflow and net outflow indicators of domestic extra-provincial inflows, outflows, import, and export trade, it can better reflect the current actual revenue and expenditure of the GWF.
FIGURE 6. Interregional virtual gray water/indirect GWF flow (outsourcing/outsourced) (unit: Bm3). S1, S2…S22 represents 22 departments, (A–D) represent 2002, 2007, 2012, and 2017.
If province A’s import plus domestic extra-provincial inflow has more GWF than its export plus domestic extra-provincial outflow through its sectoral trade, province A could be regarded as a net inflow province of GWF, meaning that province A outsources its GWF/water pollution to other regions. The same rule also applies to sector GWF outsourcing analysis. By 2012, Fujian Province being outsourced by other regions, produced an average of 11.4 Bm3 GWF flowing to other regions. However, in 2017, Fujian became an outsourcing province, outsourcing 12.5 Bm3 GWF to other regions (Figure 6). As shown in Figure 7A, sectoral GWF outsourcing varies greatly by sector and interannual variation. For example, as the largest being outsourced sector, S3 has been outsourced 7.1 Bm3 GWF annually from other regions, much larger than other sectors (2–351 times as much as other being outsourced sectors). Furthermore, the industry’s GWF varies considerably yearly, with 2012 (14.2 Bm3) more than 14 times higher than 2017 (1 Bm3). The outsourcing sector S22 (other services) outsourced an average of 0.5 Bm3 per year to other regions, but in 2012, the volume was 20 times more than in 2017. In 2002, only five of the 22 sectors outsourced water pollution to other regions, compared to seven in 2007, nine in 2012, and 14 in 2017. Also worth noting is that the number of outsourcing sectors is increasing. Specifically, in 2012 and the first 2 years, the primary industry was being an outsourced industry. In 2017, except for animal husbandry and fishery, other primary industries transformed to outsourcing industries, of which S5 (9.9 Bm3) became the second largest outsourcing sector that year (Figure 7A).
FIGURE 7. GWF outsourcing among 22 sectors during 2002, 2007, 2012, and 2017. S1, S2…S22 represents 22 departments. A positive value indicates the outsourcing sector, and a negative value indicates being outsourced by other sectors (A). IGWFp and IGWFc, respectively, represent the indirect GWF based on production and consumption (B). (B1–B4) indicate 2002, 2007, 2012, and 2017 (B).
We can also gain insights into sectoral pollution outsourcing at the production and consumption ends by comparing production-based and consumption-based indirect GWF, which helps propose pollution control measures from both production and consumption sides, and by further analysis of IGWFp and IGWFc, it can be seen that pollution outsourcing exists in specific aspects of production and consumption in the sector. For example, if the IGWFp of a sector is greater than IGWFc, it means that the sector is outsourcing a large amount of pollution on the production side, that is, other sectors provide a large number of raw materials in the production process of the sector, which is equivalent to the transfer of pollution from the sector to other sectors; conversely, if IGWFc is greater than IGWFp, it means that the sector has outsourced a large amount of pollution on the consumption side, that is, the final products are consumed by other sectors, which is equivalent to the pollution from other sectors being transferred to that sector.
Figure 7B shows that the pollution outsourcing of the primary industry is mainly concentrated in the consumption link, that is, there is pollution at the consumption end, which belongs to the being polluted outsourced sector. The pollution outsourcing of the secondary and tertiary industries is mainly concentrated in the production link, that is, there is pollution outsourcing at the production end, which belongs to the pollution outsourcing sector. During the research period, the sector with the largest proportion of pollution outsourcing on the production side was the construction sector, and the sector with the largest proportion of pollution outsourcing on the consumer side was S3 before 2012 and S6 in 2017, respectively.
Figure 8A demonstrates that the GWF in Fujian Province increased by 20.7% from 2002 to 2007, and it then remained higher from 2007 to 2012, when it increased by 87.8%. This was followed by a downward trend of 30.5% between 2012 and 2017. The increase in the Fujian’s GWF from 2002 to 2017 mainly resulted from the increased final demand per capita (QFL), while the direct GWF intensity (QE) was the key factor that drove the decrease in the Fujian’s GWF, except for 2007 to 2012. We found that the population (QP) mainly contributed to the increase in the GWF, but its influence was small. The influence of the industry structure (QL) and consumption structure (QFS) on the GWF changed over time. The final demand per capita enlarged the GWF by 180.6 Bm3 (6.3 times the net increase of 28.6 Bm3) from 2002 to 2007, by 134 Bm3 (0.9 times the net increase of 146.2 Bm3) from 2007 to 2012, and by 111.7 Bm3 (1.2 times the net decrease of 95.3 Bm3) from 2012 to 2017. The secondary industry made the largest contribution to the change of GWF, followed by the tertiary industry and the primary industry (Figure 8B).
FIGURE 8. Changes in the annual GWF (A), the three major industries (B), and the sectoral GWF (C) in 2002, 2007, 2012, and 2017, (C1) (2002–2007), (C2) (2007–2012), and (C3) (2012–2017). Contributions of five driving factors: QE, direct GWF intensity; QL, industry structure; QFS, the consumption structure, QFL, final demand per capita; and QP, population.
At the sectoral level, QFL and QP made the largest contribution to the increase in the sectoral GWF, and the contribution varied in different industries (Figure 8C). The sector most affected by the five drivers was “construction,” and was followed by “machinery and equipment manufacturing,” and “other services,” whereas the least affected sector was forestry. The contribution of QFL to various departments varied greatly. For instance, QFL’s contribution to “machinery and equipment manufacturing” declined from 25% in 2002–2007 to 8% in 2007–2012, whereas it rose rapidly to 21% in 2012–2017. QFL’s contribution to “construction” rose from 12% in 2002–2007 to 37% in 2007–2012, and then declined to 12% in 2012–2017. Except for “construction,” QP’s contribution to other sectors has decreased over time. QE and QL became the main driving forces of the sectoral GWF reduction in 2017.
The total GWFs of Fujian in 2002, 2007, 2012, and 2017 were 138.01 Bm3, 166.56 Bm3, 312.79 Bm3, 217.45 Bm3, respectively; the value of the sectoral GWF increased rapidly before 2012 and then decreased slowly. We argue that this has a lot to do with the rapid economic development before 2012, and the policy of the State Council on the implementation of the strictest water resources management system (State Council of China, 2012) after 2012. Moreover, our literature review shows that our results match well with previous research. For instance, Liu et al. (2020) estimated that the GWF of Fujian increased during 2002–2012. Li et al. (2020) found that the GWF of Fujian increased during 2006–2012, and decreased during 2012–2017. However, the total GWF obtained in this study was bigger than that of other researchers. For example, the GWF in Fujian in 2012 was calculated by us to be 312.79 Bm3, which is about 2.6 and 3.9 times bigger than the estimate of about 120 Bm3 (traditional method) and 80 Bm3 (considering the combined effects of the multiple pollutants method) in the Li et al. (2021) study and about 1.6 times larger than the 190 Bm3 of Li et al. (2020). This is mainly due to the different accounting models, the choice of data and indicators, and the different research focus. In Li et al. (2020), they used traditional GWF accounting methods and only studied the direct GWF from production. In Li et al. (2021), they considered the influence of various pollutants on the GWF, which cut the total GWF by one-third. Both Li et al. (2020) and Li et al. (2021) did not take into account the indirect GWF caused by sectoral trade in one region, which lowered their accounting results. Hence, it makes sense that our results are bigger than theirs. In addition, our study also shows that the IGWF of sectors in Fujian Province is very large (accounts for 65.9% of the total GWF), and IGWF further promotes pollution outsourcing, which deserves attention and further analysis.
Figure 4 shows that the Fujian’s GWF is mainly distributed in the primary industry, and this is consistent with the findings of Liao et al. (2019). Thus, the refinement of the primary industry subsector facilitates the identification and analysis of the key sectors that influence changes in the GWF. We found that the animal husbandry sector (S3) of the primary industry was the largest outsourced sector (Li et al., 2021), being outsourced an average of 7 Bm3 GWF to other regions annually (Figure 7A). Therefore, the animal husbandry sector was one of the hotspot sectors of the Fujian’s GWF. This is different from the study in the northern region (Zhao et al., 2021b). The most polluting sector in Fujian Province is not agriculture (Figures 4, 7B), which may be related to the small area of plains and the large scale of rice cultivation in the region (the production of this crop requires a lot of labor and water resources). We also found that the GWF outsourced between sectors are becoming more extensive (Figure 5), and, building on Li et al. (2017) and Zhao et al. (2019), we further propose that the direction of GWF flow is the opposite to that of GWF outsourcing/pollution outsourcing. For instance, the construction sector in 2017 as examples, the DGWF, DGWFf, and IGWFP of construction were 0.88 Bm3, 0.86 Bm3, and 40 Bm3, respectively. The construction sector produced 48.6 GWF (DGWFf + IGWFp), but the final product consumed only 2.1%; 2.2% was discharged into the water bodies of Fujian Province, and 97.9% of the GWF came from indirect inputs from other sectors (Figure 4). A large volume of the IGWF from other sectors is used as a raw material for the construction sector (Figure 5), which discharges a large amount of water pollution in other sectors. This is equivalent to outsourcing the pollution of construction to other departments (Zhao et al., 2019). From Figure 5, we can confirm our idea that almost all of the IGWF flow to construction little outflow. Conversely, animal husbandry sector is outsourced to other sectors, a large amount of GWF flows from animal husbandry to other departments (Figure 5), that is, a lot of water pollution flows into animal husbandry, which will inevitably increase the pollution responsibility of animal husbandry.
With excellent market allocation and more and more trade in intermediate goods/services, water pollution outsourcing in domestic sectors is becoming more common (Li et al., 2021). Physical water environment monitoring alone cannot track the transfer of water pollution from the supply chain, let alone quantify the pollution responsibility of upstream and downstream departments. By studying the flow and composition of the GWF, clarifying pollution outsourcing departments and outsourcing links, and sorting out the specific pollution responsibilities of upstream and downstream departments, it is of great significance to control the growth of the GWF. For example, we find that the agriculture sector, food and tobacco sector, textile, clothing and shoes sector, and construction sector should take additional pollution responsible for their upstream sector because these sectors use a lot of upstream products and services in their production processes (IGWFp > IGWFc) (Figure 7B), reducing the pollution of its own sector; however, it has aggravated the direct pollution of the upstream sector. Taking the construction industry as an example, it can be seen from Figure 5 that the top three industries in the upstream sector of the construction industry are S15, S3, and S6, respectively, and the DGWF of these three industries has always been higher than that of the construction industry. That is to say, to meet the production input of the construction industry, these three sectors cause more pollution to the local water bodies. To sum up, sectors with net GWF inflow and IGWFp greater than IGWFc are outsourcing sectors; and sectors with net GWF outflow and IGWFp smaller than IGWFc are outsourced sectors. GWF’s being an outsourced sector will increase the sector’s local discharge of water pollution, while GWF’s outsourced sector will reduce the sector’s water pollution discharge. Pollution outsourcing is a double-edged sword for the water environment (Mao et al., 2020). The “polluter pays” model must be changed (Wang et al., 2021).
Only in 2017 did Fujian Province become a net inflow region (12.6 Bm3), outsourcing its GWF to other regions. Before 2012, it was being an outsourced area, with an average of 11.4 Bm3 GWF being outsourced to other departments. Our observations align with Wu and Ye (2020), who argue that Fujian was a being outsourced province in 2012. In addition to policy factors (State Council of China, 2012), this is also the main reason for the downward adjustment of GWF in Fujian Province since 2012. Again, this is the root cause of the serious quality-induced water shortage (Ma et al., 2020; Yu et al., 2020) and water resources utilization pressure (Niva et al., 2020) in the province. However, the study of Li et al. (2021) showed that Fujian Province was a net inflow area of the GWF in 2012. Both Wu and Li used the import and export data to measure the flow of the GWF, but the results were completely opposite. This calls for a new index to measure the GWF flow to achieve sustainable water resources management. When the net imports are no longer a good measure of the impact of trade on the domestic economy (WTO et al., 2019), the net inflow/outflow will better display the region’s economy and water use. For example, the net outflow sector of the GWF means that pollution is outsourced, so these sectors should be hotspots for reducing water pollution; while the net inflow sector of the GWF means that the sector has outsourced its pollution. With Fujian’s sectoral GWF turning to the domestic market, considering the net inflow/outflow sectors of the GWF will become a hotspot for water resources management.
Figure 8A highlights the dominant role of the GWF change. The increase in per capita demand leads to the increase in the GWF, which shows that Fujian Province is still in a period of rapid economic development. Rapid urbanization and an increase in per capita income make people consume more types of products (Zhao et al., 2021a) and produce more GWF, which thereby aggravates the water pollution situation in Fujian Province. Given this, we advocate adjusting the consumption structure, using low-water consumption, low-intensity products, encouraging the purchase of old commodities, and promoting the recycling of goods (Liu et al., 2022).
Another interesting finding is that with the increase in the GWF intensity in Fujian Province, the technology (QE) effect is a significantly positive factor promoting the increase in the GWF in the province (2007–2012), and with the decrease in the GWF intensity (2002–2007, 2012–2017), the technology (QE) effect becomes a negative factor (Figure 8A). This shows that it is necessary to strengthen the technical input and management of the water resources in Fujian Province. The estimates of Zhang et al. (2019) are consistent with ours, which means technology has little effect on the reduction of the GWF in Fujian, and it is necessary to strengthen technological improvement. The reason for this phenomenon may probably be because they have better water endowments and no incentive or pressure to innovate.
We also found that the five driving factors had a greater contribution to all sectors of the secondary industry, and a smaller contribution to the primary industry with a larger GWF (Figure 8B). On the one hand, the interannual GWF does not change much due to the relatively stable agricultural production, whereas the secondary industry changes greatly with the market, and the type and amount of sewage discharge vary greatly from year to year. On the other hand, it is also the result of the rapid urbanization in Fujian Province. More and more rural people have moved to cities, which increases the demand for housing and stimulates the development of related industries such as construction; this leads to the rapid development of GWF emissions in these sectors. In addition, GWF outsourcing is also a major contributor to changes in the GWF.
(1) Departments with IGWFp > IGWFc are mostly downstream sectors, and with GWFc > IGWFp are mostly upstream sectors of the supply chain. GWF outsourcing intensifies the water pressure and pollution responsibility of being outsourced sectors (upstream sectors). Clarifying the pollution responsibility of the upstream and downstream sectors and encouraging the sharing of responsibilities for pollution are pivotal measures to reduce the upstream sectoral GWF and water pressure in Fujian Province.
(2) Domestic trade is the main market of the GWF flow in Fujian Province. Considering the net inflow/outflow sectors of the GWF will become a hotspot for water resources management.
(3) The changes of the GWF were mainly influenced by the per capita final demand (QFL). To adjust the consumption structure, using low-GWF consumption low-GWF intensity products and promoting moderate consumption are critical to reduce the GWF and promote sustainability in Fujian Province.
(4) According to the different links and types of pollution in each department, from the perspective of the entire supply chain, corresponding departmental water pollution countermeasures should be formulated. For instance, from the perspective of direct sewage discharge (DGWF) and GWF outsourcing, controlling the direct sewage discharge of “animal husbandry should be focused on”; from the production perspective (IGWFp), a pollution liability tax on “construction” and “machinery and equipment manufacturing” should be imposed to limit the increase in pollution in their upstream sectors; from the consumption (IGWFc), the water consumption of “production and supply of electric power, heat, gas, and water” and “mining products” should be limited. Investment in science and technology should be increased to improve water efficiency.
This article provides a preliminary discussion of the redistribution and transfer of the direct and indirect GWF/virtual gray water based on the EIO-LCA model, but there are still some limitations. First, although the subsector study of the primary industry can more accurately locate its current pollution sectors, due to a lack of data on the product services of agriculture, forestry, animal husbandry, and fisheries industry, we only used the breeding services of animal husbandry and fisheries to replace the data of the whole sector, which will undoubtedly reduce the GWF of this sector. Second, due to the lack of sectoral water consumption data, we assumed that the sectoral water consumption structure of Fujian Province was the same as that of China, and the pollutant load contained in unit sewage in the same sector is the same. On account of the limitations of the EIO-LCA model, pollution outsourcing departments cannot be associated with specific regions, but we can judge whether there is outsourcing in the departments. Next, we will adopt a multi-region input–output model to help reduce uncertainty.
The original contributions presented in the study are included in the article/Supplementary Material, further inquiries can be directed to the corresponding author.
FY: methodology, data curation, software, visualization, writing—original draft, and writing—review and editing; CZ: methodology and writing—review and editing; YW: conceptualization, supervision, investigation, and writing—review and editing; LZ, JJ, HD, and HL: writing—review and editing; RS: software and writing—review and editing; YH: visualization and editing; JY: visualization and editing; and PJ: visualization and editing.
This work was funded by the Key Research Funds for Fujian Province Public-interest Scientific Institution in China (Grant 2019R1002-7), Social Science Foundation of Fujian Province in China (FJ2021B042), and Key Project of Natural Science Foundation of Fujian Province in China (2021J02030). Additional support was provided by the Fujian Provincial Natural Science Foundation project in China (2019J05068).
The authors declare that the research was conducted in the absence of any commercial or financial relationships that could be construed as a potential conflict of interest.
All claims expressed in this article are solely those of the authors and do not necessarily represent those of their affiliated organizations, or those of the publisher, the editors, and the reviewers. Any product that may be evaluated in this article, or claim that may be made by its manufacturer, is not guaranteed or endorsed by the publisher.
The Supplementary Material for this article can be found online at: https://www.frontiersin.org/articles/10.3389/fenvs.2022.864346/full#supplementary-material
An, L., Li, X. X., and Yang, Z. X. (2018). Research on Chinese Inter-year Dynamic Input-Output Model. Stat. Decis. 24, 5–9. doi:10.13546/j.cnki.tjyjc.2018.24.001
Blackhurst, B. M., Hendrickson, C., and Vidal, J. S. i. (2010). Direct and Indirect Water Withdrawals for U.S. Industrial Sectors. Environ. Sci. Technol. 44, 2126–2130. doi:10.1021/es903147k
Cai, B., Hubacek, K., Feng, K., Zhang, W., Wang, F., and Liu, Y. (2020). Tension of Agricultural Land and Water Use in China's Trade: Tele-Connections, Hidden Drivers and Potential Solutions. Environ. Sci. Technol. 54, 5365–5375. doi:10.1021/acs.est.0c00256
Cazcarro, I., Duarte, R., and Sánchez-Chóliz, J. (2016). Downscaling the Grey Water Footprints of Production and Consumption. J. Clean. Prod. 132, 171–183. doi:10.1016/j.jclepro.2015.07.113
Deng, G., Lu, F., Wu, L., and Xu, C. (2021). Social Network Analysis of Virtual Water Trade Among Major Countries in the World. Sci. Total Environ. 753, 142043. doi:10.1016/j.scitotenv.2020.142043
Dolan, F., Lamontagne, J., Link, R., Hejazi, M., Reed, P., and Edmonds, J. (2021). Evaluating the Economic Impact of Water Scarcity in a Changing World. Nat. Commun. 12, 1915. doi:10.1038/s41467-021-22194-0
Egilmez, G., and Park, Y. S. (2014). Transportation Related Carbon, Energy and Water Footprint Analysis of U.S. Manufacturing: An Eco-Efficiency Assessment. Transport. Res. D Transport Environ. 32, 143–159. doi:10.1016/j.trd.2014.07.001
Elahi, E., Khalid, Z., Tauni, M. Z., Zhang, H., and Lirong, X. (2021). Extreme Weather Events Risk to Crop-Production and the Adaptation of Innovative Management Strategies to Mitigate the Risk: A Retrospective Survey of Rural Punjab, Pakistan. Technovation 4, 102255. doi:10.1016/j.technovation.2021.102255
Elahi, E., Zhang, Z., Khalid, Z., Xu, H., and Lirong, X. (2022a). Application of an Artificial Neural Network to Optimise Energy Inputs: An Energy- and Cost-Saving Strategy for Commercial Poultry Farms. Energy 244, 123169. doi:10.1016/j.energy.2022.123169
Elahi, E., Khalid, Z., and Zhang, Z. (2022b). Understanding Farmers' Intention and Willingness to Install Renewable Energy Technology: A Solution to Reduce the Environmental Emissions of Agriculture. Appl. Energ. 309, 118459. doi:10.1016/j.apenergy.2021.118459
Fu, T., Xu, C., Yang, L., Hou, S., and Xia, Q. (2022). Measurement and Driving Factors of Grey Water Footprint Efficiency in Yangtze River Basin. Sci. Total Environ. 802, 149587. doi:10.1016/j.scitotenv.2021.149587
Fujian Provincial Bureau of Statistics, 2002, 2007, 2012 (2017a). Fujian Province Input-Output Table. Fuzhou: Fujian Provincial Bureau of Statistics Official Website. Available at: https://tjj.fujian.gov.cn/gzcy/.
Fujian Provincial Bureau of Statistics, 2002, 2007, 2012 (2017b). Fujian Statistical Yearbook. Fuzhou: Fujian Provincial Bureau of Statistics Official Website. Available at: https://tjj.fujian.gov.cn/xxgk/ndsj/.
Han, M. Y., Chen, G. Q., Mustafa, M. T., Hayat, T., Shao, L., Li, J. S., et al. (2015). Embodied Water for Urban Economy: A Three-Scale Input-Output Analysis for Beijing 2010. Ecol. Model. 318, 19–25. doi:10.1016/j.ecolmodel.2015.05.024
Hendrickson, C., Horvath, A., Joshi, S., and Lave, L. (1998). Peer Reviewed: Economic Input-Output Models for Environmental Life-Cycle Assessment. Environ. Sci. Technol. 32, 184A–191A. doi:10.1021/es983471i
Hoekstra, A. Y., and Hung, P. Q. (2005). Globalisation of Water Resources: International Virtual Water Flows in Relation to Crop Trade. Glob. Environ. Change 15, 45–56. doi:10.1016/j.gloenvcha.2004.06.004
Hoekstra, A. Y., Chapagain, A. K., Aldaya, M. M., and Mekonnen, M. M. (2011). The Water Footprint Assessment Manual. Padstow: TJ International Ltd.
Hua, E., Wang, X., Engel, B. A., Qian, H., Sun, S., and Wang, Y. (2021). Water Competition Mechanism of Food and Energy Industries in WEF Nexus: A Case Study in China. Agric. Water Manage. 254, 106941. doi:10.1016/j.agwat.2021.106941
Huang, K., Wang, M., Zhou, Z., Yu, Y., and Bi, Y. (2021). A Decoupling Analysis of the Crop Water Footprint versus Economic Growth in Beijing, China. Front. Environ. Sci. 9, 807946. doi:10.3389/fenvs.2021.807946
Lenzen, M., Moran, D., Bhaduri, A., Kanemoto, K., Bekchanov, M., Geschke, A., et al. (2013). International Trade of Scarce Water. Ecol. Econ. 94, 78–85. doi:10.1016/j.ecolecon.2013.06.018
Li, H., Yang, Z., Liu, G., Casazza, M., and Yin, X. (2017). Analyzing Virtual Water Pollution Transfer Embodied in Economic Activities Based on gray Water Footprint: A Case Study. J. Clean. Prod. 161, 1064–1073. doi:10.1016/j.jclepro.2017.05.155
Li, S. N., Wang, Y., Luo, J., Jiang, P. P., and Chen, H. Y. (2020). Spatio-temporal Variations and Driving Factors of Grey Water Footprint in Fujian Province. Acta Eco. Sin. 40 (21), 7952–7965. doi:10.5846/stxb201910292274
Li, H., Liang, S., Liang, Y., Li, K., Qi, J., Yang, X., et al. (2021). Multi-pollutant Based Grey Water Footprint of Chinese Regions. Resour. Conserv. Recycling 164, 105202. doi:10.1016/j.resconrec.2020.105202
Liang, S., Feng, T., Qu, S., Chiu, A. S. F., Jia, X., and Xu, M. (2016). Developing the Chinese Environmentally Extended Input-Output (CEEIO) Database. J. Ind. Ecol. 21 (4), 953–965. doi:10.1111/jiec.12477
Liao, X., Chai, L., Xu, X., Lu, Q., and Ji, J. (2019). Grey Water Footprint and Interprovincial Virtual Grey Water Transfers for China's Final Electricity Demands. J. Clean. Prod. 227, 111–118. doi:10.1016/j.jclepro.2019.04.179
Liu, J., Zhao, D., Mao, G., Cui, W., Chen, H., and Yang, H. (2020). Environmental Sustainability of Water Footprint in Mainland China. Geogr. Sustain. 1, 8–17. doi:10.1016/j.geosus.2020.02.002
Liu, X., Lu, X., Feng, Y., Zhang, L., and Yuan, Z. (2022). Recycled WEEE Plastics in China: Generation Trend and Environmental Impacts. Resour. Conserv. Recycling 177, 105978. doi:10.1016/j.resconrec.2021.105978
Long, A., Yu, J., He, X., Deng, X., Su, S., Zhang, J., et al. (2021). Linking Local Water Consumption in Inland Arid Regions with Imported Virtual Water: Approaches, Application and Actuators. Adv. Water Resour. 151, 103906. doi:10.1016/j.advwatres.2021.103906
Longyan Environmental Protection Bureau (2019). Table of Discharge Coefficient of Tertiary Industry in Fujian Province. Longyan: Longyan Municipal People's Government. Available at: https://xxgk.longyan.gov.cn/hjbhj/gkml/22/201810/t20181012_1414089.htm (Accessed October 12, 2004).
Ma, G. X., Fang, Y., Cao, D., and Niu, K. Y. (2012). Calculation of Agricultural Non-point Source Pollution Emission in China and its Long-Term Forecast. Acta Sci. Circumutantiae 32 (2), 489–497. doi:10.13671/j.hjkxxb.2012.02.006
Ma, T., Sun, S., Fu, G., Hall, J. W., Ni, Y., He, L., et al. (2020). Pollution Exacerbates China's Water Scarcity and its Regional Inequality. Nat. Commun. 11, 650. doi:10.1038/s41467-020-14532-5
Mao, X., Luan, X., and Huang, X. (2020). How Does Inter-sectoral Linkage Affect the Environmental Performance of a Transitioning Region: Evidence from the Yangtze River Economic Belt, China. Resour. Environ. Sustain. 1, 100002. doi:10.1016/j.resenv.2020.100002
Mekonnen, M. M., and Hoekstra, A. Y. (2020). Blue Water Footprint Linked to National Consumption and International Trade Is Unsustainable. Nat. Food 1, 792–800. doi:10.1038/s43016-020-00198-1
Ministry of Ecological Environment (2021). Emission Source Statistics Survey Production and Emission Accounting Method and Coefficient Manual. Beijing: Ministry of Ecology and Environment of the People's Republic of China Publishing Website. Available at: https://www.mee.gov.cn/xxgk2018/xxgk/xxgk01/202106/t20210618_839512.html (Accessed June 11, 2021).
National Bureau of Statistics of China (2013). Fujian Enterprise Yearbook. Beijing: China Statistics Press.
Niva, V., Cai, J., Taka, M., Kummu, M., and Varis, O. (2020). China's Sustainable Water-Energy-Food Nexus by 2030: Impacts of Urbanization on Sectoral Water Demand. J. Clean. Prod. 251, 119755. doi:10.1016/j.jclepro.2019.119755
Okadera, T., Geng, Y., Fujita, T., Dong, H., Liu, Z., Yoshida, N., et al. (2015). Evaluating the Water Footprint of the Energy Supply of Liaoning Province, China: A Regional Input-Output Analysis Approach. Energy Policy 78, 148–157. doi:10.1016/j.enpol.2014.12.029
State Council of China (2012). Opinions of the State Council on Implementing the Strictest Water Resource Management System (Beijing: The Central People’s Government of the People’s Republic of China). Available at: http://www.gov.cn/zhuanti/2015-06/13/content_2878992.htm (Accessed February 15, 2012).
Sun, L., Cai, Y., Chen, A., Zamora, D., and Jaramillo, F. (2021). Water Footprint and Consumption of Hydropower from basin-constrained Water Mass Balance. Adv. Water Resour. 153, 103947. doi:10.1016/j.advwatres.2021.103947
UNFAOUNDPUNIDOUNESCOUN-WaterUN-HABITATet al. (2021). Valuing Water. Pairs: UNESCO Publishing Website. Available at: https://digitallibrary.un.org/record/3905489.
Wang, Y., Yuan, Z., and Tang, Y. (2021). Enhancing Food Security and Environmental Sustainability: A Critical Review of Food Loss and Waste Management. Resour. Environ. Sustain. 4, 100023. doi:10.1016/j.resenv.2021.100023
WTOIDE-JETROOECDUIBEWord Bank Group (2019). Technological Innovation, Supply Chain Trade, and Workers in a Globalized World, Global Value Chain Development Report 2019. Geneva: WTO Publishing Website. Available at: https://www.wto.org/english/res_e/booksp_e/gvc_dev_report_2019_e.pdf.
Wu, Z., and Ye, Q. (2020). Water Pollution Loads and Shifting within China's Inter-province Trade. J. Clean. Prod. 259, 120879. doi:10.1016/j.jclepro.2020.120879
Yang, L., Wang, Y., Wang, R., Klemeš, J. J., Almeida, C. M. V. B. d., Jin, M., et al. (2020). Environmental-social-economic Footprints of Consumption and Trade in the Asia-Pacific Region. Nat. Commun. 11, 4490. doi:10.1038/s41467-020-18338-3
Yu, C., Yin, X., Li, H., and Yang, Z. (2020). A Hybrid Water-Quality-index and Grey Water Footprint Assessment Approach for Comprehensively Evaluating Water Resources Utilization Considering Multiple Pollutants. J. Clean. Prod. 248, 119225. doi:10.1016/j.jclepro.2019.119225
Zhang, C., and Anadon, L. D. (2014). A Multi-Regional Input-Output Analysis of Domestic Virtual Water Trade and Provincial Water Footprint in China. Ecol. Econ. 100, 159–172. doi:10.1016/j.ecolecon.2014.02.006
Zhang, L., Dong, H., Geng, Y., and Francisco, M.-J. (2019). China's Provincial Grey Water Footprint Characteristic and Driving Forces. Sci. Total Environ. 677, 427–435. doi:10.1016/j.scitotenv.2019.04.318
Zhao, X., Liu, J., Liu, Q., Tillotson, M. R., Guan, D., and Hubacek, K. (2015). Physical and Virtual Water Transfers for Regional Water Stress Alleviation in China. Proc. Natl. Acad. Sci. U.S.A. 112, 1031–1035. doi:10.1073/pnas.1404130112
Zhao, X., Liao, X., Chen, B., Tillotson, M. R., Guo, W., and Li, Y. (2019). Accounting Global Grey Water Footprint from Both Consumption and Production Perspectives. J. Clean. Prod. 225, 963–971. doi:10.1016/j.jclepro.2019.04.037
Zhao, D., Liu, J., Sun, L., Ye, B., Hubacek, K., Feng, K., et al. (2021a). Quantifying Economic-Social-Environmental Trade-Offs and Synergies of Water-Supply Constraints: An Application to the Capital Region of China. Water Res. 195, 116986. doi:10.1016/j.watres.2021.116986
Keywords: trade, gray water footprint, virtual gray water, economic input–output life cycle, structural decomposition analysis
Citation: Yu F, Zhang C, Wang Y, Zhu L, Jin J, Deng H, Ling H, Shi R, He Y, Yu J and Jiang P (2022) Analysis of Gray Water Footprint Changes and Driving Forces Caused by Sectoral Trade in Southeast China. Front. Environ. Sci. 10:864346. doi: 10.3389/fenvs.2022.864346
Received: 28 January 2022; Accepted: 11 April 2022;
Published: 11 May 2022.
Edited by:
Anik Bhaduri, Griffith University, AustraliaCopyright © 2022 Yu, Zhang, Wang, Zhu, Jin, Deng, Ling, Shi, He, Yu and Jiang. This is an open-access article distributed under the terms of the Creative Commons Attribution License (CC BY). The use, distribution or reproduction in other forums is permitted, provided the original author(s) and the copyright owner(s) are credited and that the original publication in this journal is cited, in accordance with accepted academic practice. No use, distribution or reproduction is permitted which does not comply with these terms.
*Correspondence: Yuan Wang, eS53YW5nQGZqbnUuZWR1LmNu, eXdhbmdAbmp1LmVkdS5jbg==
†These authors share first authorship
Disclaimer: All claims expressed in this article are solely those of the authors and do not necessarily represent those of their affiliated organizations, or those of the publisher, the editors and the reviewers. Any product that may be evaluated in this article or claim that may be made by its manufacturer is not guaranteed or endorsed by the publisher.
Research integrity at Frontiers
Learn more about the work of our research integrity team to safeguard the quality of each article we publish.