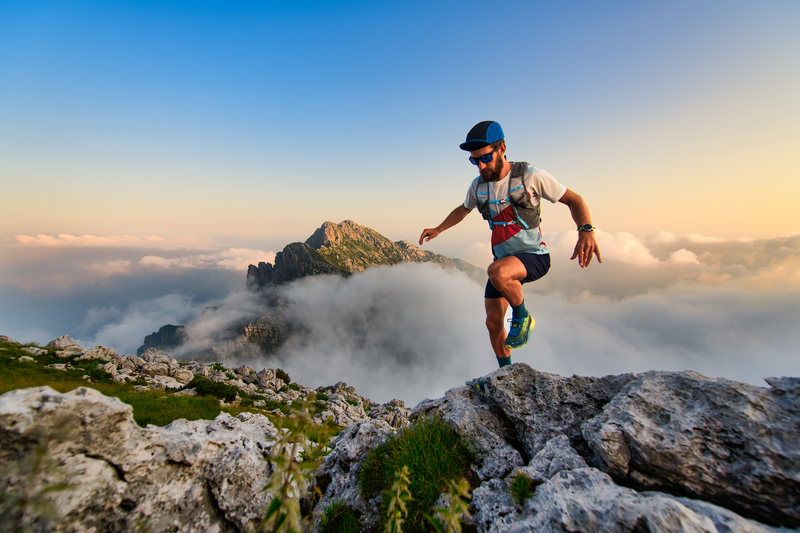
94% of researchers rate our articles as excellent or good
Learn more about the work of our research integrity team to safeguard the quality of each article we publish.
Find out more
ORIGINAL RESEARCH article
Front. Environ. Sci. , 06 June 2022
Sec. Soil Processes
Volume 10 - 2022 | https://doi.org/10.3389/fenvs.2022.852241
This article is part of the Research Topic Agricultural Diversification: Benefits and Barriers for Sustainable Soil Management View all 12 articles
There is a renewed global awareness to improve soil health through the intensification and management of organic inputs such as the application of animal waste–based digestate and other types of organic fertilizers to the soil. The objective of this study was to evaluate the influence of different types of animal waste–based digestate application on soil prokaryotic diversity and composition in an agricultural cropping system over a period of 3 years, cultivated with three different annual cereal crops (spring wheat, triticale, and barley). Treatments were laid out in a randomized design with five conditions (three replicates per condition): fertilizer treatments included three different types of digestate (pig manure, chicken manure, and cow manure digestates), synthetic mineral nitrogen, and unfertilized control. Prokaryotic soil communities were characterized by Illumina MiSeq sequencing. The three most abundant phyla identified were Actinobacteria, Acidobacteria, and Proteobacteria, which accounted for over 55% of the total prokaryotic community. Other phylogenetic groups such as Verrucomicrobia and Bacteroidetes were also identified as part of the native soil microbiota. It was observed that the period of digestate application did not significantly influence the prokaryotic diversity in the soil. On the contrary, sampling time was a major factor in driving β-diversity. A correlation with soil pH was also observed for several taxonomic groups, indicating its importance in shaping prokaryotic community composition. Our study showed that the richness and diversity of the soil prokaryotic community were not affected by digestate application, while other factors such as the yearly crop varieties and seasonal/climate changes were the major contributors to differentiating the prokaryotic community composition over time.
The consumption of animal-derived products is constantly increasing (Salter, 2017), and in the future years it is expected to rise; therefore, it is extremely important to develop sustainable systems for animal-waste product management. Biogas systems produce clean energy using organic waste such as animal byproducts and discarded food which are converted into methane and carbon dioxide (Aydin, 2017). Digestates are the end-products generated from the anerobic digestion of these organic substrates (Crolla et al., 2013; Nkoa, 2014). Digestates could be further used as fertilizer because of their high content of nutrients such as nitrogen (N), potassium (K), phosphorus (P), and organic matter, being a sustainable alternative to reduce the utilization of inorganic fertilizers (Bachmann et al., 2014; Lee et al., 2021). Moreover, digestates may contain beneficial bacteria as nitrogen fixers and phosphate solubilizers (Fernandez-Bayo et al., 2020; Raymond et al., 2020), conferring an additional value as biofertilizers (Crolla et al., 2013; Insam et al., 2015). The bacterial composition of the digestate is different with respect to the microbiota present in the primary feedstocks owing to anerobic treatments they have undergone (Fernandez-Bayo et al., 2020). This will also affect the persistence in the soil of the bacteria present in the digestate which is lower in comparison to other organic fertilizers such as woodchip compost (Akari and Uchida, 2021; Dincă et al., 2022). Organic fertilization contributes to increasing nutrient availability to plants (Chu et al., 2007), enhances soil microorganism activity (Makdi et al., 2012; Nkoa, 2014), and in turn improves crop yield (Šimon et al., 2015). Soil type and the kind of organic material applied are two critical factors influencing soil microbial activities such as respiration rate and soil microbial biomass (Li et al., 2018; Chen et al., 2019). Specifically, several studies indicated increased microbial biomass due to digestate application (Fuchs et al., 2008; Alburquerque et al., 2012; Nkoa, 2014). In terms of the time frame of continuous digestate application, there have been varying reports on the influence of digestate/organic fertilization on soil microbes from short- to long-term experiments based on the primary feedstocks and the mode of experiments (Luo et al., 2015; Möller, 2015; Nielsen et al., 2020). For instance, Möller (2015) reported that digestate with a high degradability of organic matter such as clover-grass has a stronger effect on the short-term soil microbial activity. In addition, Nielsen et al. (2020) reported in a previous review that higher effects of digestate application on some soil microbial activity define parameters such as metabolic content and basal respiration than those of their individual feedstock in the short-term involving specific experimental setup. In contrast, Luo et al. (2015) reported a shift in microbial community structure in a long-term study involving 33 years of fertilization, which was also affirmed in other studies with a different long-term period (Ruppel and Makswitat, 1999; Chu et al., 2007; Guo et al., 2019). Also, climatic fluctuations over a cultivation year result in soil bacterial communities being constantly exposed to changes and adaptations to environmental conditions such as moisture, resource availability, and temperature (Bardgett and Caruso, 2020).
The bacterial community may then be significantly altered in response to the individual components of the added waste and with respect to time. Therefore, it is important to understand the major factors in shaping the soil prokaryotic community to pave the way for improving soil quality and carrying out proper fertilization using alternative byproducts such as digestate (Peacock et al., 2001).
In previous studies, we analyzed the effects of three different types of digestates (from pig, chicken, and cow manure) on soil features and plant yield. Repeated digestate applications over 3 years of treatment lead to a slight decrease in nitrogen and carbon soil content, while a considerable increase was observed for potassium (K2O), in particular for soils treated with cow and chicken manure digestates. Another difference was related to P content which increased in all treatments (including unfertilized plots and plots fertilized with synthetic nitrogen), with the exception of the plots treated with chicken manure digestates. There were no differences observed in relation to soil pH (Doyeni et al., 2021b).
In terms of plant quality and productivity, the effects of fertilization differed depending on the tested crop. Higher grain density was observed for spring wheat and spring barley treated with digestates or synthetic nitrogen fertilizers with respect to the control. The grain protein percentage was generally higher in all the fertilized plants comparable values were observed for pig manure digestate and synthetic fertilizer in spring wheat; in triticale, synthetic fertilizer outperformed with respect to digestates, while in spring barley, chicken and cow manure digestates gave better results (Doyeni et al., 2021b). Moreover, in pot experiments, where a similar soil was used, a general increase of the soil microbial biomass with all the three types of digestates was observed (Doyeni et al., 2021a). The aim of this study was to evaluate the composite effect of fertilization with these different sources of animal waste–based digestate and seasonal/annual variation on the soil prokaryotic community diversity and composition over 3 years.
The experimental field was located at the Lithuanian Research Centre for Agriculture and Forestry (55.40 N, 23.87 E), which is characterized by a humid continental climate (Belda et al., 2014). The soil in the experimental area is loamy (Endocalcaric Epigleyic Cambisol) (Baxter, 2007), and the soil chemical composition exhibited suitable parameters for cereal cultivation: pH (7.03), organic carbon content (1.3%), and nitrogen content (0.14%). For a complete characterization of physico-chemical properties, the details are shown in (Doyeni et al., 2021b). A complete randomized block design with five treatments was used to evaluate the effects of fertilization and time on soil microbiota. The complete randomized design was characterized by 15 plots (five fertilizer treatments × three replicates). Each treatment plot was 30 m2 (3 m × 10 m). Fertilization conditions were as follows: 1) no fertilizers (Control; C), 2) synthetic nitrogen fertilizer ([NH4NO3]; SN), and three different organic fertilizers obtained from anerobic digestion of animal manure, 3) pig manure digestate (PM), 4) chicken manure digestate (ChM), and 5) cow manure digestate (CoM); for a complete characterization of the digestates used, see (Doyeni et al., 2021b). The experiment was carried on for 3 years (1–3) from April 2018 to August 2020. The samples were collected each year before fertilization (BF; April-May) and after harvest (AH; August). At the beginning of the field experiment, soil samples were randomly collected from five different spots at a depth of 0–20 cm. The samples were thoroughly mixed to form a composite, and soil specimens were immediately stored at a temperature of -80 °C. The samples were named according to fertilization conditions and sampling time. Before the beginning of the experiment, the field was cultivated with winter wheat (Triticum aestivum)-cultivar “Skagen” (Nordic seed A/S, Denmark). In the first, second, and third year, plots were cultivated with spring wheat (Triticum aestivum) cultivar “Collada” (Einbeck, Germany), in the second year with spring triticale, a hybrid between wheat and rye [cultivar “Milkaro” (Koscian, Poland)], and in the third year, spring barley (Hordeum vulgare L.) cultivar “Ema DS” (Akademija, Lithuania). The sowing rate was 270 kg ha−1 (spring wheat), 250 kg ha−1 (spring triticale), and 220 kg ha−1 (spring barley). The seeds were sown on 19 April 2018, 16 April 2019, and 16 April 2020. The field was fertilized in all years of the 3-year experiment. In all years (year 1–3), samples before fertilization (start of the cultivation year) were subdivided into two groups: control (no fertilization) and control-treated (samples fertilized the precedent year), while samples after harvest (end of the cultivation year-month of August of each year) were further subdivided with respect to the fertilization treatment used. Analysis was conducted considering two different variables: 1) the fertilization treatment used, and 2) the sampling time, a composite parameter influenced by multiple factors.
Total DNA was extracted using the FastDNA spin kit for soil (MP Biomedicals, California, United States). Briefly, around 0.5 g of soil was weighed, homogenized by bead beating in the FastPrep®-24 instrument (MP Biomedical) at 6 m/s for 40 s, and DNA was purified with the aforementioned column-based kit according to the manufacturer’s instructions. Extracted DNA was checked by agarose gel electrophoresis. The DNA purity and quantity were measured using an ND-1000 Spectrophotometer (NanoDrop Technologies, Wilmington, United States) and standardized to a concentration of 10 ng μL−1.
For each sample, the V3–V4 region of the 16S rRNA gene was amplified using primers Pro341f and Pro805R (Takahashi et al., 2014), which allow the amplification of both Bacteria and Archaea domains, and barcodes were added to the forward primer. Amplicons for each library were purified and mixed in equal proportions. Illumina MiSeq v3 chemistry 300 base paired-end (PE) amplification and sequencing were performed at BMR genomics (Padova, Italy). Briefly, PCR reactions were prepared using 0.2 U of Platinum Taq DNA Polymerase HiFi (Thermofisher, Massachusetts, United States), 10 µM of each primer, 10 mM dNTPs mix, 1X buffer, 50 mM of MgSO4, and 50 ng of genomic DNA in a final volume of 25 µL. Amplification conditions were 94°C for 1 min, 25 cycles with 94°C for 30 s, 55°C for 30 s, and 68°C for 45 s, and a final elongation step at 68°C for 7 min. Two samples (C2AH1 and CoM1AH3) were excluded from further analysis due to sequencing failure. The primer sequences were removed using Cutadapt (Martin, 2011). Read quality was evaluated using DADA2 (Callahan et al., 2016), and reads (R1 and R2) were then trimmed and filtered using the following parameters: truncLen = c (265,220), maxN = 0, maxEE = c (2,2), and truncQ = 2. The reads were merged with an overlap of at least 12 bases, identical to each other in the overlap region. Chimeras were removed and amplicon sequence variants (ASVs) were classified against the Silva database v138 (Yilmaz et al., 2014), using the function assignTaxonomy in the DADA2 package, version 1.18.0 (Callahan et al., 2016) with “R” version 4.0.3 (R Core Team, 2020). ASVs matching with chloroplast and mitochondria sequences were removed from the ASV table.
The α-diversity measures (number of observed ASVs, Chao1 value, and Shannon index) were calculated using the vegan package, version 2.5–6 (Oksanen et al., 2020) in “R” version 4.0.3 (R Core Team, 2020). Pielou’s evenness index was calculated as J = H′/ln(S), where H′ is Shannon Weiner diversity and S is the total number of species (ASVs) (Pielou, 1966). A nonmetric multidimensional scaling (NMDS) and a permutational multivariate analysis of variance (PERMANOVA) based on Hellinger-transformed ASV abundance data were performed using the metaMDS and the adonis2 functions, respectively. Both the NMDS and the PERMANOVA were performed with the Bray–Curtis dissimilarity index. The taxa with different relative abundances between sampling times and treatments were identified by using a negative binomial mixed model (method = nb) using the function mms {y, fixed = ∼ Sampling Time + Treatment + offset [log(N)], random = ∼ 1 | plots, min. p = 0.2, method = “nb”}, where y is the matrix with the number of sequences for each taxonomic group (genus or phylum), sampling times and treatments were considered fixed variables, and plots as a random variable; only taxa with a proportion of nonzero values >0.2 (min p) were included in the analysis, and differences were considered significant for p < 0.05 (Zhang and Yi, 2020). Shapiro–Wilk and Levene tests were performed to check normality and homogeneity of variance, respectively, depending on results of the ANOVA or Kruskal–Wallis group test with false discovery rate (“fdr”) p-value adjustment followed by Tukey’s HSD or Dunn’s post hoc test, respectively, were used. All tests were conducted in “R” version 4.0.3 (R Core Team, 2020). Pearson’s correlations among different taxa (at phylum and genus level) and soil chemical features such as N, C, K2O, P2O5, pH, and humus (previously measured in Doyeni et al., 2021b) were calculated in “R” using the package Hmisc (R core Team, 2020); p-values were adjusted using the Benjamini–Hochberg false discovery rate procedure (Supplementary Datasheet S1).
Illumina MiSeq v3 sequencing was performed on the variable V3–V4 region of the 16S rDNA gene, producing a total of 17,989,800 sequences (ranging from 59,530 to 208,305 sequences per library). Rarefaction curves showed sequencing coverage for all samples (Supplementary Figure S2), allowing the identification of 12,730 amplicon sequence variants (ASVs), with a range from 1,024 to 3,137 ASVs per sample (Supplementary Figure S2).
The α-diversity was calculated for the number of ASVs observed, Chao1 value, Shannon diversity, and Pielou’s evenness indexes. We considered variations in soil prokaryotic relative abundance, namely: 1) treatment used and 2) sampling time. The sampling time was indicative as a composite parameter influenced by multiple variables: 1) different crops/varieties grown each year, 2) weathering conditions, and 3) the agricultural techniques used in each segment such as tillage and split fertilization. Species richness and Shannon diversity were not significantly different throughout the experiment in terms of treatment used or sampling time (Supplementary Figure S3). In contrast, a significant difference related to the species evenness (Pielou’s evenness index) was observed for sampling time (Figure 1). Higher evenness values were found for sampling times 1 and 2, while sampling time 3 showed the lowest value (Kruskal–Wallis and Dunn test, p < 0.05). No differences were detected with respect to the different fertilizing treatments used.
FIGURE 1. Pielou’s evenness index. On the X-axis are indicated sampling times, dots are differently colored with respect to treatments: control (before fertilization, no fertilization), control-treated (before fertilization, fertilized the precedent year), control after harvest (after harvest, no fertilization), mineral nitrogen (after harvest), chicken manure digestate (after harvest), cow manure digestate (after harvest), and pig manure digestate (after harvest).
Sampling time was also the major factor in driving the β-diversity as observed with the PCoA and PERMANOVA analysis (p < 0.01), with all the sampling times differing from each other (Figure 2B; Supplementary Table S1). In relation to the fertilization treatment, significant differences were observed between the control group (no fertilization, before fertilization) and all the groups “after harvest” (control after harvest, mineral nitrogen, chicken manure digestate, cow manure digestate, and pig manure digestate; PERMANOVA, p < 0.01), reflecting differences observed in relation to sampling time (Figure 2A). A significant difference was also found between the groups control and control-treated (PERMANOVA, p < 0.01, Supplementary Table S2).
FIGURE 2. Non-metric multidimensional scaling (nMDS) plot. nMDS plot based on the Bray–Curtis index. (A) nMDS plot with samples colored with respect to the treatment used: control (before fertilization, no fertilization), control-treated (before fertilization, fertilized the precedent year), control after harvest (after harvest, no fertilization), mineral nitrogen (after harvest), chicken manure digestate (after harvest), cow manure digestate (after harvest), and pig manure digestate (after harvest). (B) nMDS plot with samples colored with respect to sampling time.
99.81% of the ASVs were identified at least at the phylum level. ASVs were classified into 47 phyla, 113 classes, 256 orders, 324 families, and 591 genera. The overall prokaryotic community composition was similar in all the conditions tested (Figure 3A). The three most abundant phyla in all the treatments were Actinobacteria, Acidobacteria, and Proteobacteria, whose relative abundance was similar within samples: Actinobacteria (18.8 ± 1.9%), Proteobacteria (19.5 ± 1.5%), and Acidobacteria (19.8 ± 1.5%) (Figure 3). Together, these three phyla accounted for 55.7 to 60.9% of the total prokaryotic community. Other phyla whose relative abundance was relatively high (>5%) were Bacteroidetes (13.1 ± 1.6%), Verrucomicrobia (8 ± 0.9%), and Chloroflexi (6.4 ± 0.7%). The 10 most representative genera were the group 41 (2.04 ± 0.05%; Acidobacteria); Nitrospira (1.71 ± 0.02%; Nitrospira); Candidatus Udaeobacter, Candidatus Xiphinematobacter, and Chthoniobacter (1.61 ± 0.04%, 1.05 ± 0.02%, and 0.99 ± 0.02%, respectively; Verrucomicrobia); Gaiella, Pseudoarthrobacter, and Nocardiodes (1.55 ± 0.02%, 1.52 ± 0.05%, and 1.16 ± 0.02%, respectively; Actinobacteria); Sphingomonas (1.29 ± 0.05%; Proteobacteria), and Chryseolinea (1.03 ± 0.02%; Bacteroidetes). However, for many sequences, it was not possible identifying at the genus rank (54.7 ± 0.3% of the sequences); in particular within the phylum Acidobacteria, we observed the higher number of unassigned ASVs (16% of the total number of sequences), with only the 19.16% of the sequences falling in the phylum Acidobacteria assigned at the genus rank. Soil chemical features (nitrogen (N), carbon (C), potassium oxide (K2O), phosphorus pentoxide (P2O5), pH, and humus content were previously measured at two time points: the beginning (sampling time 0) and the end of the trial (sampling time 5) (Doyeni et al., 2021b). Correlation analyses were performed between the different taxonomic groups identified (at phylum and genus level) and soil composition. No significant correlation was observed at the phylum level, while at the genus rank, 75 groups (out of 463 analyzed) showed a significant correlation with pH (p-value adjusted <0.05); among them only two genera, Haliangium (Mixococcota) and group TM7a (Patescibacteria), showed a negative correlation. Most of the genera correlating with pH belonged to Proteobacteria (30 genera) and Firmicutes (15 genera). The five most represented groups showing a significant correlation with pH were Sphingomonas (Proteobacteria), Haliangium (Mixococcota), Massilia (Proteobacteria), Puia (Bacteroidetes), and Arenimonas (Proteobacteria) (Supplemental Dataset 1).
FIGURE 3. Soil prokaryotic composition. Soil prokaryotic community composition with respect to (A) the treatment used or (B) sampling time. The fifteen major groups are reported at the phylum taxonomic level. Other phyla are collapsed within the group “other”.
The PERMANOVA analysis at the phylum rank showed significant differences for both variants analyzed, fertilizing treatments, and sampling time (p < 0.001). For sampling time, differences were detected for all the six groups considered. Regarding fertilizing treatments, there were significant differences between control groups before fertilization (control and control-treated) and groups after fertilization, reflecting differences observed in relation to sampling time. The group control-treated showed no significant difference between cow manure digestate and chicken manure digestate groups.
Considering groups sampled after fertilization, significant differences (at phylum rank) were found between the control group and fertilized groups, while within fertilized groups were observed differences only among plots fertilized with mineral nitrogen and pig manure digestate (PERMANOVA, p < 0.05). A negative binomial mixed model was applied to infer differences related to sampling time and/or treatment used (Figure 4). Most of the differences (12 phyla) were related to sampling time (Figure 4), while only three phyla were differently abundant in relation to the treatment used: Firmicutes, Patescibacteria, and Dependentiae (Figures 4, 5), which accounted for the 2.18, 0.3, and 0.04% of the soil community, respectively. Significant differences in Firmicutes relative abundance were observed during the first year (sampling time 1) among control plots and plots treated with cow or chicken manure digestates (Figure 5A). A higher amount of sequences belonging to the phylum Patescibacteria was detected in plots treated with pig manure digestate in the second year (Figure 5B), while in the third year, there was a significant difference for Dependentiae phylum between the control group and plots treated with mineral nitrogen (Figure 5C).
FIGURE 4. Heatmap for the effects of sampling time and fertilization treatments at the phylum level. Differences in p-values at the phylum taxonomic rank for sampling time or treatment used in samples collected after harvest are indicated in gray (no differences) or different shades of blue (p-value ranging from 0.05 to 0).
FIGURE 5. Effect of the different fertilizers used at the phylum level after harvest. Each bar is labeled respective to the treatment applied and colored respective to the year of treatment: black (2018, first year), light gray (2019, second year), and dark gray (2020, third year). (A) Firmicutes, (B) Patescibacteria, and (C) Dependentiae. Means sharing the same letter within the same year are not significantly different (post hoc Tukey’s HSD test or Dunn’s test).
Differences at the genus rank reflected what was observed at the phylum rank with most of the differences (113 genera) associated with sampling time and only a few genera (18) varying in relation to the different treatments used (Supplementary Figure S5). The 18 genera belong to eight different phyla, with six genera affiliated with Proteobacteria, five to Actinobacteria, and two to Firmicutes. We also analyzed the relative abundance of these groups in the three different years and for seven of them, we found significant differences (p < 0.05). In the first year, we observed significant differences for the genera Streptomyces, Paenisporosarcina, the subgroup 10 of the phylum Acidobacteria and Opitutus, and the group TM7a of the phylum Patescibacteria (Figure 6; Kruskal–Wallis or ANOVA, p < 0.05). The subgroup 10 relative abundance was higher in the control group with respect to the plots treated with mineral nitrogen and cow manure digestate. For the other genera, we observed a lower amount in the control group with respect to one or more fertilized groups, in particular Streptomyces relative abundance was higher in all the manure digestate groups (Figure 6B). For the second year, only Acinetobacter showed significant differences with a higher presence in plots treated with cow manure digestate with respect to control and chicken manure digestate groups (Figure 6D; Kruskal–Wallis, p < 0.05). In the third year, two genera, Gaiella and Paenisporosarcina, were characterized by higher levels of pig and cow manure digestate (respectively) versus the control group (Figures 6A,C; ANOVA, p < 0.05). Paenisporosarcina was the only genus showing a marked increase in treated groups in two different years (first and third, Figure 6C).
FIGURE 6. Effect of the different fertilizers used at the genus level after harvest. Each bar is labeled respective to the treatment applied and colored respective to the year of treatment: black (2018, first year), light gray (2019, second year), and dark gray (2020, third year). (A) Gaiella, (B) Streptomyces, (C) Paenisporosarcina, (D) Acinetobacter, (E) Acidobacteria subgroup 10, (F) Opitutus, and (G) TM7a (Patescibacteria). Means sharing the same letter within the same year are not significantly different (post hoc Tukey’s HSD test or Dunn’s test).
The overall community composition was quite similar for all the treatments used, and the major phyla detected at the initial sampling time in the year 2018 were well-known soil dominant phyla (Proteobacteria, Acidobacteria, and Actinobacteria) which are commonly found in this type of soil, and they kept their predominance throughout the duration of the project (Mhete et al., 2020; Wu et al., 2020; Li et al., 2021). These phyla accounted for over 55% of the soil’s prokaryotic composition. Proteobacteria is one the most diverse and abundant phyla present in the soil; within this phylum, many different microbes can thrive and adapt to different soil conditions and influence plant growth either as plant growth–promoting rhizobacteria or as pathogens (Spain et al., 2009). Actinobacteria are typically dominant soil microbes partaking in the biogeochemical cycling of carbon, nitrogen, phosphorus, potassium, and several other elements in the soil. Furthermore, within Actinobacteria, there are aerobic saprophytes capable of producing extracellular hydrolytic enzymes that can degrade complex compounds (Ranjani et al., 2016). Actinobacteria presence helps in sustainably improving soil health and providing an effective pathway for nutrient cycling (Bhatti et al., 2017). Acidobacteria is considered one of the most abundant soil phyla with their relative abundances ranging from ca. 20–40% in temperate soils such as forests, grasslands, and pasture soils (Janssen, 2006). Here, we observed a relative abundance ranging from 16 to 23%, the relatively low amount of Acidobacteria found could be possibly linked to the pH (7.3) of the receiving soil used as most Acidobacteria prefer lower pH (3.0–6.5) (Kalam et al., 2020). The addition of manure digestates did not affect soil pH (Doyeni et el., 2021b) and Acidobacteria relative abundance was found similar in the 3-years analysis with no difference with respect to the treatments used. Indeed, in other similar studies (Xu et al., 2016; Zhang et al., 2021), the application of the digestate does not have negative effects on their availability. However, the correlation analysis showed that several genera (75) were affected by soil pH as a slight decrease (unrelated to soil treatments) was observed between the beginning and the end of the trial (from 7.03 to ∼6.5) (Doyeni et el., 2021b). Noticeably, the decrease in pH we observed could be linked to seasonality (Wolińska et al., 2022). We did not find significant correlations between Acidobacteria and pH at phylum or genus ranks; however, this could be biased as it was not possible to assign most of the sequences falling in the Acidobacteria phylum at low taxonomic ranks (i.e., genus). Soil pH is a major driver of bacterial selection and abundance as for bacteria the pH range for optimal growth is quite narrow (Rousk et al., 2010; Tian et al., 2021). Most of the taxa influenced by acidification belonged to the Proteobacteria and Firmicutes phyla and showed a positive correlation with pH. The most represented genera showing a positive correlation with pH were Puia (Bacteroidetes) and three proteobacterial taxa (Sphingomonas, Massilia, and Arenimonas) which decreased when compared with the first year (before fertilization) and the third year (after fertilization). Massilia genus has been found to colonize root surfaces and is relatively abundant in the rhizosphere (Ofek et al., 2012; Wolińska et al., 2022). In contrast, Haliangium (Mixococcota) relative abundance increased with a lower pH, and members of this genus are commonly found in soil [e.g., in the rhizosphere of melon plants (Ling et al., 2014)] and may have different effects on soil microbiota: it has been observed that they are capable of predating on Gram-positive bacteria (Zhang and Lueders, 2017) and also have the potential to inhibit the growth of a wide spectrum of fungi (Fudou et al., 2001; Ling et al., 2014). Variations in Massilia and Haliangium relative abundance have been previously observed in relation to pH and seasonality in a trial using an intercrop mixture and a maize monoculture (Wolińska et al., 2022).
Soils are complex environments, and perturbations of their homeostasis may alter the microbial community composition. Therefore, digestate application may enrich soil phyla already present in soil and/or influence their relative abundance. Previous analysis showed a beneficial effect of digestate application on plant growth (Doyeni et al., 2021b), which was possibly due to an increase in microbial biomass (Doyeni et al., 2021a); however, the lack of direct measurement of these samples could not directly confirm this hypothesis. Moreover, it was not clear if this was also due to alterations in the prokaryotic community and/or to the presence of novel microorganisms present in the digestates. The samples were then collected at two different time points, a medium one few months after fertilization (3–4 months after digestate application, after harvest) and a long term before the fertilization of the following year. However, no significant differences were observed between the treatments. In contrast, the PERMANOVA analysis showed that most of the differences observed were related to sampling time, indicating that multiple factors related to this parameter had a major influencing role on soil bacterial community composition. Indeed, this parameter was associated to the period of sensitivity in weathering seasonal changes, cultivation years, and agricultural practices. The applied agricultural techniques such as annual tillage before the start of the cultivation season (before fertilization) and the harvesting activity (after harvest) could have impacted significant changes in microbial composition (Longepierre et al., 2021). Also, environmental factors are known to play a fundamental role in shaping microbial composition and diversity (Zhang et al., 2019).
Considering only the effects of a few months after fertilization (after harvest), the overall prokaryotic composition was similar for all treatments, with few differences observed at phylum and genus levels. For instance, for samples collected in the first year (after harvest), Firmicutes relative abundance was different in control with respect to the other treatments, but no differences were observed between the digestates and mineral nitrogen fertilizers, indicating an effect of fertilization on this group. Furthermore, Patescibacteria and Dependetiae are enriched in mineral nitrogen–treated soil in comparison to the control in the second and third years, respectively. Patescibacteria are ultra-small bacteria mostly uncultivated with reduced genomes and often found in groundwater environments (Tian et al., 2020); however, their presence has also been observed in endophytic communities (Wemheuer et al., 2019). Similarly, the phylum Dependentiae (formerly known as TM6) is a group of microorganisms widespread in different environments (mats, sediments, sulfur springs, and sinks) whose current knowledge comes from metagenomic data only (Yeoh et al., 2016). The comparative genomic analysis showed parasitism as a common feature within this group (Yeoh et al., 2016), suggesting that it could potentially affect plant growth; however, its presence was significantly higher in mineral nitrogen–treated plots only.
Regarding the differences among treatments at the genus level, similar trends (differences among control samples and mineral nitrogen and/or samples of plots treated with digestates) were noticeable. Taxa belonging to the genera Gaiella, Streptomyces, Acinetobacter, Opitutus, Acidobacteria (subgroup 10), and Streptomycetes showed differences among treatments in 1 year only, while Paenisporosarcina showed significant differences in the first and third years. The Paenisporosarcina genus has been characterized by mostly psychrophilic species (Reddy et al., 2013); however, it has been found also in soils where it may have a beneficial effect on plant growth (i.e., rice), inhibiting potential pathogens such as Rhizoctonia solani owing to VOC production (Wang et al., 2021). Also, members of the Gaiellales have been found associated to cereals in the root system of rice (Hernández et al., 2015). Microbes belonging to the genus Streptomycetes are often detected inside plant roots and can be beneficial (Olanrewaju and Babalola, 2019) while sometimes can act as plant pathogens (Seipke et al., 2012); however, the crop yield and quality of crops were not compromised in the period of digestate application (Doyeni et al., 2021b).
The identity of the host plant has a significant influence on the identity of its microbiome (Dastogeer et al., 2020), and promoting a soil microbiome for high plant production requires management of microbial abundance and activity, community composition, and specific functions (Lehmann et al., 2020). In essence, the different cereal-based crop plants cultivated in the 3 years may have played key roles in the prokaryotic relative abundance as each plant has unique requirements in terms of needs, uptake, and competitiveness with the soil microbes. These factors together with other environmental/agricultural factors were then the major drivers in influencing microbiota composition rather than digestate application.
All the three types of digestate tested gave similar results with the native prokaryotic community composition not significantly affected in a medium/long term response over the 3 years of application. The major effect on community composition was due to the sampling time possibly related to the changing environmental conditions and other agricultural management techniques factors such as the tillage before each year’s cultivation, harvesting during summer, and different cereal crops grown each year. A pH decrease was observed between the beginning and the end of the trial, and this was unrelated to the treatment used and probably linked with seasonality. However, soil pH probably played a major role in microbiota relative abundance (positively) correlating with many taxa at genus rank.
Digestate application showed then a positive effect as the short- to long-term aim was to prevent the introduction/increase of potential pathogens in the soil and avoid a perturbation of the native soil prokaryotic community.
The 16S rRNA gene amplicon sequence data are available at the National Centre for Biotechnology Information Sequence Read Archive (SRA; http://www.ncbi.nlm.nih.gov/sra) with SRA accession from SAMN24344854 to SAMN24344941.
SS and VT conceived the experiment. MD and SS performed soil sampling and soil DNA extraction. MD, CV and FP analyzed data. All authors contributed to data interpretation, drafted the manuscript, agreed with its final version, and revised the manuscript.
This research was funded by the Research Council of Lithuania (LMTLT), agreement No. S-SIT-20-5. and the APC was funded by the Research Council of Lithuania (LMTLT), agreement No. S-SIT-20-5.
The authors declare that the research was conducted in the absence of any commercial or financial relationships that could be construed as a potential conflict of interest.
All claims expressed in this article are solely those of the authors and do not necessarily represent those of their affiliated organizations, or those of the publisher, the editors, and the reviewers. Any product that may be evaluated in this article, or claim that may be made by its manufacturer, is not guaranteed or endorsed by the publisher.
The authors wish to thank Ausra Baksinskaite, Urte Stulpinaite, and the field team of the Plant Nutrition and Agroecology department (Lithuanian Research Centre for Agriculture and Forestry) for their technical support.
The Supplementary Material for this article can be found online at: https://www.frontiersin.org/articles/10.3389/fenvs.2022.852241/full#supplementary-material
Supplementary Table S1 | P-values of the PERMANOVA test show differences among sampling times.
Supplementary Table S2 | P-values of the PERMANOVA test show differences among treatments.
Akari, M., and Uchida, Y. (2021). Survival Rates of Microbial Communities from Livestock Waste to Soils: A Comparison between Compost and Digestate. Appl. Environ. Soil Sci., 1–15. doi:10.1155/2021/6645203
Alburquerque, J. A., de la Fuente, C., Campoy, M., Carrasco, L., Nájera, I., Baixauli, C., et al. (2012). Agricultural Use of Digestate for Horticultural Crop Production and Improvement of Soil Properties. Eur. J. Agron. 43, 119–128. doi:10.1016/j.eja.2012.06.001
Aydin, S. (2017). “Anaerobic Digestion,” in Waste Biomass Management - A Holistic Approach, 1–14. doi:10.1007/978-3-319-49595-8_1
Bachmann, S., Gropp, M., and Eichler-Löbermann, B. (2014). Phosphorus Availability and Soil Microbial Activity in a 3 year Field Experiment Amended with Digested Dairy Slurry. Biomass Bioenergy 70, 429–439. doi:10.1016/j.biombioe.2014.08.004
Bardgett, R. D., and Caruso, T. (2020). Soil Microbial Community Responses to Climate Extremes: Resistance, Resilience and Transitions to Alternative States. Phil. Trans. R. Soc. B 375, 20190112. doi:10.1098/rstb.2019.0112
Baxter, S. (2007). World Reference Base for Soil Resources. World Soil Resources Report 103. Rome: Food and Agriculture Organization of the United Nations (2006), Pp. 132, US$22.00 (Paperback). ISBN 92-5-10511-4. Exp. Agric. 43, 264. doi:10.1017/s0014479706394902
Belda, M., Holtanová, E., Halenka, T., and Kalvová, J. (2014). Climate Classification Revisited: from Köppen to Trewartha. Clim. Res. 59, 1–13. doi:10.3354/CR01204
Bhatti, A. A., Haq, S., and Bhat, R. A. (2017). Actinomycetes Benefaction Role in Soil and Plant Health. Microb. Pathog. 111, 458–467. doi:10.1016/j.micpath.2017.09.036
Callahan, B. J., McMurdie, P. J., Rosen, M. J., Han, A. W., Johnson, A. J. A., and Holmes, S. P. (2016). DADA2: High-Resolution Sample Inference from Illumina Amplicon Data. Nat. Methods 13, 581–583. doi:10.1038/nmeth.3869
Chen, X. D., Dunfield, K. E., Fraser, T. D., Wakelin, S. A., Richardson, A. E., and Condron, L. M. (2020). Soil Biodiversity and Biogeochemical Function in Managed Ecosystems. Soil Res. 58, 1–20. doi:10.1071/SR19067
Chu, H., Lin, X., Fujii, T., Morimoto, S., Yagi, K., Hu, J., et al. (2007). Soil Microbial Biomass, Dehydrogenase Activity, Bacterial Community Structure in Response to Long-Term Fertilizer Management. Soil Biol. Biochem. 39, 2971–2976. doi:10.1016/j.soilbio.2007.05.031
Crolla, A., Kinsley, C., and Pattey, E. (2013). “Land Application of Digestate,” in The Biogas Handbook (Elsevier), 302–325. doi:10.1533/9780857097415.2.302
Dastogeer, K. M. G., Tumpa, F. H., Sultana, A., Akter, M. A., and Chakraborty, A. (2020). Plant Microbiome-An Account of the Factors that Shape Community Composition and Diversity. Curr. Plant Biol. 23, 100161. doi:10.1016/j.cpb.2020.100161
Dincă, L. C., Grenni, P., Onet, C., and Onet, A. (2022). Fertilization and Soil Microbial Community: A Review. Appl. Sci. 12, 1198. doi:10.3390/app12031198
Doyeni, M. O., Baksinskaite, A., Suproniene, S., and Tilvikiene, V. (2021a). Effect of Animal Waste Based Digestate Fertilization on Soil Microbial Activities, Greenhouse Gas Emissions and Spring Wheat Productivity in Loam and Sandy Loam Soil. Agronomy 11, 1281. doi:10.3390/agronomy11071281
Doyeni, M. O., Stulpinaite, U., Baksinskaite, A., Suproniene, S., and Tilvikiene, V. (2021b). The Effectiveness of Digestate Use for Fertilization in an Agricultural Cropping System. Plants 10, 1734. doi:10.3390/plants10081734
Fernandez-Bayo, J. D., Simmons, C. W., and Vandergheynst, J. S. (2020). Characterization of Digestate Microbial Community Structure Following Thermophilic Anaerobic Digestion with Varying Levels of Green and Food Wastes. J. Industrial Microbiol. Biotechnol. 47, 1031–1044. doi:10.1007/s10295-020-02326-z
Fuchs, J. G., Berner, A., Mayer, J., Smidt, E., and Schleiss, K. (2008). “Influence of Compost and Digestates on Plant Growth and Health: Potentials and Limits,” in Science, 101–110. Archived at: http://orgprints.org/17977/.
Fudou, R., Iizuka, T., Sato, S., Ando, T., Shimba, N., and Yamanaka, S. (2001). Haliangicin, a Novel Antifungal Metabolite Produced by a Marine Myxobacterium. 2. Isolation and Structural Elucidation. J. Antibiot. 54 (2), 153–156. doi:10.7164/antibiotics.54.153
Guo, Z., Han, J., Li, J., Xu, Y., and Wang, X. (2019). Effects of Long-Term Fertilization on Soil Organic Carbon Mineralization and Microbial Community Structure. PLoS ONE 14, e0211163. doi:10.1371/JOURNAL.PONE.0211163
Hernández, M., Dumont, M. G., Yuan, Q., and Conrad, R. (2015). Different Bacterial Populations Associated with the Roots and Rhizosphere of Rice Incorporate Plant-Derived Carbon. Appl. Environ. Microbiol. 81, 2244–2253. doi:10.1128/AEM.03209-14
Insam, H., Gómez-Brandón, M., and Ascher, J. (2015). Manure-based Biogas Fermentation Residues - Friend or Foe of Soil Fertility? Soil Biol. Biochem. 84, 1–14. doi:10.1016/J.SOILBIO.2015.02.006
Janssen, P. H. (2006). Identifying the Dominant Soil Bacterial Taxa in Libraries of 16S rRNA and 16S rRNA Genes. Appl. Environ. Microbiol. 72, 1719–1728. doi:10.1128/AEM.72.3.1719-1728.2006
Kalam, S., Basu, A., Ahmad, I., Sayyed, R. Z., El-Enshasy, H. A., Dailin, D. J., et al. (2020). Recent Understanding of Soil Acidobacteria and Their Ecological Significance: A Critical Review. Front. Microbiol. 11, 580024. doi:10.3389/fmicb.2020.580024
Lee, J. T. E., Ok, Y. S., Song, S., Dissanayake, P. D., Tian, H., Tio, Z. K., et al. (2021). Biochar Utilisation in the Anaerobic Digestion of Food Waste for the Creation of a Circular Economy via Biogas Upgrading and Digestate Treatment. Bioresour. Technol. 333, 125190. doi:10.1016/j.biortech.2021.125190
Lehmann, J., Bossio, D. A., Kögel-Knabner, I., and Rillig, M. C. (2020). The Concept and Future Prospects of Soil Health. Nat. Rev. Earth Environ. 1, 544–553. doi:10.1038/s43017-020-0080-8
Li, L., Xu, M., Eyakub Ali, M., Zhang, W., Duan, Y., and Li, D. (2018). Factors Affecting Soil Microbial Biomass and Functional Diversity with the Application of Organic Amendments in Three Contrasting Cropland Soils during a Field Experiment. PLoS ONE 13, e0203812. doi:10.1371/journal.pone.0203812
Li, J., Wen, Y., and Yang, X. (2021). Understanding the Responses of Soil Bacterial Communities to Long-Term Fertilization Regimes Using DNA and RNA Sequencing. Agronomy 11, 2425. doi:10.3390/agronomy11122425
Ling, N., Deng, K., Song, Y., Wu, Y., Zhao, J., Raza, W., et al. (2014). Variation of Rhizosphere Bacterial Community in Watermelon Continuous Mono-Cropping Soil by Long-Term Application of a Novel Bioorganic Fertilizer. Microbiol. Res. 169, 570–578. doi:10.1016/J.MICRES.2013.10.004
Longepierre, M., Widmer, F., Keller, T., Weisskopf, P., Colombi, T., Six, J., et al. (2021). Limited Resilience of the Soil Microbiome to Mechanical Compaction within Four Growing Seasons of Agricultural Management. Isme Commun. 1, 44. doi:10.1038/s43705-021-00046-8
Luo, P., Han, X., Wang, Y., Han, M., Shi, H., Liu, N., et al. (2015). Influence of Long-Term Fertilization on Soil Microbial Biomass, Dehydrogenase Activity, and Bacterial and Fungal Community Structure in a Brown Soil of Northeast China. Ann. Microbiol. 65, 533–542. doi:10.1007/s13213-014-0889-9
Makdi, M., Tomcsik, A., and Orosz, V. (2012). “Digestate: A New Nutrient Source - Review,” in Biogas. doi:10.5772/31355
Martin, M. (2011). Cutadapt Removes Adapter Sequences from High-Throughput Sequencing Reads. EMBnet J. 17, 10. doi:10.14806/ej.17.1.200
Mhete, M., Eze, P. N., Rahube, T. O., and Akinyemi, F. O. (2020). Soil Properties Influence Bacterial Abundance and Diversity under Different Land-Use Regimes in Semi-arid Environments. Sci. Afr. 7, e00246. doi:10.1016/j.sciaf.2019.e00246
Möller, K. (2015). Effects of Anaerobic Digestion on Soil Carbon and Nitrogen Turnover, N Emissions, and Soil Biological Activity. A Review. Agron. Sustain. Dev. 35, 1021–1041. doi:10.1007/s13593-015-0284-3
Nielsen, K., Roß, C.-L., Hoffmann, M., Muskolus, A., Ellmer, F., and Kautz, T. (2020). The Chemical Composition of Biogas Digestates Determines Their Effect on Soil Microbial Activity. Agriculture 10, 244. doi:10.3390/agriculture10060244
Nkoa, R. (2014). Agricultural Benefits and Environmental Risks of Soil Fertilization with Anaerobic Digestates: a Review. Agron. Sustain. Dev. 34, 473–492. doi:10.1007/s13593-013-0196-z
Ofek, M., Hadar, Y., and Minz, D. (2012). Ecology of Root Colonizing Massilia (Oxalobacteraceae). PLoS ONE 7, e40117. doi:10.1371/journal.pone.0040117
Oksanen, J., Blanchet, F. G., Friendly, M., Kindt, R., Legendre, P., McGlinn, D., et al. (2020). Community Ecology Package.
Olanrewaju, O. S., and Babalola, O. O. (2019). Streptomyces: Implications and Interactions in Plant Growth Promotion. Appl. Microbiol. Biotechnol. 103, 1179–1188. doi:10.1007/s00253-018-09577-y
Peacock, A. D., Mullen, M. D., Ringelberg, D. B., Tyler, D. D., Hedrick, D. B., Gale, P. M., et al. (2001). Soil Microbial Community Responses to Dairy Manure or Ammonium Nitrate Applications. Soil Biol. Biochem. 33, 1011–1019. doi:10.1016/S0038-0717(01)00004-9
Pielou, E. C. (1966). The Measurement of Diversity in Different Types of Biological Collections. J. Theor. Biol. 13, 131–144. doi:10.1016/0022-5193(66)90013-0
R Core Team (2020). R: A Language and Environment for Statistical Computing. Vienna, Austria: R Foundation for Statistical Computing. https://www.R-project.org/.
Ranjani, A., Dhanasekaran, D., and Gopinath, P. M. (2016). “An Introduction to Actinobacteria,” in Actinobacteria - Basics and Biotechnological Applications. doi:10.5772/62329
Raymond, F. C., Buraimoh, O. M., Akerele, O. S., Ilori, M. O., and Ogundipe, O. T. (2020). Digestate as Biofertilizer for the Growth of Selected Vegetables and Illumina Analysis of Associated Bacterial Community. bioRxiv [Preprint]. doi:10.1101/2020.12.03.393058
Reddy, G. S. N., Manasa, B. P., Singh, S. K., and Shivaji, S. (2013). Paenisporosarcina indica sp. nov., a psychrophilic bacterium from a glacier, and reclassification of Sporosarcina antarctica Yu et al., 2008 as Paenisporosarcina antarctica comb. nov. and emended description of the genus Paenisporosarcina. Int. J. Syst. Evol. Microbiol. 63, 2927–2933. doi:10.1099/ijs.0.047514-0
Rousk, J., Bååth, E., Brookes, P. C., Lauber, C. L., Lozupone, C., Caporaso, J. G., et al. (2010). Soil Bacterial and Fungal Communities across a pH Gradient in an Arable Soil. Isme J. 4, 1340–1351. doi:10.1038/ismej.2010.58
Ruppel, S., and Makswitat, E. (1999). Effect of Nitrogen Fertilization and Irrigation on Soil Microbial Activities and Population Dynamics - A Field Study. J. Plant Nutr. Soil Sci. 162, 75–81. doi:10.1002/(sici)1522-2624(199901)162:1<75::aid-jpln75>3.0.co;2-d
Salter, A. M. (2017). Improving the Sustainability of Global Meat and Milk Production. Proc. Nutr. Soc. 76, 22–27. doi:10.1017/S0029665116000276
Seipke, R. F., Kaltenpoth, M., and Hutchings, M. I. (2012). Streptomycesas Symbionts: an Emerging and Widespread Theme? FEMS Microbiol. Rev. 36, 862–876. doi:10.1111/j.1574-6976.2011.00313.x
Šimon, T., Kunzová, E., and Friedlová, M. (2016). The Effect of Digestate, Cattle Slurry and Mineral Fertilization on the Winter Wheat Yield and Soil Quality Parameters. Plant Soil Environ. 61, 522–527. doi:10.17221/530/2015-PSE
Spain, A. M., Krumholz, L. R., and Elshahed, M. S. (2009). Abundance, Composition, Diversity and Novelty of Soil Proteobacteria. Isme J. 3, 992–1000. doi:10.1038/ismej.2009.43
Takahashi, S., Tomita, J., Nishioka, K., Hisada, T., and Nishijima, M. (2014). Development of a Prokaryotic Universal Primer for Simultaneous Analysis of Bacteria and Archaea Using Next-Generation Sequencing. PloS One 9, e105592. doi:10.1371/journal.pone.0105592
Tian, R., Ning, D., He, Z., Zhang, P., Spencer, S. J., Gao, S., et al. (2020). Small and Mighty: Adaptation of Superphylum Patescibacteria to Groundwater Environment Drives Their Genome Simplicity. Microbiome 8, 1–15. doi:10.1186/s40168-020-00825-w
Tian, Q., Jiang, Y., Tang, Y., Wu, Y., Tang, Z., and Liu, F. (2021). Soil pH and Organic Carbon Properties Drive Soil Bacterial Communities in Surface and Deep Layers along an Elevational Gradient. Front. Microbiol. 12, 1–15. doi:10.3389/fmicb.2021.646124
Wang, E., Liu, X., Si, Z., Li, X., Bi, J., Dong, W., et al. (2021). Volatile Organic Compounds from Rice Rhizosphere Bacteria Inhibit Growth of the Pathogen Rhizoctonia Solani. Agriculture 11, 368. doi:10.3390/agriculture11040368
Wemheuer, F., Wemheuer, B., Daniel, R., and Vidal, S. (2019). Deciphering Bacterial and Fungal Endophyte Communities in Leaves of Two Maple Trees with Green Islands. Sci. Rep. 9, 1–14. doi:10.1038/s41598-019-50540-2
Wolińska, A., Kruczyńska, A., Podlewski, J., Słomczewski, A., Grządziel, J., Gałązka, A., et al. (2022). Does the Use of an Intercropping Mixture Really Improve the Biology of Monocultural Soils?-A Search for Bacterial Indicators of Sensitivity and Resistance to Long-Term Maize Monoculture. Agronomy 12, 613. doi:10.3390/agronomy12030613
Wu, S.-J., Deng, J.-J., Yin, Y., Qin, S.-J., Zhu, W.-X., Zhou, Y.-B., et al. (2020). Bacterial Community Changes Associated with Land Use Type in the Forest Montane Region of Northeast China. Forests 11, 40–19. doi:10.3390/f11010040
Xu, N., Tan, G., Wang, H., and Gai, X. (2016). Effect of Biochar Additions to Soil on Nitrogen Leaching, Microbial Biomass and Bacterial Community Structure. Eur. J. Soil Biol. 74, 1–8. doi:10.1016/j.ejsobi.2016.02.004
Yeoh, Y. K., Sekiguchi, Y., Parks, D. H., and Hugenholtz, P. (2016). Comparative Genomics of Candidate Phylum Tm6 Suggests that Parasitism Is Widespread and Ancestral in This Lineage. Mol. Biol. Evol. 33, 915–927. doi:10.1093/molbev/msv281
Yilmaz, P., Parfrey, L. W., Yarza, P., Gerken, J., Pruesse, E., Quast, C., et al. (2014). The SILVA and "All-Species Living Tree Project (LTP)" Taxonomic Frameworks. Nucl. Acids Res. 42, D643–D648. doi:10.1093/nar/gkt1209
Zhang, B., Wu, X., Tai, X., Sun, L., Wu, M., Zhang, W., et al. (2019). Variation in Actinobacterial Community Composition and Potential Function in Different Soil Ecosystems Belonging to the Arid Heihe River Basin of Northwest China. Front. Microbiol. 10, 1–11. doi:10.3389/fmicb.2019.02209
Zhang, H., Li, S., Zheng, X., Zhang, J., Bai, N., Zhang, H., et al. (2021). Effects of Biogas Slurry Combined with Chemical Fertilizer on Soil Bacterial and Fungal Community Composition in a Paddy Field. Front. Microbiol. 12, 1–13. doi:10.3389/fmicb.2021.655515
Zhang, L., and Lueders, T. (2017). Micropredator Niche Differentiation between Bulk Soil and Rhizosphere of an Agricultural Soil Depends on Bacterial Prey. FEMS Microbiol. Ecol. 93, fix103. doi:10.1093/femsec/fix103
Keywords: microbiota, sampling time, crops, soil, digestate
Citation: Suproniene S, Doyeni MO, Viti C, Tilvikiene V and Pini F (2022) Characterization of the Soil Prokaryotic Community With Respect to Time and Fertilization With Animal Waste–Based Digestate in a Humid Continental Climate. Front. Environ. Sci. 10:852241. doi: 10.3389/fenvs.2022.852241
Received: 11 January 2022; Accepted: 22 April 2022;
Published: 06 June 2022.
Edited by:
María Almagro, Spanish National Research Council (CSIC), SpainReviewed by:
Jessica Cuartero Moñino, Spanish National Research Council (CSIC), SpainCopyright © 2022 Suproniene, Doyeni, Viti, Tilvikiene and Pini. This is an open-access article distributed under the terms of the Creative Commons Attribution License (CC BY). The use, distribution or reproduction in other forums is permitted, provided the original author(s) and the copyright owner(s) are credited and that the original publication in this journal is cited, in accordance with accepted academic practice. No use, distribution or reproduction is permitted which does not comply with these terms.
*Correspondence: Francesco Pini, ZnJhbmNlc2NvLnBpbmlAdW5pYmEuaXQ=
Disclaimer: All claims expressed in this article are solely those of the authors and do not necessarily represent those of their affiliated organizations, or those of the publisher, the editors and the reviewers. Any product that may be evaluated in this article or claim that may be made by its manufacturer is not guaranteed or endorsed by the publisher.
Research integrity at Frontiers
Learn more about the work of our research integrity team to safeguard the quality of each article we publish.