- 1College of Economics and Management, Zhejiang Agricultural and Forestry University, Hangzhou, China
- 2Department of Economics and Management, Qianjiang College of Hangzhou Normal University, Hangzhou, China
Given the overlapping characteristics of poverty-stricken counties within key ecological function zones and ecologically fragile areas in China, this study takes the sample of 393 state-designated poverty-stricken counties from 2011 to 2018 and uses the differences-in-differences model to test the influence of forest resource protection on economic growth and its mechanism under the carbon neutrality target. The empirical research proves how to break the “green paradox” and “poverty curse” in the process of Chinese ecological civilization construction and targeted poverty alleviation and how policy tools integrate emission reductions with carbon-sequestration increase and achieve sustainable development. The results show that 1) the economic growth of poverty-stricken counties cannot be separated from industrialization, urbanization, and financial system; 2) China’s poverty-stricken counties have achieved an initial decoupling of carbon emissions from economic growth; 3) forest resource protection represented by forest coverage and stock changes the carbon emissions through emission reductions with carbon-sequestration increase and affect the economic growth of poverty-stricken counties; and 4) the reduction of total carbon emissions in poverty-stricken counties further enhances the role of forest resource protection in promoting county economic growth. Taking the 13th Five-Year Plan for eco-environment protection as the starting point, this research reveals different paths and impacts selected by Chinese local governments in poverty alleviation.
1 Introduction
The development of a county’s economy is an important basis for the prosperity of the rural economy and the development of small towns and also an important way to absorb the transfer of the surplus rural labor force and increase farmers’ income. Since 2012, all 832 poverty-stricken counties and 98.99 million rural poor people in China have been lifted out of poverty1. In 2020, the per capita disposable income of rural residents in poor areas was 12,588 yuan, an increase of 8.8% over the previous year, showing great vitality in the economic development of poverty-stricken counties2. However, economic growth is not sufficient for poverty reduction. If growth is at the cost of excessive exploitation and uneven distribution of resources, it will cause prominent ecological damage, unsustainable economic development, and hinder the effectiveness of poverty reduction.
China has clearly defined the goal of “carbon neutrality before 2060,” which will also produce extensive and profound changes to forest ecology, environmental quality, and economic development of poverty-stricken counties (Cai et al., 2021; Shi et al., 2022). Most of China’s poverty-stricken counties overlap geographically with ecologically fragile areas and key ecological function areas (Liu et al., 2017). Against the background of the overall reduction in global forest resources, China’s forest coverage has increased from 12.7% in the early 1970s to 23.04%. The forest area and forest stock have maintained a two-fold growth for more than 30 consecutive years. The total carbon reserves of forest vegetation are 9.2 billion tons, with an average annual growth of more than 200 million tons, which is equivalent to 700 million to 800 million tons of carbon sequestration. But forest coverage is still below the global average of 31%, and forest stock per unit area is only 78% of the global average3. Forest carbon sequestrations play an increasingly important role in realizing carbon neutrality targets, and poverty-stricken counties have high potential to increase carbon sequestration in forest resource protection.
Forest ecological products and services are mostly public goods and often need government policy intervention to reverse market failure in the allocation of forest ecological resources. For the first time, China has put forward the document of “poverty” and “ecology” at the national level dating back to the year 1994 “the program of state’s storming the fortifications in the work of helping the poor (1994–2000).” The decision of the CPC Central Committee and the State Council on Winning the Battle against Poverty issued in 2015 further proposed to give ecological protection priority to poverty alleviation and development. The restrictive indicators of forest coverage and forest stock in ecological and environmental protection planning are of great significance to the balance among economic development, ecological, and environmental protection in poverty-stricken counties. However, ecological and environmental protection planning and economic development planning are inconsistent in terms of goals, tasks, and paths. Whether the forest resource protection to improve forest functions and efficiency could inhibit the economic growth of poverty-stricken counties? How does the forest resource protection act on the economic growth of poverty-stricken counties? Answering the abovementioned questions can contribute to the spillover test of the effectiveness of forest resource protection policies and provide evidence for the role of the government’s “visible hand” in promoting the sustainable economic development of poverty-stricken counties under the carbon neutrality target.
This article takes the state-designated poverty-stricken counties as an example and makes the 13th Five-Year Plan for eco-environment protection (2016–2020) issued by each province in China as a quasi-natural experiment. The statistical data of 393 state-designated, poverty-stricken counties in 12 provinces and municipalities from 2011 to 2018 are selected, testing the effect and mechanism of forest resource protection policy on the economic growth of poverty-stricken counties.
2 Literature Review and Theoretical Analysis
2.1 Literature Review
2.1.1 Industry and Regional Development Under the Carbon Neutrality Target
According to the definition of the Intergovernmental Panel on Climate Change (IPCC), carbon neutrality is a state of balance between the CO2 emitted into the atmosphere and the CO2 removed from the atmosphere4. From the perspective of economic connotation, carbon neutrality is the realistic demand of China’s economic low-carbon transformation and high-quality development (Zhang et al., 2021; Wang et al., 2022a), and it is also in line with the decoupling theory (OECD, 2002) and the environmental Kuznetz curve (EKC) hypothesis (Song et al., 2019; Ahmad et al., 2021; Wang et al., 2022b). The EKC hypothesis has certain limitations. First, it is not enough to explain the entire relationship between environmental quality and income level. Second, it cannot be applied to all environmental indicators, and some environmental damage is difficult to measure. Third, environmental problems at different stages have different relationships with economic growth. Economic development does not necessarily ultimately bring about environmental improvement, and the belief that economic growth will eventually automatically solve environmental problems is overly optimistic and unfounded (Soumyananda, 2004). There are more studies on carbon neutrality at the regional scale. From the perspective of the industry scale, the emission reduction path of power and transportation departments has been the focus of research (Zhang and Chen, 2021; Zhang and Hanaoka, 2021). In addition, low-carbon technology studies in agriculture, construction, and chemical industries have become a new focus in recent years (Li et al., 2019; Yin et al., 2022). Furthermore, there are relatively few studies on the characterization of the mutual feedback of society, economy, and ecosystem under the carbon neutrality target. The research on various policies under the carbon neutrality target and the synergy between policies and sustainable development are not deep enough (Wu et al., 2022). On the regional scale, the research on economic development of poverty-stricken counties under the carbon neutrality target has insufficient consideration in the role of forest carbon sequestration in tackling climate change (Yin et al., 2022). Due to the overlapping backgrounds of the carbon neutrality target and targeted poverty alleviation, it is bound to cause new economic development problems in poverty-stricken counties. This study verifying the influence of forest resource protection on county economic development mechanisms will help the plan for eco-environment protection anchor the carbon neutrality target and achieve sustainable development of poverty-stricken counties.
2.1.2 Environmental Effects and Spatiotemporal Variation in Forest Resource Protection
Plan for eco-environment protection is the main driving force of the environmental effect and an important part of the county’s social and economic system (Hepburn et al., 2021). The transformation of the industrial structure led by ecological civilization construction and environmental quality objectives is conducive to the efficient utilization of resources and the optimization of production technology and management methods (Kesidou and Wu, 2020). Most of the existing literature is the research on the environmental policy effect or environmental regulation efficiency (De Souza et al., 2021; Li et al., 2021; Zengagàn-Castaño et al., 2022), while the research on the strategic deployment, such as forest coverage and forest stock, is relatively few (Arndt et al., 2021; Liu and Xia, 2021). From theoretical and empirical research studies, some scholars concluded about the “green paradox,” that is, the environmental policy for emission reduction and carbon-sequestration increase can, instead, lead to the increase of pollution emissions. From the time dimension, the environmental policy directly or indirectly promotes the cost of pollution emissions in the future, which accelerates the mining of fossil energy producers and increases pollution emissions (Hamamoto, 2006; Ulucak and Khan, 2020). From the spatial dimension, the unilateral environmental policy may lead to pollution transfer into a new “pollution paradise,” with increased overall carbon emissions on a larger scale (Levinson and Taylor, 2008). While combined with the process of poverty alleviation in China’s poverty-stricken counties, the “green paradox” may have another theoretical mechanism: in the realization of the new county urbanization process with intensive and efficient production space, moderately livable living space, harmonious and secure ecological space, the allocation of land resources has not yet reached the optimal state (Guo and Liu, 2021) where industrialization, agricultural modernization, and urbanization are not coordinated (Li et al., 2022). The characteristic industries and leading industries supporting the development of the county’s economy need to be excavated or transformed into low-carbon industries. Combining the abovementioned facts with limited financial funds and weak infrastructure and public service conditions, it is reported that China’s poverty-stricken counties are generally faced with contradiction between poverty alleviation and decarbonization (Yuan and Wang, 2021), which makes the plan for eco-environment protection for the county, especially the quantity control, fall into the institutionalized poverty trap (Arndt et al., 2021).
2.1.3 The Decoupling Mechanism of Carbon Emissions From Economic Growth
Decoupling is the basic theory proposed by OECD to describe the blocking of the link between economic growth and resource consumption or environmental pollution (Grossman and Krueger, 1995; Kube et al., 2018). According to the environmental Kuznetz curve (EKC) hypothesis, economic growth generally brings increased environmental pressure and resource consumption, but when some effective policies and new technologies are adopted, lower environmental pressure and resource consumption may be exchanged for equal or even faster economic growth (Ahmad et al., 2021). This process is also known as “decoupling” (Li et al., 2016; Sun et al., 2020). There are few studies on the decoupling relationship, influencing factors, and the rebound effects of carbon emissions and economic development in various regions and industries (Boyd and Mcclelland, 1999; Jalil and Feridun, 2011; Abdulai and Abdulai, 2017); nevertheless, there are less studies on the internal mechanism of poverty reduction through the development path of “low-carbon economy,” which could break the “resource curse” and make poor areas with rich forest resources and ecological capital get out of the dilemma of low industrialization, difficult industrial transformation, and excessive dependence on a single economic structure (Adams et al., 2016). What is even more concerning is that poverty-stricken counties in China have all been lifted out of poverty by 2020, and according to the seventh national census bulletin in 2020, the rural population decreased by 14.21% compared with the sixth national census in 2010. Under the current situation, that poverty has been eliminated and rural population growth has slowed down, will the decoupling mechanism of carbon emissions from economic growth be adjusted? Among them, what roles do the industrial structure, urbanization rate, science, education, culture, and health, and other factors play? To explore the causal relationship of the abovementioned factors, we can also find the crux and application point of the future sustainable development of county economy under the carbon neutrality target.
Most scholars focus on the emission reduction effect and economic effect of environmental policy, which provide references for this study. While no unanimous conclusion can be drawn from that whether more quantity control of plan for eco-environment protection creates a “green paradox” or a “resource curse” in poor areas, in the context of carbon neutrality targets and the post-poverty alleviation era, the research on the economic growth of poverty-stricken counties also reflects the coupling relationship of the “ecology–environment–economy–social” system and the internal mechanism of ecological and environmental protection policies on sustainable economic development by the purpose of protecting the ecological environment and limiting carbon emissions. It is also worthy for the policymakers to comprehensively consider the policy coordination and regional differences in the post-poverty alleviation era. Therefore, this study starts from the county level and uses a differences-in-differences model to analyze the influence mechanism of forest resource protection on the economic growth of poverty-stricken counties to overcome endogeneity caused by measurement error and reverse causality.
2.2 Theoretical Analysis
As early as the 1990s, scholars began to discuss the mutual relationship between the economy and environment in poverty-stricken areas (Cai and Barry, 1994; Byrne et al., 1996; Rozelle et al., 1997). There is a lot of literature on the impact of economic development on environmental pollution, and “income determinism” is in the dominant position. The “environmental poverty trap” changes the carbon elements from constraints to production factors, which affects economic growth and total factor productivity (Liuzzi and Venturi, 2021); and the overlapping generation model also reveals the logic of distorted resource allocation hindering sustainable economic development (Guruswamy Babu et al., 1997). Thus, public policy plays an important role in protecting the ecological environment and optimizing resource allocation and also has an impact on sustainable economic development (Wang et al., 2020; Hajjar et al., 2021). The existing policy research under the carbon neutrality targets mainly starts from the mechanism of social capital in resource allocation and focuses on the emission reduction path of carbon emission and the incentive mechanism innovation such as green technological reform and green credit (Yang et al., 2021a; Castle and Miller, 2021). But the forest, as natural capital, should also show its due meaning in resource allocation. Although there are detailed restrictive indicators such as forest coverage and forest stock in the plan for eco-environment protection, the poverty-stricken counties rich in forest resources have not formed a perfect top-level design for the carbon neutrality path. For example, under the carbon neutrality target, does the plan for eco-environment protection affect the county’s economic growth through the change of carbon emission? This part mainly explores the abovementioned theoretical mechanism.
2.2.1 Impact of Eco-Environment Protection on the Economic Growth
China’s poor areas carry the vast majority of the absolute poor population, with serious damage to the living environment and low natural recovery capacity (Xiang et al., 2021). The forest coverage and forest stock in the 13th Five-Year Plan for eco-environment protection pose a rigid constraint on the economic development of poverty-stricken counties, and the extensive development with high energy consumption needs to be transformed into intensive with high efficiency (Yang et al., 2021b). Meanwhile, the proposal of comprehensive poverty alleviation and carbon neutrality target has also become the background of the new era of poverty-stricken counties. Guided by the plan for eco-environment protection, the poverty-stricken counties tried to turn ecological resource advantages into ecological value advantages. Some poor areas go downhill to lift themselves out of poverty and develop in different places, which are conducive to the construction of intensive village infrastructure and public and expand the living and employment space of the poor groups and accelerate urbanization (Yang et al., 2020). Some poor areas make reasonable use of capital to explore ecological industries (Lei et al., 2021), properly handle the relationships among tradition and innovation, and coordinate ecological protection and poverty alleviation (Shuai et al., 2021). These explorations form a virtuous circle of the eco-economic system, which not only breaks the curse of “following the cost theory” and jumps out of the “environmental poverty trap” but also promotes industrial revitalization, ecological construction, and sustainable development of poverty-stricken counties.
2.2.2 Conduction Effect of County Carbon Emissions
The constraints on forest coverage and forest stock in the plan for eco-environment protection have direct and indirect impacts on carbon emissions. In terms of direct impacts, according to the study of the Chinese Academy of Sciences in 2018, land ecosystems fixed 201 million tons of carbon per year from 2001 to 2010 in China, equivalent to offsetting 14.1% of domestic carbon emissions in the same period. Among them, the forest ecosystem contributes about 80% of carbon sequestration, offsetting 11.28% of domestic carbon emissions in the same period (Tang et al., 2018). The indirect impact is to accelerate the R&D of low-carbon technologies and elimination of backward production capacity to protect the forest ecosystem, which forms the adsorption effect on the green industry and the extrusion effect of the “two high” industries. On the whole, it is conducive to reducing county carbon emissions (Sharma et al., 2013; Peng et al., 2021). Wherever carbon offset or carbon reduction is present, the constraints of forest coverage and forest stock in the plan for eco-environment protection affect the economic growth of the poverty-stricken counties through the transmission of carbon emissions. The reduction of carbon emissions means the improvement of environmental quality and social welfare. It has also become the driving force for the optimal allocation of resources and the transformation of industrial structure (Lin and Ge, 2019). Under the carbon neutrality target, poverty-stricken counties may also show a decoupling relationship between carbon emissions and economic growth (Jin et al., 2020).
3 Research Design
The two restrictive indicators of forest coverage and forest stock in the 13th Five-Year Plan for eco-environment protection in all provinces are regarded as quasi-natural experiments, and the differences-in-differences model is adopted to test the influence of forest resource protection on economic growth in poverty-stricken counties.
3.1 Models and Variables
The basic idea of the differences-in-differences model is to construct the double-difference statistics reflecting the policy effect by comparing the differences between the control group and treatment group before and after the implementation of the policy (Heckman and Robb, 1985). The model in this study draws lessons from the research by Kesidou et al. (Kesidou and Wu, 2020). First, it effectively avoids endogenous problems. Compared with microeconomic subjects, the binding indicators of the 13th Five-Year Plan for eco-environment protection are exogenous, and there is no reverse causality problem. Second, the fixed-effect estimation is used to reduce the bias from missing variables. Third, the traditional method to evaluate the policy effect is mainly by setting a dummy variable of whether the policy occurs or not, and then regression. In contrast, the differences-in-differences model can estimate the policy effect more accurately (Meyer, 1995). The benchmark regression model is as follows:
In Eq. 1, i and t indicate regions and years, respectively. Y indicates the county economic growth, with the logarithm of the GDP (lnGDP) and the logarithm of the per capita GDP (lnpGDP), aiming to deal with the heteroscedasticity in the regression equation and contribute to the robustness of the results. Moreover, treat and time represent virtual variables. treat = 1 indicates that the poverty-stricken county is constrained with forest coverage and forest stock constraints in the 13th Five-Year Plan for eco-environment protection; treat = 0 indicates that there is no forest coverage or forest stock constraint in the 13th Five-Year Plan for eco-environment protection in the province to which the poverty-stricken county belongs. Since the 13th Five-Year Plan for eco-environment protection started in 2016, time = 1 indicates that the abovementioned plan has been implemented in 2016 and after, and time = 0 indicates that the abovementioned plan has not been implemented in 2015 and before. α0, α1, and α2 are unknown parameters.
Control is a series of control variables, including the number of above-scale industrial enterprises in the county (Industry) to represent the level of industrial development, the proportion of the nonfarm population (Urbanization) to reflect the urbanization rate, the proportion of fixed asset investment in GDP (Investment) to reflect the fixed asset investment rate, the per capita loan balance of financial institutions (Finance), proportion of primary and secondary school students in the total population (Education), proportion of local financial budget revenue and expenditure in GDP (Government), the number of beds in medical institutions (Medical), the total agricultural machinery power (Agricultural), and the proportion of the secondary industry in GDP (Sec_stru). λt is the time fixed-effect, which reflects time characteristics and replaces the original policy implementation variables; μi implies regional fixed-effects that do not change over time, which reflects regional characteristics and replaces the original regional grouping variables. So, monomials of treat and time are not included in the model. εit is the stochastic component.
To further study the influence mechanism of forest resource protection on the economic growth of poverty-stricken counties, the following mediation effect model is set up as follows:
where the intermediary variable Mit refers to carbon emission, which is expressed as a logarithm of total carbon dioxide emission (lnCO2) (Chen J et al., 2020). βn (n = 0, 1, and 2) and γn (n = 0, 1, 2, and 3) are all parameters to be estimated. α1 represents the overall effect of forest resource protection policies; β1 represents the effect of forest resource protection policies on carbon dioxide emissions. γ2 represents the effect of carbon dioxide emissions on the economic growth of poverty-stricken counties.
3.2 Data Source and Description Statistics
We collect 12 copies of the 13th Five-Year Plan for eco-environmental protection publicly released on the official websites of all provinces or municipalities, including Chongqing, Jilin, Hunan, Shanxi, Yunnan, Shaanxi, Tibet, Anhui, Gansu, Henan, Heilongjiang, and Sichuan. This provided conditions for setting up the treatment and control groups, and then, two restrictive indicators of forest coverage and forest stock are extracted from the abovementioned documents, and two groups are distinguished between provinces involved and not involved in the abovementioned two restrictive indicators. Last, the state-designated poverty-stricken counties within the two types of provinces such as those mentioned above are used as the treatment and control groups, respectively (Table 1).
This study analyzes the social, economic, and natural environment statistics in poverty-stricken counties in 2011–2018. The basic county-level data are taken from the Chinese County Statistical Yearbook, matched with 832 national key counties for poverty alleviation and development work of the Poverty Elimination Research database (https://www.jianpincn.com). CO2 emission data are obtained from the estimates published by Chen et al. (2020)5. We organized different sources of data, eliminated serious missing data, supplemented data with the interpolation method, and finally selected 393 state-designated poverty-stricken counties. Among them, 137 counties with restrictive indicators of forest coverage and forest stock in the 13th Five-Year Plan for eco-environmental protection belong to the treatment group, and 256 counties that do not have the previous two restrictive indicators at the same time belong to the control group. Only the short-term effects of the plan for eco-environmental protection are studied here; the longer-term effects need to be verified by additional data. Under the control of other factors, the policy effects of forest coverage and forest stock are evaluated by comparing the economic growth of the treatment and control groups.
Variable description statistics are shown in Figure 1 and Table 2 from 2011 to 2018. The average annual GDP of 393 state-designated poverty-stricken counties was 7.201 billion, and the average per capita GDP was 20,700 yuan. Despite the steady economic growth year by year, the overall economic scale and investment competitiveness of 393 state-designated poverty-stricken counties were low among nearly 2,000 counties. The average number of industrial enterprises above the designated size in the 393 counties is 33.97, which is still in the process of transformation to industrialization, and the urbanization level is also low. The average fixed asset rate at the county level is 114.5%, and the extremely low investment efficiency shows that the poverty-stricken counties conduct infrastructure construction using a large amount of fixed asset investment and reflects the weak economic foundation and low capacity to form GDP. Education is an inexhaustible driving force for economic growth. The ratio of the number of primary and secondary school students and the total population in poverty-stricken counties is on an average of 0.120, slightly higher than the national average level of 0.115 in the same period. This reflects the future demographic dividend and industrial new force. While considering the attraction of future cities to talents, it also shows the pressure of “retaining talents” in the development of poverty-stricken counties. The ratio of local fiscal budget to GDP reflects the degree of government intervention and ability to maintain rapid and stable economic growth. The ratio of local budget revenue and GDP was 55.3%. The number of beds in medical institutions has increased by 90% over the study year, on an average of 1,263 sheets per county. The average level of agricultural mechanization represented by the total power number of agricultural machinery was 327,300 W in the sample poverty-stricken counties. The secondary industry accounts for an average of 36.6% of GDP. The industrial structure and industrial development affect the county economy, and it is also an opportunity to cultivate and develop ecological industries based on the resource endowment of poverty-stricken counties.
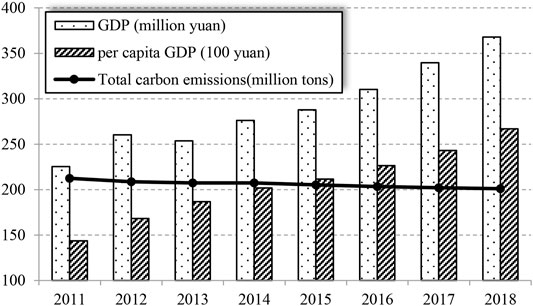
FIGURE 1. Changes in carbon emissions, GDP, and per capita GDP of poverty-stricken counties (2011–2018).
4 The Impact of Forest Resource Protection on the Economic Growth of Poverty-Stricken Counties
4.1 Benchmark Regression Model Results
By Hausman test, the results of the benchmark regression model pass the 1% significance test. Therefore, the region and year fixed-effect model was selected to test the effect of forest resource protection on the economic growth of poverty-stricken counties, and the regression results are shown in Table 3. Among them, the regression coefficients are all significant at the level of 1%. Given the possible multicollinearity problem of control variables, we use the VIF to diagnose whether there is multicollinearity. The test results show that the VIF of all explanatory variables is less than 5, that is, there is no multicollinearity between control variables. The influence coefficient of forest resource protection on GDP and per capita GDP is positive, which shows that the two restrictive indicators (forest coverage and forest stock) in the plan for eco-environment protection does not inhibit the economic growth but has a significant role in the economic growth of poverty-stricken counties. This confirms the research result of Lei et al. (2021) from the macro level, that is, China has achieved a win–win situation of poverty alleviation and ecological and environmental protection. The poverty-stricken counties with the abovementioned two restrictive indicators are 0.881% higher in GDP and 0.316% higher in per capita GDP. After the inclusion of control variables in the regression, the cross-term coefficient is still significantly negative, but the value is smaller, that is, the real impact of forest resource protection on GDP and GDP per capita in poverty-stricken counties is smaller than when the control variables were not included.
Model (1) and (2) and Model (4) and (5) use GDP and per capita GDP as dependent variables, respectively, with only the difference between the per capita loan balance of financial institutions (Finance) and the difference between the number of students in primary and secondary schools and the total population (Education). In addition, the level of industrial development, urbanization rate, loan scale of financial institutions, and proportion of industrial output value have a significant positive impact on the economic growth of poverty-stricken counties, and it reflects that the weaker the financial capacity of local governments, the stronger the economic growth of poverty-stricken counties. Some studies also show that the local fiscal revenue and expenditure of the poverty-stricken counties in China has not effectively promoted local economic development (Huang, 2018). The possible reason is that the local finance of poverty-stricken counties is weak, and there are often special funds for poverty alleviation or central finance, which plays an important role in economic growth. From the statistical description, the average investment rate of fixed assets in the sample counties exceeds 100%, indicating that the investment efficiency is very low. Combined with the results in Table 3, we can obtain results similar to the relevant research (Qin and Song, 2009). The loss of investment efficiency of fixed assets and the trend of over-investment in poverty-stricken counties in China have become the main problems restricting economic growth.
4.2 Robustness Test
Based on the benchmark regression model, from the model assumptions and data, the robustness test is carried out in the following three aspects:
The differences-in-differences (DID) method is effective, provided that the control and treatment groups need to meet the common trend hypothesis. If the treatment group is not treated, the same changes occur with the control group. The abovementioned is a counterfactual hypothesis. If there is only a difference between the treatment group and control group is whether to receive treatment and it is the same in other aspects, then this assumption is naturally true. Figures 2, 3, respectively, show the changes in GDP and per capita GDP of poverty-stricken counties before and after the implementation of the 13th Five-Year Plan for eco-environment protection. First, the definition of the treatment and control groups is consistent with the requirement of the DID model. Second, the growth trends of GDP and per capita GDP in the treatment group and control group are similar from 2011–2015, before the implementation of the 13th Five-Year Plan for eco-environment protection. Third, the growth trends are not the same between the treatment and the control groups from 2016 to 2018, after the implementation of the 13th Five-Year Plan for eco-environment protection. This discrepancy may arise from the implementation of forest resource conservation policies. It can be seen that forest resource protection promotes the economic growth of poverty-stricken counties, and the sample fits the parallel trend hypothesis.
The placebo test is used to test whether the baseline regression results are random, that is, whether the same results would be obtained without the 13th Five-Year Plan for eco-environment protection. To this end, the 13th Five-Year Plan for eco-environment protection is implemented for 1 year (2015) and 2 years (2014) in advance, and then, DID regression was used. Models (7)–(10) in Table 4 report the results from the placebo test. The core coefficients of explanatory variables are insignificant 1 or 2 years before the policy implementation. Thus, the placebo test has passed and confirmed the robustness of the benchmark regression results in this study.
The treatment and control groups are matched by the propensity score matching (PSM) method to eliminate the possible sample selection bias in the impact of the plan for eco-environment protection. After meeting the balance test, the level of industrial development, fixed asset investment rate, etc. are used as matching variables and made kernel matching with logit models. The results are shown in Table 5. The coefficients of the matching models (12) and (14) were higher than those of the models (3) and (6), respectively. This illustrates that the sample characteristics of matched groups are closer, confirming that the forest resource protection policy significantly promotes the growth of GDP and per capita GDP in poverty-stricken counties and verifies the robustness of the regression results in this article.
5 Mediating Effect of Carbon Emissions in Poverty-Stricken Counties
The abovementioned study confirms the positive role of forest resource protection in the economic growth of poverty-stricken counties, while in what ways do the restrictive indicators of forest cover and forest stock affect the GDP and per capita GDP of poverty-stricken counties? Based on the theory of this study, there may be mediating effects of carbon emissions in poverty-stricken counties: 1) the forest resource protection policy accelerated carbon neutralization; 2) carbon fixation and oxygen release of forest and industrial green transformation reduce the county carbon emissions; and 3) ecological enrichment and the low-carbon economic development path promote the optimal allocation of resources in poverty-stricken counties and unleash more dividends.
Whether there is a mediating effect depends on the significance test of Eqs 1–3. If α1 and β1 are significant, it indicates the existence of the mediating effect. At this point, if γ1 is not significant, it indicates full mediation; if γ1 is significant and β1 and γ2 have the same sign, there is partial mediation; if γ1 is significant and β1γ2 and γ1 have the different signs, there is suppressing effects (Kenny et al., 2003).
5.1 The Role of Forest Resource Protection on Carbon Emissions
We test whether forest resource protection has a direct effect on carbon emissions. The results (as in Table 6) demonstrate that forest resource protection policies help reduce carbon emissions in poverty-stricken counties. After the inclusion of control variables, the coefficient β1 declines slightly, which confirms the robustness of the estimated results. It also shows that forest resource conservation has a suppressive effect on carbon emissions, which confirms the research results of Lin and Ge. (2019). The two restrictive indicators of forest cover and forest stock in the 13th Five-Year Plan for eco-environment protection achieve the carbon neutrality targets, which have the dual effects of increasing carbon sequestration and reducing emissions.
5.2 The Role of Forest Resource Conservation on Economic Growth Through Carbon Emissions
The test results of the conduction effect of county carbon emissions on economic growth are shown in Table 7. After the inclusion of carbon emissions in the model, forest resource protection is still playing a significant and positive role on the GDP and per capita GDP in poverty-stricken counties. The impact of carbon emissions on economic growth passes the test of significance at 1% level, and it has a positive coefficient, opposite to the coefficient sign of the direct effect. This represents that carbon emissions have a suppressing effect between forest resource protection and economic growth in poverty-stricken counties. By the bootstrapping test, 95% confidence intervals are (0.1261,0.1861) and (0.2078,0.2709), excluding zero and significant at 1% level, indicating the existence of the suppressing effect. The suppressing effect accounts for 7.07 and 1.18% of the direct effect (|β1γ2/γ1|), all less than one. Thus, the suppressing effect does not change the promoting role of forest resource protection on the GDP and per capita GDP in poverty-stricken counties.
From the benchmark regression of forest resource protection on economic growth in poverty-stricken counties, the total effects are 0.0985 and 0.165. But, in fact, this is the result of being “covered up” by carbon emissions. If the variable of carbon emission is controlled, the direct effects are 0.106 and 0.167, and the influence is heightened when the coefficient values increase. As the coefficient is closer to the real result after stripping the suppressing effect, it is concluded that if the total carbon emissions decrease in poverty-stricken counties, the forest resource protection policy will play a more positive role in promoting the increase of county economy.
The test results confirmed the mediating effect of carbon emissions, that is, the constraints on forest coverage and forest stock promote the growth of GDP and per capita GDP in poverty-stricken counties by reducing carbon emissions. Combined with the positive role of China’s forestry policies in promoting farmers to get rid of poverty (Lu et al., 2020), forest resource protection plays to the advantage of forest resources and reflects the value of ecological capital. Therefore, the restrictive indicators do not inhibit economic growth but help achieve coordinated development among ecological civilization, rural revitalization, and common prosperity.
6 Conclusions and Implications
This study analyzes the influence and its mechanism of forest resource protection on the county economy. The following are conclusions:
1)Forest resource protection does not only rely on the 13th Five-Year Plan for eco-environment protection, and it also does not take reducing carbon emissions as the specific goal or carbon neutrality as the overall goal. The restrictive indicators of forest coverage and forest stock are not policy tools to directly promote the economic growth of poverty-stricken counties, while these representing the forest resources protection are proven not to inhibit the county economic growth. That breaks the curse of “following the cost theory” and jumps out of the “environmental poverty trap,” and contributes to the sustainable development of the county.
2) The economic growth of poverty-stricken counties cannot leave industrialization, urbanization, and financial support. But in the short term, the effect of basic education and agricultural mechanization on the county economy is not sufficient. The basic social security and public services, ecological civilization construction, and industrial structure transformation complement each other, all of which play an important role in the governance of the endogenous development ability fragile.
3) Under the carbon neutrality target, carbon emission plays the mediating role in the positive impact of forest resource protection on the economic growth of poverty-stricken counties. The suppressing effect of carbon emissions illustrates that the decrease of the total carbon emissions in the poverty-stricken counties can enhance the promotion of the forest resource protection policy on county economy and realize the synergy of poverty reduction and emission reduction, and the internal decoupling mechanism of county carbon emissions and economic growth has also been verified.
Based on the research of this article, local governments should pay attention to planning first in the protection of forest resources, transforming the advantages of ecological resources into ecological values of the poverty-stricken counties. The starting points in the post-poverty alleviation era are the innovation of the poverty alleviation system and upgrading of low-carbon industries, and the guarantee includes the improvements of infrastructure and public service. To further enhance the economic and ecological environmental effects of forest resource protection policies, it is essential to improve the market mechanism of ecological products and bring green GDP into the assessment standards of the government and industries.
The limitation of this study is that the mediating variable inevitably has endogenous problems. Therefore, the effect of carbon emissions on GDP depends on the relative theory and practice, to be convincing. This study combines the specific problems in the development process of poverty-stricken counties in China under the carbon neutrality target to reduce the rote application of methods in mechanism analysis. What can be further discussed is the heterogeneity of the long-term effects of different forest resource protection policies on the economic growth of poverty-stricken counties to prevent elite capture and targeting deviation in the process of emission reduction and poverty reduction.
Data Availability Statement
The original contributions presented in the study are included in the article/Supplementary Material, further inquiries can be directed to the corresponding author.
Author Contributions
Conceptualization, HQ and LS; methodology, LS and FL; formal analysis, HQ, XG, and LH; writing—original draft preparation, HQ; writing—review and editing, HQ and FL. All authors have read and agreed to the published version of the manuscript.
Funding
This manuscript was supported by the National Natural Science Foundation of China (Grant Nos. 71473230 and 71803180) and the Philosophy and Social Sciences Planning Project of the Ministry of Education in China (Grant Nos. 18YJCZH140 and 17YJCZH048).
Conflict of Interest
The authors declare that the research was conducted in the absence of any commercial or financial relationships that could be construed as a potential conflict of interest.
Publisher’s Note
All claims expressed in this article are solely those of the authors and do not necessarily represent those of their affiliated organizations, or those of the publisher, the editors, and the reviewers. Any product that may be evaluated in this article, or claim that may be made by its manufacturer, is not guaranteed or endorsed by the publisher.
Footnotes
1All 832 poor counties were lifted out of poverty-International Online (cri.cn).
2The Statistical Bulletin of the 2020 National Economic and Social Development, released on the website of the National Bureau of Statistics, on 28 February 2021.
3Realize carbon neutralization Large forest action (people.com.The cn), People’s Daily (14 January 2021).
4IPCC, Special Report on Global Warming of 1.5°C, 2018, https://www.ipcc.ch/sr15/.
5Chen, Jiandong; Gao, Ming; Cheng, Shulei; Hou, Wenxuan; Song, Malin; Liu, Xin; et al. (2020) County-level CO2 emissions and sequestration in China. doi: 10.6084/m9.figshare.c.5136302.v.
References
Abdulai, A.-N., and Abdulai, A. (2017). Examining the Impact of Conservation Agriculture on Environmental Efficiency Among maize Farmers in Zambia. Envir. Dev. Econ. 22 (2), 177–201. doi:10.1017/s1355770x16000309
Adams, C., Rodrigues, S. T., Calmon, M., and Kumar, C. (2016). Impacts of Large-Scale forest Restoration on Socioeconomic Status and Local Livelihoods: what We Know and Do Not Know. Biotropica 48 (6), 731–744. doi:10.1111/btp.12385
Ahmad, M., Muslija, A., and Satrovic, E. (2021). Does Economic prosperity lead to Environmental Sustainability in Developing Economies? Environmental Kuznets Curve Theory. Environ. Sci. Pollut. Res. 28 (18), 22588–22601. doi:10.1007/s11356-020-12276-9
Arndt, F., Arun, R., Hans, L., Klas, S., and Harald, G. (2021). Policies to Reconcile forest Conservation and Rural Development: A Pathway to Bridge the forest Transition in Bhutan? Land Use Policy 109, 105647.
Boyd, G. A., and Mcclelland, J. D. (1999). The Impact of Environmental Constraints on Productivity Improvement in Integrated Paper Plants. J. Environ. Econ. Manag. 38 (2), 121–142. doi:10.1006/jeem.1999.1082
Byrne, J., Shen, B., and Li, X. (1996). The challenge of Sustainability. Energy Policy 24 (5), 455–462. doi:10.1016/0301-4215(96)00011-0
Cai, W. X., He, N. P., and Li, M. X. (2021). Carbon Sequestration of Chinese Forests from 2010 to 2060: Spatiotemporal Dynamics and its Regulatory Strategies. Sci. Bull. doi:10.1016/j.scib.2021.12.012
Cai, Y. L., and Barry, S. (1994). Sustainability in Chinese Agriculture: challenge and hope. Agric. Ecosyst. Environ. 49 (3), 279–288.
Castle, S. E., and Miller, D. C. (2021). The Impacts of Agroforestry Interventions on Agricultural Productivity, Ecosystem Services, and Human Well-Being in Low- and Middle-Income Countries: a Systematic Review. Campbell Syst. Rev. 17, e1167. doi:10.1002/cl2.1167
De Souza, V. M., Bloemhof, J., and Borsato, M. (2021). Assessing the Eco-Effectiveness of a Solid Waste Management Plan Using Agent-Based Modelling. Waste Manag. 125, 235–248. doi:10.1016/j.wasman.2021.02.019
Grossman, G. M., and Krueger, A. B. (1995). Economic Growth and the Environment. Q. J. Econ. 110 (2), 353–377. doi:10.2307/2118443
Guo, Y., and Liu, Y. (2021). Poverty Alleviation through Land Assetization and its Implications for Rural Revitalization in China. Land Use Policy 105, 105418. doi:10.1016/j.landusepol.2021.105418
Guruswamy Babu, P., Kavi Kumar, K. S., and Murthy, N. S. (1997). An Overlapping Generations Model with Exhaustible Resources and Stock Pollution. Ecol. Econ. 21 (1), 35–43. doi:10.1016/s0921-8009(96)00089-4
Hajjar, R., Oldekop, J. A., Cronkleton, P., Newton, P., Russell, A. J. M., and Zhou, W. (2021). A Global Analysis of the Social and Environmental Outcomes of Community Forests. Nat. Sustain. 4 (3), 216–224. doi:10.1038/s41893-020-00633-y
Hamamoto, M. (2006). Environmental Regulation and the Productivity of Japanese Manufacturing Industries. Resource Energ. Econ. 28 (4), 299–312. doi:10.1016/j.reseneeco.2005.11.001
Heckman, J., and Robb, R. J. (1985). Alternative Methods for Evaluating the Impact of Interventions. J. Econ. 30 (1–2), 239–267. doi:10.1016/0304-4076(85)90139-3
Hepburn, C., Qi, Y., Stern, N., Ward, B., Xie, C., and Zenghelis, D. (2021). Towards Carbon Neutrality and China's 14th Five-Year Plan: Clean Energy Transition, Sustainable Urban Development, and Investment Priorities. Environ. Sci. Ecotechnology 8, 100130. doi:10.1016/j.ese.2021.100130
Huang, Z. P. (2018). Does the Establishment of National Poverty-Stricken Counties Promote Local Economic Development? an Empirical Analysis Based on PSM-DID. China's rural economy (Chinese) 5, 1–14.
Jalil, A., and Feridun, M. (2011). The Impact of Growth, Energy and Financial Development on the Environment in China: a Cointegration Analysis. Energ. Econ. 33 (22), 284–291. doi:10.1016/j.eneco.2010.10.003
Jin, G., Guo, B., and Deng, X. (2020). Is There a Decoupling Relationship between CO2 Emission Reduction and Poverty Alleviation in China? Technol. Forecast. Soc. Change 151, 119856. doi:10.1016/j.techfore.2019.119856
Kenny, D. A., Korchmaros, J. D., and Bolger, N. (2003). Lower Level Mediation in Multilevel Models. Psychol. Methods 8, 115–128. doi:10.1037/1082-989x.8.2.115
Kesidou, E., and Wu, L. (2020). Stringency of Environmental Regulation and Eco-Innovation: Evidence from the Eleventh Five-Year Plan and green Patents. Econ. Lett. 190, 109090. doi:10.1016/j.econlet.2020.109090
Kube, R., Löschel, A., Mertens, H., and Requate, T. (2018). Research Trends in Environmental and Resource Economics: Insights from Four Decades of JEEM. J. Environ. Econ. Manag. 92, 433–464. doi:10.1016/j.jeem.2018.08.001
Lei, M., Yuan, X.-y., and Yao, X.-y. (2021). Synthesize Dual Goals: A Study on China's Ecological Poverty Alleviation System. J. Integr. Agric. 20 (4), 1042–1059. doi:10.1016/s2095-3119(21)63635-3
Levinson, A., and Taylor, M. S. (2008). Unmasking the Pollution haven Effect. Int. Econ. Rev 49 (1), 223–254. doi:10.1111/j.1468-2354.2008.00478.x
Li, H., Pang, S., Cao, Y., and Gao, J. (2021). Research on the Evaluation of Comprehensive Efficiency of Technological Innovation and Eco-Environment in China. J. Clean. Prod. 283, 124603. doi:10.1016/j.jclepro.2020.124603
Li, L., Fan, Z., Feng, W., Yuxin, C., and Keyu, Q. (2022). Coupling Coordination Degree Spatial Analysis and Driving Factor between Socio-Economic and Eco-Environment in Northern China. Ecol. Indicators 135, 108555. doi:10.1016/j.ecolind.2022.108555
Li, X., Yan, X., An, Q., Chen, K., and Shen, Z. (2016). The Coordination between China's Economic Growth and Environmental Emission from the Environmental Kuznets Curve Viewpoint. Nat. Hazards 83 (1), 233–252. doi:10.1007/s11069-016-2314-0
Li, Y., Bao, W., Bongers, F., Chen, B., Chen, G., Guo, K., et al. (2019). Drivers of Tree Carbon Storage in Subtropical Forests. Sci. Total Environ. 654 (654), 684–693. doi:10.1016/j.scitotenv.2018.11.024
Lin, B., and Ge, J. (2019). Valued forest Carbon Sinks: How Much Emissions Abatement Costs Could Be Reduced in China. J. Clean. Prod. 224, 455–464. doi:10.1016/j.jclepro.2019.03.221
Liu, S., and Xia, J. (2021). Forest Harvesting Restriction and forest Restoration in China. For. Policy Econ. 129, 102516. doi:10.1016/j.forpol.2021.102516
Liu, Y., Liu, J., and Zhou, Y. (2017). Spatio-temporal Patterns of Rural Poverty in China and Targeted Poverty Alleviation Strategies. J. Rural Stud. 52, 66–75. doi:10.1016/j.jrurstud.2017.04.002
Liuzzi, D., and Venturi, B. (2021). Pollution-induced Poverty Traps via Hopf Bifurcation in a Minimal Integrated Economic-Environment Model. Commun. Nonlinear Sci. Numer. Simulation 93, 105523. doi:10.1016/j.cnsns.2020.105523
Lu, S., Sun, H., Zhou, Y., Qin, F., and Guan, X. (2020). Examining the Impact of Forestry Policy on Poor and Non-poor Farmers' Income and Production Input in Collective forest Areas in China. J. Clean. Prod. 276, 123784. doi:10.1016/j.jclepro.2020.123784
Meyer, B. D. (1995). Natural and Quasi-Experiments in Economics. J. Business Econ. Stat. 13 (2), 151–161. doi:10.1080/07350015.1995.10524589
Peng, J., Song, Y., Tu, G., and Liu, Y. (2021). A Study of the Dual-Target Corporate Environmental Behavior (DTCEB) of Heavily Polluting Enterprises under Different Environment Regulations: Green Innovation vs. Pollutant Emissions. J. Clean. Prod. 297, 126602. doi:10.1016/j.jclepro.2021.126602
Qin, D., and Song, H. (2009). Sources of Investment Inefficiency: The Case of Fixed-Asset Investment in China. J. Develop. Econ. 90 (1), 94–105. doi:10.1016/j.jdeveco.2008.06.001
Rozelle, S., Huang, J., and Zhang, L. (1997). Poverty, Population and Environmental Degradation in China. Food Policy 22 (3), 229–251. doi:10.1016/s0306-9192(97)00011-0
Sharma, T., Kurz, W. A., Stinson, G., Pellatt, M. G., and Li, Q. (2013). A 100-year Conservation experiment: Impacts on forest Carbon Stocks and Fluxes. For. Ecol. Manag. 310, 242–255. doi:10.1016/j.foreco.2013.06.048
Shi, Y., Guan, Y., Li, L., and Huang, M. (2022). Empirical Analysis on the Impacts of Carbon Sink Afforestation Project on County Industrial Structural Upgrading. Alexandria Eng. J. 61 (1), 207–216. doi:10.1016/j.aej.2021.04.091
Shuai, J., Liu, J., Cheng, J., Cheng, X., and Wang, J. (2021). Interaction between Ecosystem Services and Rural Poverty Reduction: Evidence from China. Environ. Sci. Policy 119, 1–11. doi:10.1016/j.envsci.2021.01.011
Song, Y., Zhang, M., and Zhou, M. (2019). Study on the Decoupling Relationship between CO2 Emissions and Economic Development Based on Two-Dimensional Decoupling Theory: A Case between China and the United States. Ecol. Indicators 102, 230–236. doi:10.1016/j.ecolind.2019.02.044
Soumyananda, D. (2004). Environmental Kuznets Curve Hypothesis: A Survey. Ecol. Econ. 49 (4), 431–455.
Sun, Y., Li, M., Zhang, M., Khan, H. S. U. D., Li, J., Li, Z., et al. (2020). A Study on China's Economic Growth, green Energy Technology, and Carbon Emissions Based on the Kuznets Curve (EKC). Environ. Sci. Pollut. Res. 28 (6), 7200–7211. doi:10.1007/s11356-020-11019-0
Tang, X., Zhao, X., Bai, Y., Tang, Z., Wang, W., Zhao, Y., et al. (2018). Carbon Pools in China's Terrestrial Ecosystems: New Estimates Based on an Intensive Field Survey. Proc. Natl. Acad. Sci. U.S.A. 115 (16), 4021–4026. doi:10.1073/pnas.1700291115
Ulucak, R., and Khan, S. U. (2020). Mitigation Pathways toward Sustainable Development: Is There Any Trade-Off between Environmental Regulation and Carbon Emissions Reduction? Sust. Develop. 28 (4), 813–822. doi:10.1002/sd.2041
Wang, G., Qian, Z., and Deng, X. (2020). Analysis of Environmental Policy and the Performance of Sustainable Agricultural Development in China. Sustainability 12 (24), 10453. doi:10.3390/su122410453
Wang, X., Huang, J., and Liu, H. (2022). Can China's Carbon Trading Policy Help Achieve Carbon Neutrality? A Study of Policy Effects from the Five-Sphere Integrated Plan Perspective. J. Environ. Manage. 305 (1), 114357. doi:10.1016/j.jenvman.2021.114357
Wang, X., Zhang, T., Nathwani, J., Yang, F., and Shao, Q. (2022). Environmental Regulation, Technology Innovation, and Low Carbon Development: Revisiting the EKC Hypothesis, Porter Hypothesis, and Jevons' Paradox in China's Iron & Steel Industry. Technol. Forecast. Soc. Change 176, 121471. doi:10.1016/j.techfore.2022.121471
Wu, X., Tian, Z., and Guo, J. (2022). A Review of the Theoretical Research and Practical Progress of Carbon Neutrality. Sust. Operations Comput. 3, 54–66. doi:10.1016/j.susoc.2021.10.001
Xiang, J., Li, X., Xiao, R., and Wang, Y. (2021). Effects of Land Use Transition on Ecological Vulnerability in Poverty-Stricken Mountainous Areas of China: A Complex Network Approach. J. Environ. Manage. 297, 113206. doi:10.1016/j.jenvman.2021.113206
Yang, J., Hao, Y., and Feng, C. (2021). A Race between Economic Growth and Carbon Emissions: What Play Important Roles towards Global Low-Carbon Development? Energ. Econ. 100, 105327. doi:10.1016/j.eneco.2021.105327
Yang, Y., De Sherbinin, A., and Liu, Y. (2020). China's Poverty Alleviation Resettlement: Progress, Problems and Solutions. Habitat Int. 98, 102135. doi:10.1016/j.habitatint.2020.102135
Yang, Y., Wang, L., Yang, F., Hu, N., and Liang, L. (2021). Evaluation of the Coordination between Eco-Environment and Socioeconomy under the "Ecological County Strategy" in Western China: A Case Study of Meixian. Ecol. Indicators 125, 107585. doi:10.1016/j.ecolind.2021.107585
Yin, S., Gong, Z., Gu, L., Deng, Y., and Niu, Y. (2022). Driving Forces of the Efficiency of forest Carbon Sequestration Production: Spatial Panel Data from the National forest Inventory in China. J. Clean. Prod. 330, 129776. doi:10.1016/j.jclepro.2021.129776
Yuan, R., and Wang, J. (2021). Impacts of Poverty Alleviation on Household GHG Footprints in China. Energ. Econ. 103, 105602. doi:10.1016/j.eneco.2021.105602
Zengagàn-Castaño, J. E., Pagàn-Castaño, E., and Ribeiro-Navarrete, S. (2022). Merits of Intercity Innovation Cooperation of Environment-Friendly Patents for Environmental Regulation Efficiency. Technol. Forecast. Soc. Change, 121404. doi:10.1016/j.techfore.2021.121404
Zhang, R., and Hanaoka, T. (2021). Deployment of Electric Vehicles in China to Meet the Carbon Neutral Target by 2060: Provincial Disparities in Energy Systems, CO2 Emissions, and Cost Effectiveness. Resour. Conservation Recycling 170, 105622. doi:10.1016/j.resconrec.2021.105622
Zhang, S., and Chen, W. Y. (2021). China’s Energy Transition Pathway in a Carbon Neutral Vision. Engineering.
Keywords: forest resource protection, poverty-stricken counties, economic growth, carbon neutral, carbon emissions
Citation: Qi H, Sun L, Long F, Gao X and Hu L (2022) Does Forest Resource Protection Under the Carbon Neutrality Target Inhibit Economic Growth? Evidence of Poverty-Stricken County From China. Front. Environ. Sci. 10:858632. doi: 10.3389/fenvs.2022.858632
Received: 20 January 2022; Accepted: 07 March 2022;
Published: 13 April 2022.
Edited by:
Faik Bilgili, Erciyes University, TurkeyReviewed by:
Munir Ahmad, Zhejiang University, ChinaMofakhar Hussain, University of Toronto, Canada
Abdul Rauf, Nanjing University of Information Science and Technology, China
Copyright © 2022 Qi, Sun, Long, Gao and Hu. This is an open-access article distributed under the terms of the Creative Commons Attribution License (CC BY). The use, distribution or reproduction in other forums is permitted, provided the original author(s) and the copyright owner(s) are credited and that the original publication in this journal is cited, in accordance with accepted academic practice. No use, distribution or reproduction is permitted which does not comply with these terms.
*Correspondence: Fei Long, bG9uZ2ZlaV96YWZ1QDE2My5jb20=