- 1Joint National-Local Engineering Research Centre for Safe and Precise Coal Mining, Anhui University of Science & Technology, Huainan, China
- 2School of Earth and Environment, Anhui University of Science & Technology, Huainan, China
- 3Institute of Energy, Hefei Comprehensive National Science Center, Hefei, China
- 4State Key Laboratory of Mining Response and Disaster Prevention and Control in Deep Coal Mines, Anhui University of Science & Technology, Huainan, China
Coal mine dust is an important factor leading to occupational diseases of mine workers, however, it remains poorly understood about the non-viral pathogens in coal mine dust. In this study, bacterial communities in total dust and respirable dust from different laneways in Hongliulin coal mine (Shaanxi, China) were analyzed by PacBio high-throughput sequencing. The results showed no significant differences in the number, diversity and structure of bacterial communities in different laneways. Gammaproteobacteria, Alphaproteobacteria and Betaproteobacteria were the numerically dominant groups in dust samples which accounted for 72.5% of the total sequences. Among them, a total of seven known bacterial pathogens species including Aeromonas hydrophila, Burkholderia cenocepacia, Klebsiella pneumoniae, Proteus vulgaris, Serratia marcescens, Staphylococcus epidermidis and Staphylococcus saccharolyticus and two bacterial genera (Actinomyces and Peptostreptococcus) with extensive pathogenicity were detected in the respirable dust samples. And linear discriminant analysis indicated that prominent pathogens were detected in the respirable dust for the return laneway and the digging tunnel, such as K. pneumoniae and S. saccharolyticus in return laneway and B. cenocepacia in the digging tunnel. In parallel, PICRUST2 was performed to predict the functions in dust, and the human diseases count accounted for 4.31% of the total predictive function. In addition, the predictive count of human diseases in the respirable dust was closely related to bacterial diversity and structure. In summary, this study complemented the information of pathogenic bacteria in dust, especially in respirable dust, and suggested that non-viral pathogens should be considered in the process of mine dust prevention and control.
Introduction
Most epidemics are closely related to microbes in the environment, particularly, the current novel coronavirus epidemic COVID-19 caused by SARS-CoV-2 has a major impact on human health and economy in the world (Akter et al., 2022). But these epidemics caused by viruses, which threaten all mankind, have always been accompanied by the development of human society, such as AIDS, plague (such as the third plague, Antonine plague, 17th century great plagues, 18th century great plagues), flu (incluing Asian flu, Russian flu, Hong Kong flu, Swine flu), MERS, SARS and so on. In addition to viruses, non-viral pathogenic bacteria also threaten human health, many of which are closely related to respiratory diseases (Guo et al., 2021), skin diseases (Lunjani et al., 2021), gastrointestinal diseases (Imai et al., 2021), and blood diseases (Matsuoka et al., 2021).
Air, especially indoor air, can also provide a dependent environment for pathogens, such as dust (Viegas et al., 2020) and aerosols (Yan et al., 2021). Previous studies have shown that dust and bioaerosols in the air may increase the potential occupational health risks related to infection, toxicity and allergenicity (Eduard et al., 2012; Heederik and Von Mutius, 2012). Therefore, the monitoring and control of microorganisms in the air is particularly important, especially in some specific environments, such as medical (Cocarta et al., 2021) and dining establishments (Viegas et al., 2020).
Coal mine dust is a small solid particle that is emitted into the air during the production, storage, transportation, and roadway excavation of coal mines, which seriously affects the safety of the mine and the health of workers (Shekarian et al., 2021). Previous studies have shown that long-term exposure to the coal mine dust is the cause of many occupational diseases (Dai et al., 2012). The most serious hazard to human health is respirable dust, long-term inhalation of respirable dust could cause lung fibrosis and pneumoconiosis (Petsonk et al., 2013; Graber et al., 2014; Ciocan et al., 2022). However, people pay more attention to the impact of the physical properties of coal mine dust on human health, and often ignore that the dust carries a large number of microorganisms that have been stored underground for hundreds of millions of years. The recovery of these microorganisms poses a potential threat to humans, especially mine workers. Recent studies have shown that many pathogens are contained in the dust microflora and can cause many healthy problems, such as asthma, respiratory infections, skin and wound infections, acne and allergic reactions (Dijkshoorn et al., 2007; Nazaroff, 2019). However, there are very few microbiome studies on coal mine dust. Among the known literatures, only Wei et al. (2015) reported the rich and diverse microbial communities in coal mine aerosols by 454 pyrosequencing. Due to the limitation of read length, sequencing strategies such as 454 and Illumina can only generate partial marker gene regions, resulting in low classification resolution (Knight et al., 2018). The third-generation sequencing, such as PacBio, can produce a full-length sequence of amplified genes and species-level resolution, which is of great significance in our discussion of functional taxa in the coal mine environment. In addition, the research of Wei et al. (2015) did not evaluate the coal related pathogens.
In summary, the study of the microbiome in coal mine dust is meaningful for understanding the occupational health risks of coal miners. In this study, PacBio high-throughput sequencing were used to deeply investigate the microbial communities of coal mine dust based on 16S rRNA analysis. According to the comprehensive understanding of the microbial communities, potential pathogens in coal mine dust would be especially concerned.
Materials and Methods
Sampling
The dust samples were sampled at No. 25210 comprehensive working face and No. 25211 successive coal-mining face from Shaanxi Coal Group Shenmu Hongliulin coal mine (38.94°N, 110.41°E) in Shaanxi Province, China. The ground elevation was +1,163∼+1,276 m, and the elevation of working faces were +1,059∼+1,065 m. The wind speed of working faces was 1 m/s. In this study, a mine CCZ-20A dust sampler and a respirable dust pre-trap with sterilized filter membranes were used to collect the total dust and respirable dust samples. A total of 20 total dust samples and 20 respirable samples were collected, namely, six total dust samples (W) and respirable dust samples (H) in digging tunnel (DR) from No. 25211 successive coal-mining face; three total dust samples and three respirable samples in mining working face (CF), three total dust samples and three respirable dust samples in intake airflow roadway (IN) and eight total dust samples and eight respirable dust samples in return laneway (RE) from No. 25210 comprehensive working face, respectively. Each sampling time was 15 min at a flow rate of 20 L/min, and the sample collection location was 1.5 m above the ground. The collected dust samples were immediately transported to the laboratory using an ice cooler. The concentrations of total dust were 71.25 ± 5.59 mg m−3 (DR), 86.94 ± 6.62 mg m−3 (CF), 15.96 ± 3.79 mg m−3 (IN) and 89.87 ± 5.61 mg m−3 (RE); and the concentrations of respirable dust were 40.42 ± 2.30 mg m−3 (DR), 28.61 ± 16.11 mg m−3 (CF), 10.33 ± 0.99 mg m−3 (IN) and 26.08 ± 1.35 mg m−3 (RE).
DNA Extraction, 16S rRNA Gene Abundance Analysis and High-Throughput Sequencing
Total DNA was extracted from each sample with the FastDNA® SPIN Kit for Soil (MP Biomedicals, Cleveland, OH, United States) according to the manufacturer’s instructions.
The 16S rRNA gene abundance was analyzed by a LightCycler 96 optical real-time detection system (Roche, Basel, Switzerland). For real-time detection, the 515F/907R primer pair (515F: 5′-GTG CCA GCM GCC GCG G-3′, and 907R: 5′-CCG TCA ATT CMT TTR AGT TT-3′) were used to amplify the 16S rRNA gene fragments. The reactions were performed in a 20-μL mixture containing 10.0 μL of SYBR Premix Ex Taq (Takara), 0.5 μM of each primer, and 1 μl of DNA template. The amplification efficiencies were 90–105%, which were obtained with R2 values >0.99.
High-throughput sequencing was conducted by PacBio platform from Nextomics Biosciences Co., Ltd (Wuhan, China). For sequencing, the 27F/1492R (27F: 5′-AGR GTT YGA TYM TGG CTC AG-3′; 1492R: 5′-RGY TAC CTT GTT ACG ACT T-3′) primer pair was used to amplify the full-length fragment of 16S rRNA gene. The specific process of the experiment was as follows: 1) agarose gel electrophoresis was used to detect the extracted total DNA and specific primer 27F/1492R with barcode was used to amplify the total DNA; 2) AxyPrepDNA Gel Recovery Kit (AXYGEN Company) was used to cut the gel to recover the PCR products, and Tris-HCl was used to elute the DNA; and then the PCR products were detected by agarose electrophoresis and quantified with QuantiFluor™-ST blue fluorescence quantification system (Promega); 3) the quality-controlled product DNA was library constructed through end repair, adaptor ligation and library fixation; 4) finally, real-time single-molecule sequencing was achieved through the PacBio platform.
Bioinformatics Analysis
The original image data obtained by PacBio sequencing was converted into sequence data by Base calling, stored in BAM file, and converted into the corresponding fastq/fasta file format for storage, barcode splitting and data quality control. High-accuracy raw circular consensus sequences (CCS) were obtained by CCS software from SMRT Link and Arrow algorithm. And then the raw CCS sequences for each sample were obtained by identifying different barcode sequences with lima software (version 2.2.0).
In-depth quality control was mainly carried out through the following steps to obtain the clean CCS sequence for each sample. 1) CCS sequences with ≥ 99% accuracy were selected; 2) Blast software was used to match and intercept the target product sequence, and corrected the sequence direction; 3) sequences less than 1,000 bp or greater than 2000 bp were removed; 4) UCHIME algorithm was used to remove chimera sequences.
De-redundant CCS sequences according to 99% similarity were performed by CD-HIT software. Operational taxonomic units (OTUs) were clustered at 97% similarity, and OTU selection and taxonomic assignment were performed according to the SILVA reference data (version 128) by QIIME. And the Shannon diversity index was also determined.
The predictive functional abundance was predicted by PICRUSt2 (Phylogenetic Investigation of Communities by Reconstruction of Unobserved States) with “picrust2_pipeline.py” (https://github.com/picrust/picrust2) (Douglas et al., 2020), which performed four key steps including sequence placement, hidden-state prediction of genomes, metagenome prediction and pathway-level predictions. And the levels of KEGG pathways were further categorized by “summarizeAbundance.py” with EasyMicrobiome (v1.12, https://github.com/YongxinLiu/EasyMicrobiome) (Liu et al., 2021), which was used to merge genes by KEGG Orthologs (KOs).
Data Analysis
One-way analysis of variance (ANOVA, TukeyHSD with significance at p < 0.05) was used to analyze the shannon diversity index and 16S rRNA gene abundance among laneway, and to compare the predictive human diseases count between total dust and respirable dust. Nonmetric multidimensional scaling (NMDS) was performed according to Bray-Curtis dissimilarities for bacterial community composition and predictive functional abundance composition, and the Bray-Curtis dissimilarities were calculated by the vegan package (Oksanen et al., 2020) in R v 3.3.2. Heatmaps of the microbial phylum and predictive functional abundance at level 1 were constructed with the “pheatmap” package (Kolde, 2019) in R. The linear discriminant analysis effect size (LEfSe) method was performed to identify and interpret high-dimensional biomarkers, and the linear discriminant analysis (LDA) threshold of 3.0 (p < 0.05) was applied for LEfSe analysis. Linear regressions were used to analyze the relationships between predictive human disease count and bacterial diversity.
Results
Microbial Diversity of Coal Mine Dust
The mean number of high-quality clean CCS sequences per sample was 19 224, ranging from 11 013 to 20 912. The average length of the clean CCS sequences was ranged from 1,454 to 1,488 bp. A total of 612 distinct OTUs were identified. The goods coverages of all samples were higher than 99.6%, indicating that the sequencing depth satisfied the subsequent analysis. The shannon diversity index for coal mine dust was 4.65 ± 1.17, ranging from 1.31 to 6.60. However, there was no significant difference of shannon diversity index between the total dust and the respirable dust among laneways (Figure 1A).
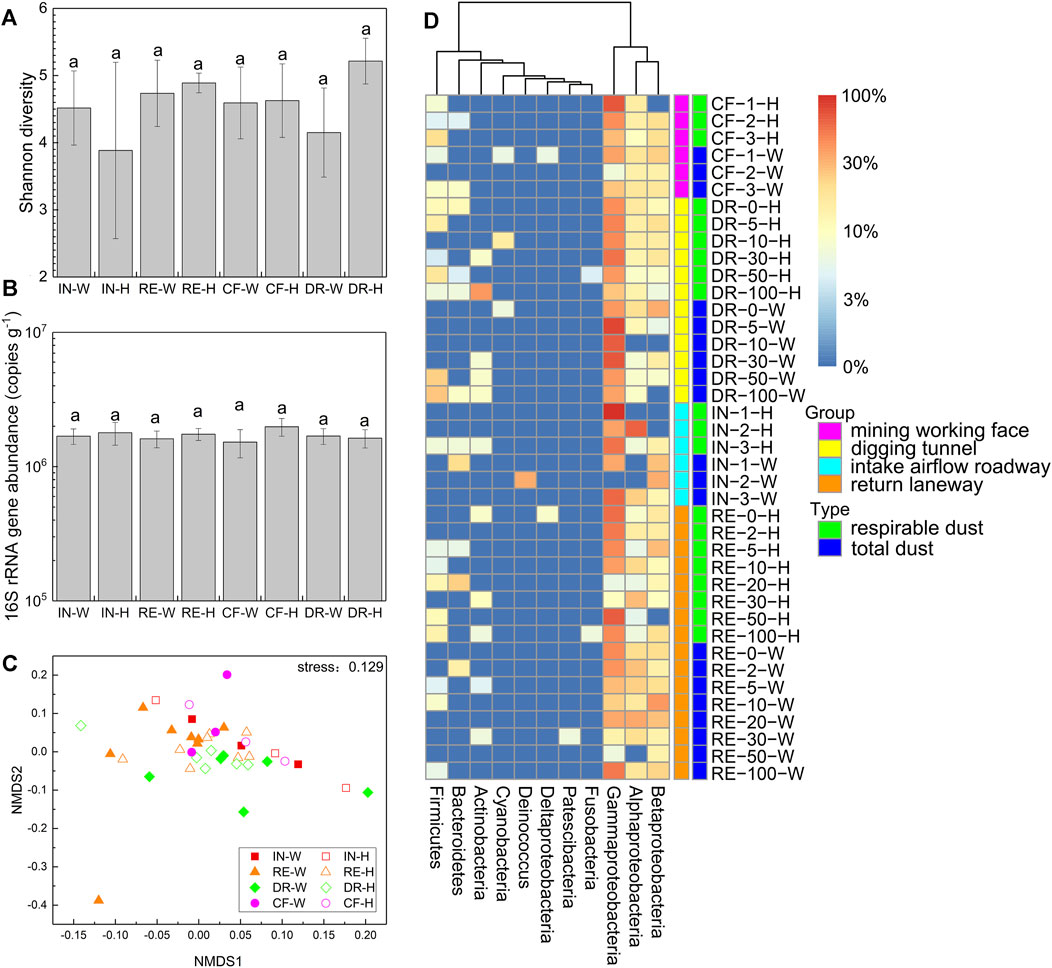
FIGURE 1. Shannon diversity based on 16S rRNA gene in dust samples from different laneways (A); 16S rRNA gene abundance (copies g−1) in dust samples from different laneways (B); Nonmetric multidimensional scaling (NMDS) of the bacterial community composition by Bray-Curtis dissimilarities in dust samples (C); Heat map of the dominant bacterial group in dust samples (D). IN-W, total dust in intake airflow roadway; IN-H, respirable dust in intake airflow roadway; RE-W, total dust in return laneway; RE-H, respirable dust in return laneway; CF-W, total dust in mining working face; CF-H, respirable dust in mining working face; DR-W, total dust in digging tunnel; DR-H, respirable dust in digging tunnel. Different lowercase letters were significantly different from each other (p < 0.05, TukeyHSD).
The 16S rRNA gene abundance was ranged from 9.51 × 105 to 2.58 × 106 copies g−1 in the total dust and 9.83 × 105 to 2.56 × 106 copies g−1in the respirable dust. And there was no significant difference in the 16S rRNA gene abundance among laneways (Figure 1B).
The ordering of dust samples by NMDS based on their Bray-Curtis distance showed the sample distribution mainly on the first axis nmds1, but there was no separation of dust samples among laneways (Figure 1C).
The high-quality CCS sequences reads were taxonomically assigned to 11 groups including phyla Actinobacteria, Deinococcus, Firmicutes, Bacteroidetes, Cyanobacteria, Patescibacteria and Fusobacteria and classes Gammaproteobacteria, Alphaproteobacteria, Betaproteobacteria and Deltaproteobacteria (Figure 1D). Among them, Gammaproteobacteria, Alphaproteobacteria and Betaproteobacteria were the numerically dominant groups accounted for 72.5% of the total sequences. Followingly, a certain number of Firmicutes, Bacteroidetes and Actinobacteria can often been detected in dust samples. However, there was no significant difference of relative abundance for these groups between the total dust and the respirable dust among laneways (Table 1).

TABLE 1. The relative abundance (mean ± se) of the dominant bacterial group in dust samples among laneways.
Predictive Function of Coal Mine Dust
We analyzed the predictive function in different dust samples according to PICRUST2. However, the ordering of dust samples by NMDS based on the Bray-Curtis distance of predictive function composition among samples also showed no separation of dust samples among laneways (Figure 2A). The predictive function of coal mine dust mainly participated in seven KEGG pathways at the level 1, including brite hierarchies, cellular processes, environmental information processing, genetic information processing, human diseases, metabolism and organismal systems (Figure 2B). Among them, brite hierarchies and metabolism were the dominant predictive functions accounted for 69.1% of the total predictive counts. In addition, human diseases and organismal systems were most overlooked predictive functions with the lowest count percentage (4.31 and 1.85%, respectively). However, there was also no significant difference of counts for these predictive functions between the total dust and the respirable dust among laneways.
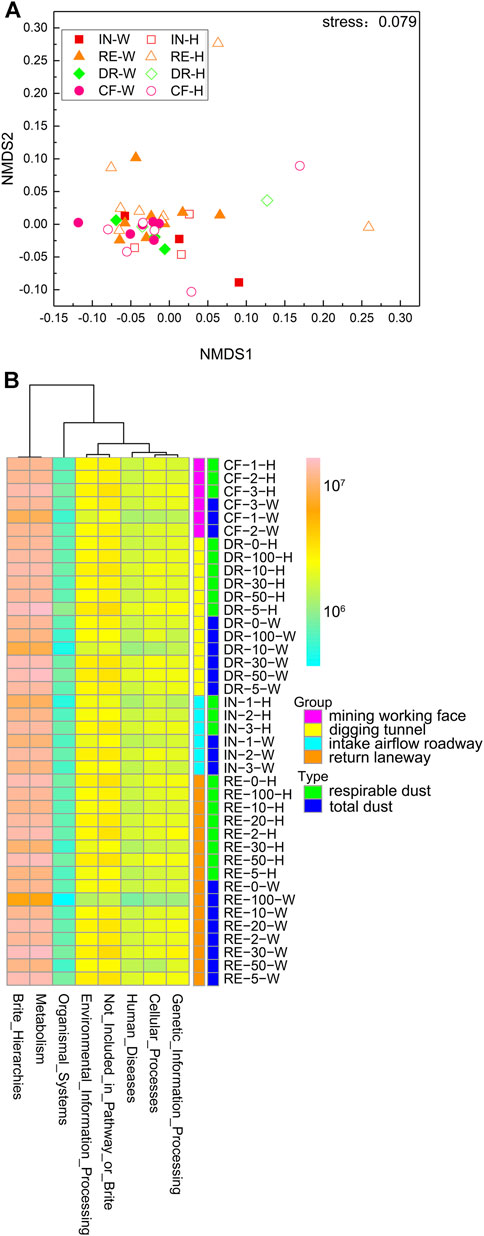
FIGURE 2. NMDS of the predictive function composition by Bray-Curtis dissimilarities in dust samples (A); Heat map of the predictive functions at level 1 in dust samples (B). IN-W, total dust in intake airflow roadway; IN-H, respirable dust in intake airflow roadway; RE-W, total dust in return laneway; RE-H, respirable dust in return laneway; CF-W, total dust in mining working face; CF-H, respirable dust in mining working face; DR-W, total dust in digging tunnel; DR-H, respirable dust in digging tunnel. Different lowercase letters were significantly different from each other (p < 0.05, TukeyHSD).
Human Diseases and Pathogenic Bacteria
The relationship between human diseases counts and bacterial diversity were constructed (Figure 3). The results showed that the predictive count of human diseases in the respirable dust was closely related to shannon diversity index, nmds1 and nmds2 (Figures 3A,C,E), and the difference of human diseases predictive count and Bray-Curtis distance in the respirable dust was also linearly correlated (Figure 3G). However, these correlations were not reflected in that from the total dust (Figures 3B,D,F,H).
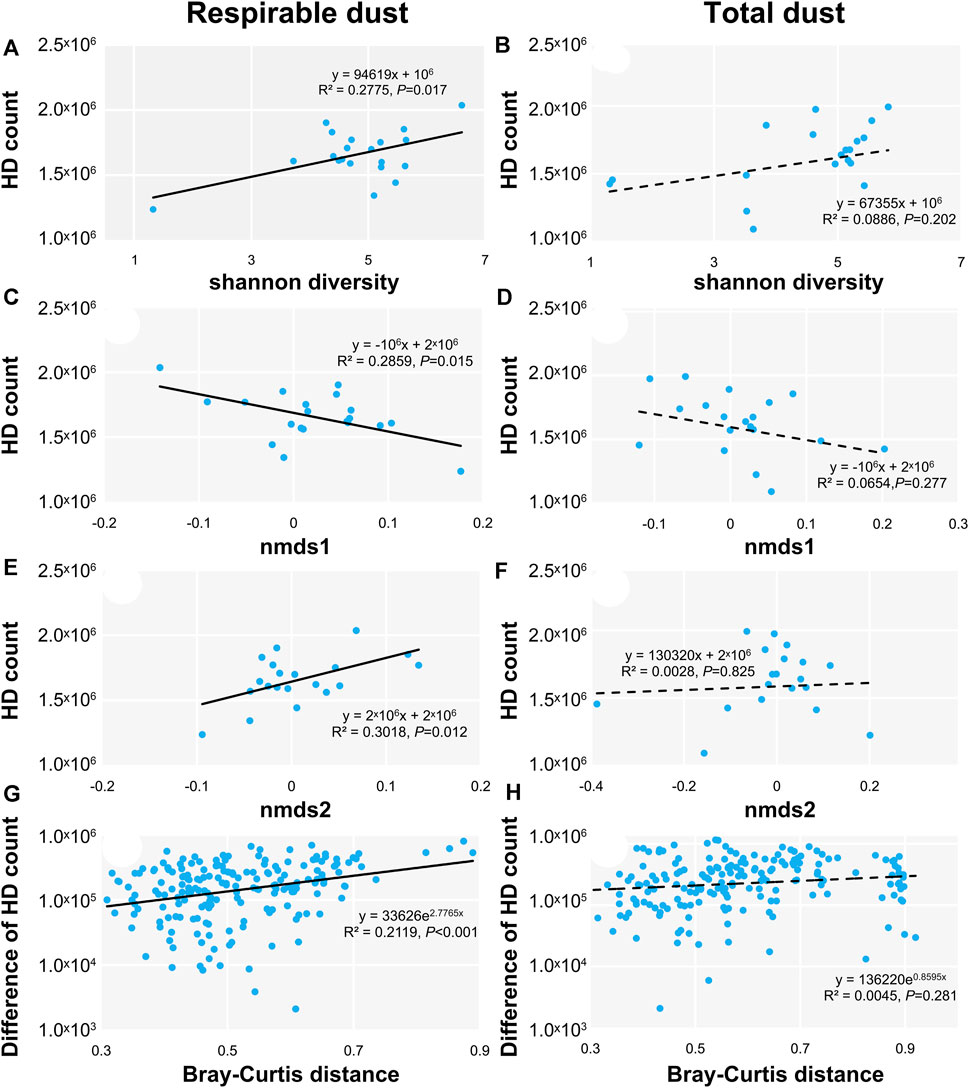
FIGURE 3. The relationship between the human diseases (HD) count and shannon diversity, the first two axes of NMDS in respirable dust (A,C,E) and total dust (B,D,F); the relationship between the different of HD count and the bacterial Bray-Curtis distance in respirable dust (G) and total dust (H).
Twelve human diseases pathways at the level 2 were predicted. According to their predicted count, the order was Drug resistance: antimicrobial, Infectious disease: bacterial, Neurodegenerative disease, Cancer: overview, Drug resistance: antineoplastic, Infectious disease: viral, Cardiovascular disease, Cancer: specific types, Endocrine and metabolic disease, Immune disease, Infectious disease: parasitic and Substance dependence (Figure 4). Among them, Drug resistance: antimicrobial and Infectious disease: bacterial were the two highest predicted human disease pathways. However, there is no difference in the counts of these human diseases pathways between total dust and respirable dust.
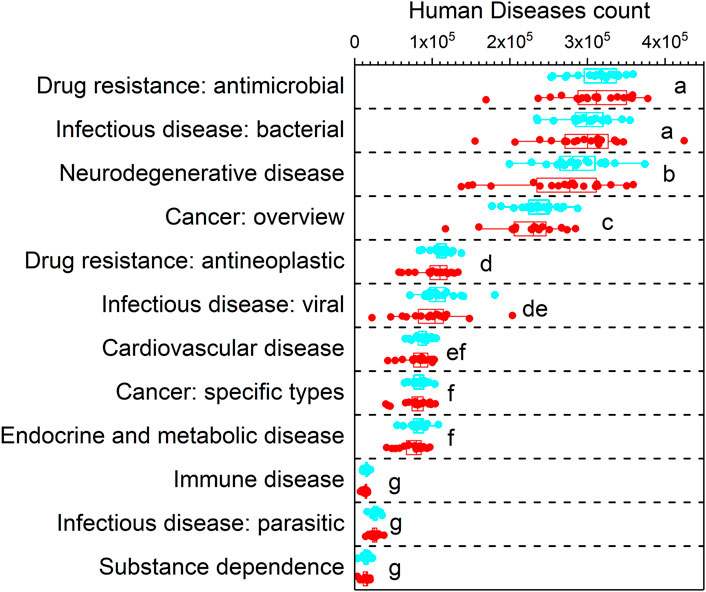
FIGURE 4. The predictive functions at level 2 in dust samples. Cyan showed the human diseases count in respirable dust and red showed the human diseases count in total dust. Different lowercase letters were significantly different from each other (p < 0.05, TukeyHSD).
We further detected seven known bacterial pathogens species including Aeromonas hydrophila, Burkholderia cenocepacia, Klebsiella pneumoniae, Proteus vulgaris, Serratia marcescens, Staphylococcus epidermidis and Staphylococcus saccharolyticus and two bacterial genera (Actinomyces and Peptostreptococcus) with extensive pathogenicity based on the specific bacterial pathogens database (https://globalrph.com/bacteria/). Linear discriminant analysis (Figure 5) indicated that there are differences in the specific bacteria between the total dust and the respirable dust in the return laneway, the digging tunnel and the mining working face, and the different bacteria were also diverse in laneways. Furthermore, prominent pathogens were detected in the respirable dust for the return laneway and the digging tunnel, such as K. pneumoniae and S. saccharolyticus in return laneway (Figure 5A) and B. cenocepacia in the digging tunnel (Figure 5B).
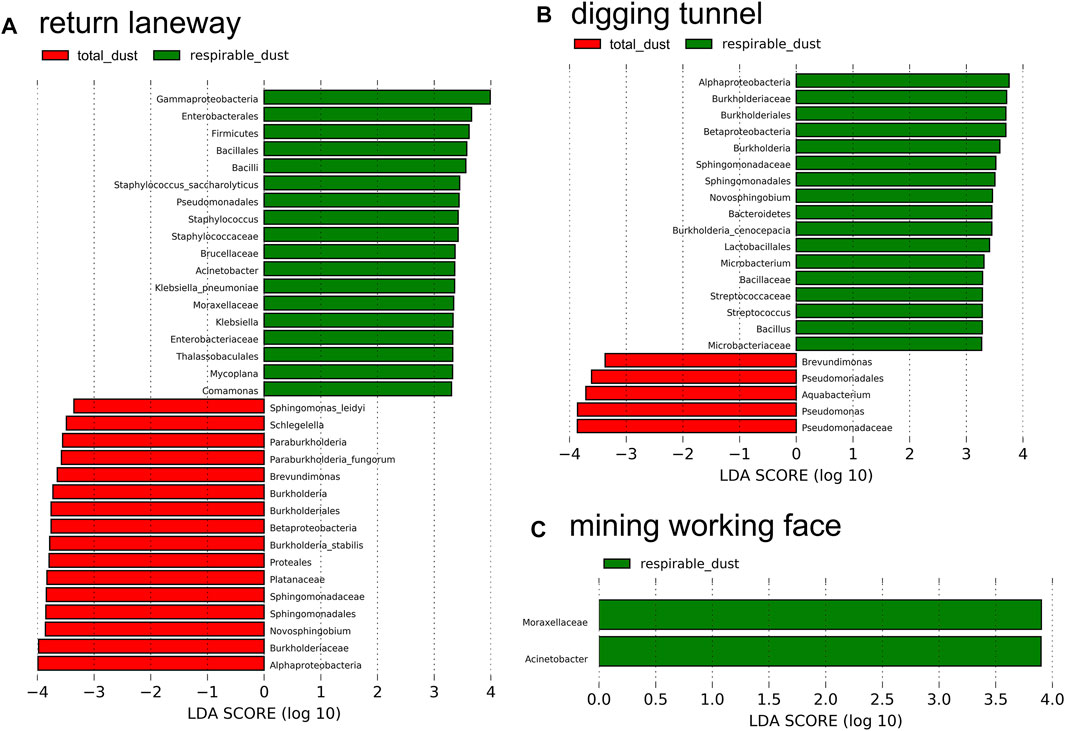
FIGURE 5. Linear discriminant analysis (LDA, threshold of 3.0, p < 0.5) for high-dimensional biomarkers in return lanway (A), digging tunnel (B) and mining working face (C). And there was no high-dimensional biomarker in intake airflow roadway between the total dust and the respirable dust.
Discussion
In this study, we observed the microbial diversity and abundance of dust in different laneways. The special loading medium of dust and the better ventilation environment in the laneways may not be suitable for the bacterial survival compared to soil, water and other habitats, resulting in a lower abundance. However, the bacterial diversity in this study (shannon diversity index 4.65 ± 1.17) was at a similar level to that of the sandy soil (Wang W et al., 2020) semiarid farmland (Shen et al., 2016) and phyllospheric habitat (Zhang et al., 2015) in Shaanxi, China, which was also higher than that in coal seams from the majority coal mining areas in China such as Anhui, Henan, Inner Mongolia, Shanxi and Guizhou (Su et al., 2018; Liu et al., 2019). It also showed the rich bacterial diversity in mine laneways.
Respiratory dust is particulate matter that can enter the alveolar area of human. The particle size is smaller, but this study indicated that the bacterial diversity and abundance of respiratory dust were not significantly decreased. In parallel, the bacterial structure and composition will not be different among different laneways, thus the bacteria in respiratory dust that affecting the health of mine workers cannot be ignored. Proteobacteria were the main microbial group in dust in this study, especially Beta-, Alpha- and Gamma-Proteobacteria. The microorganisms in mine dust mainly come from coal seams, and there is still a lack of reports on the study of coal seam microorganisms in this area. But in other coal seams from other areas of China (including Shanxi, Guizhou, Anhui, Inner Mongolia, Henan, etc.), Proteobacteria were also found to be the main microbial group (Su et al., 2018; Liu et al., 2019). Respiratory dust is considered to be an important factor in the lung injury of mine workers (Petsonk et al., 2013; Graber et al., 2014). Through a follow-up study of 1 534 005 person-years (range, 4.8–43.9 years), Wang D et al. (2020) further found that mine dust exposure significantly increased the risk of death from diseases such as lung cancer, respiratory tuberculosis, cardiovascular diseases, and respiratory diseases. However, we often ignore that pathogenic bacteria could increase the harm to human health with the inhalation of dust, such as asthma, respiratory tract infections (Dijkshoorn et al., 2007; Nazaroff, 2019). In other industries, though, pathogens have been of great concern. For example, Panton-Valentine leukocidin-producing methicillin-susceptible Staphylococcus aureus could cause cutaneous abscesses for gold mine workers (Ismail et al., 2020).
In order to understand the pathogenic bacteria of dust, PICRUSt2 was used to predict the genome of 16S rRNA gene sequences sampled from the environment. The PICRUSt2 algorithm includes steps to optimize genome prediction, such as placing sequences in a reference phylogenetic tree, predicting based on larger reference genomes and gene family databases, rigorously predicting pathway abundance, and being able to predict complex phenotypes and integrations Custom database (Douglas et al., 2020). It is considered to be an effective tool to evaluate the functional gene composition of the sample, and the result of its function prediction analysis is quite close to the result obtained by metagenomic sequencing (Langille et al., 2013). The prediction results found that human disease-causing genes also require extensive attention, and their predicted absolute abundance is still not low (ranged from 8.25 × 105 to 2.04 × 106). And its abundance does not differ among different laneways. These genes mainly included drug resistance, infectious disease, neurodegenerative disease and cancer.
At the same time, this study detected seven known bacterial pathogens species which involve digestive tract diseases, skin diseases, respiratory diseases, blood infections, genitourinary diseases, oral and dental diseases and drug resistance (https://globalrph.com/bacteria/). A survey in the United States showed that all cardiovascular disease, chronic obstructive pulmonary disorder, cardiovascular stroke, heart failure, ischemic heart disease, and respiratory tract infections decreased significantly after coal exposure decreased during 2005–2012 (Henneman et al., 2019). At the same time, poor ventilation in the mine was an important factor leading to latent tuberculosis infection (Jin et al., 2018). However, the presence of pathogenic bacteria in dust might also be an important factor that aggravated the disease or increases the prevalence of pulmonary disease (Hailemariam et al., 2021). In addition, mining and coal mine movers have been found to be one of the most frequent occupations for skin diseases (Camille et al., 2019). Among the bacterial pathogens species, the ESKAPE pathogen K. pneumoniae (Meade et al., 2021) was obviously prominent in the return laneway. The infection of these pathogenic bacteria will undoubtedly increase the difficulty of treatment for sick workers. The infections of A. hydrophila that detected in this study have a distinct clinical manifestation in wood, besides some other symptoms were often found such as hepatobiliary diseases, diarrhea, and even bacteremia (Rosso et al., 2019). Previous studies reported a mortality rate of 30% due to this infection (Sinclair et al., 2016). Furthermore, S. marcescens often detected in the study area can penetrated the skin barrier and overwhelm the human immune system to cause skin and soft tissue infections (Soria et al., 2008).
Besides, dehydration is an important health risk for mine workers (Polkinghorne et al., 2013), which can also end up taxing the workers’ urethra and kidneys (Edwin et al., 2019). In this study, the presence of K. pneumonia (Daca et al., 2021; Lorenzo-Gomez et al., 2021), Proteus Vulgaris (Lorenzo-Gomez et al., 2021), S. Marcescens (Jimenez-Guerra et al., 2020) and S. saccharolyticus (Loes et al., 2014) in dust in this study area also increased the risk of urethral disease among workers. Additionally, the pathogen is often detected in the serum of mine workers in other areas and was potentially associated with specific diseases (Yih et al., 2019). Therefore, health problems of mine workers are influenced by a variety of factors in their working environment, as well as the potential threat of pathogenic bacteria.
From the perspective of pathogen prevention and control, controlling the influencing factors of pathogen gene abundance is very important. Dust reduction (Heidenreich, 2013) is undoubtedly an effective control of the total amount of pathogenic genes exposed to workers. In addition, our research also found that the relative abundance of pathogenic genes in respiratory dust is closely related to bacterial diversity, and higher microbial diversity will also increase the abundance of disease related genes in dust. Therefore, reducing the spread of pathogenic genes should not be neglected in the work of dust suppression in mines.
Data Availability Statement
The datasets presented in this study can be found in online repositories. The names of the repository/repositories and accession number(s) can be found below: NCBI (accession: PRJNA794272).
Author Contributions
SX, XL, and YL had full access to all of the data in the study and take responsibility for the integrity of the data and the accuracy of the data analysis. SX and YL drafted the manuscript; BL and YL conducted the bulk of the data analysis for the study; XL, QT, and CL participated in sample collection; all authors have substantial contributions to the conception or the design of the work. All authors contributed to the acquisition analysis and interpretation of data; revising critically and final approval of the version; and agreement to be accountable for all aspects of the work.
Funding
This study was funded by the key program of National Natural Science Foundation of China (51934007), major scientific and technological innovation projects in Shandong Province (2019JZZY020504), the Independent Research Fund of the State Key Laboratory of Mining Response and Disaster Prevention and Control in Deep Coal Mines in Anhui University of Science and Technology (SKLMRDPC20ZZ08), and the Institute of Energy, Hefei Comprehensive National Science Center under Grant No. 21KZS216.
Conflict of Interest
The authors declare that the research was conducted in the absence of any commercial or financial relationships that could be construed as a potential conflict of interest.
Publisher’s Note
All claims expressed in this article are solely those of the authors and do not necessarily represent those of their affiliated organizations, or those of the publisher, the editors and the reviewers. Any product that may be evaluated in this article, or claim that may be made by its manufacturer, is not guaranteed or endorsed by the publisher.
Acknowledgments
The authors thank Shaanxi Coal Group Shenmu Hongliulin Mining Co. Ltd. for providing help for sample collection of this study.
References
Akter, R., Rahman, M. H., Bhattacharya, T., Kaushik, D., Mittal, V., Parashar, J., et al. (2021). Novel Coronavirus Pathogen in Humans and Animals: an Overview on its Social Impact, Economic Impact, and Potential Treatments. Environ. Sci. Pollut. Res 28, 68071–68089. doi:10.1007/s11356-021-16809-8
Camille, C., Catherine, V. E., Isabelle, L., and Isabelle, B. (2019). Occupational Cancers: Risks and Prevention. Bull. Du Cancer 106, 665–677. doi:10.1016/j.bulcan.2018.10.010
Ciocan, C., Pira, E., Coggiola, M., Franco, N., Godono, A., La Vecchia, C., et al. (2022). Mortality in the Cohort of Talc Miners and Millers from Val Chisone, Northern Italy: 74 Years of Follow-Up. Environ. Res. 203, 111865. doi:10.1016/j.envres.2021.111865
Cocarta, D. M., Prodana, M., Demetrescu, I., Lungu, P. E. M., and Didilescu, A. C. (2021). Indoor Air Pollution with Fine Particles and Implications for Workers' Health in Dental Offices: A Brief Review. Sustainability 13 (2), 599. doi:10.3390/su13020599
Daca, A., Golebiewska, J., Bronk, M., and Jarzembowski, T. (2021). Changes of Urine Isolates of Escherichia coli and Klebsiella pneumoniae Biofilm Affect Monocytes' Response. World J. Microbiol. Biotechnol. 37, 181. doi:10.1007/s11274-021-03150-y
Dai, S., Ren, D., Chou, C.-L., Finkelman, R. B., Seredin, V. V., and Zhou, Y. (2012). Geochemistry of Trace Elements in Chinese Coals: A Review of Abundances, Genetic Types, Impacts on Human Health, and Industrial Utilization. Int. J. Coal Geology. 94, 3–21. doi:10.1016/j.coal.2011.02.003
Dijkshoorn, L., Nemec, A., and Seifert, H. (2007). An Increasing Threat in Hospitals: Multidrug-Resistant Acinetobacter Baumannii. Nat. Rev. Microbiol. 5, 939–951. doi:10.1038/nrmicro1789
Douglas, G. M., Maffei, V. J., Zaneveld, J. R., Yurgel, S. N., Brown, J. R., Taylor, C. M., et al. (2020). PICRUSt2 for Prediction of Metagenome Functions. Nat. Biotechnol. 38, 685–688. doi:10.1038/s41587-020-0548-6
Eduard, W., Heederik, D., Duchaine, C., and Green, B. J. (2012). Bioaerosol Exposure Assessment in the Workplace: the Past, Present and Recent Advances. J. Environ. Monit. 14, 334–339. doi:10.1039/c2em10717a
Edwin, M., Moore, D., and Guard, D. (2019). Dehydration in New Zealand Fishing Vessel Crews. Saf. Sci. 117, 314–319. doi:10.1016/j.ssci.2019.04.019
Graber, J. M., Stayner, L. T., Cohen, R. A., Conroy, L. M., and Attfield, M. D. (2014). Respiratory Disease Mortality Among US Coal Miners; Results after 37 Years of Follow-Up. Occup. Environ. Med. 71, 30–39. doi:10.1136/oemed-2013-101597
Guo, M. Y., Chen, H. K., Ying, H. Z., Qiu, F. S., and Wu, J. Q. (2021). The Role of Respiratory Flora in the Pathogenesis of Chronic Respiratory Diseases. Biomed. Res. Int. 2021, 6431862. doi:10.1155/2021/6431862
Hailemariam, M., Yu, Y., Singh, H., Teklu, T., Wondale, B., Worku, A., et al. (2021). Protein and Microbial Biomarkers in Sputum Discern Acute and Latent Tuberculosis in Investigation of Pastoral Ethiopian Cohort. Front Cel Infect Microbiol 11, 595554. doi:10.3389/fcimb.2021.595554
Heederik, D., and Von Mutius, E. (2012). Does Diversity of Environmental Microbial Exposure Matter for the Occurrence of Allergy and Asthma? J. Allergy Clin. Immunol. 130, 44–50. doi:10.1016/j.jaci.2012.01.067
Heidenreich, S. (2013). Hot Gas Filtration - A Review. Fuel 104, 83–94. doi:10.1016/j.fuel.2012.07.059
Henneman, L. R. F., Choirat, C., and Zigler, C. M. (2019). Accountability Assessment of Health Improvements in the United States Associated with Reduced Coal Emissions between 2005 and 2012. Epidemiology 30, 477–485. doi:10.1097/ede.0000000000001024
Imai, J., Kitamoto, S., and Kamada, N. (2021). The Pathogenic Oral-Gut-Liver axis: New Understandings and Clinical Implications. Expert Rev. Clin. Immunol. 17, 727–736. doi:10.1080/1744666x.2021.1935877
Ismail, H., Govender, N. P., Singh-Moodley, A., Van Schalkwyk, E., Shuping, L., Moema, I., et al. (2020). An Outbreak of Cutaneous Abscesses Caused by Panton-Valentine Leukocidin-Producing Methicillin-Susceptible Staphylococcus aureus Among Gold Mine Workers, South Africa, November 2017 to March 2018. Bmc Infect. Dis. 20, 621. doi:10.1186/s12879-020-05352-5
Jiménez-Guerra, G., Borrego-Jiménez, J., Gutiérrez-Soto, B., Expósito-Ruiz, M., Navarro-Marí, J. M., and Gutiérrez-Fernández, J. (2020). Susceptibility Evolution to Antibiotics of Enterobacter cloacae, Morganella morganii, Klebsiella Aerogenes and Citrobacter Freundii Involved in Urinary Tract Infections: an 11-year Epidemiological Surveillance Study. Enfermedades Infecciosas y Microbiologia Clinica (English ed.) 38, 166–169. doi:10.1016/j.eimce.2019.07.003
Jin, Y., Wang, H., Zhang, J., Ding, C., Wen, K., Fan, J., et al. (2018). Prevalence of Latent Tuberculosis Infection Among Coal Workers' Pneumoconiosis Patients in China: a Cross-Sectional Study. Bmc Public Health 18, 473. doi:10.1186/s12889-018-5373-1
Knight, R., Vrbanac, A., Taylor, B. C., Aksenov, A., Callewaert, C., Debelius, J., et al. (2018). Best Practices for Analysing Microbiomes. Nat. Rev. Microbiol. 16, 410–422. doi:10.1038/s41579-018-0029-9
Langille, M. G. I., Zaneveld, J., Caporaso, J. G., Mcdonald, D., Knights, D., Reyes, J. A., et al. (2013). Predictive Functional Profiling of Microbial Communities Using 16S rRNA Marker Gene Sequences. Nat. Biotechnol. 31, 814–821. doi:10.1038/nbt.2676
Liu, B., Yuan, L., Shi, X., Li, Y., Jiang, C., Ren, B., et al. (2019). Variations in Microbiota Communities with the Ranks of Coals from Three Permian Mining Areas. Energy Fuels 33, 5243–5252. doi:10.1021/acs.energyfuels.8b04413
Liu, Y.-X., Qin, Y., Chen, T., Lu, M., Qian, X., Guo, X., et al. (2021). A Practical Guide to Amplicon and Metagenomic Analysis of Microbiome Data. Protein Cell 12, 315–330. doi:10.1007/s13238-020-00724-8
Loes, A. N., Ruyle, L., Arvizu, M., Gresko, K. E., Wilson, A. L., and Deutch, C. E. (2014). Inhibition of Urease Activity in the Urinary Tract Pathogen Staphylococcus Saprophyticus. Lett. Appl. Microbiol. 58, 31–41. doi:10.1111/lam.12153
Lorenzo-Gómez, M. F., Padilla-Fernández, B., Flores-Fraile, J., Valverde-Martínez, S., González-Casado, I., Hernández, J.-M. D. D., et al. (2021). Impact of Whole-Cell Bacterial Immunoprophylaxis in the Management of Recurrent Urinary Tract Infections in the Frail Elderly. Vaccine 39, 6308–6314. doi:10.1016/j.vaccine.2021.08.093
Lunjani, N., Ahearn-Ford, S., Dube, F. S., Hlela, C., and O’Mahony, L. (2021). Mechanisms of Microbe-Immune System Dialogue within the Skin. Genes Immun. 22, 276–288. doi:10.1038/s41435-021-00133-9
Matsuoka, T., Shimizu, T., Minagawa, T., Hiranuma, W., Takeda, M., Kakuta, R., et al. (2021). First Case of an Invasive Bacteroides Dorei Infection Detected in a Patient with a Mycotic Aortic Aneurysm-Raising a Rebellion of Major Indigenous Bacteria in Humans: a Case Report and Review. BMC Infect. Dis. 21, 625. doi:10.1186/s12879-021-06345-8
Meade, E., Slattery, M. A., and Garvey, M. (2021). Biocidal Resistance in Clinically Relevant Microbial Species: A Major Public Health Risk. Pathogens 10, 598. doi:10.3390/pathogens10050598
Nazaroff, W. W. (2019). Embracing Microbes in Exposure Science. J. Expo. Sci. Environ. Epidemiol. 29, 1–10. doi:10.1038/s41370-018-0075-4
Oksanen, J., Blanchet, F. G., Friendly, M., Kindt, R., Legendre, P., Mcglinn, D., et al. (2020). Vegan: Community Ecology Package. R package version 2.5-7.
Petsonk, E. L., Rose, C., and Cohen, R. (2013). Coal Mine Dust Lung Disease. New Lessons from an Old Exposure. Am. J. Respir. Crit. Care Med. 187, 1178–1185. doi:10.1164/rccm.201301-0042ci
Polkinghorne, B. G., Gopaldasani, V., Furber, S., Davies, B., and Flood, V. M. (2013). Hydration Status of Underground Miners in a Temperate Australian Region. Bmc Public Health 13, 426. doi:10.1186/1471-2458-13-426
Rosso, F., Cedano, J. A., Parra-Lara, L. G., Sanz, A. M., Toala, A., Velez, J. F., et al. (2019). Emerging Carbapenem-Resistant Aeromonas Spp. Infections in Cali, Colombia. Braz. J. Infect. Dis. 23, 336–342. doi:10.1016/j.bjid.2019.08.005
Shekarian, Y., Rahimi, E., Rezaee, M., Su, W. C., and Roghanchi, P. (2021). Respirable Coal Mine Dust: A Review of Respiratory Deposition, Regulations, and Characterization. Minerals 11 (7), 696. doi:10.3390/min11070696
Shen, Y., Chen, Y., and Li, S. (2016). Microbial Functional Diversity, Biomass and Activity as Affected by Soil Surface Mulching in a Semiarid Farmland. Plos One 11, e0159144. doi:10.1371/journal.pone.0159144
Sinclair, H. A., Heney, C., Sidjabat, H. E., George, N. M., Bergh, H., Anuj, S. N., et al. (2016). Genotypic and Phenotypic Identification of Aeromonas Species and CphA-Mediated Carbapenem Resistance in Queensland, Australia. Diagn. Microbiol. Infect. Dis. 85, 98–101. doi:10.1016/j.diagmicrobio.2016.02.005
Soria, X., Bielsa, I., Ribera, M., Herrero, M. J., Domingo, H., Carrascosa, J. M., et al. (2008). Acute Dermal Abscesses Caused by Serratia marcescens. J. Am. Acad. Dermatol. 58, 891–893. doi:10.1016/j.jaad.2008.01.011
Su, X., Zhao, W., and Xia, D. (2018). The Diversity of Hydrogen-Producing Bacteria and Methanogens within an In Situ Coal Seam. Biotechnol. Biofuels 11, 245. doi:10.1186/s13068-018-1237-2
Viegas, C., Fleming, G. T. A., Kadir, A., Almeida, B., Caetano, L. A., Quintal Gomes, A., et al. (2020). Occupational Exposures to Organic Dust in Irish Bakeries and a Pizzeria Restaurant. Microorganisms 8, 118. doi:10.3390/microorganisms8010118
Wang, D., Yang, M., Liu, Y., Ma, J., Shi, T., and Chen, W. (2020). Association of Silica Dust Exposure and Cigarette Smoking with Mortality Among Mine and Pottery Workers in China. JAMA Netw. Open 3, e202787. doi:10.1001/jamanetworkopen.2020.2787
Wang, W., Han, L., and Zhang, X. (2020). Winter Cover Crops Effects on Soil Microbial Characteristics in sandy Areas of Northern Shaanxi, China. Rev. Bras. Cienc. Solo 44, e0190173. doi:10.36783/18069657rbcs20190173
Wei, M., Yu, Z., and Zhang, H. (2015). Molecular Characterization of Microbial Communities in Bioaerosols of a Coal Mine by 454 Pyrosequencing and Real-Time PCR. J. Environ. Sci. 30, 241–251. doi:10.1016/j.jes.2014.07.035
Yan, C., Leng, Y. L., and Wu, J. T. (2021). Quantitative Microbial Risk Assessment for Occupational Health of Temporary Entrants and Staffs Equipped with Various Grade PPE and Exposed to Microbial Bioaerosols in Two WWTPs. Int. Arch. Occup. Environ. Health 94, 1327–1343. doi:10.1007/s00420-021-01663-5
Yih, W. K., Kulldorff, M., Friedman, D. J., Leibler, J. H., Amador, J. J., López-Pilarte, D., et al. (2019). Investigating Possible Infectious Causes of Chronic Kidney Disease of Unknown Etiology in a Nicaraguan Mining Community. Am. J. Trop. Med. Hyg. 101, 676–683. doi:10.4269/ajtmh.18-0856
Keywords: coal mine, respirable dust, non-viral pathogens, PacBio, picrust2
Citation: Xue S, Liu X, Li Y, Liu B, Tu Q and Li C (2022) Pathogenic Bacterial Communities of Dust in a Coal Mine. Front. Environ. Sci. 10:857744. doi: 10.3389/fenvs.2022.857744
Received: 19 January 2022; Accepted: 28 March 2022;
Published: 27 April 2022.
Edited by:
Nur A. Hasan, EzBiome Inc., United StatesReviewed by:
Anamul Haque, Clemson University, United StatesHasan C. Tekedar, Mississippi State University, United States
Copyright © 2022 Xue, Liu, Li, Liu, Tu and Li. This is an open-access article distributed under the terms of the Creative Commons Attribution License (CC BY). The use, distribution or reproduction in other forums is permitted, provided the original author(s) and the copyright owner(s) are credited and that the original publication in this journal is cited, in accordance with accepted academic practice. No use, distribution or reproduction is permitted which does not comply with these terms.
*Correspondence: Yang Li, bGl5YW5nX2F1c3RAMTYzLmNvbQ==, b3JjaWQub3JnLzAwMDAtMDAwMi04OTQ2LTM5NjI=