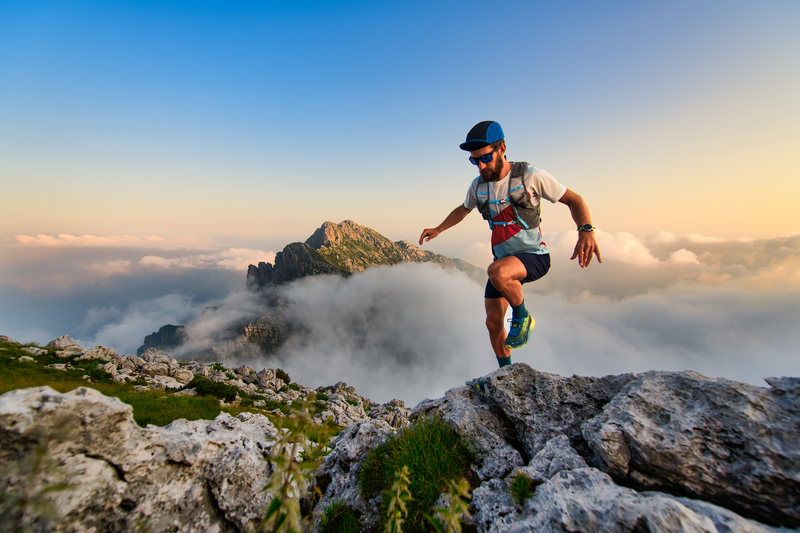
94% of researchers rate our articles as excellent or good
Learn more about the work of our research integrity team to safeguard the quality of each article we publish.
Find out more
ORIGINAL RESEARCH article
Front. Environ. Sci. , 23 March 2022
Sec. Environmental Economics and Management
Volume 10 - 2022 | https://doi.org/10.3389/fenvs.2022.857225
Since the GDP-oriented evaluation system was introduced in China, setting ambitious economic growth targets has become a norm for governments at all levels, which promotes rapid economic growth but also causes serious carbon emissions. To achieve high-quality sustainable development, a key issue is to optimize the role of economic growth targets by studying their impact on the environment. In this context, this paper employs the spatial Durbin model to investigate the impact of economic growth targets on CO2 emissions based on panel data of 285 prefecture-level and above cities in China during the period 2005–2018. The results show that economic growth targets have a negative effect and a positive impact on CO2 emissions locally and adjacently, respectively, although the spillover effect decays rapidly in space. Heterogeneity analysis suggests that the adverse impact of economic growth targets on carbon emission reduction varies by city, and is generally lower in cities that have more abundant human, financial, and material resources. Additionally, the mechanistic analysis reveals that fiscal decentralization and environmental decentralization are the key channels through which economic growth targets impact carbon emissions, serving as a mediating and masking effect in local and adjacent regions, respectively. Based on the above findings, this study provides policy recommendations for the government to reduce carbon emissions by optimizing the target management system.
As increasingly more countries have entered into an accelerated period of industrialization and urbanization, the world’s total energy production and consumption have continuously expanded in the past few decades, which provides a reliable safeguard for the stable development of human society while putting tremendous pressure on the ecological environment (Song et al., 2021). In particular, a large amount of CO2 produced by the excessive burning of fossil energy sources is entering the atmosphere, becoming the main cause of the greenhouse effect and aggravating a series of climate problems, such as glacier melting, sea-level rise, land desertification, and extreme weather (Le Xu et al., 2021). Under the no policy scenario, there is nearly a 75% probability that the global temperature will rise by 4°C and a 21% chance that it will change by more than 8°C (Waldhoff and Fawcett, 2011). Such a temperature rise could disrupt the lives of millions of people and even destroy the planet’s ecological balance. Clearly, carbon emission reduction is a major global issue that urgently needs to be addressed (Wang et al., 2020).
Notably, it is more than 90% certain that the global temperature rise since 1950 has been caused by human activities (Hileman, 2007), which implies that the adverse effect of climate change may be delayed or even averted by reducing man-made emissions of greenhouse gases (Le Xu et al., 2021). This situation has aroused the great enthusiasm of international organizations represented by the United Nations to promote cooperative carbon emission reduction among various countries, and the major global economies have actively participated and consciously assumed their corresponding responsibilities. For example, as the world’s largest energy consumer and carbon emitter, China has repeatedly pledged at various international conferences that its carbon intensity will be reduced by 60–65% by 2030 compared to 2005 (Huarong Peng et al., 2021), and its carbon peak and carbon neutrality will be achieved by 2030 and 2060, respectively (Cao et al., 2021).
However, CO2 emissions commonly occur in conjunction with economic growth (Dong et al., 2020), and the latter is also a chief aim pursued by various countries, especially developing countries (Xin Xu et al., 2021). For example, in Chinese culture, economic growth is the key to realizing the vision of a well-off society, and therefore, economic construction is continuously regarded as the central task (Pang et al., 2019). More importantly, with the formation of a GDP-oriented evaluation system, China began to implement economic growth target management in the late 1980s, which became the core mechanism for governments at all levels to manage the economy of their jurisdictions. The basic feature of economic growth target management is that the government presets economic growth targets and formulates a series of development plans and supporting policies to ensure their realization (Liu et al., 2020). However, under promotion tournaments, it has become the norm for local officials to set ambitious economic growth targets and seek short-term rapid economic growth at the expense of the environment. In this sense, governments’ targets seem to fall into a game dilemma between economic growth and carbon emission reduction (Wenbin Peng et al., 2021).
Unfortunately, although economic target management is one of the primary methods of macroeconomic management and is implicitly found to be one of the potential contributors to environmental pollution, its impact on CO2 emissions has not been systematically demonstrated. Since 1950, at least 49 economies, including China, India, and some countries in the European Union, have announced or regularly published economic growth targets to stimulate their economic development. This indicates that exploring the causality between economic growth targets and pollutant emissions is crucial for both developed and developing countries. With this in mind, we attempt to answer the following four questions through rigorous empirical analysis: Do economic growth targets influence CO2 emissions? If so, what are the characteristic effect of economic growth targets on CO2 emissions, such as spatial spillover and regional heterogeneity? Furthermore, what are the mechanisms by which economic growth targets affect CO2 emissions? How do strategic interactions among local governments regarding target management affect carbon emissions?
To fill the abovementioned knowledge gap, this paper first employs the spatial Durbin approach to explore the influence of economic growth targets on CO2 emissions. Second, the geographic distance weight matrix is used to estimate the spillover effect of economic growth targets on CO2 emissions and its geographical distance attenuation. Moreover, according to human, financial, and material resources, the samples are divided into high- and low-resource groups to analyze the heterogeneous effect of economic growth targets on CO2 emissions. Third, from the perspectives of fiscal decentralization and environmental decentralization, the transmission channels through which economic growth targets affect CO2 emissions are discussed.
The marginal contributions of this paper to the literature are as follows: First, this study examines the impact of economic growth targets on CO2 emissions at the prefecture-level city level, enriching the research content related to carbon emissions influencing factors, and providing reliable insights into the link between economic growth targets and carbon emissions. More importantly, we are not aware of any prior literature that explores carbon emissions from the perspective of economic growth target management. Second, the spatial Durbin model is introduced to explore the spillover effect and heterogeneous effect of economic growth targets on CO2 emissions. These results may provide important evidence for local governments to coordinate emissions reduction and implement local policies according to their own actual conditions. Third, a mediation effect model is introduced to determine the mechanisms by which economic growth targets affect CO2 emissions. By doing so, we can track the internal transmission path of economic growth targets, and provide practical solutions that help the government reduce carbon emissions. Thus, the study aims to report reliable results and policy recommendations.
The rest of this paper is structured as follows. Section 2 provides a literature review. Section 3 introduces the method and data. Section 4 provides and summarizes the empirical results. Section 5 concludes and gives some policy implications based on our findings.
The impact of economic growth targets on CO2 emissions is the focus of this paper. Based on this theme, this paper mainly reviews the literature related to economic growth targets and carbon emissions. First, regarding the setting of economic growth targets, the existing literature shows that many factors may affect target setting, such as historical economic growth targets, actual economic growth rates, local leaders’ characteristics, environmental protection targets, and expected targets set by neighboring regions. Ma (2016) finds that the economic growth target last year and its realization have a positive effect on the economic growth target set for this year. Ma (2013) believes that there is a U-shaped curve between officials’ tenure and economic growth targets, and officials who are promoted locally tend to set higher economic growth targets than those who are transferred from the central government and other provinces. Zhang (2021) argues that environmental targets constrain GDP growth targets; however, the substitutive relationship between the two turns into a complementary relationship when environmental performance is incorporated into the cadre evaluation system (Du and Yi, 2021). Additionally, Wang and Huang (2019) point out that the setting of economic growth targets is radical, interactive, and volatile. A region’s target is not only a response to its own economic growth rate, but is also positively related to its competitors’ targets.
Regarding the impact of economic growth targets, academic researchers analyze them from the macro and micro perspectives. At the macro level, Liu et al. (2020) hold that economic growth targets will distort fiscal expenditure, which stalls human capital and technological progress, ultimately hindering long-term economic growth and inducing local governments to manipulate GDP data (Lyu et al., 2018). Chai et al. (2021) argue that economic growth targets may affect sustainable development because of the U-shaped relationship between it and air pollution, and this impact is influenced by human capital, industrial structure, and foreign direct investment. Using panel data of 30 provinces in China during the period 2000–2017, Su et al. (2021) conclude that economic growth targets significantly reduce energy efficiency by distorting marketization. At the micro level, Chen et al. (2021) deem that economic growth targets exhibit a persistent pattern of top-down amplification along with different jurisdictions, which results in enterprise overcapacity in the jurisdiction. Zhong et al. (2021) believe that the pressure to achieve economic growth targets increases PM2.5 concentration and threatens public health, so the government should raise public awareness of environmental protection to reduce this adverse effect.
Furthermore, the mechanism by which economic growth targets work has also been analyzed theoretically. Under the Chinese-style fiscal decentralization system, local taxes are levied by local tax authorities, and local governments are given wide discretionary powers. Thus, they can lower tax rates to reduce corporate tax burdens and expand corporate investment, thereby stimulating local economic growth. Local governments have greater autonomy in fiscal expenditures than revenue, and can independently adjust their fiscal expenditure structures in order to promote economic growth (Jia et al., 2020). Meanwhile, China has a top-down environmental regulatory system, in which the central government formulates regulations and the local governments implement them (Ran, 2013; Sun et al., 2021a). Under the pressure of economic growth targets, environmental governance tools developed by the central government may be distorted by local governments when they are implemented. For example, local governments have considerable control over how pollution fees are collected (Lian et al., 2018), and they can adjust tax categories and collection standards so that pollution fees can serve as an incentive tool for enterprises to expand production (Huang et al., 2020).
Second, the discussion on carbon emissions focuses more on the influencing factors. Several factors have been identified as influencing CO2 emissions, including urbanization, globalization, financial development, economic growth, and corporate social responsibility. Yao et al. (2021) analyze economic urbanization, land urbanization, and population urbanization in China, and believe that there is a nonlinear relationship between the three and carbon emissions. Using an econometric model, Chen et al. (2020) show that political globalization reduces CO2 emissions, and You and Lv (2018) report that economic globalization has a significant negative impact. Farouq et al. (2021) argue that financial globalization uncertainty is inversely related to CO2 emissions, and enhancing the financial market can reduce carbon emissions by improving carbon sequestration capacity and industrial structure (Yang et al., 2022). Many scholars evaluate the environmental Kuznets curve hypothesis by analyzing whether there is an inverted U-shaped relationship between economic growth and carbon emissions. Some of them argue that economic development and CO2 emissions are mutually causal (Kacprzyk and Kuchta, 2020). However, some scholars hold different views (Raza and Lin, 2020; Tinghui Li et al., 2021). Román et al. (2021) assert that there is no significant difference between developed and developing economies when it comes to carbon management, but the existence of a corporate social responsibility committee has a substantial impact. Consequently, enterprises should disclose their corporate social responsibility reports to reduce the likelihood of hidden carbon emissions (Zhenghui Li et al., 2021).
Besides the ones mentioned above, structural, technical, and policy factors also play a significant role in carbon emissions. Sun and Ren (2021) use the Shannon–Wiener diversity index to measure the energy consumption structure, and they find that the more reasonable the energy consumption structure, the lower the carbon emissions levels. Wu et al. (2021) contend that energy-dependent industrial structure plays a partial mediating role in the promotion of carbon emissions by energy endowment, so solar energy might serve as a solution for low-carbon sustainable development (Sun et al., 2021a). As for technical factors, Erdogan (2021) reveals a long-term correlation between technological innovation and carbon emissions in BRIC countries. Sun et al. (2021b) identify a positive correlation between technological innovation and energy efficiency that can significantly reduce carbon intensity (Li and Lin, 2016). The literature on policy factors is arising with the development of global carbon markets and carbon finance, including carbon taxes (O’mahony, 2020; Runst and Thonipara, 2020), carbon pricing (Tvinnereima and Mehling, 2018; Kanamura, 2019), and carbon trading (Jia and Lin, 2020; Yang and Luo, 2020). Using a quasi-natural experiment, Guo et al. (2021) demonstrate that carbon emissions trading policy promotes carbon finance and reduces carbon emissions through technological innovation and industrial upgrading, and has beneficial spillover effects on non-pilot areas.
Throughout the abovementioned literature, it appears that economic growth targets are investigated primarily for their setting, effects, and mechanisms, whereas carbon emissions are discussed based on their affecting factors. However, the relationship between economic growth targets and CO2 emissions is unclear. There are several possible reasons for this ambiguity. One is that the spatial effect of economic growth targets is not taken into account, resulting in biased estimates. Another is that there are significant differences among cities regarding the total amount of various resources, such as human, financial, and material resources (Zameer et al., 2020; Wang et al., 2021), which results in heterogeneous effects of preset targets. In addition, the mechanism by which economic growth targets affect carbon emissions remains an open question. Based on this, this paper examines the impact and mechanism of economic growth targets on CO2 emissions using a spatial econometric model to provide an empirical basis for improving economic growth target management and promoting carbon emission reduction.
To empirically examine the impact of economic growth targets on CO2 emissions, the study employs panel data of 285 prefecture-level and above Chinese cities from 2005 to 2018. According to Shahnazi and Shabani (2021), CO2 would spill over because of inter-regional supply chains, pollution haven hypothesis, and strategic interaction behaviors including competition and imitation, resulting in emissions that are not independent across regions. Therefore, this study employs spatial econometric analysis to investigate the spatial effect of economic growth targets on CO2 emissions. The spatial Durbin model (SDM) encompasses both the spatial autoregressive (SAR) model and the spatial error model (SEM) (LeSage and Pace, 2009), and should therefore be prioritized in empirical analysis according to a general-to-specific strategy (Mur and Angulo, 2009; Chen et al., 2022). The baseline model is set as follows:
where Y and X are the explained and explanatory variables, respectively. WY and WX denote the spatial lag terms of the explained and explanatory variables. ρ indicates the spatial autocorrelation coefficient, and α and θ are spatial regressive coefficients. W denotes a row-normalized spatial weight matrix.
where lnPCE indicates CO2 emissions, lnEGT denotes economic growth targets. α is the linear parameter, ρ denotes the spatial regressive parameter of CO2 emissions, and θ indicates the spatial spillover parameter of economic growth targets on CO2 emissions.
To identify the transmission mechanisms through which economic growth targets impact CO2 emissions, we use the mediation effect proposed by Baron and Kenny (1986). Considering the spatial effect, we refer to Huang et al. (2020) and introduce lag terms for various variables. The model is constructed as follows:
where M denotes the mediating variables, including fiscal decentralization and environmental decentralization.
The distance in geographical space determines the frequency of economic connection, and the difference in economic development affects the degree of resource competition. Consequently, the correlation of carbon emissions between regions may gradually deteriorate with an increasing geographical distance or a growing development gap (Shao et al., 2020). To highlight this feature, we use a geographical distance weight matrix (W1) to estimate the spatial effect and an economic distance weight matrix (W2) for robustness checks. Their construction is as follows:
where dij stands for the great circle distance based on longitude and latitude between city i and city j.
CO2 emissions per capita (lnPCE) are used as the explained variable, which is calculated by dividing total CO2 emissions by population (Churchill et al., 2020; Ren et al., 2021). Although the emission data from the environmental monitor provide an ideal dataset, they have not been released at the prefecture-level city, so we estimate total CO2 emissions using an indirect method. Following Wang and Zhang (2022), the equation is derived as follows:
where CE indicates CO2 emissions, and Cn, Cp, and Ce represent CO2 emissions caused by natural gas, liquefied petroleum gas, and industrial power consumption, respectively. En and Ep denote the natural gas and liquefied petroleum gas consumed, respectively, and Ee is the industrial power consumption.
The explanatory variable is economic growth targets (lnEGT) announced by local governments at the beginning of each year after being approved by the National People’s Congress (Liu et al., 2020). The annual targets are manually collected from the official websites, government work reports, and statistical yearbooks of various cities. The interval data are filled with the mean between upper and lower bounds, and the missing data are filled with the value in the 5-year plan for national economic and social development.
According to previous theoretical research, economic growth targets may affect pollution emissions via decentralization in the fiscal system and environmental governance, so we examine whether these may act as mediators. Fiscal decentralization (lnFD) is measured by the ratio of local budgetary revenue to budgetary expenditure (Wu and Heerink, 2016). The lower the value, the higher the fiscal gap and the more reliant the government is on transfers. Environmental decentralization (lnED) is measured by the number of employees in the water conservancy, environment, and public facilities management industries (Hao et al., 2021). Drawing on Guoxiang Li et al. (2021), the calculation proceeds as follows:
where i and t denote the city and year, respectively; WEPP represents the number of employees in the water conservancy, environment, and public facilities management industry. POP and GDP indicate the registered population and gross domestic product, respectively.
According to Le Xu et al. (2021), five indicators that could influence carbon emissions, namely, economic level (lnEL), industrial structure (lnIS), economic openness (lnEO), technological innovation (lnTI), and population density (lnPD), are added as control variables. The environmental Kuznets curve hypothesis suggests a significant correlation between economic development and environmental pollution; thus, the economic level measured by per capita GDP is controlled (Aller et al., 2021). The industrial structure is expressed by the ratio of secondary industry output to GDP, which illustrates how industrial sectors influence CO2 emissions (Gan et al., 2021). Economic openness may change a region’s comparative advantage by introducing advanced production technology and management experience, which contributes to energy conservation and emission reduction, as measured by the ratio of foreign direct investment (FDI) to GDP (Wenbin Peng et al., 2021). Technological innovation is beneficial for improving energy efficiency and reducing carbon emissions, and it can be represented by the number of patents granted (Cheng et al., 2021). Population density, defined as the proportion of the registered population in the administrative area, can control pollution emissions caused by demographics (Yi et al., 2020).
Since most Chinese cities do not set economic growth targets before 2005 or release the number of employees used to measure environmental decentralization after 2018, and 12 cities, including Lhasa, Haidong, and Danzhou, have many missing values in CO2 emissions, we conduct the empirical analysis using panel data from 285 prefecture-level and above cities from 2005 to 2018. The data come from the EPS database, CEIC database, statistical yearbooks, and government reports. Missing values are supplemented by interpolation and mean. All variables are used as the natural logarithm to process potential heteroscedasticity. For illustration purposes, the definitions and descriptive statistics of variables are presented in Table 1.
The Moran’s I index is employed to analyze the spatial autocorrelation of explained and explanatory variables, and the results with the geographical distance weight matrix are reported in Table 2. From 2005 to 2018, the Moran’s I indexes of lnPCE and lnEGT are positive and pass the 1% significance test, indicating that there is a positive spatial autocorrelation among regions regarding CO2 emissions and expected targets. Notably, the Moran’s I statistics of lnPCE present a U-shaped curve, and the Moran’s I statistics of lnEGT show a fluctuating upward trend. This indicates the spatial autocorrelation among regions in both CO2 emissions and economic growth targets appears to be strengthening. As ecological pollution and environmental degradation become increasingly serious, the central government has introduced environmental protection indicators into the official performance evaluation system to transform the GDP-oriented development model, which results in a stronger strategic interaction between local governments on economic growth and environmental governance.
To identify spatial heterogeneity, one needs to perform spatial autocorrelation tests using the local Moran’s index. Figures 1, 2 are scatter plots of the local Moran’s index for lnPCE and lnEGT under the geographical distance weight matrix in 2005 and 2018, respectively. The results show that most of the samples are situated in the first and third quadrants, suggesting that CO2 emissions and economic growth targets are characterized by high-high and low-low agglomeration. Accordingly, the local Moran’s index illustrates a clear positive spatial autocorrelation among regions in both CO2 emissions and economic growth targets, in line with the global Moran’s index.
The spatial autocorrelation test indicates that spatial econometric analysis should be used in this paper, and therefore, a series of tests are conducted to select the optimal model. Drawing on LeSage and Pace (2009), the specific steps are shown in Table 3. First, the LM lag, robust-LM lag, LM error, and robust-LM error tests suggest that spatial econometric analysis is more suitable than ordinary regression. Second, the Wald lag, Wald error, LR lag, and LR error tests reject the null hypothesis that the SDM degenerates to SAR or SEM at the 1% significance level. Third, the Hausman test implies that the fixed effect should be adopted over the random effect, and the R-squared and log-likelihood tests show that the space-fixed effect is optimal. Thus, the SDM with a space-fixed effect is selected for the empirical analysis.
The estimation results using SDM are presented in Column (4) of Table 3, and as a comparison, the results using OLS, SAR, and SEM are also given in Columns (1)–(3). As shown in Column (4), the spatial autocorrelation coefficient ρ is 0.275 and is significant at the 1% level, suggesting that every 1% increase in local CO2 emissions corresponds to a 0.275% increase in surrounding areas. This finding is in keeping with the results of the spatial autocorrelation test. A city’s carbon emissions are influenced not only by the local environment but also by the spatial effects of various factors, such as technology innovation, government intervention, and environmental regulations in neighboring regions (Wenbin Peng et al., 2021).
The decomposition effect results are given in Table 4. The direct effect indicates that a 1% increase in the local economic growth target causes a 0.165% decrease in local CO2 emissions. That is, economic growth targets have a negative local effect, which reduces local carbon emissions. As environmental governance is linked to performance appraisal, local governments have expanded the single indicator of maximizing GDP into a hybrid indicator that includes prospective economic goals and constrained environmental goals. Thus, local governments tend to develop the economy more rationally than they used to, such as by promoting technological innovation and optimizing industrial structure, and they also use these results to reduce pollution. Notably, the coefficient of lnEGT in Table 4 is slightly different from that in Table 3. This is due to the feedback effect that results from impacts caused by the local region that then spread to neighboring regions and are acted on again in the local region, and it is numerically equal to the difference between the direct and main effects. For example, the direct and main effects of lnEGT are −0.165 and −0.167, respectively, so the feedback effect is equal to 0.002, which means that it weakens the inhibiting effect of economic growth targets on CO2 emissions.
The indirect effect indicates that a 1% increase in the local economic growth target may induce a 0.909% increase in CO2 emissions in neighboring regions. This result implies that economic growth targets have a positive spillover effect. On the one hand, local governments will compete for liquidity resources to develop their own economy, which leads to a siphon effect that lowers energy efficiency in neighboring regions. On the other hand, local governments may force high-polluting and high-emission enterprises to relocate to downstream border areas, thereby realizing pollution transfer. Additionally, the indirect effect is much stronger than the direct effect, indicating that the total effect is significantly positive; that is, economic growth targets severely exacerbate carbon emissions.
As for the control variables, the coefficient of the secondary industry’s GDP ratio is statistically insignificant, as determined by Huang et al. (2020). Although there are a variety of high-pollution sectors in the secondary industry, energy consumption can be reduced by restraining the development of other industrial sectors (Shao et al., 2020). The direct effect of lnEO is significantly positive, suggesting that FDI increases local CO2 emissions. This is in line with the pollution haven hypothesis. The direct and indirect effects of lnEL are significantly negative and positive, respectively, which means that economic development reduces local CO2 emissions but increases neighbors’ CO2 emissions. The direct and indirect effects of lnTI are significantly negative and positive, respectively, indicating that technological innovation inhibits CO2 emissions in the local area but promotes emissions in neighboring areas, respectively. The direct and indirect effects of lnPD are insignificantly and significantly negative, implying that the larger population size increases neighbors’ carbon emissions. As mentioned by Zheng et al. (2014), economic development, population growth, and technological innovation are associated with agglomeration forces that increase local resource efficiency but exacerbate neighbors’ carbon emissions by reducing energy efficiency (Chica-Olmo et al., 2020).
This paper conducts a robustness test by replacing the explained variable and matrix, and the estimation results are presented in Table 5. Column (1) uses total CO2 emissions rather than per capita CO2 emissions are the explained variable. The spatial matrix in Column (2) is replaced by the economic distance weight matrix. In Column (3), both the explained variable and the spatial matrix are replaced. As shown in Table 5, the coefficients of lnEGT and WlnEGT are statistically negative and positive, respectively, indicating that economic growth targets are conducive to carbon emission reduction in the local area but not in the neighboring areas. Moreover, the decomposition results indicate that the direct and indirect effects of lnEGT are negative and positive, respectively, which is in line with the baseline regression. For example, the direct and indirect effects of lnEGT are -0.114 and 0.436 in Column (3), respectively, which means that a 1% increase in economic growth targets can result in a 0.114% decrease and 0.436% increase in CO2 emissions for local and neighboring areas, respectively. In short, the robustness test results are consistent with the baseline regression results, demonstrating the reliability of our findings.
To capture the characteristics of spatial spillover effects, the spatial matrix is reset as follows: The element is recorded as the reciprocal of the geographic distance between two cities if that distance is less than the threshold distance; otherwise, it is recorded as 0. The initial value of threshold distance and progressive distance are both set to 100 km, The threshold distance and progressive distance are both set to 100 km, so the spatial econometric analysis is conducted from 100 km and performed every 100 km thereafter.
Table 6 presents the decomposition results within 1,400 km. The local and spillover effects can be divided into three intervals. The first interval is within 400 km, the direct and indirect effects are statistically negative and positive, respectively, and the inhibiting and promoting effects of economic growth targets on local and neighboring carbon emissions increase with geographic distance. Most cities within 400 km are geographically adjacent or are part of the same province. While they engage in vicious competition for social resources and political promotion, they also participate in cross-regional cooperation to reduce carbon emissions. The second interval ranges between 500 and 1,200 km. The direct and indirect effects of preset targets on CO2 emissions in local and nearby areas diminish as distance increases. In terms of career advancement, local officials are mainly competing with their peers from their provinces. Consequently, there is little or no strategic interaction between cross-regional cities in setting economic growth targets, which reduces the amplification of preset targets and the competitiveness for liquidity resources. More than 1,200 km is the third interval. The direct effects are irregular, and the indirect effects are no longer significant. This is mainly because most of the elements in the geographical distance weight matrix are 0, which leads to the white noise sequence. In summary, the spatial spillover effect of economic growth targets on CO2 emissions is attenuated by geographical distance.
To investigate the difference of economic growth target on carbon emissions among cities with different resource endowments, we use per capita education expenditure, deposits and loans to GDP, and fixed-asset investment to GDP as substitutes for human, financial, and material resources, respectively, and then divide the samples into high- and low-resource groups according to the median value for analysis.
We pay more attention to the role of the core variable, namely, economic growth targets. In Columns (1)–(2), the direct effects are significantly negative, indicating that economic growth targets increase CO2 emissions in cities with different human resources. The indirect effects in Columns (1)–(2) are insignificant and significant, respectively, which means that preset targets in cities with high human resources have no spillover effects, whereas those in cities with low human resources worsen neighbors’ carbon emissions. Carbon emission reduction requires not only well-educated labor forces to develop clean technologies to reduce energy consumption, but also highly skilled workers to put these technologies into practice to improve energy efficiency. Economic growth targets and environmental protection goals put great pressure on many cities, especially those with limited human resources, to compete for highly skilled workers, resulting in a reduction in local carbon emissions and an increase in neighbors’ carbon emissions.
In Columns (3)–(4), the direct effects of lnEGT are statistically negative, suggesting that economic growth targets reduce CO2 emissions in cities with various financial resources. The indirect effects of lnEGT are significantly positive, which means that local economic growth targets worsen neighbors’ CO2 emissions. Notably, the direct and indirect effects are higher in cities with low financial resources than in cities with high financial resources. This means that local inhibition and adjacent promotion of preset targets are weaker in cities with high financial resources than in cities with low financial resources. Several initiatives that contribute to carbon emission reduction, including industrial upgrading and technological innovation, are inseparable from financial support. However, under promotion tournaments, local officials may demonstrate their abilities to superiors for career advancement by pursuing high economic growth rates. In turn, this will prompt them to intervene in bank management decisions, such as loan issuance, resulting in less support for carbon emission reduction investments and financing activities.
Third, the direct effects of lnEGT in Columns (5)–(6) are significant and insignificantly negative, respectively, indicating that the economic growth target reduces CO2 emissions in cities with high material resources, but does not reduce CO2 emissions in cities with low material resources. The indirect effects of lnEGT are significantly positive in Columns (5)–(6), which means that economic growth targets in the two types of cities increase their neighbors’ CO2 emissions. These results imply that carbon emissions can only be materially reduced when the infrastructure construction is improved in the fields of environmental protection and carbon markets; that is, material resources are essential for low-carbon development. Overall, the findings not only verify baseline results on a whole, but also indicate that there are heterogeneous effects in cities with differently endowed resources Table 7.
In this section, we reveal we reveal how economic growth targets affect CO2 emissions. The results with fiscal decentralization as a mediation variable are presented in Columns (1)–(2) of Table 8. The coefficient of lnEGT is significantly positive in Column (1), indicating that economic growth targets contribute to fiscal decentralization. The coefficients of lnFD and WlnFD in Column (2) are negative, although the latter is not statistically significant. Additionally, the coefficients of lnEGT and WlnEGT remain significantly negative and positive after introducing the fiscal decentralization variable. The sign of the product of lnEGT in Column (1) and lnFD in Column (2) is the same as that of lnEGT in Column (2), whereas the sign of the product of lnEGT in Column (1) and WlnFD in Column (2) is opposite to that of WlnEGT in Column (2). These results mean that fiscal decentralization plays a mediating and masking role in linking economic growth targets and CO2 emissions in local and neighboring regions, respectively. In other words, economic growth targets improve local inhibition and weaken adjacent promotion by promoting fiscal decentralization. For example, the coefficient of lnEGT is 0.153, and the direct and indirect effects of lnFD are -0.117 and -0.314, which means that a 1% increase in fiscal decentralization could reduce CO2 emissions in local and neighboring regions by 0.018 and 0.048%, respectively.
Columns (3)–(4) show the results of environmental decentralization as a mediation variable. The coefficient of lnEGT is negative and passes the 5% significance test in Column (3), indicating that economic growth targets could hinder environmental decentralization. The coefficients of lnED and WlnED in Column (4) are positive and pass the 1% significance test. Furthermore, after introducing the environmental decentralization variable, the coefficients of lnEGT and WlnEGT remain significantly negative and positive, respectively. Thus, the sign of the product of lnEGT in Column (3) and lnED in Column (4) is the same as that of lnEGT in Column (4), while the sign of the product of lnEGT in Column (3) and WlnED in Column (4) is different from that of WlnEGT in Column (4). These findings imply that environmental decentralization plays a mediating and masking role in the relationship between economic growth targets and local and neighbors’ CO2 emissions, respectively. That is, environmental decentralization increases the inhibition of economic growth targets on local CO2 emissions and decreases the promotion of economic growth targets on neighbors’ CO2 emissions. For instance, the lnEGT coefficient is -0.042, and the direct and indirect effects of lnED are 0.143 and 1.763, indicating that a 1% increase in environmental decentralization could reduce local and neighbors’ CO2 emissions by 0.006 and 0.074%, respectively.
Based on these findings, we conclude that economic growth targets exert their influence on carbon emissions through fiscal decentralization and environmental decentralization. Specifically, economic growth targets reduce local CO2 emissions by promoting fiscal decentralization and inhibiting environmental decentralization. Meanwhile, economic growth targets increase neighbors’ CO2 emissions by improving the negative spillover effect of fiscal decentralization and the positive spillover effect of environmental decentralization. Environmental pollution will not only impact regional economic development by limiting foreign investment and talent introduction, but also restrict local officials’ career development as the central government implements a one-vote veto system for environmental protection. Thus, with sufficient political incentives, local governments will strengthen pollution control while developing the local economy. Fiscal decentralization and environmental decentralization mean that local governments have the initiative to promote coordinated development between the economy and the environment, and they can optimize fiscal expenditure structure and formulate environmental protection policies based on local circumstances, which may reduce pollution more effectively.
This paper uses the spatial Durbin model to explore the impact of economic growth targets on CO2 emissions based on panel data of 285 prefecture-level and above cities in China from 2005 to 2018. This study suggests that economic growth targets have a negative local effect and a positive spillover effect on CO2 emissions. That is, economic growth targets contribute to local carbon emission reduction, but exacerbate neighbors’ carbon emissions. Notably, the spatial spillover effect decays with geographic distance and is only significant within 1,200 km. Furthermore, when the sample is divided into high- and low-resource groups, this study shows that the impact of economic growth targets on CO2 emissions is heterogeneous, and is generally lower in cities with more abundant human, financial, and material resources. Finally, our mechanism analysis indicates that economic growth targets reduce local CO2 emissions by improving the inhibition of fiscal decentralization and the promotion of environmental decentralization, and increase neighbors’ CO2 emissions by improving the negative spillover effect of fiscal decentralization and the positive spillover effect of environmental decentralization. From this outcome, fiscal decentralization and environmental decentralization will impact carbon emissions. The government should set appropriate economic growth targets to promote environmental sustainability.
The above findings have some policy implications for carbon emission reduction. First, the central government should adjust the weight of economic growth in the performance evaluation system, and reverse local governments’ tendency to pursue high economic growth goals, so as to correct their distorted behavior and production bias. Meanwhile, some hard constraints that can encourage pollution reduction and environmental optimization, such as total energy consumption and energy consumption intensity, should be given more weight, and several indicators that illustrate ecological civilization, high-quality development, and public demands must be set, such as environmental protection, technological progress, and basic services. More importantly, it is crucial to supervise the completion of environmental goals by rewarding areas that meet the standards and punishing those that do not, so that local governments are encouraged to adjust their target management and regional development strategy. Second, the central government should decentralize its administrative power to exploit the cost and information advantages of local governments in carbon emission reduction, and adopt fiscal policies to increase the enthusiasm of local governments in environmental governance. For example, it could increase special transfer payments and reform environmental protection tax. Meanwhile, local governments should increase environmental governance investment by adjusting the structure of fiscal expenditure, and promote technological upgrading by providing R&D subsidies to polluting enterprises. Finally, the government should introduce a cross-regional carbon emissions governance mechanism and carbon emissions trading system.
Although we conclude that preset targets are not conducive to carbon emission reduction and put forward policy suggestions for target management, whether and to what extent this has an impact has not been investigated. In addition, China is not the only country that implements economic growth target management. Future research can be conducted by collecting data from other countries that set expected targets. There may be new evidence related to our findings in this paper or novel insights into the association between target management and carbon emissions (Chen et al., 2020).
The raw data supporting the conclusions of this article will be made available by the authors, without undue reservation.
TG: software, methodology, writing original draft, and project administration. LM: data curation, writing—review and editing. CW: conceptualization, investigation, supervision.
This study was supported by the National Social Science Foundation of China (No. 21CJL016), and the Social Science Foundation of Nantong (No. 2021CNT002).
The authors declare that the research was conducted in the absence of any commercial or financial relationships that could be construed as a potential conflict of interest.
All claims expressed in this article are solely those of the authors and do not necessarily represent those of their affiliated organizations, or those of the publisher, the editors and the reviewers. Any product that may be evaluated in this article, or claim that may be made by its manufacturer, is not guaranteed or endorsed by the publisher.
Aller, C., Ductor, L., and Grechyna, D. (2021). Robust Determinants of CO2 Emissions. Energ. Econ. 96, 105154. doi:10.1016/j.eneco.2021.105154
Baron, R. M., and Kenny, D. A. (1986). The Moderator-Mediator Variable Distinction in Social Psychological Research: Conceptual, Strategic, and Statistical Considerations. J. Personal. Soc. Psychol. 51 (6), 1173–1182. doi:10.1037/0022-3514.51.6.1173
Cao, J., Dai, H., Li, S., Guo, C., Ho, M., Cai, W., et al. (2021). The General Equilibrium Impacts of Carbon Tax Policy in China: A Multi-Model Comparison. Energ. Econ. 99, 105284. doi:10.1016/j.eneco.2021.105284
Chai, J., Hao, Y., Wu, H., and Yang, Y. (2021). Do Constraints Created by Economic Growth Targets Benefit Sustainable Development? Evidence from China. Bus Strat Env 30 (8), 4188–4205. doi:10.1002/bse.2864
Chen, J., Chen, X., Hou, Q., and Hu, M. (2021). Haste Doesn't Bring Success: Top-Down Amplification of Economic Growth Targets and Enterprise Overcapacity. J. Corporate Finance 70, 102059. doi:10.1016/j.jcorpfin.2021.102059
Chen, T., Gozgor, G., Koo, C. K., and Lau, C. K. M. (2020). Does International Cooperation Affect CO2 Emissions? Evidence from OECD Countries. Environ. Sci. Pollut. Res. 27, 8548–8556. doi:10.1007/s11356-019-07324-y
Chen, Y., Shao, S., Fan, M., Tian, Z., and Yang, L. (2022). One Man's Loss Is Another's Gain: Does Clean Energy Development Reduce CO2 Emissions in China? Evidence Based on the Spatial Durbin Model. Energ. Econ. 107, 105852. doi:10.1016/j.eneco.2022.105852
Cheng, C., Ren, X., Dong, K., Dong, X., and Wang, Z. (2021). How Does Technological Innovation Mitigate CO2 Emissions in OECD Countries? Heterogeneous Analysis Using Panel Quantile Regression. J. Environ. Manage. 280, 111818. doi:10.1016/j.jenvman.2020.111818
Chica-Olmo, J., Sari-Hassoun, S., and Moya-Fernández, P. (2020). Spatial Relationship between Economic Growth and Renewable Energy Consumption in 26 European Countries. Energ. Econ. 92, 104962. doi:10.1016/j.eneco.2020.104962
Churchill, S. A., Inekwe, J., and Ivanovski, K. (2020). Stochastic Convergence in Per Capita CO2 Emissions: Evidence from Emerging Economies, 1921-2014. Energ. Econ. 86, 104659. doi:10.1016/j.eneco.2019.104659
Dong, B., Xu, Y., and Fan, X. (2020). How to Achieve a Win-Win Situation between Economic Growth and Carbon Emission Reduction: Empirical Evidence from the Perspective of Industrial Structure Upgrading. Environ. Sci. Pollut. Res. 27, 43829–43844. doi:10.1007/s11356-020-09883-x
Du, J., and Yi, H. (2021). Target‐setting, Political Incentives, and the Tricky Trade‐off between Economic Development and Environmental protection. Public Admin. doi:10.1111/padm.12768
Erdogan, S. (2021). Dynamic Nexus between Technological Innovation and Building Sector Carbon Emissions in the BRICS Countries. J. Environ. Manage. 293, 112780. doi:10.1016/j.jenvman.2021.112780
Farouq, I. S., Sambo, N. U., Umar Sambo, N., Ahmad, A. U., Jakada, A. H., and Danmaraya, I. i. A. (2021). Does Financial Globalization Uncertainty Affect CO2 Emissions? Empirical Evidence from Some Selected SSA Countries. Quant. Financ. Econ. 5 (2), 247–263. doi:10.3934/QFE.2021011
Gan, T., Yang, H., and Liang, W. (2021). How Do Urban Haze Pollution and Economic Development Affect Each Other? Empirical Evidence from 287 Chinese Cities during 2000-2016. Sust. Cities Soc. 65, 102642. doi:10.1016/j.scs.2020.102642
Guo, Q., Su, Z., and Chiao, C. (2021). Carbon Emissions Trading Policy, Carbon Finance, and Carbon Emissions Reduction: Evidence from a Quasi-Natural Experiment in China. Econ. Change Restruct. doi:10.1007/s10644-021-09353-5
Guoxiang Li, G., Guo, F., and Di, D. (2021). Regional Competition, Environmental Decentralization, and Target Selection of Local Governments. Sci. Total Environ. 755, 142536. doi:10.1016/j.scitotenv.2020.142536
Hao, Y., Gai, Z., Yan, G., Wu, H., and Irfan, M. (2021). The Spatial Spillover Effect and Nonlinear Relationship Analysis between Environmental Decentralization, Government Corruption and Air Pollution: Evidence from China. Sci. Total Environ. 763, 144183. doi:10.1016/j.scitotenv.2020.144183
Hileman, B. (2007). UN Issues Dire Climate Warnings. Chem. Eng. News Archive 85 (7), 17. doi:10.1021/cen-v085n007.p017
Huang, G., Zhang, J., Yu, J., and Shi, X. (2020). Impact of Transportation Infrastructure on Industrial Pollution in Chinese Cities: A Spatial Econometric Analysis. Energ. Econ. 92, 104973. doi:10.1016/j.eneco.2020.104973
Huarong Peng, H., Qi, S., and Cui, J. (2021). The Environmental and Economic Effects of the Carbon Emissions Trading Scheme in China: The Role of Alternative Allowance Allocation. Sustainable Prod. Consumption 28, 105–115. doi:10.1016/j.spc.2021.03.031
Jia, J., Ding, S., and Liu, Y. (2020). Decentralization, Incentives, and Local Tax Enforcement. J. Urban Econ. 115, 103225. doi:10.1016/j.jue.2019.103225
Jia, Z., and Lin, B. (2020). Rethinking the Choice of Carbon Tax and Carbon Trading in China. Technol. Forecast. Soc. Change 159, 120187. doi:10.1016/j.techfore.2020.120187
Kacprzyk, A., and Kuchta, Z. (2020). Shining a New Light on the Environmental Kuznets Curve for CO2 Emissions. Energ. Econ. 87, 104704. doi:10.1016/j.eneco.2020.104704
Kanamura, T. (2019). Supply-side Perspective for Carbon Pricing. Quant. Financ. Econ. 3 (1), 109–123. doi:10.3934/QFE.2019.1.109
Le Xu, L., Fan, M., Yang, L., and Shao, S. (2021). Heterogeneous Green Innovations and Carbon Emission Performance: Evidence at China's City Level. Energ. Econ. 99, 105269. doi:10.1016/j.eneco.2021.105269
LeSage, J., and Pace, R. K. (2009). Introduction to Spatial Econometrics. Boca Raton, FL: CRC Press.
Li, K., and Lin, B. (2016). China's Strategy for Carbon Intensity Mitigation Pledge for 2020: Evidence from a Threshold Cointegration Model Combined with Monte-Carlo Simulation Methods. J. Clean. Prod. 118, 37–47. doi:10.1016/j.jclepro.2015.12.093
Lian, X., Gong, Q., and Wang, L. F. S. (2018). Consumer Awareness and Ex-Ante versus Ex-Post Environmental Policies Revisited. Int. Rev. Econ. Finance 55, 68–77. doi:10.1016/j.iref.2018.01.014
Liu, D., Xu, C., Yu, Y., Rong, K., and Zhang, J. (2020). Economic Growth Target, Distortion of Public Expenditure and Business Cycle in China. China Econ. Rev. 63, 101373. doi:10.1016/j.chieco.2019.101373
Lyu, C., Wang, K., Zhang, F., and Zhang, X. (2018). GDP Management to Meet or Beat Growth Targets. J. Account. Econ. 66 (1), 318–338. doi:10.1016/j.jacceco.2018.07.001
Ma, L. (2013). Promotion Incentive of Government Officials and Government Performance Target-Setting: An Empirical Analysis of Provincial Panel Data in China. J. Public Manag. 10 (2), 28–39. (In Chinese).
Ma, L. (2016). Performance Feedback, Government Goal-Setting and Aspiration Level Adaptation: Evidence from Chinese Provinces. Public Admin 94 (2), 452–471. doi:10.1111/padm.12225
Mur, J., and Angulo, A. (2009). Model Selection Strategies in a Spatial Setting: Some Additional Results. Reg. Sci. Urban Econ. 39 (2), 200–213. doi:10.1016/j.regsciurbeco.2008.05.018
O’Mahony, T. (2020). State of the Art in Carbon Taxes: A Review of the Global Conclusions. Green. Finance 2 (4), 409–423. doi:10.3934/GF.2020022
Pang, R., Zheng, D., Shi, M., and Zhang, X. (2019). Pollute First, Control Later? Exploring the Economic Threshold of Effective Environmental Regulation in China's Context. J. Environ. Manage. 248, 109275. doi:10.1016/j.jenvman.2019.109275
Ran, R. (2013). Perverse Incentive Structure and Policy Implementation Gap in China's Local Environmental Politics. J. Environ. Pol. Plann. 15 (1), 17–39. doi:10.1080/1523908X.2012.752186
Raza, M. Y., and Lin, B. (2020). Decoupling and Mitigation Potential Analysis of CO2 Emissions from Pakistan's Transport Sector. Sci. Total Environ. 730, 139000. doi:10.1016/j.scitotenv.2020.139000
Ren, Y.-S., Ma, C.-Q., Apergis, N., and Sharp, B. (2021). Responses of Carbon Emissions to Corruption across Chinese Provinces. Energ. Econ. 98, 105241. doi:10.1016/j.eneco.2021.105241
Román, C., Zorio-Grima, A., and Merello, P. (2021). Economic Development and CSR Assurance: Important Drivers for Carbon Reporting… yet Inefficient Drivers for Carbon Management? yet Inefficient Drivers for Carbon Management? Technol. Forecast. Soc. Change 163, 120424. doi:10.1016/j.techfore.2020.120424
Runst, P., and Thonipara, A. (2020). Dosis Facit Effectum Why the Size of the Carbon Tax Matters: Evidence from the Swedish Residential Sector. Energ. Econ. 91, 104898. doi:10.1016/j.eneco.2020.104898
Shahnazi, R., and Dehghan Shabani, Z. (2021). The Effects of Renewable Energy, Spatial Spillover of CO2 Emissions and Economic freedom on CO2 Emissions in the EU. Renew. Energ. 169, 293–307. doi:10.1016/j.renene.2021.01.016
Shao, S., Zhang, Y., Tian, Z., Li, D., and Yang, L. (2020). The Regional Dutch Disease Effect within China: A Spatial Econometric Investigation. Energ. Econ. 88, 104766. doi:10.1016/j.eneco.2020.104766
Song, Y., Cheng, J., Zhang, Y., Dai, T., and Huang, J. (2021). Direct and Indirect Effects of Heterogeneous Technical Change on Metal Consumption Intensity: Evidence from G7 and BRICS Countries. Resour. Pol. 71, 101995. doi:10.1016/j.resourpol.2021.101995
Su, X., Yang, X., Zhang, J., Yan, J., Zhao, J., Shen, J., et al. (2021). Analysis of the Impacts of Economic Growth Targets and Marketization on Energy Efficiency: Evidence from China. Sustainability 13 (8), 4393. doi:10.3390/su13084393
Sun, H., Awan, R. U., Nawaz, M. A., Mohsin, M., Rasheed, A. K., and Iqbal, N. (2021a). Assessing the Socio-Economic Viability of Solar Commercialization and Electrification in South Asian Countries. Environ. Dev. Sustain. 23, 9875–9897. doi:10.1007/s10668-020-01038-9
Sun, H., Edziah, B. K., Kporsu, A. K., Sarkodie, S. A., and Taghizadeh-Hesary, F. (2021b). Energy Efficiency: The Role of Technological Innovation and Knowledge Spillover. Technol. Forecast. Soc. Change 167, 120659. doi:10.1016/j.techfore.2021.120659
Sun, W., and Ren, C. (2021). The Impact of Energy Consumption Structure on China's Carbon Emissions: Taking the Shannon-Wiener index as a New Indicator. Energ. Rep. 7, 2605–2614. doi:10.1016/j.egyr.2021.04.061
Tinghui Li, T., Li, X., and Liao, G. (2021). Business Cycles and Energy Intensity. Evidence from Emerging Economies. Borsa Istanbul Rev.. doi:10.1016/j.bir.2021.07.005
Tvinnereim, E., and Mehling, M. (2018). Carbon Pricing and Deep Decarbonisation. Energy Policy 121, 185–189. doi:10.1016/j.enpol.2018.06.020
Waldhoff, S. T., and Fawcett, A. A. (2011). Can Developed Economies Combat Dangerous Anthropogenic Climate Change without Near-Term Reductions from Developing Economies? Climatic Change 107, 635–641. doi:10.1007/s10584-011-0132-7
Wang, H., Cao, R., and Zeng, W. (2020). Multi-Agent Based and System Dynamics Models Integrated Simulation of Urban Commuting Relevant Carbon Dioxide Emission Reduction Policy in China. J. Clean. Prod. 272, 122620. doi:10.1016/j.jclepro.2020.122620
Wang, H., and Zhang, R. (2022). Effects of Environmental Regulation on CO2 Emissions: An Empirical Analysis of 282 Cities in China. Sust. Prod. Consumption 29, 259–272. doi:10.1016/j.spc.2021.10.016
Wang, R., Tan, J., and Yao, S. (2021). Are Natural Resources a Blessing or a Curse for Economic Development? the Importance of Energy Innovations. Resour. Pol. 72, 102042. doi:10.1016/j.resourpol.2021.102042
Wang, X., and Huang, L. (2019). Local Economic Growth Target Management——A Triple-Factor Framework of Theoretical Construction and Empirical Test. Econ. Theor. Business Manag. 9, 30–44. (In Chinese). doi:10.3969/j.issn.1000-596X.2019.09.004
Wenbin Peng, W., Yin, Y., Kuang, C., Wen, Z., and Kuang, J. (2021). Spatial Spillover Effect of Green Innovation on Economic Development Quality in China: Evidence from a Panel Data of 270 Prefecture-Level and above Cities. Sust. Cities Soc. 69, 102863. doi:10.1016/j.scs.2021.102863
Wu, L., Sun, L., Qi, P., Ren, X., and Sun, X. (2021). Energy endowment, industrial structure upgrading, and CO2 emissions in China: Revisiting resource curse in the context of carbon emissions. Resour. Pol. 74, 102329. doi:10.1016/j.resourpol.2021.102329
Wu, Y., and Heerink, N. (2016). Foreign Direct Investment, Fiscal Decentralization and Land Conflicts in China. China Econ. Rev. 38, 92–107. doi:10.1016/j.chieco.2015.11.014
Xin Xu, X., Huang, S., and An, H. (2021). Identification and causal analysis of the influence channels of financial development on CO2 emissions. Energy Policy 153, 112277. doi:10.1016/j.enpol.2021.112277
Yang, J., Luo, P., and Luo, P. (2020). Review on International Comparison of Carbon Financial Market. Green. Finance 2 (1), 55–74. doi:10.3934/GF.2020004
Yang, Z., Yu, L., Liu, Y., Yin, Z., and Xiao, Z. (2022). Financial Inclusion and Carbon Reduction: Evidence from Chinese Counties. Front. Environ. Sci. 9, 221. doi:10.3389/fenvs.2021.793221
Yao, F., Zhu, H., and Wang, M. (2021). The Impact of Multiple Dimensions of Urbanization on CO2 Emissions: A Spatial and Threshold Analysis of Panel Data on China's Prefecture-Level Cities. Sust. Cities Soc. 73, 103113. doi:10.1016/j.scs.2021.103113
Yi, F., Ye, H., Wu, X., Zhang, Y. Y., and Jiang, F. (2020). Self-Aggravation Effect of Air Pollution: Evidence from Residential Electricity Consumption in China. Energ. Econ. 86, 104684. doi:10.1016/j.eneco.2020.104684
You, W., and Lv, Z. (2018). Spillover effects of economic globalization on CO2 emissions: A spatial panel approach. Energ. Econ. 73, 248–257. doi:10.1016/j.eneco.2018.05.016
Zameer, H., Yasmeen, H., Wang, R., Tao, J., and Malik, M. N. (2020). An Empirical Investigation of the Coordinated Development of Natural Resources, Financial Development and Ecological Efficiency in China. Resour. Pol. 65, 101580. doi:10.1016/j.resourpol.2020.101580
Zhang, P. (2021). Target Interactions and Target Aspiration Level Adaptation: How Do Government Leaders Tackle the "Environment‐Economy" Nexus? Public Admin. Rev. 81 (2), 220–230. doi:10.1111/puar.13184
Zheng, X., Yu, Y., Wang, J., and Deng, H. (2014). Identifying the Determinants and Spatial Nexus of Provincial Carbon Intensity in China: A Dynamic Spatial Panel Approach. Reg. Environ. Change 14 (4), 1651–1661. doi:10.1007/s10113-014-0611-2
Zhenghui Li, Z., Zou, F., and Mo, B. (2021). Does mandatory CSR disclosure affect enterprise total factor productivity? Econ. Research-Ekonomska Istraživanja, 1–20. doi:10.1080/1331677X.2021.2019596
Keywords: economic growth target, CO 2 emissions, spillover effect, spatial econometric model, China
Citation: Ge T, Ma L and Wang C (2022) Spatial Effect of Economic Growth Targets on CO2 Emissions: Evidence From Prefectural-Level Cities in China. Front. Environ. Sci. 10:857225. doi: 10.3389/fenvs.2022.857225
Received: 18 January 2022; Accepted: 28 February 2022;
Published: 23 March 2022.
Edited by:
Guo Wei, University of North Carolina at Pembroke, United StatesCopyright © 2022 Ge, Ma and Wang. This is an open-access article distributed under the terms of the Creative Commons Attribution License (CC BY). The use, distribution or reproduction in other forums is permitted, provided the original author(s) and the copyright owner(s) are credited and that the original publication in this journal is cited, in accordance with accepted academic practice. No use, distribution or reproduction is permitted which does not comply with these terms.
*Correspondence: Cang Wang, ZGF5aW4zMjRAMTI2LmNvbQ==
Disclaimer: All claims expressed in this article are solely those of the authors and do not necessarily represent those of their affiliated organizations, or those of the publisher, the editors and the reviewers. Any product that may be evaluated in this article or claim that may be made by its manufacturer is not guaranteed or endorsed by the publisher.
Research integrity at Frontiers
Learn more about the work of our research integrity team to safeguard the quality of each article we publish.