- 1Key Laboratory of Ecosystem Network Observation and Modeling, Institute of Geographic Sciences and Natural Resources Research, Chinese Academy of Sciences, Beijing, China
- 2University of Chinese Academy of Sciences, Beijing, China
- 3Key Laboratory for Resources Use and Environmental Remediation, Institute of Geographic Sciences and Natural Resources Research, Chinese Academy of Sciences, Beijing, China
- 4Key Laboratory of Agricultural Water Resources, Hebei Laboratory of Agricultural Water-Saving, Center for Agricultural Research, Institute of Genetics and Development Biology, Chinese Academy of Sciences, Shijiazhuang, Hebei, China
- 5Department of Civil and Environmental Engineering, College of Engineering, Florida A&M University-Florida State University, Tallahassee, FL, United States
- 6Department of Agriculture and Environmental Sciences, School of Agriculture, Haramaya University, Dire Dawa, Ethiopia
- 7Africa Center of Excellence for Climate Smart Agriculture and Biodiversity Conservation, Haramaya, Ethiopia
Climate change impacts on drylands pose more vexing risks to socio-ecological systems, resulting in food security issues, biodiversity loss, and livelihood shifts in Africa. This study critically reviewed relevant literature to evaluate the complexities and feedback loops between the climate–drylands–food security (CDF) nexus, which helps assess tactics to attain sustainable dryland ecosystem management under the changing environment. Comprehensive CDF frameworks are explored for dryland dynamics, ecosystem services, and food security (FS), and current high-precision ecosystem observation networks are used to detect regional-level climate variability and identify hotspots. In addition, this review also examines challenges and uncertainties for CDF systems and effective agrarian innovations as a way forward. To bridge the gap from science to policy making in the CDF nexus, it is vital to enhance the impacts and feedbacks of ecohydrological processes on agrarian production, ecosystem service tradeoffs and their effects on livelihoods, and regional development and preservation by optimization of the ecological water security pattern. This state-of-the-art assessment uses acquired information and knowledge to conceptually evaluate the past, current, and future impacts and risks and facilitates decision making through the delivery of long-term sustainability and socio-ecological resilience.
1 Introduction
Climate change is one of the world’s most pressing real threats to the drylands, which may jeopardize food security (FS), that is, physical, social, and economic access to sufficient, safe, and nutritious food by people for an active and healthy life (Samuel et al., 2019) and other sectors of our civilization (Tachiiri et al., 2021; Ukhurebor et al., 2021). Drylands are areas where precipitation is balanced by evaporation from surfaces and evapotranspiration (Middleton and Thomas, 1997). They are generally characterized by sparse vegetation, water scarcity, and unpredictability (Berg and McColl, 2021). The distinct biophysical features of drylands make them highly susceptible (Robertson et al., 2018) and complex to unanimous climate change drivers (Berdugo et al., 2020). Upsurging temperatures, changes in precipitation and rainfall patterns, land use, nutrient availability, atmospheric CO2 (Classen et al., 2015; Copeland et al., 2017; Schlaepfer et al., 2017; Leisner, 2020), and other greenhouse gases emissions (GHGs) are key driving factors of unprecedented dryland expansion (Maestre et al., 2012; Li et al., 2019; Lian et al., 2021). Drylands are associated with substantial land degradation and extremely vulnerable to severe environmental shocks and socioeconomic crises (Fraser et al., 2011; UNU-WIDER, 2017). Due to anthropogenic change and non-climatic stressors, in tandem with other stimuli, the mean global temperature has increased by ∼1.0°C and is expected to further increase over the next century (IPCC, 2018). As a result, many dryland habitats are faced with severe threats that lead to reduced carbon sequestration and high water scarcity (UNEP, 2007; UNEP-WCMC, 2011; Bradford et al., 2020). Moreover, by the late 21st century, it is projected that ∼78% of dryland expansion will befall under the representative concentration pathways (RCPs) 8.5 scenario in developing countries (Huang J et al., 2016; Huang et al., 2017). The impaired climate–drylands connection could impact FS in all four dimensions: availability, access, utilization, and food system stability, negatively influencing the efforts toward sustainability and ecosystem resilience in Africa (Connolly-Boutin and Smit, 2016; Niles and Brown, 2017; Mbow et al., 2019). Multi-disciplinary investigations are in need to identify effective techniques and practices, including coupled earth-anthropogenic processes in conjunction with careful management and adaptation measures of potential ecological risks, to enable mitigating the repercussions.
Meanwhile, the two dimensions of the nexus approach are interdisciplinary and transdisciplinary (Pahl-Wostl, 2019). By highlighting the trade-offs and synergies between the components, the primary dimension assesses the complexity of linkages among climate, dryland, and food systems. The second dimension strengthens driving forces such as population growth, socio-economic progress, and climate change, as well as innovation, technology, and policies (Endo et al., 2020). Nonetheless, a three-node nexus of climate change–dryland variation-FS leads to complexity. It also apprehends a “wide portrayal” and facilitates bringing in the socio-economic and ecological dimensions. This approach is considered a flexible and open option (Bleischwitz and Miedzinski, 2018). Tools and methodologies are varied and context-specific, but the linkages from climate change to social and environmental impacts are difficult to model, given the unpredictable anthropogenic activities affecting the outcomes (Devereux and Edwards, 2004). Conversely, new techniques are compelled to understand the complexities that lead to abrupt non-linear/correlation between Earth’s systems (Randall et al., 2007; Stephens et al., 2020) and thresholds due to bulky and/or irretrievable effects (Devereux and Edwards, 2004). In addition to their implicitly multi-scale structure, linkage processes are difficult to simulate and/or emulate because they are rarely at the required spatial and temporal scale to establish specific reference as to the underlying changing aspects. To fully comprehend the CDF linkages, key factors (e.g., population growth, agricultural transformations and industrial development, technology and innovations, livelihood shifts, and governance and policy implementation) that drive those nexus complexities must be assessed and described for the entire system through the lens of climate change.
Correspondingly, a wide range of multi-spatiotemporal scale integrated frameworks focused on dryland changes, climate–land–energy–water (CLEW) nexus (Vinca et al., 2021), water–energy–food (WEF) nexus (Kogan et al., 2017; He et al., 2019; Kogan, 2019), water–energy–food–environment nexus (WEFE) (Malagó et al., 2021; Mirzaei et al., 2021), water–energy–food–biodiversity–health (WEFBH) nexus (Hirwa et al., 2021), and others have been set up. However, they are not sufficient anymore (Fernández-Ríos et al., 2021). Instead, current advances in climate change, dryland ecosystem management, and FS are hindered by the limitations of inadequate data on dryland environments and the methodologies commonly used for scientific data analysis, some of which are ill-equipped for capturing complex relationships present in the huge volumes of available data. Coupling large-scale field spatial observations with model simulations is now considered the most viable opportunity and accurate technique to identify dryland ecosystem shifts and evaluate dryland ecosystem stability. But, resistance and recovery after extreme events such as droughts, as a high priority needs urgent attention (Ruppert et al., 2015; Burrell et al., 2017; Wei et al., 2022). Development of using geospatial tools by multiscale frameworks continues to present key fundamental gaps (Fritz et al., 2019). In addition, various methods have been used to assess the influence of extreme events on dryland degradation (Wang et al., 2012; Dubovyk, 2017; He et al., 2019). Global FS requires transdisciplinary responses and interventions at different types of scale (Drimie and McLachlan, 2013), that is, globally (Schmidhuber and Tubiello, 2007; Yadav and Congalton, 2018), regionally (Ingram, 2011), and locally (Moore et al., 2012). With global climate change, dryland variation, and FS, there is the additional challenge of uncertainties, which is unlikely to decrease in the next coming decades (Campbell et al., 2016). There are gaps between research and technology transfer, research and implementation, research and practice, and science and policy. It is, therefore, urgent to seek alternative resources, efforts, and procedures that combine local with emerging scientific knowledge through more effective dissemination of information and technology, appropriate participatory learning, and partnerships.
Few current research on climate change, dryland variation, and FS in Africa have been published (Wheeler and von Braun, 2013; Cervigni and Morris, 2016; Guilpart et al., 2017; Li and Zhang, 2017; Leakey, 2018; Schouten et al., 2018; Nyberg et al., 2019; Chimwamurombe and Mataranyika, 2021). Consequently, developed and developing nations started focusing on new tools and strategies for boosting agricultural production to meet future challenges, and improving or advancing techniques that would help deal with food (in) security and monitor the expansion of drylands (Peng et al., 2021). The apparent potential for developing more holistic and cost-effective tactics, including using existing strategies and procedures as foundations, through developing novel methods that integrate RS and local participation, necessitates a suitable synopsis of dryland dynamics and FS on distinctive spatiotemporal scales.
In a nutshell, this succinct review aims to address both the vexing and progressive threats between climate change, dryland dynamics, and FS through the lens of novel systems approach, advances, challenges, and future opportunities. The CDF nexus provides a strong foundation for scientists, environmental decision-makers, and activists and actors who are interested in achieving all targets of the 17 sustainable development goals (SDGs), particularly SDG 13 (climate action), SDG 15 (use of ecosystem services), SDG 2 (zero hunger), and the Paris Climate Agreement, thereby devising effective policy for action and planetary well-being. This study also proposes a conceptual framework clarifying the interlinkages between influencing systems (i.e., drylands, climate, ecosystems, socio-ecological, and food systems) that consistently unravel and build greater resilience to the confounding vulnerabilities, shocks, and stresses within the food networks.
This study employs various research publications, books, reports, and case studies collected from official websites. Hence, we organize our review into four major aspects and then discuss them using past and current literature. These aspects are: 1) the CDF nexus, including dryland distribution and their associated impacts factors, the relationship between compounded climate, dryland, and FS; 2) technique advances in drylands monitoring methods, including regional observation networks and innovative technologies; 3) challenges and uncertainties for climate change, dryland dynamics, and FS measurements; and 4) future directions and research opportunities to improve dryland ecosystems management and cope with ongoing risks related to FS under climate change conditions.
2 Methodology
2.1 Study area
Africa’s inhabited dryland areas (mainly arid, semi-arid, and subhumid zones) cover 11% of Earth’s surface, 27% of the planet’s drylands, and 40% of the continent’s surface (Figure 1) (Wei et al., 2021). In these regions, the majority of the population (∼85%) relies on subsistence rainfed agriculture and pastoralism (Kogo et al., 2021). The main staple subsistence crops are wheat, rice, maize, sorghum, and millet (Tsusaka and Otsuka, 2013). Increase of multiple climatic extreme events, including rainfall variability (Bradford et al., 2020), high temperatures (Webb et al., 2017), erratic droughts (Adhikari et al., 2015), and changing agro-ecological conditions (Schmidhuber and Tubiello, 2007; Scheelbeek et al., 2018) has significantly affected dryland agriculture with high uncertainties since the 1980s (Defrance et al., 2020). Ultimately, model simulations and other evidence clearly show that continued global warming will make the earth’s drylands drier over time (Overpeck and Udall, 2010; Huang et al., 2012; Feng and Fu, 2013; Koutroulis, 2019). Climate models predict high evapotranspiration and lower soil moisture levels in arid and semi-arid regions of Africa (McCarthy et al., 2001; Bathiany et al., 2018), suggesting some tropical grasslands could become drier and unsuitable for farming (Schmidhuber and Tubiello, 2007).
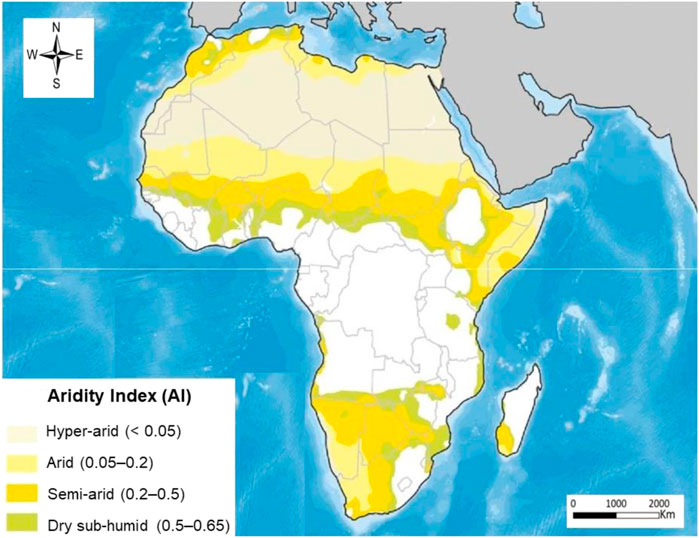
FIGURE 1. Distribution of Africa’s drylands based on aridity index (AI). The AI was calculated using the annual precipitation (P) and potential evapotranspiration (PET) based on the 1970–2000 mean global-AI and global_ET0 datasets (Trabucco and Zomer, 2018). The hyper-arid zones are not considered in this study.
2.2 Methods
VOSviewer, a software tool for constructing and visualizing bibliometric networks (Perianes-Rodriguez et al., 2016), was used to conduct the similarity analysis of high frequency terms in the titles and abstracts of the articles and to generate a keyword tagging map. Studies published from 1980 to 2022 were analyzed. The keywords were mainly categorized into four subjects: “climate change,” “drylands,” “food security,” and “Africa”. Databases used for extraction of studies, reports, and published articles included Web of Science™ (WoS) Core Collection database, Scopus, and ScienceDirect. In addition, reports on the impacts of climate change on either drylands or food security in Africa were also extracted from official websites of international organizations such as the Intergovernmental Panel on Climate Change (IPCC), Intergovernmental Science-Policy Platform on Biodiversity and Ecosystem Services (IPBES), United Nations Framework Convention on Climate Change (UNFCCC), and the Food and Agriculture Organization (FAO). Moreover, 2,820 studies were initially extracted. Referring to their abstracts, 152 studies were considered pertinent to this study. Out of the 152 publications, 88 studies were used to provide a summary of the nexus between the impacts of climate change on dryland variation, and food (in)security in Africa. In the context of interconnections, climate change, dryland ecosystem shifts, and food security in Africa are studied because there is a strong connection between the three systems to form a nexus. The analysis reveals that the dryland variation is mostly influenced by climate change, which results in food insecurity in Africa (Figure 2). As shown in Figure 2, it is clear that more prior research has been focused on climate change, household, and household food security.
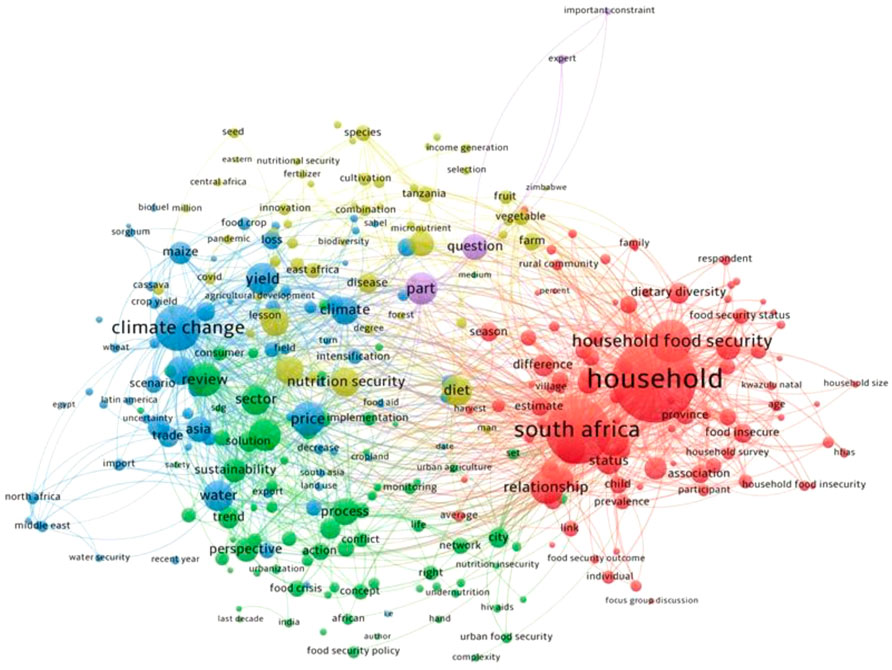
FIGURE 2. Diagram of clusters of topic terms for climate change, dryland variation, and food security nexus. The larger the node, the more frequently keywords appear in research. Lines stand for the co-citation relationship. Different colors denote research topics.
3 Overview on impacts of climate change on drylands and food security in Africa
3.1 Climatic changes in arid and semi-arid environments
Progressive shifts in climatic or weather variability influence both dryland biophysical and socioeconomic reciprocities (Berg et al., 2016; Greve et al., 2019; Overpeck and Udall, 2020). The main drivers of dryland variation include climatic factors (i.e., high temperature, changing rainfall patterns, and infertile soils) and anthromes (e.g., agriculture, urbanization, livestock grazing, and wildfires). The interconnected natural processes of degradation are water and wind erosion, salinization, and organic matter (OM) loss, which furtherance results in a decrease in soil health, agrarian productivity, and the ability to reduce carbon (C) emissions into the atmosphere (Reynolds et al., 2007). Over-exploitation and land degradation of ∼4 × 109 ha (∼73% of the total area of rangelands) resulted in soil loss of ∼216 × 106 ha (∼47% of SSA’s drylands), degradation of 43 × 106 of irrigated croplands (∼30% of total SSA’s drylands) (UNEP, 2021). Nevertheless, dryland ecosystems and their biodiversity are strongly shaped by interdependent components such as topography, geology, rainfall, herbivores, fires, and human management (Davies et al., 2012). For instance, in Southern Africa, dry forests particularly, the Miombo woodland have the capability of storing ∼100 tons of carbon/hectare. In addition, the coupled human biomes development leads to land degradation and a net loss of carbon storage, deteriorating the impacts of climate change (Stringer et al., 2012).
3.1.1 Increase in temperatures
Global warming trend has indeed been detected over drylands since the 1980s, with further warming predicted in the near future (Huang et al., 2016). Africa’s drylands are known for their high temperatures (Put et al., 2004; Zhang et al., 2021). Climate change-induced temperature rises are likely to aggravate existing vulnerabilities of natural semiarid systems like droughts, water scarcity, and floods (Koohafkan and Stewart, 2008). The extreme variation in rainfall and the overall water shortage constrain nutrient accumulation in dryland ecosystems, impeding biogeochemical nutrient cycling (Laban et al., 2018). The study by Daramola and Xu (2021) reported that temperature generally increased across all dryland areas, with the warmest years identified between 2015 and 2017 except for the hyper-arid zones where the highest temperature increase occurred in 2010. Extreme temperature occurrences have a severe impact on agriculture in Africa since many crops are already planted at the boundaries of their thermal tolerance and water stress resilience (Scholes et al., 2015).
3.1.2 Decrease in rainfall patterns and poor nutrient soils
Prior research showed that precipitation generally decreased over the drylands and summer precipitation increased over Southern Africa as well as Northern Africa’s dryland areas (Daramola and Xu, 2021). High precipitation years in Southern Africa caused an initial spike in fire rates, which then declined in subsequent years (Wei et al., 2020). Dryland soils are defined as having low organic matter (limiting microbial processing of nutrients for plants), weak structure and high salt content, and limited moisture retention capabilities (Safriel, 2017; Plaza et al., 2018). These, however, are enhanced by extreme variations in rainfall and overall water deficiency (Hartley et al., 2007).
3.1.3 Wildfires
Wildfires are an extreme threat to dryland environments (e.g., grasslands, savannas, or dry forests) and the threat is increasing due to increased ignition potentials by humans, the spread of fire-prone invasive grasses, and shrubs, surface temperature, and dry conditions. The dramatic increase in wildfire prevalence in recent decades poses serious threats to human safety, infrastructure, agricultural production, cultural resources, native ecosystems, and watershed functioning. It is especially prevalent in Africa, with up to 9% of the continent burnt on an annual basis (Andela et al., 2013), which contributes to 70% of the global burned area (Andela and van der Werf, 2014). More extensive dry season fires lead to wet season rainfall deficits of up to 30 mm (Saha et al., 2016). Subsequently, the occurrence and impacts of wildfires must be reduced through prevention, preparedness, and pre-fire management. The post-fire response such as erosion control and replanting in burned areas also helps reduce the immediate impacts of wildfire and establish non-native grasses, reducing the risk of future fires.
Variations in surface temperatures change the water dynamics in the soil, impacting crop yields directly. The warming trend will also lead to soil surface temperature increase, resulting in a decrease in rainfall patterns and soil nutrients. Although wildfire occurrence and extent have been linked to rainfall and temperature on regional scales, the atmospheric mechanisms that drive regional patterns of rainfall and temperature need to be further investigated.
3.2 Climate change and food security
3.2.1 Food availability
Availability refers to the physicality of food. Different foods can be produced in different ways. Agriculture in the drylands is dominated by small-scale and resource-poor farming, which is characterized by declining crop yields and livestock productivity and suffers from limited investments in agricultural technologies and inputs (Mortimore et al., 2009). Heat and drought stress, as well as increased insects (Salih et al., 2020), plant diseases (Graziosi et al., 2020), and flood damage (Atanga and Tankpa, 2021), thus have significant consequences for regional, national, and household food security and livelihoods (Blunden and Arndt, 2020). Under RCP 8.5, reductions of 13, 11, and 8% in mean cereal yields are projected in West and Central Africa, Northern Africa, and Southeastern Africa, respectively, based on the yield indicator of crop production per area of harvested land (WMO, 2020; Stuch et al., 2021). In addition, climate impacts the production of roots and tuber crops in different ways, such as changes in sowing time, pest and disease infestation of crops, and low crop yields (Owusu et al., 2020). Concerns have been raised that converting Africa’s dry tropical forests and savannahs to croplands for agricultural production may undermine the biomes’ natural carbon reserves (IPCC, 2019). Livestock has both positive and negative effects on dryland resources. Nevertheless, about 25 × 106 pastoralists and 24 × 107 agro-pastoralists rely on livestock as their main source of income. In sub-Saharan Africa (SSA), 35% is permanent pasture (Kiage, 2013).
According to the study by Fischer et al. (2002), land suitable for double cropping would be reduced by 2 × 107 ha whereas for triple cropping would decrease from 5 × 106 to 1 × 107 ha (Grote et al., 2021) in SSA. From 2000 to 2050 in SSA, due to combined high temperatures and rainfall shortages, maize, millet, and wheat production is expected to decline by 5, 10, and 15%, respectively (Shiferaw et al., 2013). In Tanzania in eastern Africa, the maize yields will shrink by about 33% for the overall country. For the central regions, there will be an 84% decrease. Moreover, a decline in mean maize yields is projected for over 85% and 25% of harvested maize areas in Southern Africa and West Africa, respectively (Stuch et al., 2021). In many instances, crop production is not only affected by climate change and abiotic stresses, such as warmth and water scarcity (Cairns and Prasanna, 2018; Deutsch et al., 2018), but also biotic factors such as novel viral pests, insects, and diseases [e.g., Case of deserts locusts in Eastern Africa drylands (Kassegn and Endris, 2021)]. Finally, all these fluctuations continue to adversely affect food supplies, food prices, and malnutrition-related diseases (Levy et al., 2016). In light of these results, it is clear that there is much uncertainty regarding future forecasts of food production under climate change. Therefore, the implications for agro-socio-ecological linkages are important to accurately predict system dynamics from climate change.
3.2.2 Food accessibility
Generally, accessibility refers to the ease of acquiring foods in a form and location that enable their consumption. Weather-related shocks might undermine food security through various levels of change and food price volatility (Porter et al., 2014). Local food supply in many nations is mostly reliant on global food exchanges (or trade) and adverse climatic conditions such as floods, cyclones, and hailstorms alter agricultural commodities and transportation infrastructures at national to regional scales, thus influencing food supply at variable levels. However, changing climate affects food production, farmers’ income, access to food, supply, and safety (Affoh et al., 2022). COVID-19 has had a vexing effect on food security and marginalized dryland communities across Africa, which serves as an external driver of FS (Ukhurebor et al., 2021). Food, livestock traders, and consumers have experienced restrictions on cross-border mobility and relations leading to a surge of spoiled goods due to prolonged transit times. For instance, in Eastern African countries, truckers regularly line up for miles when crossing the borders of neighboring countries. In addition, the effects of political instability in the Democratic Republic of Congo, Ethiopia, and South Sudan have caused the people to flee across the borders (O’Grady, 2021). From 2019 to 2020, the acute food insecurity induced by population change has increased to 1,033, 883, 600, 333, and 250% in Mali, Chad, Burundi, Sierra Leone, and Cameroon, respectively (WFP&FAO, 2020).
Climatic instability in SSA, however, could destabilize local markets, curb economic growth, and heighten the risk for agricultural investors in the north and south arid regions, which will lead to increased childhood hunger by rising food prices. It is projected that the price of maize will increase by 104% between 2005 and 2050 (Rosegrant et al., 2014), although the systematic analysis of the relationship between weather shocks and domestic food prices is rather limited (Mirzabaev and Tsegai, 2012). Advances in well-being may result from a more equitable allocation of benefits among many stakeholders and beneficiaries. Furthermore, a policy environment that fosters construction of better storage, and freer trade and promotes investments in transportation and irrigation infrastructure can help deal with these problems early on.
3.2.3 Food utilization
Food utilization is closely linked with the general health environment, water, and sanitation (Vilakazi et al., 2019), which is indirectly impaired by climate change (Wheeler and von Braun, 2013). Climate change could have a direct effect on micronutrient consumption in three forms: by lowering important micronutrient source crop yields, altering the nutritional balance of a particular crop, or influencing crop selection decisions (Felix and Romuald, 2012). Due to uneven actual food distribution across Africa and diverse populations and households, food utilization is understudied (Myers et al., 2017). In response to food price shocks, urban and rural households adjust their consumption patterns in a number of ways such as decreasing caloric intake, decreasing the number of meals per day, decreasing food diversity, or substituting with less preferred foods (Matz et al., 2015; Kubik and May, 2018). Across most dryland areas in Africa, many poor people still face difficulties in obtaining adequate calorie intake and/or diverse quality diets. The proliferation of small-scale agro-processing industries and modern storage techniques in both rural and urban areas can increase food security by diversifying agrarian products and enhancing nutritional standards as well as creating the employment (Adeyeye, 2017). The “atta” for cowpea in Benin is a typical example (Kpossilande et al., 2020). Moreover, processed food can be purchased in various forms for each and every category of household (Reardon et al., 2021). Importantly, several studies revealed that agricultural policies have contributed in many SSA countries to increased food production, which helps the population to acquire more nutritious diets and improve livelihoods (Pernechele et al., 2018).
3.2.4 Food stability
Food stability is established when food supply and people’s ability to access and consume food remain stable and consistent over time (Bonuedi et al., 2020). The major causes of food instability include recurrent droughts, geopolitical instability, conflicts, lack of investments in agriculture, unstable markets, and poverty (WFP, 2019). More importantly, even temporary disruptions of food access resulting from food inflation can entail long-term, often irreversible nutritional damage, especially amongst infants and young children during the period of critical growth and development (Arndt et al., 2016). Because of the short-term supply fluctuations, the stability of complete food systems may be jeopardized as a result of climate change (Grote et al., 2021). Furthermore, abiotic (e.g., weather) and biotic (e.g., pests) shocks can compromise cereal stability. As staple crops like wheat and maize are planted in large areas, losses from pests, diseases, and climate change may be catastrophic (Conceição et al., 2016).
3.3 Feedbacks between climate–drylands–food security interactions and drivers
Dryland FS is driven by several factors. Changing climate is just one of many interconnected trends and drivers that shape dryland agricultural systems, including FS and nutrition (Brown et al., 2018). The dryland socio-ecological system comprises a food system, which is, furthermore, a complex adaptive system (Pereira, 2013; Allen and Prosperi, 2016). The most noteworthy technical advances and socio-economic factors that drive changes in food systems include technological and structural changes in the food system, food production, processing, distribution, and markets, population growth, wealth shifts, changing demographics, globalization, catastrophe management, and energy production, availability and use (Ingram, 2011; Pingali, 2012). Likewise, a sustainable food system is critical to the households’ survival and community resilience in Africa (Smit, 2016).
Bringing various fundamentals together, the integrated conceptual framework, illustrated in Figure 3, shows how climate, dryland, and food system give rise to a set of socioeconomic, ecosystem, food, livelihoods, and policy systems. The ecosystem services are stratified according to the livelihood outcome and other factors and the climate, in turn, shape-specific elements of dryland expansion and FS. Food system development must be evaluated not only in terms of economic efficiency and capacity to enhance FS but also in terms of their environmental impacts throughout the food chain. The climate change implications on drylands, ecosystem, food, and the socio-economic systems could gain from facets of all FS and improved livelihoods, thus providing a certain comprehensive understanding of the whole system as vividly illustrated in Figure 3.
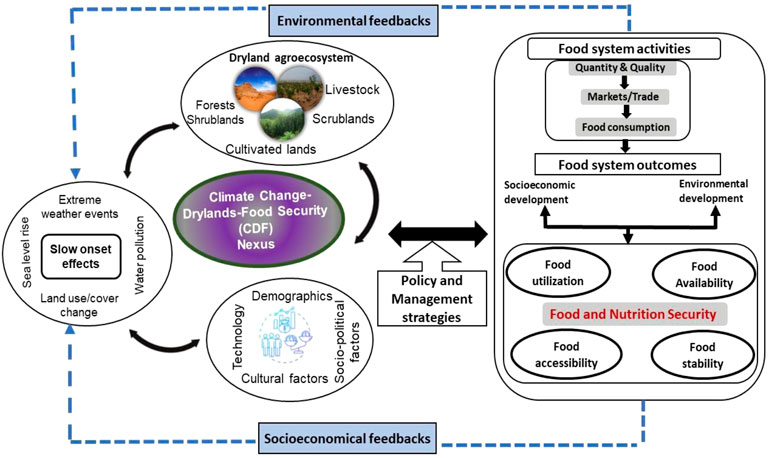
FIGURE 3. Interrelationship among the dryland agroecosystem, climate system, socio-economic system, and food system. Here, we present the CDF nexus (epicenter) from the viewpoint of environmental and socioeconomic feedback (above and below) and food system outcomes (right side). In particular, the anthropogenic activities, natural processes, and socioeconomic operations together drive dryland agroecosystem changes in African drylands. All these combined factors, however, positively/negatively impact the stability and income level, via effects on productivity, production costs, and market prices resulting in food insecurity and malnutrition.
4 Technical advances in climate change, dryland ecosystem monitoring, and food security
4.1 Progresses of dryland agroecosystem dynamics detection techniques
Over the past four decades, methods to detect and quantify the relative dryland expansion and land degradation have been developed (Wang et al., 2012; AghaKouchak et al., 2015). The RS techniques and spatial modeling are commonly used tools for quantifying spatio-temporal trends of LU/LC change in drylands (Ohana-Levi et al., 2019). Over the last four decades, the advent of earth observations (EOs) has been highly relevant for enhancing data availability in drylands globally (Figure 4). Cost-effective atmospheric conditions in drylands complimented the extra huge need by providing the improved probability of high-quality data due to decreasing cloud cover for optical RS (Smith et al., 2019). Henceforward, several RS integrated tools have been introduced in drylands, including sensors such as Advanced Spaceborne Thermal Emission and Reflection Radiometer (ASTER), Compact High-Resolution Imaging Spectrometer (CHRIS), Landsat thematic mapper (TM), Landsat multispectral scanner (MSS), Landsat operational land imager (OLI), and thermal infrared sensor (TIRS) with different multi-spectral satellite products such as atmospheric profiles product (MOD), Moderate Resolution Imaging Spectroradiometer (MODIS), modified atmospheric profiles from reanalysis information (MAPRI), and Atmospheric Correction Parameter Calculator (ACPC) (Kowalik et al., 1982; Jiménez-Muñoz et al., 2010; Fritz et al., 2019). Quantitative estimates of Vegetation Health and biomass dynamics based on the Visible Infrared Imaging Radiometer Suite (VIIRS) of Landsat MSS offer a dimensionless measure of greenness with normalized difference vegetation index (NDVI) (Rouse et al., 1974; Tian et al., 2016). Multi-source and multi-scale data sets, and fusion algorithms that intelligently integrate in situ data, remote sensing observations, and modeling results, are required to capture the complex spatial and temporal land and vegetation dynamics processes.
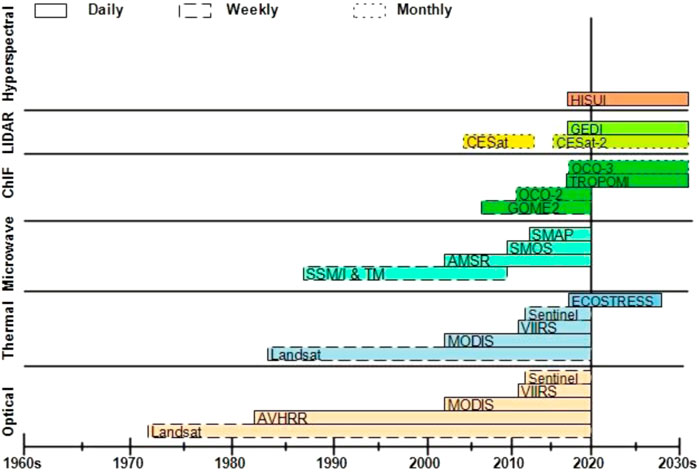
FIGURE 4. Advances in satellite imagery and multi-spectral RS of dryland vegetation dynamics. Historical milestones are provided from the 1960s to the 2030s. Timelines of LiDAR, chlorophyll fluorescence (ChIF), thermal infrared (TIR), microwave, optical, and hyperspectral earth observation satellites are shown. The progression of satellite capabilities from optical to hyperspectral indicates both the rising spatial and temporal resolution of sensor information as well as the extension of RS techniques in drylands. Modified and adapted from (Kuenzer et al., 2014; Smith et al., 2019). The color differentiates the type of satellite and its capability. The solid, semi-solid, and dash-dash lines represent daily, weekly, and monthly scales, respectively, as the data acquisition time.
Dryland RS satellites are defined in terms of spatial and temporal resolution (Figure 5). Apart from the Advanced Very High-Resolution Radiometer (AVHRR) and Landsat, no sensor line allows for three to four decades of long-term monitoring of thermal patterns. While AVHRR provides two thermal observations per day on average, Landsat also has a 16-day repeat cycle. Thus, cloud-free data may only be accessible a few times annually, especially in overcast latitudes (Kuenzer et al., 2014). Several studies employed the AVHRR NDVI time series to assess the long-term patterns in regional vegetation heterogeneity and drivers in African drylands (Anyamba and Tucker, 2005; Donohue et al., 2009; Fensholt and Rasmussen, 2011).
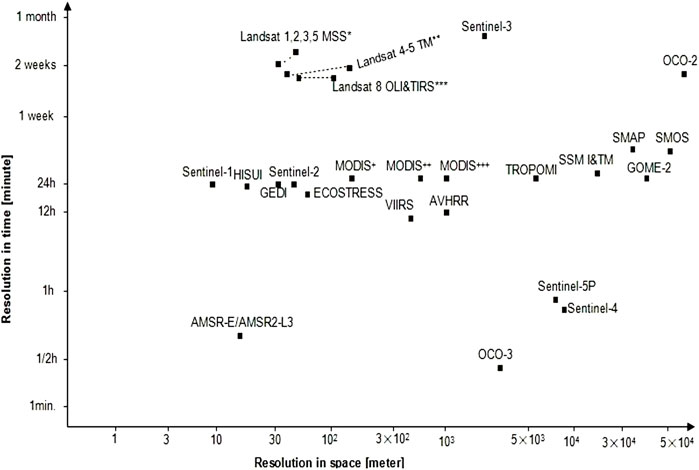
FIGURE 5. Comparison of RS satellites. The multispectral scanner (MSS)* its original pixel size was 79 m × 57 m, where the production systems now resampled the data to 60 m. The thematic mapper (TM)** band 6 was acquired at 120 m resolution, but products are resampled to 30 m pixels. Therefore, the Landsat 8 operational land imager (OLI) and thermal infrared sensor (TIRS)***, the TIRS bands are acquired at 100 m resolution but are resampled to 30 m in the delivered data product. The spectral band placement for each sensor is visually displayed. The MODIS consists of several bands, including bands 1–2+, bands 3–7++, and bands 8–36+++.
4.2 Observational networks as essential to Africa dryland ecosystem management
Long-term ecological research (LTER) is a method of assessing biophysical interactions with human activities and how they affect the ecological integrity, particularly environmental processes and humanity’s carrying capacity (Vanderbilt and Gaiser, 2017). These networks deal with climate and anthropological impacts on grassland, forests, freshwater, deserts, coasts, and other ecosystems that span a wide topographical range (Yevide et al., 2015). Some ecosystem research networks (ERNs) have been established in Africa (Table 1). These monitoring initiatives are intended to develop response strategies for any potential consequences such as biodiversity loss, land degradation, desertification, and extreme events (Li et al., 2015). Ecosystem monitoring via international LTER (ILTER) emerges in countries and is now applied all over the world (Yevide et al., 2016). Currently, the ILTER covers about 44 nations and 700 experimental stations, integrating observation of ecological aspects to serve the needs worldwide (Mirtl et al., 2018). Therefore, the advancement of dryland-specific models and novel assessment technologies for drylands cannot act without substantial and specialized observational networks (Smith et al., 2019). These networks currently exist in some regions, for example, HiWATER, OZFlux, and Semiarid ECohydrology Array (SECA). Africa is, however, one of the continents that owns continental and regional-scale monitoring networks, including the South African environmental observation network (SAEON) (Gray and Kalpers, 2005; Jürgens et al., 2012). Rapidly advancing technology will continue to impact LTER’s tasks. The monitoring sites generally continue to be sparse, scattered, and biased toward dryland ecosystems. Development of ecotechnologies is needed. A need for scientifically-based peer-reviewed research using ‘‘bottom-up’’ rather than ‘‘top-down’’ help answer pressing.
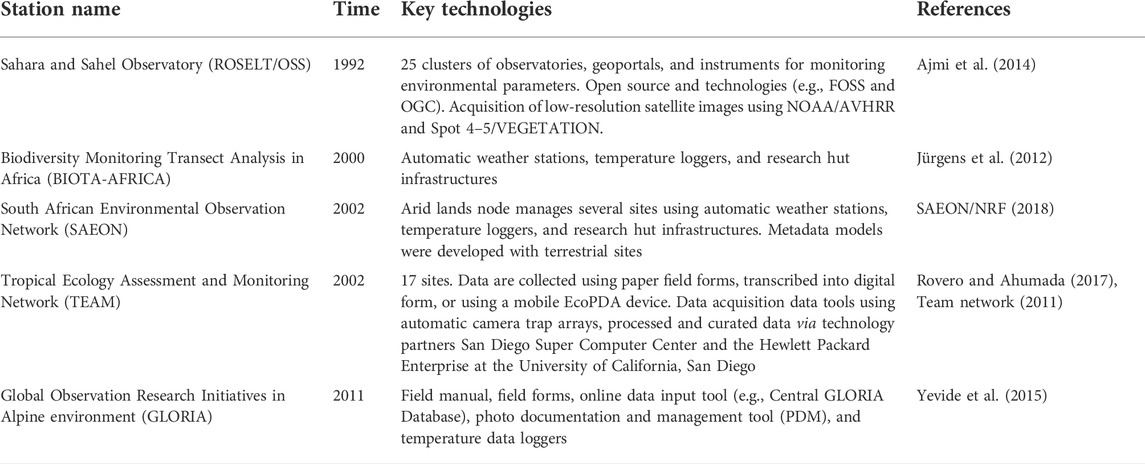
TABLE 1. Examples of African Ecosystem Research Networks (AERNs) dealing with climate change, dryland monitoring, and FS.
In 1992, the Global Climate Observing System (GCOS) was created. All stakeholders that require climate information, from research to forecasting and impacts to mitigation and adaptation, are ensured to have access to adequate information and trends in the climate system. (Verstraete et al., 2009). Various technologies have also been developed to help address concerns about dryland expansion, climate variability, FS, and other environmental assessments (Smith et al., 2019). However, long-term ecological research infrastructures are often fragmented, unevenly distributed in space, and restricted to particular scientific objectives (Hass et al., 2018). Multiple global ecosystem research networks that help enhance investigations related to climate change, environment, and FS necessitate funds in research and development, human capital, knowledge flows, and infrastructure.
5 Challenges and uncertainties for climate change, dryland dynamics, and food security
Climate models (CMs) are weather forecasting extensions. Moreover, these models provide information on hydro-biogeochemical cycles (Foley, 2010; Wang et al., 2015). Scientists utilize the CMs to draw past, current, and future conclusions about complex earth systems (Huang et al., 2017). The most intricate and reliable models for understanding climate systems and forecasting climate change are General circulation models (GCMs) and regional climate models (RCMs), which may need bias corrections and model output statistics (MOS) (Eden and Widmann, 2014). For instance, Keenan et al. (2016) testified that during the last decades, in the warming break of drylands, a current hiatus of crop growth rate was linked to a rise of atmospheric CO2 in the terrestrial sink, which was attributed to the effects of atmospheric CO2 on vegetation (Ballantyne et al., 2017). Consequently, because global carbon cycle dynamics are not included in some CMIP5 models, CMIP5 cannot duplicate this trend without significant uncertainty (Huang et al., 2017). Even for state-of-the-art models of global carbon cycling, the carbon concentration still has a lot of uncertainty. The case of the West African monsoon is an example (Klein et al., 2017). Nonetheless, various models are built based on the same modeling institutions. Thus, the ensemble of CMs is not weighted. There are great uncertainties remaining in evaluations of the global trends in dryness and wetness under climate change conditions (Trenberth et al., 2014). To handle the uncertainties in aridity projections and the aridity index (AI) calculation against the hydro-ecological variables, there is a need to consider regions where the overwhelming of models agree in sign (Greve et al., 2019). Moreover, the use of time series precipitation and evapotranspiration datasets from meteorological stations could be helpful to reduce uncertainties in AI projections and regional dryland climate modeling (Tarek et al., 2021).
Dryland climate system uncertainty over human action possesses two main sources, including uncertainty due to unknown future emission concentrations of greenhouse gases and aerosols, and uncertainty of the climate system’s response to our actions (Trenberth and Trenberth, 1992; Smith et al., 2009). This information, combined with climate models, allows decision makers at all levels of governance to determine how both natural and manmade influences have and will impact changes in our climate.
6 Future directions and research needs
In the past four decades, drastic population development has been observed in drylands (Smit, 2016; Ellis et al., 2021). Subsequently, modern dryland farming and intensive land use are necessary. Sustainable agriculture comprises multiple components, including the introduction of climate-adapted cultivars and sustainable environmental protection that integrates provision and preservation of ecosystem services by enhancing durable intensification programs based on conservation agriculture and community-based adaptation and mitigation with operational support services (e.g., biodiversity, food production, and reduction of GHG emissions) (Mbow et al., 2014; Sanz et al., 2017). Therefore, planning of the so-called food–energy–water–biodiversity–human health (WEFBH) nexus has revealed practicality in evaluating strategic policy to achieve the SDGs prior to the rising demands, dryland resource scarcity, and climate variability (Albrecht et al., 2018; Hirwa et al., 2021).
Remote sensing data have been utilized to provide information in data-scarce areas to address climate variability and FS induced by shifts in foundational dynamic ecosystems. The extension of dryland-specific modern observation models, networks, and evaluations of new RS technologies is a key to successful dryland ecosystem management. These technologies exist in some areas across Africa (e.g., AngoSat-2, NileSat-301, and NARSSCube-2) (Woldai, 2020). Owing to advances in model development from the late 1990s until now, modeling efforts have inspired more current observational investigations. In this instance, measurements are frequently provided apropos of regression models, and multidecadal aerial images are used to identify vegetation changes, for example, in the case of Niger over a forty-year interval. Nonetheless, some models (e.g., Brusselator model) can be overly mechanistic in their representation of many processes at hand, resulting in a high dimensionality that must be calculated from data. As a result, this modeling approach is frequently linked to observation and involves comparisons to field-based assessments (Figure 6). Ultimately, we recommend close collaboration between geo-information data-driven modeling approaches and terrestrial ecosystem modelers to more swiftly categorize model structural deficiencies and hence intrinsically empower more precise dryland ecosystem functioning model projections with the social and ecological system.
Commonly known technologies are categorized into two main types of hardware and software resources, including open-source and affordable tools and scanners, sensors, and platform networks. Therefore, an increasingly growing pool of comparatively low-cost innovations is spurring the transition from catchment to subnational measures (Richardson et al., 2018). The ecosystem phenology camera network can be used to estimate the carbon flux, photosynthesis, and canopy greenness in dryland vegetation (Richardson et al., 2013), and mobile devices can be redeployed to record and capture ecological data. For instance, the Land-Potential Knowledge System (LandPKS) recognizes soil and land types, monitors soil health and vegetation, and identifies management options (Herrick et al., 2016). This could be used to verify remote sensing products, assess earth surface model projections, seasonally explain ecosystem-scale data, and investigate the climate change effects on the terrestrial ecosystem (Seyednasrollah et al., 2019). Public and private institutions can reduce expenditures on design, research, and development, via surplus non-custom devices that are relatively inexpensive and widely available.
7 Conclusion
At present, dryland ecosystem degradation meets increasingly severe climate change. Increasingly, widespread, frequent, and extreme weather events substantially impact food security, especially the sufficiency and regularity of food production. In this review, the bibliometric approach was used to assess the research trends, which identified that research demand on the impacts of climate change on drylands and FS has been increasing. African drylands harbor enormous exceptional levels of biodiversity via diverse land-use systems and provide a variety of ecosystem services. However, they are ecologically fragile in a plethora of ways. There is a strong relationship between climate change, dryland change, and food systems. With regards to the digital revolution in the RS field, in addition to continuing to use conventional methods to detect the impact of climate change on arid and semi-arid regions, technical innovations (e.g., ecosystem observational/research networks) and modern practices (e.g., climate modeling tools) focusing on dryland changes and FS in Africa are very rare across all sectors. Novel methods, such as coupling different vegetation indices, are urgently needed and encouraged to support conventional dryland RS and FS assessment, from basin to regional scales. With particular efforts to the tactically explored studies, we propose an integrated conceptual framework of different systems (i.e., drylands, climate, ecosystem, socio-economic, and food system) (Figure 3). The foresight and prediction assessment of driving forces of the climate, drylands, and FS needs further research (Section 6). The framework has the potential to reveal new insights into climate change, dryland ecosystem dynamics, and FS with the availability and accuracy of data in the entire system. The nexus approach combines intradisciplinary sections involving all socioeconomic and ecological fields to better understand the regional impacts and develop adaptive strategies while mitigating the climate change impacts on drylands. Herein, we propose new research opportunities to strengthen the CDF nexus: ① promoting sustainable agricultural best management practices and innovations as a tool to enhance community resilience and cope with climate change impacts on FS, ② using modern observational data and developing idealistic models to better understand the CDF nexus approaches, and ③ strengthening dryland research and management effectiveness through emerging and affordable technologies. By combining these research directions, we may gain new insights into dryland dynamics, ecosystem services, and FS. We recommend decision makers design policy instruments that consider CDF fields as a multidisciplinary nexus.
Author contributions
HH: conceptualization, methodology, investigation, acquisition of data, formal analysis, writing—original draft, and review and editing. FL: conceptualization, methodology, formal analysis, writing—original draft, review and editing, supervision, and funding acquisition. YQ, SM, FM, CT, PL, AI, RI, GC, and BT: writing—review and editing.
Funding
This research was supported by the National Natural Science Foundation of China (Grant Nos. 41761144053, 41561144011, U1906219, and U1803244).
Acknowledgments
The first author was sponsored by the Chinese Academy of Sciences (CAS)—The World Academy of Sciences (TWAS) President’s Fellowship Programme for his Ph.D. study at the University of Chinese Academy of Sciences.
Conflict of interest
The authors declare that the research was conducted in the absence of any commercial or financial relationships that could be construed as a potential conflict of interest.
Publisher’s note
All claims expressed in this article are solely those of the authors and do not necessarily represent those of their affiliated organizations, or those of the publisher, the editors, and the reviewers. Any product that may be evaluated in this article, or claim that may be made by its manufacturer, is not guaranteed or endorsed by the publisher.
References
Adeyeye, S. A. O. (2017). The role of food processing and appropriate storage technologies in ensuring food security and food availability in Africa. Nutr. Food Sci. 47, 122–139. doi:10.1108/nfs-03-2016-0037
Adhikari, U., Nejadhashemi, A. P., and Woznicki, S. A. (2015). Climate change and eastern Africa: A review of impact on major crops. Food Energy secur. 4, 110–132. doi:10.1002/fes3.61
Affoh, R., Zheng, H., Dangui, K., and Dissani, B. M. (2022). The impact of climate variability and change on food security in sub-saharan Africa: Perspective from panel data analysis. Sustainability 14, 759. doi:10.3390/su14020759
AghaKouchak, A., Farahmand, A., Melton, F. S., Teixeira, J., Anderson, M. C., Wardlow, B. D., et al. (2015). Remote sensing of drought: Progress, challenges and opportunities. Rev. Geophys. 53, 452–480. doi:10.1002/2014rg000456
Ajmi, M., Hamza, M. H., Labiadh, M., Yermani, M., Khatra, N. B., Al-Thubaiti, A. S., et al. (2014). Setting up a spatial data infrastructure (SDI) for the ROSELT/OSS network. J. Geogr. Inf. Syst. 02, 150–161. doi:10.4236/jgis.2014.62016
Albrecht, T. R., Crootof, A., and Scott, C. A. (2018). The water-energy-food nexus: A systematic review of methods for nexus assessment. Environ. Res. Lett. 13, 043002. doi:10.1088/1748-9326/aaa9c6
Allen, T., and Prosperi, P. (2016). Modeling sustainable food systems. Environ. Manag. 57, 956–975. doi:10.1007/s00267-016-0664-8
Andela, N., Liu, Y., Van Dijk, A., De Jeu, R., and McVicar, T. (2013). Global changes in dryland vegetation dynamics (1988–2008) assessed by satellite remote sensing: Comparing a new passive microwave vegetation density record with reflective greenness data. Biogeosciences 10, 6657–6676. doi:10.5194/bg-10-6657-2013
Andela, N., and van der Werf, G. R. (2014). Recent trends in African fires driven by cropland expansion and El Niño to La Niña transition. Nat. Clim. Chang. 4, 791–795. doi:10.1038/nclimate2313
Anyamba, A., and Tucker, C. J. (2005). Analysis of Sahelian vegetation dynamics using NOAA-AVHRR NDVI data from 1981–2003. J. Arid Environ. 63, 596–614. doi:10.1016/j.jaridenv.2005.03.007
Arndt, C., Hussain, M. A., Salvucci, V., and Østerdal, L. P. (2016). Effects of food price shocks on child malnutrition: The Mozambican experience 2008/2009. Econ. Hum. Biol. 22, 1–13. doi:10.1016/j.ehb.2016.03.003
Atanga, R. A., and Tankpa, V. (2021). Climate change, flood disaster risk and food security nexus in northern Ghana. Front. Sustain. Food Syst. 5. doi:10.3389/fsufs.2021.706721
Ballantyne, A., Smith, W., Anderegg, W., Kauppi, P., Sarmiento, J., Tans, P., et al. (2017). Accelerating net terrestrial carbon uptake during the warming hiatus due to reduced respiration. Nat. Clim. Chang. 7, 148–152. doi:10.1038/nclimate3204
Bathiany, S., Dakos, V., Scheffer, M., and Lenton, T. M. (2018). Climate models predict increasing temperature variability in poor countries. Sci. Adv. 4, eaar5809. eaar5809. doi:10.1126/sciadv.aar5809
Berdugo, M., Delgado-Baquerizo, M., Soliveres, S., Hernández-Clemente, R., Zhao, Y., Gaitán, J. J., et al. (2020). Global ecosystem thresholds driven by aridity. Science 367, 787–790. doi:10.1126/science.aay5958
Berg, A., Findell, K., Lintner, B., Giannini, A., Seneviratne, S. I., van den Hurk, B., et al. (2016). Land–atmosphere feedbacks amplify aridity increase over land under global warming. Nat. Clim. Chang. 6, 869–874. doi:10.1038/nclimate3029
Berg, A., and McColl, K. A. (2021). No projected global drylands expansion under greenhouse warming. Nat. Clim. Chang. 11, 331–337. doi:10.1038/s41558-021-01007-8
Bleischwitz, R., and Miedzinski, M. (2018). “The resource nexus and resource efficiency: What a nexus perspective adds to the story,” in Factor X: Challenges, implementation strategies and examples for a sustainable use of natural resources. Editor H. Lehmann Cham: Springer International Publishing, 199–212.
Blunden, J., and Arndt, D. (2020). A look at 2019: Takeaway points from the state of the climate. Bull. Am. Meteorological Soc. 101, 612–622. doi:10.1175/bams-d-20-0203.1
Bonuedi, I., Kamasa, K., and Opoku, E. E. O. (2020). Enabling trade across borders and food security in Africa. Food Secur. 12, 1121–1140. doi:10.1007/s12571-020-01095-y
Bradford, J. B., Schlaepfer, D. R., Lauenroth, W. K., and Palmquist, K. A. (2020). Robust ecological drought projections for drylands in the 21st century. Glob. Chang. Biol. 26, 3906–3919. doi:10.1111/gcb.15075
Brown, M., Antle, J., Backlund, P., Carr, E., Easterling, W., Walsh, M., et al. (2018). Climate change, global food security, and the US food system. United States: Department of Agriculture (USDA). doi:10.J0862/DC0867
Burrell, A. L., Evans, J. P., and Liu, Y. (2017). Detecting dryland degradation using time series segmentation and residual trend analysis (TSS-RESTREND). Remote Sens. Environ. 197, 43–57. doi:10.1016/j.rse.2017.05.018
Cairns, J. E., and Prasanna, B. M. (2018). Developing and deploying climate-resilient maize varieties in the developing world. Curr. Opin. Plant Biol. 45, 226–230. doi:10.1016/j.pbi.2018.05.004
Campbell, B. M., Vermeulen, S. J., Aggarwal, P. K., Corner-Dolloff, C., Girvetz, E., Loboguerrero, A. M., et al. (2016). Reducing risks to food security from climate change. Glob. Food Secur. 11, 34–43. doi:10.1016/j.gfs.2016.06.002
Cervigni, R., and Morris, M. (2016). Confronting drought in Africa's drylands: Opportunities for enhancing resilience. Washington, DC: The World Bank.
Chimwamurombe, P. M., and Mataranyika, P. N. (2021). Factors influencing dryland agricultural productivity. J. Arid Environ. 189, 104489. doi:10.1016/j.jaridenv.2021.104489
Classen, A. T., Sundqvist, M. K., Henning, J. A., Newman, G. S., Moore, J. A. M., Cregger, M. A., et al. (2015). Direct and indirect effects of climate change on soil microbial and soil microbial-plant interactions: What lies ahead? Ecosphere 6, art130. doi:10.1890/es15-00217.1
Conceição, P., Levine, S., Lipton, M., and Warren-Rodríguez, A. (2016). Toward a food secure future: Ensuring food security for sustainable human development in Sub-Saharan Africa. Food Policy 60, 1–9. doi:10.1016/j.foodpol.2016.02.003
Connolly-Boutin, L., and Smit, B. (2016). Climate change, food security, and livelihoods in sub-Saharan Africa. Reg. Environ. Change 16, 385–399. doi:10.1007/s10113-015-0761-x
Copeland, S. M., Bradford, J. B., Duniway, M. C., and Schuster, R. M. (2017). Potential impacts of overlapping land-use and climate in a sensitive dryland: A case study of the Colorado plateau, USA. Ecosphere 8, e01823. doi:10.1002/ecs2.1823
Daramola, M. T., and Xu, M. (2021). Recent changes in global dryland temperature and precipitation. Int. J. Climatol. 42, 1267–1282. n/a. doi:10.1002/joc.7301
Davies, J., Poulsen, L., Schulte-Herbrüggen, B., Mackinnon, K., Crawhall, N., Henwood, W., et al. (2012). Conserving dryland biodiversity. Nairobi, Kenya: International Union for the Conservation of Nature (IUCN).
Defrance, D., Sultan, B., Castets, M., Famien, A. M., and Baron, C. (2020). Impact of climate change in West Africa on cereal production per capita in 2050. Sustainability 12, 7585. doi:10.3390/su12187585
Deutsch, C. A., Tewksbury, J. J., Tigchelaar, M., Battisti, D. S., Merrill, S. C., Huey, R. B., et al. (2018). Increase in crop losses to insect pests in a warming climate. Science 361, 916–919. doi:10.1126/science.aat3466
Devereux, S., and Edwards, J. (2004). Climate change and food security. IDS Bull.. Institute of Development Studies (IDS) 35 (3), 22–30.
Donohue, R. J., McVicar, T. R., and Roderick, M. L. (2009). Climate-related trends in Australian vegetation cover as inferred from satellite observations, 1981–2006. Glob. Change Biol. 15, 1025–1039. doi:10.1111/j.1365-2486.2008.01746.x
Drimie, S., and McLachlan, M. (2013). Food security in South Africa—First steps toward a transdisciplinary approach. Food Secur. 5, 217–226. doi:10.1007/s12571-013-0241-4
Dubovyk, O. (2017). The role of remote sensing in land degradation assessments: Opportunities and challenges. Eur. J. Remote Sens. 50, 601–613. doi:10.1080/22797254.2017.1378926
Eden, J. M., and Widmann, M. (2014). Downscaling of GCM-simulated precipitation using model Output Statistics. J. Clim. 27, 312–324. doi:10.1175/jcli-d-13-00063.1
Ellis, E. C., Gauthier, N., Klein Goldewijk, K., Bliege Bird, R., Boivin, N., Díaz, S., et al. (2021). People have shaped most of terrestrial nature for at least 12, 000 years. Proc. Natl. Acad. Sci. U. S. A. 118, e2023483118. doi:10.1073/pnas.2023483118
Endo, A., Yamada, M., Miyashita, Y., Sugimoto, R., Ishii, A., Nishijima, J., et al. (2020). Dynamics of water–energy–food nexus methodology, methods, and tools. Curr. Opin. Environ. Sci. Health 13, 46–60. doi:10.1016/j.coesh.2019.10.004
Felix, B., and Romuald, K. S. (2012). Rainfall shocks, food prices vulnerability and food security: Evidence for sub-saharan african countries. Clermont Ferrand, France: Centre d’Etude et de recherche sur le Développement International (CERDI).
Feng, S., and Fu, Q. (2013). Expansion of global drylands under a warming climate. Atmos. Chem. Phys. 13, 10081–10094. doi:10.5194/acp-13-10081-2013
Fensholt, R., and Rasmussen, K. (2011). Analysis of trends in the Sahelian ‘rain-use efficiency’ using GIMMS NDVI, RFE and GPCP rainfall data. Remote Sens. Environ. 115, 438–451. doi:10.1016/j.rse.2010.09.014
Fernández-Ríos, A., Laso, J., Campos, C., Ruiz-Salmón, I., Hoehn, D., Cristóbal, J., et al. (2021). Towards a water-energy-food (WEF) nexus index: A review of nutrient profile models as a fundamental pillar of food and nutrition security. Sci. Total Environ. 789, 147936. doi:10.1016/j.scitotenv.2021.147936
Fischer, G., Shah, M. M., and Van Velthuizen, H. (2002). Climate change and agricultural vulnerability. Vienna: International Institute for Applied Systems Analysis (IIASA).
Foley, A. M. (2010). Uncertainty in regional climate modelling: A review. Prog. Phys. Geogr. Earth Environ. 34, 647–670. doi:10.1177/0309133310375654
Fraser, E. D., Dougill, A. J., Hubacek, K., Quinn, C. H., Sendzimir, J., and Termansen, M. (2011). Assessing vulnerability to climate change in dryland livelihood systems: Conceptual challenges and interdisciplinary solutions. Ecol. Soc. 16, art3. doi:10.5751/es-03402-160303
Fritz, S., See, L., Bayas, J. C. L., Waldner, F., Jacques, D., Becker-Reshef, I., et al. (2019). A comparison of global agricultural monitoring systems and current gaps. Agric. Syst. 168, 258–272. doi:10.1016/j.agsy.2018.05.010
Gray, M., and Kalpers, J. (2005). Ranger based monitoring in the virunga–bwindi region of east-central Africa: A simple data collection tool for park management. Biodivers. Conserv. 14, 2723–2741. doi:10.1007/s10531-005-8406-x
Graziosi, I., Tembo, M., Kuate, J., and Muchugi, A. (2020). Pests and diseases of trees in Africa: A growing continental emergency. Plants, People, Planet 2, 14–28. doi:10.1002/ppp3.31
Greve, P., Roderick, M. L., Ukkola, A. M., and Wada, Y. (2019). The aridity Index under global warming. Environ. Res. Lett. 14, 124006. doi:10.1088/1748-9326/ab5046
Grote, U., Fasse, A., Nguyen, T. T., and Erenstein, O. (2021). Food security and the dynamics of wheat and maize value chains in Africa and asia. Front. Sustain. Food Syst. 4, 617009. doi:10.3389/fsufs.2020.617009
Guilpart, N., Grassini, P., van Wart, J., Yang, H., van Ittersum, M. K., van Bussel, L. G. J., et al. (2017). Rooting for food security in sub-saharan Africa. Environ. Res. Lett. 12, 114036. doi:10.1088/1748-9326/aa9003
Hartley, A., Barger, N., Belnap, J., and Okin, G. S. (2007). Dryland ecosystems, Nutrient cycling in terrestrial ecosystems. Berlin, Heidelberg: Springer-Verlag, 271
Hass, A. L., Kormann, U. G., Tscharntke, T., Clough, Y., Baillod, A. B., Sirami, C., et al. (2018). Landscape configurational heterogeneity by small-scale agriculture, not crop diversity, maintains pollinators and plant reproduction in western Europe. Proc. R. Soc. B 285, 20172242. doi:10.1098/rspb.2017.2242
He, X., Estes, L., Konar, M., Tian, D., Anghileri, D., Baylis, K., et al. (2019). Integrated approaches to understanding and reducing drought impact on food security across scales. Curr. Opin. Environ. Sustain. 40, 43–54. doi:10.1016/j.cosust.2019.09.006
Herrick, J. E., Beh, A., Barrios, E., Bouvier, I., Coetzee, M., Dent, D., et al. (2016). The land-potential knowledge system (LandPKS): Mobile apps and collaboration for optimizing climate change investments. Ecosyst. Health Sustain. 2, e01209. doi:10.1002/ehs2.1209
Hirwa, H., Zhang, Q., Qiao, Y., Peng, Y., Leng, P., Tian, C., et al. (2021). Insights on water and climate change in the greater horn of Africa: Connecting virtual water and water-energy-food-biodiversity-health nexus. Sustainability 13, 6483. doi:10.3390/su13116483
Huang, J., Guan, X., and Ji, F. (2012). Enhanced cold-season warming in semi-arid regions. Atmos. Chem. Phys. 12, 5391–5398. doi:10.5194/acp-12-5391-2012
Huang, J., Li, Y., Fu, C., Chen, F., Fu, Q., Dai, A., et al. (2017). Dryland climate change: Recent progress and challenges. Rev. Geophys. 55, 719–778. doi:10.1002/2016rg000550
Huang, J, J., Ji, M., Xie, Y., Wang, S., He, Y., and Ran, J. (2016). Global semi-arid climate change over last 60 years. Clim. Dyn. 46, 1131–1150. doi:10.1007/s00382-015-2636-8
Huang, J. Y, J. Y., Guan, H., Wang, X., and Guo, G. (2016). Accelerated dryland expansion under climate change. Nat. Clim. Chang. 6, 166–171. doi:10.1038/nclimate2837
Ingram, J. (2011). A food systems approach to researching food security and its interactions with global environmental change. Food Secur. 3, 417–431. doi:10.1007/s12571-011-0149-9
IPCC (2019). Climate change and land. An IPCC Special Report on climate change, desertification, land degradation, sustainable land management, food security, and greenhouse gas fluxes in terrestrial ecosystems. Geneva, Switzerland: Summary for policymakers, WMO, UNEP.
IPCC (2018). “Special Report on the impacts of global warming of 1.5 C above pre-industrial levels and related global greenhouse gas emission pathways the context of strengthening the global response to the threat of climate change, sustainable development, and efforts to eradicate poverty,” in Intergovernmental Panel on climate change.
Jiménez-Muñoz, J. C., Sobrino, J. A., Mattar, C., and Franch, B. (2010). Atmospheric correction of optical imagery from MODIS and Reanalysis atmospheric products. Remote Sens. Environ. 114, 2195–2210. doi:10.1016/j.rse.2010.04.022
Jürgens, N., Schmiedel, U., Haarmeyer, D. H., Dengler, J., Finckh, M., Goetze, D., et al. (2012). The BIOTA biodiversity observatories in Africa—A standardized framework for large-scale environmental monitoring. Environ. Monit. Assess. 184, 655–678. doi:10.1007/s10661-011-1993-y
Kassegn, A., and Endris, E. (2021). Review on socio-economic impacts of ‘triple threats’ of COVID-19, desert locusts, and floods in East Africa: Evidence from Ethiopia. Cogent Soc. Sci. 7, 1885122. doi:10.1080/23311886.2021.1885122
Keenan, T., Prentice, I., Canadell, J., Williams, C., Wang, H., Raupach, M., et al. (2016). Recent pause in the growth rate of atmospheric CO2 due to enhanced terrestrial carbon uptake. Nat. Commun. 7, 13428. doi:10.1038/ncomms13428
Kiage, L. M. (2013). Perspectives on the assumed causes of land degradation in the rangelands of Sub-Saharan Africa. Prog. Phys. Geogr. Earth Environ. 37, 664–684. doi:10.1177/0309133313492543
Klein, C., Bliefernicht, J., Heinzeller, D., Gessner, U., Klein, I., and Kunstmann, H. (2017). Feedback of observed interannual vegetation change: A regional climate model analysis for the West african monsoon. Clim. Dyn. 48, 2837–2858. doi:10.1007/s00382-016-3237-x
Kogan, F., Guo, W., and Yang, W. (2017). SNPP/VIIRS vegetation health to assess 500 California drought. Geomatics, Nat. Hazards Risk 8, 1383–1395. doi:10.1080/19475705.2017.1337654
Kogo, B. K., Kumar, L., and Koech, R. (2021). Climate change and variability in Kenya: A review of impacts on agriculture and food security. Environ. Dev. Sustain. 23, 23–43. doi:10.1007/s10668-020-00589-1
Koohafkan, P., and Stewart, B. A. (2008). Water and cereals in drylands. Cambridge, United Kingdom: Cambridge University Press.
Koutroulis, A. G. (2019). Dryland changes under different levels of global warming. Sci. Total Environ. 655, 482–511. doi:10.1016/j.scitotenv.2018.11.215
Kowalik, W. S., Marsh, S. E., and Lyon, R. J. P. (1982). A relation between landsat digital numbers, surface reflectance, and the cosine of the solar zenith angle. Remote Sens. Environ. 12, 39–55. doi:10.1016/0034-4257(82)90006-2
Kpossilande, C. E., Honfoga, B. G., and Ferre, T. (2020). Economic potentials of artisanal food processing microenterprises in West Africa: Case of “atta” production in cotonou (Benin). Agric. Food Econ. 8, 24. doi:10.1186/s40100-020-00168-y
Kubik, Z., and May, J. (2018). Weather shocks, food prices and food security: Evidence from South Africa. Somerset West, South Africa: Agricultural Economics Association of South Africa (AEASA).
Kuenzer, C., Ottinger, M., Wegmann, M., Guo, H., Wang, C., Zhang, J., et al. (2014). Earth observation satellite sensors for biodiversity monitoring: Potentials and bottlenecks. Int. J. Remote Sens. 35, 6599–6647. doi:10.1080/01431161.2014.964349
Laban, P., Metternicht, G., and Davies, J. (2018). Soil biodiversity and soil organic carbon: Keeping drylands alive. Gland, Switzerland: IUCN.
Leakey, R. R. B. (2018). Converting ‘trade-offs’ to ‘trade-ons’ for greatly enhanced food security in Africa: Multiple environmental, economic and social benefits from ‘socially modified crops. Food Secur. 10, 505–524. doi:10.1007/s12571-018-0796-1
Leisner, C. P. (2020). Review: Climate change impacts on food security- focus on perennial cropping systems and nutritional value. Plant Sci. 293, 110412. doi:10.1016/j.plantsci.2020.110412
Levy, K., Woster, A. P., Goldstein, R. S., and Carlton, E. J. (2016). Untangling the impacts of climate change on waterborne diseases: A systematic review of relationships between diarrheal diseases and temperature, rainfall, flooding, and drought. Environ. Sci. Technol. 50, 4905–4922. doi:10.1021/acs.est.5b06186
Li, B., Parr, T., and Rozzi, R. (2015). “Geographical and thematic distribution of publications generated at the international long-term ecological research network (ILTER) sites,” in Earth stewardship: Linking ecology and ethics in theory and practice. Editors R. Rozzi, F. S. ChapinIii, J. B. Callicott, S. T. A. Pickett, M. E. Power, J. J. Armestoet al. (Cham Springer International Publishing), 195–216.
Li, H., and Zhang, X. (2017). A spatial explicit assessment of food security in Africa based on simulated crop production and distribution. J. Clean. Prod. 147, 628–636. doi:10.1016/j.jclepro.2017.01.124
Li, Y., Chen, Y., and Li, Z. (2019). Dry/wet pattern changes in global dryland areas over the past six decades. Glob. Planet. Change 178, 184–192. doi:10.1016/j.gloplacha.2019.04.017
Lian, X., Piao, S., Chen, A., Huntingford, C., Fu, B., Li, L. Z., et al. (2021). Multifaceted characteristics of dryland aridity changes in a warming world. Nat. Rev. Earth Environ. 2, 232–250. doi:10.1038/s43017-021-00144-0
Maestre, F. T., Salguero-Gómez, R., and Quero, J. L. (2012). It is getting hotter in here: Determining and projecting the impacts of global environmental change on drylands. Phil. Trans. R. Soc. B 367, 3062–3075. doi:10.1098/rstb.2011.0323
Malagó, A., Comero, S., Bouraoui, F., Kazezyılmaz-Alhan, C. M., Gawlik, B. M., Easton, P., et al. (2021). An analytical framework to assess SDG targets within the context of WEFE nexus in the Mediterranean region. Resour. Conservation Recycl. 164, 105205. doi:10.1016/j.resconrec.2020.105205
Matz, J. A., Kalkuhl, M., and Abegaz, G. A. (2015). The short-term impact of price shocks on food security-Evidence from urban and rural Ethiopia. Food Secur. 7, 657–679. doi:10.1007/s12571-015-0467-4
Mbow, C., Rosenzweig, C., Barioni, L. G., Benton, T. G., Herrero, M., Krishnapillai, M., et al. (2019). Food security, climate change and land: An IPCC special report on climate change, desertification, land degradation, sustainable land management, food security and greenhouse gas fluxes in terrestrial ecosystems. Geneva, Switzerland: IPCC.
Mbow, C., Van Noordwijk, M., Luedeling, E., Neufeldt, H., Minang, P. A., and Kowero, G. (2014). Agroforestry solutions to address food security and climate change challenges in Africa. Curr. Opin. Environ. Sustain. 6, 61–67. doi:10.1016/j.cosust.2013.10.014
McCarthy, J. J., Canziani, O. F., Leary, N. A., Dokken, D. J., and White, K. S. (2001). Climate change 2001: Impacts, adaptation, and vulnerability: Contribution of working group II to the third assessment report of the intergovernmental Panel on climate change. Cambridge, United Kingdom: Cambridge University Press.
Middleton, N., and Thomas, D. (1997). World atlas of desertification. Oxford, United Kingdom: Hodder Headline, PLC Arnold.
Mirtl, M., Borer, T. E., Djukic, I., Forsius, M., Haubold, H., Hugo, W., et al. (2018). Genesis, goals and achievements of long-term ecological research at the global scale: A critical review of ILTER and future directions. Sci. Total Environ. 626, 1439–1462. doi:10.1016/j.scitotenv.2017.12.001
Mirzabaev, A., and Tsegai, D. W. (2012). Effects of weather shocks on agricultural commodity prices in Central Asia. Bonn, Germany: ZEF-Discussion Papers on Development Policy.
Mirzaei, A., Abdeshahi, A., Azarm, H., and Naghavi, S. (2021). New design of water-energy-food-environment nexus for sustainable agricultural management. Stoch. Environ. Res. Risk Assess. 35, 1–14.
Moore, N., Alagarswamy, G., Pijanowski, B., Thornton, P., Lofgren, B., Olson, J., et al. (2012). East African food security as influenced by future climate change and land use change at local to regional scales. Clim. change 110, 823–844. doi:10.1007/s10584-011-0116-7
Mortimore, M., Anderson, S., Cotula, L., Davies, J., Faccer, K., Hesse, C., et al. (2009). Dryland opportunies: A new paradigm for people, ecosystems and development. London, United Kingdom: International Union for Conservation of Nature IUCN.
Myers, S. S., Smith, M. R., Guth, S., Golden, C. D., Vaitla, B., Mueller, N. D., et al. (2017). Climate change and global food systems: Potential impacts on food security and undernutrition. Annu. Rev. Public Health 38, 259–277. doi:10.1146/annurev-publhealth-031816-044356
Niles, M. T., and Brown, M. E. (2017). A multi-country assessment of factors related to smallholder food security in varying rainfall conditions. Sci. Rep. 7, 16277. doi:10.1038/s41598-017-16282-9
Nyberg, G., Mureithi, S. M., Muricho, D. N., and Ostwald, M. (2019). Enclosures as a land management tool for food security in African drylands. J. Land Use Sci. 14, 110–121. doi:10.1080/1747423x.2019.1636147
O’Grady, Siobhán (2021). The coronavirus intensified a hunger crisis last year, but 2021 could be worse,” the Washington post. Washington, DC: The Washington Post.
Ohana-Levi, N., Paz-Kagan, T., Panov, N., Peeters, A., Tsoar, A., and Karnieli, A. (2019). Time series analysis of vegetation-cover response to environmental factors and residential development in a dryland region. GIScience Remote Sens. 56, 362–387. doi:10.1080/15481603.2018.1519093
Overpeck, J. T., and Udall, B. (2020). Climate change and the aridification of North America. Proc. Natl. Acad. Sci. U. S. A. 117, 11856–11858. doi:10.1073/pnas.2006323117
Overpeck, J., and Udall, B. (2010). Dry times ahead. Science 328, 1642–1643. doi:10.1126/science.1186591
Owusu, K., Obour, P. B., Oppong, R., and Boadi, S. A. (2020). Climate change impacts on staple root and tuber crops production: Implications for smallholder farmers' livelihoods in rural Ghana. Int. J. Sustain. Soc. 12, 253–265. doi:10.1504/ijssoc.2020.109771
Pahl-Wostl, C. (2019). Governance of the water-energy-food security nexus: A multi-level coordination challenge. Environ. Sci. Policy 92, 356–367. doi:10.1016/j.envsci.2017.07.017
Peng, Y., Hirwa, H., Zhang, Q., Wang, G., and Li, F. (2021). Dryland food security in Ethiopia: Current status, opportunities, and a roadmap for the future. Sustainability 13, 6503. doi:10.3390/su13116503
Pereira, L. M. (2013). The future of the food system: Cases involving the private sector in South Africa. Sustainability 5, 1234–1255. doi:10.3390/su5031234
Perianes-Rodriguez, A., Waltman, L., and van Eck, N. J. (2016). Constructing bibliometric networks: A comparison between full and fractional counting. J. Inf. 10, 1178–1195. doi:10.1016/j.joi.2016.10.006
Pernechele, V., Balié, J., and Ghins, L. (2018). Monitoring and analysing food and agricultural policies (MAFAP) synthesis study.Agricultural policy incentives in sub-Saharan Africa in the last decade (2005–2016)
Pingali, P. L. (2012). Green revolution: Impacts, limits, and the path ahead. Proc. Natl. Acad. Sci. U. S. A. 109, 12302–12308. doi:10.1073/pnas.0912953109
Plaza, C., Zaccone, C., Sawicka, K., Méndez, A. M., Tarquis, A., Gascó, G., et al. (2018). Soil resources and element stocks in drylands to face global issues. Sci. Rep. 8, 13788. doi:10.1038/s41598-018-32229-0
Porter, J. R., Xie, L., Challinor, A. J., Cochrane, K., Howden, S. M., Iqbal, M. M., et al. (2014). Food security and food production systems. Cambridge, United Kingdom and New York, NY, United States: Cambridge University Press.
Put, M., Verhagen, J., Veldhuizen, E., and Jellema, P. (2004). “Climate change in dryland West Africa?,” in The impact of climate change on drylands: With a focus on West Africa. Editors A. J. Dietz, R. Ruben, and A. Verhagen (Dordrecht: Springer Netherlands), 27
Randall, D. A., Wood, R. A., Bony, S., Colman, R., Fichefet, T., Fyfe, J., et al. (2007). “Climate models and their evaluation, Climate change 2007: The physical science basis,” in Contribution of working group I to the fourth assessment report of the IPCC (FAR) (Cambridge University Press), 589
Reardon, T., Tschirley, D., Liverpool-Tasie, L. S. O., Awokuse, T., Fanzo, J., Minten, B., et al. (2021). The processed food revolution in African food systems and the double burden of malnutrition. Glob. Food Secur. 28, 100466. doi:10.1016/j.gfs.2020.100466
Reynolds, J. F., Smith, D. M. S., Lambin, E. F., Turner, B. L., Mortimore, M., Batterbury, S. P. J., et al. (2007). Global desertification: Building a science for dryland development. Science 316, 847–851. doi:10.1126/science.1131634
Richardson, A. D., Hufkens, K., Milliman, T., Aubrecht, D. M., Chen, M., Gray, J. M., et al. (2018). Tracking vegetation phenology across diverse North American biomes using PhenoCam imagery. Sci. Data 5, 180028–180124. doi:10.1038/sdata.2018.28
Richardson, A. D., Keenan, T. F., Migliavacca, M., Ryu, Y., Sonnentag, O., and Toomey, M. (2013). Climate change, phenology, and phenological control of vegetation feedbacks to the climate system. Agric. For. Meteorology 169, 156–173. doi:10.1016/j.agrformet.2012.09.012
Robertson, A. D., Zhang, Y., Sherrod, L. A., Rosenzweig, S. T., Ma, L., Ahuja, L., et al. (2018). Climate change impacts on yields and soil carbon in row crop dryland agriculture. J. Environ. Qual. 47, 684–694. doi:10.2134/jeq2017.08.0309
Rosegrant, M. W., Koo, J., Cenacchi, N., Ringler, C., Robertson, R. D., Fisher, M., et al. (2014). Food security in a world of natural resource scarcity: The role of agricultural technologies. Washington, DC: Intl Food Policy Res Inst.
Rouse, J. W., Haas, R. H., Schell, J. A., Deering, D. W., and Harlan, J. C. (1974). Monitoring the vernal advancement and retrogradation (green wave effect) of natural vegetation. Greenbelt: NASA/GSFC Type III Final Report, 371
Rovero, F., and Ahumada, J. (2017). The Tropical Ecology, Assessment and Monitoring (TEAM) Network: An early warning system for tropical rain forests. Sci. Total Environ. 574, 914–923. doi:10.1016/j.scitotenv.2016.09.146
Ruppert, J. C., Harmoney, K., Henkin, Z., Snyman, H. A., Sternberg, M., Willms, W., et al. (2015). Quantifying drylands' drought resistance and recovery: The importance of drought intensity, dominant life history and grazing regime. Glob. Change Biol. 21, 1258–1270. doi:10.1111/gcb.12777
SAEON/NRF (2018). SAEON annual report 2016/17. South african environmental observation network (SAEON), national research foundation (NRF) and republic of South Africa. South Africa: National Research Foundation.
Safriel, U. (2017). Land Degradation Neutrality (LDN) in drylands and beyond–where has it come from and where does it go. Silva Fenn. Hels. 51, 20–24. doi:10.14214/sf.1650
Saha, M. V., Scanlon, T. M., and D'Odorico, P. (2016). Suppression of rainfall by fires in African drylands. Geophys. Res. Lett. 43, 8527–8533. doi:10.1002/2016gl069855
Salih, A. A. M., Baraibar, M., Mwangi, K. K., and Artan, G. (2020). Climate change and locust outbreak in East Africa. Nat. Clim. Chang. 10, 584–585. doi:10.1038/s41558-020-0835-8
Samuel, O. O., Sylvia, T. S., and Ricart Casadevall, S. (2019). Establishing the nexus between climate change adaptation strategy and smallholder farmers’ food security status in South Africa: A bi-casual effect using instrumental variable approach. Cogent Soc. Sci. 5, 1656402. doi:10.1080/23311886.2019.1656402
Sanz, M., De Vente, J., Chotte, J.-L., Bernoux, M., Kust, G., Ruiz, I., Almagro, M., Alloza, J.-A., Vallejo, R., and Castillo, V. (2017). Sustainable Land Management contribution to successful land-based climate change adaptation and mitigation.” in A report of the science-policy interface. Bonn, Germany: United Nations Convention to Combat Desertification United Nations.
Scheelbeek, P. F. D., Bird, F. A., Tuomisto, H. L., Green, R., Harris, F. B., Joy, E. J. M., et al. (2018). Effect of environmental changes on vegetable and legume yields and nutritional quality. Proc. Natl. Acad. Sci. U. S. A. 115, 6804–6809. doi:10.1073/pnas.1800442115
Schlaepfer, D. R., Bradford, J. B., Lauenroth, W. K., Munson, S. M., Tietjen, B., Hall, S. A., et al. (2017). Climate change reduces extent of temperate drylands and intensifies drought in deep soils. Nat. Commun. 8, 14196. doi:10.1038/ncomms14196
Schmidhuber, J., and Tubiello, F. N. (2007). Global food security under climate change. Proc. Natl. Acad. Sci. U. S. A. 104, 19703–19708. doi:10.1073/pnas.0701976104
Scholes, M., Scholes, R. B., and Lucas, M. (2015). Climate change: Briefings from southern Africa. Johannesburg, South Africa: Witwatersrand University Press.
Schouten, G., Vink, M., and Vellema, S. (2018). Institutional diagnostics for African food security: Approaches, methods and implications. NJAS Wageningen J. Life Sci. 84, 1–5. doi:10.1016/j.njas.2017.11.002
Seyednasrollah, B., Young, A. M., Hufkens, K., Milliman, T., Friedl, M. A., Frolking, S., et al. (2019). Tracking vegetation phenology across diverse biomes using Version 2.0 of the PhenoCam Dataset. Sci. Data 6, 222. doi:10.1038/s41597-019-0229-9
Shiferaw, B., Smale, M., Braun, H.-J., Duveiller, E., Reynolds, M., and Muricho, G. (2013). Crops that feed the world 10. Past successes and future challenges to the role played by wheat in global food security. Food Secur. 5, 291–317. doi:10.1007/s12571-013-0263-y
Smit, W. (2016). Urban governance and urban food systems in Africa: Examining the linkages. Cities 58, 80–86. doi:10.1016/j.cities.2016.05.001
Smith, D. M. S., Abel, N., Walker, B., and Chapin, F. S. (2009). Drylands: Coping with uncertainty, thresholds, and changes in state, principles of ecosystem stewardship. New York, NY, United States: Springer Science+Business Media, 171.
Smith, W. K., Dannenberg, M. P., Yan, D., Herrmann, S., Barnes, M. L., Barron-Gafford, G. A., et al. (2019). Remote sensing of dryland ecosystem structure and function: Progress, challenges, and opportunities. Remote Sens. Environ. 233, 111401. doi:10.1016/j.rse.2019.111401
Stephens, C., Lall, U., Johnson, F., and Marshall, L. (2020). Landscape changes and their hydrologic effects: Interactions and feedbacks across scales. Earth-Science Rev. 212, 103466. doi:10.1016/j.earscirev.2020.103466
Stringer, L. C., Dougill, A. J., Thomas, A. D., Spracklen, D., Chesterman, S., Speranza, C. I., et al. (2012). Challenges and opportunities in linking carbon sequestration, livelihoods and ecosystem service provision in drylands. Environ. Sci. policy 19, 121–135. doi:10.1016/j.envsci.2012.02.004
Stuch, B., Alcamo, J., and Schaldach, R. (2021). Projected climate change impacts on mean and year-to-year variability of yield of key smallholder crops in Sub-Saharan Africa. Clim. Dev. 13, 268–282. doi:10.1080/17565529.2020.1760771
Tachiiri, K., Su, X., and Matsumoto, K. i. (2021). Identifying key processes and sectors in the interaction between climate and socio-economic systems: A review toward integrating earth–human systems. Prog. Earth Planet. Sci. 8, 24. doi:10.1186/s40645-021-00418-7
Tarek, M., Brissette, F., and Arsenault, R. (2021). Uncertainty of gridded precipitation and temperature reference datasets in climate change impact studies. Hydrol. Earth Syst. Sci. 25, 3331–3350. doi:10.5194/hess-25-3331-2021
TEAM Network (2011). TEAM network sampling design guidelines. Arlington, VA, United States: Tropical Ecology, Assessment and Monitoring Network, Science and Knowledge Division, Conservation International.
Tian, F., Brandt, M., Liu, Y. Y., Verger, A., Tagesson, T., Diouf, A. A., et al. (2016). Remote sensing of vegetation dynamics in drylands: Evaluating vegetation optical depth (VOD) using AVHRR NDVI and in situ green biomass data over West African Sahel. Remote Sens. Environ. 177, 265–276. doi:10.1016/j.rse.2016.02.056
Trabucco, A., and Zomer, R. J. (2018). Global aridity index and potential evapotranspiration (ET0) climate database v2. Montpellier, France: CGIAR Consort Spat Inf.
Trenberth, K. E., Dai, A., van der Schrier, G., Jones, P. D., Barichivich, J., Briffa, K. R., et al. (2014). Global warming and changes in drought. Nat. Clim. Chang. 4, 17–22. doi:10.1038/nclimate2067
Tsusaka, T. W., and Otsuka, K. (2013). The changes in the effects of temperature and rainfall on cereal crop yields in sub-saharan Africa: A country level panel data study, 1989 to 2004. Environ. Econ. 4 (2), 70.
Ukhurebor, K. E., Singh, K. R. B., Nayak, V., and Uk-Eghonghon, G. (2021). Influence of the SARS-CoV-2 pandemic: A review from the climate change perspective. Environ. Sci. Process. Impacts 23, 1060–1078. doi:10.1039/d1em00154j
Unep-Wcmc, (2011). Global drylands: A UN system-wide response. Nairobi, Kenya: United Nations Environment Management Group.
Vanderbilt, K., and Gaiser, E. (2017). The international long term ecological research network: A platform for collaboration. Ecosphere 8, e01697. doi:10.1002/ecs2.1697
Verstraete, M., Stafford Smith, M., and Scholes, R. (2009). “Designing an integrated global monitoring system for drylands,” in Proceedings, 33rd International Symposium on Remote Sensing of Environment (Stresa, Italy: ISRSE), 898–901.
Vilakazi, N., Nyirenda, K., and Vellemu, E. (2019). Unlocking water issues towards food security in Africa, food security in Africa. London, United Kingdom: IntechOpen.
Vinca, A., Riahi, K., Rowe, A., and Djilali, N. (2021). Climate-land-energy-water nexus models across scales: Progress, gaps and best accessibility practices. Front. Environ. Sci. 9, 691523. doi:10.3389/fenvs.2021.691523
Wang, L., D'Odorico, P., Evans, J. P., Eldridge, D. J., McCabe, M. F., Caylor, K. K., et al. (2012). Dryland ecohydrology and climate change: Critical issues and technical advances. Hydrol. Earth Syst. Sci. 16, 2585–2603. doi:10.5194/hess-16-2585-2012
Wang, L., Manzoni, S., Ravi, S., Riveros-Iregui, D., and Caylor, K. (2015). Dynamic interactions of ecohydrological and biogeochemical processes in water-limited systems. Ecosphere 6, art133. doi:10.1890/es15-00122.1
Webb, N. P., Marshall, N. A., Stringer, L. C., Reed, M. S., Chappell, A., and Herrick, J. E. (2017). Land degradation and climate change: Building climate resilience in agriculture. Front. Ecol. Environ. 15, 450–459. doi:10.1002/fee.1530
Wei, F., Wang, S., Brandt, M., Fu, B., Meadows, M. E., Wang, L., et al. (2021). Responses and feedbacks of African dryland ecosystems to environmental changes. Curr. Opin. Environ. Sustain. 48, 29–35. doi:10.1016/j.cosust.2020.09.004
Wei, F., Wang, S., Fu, B., Brandt, M., Pan, N., Wang, C., et al. (2020). Nonlinear dynamics of fires in Africa over recent decades controlled by precipitation. Glob. Change Biol. 26, 4495–4505. doi:10.1111/gcb.15190
Wei, M., Li, H., Akram, M. A., Dong, L., Sun, Y., Hu, W., et al. (2022). Quantifying drought resistance of drylands in northern China from 1982 to 2015: Regional disparity in drought resistance. Forests 13, 100. doi:10.3390/f13010100
WFP, 2019. Africa hunger, famine: Facts, FAQs, and how to help?. Rome, Italy: World Vision International.
WFP&FAO (2020). FAO-WFP early warning analysis of acute food insecurity hotspots. Washington, DC: World Food Programme.
Wheeler, T., and von Braun, J. (2013). Climate change impacts on global food security. Science 341, 508–513. doi:10.1126/science.1239402
WMO (2020). State of the climate in Africa 2019. Geneva, Switzerland: World Meteorological Organization.
Woldai, T. (2020). The status of earth observation (EO) & geo-information sciences in Africa – trends and challenges. Geo-spatial Inf. Sci. 23, 107–123. doi:10.1080/10095020.2020.1730711
Yadav, K., and Congalton, R. G. (2018). Accuracy assessment of global food security-support analysis data (GFSAD) cropland extent maps produced at three different spatial resolutions. Remote Sens. 10, 1800. doi:10.3390/rs10111800
Yevide, A. S. I., Wu, B., Khan, A. S., Zeng, Y., and Liu, J. (2016). Bibliometric analysis of ecosystem monitoring-related research in Africa: Implications for ecological stewardship and scientific collaboration. Int. J. Sustain. Dev. World Ecol. 23, 412–422. doi:10.1080/13504509.2015.1129998
Yevide, A. S. I., Wu, B., Yu, X., Li, X., Liu, Y., and Liu, J. (2015). Building African Ecosystem Research Network for sustaining local ecosystem goods and services. Chin. Geogr. Sci. 25, 414–425. doi:10.1007/s11769-015-0767-9
Keywords: Africa, aridity index, CDF nexus, drylands, observation networks, sustainability
Citation: Hirwa H, Li F, Qiao Y, Measho S, Muhirwa F, Tian C, Leng P, Ingabire R, Itangishaka AC, Chen G and Turyasingura B (2022) Climate change–drylands–food security nexus in Africa: From the perspective of technical advances, challenges, and opportunities. Front. Environ. Sci. 10:851249. doi: 10.3389/fenvs.2022.851249
Received: 09 January 2022; Accepted: 08 August 2022;
Published: 06 September 2022.
Edited by:
Shuai Wang, Case Western Reserve University, United StatesReviewed by:
Kingsley Eghonghon Ukhurebor, Edo University, NigeriaChristian Baron, Institut National de la Recherche Agronomique (INRA), France
Athanase Nduwumuremyi, Rwanda Agriculture and Animal Resources Development Board, Rwanda
Copyright © 2022 Hirwa, Li, Qiao, Measho, Muhirwa, Tian, Leng, Ingabire, Itangishaka, Chen and Turyasingura. This is an open-access article distributed under the terms of the Creative Commons Attribution License (CC BY). The use, distribution or reproduction in other forums is permitted, provided the original author(s) and the copyright owner(s) are credited and that the original publication in this journal is cited, in accordance with accepted academic practice. No use, distribution or reproduction is permitted which does not comply with these terms.
*Correspondence: Fadong Li, lifadong@igsnrr.ac.cn; Hubert Hirwa, hhirwa2019@igsnrr.ac.cn