- 1SAMRC Microbial Water Quality Monitoring Centre, University of Fort Hare, Alice, South Africa
- 2Applied and Environmental Microbiology Research Group, Department of Biochemistry and Microbiology, University of Fort Hare, Alice, South Africa
- 3Department of Microbiology and Biotechnology, Western Delta University, Oghara, Nigeria
- 4Department of Environmental Health Sciences, University of Sharjah, Sharjah, United Arab Emirates
The preharvest environment provides a suitable platform for the emergence and dissemination of multidrug resistant pathogenic bacteria. This could be detrimental to public health and may lead to fresh produce related disease outbreaks when transferred to the food web. This study evaluated the occurrence and antibiogram fingerprints of the pathogenic strains of Escherichia coli (E. coli) recovered from irrigation water and agricultural soil samples in fresh produce farms in the Eastern Cape Province, South Africa. E. coli was isolated, pathotyped and screened for antibiogram fingerprints using standard cultural and molecular methods. An antibiogram-based K-means cluster analysis and neighbour-joining dendrogram was used to elucidate the relatedness of the isolates. Of the confirmed isolates, 37% harboured at least one of the screened virulence genes (VGs) that encode for intestinal and extraintestinal E. coli pathovars. The most prevalent pathovar/associated VG in all the samples was Enterohemorrhagic E. coli/stx2. Of all the antimicrobials tested, E. coli pathovars conferred the highest resistance against ampicillin with a median single antimicrobial resistance (AMR) index of 0.23 (IQR: 0.10–0.38, 95% CI: 0.16–0.27) for pathovars from irrigation water and 0.01 (IQR: 0.01–0.03, 95% CI: 0.01–0.03) for pathovars from agricultural soil. About 60 and 20% of the pathovars from irrigation water and agricultural soil were multi drug resistant with a median multiple AMR index of 0.41 (IQR: 0.25–0.52, 95% CI: 0.31–0.47) and 0.38 (IQR: 0.23–0.66, 95% CI: 0.19–0.69) respectively. The most prevalent resistance genes detected were sulI, catII, tetA and AmpC across all sample types. The blaTEM was the most prevalent extended-spectrum β-lactamase resistance gene detected. A positive correlation between the phenotypic and genotypic AMR profiles of the pathovars in irrigation water samples, r = 0.6, p ≤ 0.01 and agricultural soil samples, r = 0.8, p ≤ 0.01 was recorded. The antibiogram based dendrogram generated 11 and 7 clades from irrigation water and agricultural soil samples respectively, indicating that the isolates are highly diversified. The findings revealed the occurrence of highly diversified multidrug resistant E. coli pathovars in the preharvest environment, indicating potential public health threats.
Introduction
Antimicrobial resistance (AMR) is a multifaceted global health problem that increases hospitalization time, treatment costs, morbidity and mortality. Its containment requires a multi-pronged approach which involves the use of the One Health concept. This concept indicates the interrelatedness between the human, animal, food and environmental health spheres, and fosters collaborative and multisectoral efforts to tackle the challenge (Taneja and Sharma, 2019). Sadly, AMR engendered from the environmental sphere has received less attention compared to that from the human and animal health spheres despite the significant variation in the geographical spread of AMR contributed by the environment (O ’Neill, 2015).
The environment serves as a key reservoir of antimicrobial resistance genes (ARGs) which are transferable to clinically significant pathogenic bacteria. In terms of the uptake of the novel resistance determinants, the varying ecological niches of the environment provides a highly diversified gene pool that is unmatched to that of the human and animal spheres (Schulz et al., 2017). Of all the environmental niches, the agricultural ecosystem is more complex considering the various activities that influence the tenacity and spread of AMR within the system (Mafiz et al., 2018). The release of wastewater treatment plant (WWTP) effluents to surface water bodies typically used for recreational, domestic and irrigation purposes; the application of bio-solids and organic manure to agricultural soil; animal intrusion on farmlands and water bodies; and the use of reclaimed wastewater for irrigation purposes have led to the expansion of AMR within and beyond the agroecosystem (Iwu et al., 2020). Because fresh produce is almost always in contact with irrigation water and agricultural soil during the preharvest agricultural production, these environmental niches, therefore, serve as key transmission pathways for ARB and their determinants to the food web.
In the past decade, the consumption of fresh produce has been recognized as a potential exposure route of not only foodborne pathogens but also ARB (Pesavento et al., 2014; Araújo et al., 2017). Foodborne diseases caused by pathogenic bacteria in field-grown produce have tremendously increased, leading to significant public health burden and multiple disease outbreaks (CDC, 2014; EFSA, 2017). Pathogenic E. coli strains have been implicated in several fresh produce related disease outbreaks, some of which were traced back to the preharvest environment. In 2005, an outbreak in Sweden with about 135 cases was caused by E. coli O157:H7 in iceberg lettuce and was traced back to the irrigation water used during the primary production (Söderström et al., 2008). In 2013, another outbreak was caused by enterohaemorrhagic E. coli (EHEC) in a fresh salad and the irrigation water used during the primary production was identified as the plausible source of the outbreak (Edelstein et al., 2014).
E. coli is a Gram-negative bacterium and a commensal in the human microbiota. Its genomic plasticity has enabled the evolution of the pathogenic strains that can cause human and animal diseases (Rojas-Lopez et al., 2018). The pathogenicity of E. coli is associated with specific virulence factors including cytotoxic necrotic factors, toxins, effacement factors, adhesins, capsules, haemolysins and invasins. These virulence factors alone are insufficient in determining the pathogenicity of E. coli, but targeting them is ideal in characterizing the virulence potential of a given E. coli isolate (Kuhnert et al., 2000). The pathogenic strains of E. coli are divided into two main groups depending on the location of the disease: intestinal pathogenic E. coli (InPEC) and extraintestinal pathogenic E. coli (ExPEC). The InPEC strains are transmitted through the faecal-oral route and are associated with diarrheal disease. They are sub-divided into six well-known pathotypes: enterotoxigenic E. coli (ETEC), enteroaggregative E. coli (EAEC), diffusely adherent E. coli (DAEC), enterohemorrhagic E. coli (EHEC), enteroinvasive E. coli (EIEC) and enteropathogenic E. coli (EPEC). The ExPEC strains are mainly associated with diseases outside of the intestinal tract. They are subdivided into the uropathogenic E. coli (UPEC) which is associated with urinary tract infections and neonatal meningitis E. coli (NMEC) which is associated with neonatal meningitis (Rojas-Lopez et al., 2018). Since E. coli is found in diverse hosts and environments and can easily acquire resistance, it is well suited for AMR surveillance (Erb et al., 2007).
There is a paucity of data regarding the occurrence of multidrug resistant pathogenic strains of E. coli in the preharvest environment in South Africa, despite its significance in the transmission of pathogens to the food web. This study was, therefore, carried out to evaluate the prevalence and diversities of multidrug resistant pathogenic strains of E. coli in irrigation water and agricultural soil in fresh produce farms so that appropriate measures can be taken to prevent their transmission to the food web.
Methods
Study Design and Setting
This study involved a cross-sectional laboratory-based analysis of preharvest environmental samples including irrigation water and agricultural soil collected from fresh produce farms in Amathole and Chris Hani District Municipalities, Eastern Cape Province. Eastern Cape Province is the second largest province in South Africa with an area of about 170,000 km2 and 6 676 590 people according to the 2021 Mid-year population estimates (Statssa, 2021). Amathole District Municipality is located in the central part of the province while Chris Hani District Municipality is located in the north-eastern part of the province. The agricultural and agro-processing sectors in the province are deeply rooted in the Provincial Growth and Development Program (PGDP) (EC Provincial Website, 2020). Fifteen sampling sites (S1-S15) were in Amathole District Municipality, while 4 sampling sites (S16-S19) were in Chris Hani District Municipality. This makes a total of 19 sampling sites as shown in Figure 1. These sampling sites were selected after reconnaissance visits were done and permits were granted by the farm owners. The anthropogenic activities identified in the irrigation water sources were animal intrusions, swimming, discharge of poultry and swine effluents and dumping of refuse while the anthropogenic activities identified on the farmlands were animal intrusion and soil amendment with organic and inorganic fertilizers as detailed in Supplementary Table S1.
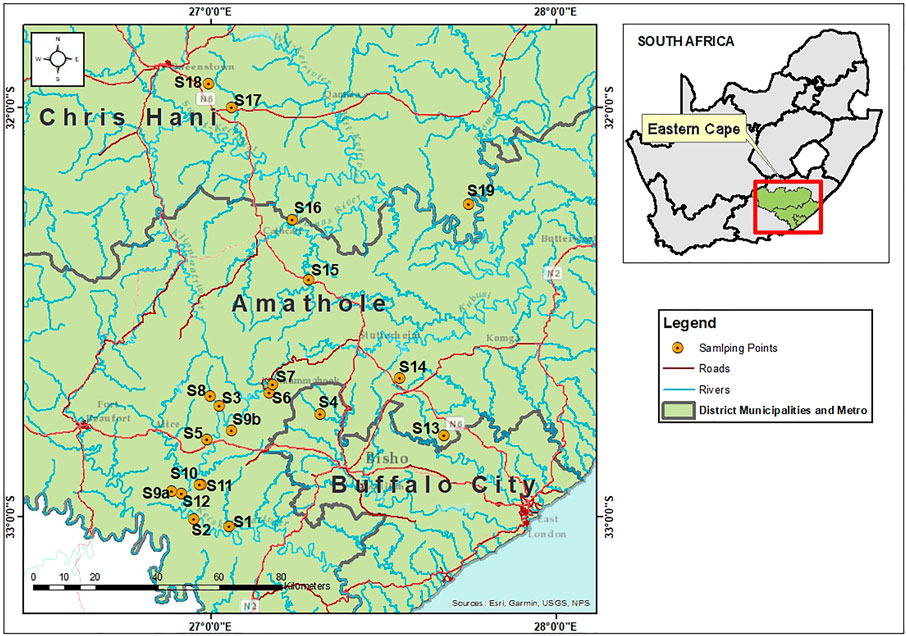
FIGURE 1. Map showing the sampling sites in Amathole (S1-S15) and Chris Hani (S16-S19) District Municipalities, Eastern Cape Province, South Africa.
Sample Collection
A grab sampling technique was employed in this study. Nineteen irrigation water samples (1 L each) and 13 agricultural soil samples (30 g each) were collected from the sampling sites using sterile 1L sampling bottles and sterile plastic bags respectively. The farms in S1, S4, S6, S10, S15, and S19 were inaccessible. As such, agricultural soil samples were not collected and only irrigation water samples were collected. All the samples were collected in triplicates, stored in a cooler box with ice and shipped to the laboratory within 4 h for microbiological analysis.
Isolation of Presumptive E. coli
In the laboratory, 10 ml of the irrigation water samples and 10 g of the agricultural soil samples were transferred to 90 ml of trypticase Soy Broth (TSB) (Laboratorios Conda, Pronadisa, South Africa) for the enrichment of E. coli in the samples. The broth was incubated at 37°C for 24 h after which a loop full was streaked on E. coli chromogenic agar (ECA) (Laboratorios Conda, Pronadisa, South Africa). After 24 h of incubation at 37°C, blue colonies on ECA were further purified on Eosin Methylene Blue (EMB) Agar (Laboratorios Conda, Pronadisa, South Africa) and incubated at 37°C for 24 h. At least 10 morphologically clear and distinct isolates from each sample with green metallic sheen were randomly picked as presumptive E. coli and stored in 25% glycerol stock at −80°C for further analysis.
Molecular Confirmation of the Presumptive E. coli Isolates and the Characterization of the Pathogenic Strains
The presumptive E. coli isolates were confirmed by targeting the housekeeping 4-methyl umbelliferyl-glucuronide (uidA) gene marker in a polymerase chain reaction (PCR) assay as described by Nontongana et al. (2014). Before this, the isolates were resuscitated using nutrient broth (Merck, SA) and the DNA of the isolates were extracted using the boiling technique as described by Maugeri et al. (2004) and López-Saucedo et al. (2003). The confirmed E. coli isolates were delineated into 6 intestinal pathogenic strains (EAEC, EIEC, DAEC, ETEC, EPEC, EHEC) and 2 extraintestinal pathogenic strains (NMEC, UPEC) as described by Titilawo et al. (2015b) in simplex PCR assays. The housekeeping gene, virulence genes, primer sequences, amplicon sizes and cycling conditions used to confirm the presumptive E. coli isolates and characterize them into their pathotypes are shown in Table 1. All the PCR assays were carried out using the MyCycler™ thermal Cycler PCR system (BioRad, United States) while the electrophoresed gels were visualized under the ultraviolet trans-illuminator (Alliance 4.7).
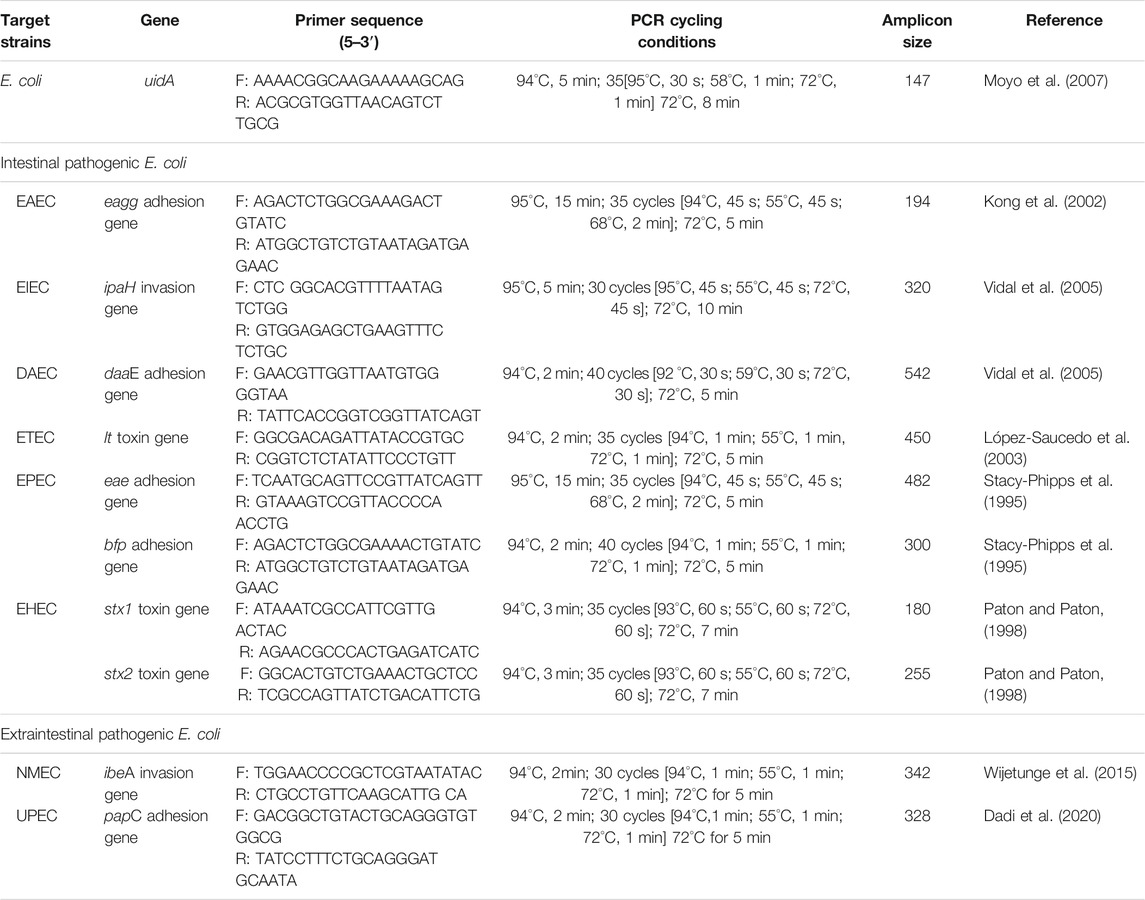
TABLE 1. Primer sequence, PCR cycling conditions and expected amplicon sizes for the confirmation and delineation of E. coli into its pathotypes.
Antimicrobial Susceptibility Testing and the Single Antimicrobial Resistance Indexing of the Pathogenic E. coli Strains
The disk diffusion test was used to evaluate the AS patterns of the pathogenic strains of E. coli following the recommendation of the Clinical Laboratory Standards Institute (CLSI, 2018). Confirmed E. coli pathotypes were picked from an overnight pure culture on nutrient agar, emulsified in sterile normal saline and adjusted to match a 0.5 McFarland standard. The mixture was evenly inoculated on Mueller-Hinton agar (Merck, South Africa) using sterile swabs. The test antibiotics were then placed on the agar using a disc dispenser. This was then incubated at 37°C for 24 h. All the zone of inhibition were measured in millimeters (mm) and the values were interpreted using the CLSI recommended standards (CLSI, 2018). The outcomes of the susceptibility tests were categorized as either Resistant (R), Intermediate (I) or Susceptible (S) to the test antibiotics. A total of 16 antibiotics belonging to 10 classes that are commonly used to treat infections caused by pathogenic E. coli were used to carry out the AS testing. They include:, tetracyclines [doxycycline (DXT/30 µg), tetracycline (T/30 µg)], carbapenems [meropenem (MEM/10 µg), imipenem (IMI/10 µg)], fluoroquinolones [norfloxacin (NOR/30 µg), ciprofloxacin (CIP/5 µg)], phenicols [chloramphenicol (C/30 µg)], quinolones [nalidixic acid (NA/30 µg)], cephems [cefuroxime (CXM/30 µg), cefotaxime (CTX/30 µg)], β-lactams [ampicillin (AP/10 µg), amoxicillin-clavulanic acid (AUG/30 µg)], sulfonamides [trimethoprim/sulphamethoxazole (TS/25 µg:25 µg)], aminoglycosides [amikacin (AK/30 µg), gentamycin (GM/10 µg)] and nitrofurans [nitrofurantoin (NI/300 µg)].
The Single Antimicrobial Resistance Index (SARI) of the pathogenic strains of E. coli retrieved from the preharvest environmental samples were evaluated using Eq. 1. The SARI provides values that range from 0 to 1. Isolates with SARI close to 1 are highly resistant against the test antimicrobials (Hernandez et al., 2021).
R, I and S represent the number of isolates with resistant, intermediate and susceptible outcomes respectively.
Multiple Antibiotic Resistance Phenotyping and Indexing of the E. coli Pathovars
E. coli pathovars that were non-susceptible to one or more antibiotics in three or more antimicrobial categories were considered multidrug resistant (MDR) (Magiorakos et al., 2012).
The multiple antimicrobial resistance phenotypes (MARPs) of the MDR isolates were evaluated as described by Titilawo et al. (2015c), while their multiple antimicrobial resistance indices (MARI) were evaluated using Eq. 2 (Krumperman, 1983).
MARI ≥0.2 indicate that the pathogen is from a “high-risk environment” where antimicrobials are indiscriminately used (Krumperman, 1983).
Molecular Screening of Antimicrobial Resistance Genes in Antibiotic Resistant E. coli Pathovars
Phenotypic antimicrobial resistant of E. coli pathovars were screened for putative antimicrobial resistance genes (ARGs) using PCR assays. The simplex, duplex and multiplex PCR assays were used to screen for 19 ARGs encoding tetracycline (tetA, tetB, tetC, tetD, tetE, tetG, tetK, tetL, tetM), sulfonamide (sulI, sulII), phenicol (cmlA1, catI, catII) and aminoglycoside [strA, aadA, aac(3)-IIa(aacC2), aph(3)-Ia(aphA1), aph(3)-IIa(aphA2)] resistance as described by (Titilawo et al., 2015a). Supplementary Table S2 details the genomic sequences of these genes, amplicon sizes, and the PCR cycling conditions. Additionally, 20 ARGs encoding for AmpC β-lactamase., ESBLs including blaTEM (TEM-1 and TEM-2), blaSHV (SHV-1), blaOXA-1-like (OXA-1, OXA-4, OXA-30), blaOXA-48-like (OXA-48), blaCTX-M-1 (CTX-M-1, CTX-M-3, CTX-M-15), blaCTX-M-2 (CTX-M-2), blaCTX-M-9 (CTX-M-9, CTX-M-14), blaCTX-M group 8/25 (CTX-M-8, CTX-M-25, CTX-M-26, CTX-M-39 to CTX-M-41), blaVEB (VEB-1 to VEB-6), blaPER (PER-1, PER-3), blaGES (GES-1 to 8, GES-11)., plasmid-mediated AmpC including blaACC (ACC-1, ACC-2), blaFOX (FOX-1 to FOX-5), blaMOX (MOX-1, MOX-2, CMY-1, CMY-8 to CMY-11, CMY-19), blaDHA (DHA-1, DHA-2), blaCIT (LAT-1 to LAT-3, BIL-1, CMY-2 to MY-7, CMY-12 to CMY-18 and CMY-21 to CMY-23), blaEBC (ACT-1, MIR-1) and carbapenemases including blaIMP (IMP variants except for IMP-9, IMP-16, IMP-18, IMP-22 and IMP-25), blaVIM (VIM-1, VIM-2), blaKPC (KPC-1 to KPC-5) were screened for in a simplex and multiplex PCR assays as described by Dallenne et al. (2010). The genomic sequences of these genes, amplicon sizes, and PCR cycling conditions are detailed in Supplementary Table S3.
Assessment of the Multiple Antibiotic Resistance Genotypes in Pathogenic E. coli Strains
The multiple antimicrobial resistance genotypes (MARGs) of the pathogenic E. coli strains harbouring ≥2 ARGs in each sample type were evaluated. The patterns of the MARGs were elucidated as described by Iwu and Okoh (2020).
Evaluation of the Correlation Between the Phenotypic and Genotypic Characteristics of the E. coli Pathovars and Their Antibiogram-Based Diversities
The correlation between the phenotypic and genotypic AMR characteristics of the pathogenic strains of E. coli retrieved from irrigation water and agricultural soil was evaluated using appropriate statistical analysis. A heatmap was constructed to display the phenotypic and genotypic AMR characteristics of all the pathogenic strains of E. coli retrieved from irrigation water and agricultural soil samples. Based on the antibiogram fingerprints of the isolates, a cluster analysis was done using the k-means clustering technique to elucidate the relatedness of the isolates. This was portrayed by a neighbour-joining dendrogram using a Jaccard similarity index and the generated clades.
Statistical Analysis
Data obtained from this study were captured in Microsoft Excel (version 2016) and subjected to descriptive and inferential statistical analysis using STATA 15 (Stata Corp LLC 4905, Lakeway Drive College Station, Texas 77,845, United States). The data were analysed for normality using the Shapiro-Wilk test, which rejects the null hypothesis of normality when p < 0.05 (Ahad et al., 2011). Normally distributed continuous variables were described using means, standard deviation (SD) and 95% confidence interval (CI), otherwise described using median, interquartile range (IQR) and 95% confidence interval (CI). Dichotomous and categorical variables were described using percentage proportions. The SARI and MARI of the pathogenic strains of E. coli retrieved from irrigation water and agricultural soil samples were compared using an unpaired samples t-test. The Spearman’s rank correlation test was used to assess the correlation between the phenotypic and genotypic AMR characteristics of the pathogenic strains of E. coli retrieved from the preharvest environmental samples. A two-tailed p-value of 0.05 was set to be statistically significant. The antibiogram-based K-means cluster analysis and neighbour-joining dendrogram was carried out using the Paleontological statistics software (version 3.14, Oslo, Norway).
Results
Confirmation and Pathogenic Characterization of E. coli Isolates Retrieved From the Preharvest Environmental Samples
A total of 350 presumptive E. coli isolates were retrieved, of which 200 were from irrigation water samples and 150 were from agricultural soil samples. Of this, 300 (86%) were confirmed to be E. coli. Specifically, 180 (60%) confirmed isolates were retrieved from irrigation water samples while 120 (40%) confirmed isolates were retrieved from agricultural soil samples. Out of the confirmed E. coli isolates (n = 300), 110 (37%) were found to be pathogenic harbouring at least one of the screened virulence genes. Of this, 70 (64%) pathogenic strains were retrieved from irrigation water samples while 40 (36%) pathogenic strains were retrieved from agricultural soil samples. The PCR amplification of the housekeeping uidA gene used in the confirmation of E. coli as well as the virulence genes used in the characterization of its pathotypes are shown in Figure 2A.
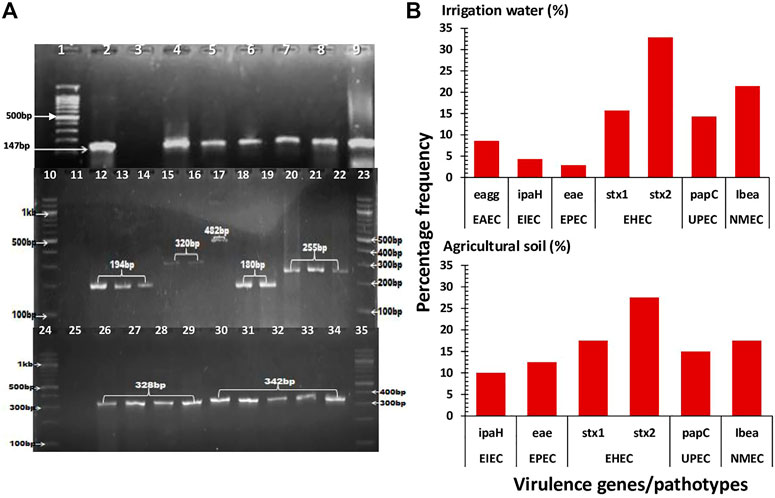
FIGURE 2. The confirmation, pathotype characterization and prevalence of the pathogenic strains of E. coli in irrigation water and agricultural soil samples. (A): A representative gel image showing the PCR amplification of the housekeeping uidA gene (lane 4–9) and VGs including eagg (lane 12–14), ipaH (lane 15 and 16), eae (lane 17), stx1 (lane 18 and 19), stx2 (lane 20–22), papC (lane 26–29) and ibeA (lane 30–34). Lane 1, 10, 23, 24 and 35 represents 100bp DNA ladder, lane 2 represents positive control (E. coli ATCC 25922), lane 3, 11 and 25 represents negative control. (B): The prevalence of the detected VGs encoding for the various pathotypes.
In irrigation water samples, the InPEC pathotypes/encoded VGs detected include EAEC/eagg, EIEC/ipaH, EPEC/eae, EHEC/stx1 and EHEC/stx2 while the ExPEC pathotype/encoded VGs detected include UPEC/papC and NMEC/Ibea. In agricultural soil samples, the InPEC pathotypes/encoded VGs detected include EIEC/ipaH, EPEC/eae, EHEC/stx1 and EHEC/stx2 while the ExPEC pathotypes/encoded VGs detected include UPEC/papC and NMEC/Ibea. The prevalence of the detected pathotypes in irrigation water samples ranged from 2.9% (EPEC/eae) to 32.9% (EHEC/stx2) while the prevalence of the detected pathotypes in agricultural soil samples ranged from 10% (EIEC/ipaH) to 27.5% (EHEC/stx2) as shown in Figure 2B.
Antimicrobial Susceptibility Profiles and Single Antimicrobial Resistance Indices (SARI) of the Pathogenic Strains of E. coli Isolates in Preharvest Environmental Samples
The antimicrobial susceptibility profiles and SARI of the pathogenic strains of E. coli retrieved from irrigation water and agricultural soil samples are shown in Figure 3. In irrigation water samples, the percentage susceptibility of the isolates to the test antimicrobials ranged from 25.7% (ampicillin) to 98.6% (amikacin), while the percentage resistance of the isolates against the test antimicrobials ranged from 1.4% (amikacin and imipenem) to 72.9% (ampicillin). High susceptibility rates were also observed for gentamicin (94.3%), imipenem (94.3%) and meropenem (91.4%), and high resistance rates were also observed for amoxicillin-clavulanic acid (70.0%) and tetracycline (58.6%). The SARI of the pathogenic E. coli retrieved from irrigation water samples ranged from 0.0 to 0.70 with a median score of 0.23 (IQR: 0.10 to 0.38, 95% CI: 0.16–0.27).
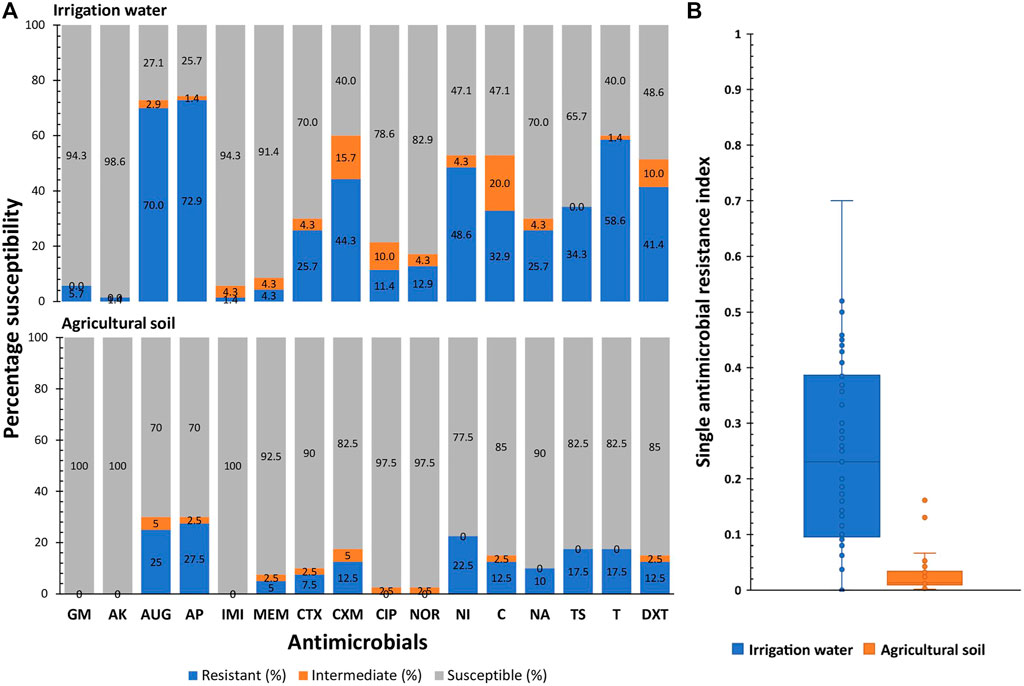
FIGURE 3. The antimicrobial susceptibility profiles (A) and single antimicrobial resistance index (B) of the pathogenic strains of E. coli retrieved from irrigation water and agricultural soil samples. GM-gentamycin, AK-amikacin, AUG-amoxycillin-clavulanic acid, AP-ampicillin, IMI-imipenem, MEM-meropenem, CTX-cefotaxime, CXM-cefuroxime, CIP-ciprofloxacin, NOR-norfloxacin, NI-nitrofurantoin, C-chloramphenicol, NA-nalidixic acid, TS-trimethoprim/sulphamethoxazole, T-tetracycline, DXT-doxycycline.
In agricultural soil samples, the percentage susceptibility of the isolates to the test antimicrobials ranged from 70% (amoxicillin-clavulanic acid and ampicillin) to 100% (gentamicin, amikacin and imipenem), while the percentage resistance of the isolates against the test antimicrobials ranged from 0.0% (gentamicin, amikacin and imipenem) to 27.5% (ampicillin). High susceptibility rates were also observed for ciprofloxacin (97.5%), norfloxacin (97.5%), meropenem (92.5%), cefotaxime (90%) and nalidixic acid (90%) and high resistance rates were also observed for amoxicillin-clavulanic acid (25%), nitrofurantoin (22.5%), tetracycline (17.5%) and trimethoprim/sulphamethoxazole (17.5%). The SARI of the pathogenic E. coli retrieved from agricultural soil samples ranged from 0.002 to 0.16 with a median score of 0.01 (IQR: 0.01 to 0.03, 95% CI: 0.01–0.03).
The SARI of the antimicrobial resistant pathogenic E. coli strains retrieved from irrigation water was significantly higher than that of the pathogenic E. coli strains retrieved from agricultural soil; t (108) = 7.8, p ≤ 0.01.
The Multiple Antimicrobial Resistance Phenotypes and Multiple Antimicrobial Resistance Indices of the Pathogenic Strains of E. coli Recovered From the Preharvest Environmental Samples
Of the pathogenic E. coli strains (n = 70) recovered from the irrigation water samples, 60 (85.7%) were phenotypically resistant against at least one antimicrobial, while 42 (60.0%) were multidrug resistant (MDR). The majority of the MDR E. coli pathovars in irrigation water samples were retrieved from S5 and S18 as shown in Figure 4. The MARPs and MARI of the MDR isolates are shown in Table 2. A total of 38 patterns of MARPS was observed most of which (n = 34) appeared uniquely. Four MARPS patterns including “NI-T-DXT”, “AUG-AP-CTX-NI-C”, “AUG-AP-CXM-NI-C-NA-TS-T-DXT”, “GM-AUG-AP-CIP-NOR-C-NA-TS-T-DXT” were observed in duplicates. The MARI of the MDR pathogenic E. coli from irrigation water samples ranged from 0.2 to 0.8 with a median score of 0.41 (IQR: 0.25 to 0.52, 95% CI: 0.31–0.47).
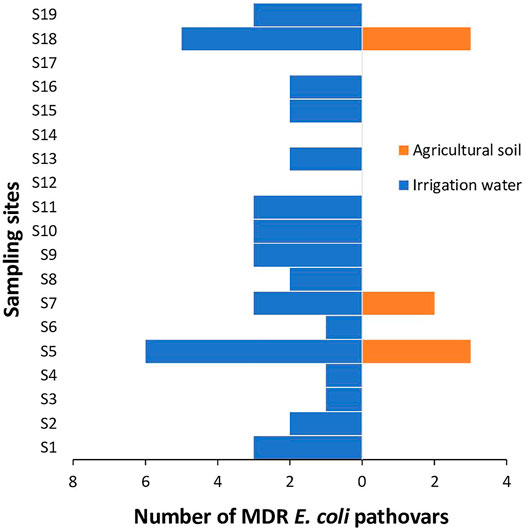
FIGURE 4. The distribution of MDR pathogenic strains of E. coli in irrigation water and agricultural soil across the sampling sites.
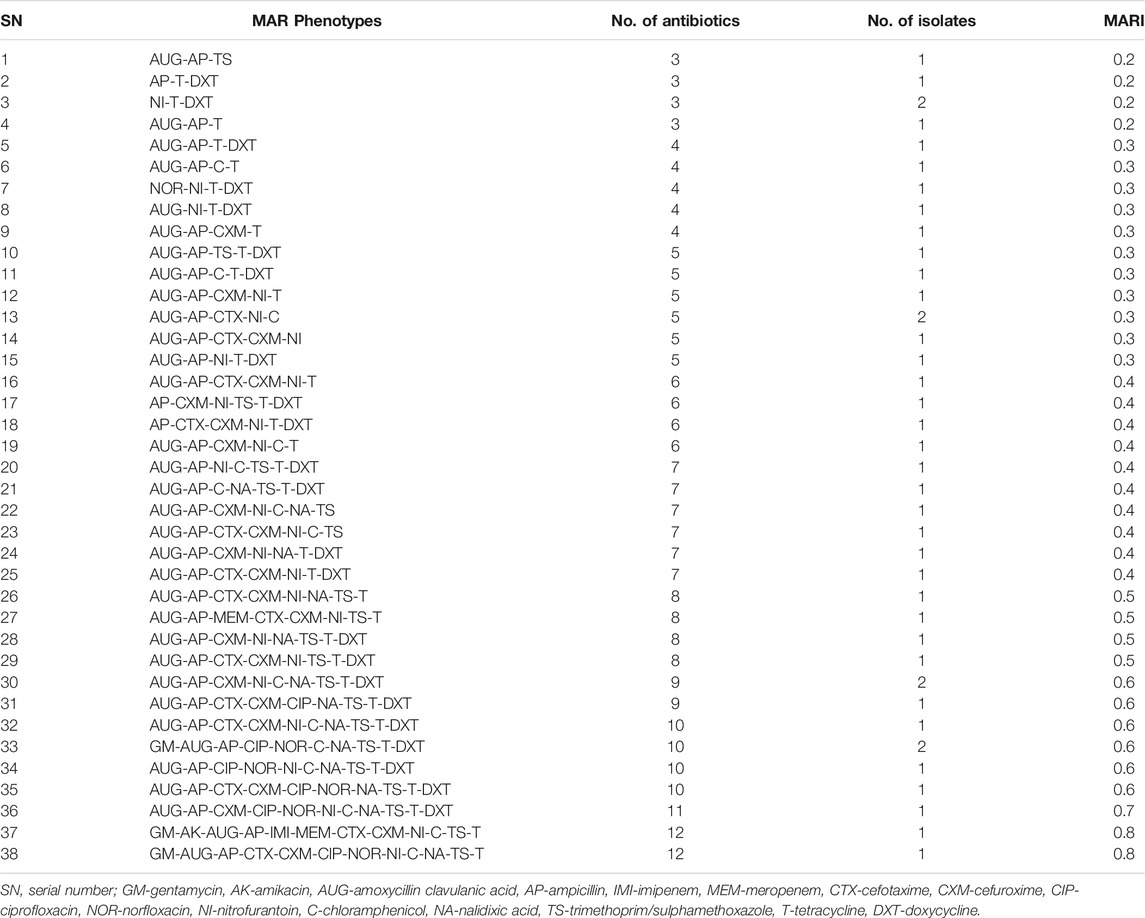
TABLE 2. The MARPs and MARI of the pathogenic strains of E. coli retrieved from irrigation water samples.
Of the pathogenic E. coli strains (n = 40) recovered from the agricultural soil samples, 15 (37.5%) were phenotypically resistant against at least one antimicrobial, while 8 (20.0%) were (MDR). The MDR E. coli pathovars in agricultural soil samples were retrieved from S5, S7 and S18 as shown in Figure 4. The MARPs and MARI of the MDR isolates are shown in Table 3. A total of 6 patterns of MARPs was observed most of which (n = 4) appeared uniquely. Two MARPS patterns including “AUG-AP-NI-TS-T-DXT” and “AUG-AP-MEM-CTX-CXM-NI-C-NA-TS-T-DXT” were observed in duplicates. The MARI of the MDR pathogenic E. coli from agricultural soil samples ranged from 0.2 to 0.7 with a median score of 0.38 (IQR: 0.23 to 0.66, 95% CI: 0.19–0.69).
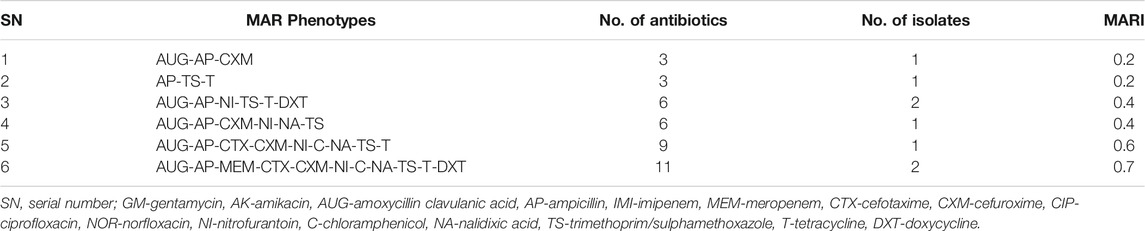
TABLE 3. The MARPs and MARI of the pathogenic strains of E. coli retrieved from agricultural soil samples.
There was no significant difference between the MARI of the MDR pathogenic E. coli strains retrieved from irrigation water and that from agricultural soil; t (56) = 0.3, p = 0.7.
Detection and Prevalence of Antimicrobial Resistance Genes in Phenotypically Resistant Pathogenic Strains of E. coli
The ARGs detected in phenotypically resistant pathogenic strains of E. coli recovered from irrigation water samples include catII phenicol resistance encoding gene, tetA and tetB tetracycline resistance-encoding genes, sulI and sulII sulfonamide resistance-encoding genes, AmpC β-lactam resistance-encoding gene and blaTEM, blaPER, and blaGES ESBLs. The frequency of occurrence of these genes ranged from 4.2% for blaPER and blaGES to 75.0% for sulI as shown in Figure 5.
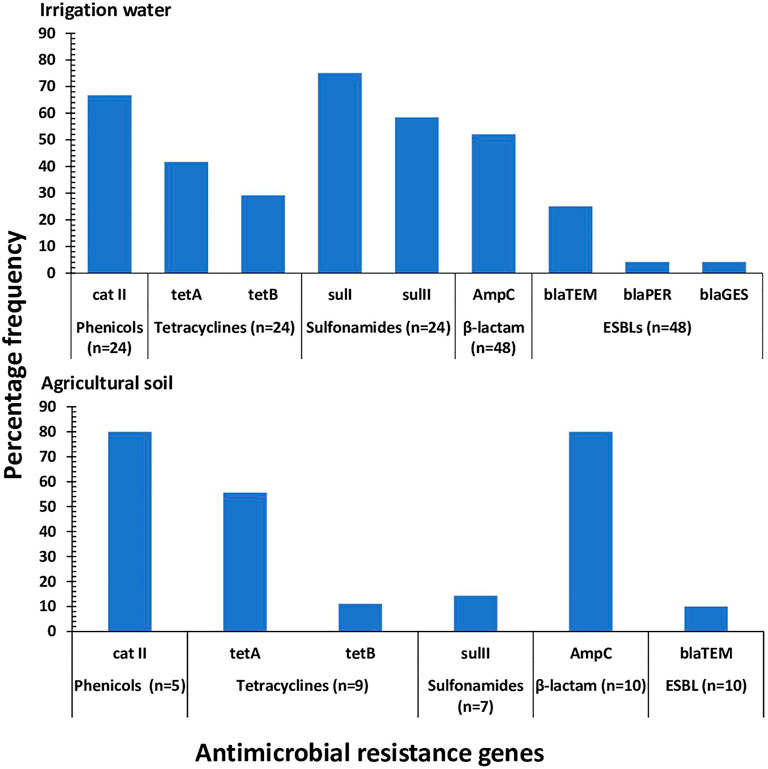
FIGURE 5. The frequency of occurrence of ARGs detected in the pathogenic strains of E. coli recovered from irrigation water and agricultural soil. n: the number of isolates phenotypically resistant against the associated antimicrobial.
In phenotypically resistant pathogenic strains of E. coli recovered from agricultural soil, the ARGs detected include catII phenicol resistance encoding gene, tetA and tetB tetracycline resistance-encoding genes, sulII sulfonamide resistance-encoding genes, AmpC β-lactam resistance-encoding gene and blaTEM ESBL. The frequency of occurrence of these genes ranged from 10.0% for blaTEM to 80.0% for catII and AmpC as shown in Figure 5.
The Multiple Antimicrobial Resistance Genotypes in the pathogenic Strains of E. coli Recovered From Preharvest Environmental Samples
In irrigation water samples, 26 (43.3%) of the phenotypically resistant pathogenic strains of E. coli (n = 60) harboured multiple (≥2) ARGs, some of which were a combination of non-β-lactams/ESBLs and β-lactams/ESBLs. The MARGs of these isolates were mapped as shown in Table 4 and a total of 18 patterns were observed, most of which occurred uniquely. Four of the patterns were observed in duplicates while two of the patterns were observed in triplicates.
In agricultural soil samples, 5 (33.3%) of the phenotypically resistant pathogenic strains of E. coli (n = 15) harboured multiple (≥2) ARGs, some of which were a combination of non-β-lactams/ESBLs and β-lactams/ESBLs. The MARGs of these isolates were mapped as shown in Table 5 and a total of five patterns were observed, all of which occurred uniquely.
The Correlation Between the Phenotypic and Genotypic Characteristics of E. coli Pathovars in the Preharvest Environmental Samples and Their Antibiogram-Based Diversities
There was a positive correlation between the phenotypic and genotypic AMR profiles of the pathogenic strains of E. coli retrieved from irrigation water samples, r = 0.6, p ≤ 0.01 and agricultural soil samples, r = 0.8, p ≤ 0.01. Based on the antibiogram fingerprints of the pathogenic strains of E. coli retrieved from the pre-harvest environmental samples, 11 clades (A to K) of the isolates from irrigation water samples were identified as shown in Figure 6, while 7 clades (A to G) of the isolates from the agricultural soil samples were identified as shown in Figure 7. Two isolates from the irrigation water samples and only one isolate from the agricultural soil samples did not cluster with other isolates.
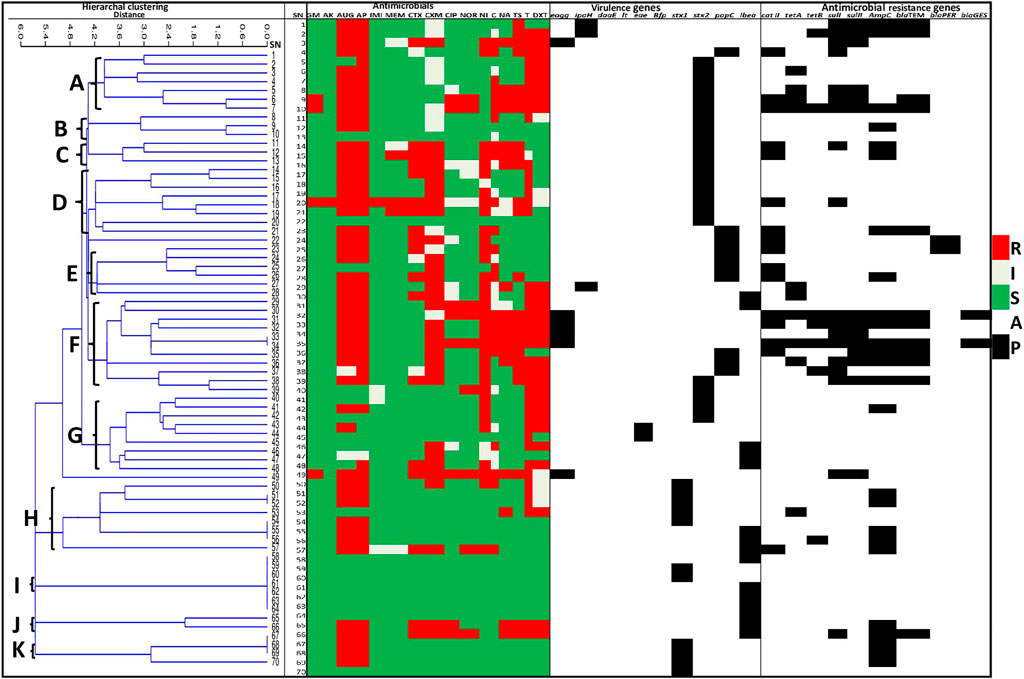
FIGURE 6. A heatmap cluster analysis of the antibiogram profiles of the pathogenic strains of E. coli retrieved from irrigation water samples. The clusters grouped related isolates into clades A to K based on their response/activity to the test antimicrobials. SN: Isolate numbers, R: Resistance, I: Intermediate, S: Susceptible, A: Absent, P: Present, GM-gentamycin, AK-amikacin, AUG-amoxycillin clavulanic acid, AP-ampicillin, IMI-imipenem, MEM-meropenem, CTX-cefotaxime, CXM-cefuroxime, CIP-ciprofloxacin, NOR-norfloxacin, NI-nitrofurantoin, C-chloramphenicol, NA-nalidixic acid, TS-trimethoprim/sulphamethoxazole, T-tetracycline, DXT-doxycycline.
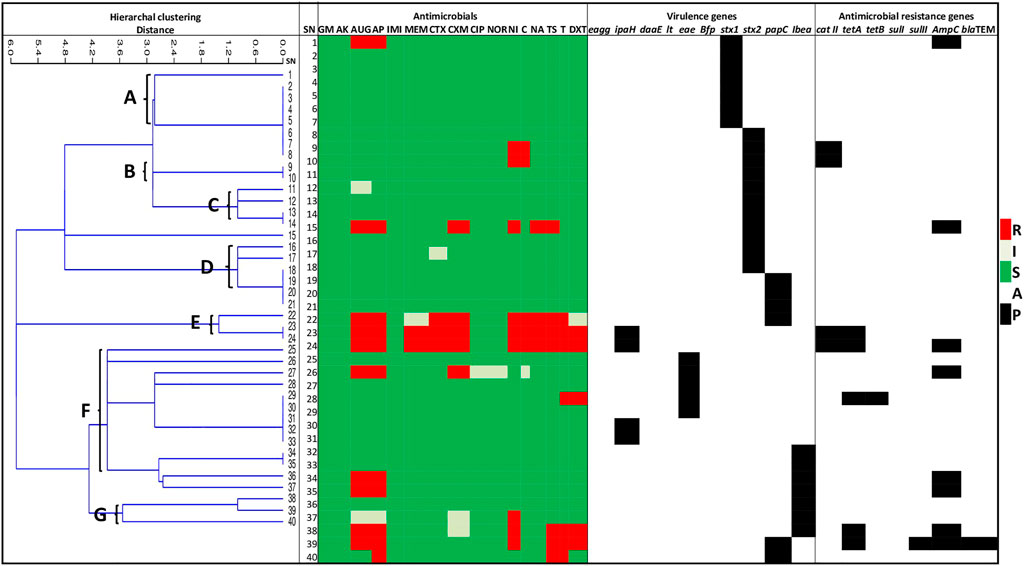
FIGURE 7. A heatmap cluster analysis of the antibiogram profiles of the pathogenic strains of E. coli retrieved from agricultural soil samples. The clusters grouped related isolates into clades A to G based on their response/activity to the test antimicrobials. SN: Isolate numbers, R: Resistance, I: Intermediate, S: Susceptible, A: Absent, P: Present, GM-gentamycin, AK-amikacin, AUG-amoxycillin-clavulanic acid, AP-ampicillin, IMI-imipenem, MEM-meropenem, CTX-cefotaxime, CXM-cefuroxime, CIP-ciprofloxacin, NOR-norfloxacin, NI-nitrofurantoin, C-chloramphenicol, NA-nalidixic acid, TS-trimethoprim/sulphamethoxazole, T-tetracycline, DXT-doxycycline.
Discussion
The occurrence of antimicrobial resistant E. coli in the environment has been proposed to indicate the presence of other public health and environmentally significant antimicrobial resistant bacteria and clinically relevant ARGs (EFSA, 2008; Gekenidis et al., 2018). In this study, we evaluated the prevalence and diversities of multidrug resistant pathogenic strains of E. coli in preharvest environmental samples including irrigation water and agricultural soil so that appropriate measures can be taken to minimize their occurrence and transmission to the food web.
A high proportion of the presumptive isolates in both irrigation water and agricultural soil in this study were confirmed to be E. coli, many of which were pathogenic strains harbouring at least one of the screened virulence genes. Four InPEC including EAEC, EIEC, EPEC, EHEC and 2 ExPEC including UPEC and NMEC were detected in the irrigation water samples in this study. Similar pathotypes were detected in a surface water body which may be used for irrigation purposes in Australia (Sidhu et al., 2013). Three InPEC including EIEC, EPEC, EHEC and 2 ExPEC including UPEC and NMEC were detected in the agricultural soil samples in this study. This poses a huge risk to food safety and public health considering the role the preharvest environmental niches play in the transmission of infectious disease pathogens to the food web (Iwu and Okoh, 2019).
The prevalence of E. coli pathotypes and their associated virulence genes in irrigation water samples ranged from 2.9% (EPEC/eae) to 32.9% (EHEC/stx2) while that in agricultural soil samples ranged from 10% (EIEC/ipaH) to 27.5% (EHEC/stx2). This implies that EHEC encoded by the stx2 genes were predominantly detected in this study. This contrasts a similar study in Johannesburg, South Africa whereby EHEC at a prevalence rate of 3% was the least prevailing pathotype in environmental water samples while EPEC was the most prevailing pathotype at a prevalence rate of 19.2% (Bolukaoto et al., 2021). Another contrasting finding in South Africa indicated that EAEC encoded by the aatA and/or aaiC genes was the predominant pathotype in irrigation water and food products at a prevalence rate of 2% (Aijuka et al., 2018). These contrasts may be attributed to the differences in the geographical spread of E. coli pathotypes which is largely impacted by the varying levels of anthropogenic activities specific to the study areas. Considering that EHEC is generally involved in large outbreaks globally (Torres, 2017), detecting them at a high prevalence rate in the preharvest environment in this study is a ticking time bomb for fresh produce related disease outbreaks. It also indicates that the study sites are potential hotspots for the spread of pathogenic strains of E. coli with outbreak potential. This requires urgent attention as EHEC does not only cause diarrheal disease but also cause medical complications such as hemorrhagic colitis and hemolytic uremic syndrome among the human population (Torres, 2017).
The emergence of antimicrobial resistant pathogenic bacteria has prompted the need to continually investigate their presence in the environment, from where they can reach humans. The pathogenic strains of E. coli retrieved from the preharvest environmental samples in this study conferred a high resistance against the β-lactams including ampicillin and amoxicillin-clavulanic acid, tetracyclines, nitrofurantoin and trimethoprim/sulphamethoxazole. This implies that these antimicrobials are indiscriminately used in this study area. A similar study in Texas, United States recorded a high resistance against ampicillin among 81% of their isolates (Summerlin et al., 2021). Additionally, a similar study in Spain recorded a high resistance against sulfamethoxazole, ampicillin and tetracycline (Amato et al., 2021). On the flip side, the pathogenic strains of E. coli retrieved from the preharvest environmental samples in this study were highly susceptible to aminoglycosides including amikacin and gentamicin, carbapenems including imipenem and meropenem, fluoroquinolones including ciprofloxacin and norfloxacin, cephems including cefotaxime and quinolones including nalidixic acid. Fortunately, the median scores of the SARI of the E. coli pathogens recovered from the preharvest environmental samples in this study were low. This indicates that the isolates conferred resistance against the test antimicrobials at a low rate. In other words, only a few of the isolates were resistant against the test antimicrobials. The median SARI score of the E. coli pathogens recovered from irrigation water was significantly higher than that of the E. coli pathogens recovered from agricultural soil. This indicates that the irrigation water are better reservoirs of antimicrobial resistant bacteria. This is attributed to the numerous anthropogenic activities observed at the irrigation water sources which included animal intrusion, recreational activities, dumping of refuse, discharge of poultry and swine effluents and discharge of WWTP effluents. Additionally, the irrigation water sources across all the sampling sites aside S12 and S17 were surface water bodies which are open to various contamination sources.
Although the E. coli pathovars recovered from the preharvest environment in this present study had relatively low SARI, most of the resistant isolates were highly multidrug resistant against the test antimicrobials. The MDR E. coli pathovars in irrigation water samples were majorly detected in S5 and S18. This is not surprising as the irrigation water sources in these sampling sites receives effluents from livestock farms and WWTPs. Interestingly, no MDR E. coli pathovar were detected in irrigation water samples collected from S12 and S17. This is probably because the source of irrigation water in these sampling sites were treated municipal water and borehole, indicating that they are the ideal sources of water required for the primary production of fresh produce. In the same vein, The MDR E. coli pathovars in agricultural soil samples were detected in S5 (loamy soil), S7 (clay soil) and S18 (loamy soil). The agricultural soil in these sampling sites were found to be amended using poultry and swine faeces and organic composts of animal origin. This may have attributed to the occurrence of the MDR E. coli pathovars in the agricultural soil in the sampling sites.
The majority of the MARP patterns of the MDR isolates in the preharvest environmental samples occurred uniquely while only a few occurred in duplicates. This suggests that each MDR isolate in the preharvest environment were exposed to unique, yet highly diversified antimicrobial selective pressure which may have led to the rapid evolution and transmission of diverse resistance determinants between the isolates. The MARI of all the MDR isolates in this study were all greater than the benchmark of 0.2. This confirms that antimicrobials are indiscriminately used in the study area. As a result, the preharvest environments in the study area are high-risk environments that favour the evolution and dissemination of MDR pathogens. This is plausible as antimicrobials dispensed to humans and animals are often not completely broken down. The metabolites are discharged into the environment which eventually finds their way to the preharvest environmental niches such as irrigation water and agricultural soil where they cause natural selective pressure for the resident bacteria (Iwu et al., 2020). There was no significant difference between the MARI of the E. coli pathogens in irrigation water and that of the E. coli pathogens in agricultural soil. This indicates that irrigation water and agricultural soil are equally high-risk environments as they are great receptacles of antimicrobial metabolites.
The presence of antimicrobials in the environment encourages the horizontal transfer of antimicrobial resistance genes between related and unrelated bacteria. This increases the prevalence of resistant bacteria in the environment and exacerbates the risk of super-resistant infections (Lerminiaux and Cameron, 2019). In this study, sulI sulfonamide resistance-encoding gene was the most prevailing ARG in the pathogenic strains of E. coli retrieved from irrigation water samples while catII phenicol resistance-encoding gene and AmpC β-lactam resistance-encoding gene were the most prevailing ARGs in the pathogenic strains of E. coli retrieved from agricultural soil samples. The tetA and tetB tetracycline resistance-encoding genes were also detected from the pathogenic strains of E. coli in both irrigation water and agricultural soil at a relatively high prevalence rate. These genes including macrolide and fluoroquinolone resistance encoding genes have been identified as the most abundant ARGs in the aquatic environments (Zhai et al., 2016; Christou et al., 2017; Dang et al., 2017). Despite the scarcity of studies on the prevalence of ARGs in agricultural soil, the abundance of the aforementioned ARGs in the preharvest environment is not surprising since the associated antimicrobials (sulfonamides, phenicols, tetracyclines, and ampicillin) are traditionally used as a first-line treatment of gastrointestinal infections. Additionally, the associated antimicrobials are used as growth promoters in the Eastern Cape Province in South Africa (Iweriebor et al., 2015).
β-lactams are the most widely used antimicrobials in the treatment of a wide variety of infectious diseases. This is because of their low toxicity and high effectiveness. This has led to an increased prevalence of the various variants of AmpC and ESBLs in the environment (Livermore, 1996; Iwu et al., 2021). The ESBLs in the pathogenic strains of E. coli detected in the preharvest environmental samples in this study were blaTEM, blaPER, and blaGES. This corroborates similar studies whereby blaTEM producing E. coli was detected in irrigation water and vegetables in household farms (Araújo et al., 2017) as well as in agricultural irrigation waters from Valencia city, Spain (Amato et al., 2021). Although blaSHV, blaTEM, and blaCTX-M have been identified as the most prevalent ESBLs in clinical and agricultural settings globally (Njage and Buys, 2015; Ye et al., 2017), our study, as a contrast, indicates the presence of blaPER, and blaGES producing E. coli in the preharvest environment, hence the need for constant surveillance of the ESBLs in the environment. Sadly, a high proportion of the pathogenic E. coli in this study harboured multiple ARGs, some of which were a combination of non-β-lactams/ESBLs with β-lactams/ESBLs. The majority of the MARGs patterns occurred uniquely, suggesting a rapid genetic exchange of ARGs between the pathogenic strains of E. coli in the preharvest environment.
Our study indicated a strong positive correlation between the genetic and phenotypic characteristics of the pathogenic strains of E. coli in the preharvest environment. This suggests that the detected ARGs were responsible for the resistance phenotypically observed among the isolates. Our antibiogram-based cluster analysis indicated that the isolates were highly distinct. This suggests that the preharvest environment is saturated with expanded classes of antimicrobials, which in turn causes a highly diversified AMR selective pressure for the evolution and dissemination of diverse multidrug resistant pathogenic E. coli strains and their determinants. The strength of this study is that it has provided more insight into the occurrence and diversities of multidrug resistant pathogenic strains of E. coli in the preharvest environment, an area that is still understudied despite its public health importance. The study also employed a robust statistical analysis to explore the epidemiological and correlational distribution of the multidrug resistant pathogenic strains of E. coli in the preharvest environment. The study, nonetheless, had a limitation. The grab sampling technique used in this study provided only a snapshot of the distribution of multidrug resistant E. coli pathovars in the preharvest environmental samples collected from the study sites.
Conclusion
This study revealed the high levels of diversified multidrug resistant E. coli pathovars in the preharvest environmental samples including irrigation water and agricultural soil collected from fresh produce farms in two District Municipalities in the Eastern Cape Province, South Africa. These agricultural niches were found to be high risk environments that support the evolution and dissemination of AMR. EHEC encoded by the stx2 toxin gene was the most prevalent pathovar in the preharvest environment indicating an outbreak potential when transferred to the food web. This calls for a multisectoral and evidence-based approach to minimize the occurrence of multidrug resistant pathogenic bacteria in the preharvest environment and their potential spread to the food web.
Data Availability Statement
The original contributions presented in the study are included in the article/Supplementary Material, further inquiries can be directed to the corresponding author.
Author Contributions
Conceptualization: CI and AI; methodology: CI and AI; investigation: CI; data curation: CI; data analysis: CI; writing—original draft preparation: CI; writing—review and editing, CI, AK, BI, and AI; supervision: AI; funding acquisition: AI. All authors have read and agreed to the published version of the manuscript.
Funding
This study was funded by the South African Medical Research Council, the Department of Science and Technology of South Africa, and the United States Agency for International Development. These funding agencies did not partake in the study’s design, and conclusions arrived herein are entirely those of the authors.
Conflict of Interest
The authors declare that the research was conducted in the absence of any commercial or financial relationships that could be construed as a potential conflict of interest.
Publisher’s Note
All claims expressed in this article are solely those of the authors and do not necessarily represent those of their affiliated organizations, or those of the publisher, the editors and the reviewers. Any product that may be evaluated in this article, or claim that may be made by its manufacturer, is not guaranteed or endorsed by the publisher.
Supplementary Material
The Supplementary Material for this article can be found online at: https://www.frontiersin.org/articles/10.3389/fenvs.2022.858964/full#supplementary-material
References
Ahad, N. A., Yin, T. S., Abdul, R. O., and Yaacob, C. R. (2011). Sensitivity of Normality Tests to Non-normal Data (Kepekaan Ujian Kenormalan Terhadap Data Tidak Normal). Sains Malaysiana. 40, 637–641.
Aijuka, M., Santiago, A. E., Girón, J. A., Nataro, J. P., and Buys, E. M. (2018). Enteroaggregative Escherichia coli Is the Predominant Diarrheagenic E. coli Pathotype Among Irrigation Water and Food Sources in South Africa. Int. J. Food Microbiol. 278, 44–51. doi:10.1016/J.IJFOODMICRO.2018.04.018
Amato, M., Dasí, D., González, A., Ferrús, M. A., and Castillo, M. Á. (2021). Occurrence of Antibiotic Resistant Bacteria and Resistance Genes in Agricultural Irrigation Waters from Valencia City (Spain). Agric. Water Management. 256, 107097. doi:10.1016/J.AGWAT.2021.107097
Araújo, S., A.T. Silva, I. I., Tacão, M., Patinha, C., Alves, A., and Henriques, I. (2017). Characterization of Antibiotic Resistant and Pathogenic Escherichia coli in Irrigation Water and Vegetables in Household Farms. Int. J. Food Microbiol. 257, 192–200. doi:10.1016/j.ijfoodmicro.2017.06.020
Bolukaoto, J. Y., Singh, A., Alfinete, N., and Barnard, T. G. (2021). Occurrence of Hybrid Diarrhoeagenic Escherichia coli Associated with Multidrug Resistance in Environmental Water, Johannesburg, South Africa. Microorganisms. 9, 2163. doi:10.3390/MICROORGANISMS9102163
CDC (2014). Centers for Disease Control and Prevention. Surveillance for Foodborne Disease Outbreaks, United States, 2012, Annual Report. Atlanta, Georgia: US Department of Health and Human Services. Available at: https://www.cdc.gov/foodsafety/pdfs/foodborne-disease-outbreaks-annual-report-2012-508c.pdf (Accessed October 6, 2018).
Christou, A., Agüera, A., Bayona, J. M., Cytryn, E., Fotopoulos, V., Lambropoulou, D., et al. (2017). The Potential Implications of Reclaimed Wastewater Reuse for Irrigation on the Agricultural Environment: The Knowns and Unknowns of the Fate of Antibiotics and Antibiotic Resistant Bacteria and Resistance Genes - A Review. Water Res. 123, 448–467. doi:10.1016/J.WATRES.2017.07.004
CLSI (2018). Clinical and Laboratory Standards Institute. Performance Standards for Antimicrobial Susceptibility Testing. 28th Ed. CLSI Supplement M100. Wayne, PA: Clinical and Laboratory Standards Institute.
Dadi, B. R., Abebe, T., Zhang, L., Mihret, A., Abebe, W., and Amogne, W. (2020). Distribution of Virulence Genes and Phylogenetics of Uropathogenic Escherichia coli Among Urinary Tract Infection Patients in Addis Ababa, Ethiopia. BMC Infect. Dis. 20, 20–108. doi:10.1186/S12879-020-4844-Z
Dallenne, C., da Costa, A., Decré, D., Favier, C., and Arlet, G. (2010). Development of a Set of Multiplex PCR Assays for the Detection of Genes Encoding Important β-lactamases in Enterobacteriaceae. J. Antimicrob. Chemother. 65, 490–495. doi:10.1093/jac/dkp498
Dang, B., Mao, D., Xu, Y., and Luo, Y. (2017). Conjugative Multi-Resistant Plasmids in Haihe River and Their Impacts on the Abundance and Spatial Distribution of Antibiotic Resistance Genes. Water Res. 111, 81–91. doi:10.1016/J.WATRES.2016.12.046
EC Provincial Website (2020). The Eastern Cape- Hope of Legends. Available at: https://ecprov.gov.za/the-eastern-cape.aspx (Accessed October 10, 2021).
Edelstein, M., Sundborger, C., Hergens, M.-P., Ivarsson, S., Dryselius, R., Insulander, M., et al. (2014). Barriers to Trace-Back in a Salad-Associated EHEC Outbreak, Sweden, June 2013. Plos Curr. 6. doi:10.1371/currents.outbreaks.80bbab3af3232be0372ea0e904dcd1fe
EFSA (2008). European Food Safety Authority. Report from the Task Force on Zoonoses Data Collection Including Guidance for Harmonized Monitoring and Reporting of Antimicrobial Resistance in Commensal Escherichia coli and Enterococcus Spp. From Food Animals. EFSA J. 6. doi:10.2903/J.EFSA.2008.141R
EFSA (2017). The European Union Summary Report on Trends and Sources of Zoonoses, Zoonotic Agents and Food-Borne Outbreaks in 2016. EFSA J. 15, e05077. doi:10.2903/j.efsa.2017.5077
Erb, A., Stürmer, T., Marre, R., and Brenner, H. (2007). Prevalence of Antibiotic Resistance in Escherichia coli: Overview of Geographical, Temporal, and Methodological Variations. Eur. J. Clin. Microbiol. Infect. Dis. 26, 83–90. doi:10.1007/S10096-006-0248-2/TABLES/2
Gekenidis, M.-T., Qi, W., Hummerjohann, J., Zbinden, R., Walsh, F., and Drissner, D. (2018). Antibiotic-resistant Indicator Bacteria in Irrigation Water: High Prevalence of Extended-Spectrum Beta-Lactamase (ESBL)-producing Escherichia coli. PLoS One. 13, e0207857. doi:10.1371/JOURNAL.PONE.0207857
Hernandez, B., Herrero-Viñas, P., Rawson, T. M., Moore, L. S. P., Holmes, A. H., and Georgiou, P. (2021). Resistance Trend Estimation Using Regression Analysis to Enhance Antimicrobial Surveillance: A Multi-Centre Study in London 2009-2016. Antibiotics. 10, 1267. doi:10.3390/ANTIBIOTICS10101267
Iweriebor, B. C., Iwu, C. J., Obi, L. C., Nwodo, U. U., and Okoh, A. I. (2015). Multiple Antibiotic Resistances Among Shiga Toxin Producing Escherichia coli O157 in Feces of Dairy Cattle Farms in Eastern Cape of South Africa. BMC Microbiol. 15, 2131–2139. doi:10.1186/s12866-015-0553-y
Iwu, C. D., du Plessis, E., Korsten, L., and Okoh, A. I. (2021). Antibiogram Imprints of E. coli O157:H7 Recovered from Irrigation Water and Agricultural Soil Samples Collected from Two District Municipalities in South Africa. Int. J. Environ. Stud. 78, 940–953. doi:10.1080/00207233.2020.1854522
Iwu, C. D., Korsten, L., and Okoh, A. I. (2020). The Incidence of Antibiotic Resistance within and beyond the Agricultural Ecosystem: A Concern for Public Health. Microbiologyopen. 9, e1035. doi:10.1002/mbo3.1035
Iwu, C. D., and Okoh, A. I. (2019). Preharvest Transmission Routes of Fresh Produce Associated Bacterial Pathogens with Outbreak Potentials: A Review. Int. J. Environ. Res. Public Health. 16, 4407. doi:10.3390/ijerph16224407
Iwu, C. D., and Okoh, A. I. (2020). Characterization of Antibiogram Fingerprints in Listeria Monocytogenes Recovered from Irrigation Water and Agricultural Soil Samples. PLoS One. 15, e0228956. doi:10.1371/journal.pone.0228956
Kong, R., Lee, S., Law, T., Law, S., and Wu, R. (2002). Rapid Detection of Six Types of Bacterial Pathogens in marine Waters by Multiplex PCR. Water Res. 36, 2802–2812. doi:10.1016/S0043-1354(01)00503-6
Krumperman, P. H. (1983). Multiple Antibiotic Resistance Indexing of Escherichia coli to Identify High-Risk Sources of Fecal Contamination of Foods. Appl. Environ. Microbiol. 46, 165–170. doi:10.1007/s11356-014-3887-310.1128/aem.46.1.165-170.1983
Kuhnert, P., Boerlin, P., and Frey, J. (2000). Target Genes for Virulence Assessment ofEscherichia Coliisolates from Water, Food and the Environment. FEMS Microbiol. Rev. 24, 107–117. doi:10.1111/J.1574-6976.2000.TB00535.X
Lerminiaux, N. A., and Cameron, A. D. S. (2019). Horizontal Transfer of Antibiotic Resistance Genes in Clinical Environments. Can. J. Microbiol. 65, 34–44. doi:10.1139/CJM-2018-0275/ASSET/IMAGES/CJM-2018-0275TAB1
Livermore, D. M. (1996). Are All Beta-Lactams Created Equal? Scand. J. Infect. Dis. Suppl. 101, 33–43. Available at: http://www.ncbi.nlm.nih.gov/pubmed/9060050 (Accessed June 13, 2019).
López-Saucedo, C., Cerna, J. F., Villegas-Sepulveda, N., Thompson, R., Velazquez, F. R., Torres, J., et al. (2003). Single Multiplex Polymerase Chain Reaction to Detect Diverse Loci Associated with DiarrheagenicEscherichia Coli. Emerg. Infect. Dis. 9, 127–131. doi:10.3201/eid0901.010507
Mafiz, A. I., Perera, L. N., He, Y., Zhang, W., Xiao, S., Hao, W., et al. (2018). Case Study on the Soil Antibiotic Resistome in an Urban Community Garden. Int. J. Antimicrob. Agents. 52, 241–250. doi:10.1016/j.ijantimicag.2018.05.016
Magiorakos, A.-P., Srinivasan, A., Carey, R. B., Carmeli, Y., Falagas, M. E., Giske, C. G., et al. (2012). Multidrug-resistant, Extensively Drug-Resistant and Pandrug-Resistant Bacteria: an International Expert Proposal for Interim Standard Definitions for Acquired Resistance. Clin. Microbiol. Infect. 18, 268–281. doi:10.1111/J.1469-0691.2011.03570.X
Maugeri, T. L., Carbone, M., Fera, M. T., Irrera, G. P., and Gugliandolo, C. (2004). Distribution of Potentially Pathogenic Bacteria as Free Living and Plankton Associated in a marine Coastal Zone. J. Appl. Microbiol. 97, 354–361. doi:10.1111/j.1365-2672.2004.02303.x
Moyo, S. J., Maselle, S. Y., Matee, M. I., Langeland, N., and Mylvaganam, H. (2007). Identification of Diarrheagenic Escherichia coli Isolated from Infants and Children in Dar Es Salaam, Tanzania. BMC Infect. Dis. 7, 92. doi:10.1186/1471-2334-7-92
Njage, P. M. K., and Buys, E. M. (2015). Pathogenic and Commensal E Scherichia Coli from Irrigation Water Show Potential in Transmission of Extended Spectrum and AmpC β‐lactamases Determinants to Isolates from Lettuce. Microb. Biotechnol. 8, 462–473. doi:10.1111/1751-7915.12234
Nontongana, N., Sibanda, T., Ngwenya, E., and Okoh, A. (2014). Prevalence and Antibiogram Profiling of Escherichia coli Pathotypes Isolated from the Kat River and the Fort Beaufort Abstraction Water. Ijerph 11, 8213–8227. doi:10.3390/ijerph110808213
O ’Neill, J. (2015). Antimicrobials in Agriculture and the Environment: Reducing Unnecessary and Waste. Rev. Antimicrob. Resist., 1–40. Available at: https://amr-review.org/sites/default/files/Antimicrobials in agriculture and the environment - Reducing unnecessary use and waste.pdf (Accessed January 12, 2018).
Paton, A. W., and Paton, J. C. (1998). Detection and Characterization of Shiga Toxigenic Escherichia coli by Using Multiplex PCR Assays for Stx 1 , Stx 2 , eaeA , Enterohemorrhagic E. coli hlyA , Rfb O111 , and Rfb O157. J. Clin. Microbiol. 36, 598–602. doi:10.1128/jcm.36.2.598-602.1998
Pesavento, G., Calonico, C., Ducci, B., Magnanini, A., and Lo Nostro, A. (2014). Prevalence and Antibiotic Resistance of Enterococcus Spp. Isolated from Retail Cheese, Ready-To-Eat Salads, Ham, and Raw Meat. Food Microbiol. 41, 1–7. doi:10.1016/j.fm.2014.01.008
Rojas-Lopez, M., Monterio, R., Pizza, M., Desvaux, M., and Rosini, R. (2018). Intestinal Pathogenic Escherichia coli: Insights for Vaccine Development. Front. Microbiol. 9, 440. doi:10.3389/FMICB.2018.00440
Schulz, F., Eloe-Fadrosh, E. A., Bowers, R. M., Jarett, J., Nielsen, T., Ivanova, N. N., et al. (2017). Towards a Balanced View of the Bacterial Tree of Life. Microbiome. 5, 140. doi:10.1186/S40168-017-0360-9/FIGURES/2
Sidhu, J. P. S., Ahmed, W., Hodgers, L., and Toze, S. (2013). Occurrence of Virulence Genes Associated with Diarrheagenic Pathotypes in Escherichia coli Isolates from Surface Water. Appl. Environ. Microbiol. 79, 328–335. doi:10.1128/AEM.02888-12
Söderström, A., Österberg, P., Lindqvist, A., Jönsson, B., Lindberg, A., Blide Ulander, S., et al. (2008). A LargeEscherichia coliO157 Outbreak in Sweden Associated with Locally Produced Lettuce. Foodborne Pathog. Dis. 5, 339–349. doi:10.1089/fpd.2007.0065
Stacy-Phipps, S., Mecca, J. J., and Weiss, J. B. (1995). Multiplex PCR Assay and Simple Preparation Method for Stool Specimens Detect Enterotoxigenic Escherichia coli DNA during Course of Infection. J. Clin. Microbiol. 33, 1054–1059. doi:10.1128/jcm.33.5.1054-1059.1995
Statssa (2021). Statistical Release P0302. Mid-year Population Estimates 2021. Available at: ,info@statssa.gov">www.statssa.gov.zaLGluZm9Ac3RhdHNzYS5nb3Y= (Accessed October 10, 2021).
Summerlin, H. N., Pola, C. C., McLamore, E. S., Gentry, T., Karthikeyan, R., and Gomes, C. L. (2021). Prevalence of Escherichia coli and Antibiotic-Resistant Bacteria During Fresh Produce Production (Romaine Lettuce) Using Municipal Wastewater Effluents. Front. Microbiol. 12, 1186. doi:10.3389/FMICB.2021.660047/BIBTEX
Taneja, N., and Sharma, M. (2019). Antimicrobial Resistance in the Environment: The Indian Scenario. Indian J. Med. Res. 149, 119. doi:10.4103/IJMR.IJMR_331_18
Titilawo, Y., Obi, L., and Okoh, A. (2015a). Antimicrobial Resistance Determinants of Escherichia coli Isolates Recovered from Some Rivers in Osun State, South-Western Nigeria: Implications for Public Health. Sci. Total Environ. 523, 82–94. doi:10.1016/j.scitotenv.2015.03.095
Titilawo, Y., Obi, L., and Okoh, A. (2015b). Occurrence of Virulence Gene Signatures Associated with Diarrhoeagenic and Non-diarrhoeagenic Pathovars of Escherichia coli Isolates from Some Selected Rivers in South-Western Nigeria. BMC Microbiol. 15, 204. doi:10.1186/s12866-015-0540-3
Titilawo, Y., Sibanda, T., Obi, L., and Okoh, A. (2015c). Multiple Antibiotic Resistance Indexing of Escherichia coli to Identify High-Risk Sources of Faecal Contamination of Water. Environ. Sci. Pollut. Res. 22, 10969–10980. doi:10.1007/s11356-014-3887-3
Torres, A. G. (2017). Escherichia coli Diseases in Latin America-a 'One Health' Multidisciplinary Approach. Pathog. Dis. 75, 12. doi:10.1093/FEMSPD/FTX012
Vidal, M., Kruger, E., Durán, C., Lagos, R., Levine, M., Prado, V., et al. (2005). Single Multiplex PCR Assay to Identify Simultaneously the Six Categories of Diarrheagenic Escherichia coli Associated with Enteric Infections. J. Clin. Microbiol. 43, 5362–5365. doi:10.1128/jcm.43.10.5362-5365.2005
Wijetunge, D. S. S., Gongati, S., DebRoy, C., Kim, K. S., Couraud, P. O., Romero, I. A., et al. (2015). Characterizing the Pathotype of Neonatal Meningitis Causing Escherichia coli (NMEC). BMC Microbiol. 15, 15–211. doi:10.1186/S12866-015-0547-9
Ye, Q., Wu, Q., Zhang, S., Zhang, J., Yang, G., Wang, H., et al. (2017). Antibiotic-Resistant Extended Spectrum SS-Lactamase- and Plasmid-Mediated AmpC-Producing Enterobacteriaceae Isolated from Retail Food Products and the Pearl River in Guangzhou, China. Front. Microbiol. 8, 1–12. doi:10.3389/fmicb.2017.00096
Keywords: environment, antibiotic resistance, public health, disease outbreak, microbial disease
Citation: Iwu CD, Kayode AJ, Igere BE and Okoh AI (2022) High Levels of Multi Drug Resistant Escherichia coli Pathovars in Preharvest Environmental Samples: A Ticking Time Bomb for Fresh Produce Related Disease Outbreak. Front. Environ. Sci. 10:858964. doi: 10.3389/fenvs.2022.858964
Received: 20 January 2022; Accepted: 21 February 2022;
Published: 17 March 2022.
Edited by:
Timothy Sibanda, University of Namibia, NamibiaReviewed by:
Joshua Mbanga, National University of Science and Technology, ZimbabweMagdalena Popowska, University of Warsaw, Poland
Copyright © 2022 Iwu, Kayode, Igere and Okoh. This is an open-access article distributed under the terms of the Creative Commons Attribution License (CC BY). The use, distribution or reproduction in other forums is permitted, provided the original author(s) and the copyright owner(s) are credited and that the original publication in this journal is cited, in accordance with accepted academic practice. No use, distribution or reproduction is permitted which does not comply with these terms.
*Correspondence: Chidozie Declan Iwu, Y2hpZG96aWVkZWxhbkBnbWFpbC5jb20=