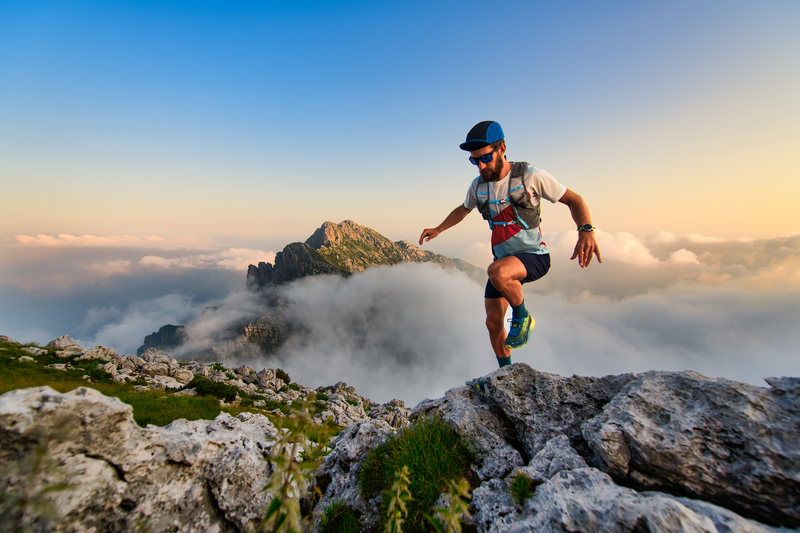
95% of researchers rate our articles as excellent or good
Learn more about the work of our research integrity team to safeguard the quality of each article we publish.
Find out more
SYSTEMATIC REVIEW article
Front. Environ. Sci. , 31 March 2022
Sec. Environmental Economics and Management
Volume 10 - 2022 | https://doi.org/10.3389/fenvs.2022.846742
The new energy demonstration city policy is a significant pilot measure to promote the transition of China’s energy system, aiming at developing new, green, and low-carbon sources of energy. In this paper, the Non-radial Directional Distance Function (NDDF) was adopted to calculate the Energy-Carbon Performance Index (ECPI) of Chinese 182 cities, for measuring the Energy-Carbon Performance (ECP) level of each city. On this basis, it is possible to empirically analyse the impact that the policy orientation of constructing new energy demonstration cities has had on urban energy carbon performance by using a combination of Propensity Score Matching and Difference-in-Difference. Moreover, a mediating effect model is utilised to test the mediating effect of technological innovation. The results show that the new energy demonstration city policy can significantly improve the ECP. Technological innovation has a partial mediating effect between the policy orientation of new energy demonstration city construction and ECP, which accounts for 12.92% of the total effect. Optimising the industrial structure, improving the level of economic development, increasing carbon sink resources, and attracting foreign direct investment all have significant impacts on the improvement of China’s ECP, while the urbanisation process has an inhibitory effect on the improvement of ECP. Heterogeneity analysis shows that policy orientation has a better driving effect on eastern cities and western cities in promoting the improvement of ECP. The policy implications of this paper are that 1) The government should expand the scope of new energy city pilots in an orderly manner; 2) The lasting and long-term influence of policy orientation on ECP should make use of technological innovation intermediary channels; 3) Support policies are supposed to formulate according to local conditions.
At present, the earth is facing difficult environmental problems such as climate change and global warming, and the burning of fossil fuels and CO2 emissions are the main causes of environmental deterioration (Anser et al., 2020). Renewable energy technology innovation and renewable energy consumption can promote sustainable development of the global environment by reducing fossil fuel combustion and greenhouse gas emissions (Kirikkaleli and Adebayo, 2020; Shan et al., 2021). Energy security and climate change issues have urged governments to give priority to the development of new energy sources and actively implement reform energy policies. Since 2009, China’s government has implemented a number of new energy policies and measures to guide the healthy and rapid development of the new energy industry and promote the transition of the energy system through innovative mechanisms and policy subsidies. In 2020, China’s renewable energy consumption reached the equivalent of 266 million tons of standard coal, an increase of 15.41% over 2019, accounting for 5.36% of the total primary energy consumption and a rise of 0.61% over 2019 (BP, 2021). The high-quality growth of the new energy industry has enhanced China’s energy supply capacity and effectively alleviated energy shortage and solved energy security problems. Meanwhile, the vigorous development of new energy has replaced some traditional fossil energy, which can reduce CO2 emissions and pressure on the ecological environment. Finally, it can also improve energy-carbon performance.
In the background of “carbon peak and carbon neutrality” (also called dual-carbon target), ECP has been accepted by more and more countries as a tool for energy policy governance (Malinauskaite et al., 2017). Through the implementation of public policy, the government can effectively guide society towards green, high-quality and low-carbon development, especially in reducing carbon dioxide emissions and improving energy efficiency. Although policy does not directly increase production resources, it can optimise efficiency, speed, and direction of social production by changing the market price and the configuration environment of production factors. The government is the macro regulator of social production behaviour. It uses the policy-oriented effect and its policies and regulations to regulate social production behaviour (Song et al., 2021). Policy orientation means that governments can regulate the production behaviour of market economy and make social production develop in an expected direction. Governments adopt policies and requirements to restrict and regulate production behaviour. The policy orientation focused on in this article is defined as the behavioural principle that social producers and operators adapt to the new energy demonstration city policy and adjust their social activities according to policy changes to ensure social benefits.
In accordance with the requirements of the 12th Five-Year Plan for renewable energy development, the Chinese government has established the policy orientation for the pilot projects of new energy demonstration cities. In January 2014, the National Energy Administration announced the list of the first batch of new energy demonstration cities, including Changping District of Beijing, Zhengzhou, Datong and other 81 cities (districts). In November 2016, the second batch of new energy demonstration cities, was announced Xigaze city, Dunhuang City and Yangzhong City. The new energy demonstration city policy aims to improve energy efficiency, optimise energy structure, reduce carbon dioxide emissions by reducing dependence on fossil energy and increasing utilisation of renewable resources such as solar energy and geothermal energy.
During the construction of new energy demonstration cities, technological innovation, as the key to innovation-driven green and low-carbon development, is the core factor to achieve high-quality economic development (Khan et al., 2020). In addition to the transition and upgrading of energy structures, a consensus has been reached to achieve the dual-carbon target by relying on technological innovation. Because China’s economic development has the typical characteristics of “government leading,” government behaviour is bound to have a great impact on the economy and society. Technological innovation and new energy development have strong externalities, which are not only the result of spontaneous choice of the market, but also the result led by the government. The discussion of government behaviour cannot be separated from the background of China’s policy orientation, which not only has a significant impact on technological innovation, but also is an important factor affecting urban ECP. At present, when studying the policy impact of new energy demonstration cities, no scholar has explored new energy demonstration city construction policy-oriented effect from the perspective of ECP. It is also essential to identify the role of urban technological innovation in the impact of new energy demonstration city policies on urban ECP.
To sum up the above arguments, this paper’s contribution to existing literature is twofold. Firstly, it is the first time to explore the impact of policy orientation for the construction of new energy demonstration cities on the city’s ECP. In the evaluation process, not only is the ECP index (ECPI) of 182 prefecture cities in China used for measuring the ECP level of each city, which is calculated by the non-radial directional distance function (NDDF), but also the impact effect and robustness test of the new energy demonstration city policy on ECP are analysed by Propensity Score Matching (PSM) and Difference-in-Difference (DID) methods, in order to overcome the endogenous problems and selection bias. It provides a factual reference for gradually promoting the pilot construction of new energy demonstration cities and improving the performance of urban ECP. Secondly, it establishes a mediating effect model to explore the impact mechanism of new energy demonstration city policy on ECP improvement from the perspective of urban technological innovation, so as to provide reference for further optimising the construction path of new energy demonstration cities.
The structure of the paper is as follows. Literature review and research assumptions are described in Section 2. Section 3 introduces the measurement model and variable selection. Section 4 shows and analyses the empirical results. Section 5 verifies the mediating effect of technological innovation. Section 6 explores the heterogeneity of policy effects across regions. Section 7 summarises the main conclusions and policy recommendations.
Energy-carbon (energy and carbon dioxide emission) performance is the production relationship ratio of minimum energy consumption, carbon dioxide emission, and maximum economic output without increasing labor and capital input (Zhu and Lin, 2021). It is calculated by combining concurrent energy consumption and CO2 emissions (Chen and Lin, 2020). By studying existing research, it is found that the literature on ECP mainly involves three research perspectives. First, ECP is measured by different theoretical models. Zhou et al. (2012) proposed a NDDF model to measure the ECP of power generation in 126 countries. Related scholars used this model to measure the ECP of China’s construction sectors (Chen et al., 2019), manufacturing sectors (Lin and Chen, 2020), mining sectors (Zhu and Lin, 2021), agricultural sectors (Fei and Lin, 2017), and commercial sectors (Wang and Lin, 2018). Wang et al. (2013) used a range-adjusted model (RAM) to assess China’s provincial energy and carbon performance. Ding et al. (2020) proposed a constrained performance index model (CPIM) to measure the ECP of 30 provinces and cities in China. Wang et al. (2017) assessed the ECP of 18 APEC’s countries by constructing non-parametric global production technology exhibiting variable returns to scale and a non-radial directional distance function model. Choi et al. (2012) used the SBM-DEA method to estimate the efficiency and abatement costs of energy-related CO2 emissions in China. Iftikhar et al. (2018) used the network DEA (NDEA) method to estimate the ECP of the world’s 19 major economies. Second, the impact mechanism of energy-carbon performance is explored from different perspectives. Chen and Lin (2020) analysed the static and dynamic ECP of China’s non-ferrous metal industry based on NDDF model, and found that technological innovation is the most significant factor driving ECP. Lin and Wu (2020) measured the ECP of China’s iron and steel industry based on NDDF. Their empirical results show that the driving factors to improve ECP are technical efficiency and technological progress. Lin and Zhou (2021a) found that internet development through industrial structure upgrading and technology diffusion, improves energy and carbon emission performances. Salehi et al. (2021) used the method of Life Cycle Energy Analysis (LCEA) to evaluate the energy and carbon efficiency of six luxury hotels in Iran. The study found that promoting renewable energy technology is an important way to achieve energy and carbon efficiency. Zhang et al. (2013) proposed a meta-frontier non-radial directional distance function (MNDDF) to measure ECP in the context of power generation in Korea, and found that ECP can be improved by promoting technological innovation. Third, the impacts of relevant policies on ECP are studied. Chen and Lin (2021) measured the energy-carbon performance index of 26 provinces in China by constructing NDDF. Their results showed that a carbon emission trading scheme can significantly improve ECP. Wu and Lin (2022) found that the intensity of environmental regulation inhibits the improvement of energy-environmental performance, and environmental regulation affects the industrial energy-environmental performance through technological innovation. Lee (2021) used the NDDF-based Meta frontier Malmquist–Luenberger (MML) index model to measure the CO2 emission performance of the Korean manufacturing industry. The results show that the emissions trading scheme (ETS) regulatory policy can effectively promote the improvement of CO2 emission performance, but it cannot show that it is the best path to achieve a carbon neutral economy. Iftikhar et al. (2016) used the SBM-DEA method to estimate the ECP of 26 major economies in the world, and the study showed that economies that implement carbon tax policies can improve their ECP.
The development and utilisation of new energy has positive externalities and public characteristics. Affected by capital, technology, and other factors, market subjects have little initiative in the development and utilisation of new energy. New energy belongs to the market failure field in many aspects and needs the macro-control of government policies. As a method of government macro-control, new energy demonstration cities have played a significant role in the development and utilisation of new energy and technological innovation. It can effectively alleviate the problem of urban energy shortage and control fossil energy consumption and carbon emission (Dingbang et al., 2021). Yang X. et al. (2021) found that the new energy demonstration city policy can significantly decrease environmental pollution through the technological innovation effect, the structure effect, and the resource allocation effect. Among them, one of the strongest effects is the technological innovation effect, followed by the resource allocation effect and the industrial structure effect. Based on the research of new energy demonstration city construction, Wu et al. (2018) found that local governments need to promote the implementation of national energy transition policies in energy transition.
Through the review of existing literature, most use NDDF to measure ECP, which lays an important theoretical and methodological foundation for the study of ECP in this paper. At the same time, relevant researchers studied the policy benefits brought by the new energy demonstration city policy from the perspective of economic development and environmental pollution. Existing research did not explore the policy-oriented effect and influence mechanism of the construction of new energy demonstration cities from the perspective of ECP. In view of that, this study takes the new energy demonstration city policy as research object to measure ECP based on the NDDF. Additionally, the impact of the new energy demonstration city policy on the ECP and its path relationship were empirically tested.
Policy orientation is essential to realise the green and low-carbon development of energy economy. As a comprehensive policy orientation, the pilot construction of new energy demonstration cities can realise the high-quality development of a new energy industry driven by these preferential policies and target requirements. New energy demonstration cities, through new energy industry development planning, set a new energy capacity target and encourage green and low carbon technology development, setting up a national research centre for new energy technology and application, so as to advancing the urban energy structure optimisation and urban industrial transition and upgrading (Wu et al., 2018). It can also accelerate the replacement of traditional fossil energy and reduce carbon emissions. Finally, it would indirectly promote ECP improvement. The government’s supporting policy direction can encourage the market to adjust its own production behaviour and strengthen the R&D and application of low-carbon technologies such as wind power, photovoltaic power generation, and electric vehicles. It can effectively reduce the fossil energy consumption in social production and operation, obtain policy subsidies, and improve its own income. Meanwhile, under the target constraint of new energy consumption, new energy demonstration cities restrict the energy consumption of market subjects to promote the improvement of ECP by eliminating high energy consuming industries and enterprises, increasing investment in new energy technology innovation, and improving the market access threshold. Accordingly, this paper puts forward hypothesis H1:
H1: The new energy demonstration city policy has a significant positive impact on improvement of ECP.
Policy support is crucial to technological innovation (Samant et al., 2020). First of all, government orientation can stimulate the output and input of technological innovation of high-tech enterprises, strengthen research on basic science and technological knowledge to promote the technological frontier, accelerate the development and utilisation of green energy technology, and enhance the technological innovation ability of enterprises (Szczygielski et al., 2017). Meanwhile, with the rapid development of technological innovation, the cost of new energy development has been reduced and the internal driving force for enterprises to carry out independent innovation and improve business efficiency has been increased. The second, the construction of new energy demonstration cities has target requirements for the utilisation of renewable energy such as solar energy, biomass energy, and wind energy, which urges the government to take certain policies and measures to strengthen the elimination of enterprises relying on traditional energy. Meanwhile, the rising cost of traditional fossil energy consumption and the potential cost of carbon emissions have exerted enormous external pressure on market players, prompting enterprises to increase technological innovation. Accordingly, this paper puts forward hypothesis H2:
H2: The new energy demonstration city policy has positive influence on technological innovation.
Energy is not only the driving force of economic output, but also the source of carbon emission pollution (Ding et al., 2020). Technological innovation driven by green and low-carbon energy demand, government intervention, and economies of scale is the key factor to promote the development of renewable energy (Dodd et al., 2018). Through the innovation of new energy technology, the new energy supply capacity of the city can be improved to meet the energy demand (Lewis, 2010). Technological innovation can also improve energy efficiency and the proportion of low-carbon energy utilisation to optimise the energy structure (Yang X. et al., 2021). By adopting new energy technologies to improve energy efficiency, it is possible to replace highly polluting energy, reduce energy use per unit of economic output, and reduce carbon emission pollution per unit of energy use, ultimately improving ECP. Accordingly, this paper puts forward hypothesis H3:
H3: Technological innovation has a positive impact on ECP.
Policy orientation is a kind of government behaviour whose fundamental purpose is to support economic and social progress with high-quality energy development (Zingher and Flynn, 2019). In the context of a dual-carbon target, the policy orientation of new energy demonstration cities is an important driving force to promote green technology innovation and achieve energy-saving and low-carbon development. Combined with the logical causality among H1, H2, and H3, this study assumes that technological innovation plays a mediating role between the new energy demonstration city policy and ECP. Therefore, the following hypotheses are proposed:
H4: Technological innovation plays a mediating role between new energy demonstration city policy and ECP.
The implementation of the new energy demonstration city policy can be regarded as a quasi-natural experiment exogenous to the energy economic system. However, because the selection of the new energy demonstration city is not completely random, there are certain endogenous problems. The DID method is currently the mainstream method for evaluating policy effects. DID can solve the endogenous problem and alleviate the problem of omission variable bias, but it still has the problem of selection bias (Wang and Qiu, 2021; Yang D. et al., 2021). Econometric economists represented by Heckman (1976), Rosenbaum, and Rubin (Chung et al., 1997) proposed the Propensity Score Matching (PSM) method, which eliminate the problem of selection deviation. Combining the advantages of DID and PSM, this paper performs Propensity Score Matching on panel data before implementation of the policy, and makes a DID regression on panel data after matching. In order to keep the data stable and eliminate heteroscedasticity, all variables are treated with logarithm. Based on the above analysis and research hypothesis H1, the benchmark regression model is established as follows:
In addition, in order to test whether the policy orientation for the construction of new energy demonstration cities will indirectly affect urban ECP through technological innovation, this paper draws lessons from the design of the three-stage mediating effect model by Baron and Kenny (1986) and the phased regression method. The first stage is to test whether China’s new energy demonstration city policy is conducive to improving ECP, with ECPI as the dependent variable and the new energy demonstration city policy as the independent variable. The model is consistent with the benchmark regression model. The second stage is to take technological innovation as the dependent variable and the new energy demonstration city policy as the independent variable to test the impact of the approved new energy demonstration city pilot on urban technological innovation ability. In the third stage, ECPI is taken as the explained variable and technological innovation is taken as the explanatory variable to test the impact of technological innovation on ECP (Chen S. et al., 2020). Based on research hypotheses H2 and H3 and the idea of the mediating effect model, this paper sets the mediation effect model as shown in Eqs 2,3.
In the above two equations,
Moreover, in order to test whether the mediating effect of technological innovation is full mediating effect, namely whether the influence of policy orientation on ECP is still significant after controlling the indirect effect of technological innovation, this paper further constructs a regression model with ECPI as dependent variable. Multiplier term
If both
When some cities create new energy demonstration cities, they only set up a county or district within the urban area as pilot cities; For example, Beijing has set up Changping District as a pilot new energy demonstration city. As this study employs the data of prefecture-level cities to study the policy effect, the sample cities of county-level cities and districts are excluded to ensure the reliability of the research results. Base on the availability of data, only the first batch of new energy demonstration cities published by China’s national energy administration in 2014 were selected as the research object of policy implementation effect. The sample in this paper includes 2,366 observations in 182 cities in China from 2005 to 2017, of which the treatment group consists of 38 cities and the control group consists of 144 cities. All data comes from the Chinese Urban Yearbook from 2005 to 2017 and the statistical yearbook and statistical bulletin of each city. The missing values of a few variables in individual years are supplemented by the interpolation method.
Zhou et al. (2012) proposed a NDDF method to measure the ECP of electricity generation in different countries. On this basis, Zhang et al. (2014) proposed a common frontier NDDF approach to measure the power generation industries’ energy and carbon efficiency. They also analysed the size control policy’s impact on efficiency of China’s fossil fuel power generation. NDDF has been widely used for it can effectively solve the problem that all input and output factors change in the same direction (Liu et al., 2020). For example, Chen et al. (2019) used the NDDF method to measure the ECP of China’s construction industry. Therefore, this paper establishes a NDDF model to measure ECP.
In the empirical part, the 182 studied cities are treated as research objects. Take labour force(L), contain capital(K), and total energy consumption(E) as input elements, GDP of each city as desirable output, and carbon dioxide emission of each city as undesirable output. Based on the research of Lin and Zhou (2021b), the set production technology form is as follows:
In reference to Zhou et al. (2012), the NDDF is defined as:
Where
The optimal solution
In the process of measuring ECPI, the average number of employees is selected in each city to measure the level of labour force(L). Considering data availability at the city level, total energy consumption (E) is measured by the total urban energy consumption from Beijing Digital Huitong Environmental Technology Research Institute (Data Service Network, 2021). The stock of fixed capital is used to measure Capital. The calculation is:
The multiplier term
Technological innovation (TI) is the key to improving ECP. With improvement on the scientific and technological level, new energy and green production technologies are innovated and energy utilisation efficiency is improved. The large-scale use of new energy can reduce carbon dioxide emission and promote the improvement of ECP. The input of scientific and technological talents is an important source of promoting technological development and an important embodiment of technological innovation ability. Therefore, in this paper, the proportion of scientific research, technical service, and geological exploration employee to year-end employee at the end of the year is used to measure the city’s technological innovation ability (Qu et al., 2020).
ECP is also affected by many factors. Studies have shown that the increase in the proportion of the tertiary industry and the secondary industry can indirectly reduce CO2 emissions by improving energy efficiency (Zhao et al., 2022). The introduce of foreign capital can not only bring advanced technology and capital, but also promote the improvement of energy efficiency (Pan et al., 2020). Namahoro et al. (2021) believes that renewable energy consumption and economic growth can effectively curb CO2 emissions. In addition, carbon sink resources and urbanization levels are also important factors affecting ECP (Teng et al., 2021; Liu et al., 2021). Based on the above research, this paper selects industrial structure (IND), urban economic development level (PGDP), urbanization rate (URBAN), foreign direct investment (FDI), and carbon sink resources (CSR) as control variables. The ratio of the added value of the tertiary industry to the added value of the secondary industry was adopted as the proxy variable of IND (Lin and Zhou, 2021b). For urban economic development level, the study is measured by GDP per capita (Wan et al., 2022). Urbanisation rate refers to the proportion of urban population to total population (Wan et al., 2022). This article measures foreign direct investment by the proportion of FDI to gross domestic product (Tan et al., 2021). Carbon sink resources are measured by the urban greening coverage of the studied city. The descriptive statistical analysis of each variable is shown in Table 1.
Firstly, PSM was conducted in 182 cities, including 38 processing cities and 144 control cities to eliminate the selection deviation and ensure the accuracy of DID analysis. Logit regression was used to calculate the propensity score of the sample. Finally, the economic development level, urbanisation rate, industrial structure, foreign direct investment, and carbon sink resources are used as the matching variable for 1:1 within the calliper of neighbour matching.
Figure 1 shows the kernel density map before and after matching between new energy demonstration cities (treatment group) and non-demonstration cities (control group). According to PSM matching results, sample cities of the experimental group and control group were basically matched. Before matching, there are great differences in the distribution of propensity scores between the experimental group and the control group. If the DID analysis is carried out directly, the policy effect may have the problem of sample deviation. Instead, we can find the city with the similarity in the control group, and then retain the urban individuals with overlapping tendency scores. After the above matching treatment, the probability distribution of tendency scores in the experimental group and the control group has basically tended to coincide. It shows no significant systematic error, which is meeting the common support hypothesis of the PSM model.
FIGURE 1. Kernel density distribution comparison of propensity score values before and after kernel matching.
In order to test the rationality of the selected matching variables, the balance test is carried out on the matched samples. Table 2 shows the balance test results. The standard error of most variable after matching is significantly reduced, and the absolute values of the standard deviation after matching are all less than 10%. The experimental group and the control group tend to be the same in all dimensions. To sum up, the selected variables and the overall sample size have passed the balance test. The selected matching variables and matching methods are appropriate, which shows matching results are reliable and effective.
Table 3 is the benchmark regression results of Eq. 1. Models (1) and (2) are DID regression results without propensity score matching, while Models (3) and (4) present a control group with similar characteristics to the experimental group through PSM. DID is then used to estimate the net effect of the policy orientation of new energy demonstration city construction on the improvement of urban ECP. Models (1) and (3) are regression models without added control variables, while Models (2) and (4) are regression models with added control variables. Through the Hausman test, it is determined that the regression model adopts the fixed effect model.
The regression coefficients of the four models’ explanatory variable (
It can be seen from Model (4) that the coefficient of the multiplier term (
Industrial structure, economic development level, urbanization level and foreign direct investment can promote the improvement of ECP, which shows that new energy demonstration cities can promote the improvement of ECP through the channels of industrial transformation and attracting foreign direct investment with the support of the dual policies of the central and local governments, so as to provide a steady source of endogenous growth power for the improvement of ECP. However, improvement of the urbanisation rate can inhibit the improvement of ECP. The possible reasons are that, with the development of urbanisation, forest carbon sink resources are continuously reduced, and environmental pollutants such as carbon dioxide generated by energy consumption are continuously accumulated, resulting in “crowding effect”. Thus, it will inhibit the improvement of urban ECP. Another potential reason is that promoting urbanisation requires large-scale infrastructure and housing construction, which indirectly promotes the development of industries such as real estate, construction, automobile, and household appliances. This also promotes urban energy consumption and CO2 emission, and reduces ECP. Therefore, more attention should be paid to high-quality urbanization in the construction of new energy demonstration cities.
The regression results of the DID method are often disturbed by some omitted variables, resulting in the deviation of the operation results. In order to eliminate the interference of all omitted variables, this paper randomly selected pilot cities to conduct a placebo test (Li et al., 2016). Specifically, random sampling was conducted 1,000 times in 182 prefecture-level cities. 38 sample cities were randomly selected from each sampling as the virtual experimental group, and the remaining 144 cities were used as the virtual control group for regression according to Eq. 1 (Shi and Li, 2020). As shown in Figure 2, the absolute value of the T value of regression coefficient in most virtual experiments is within two and follows normal distribution, indicating that the new energy demonstration city policy has no significant effect in these 1,000 virtual experiments. Therefore, through the placebo test of the virtual experimental group, the conclusion of this study verifies again that the influence of new energy demonstration city policy on ECP has little causal relationship with other unknown variables.
Satisfying the parallel trend hypothesis test is an important premise of the DID model. This paper refers to the research of Qu et al. (2022) and Xiao et al. (2022) to test the parallel trend hypothesis. Specifically, by constructing a set of annual dummy variables
According to Figure 3, before the policy was implemented, the regression coefficient value of
In order to eliminate the interference of other policies and unobservable omitted variables on the policy orientation effect, and further support the causal effect of benchmark regression in this paper, the time of the new energy demonstration policy is simulated to years before 2014. And on this basis, the regression estimation of the benchmark model is carried out to conduct a counterfactual test (Zheng et al., 2021). The premise of the DID method is that the experimental group and control group sample are comparable, that is, if there is no new energy demonstration policy, the ECP of the experimental group and control group cities will not show significant differences over time. In order to verify this premise, the time of policy impact was successively advanced to 2013 and 2012 to conduct tests consistent with benchmark regression. According to the counterfactual test results in Table 4, when the time of policy implementation is advanced to 2013 or 2012, the coefficient of
In order to promote the sustainable development of energy economy, the Chinese government has introduced a number of Energy Conservation and Pollution Emissions Reduction policies, such as carbon emission trading pilot policy and low-carbon city pilot policy. The relevant policy orientation also plays a significant role in ECP. In order to accurately identify the effect of policy orientation, it is necessary to exclude the interference of other policies. Therefore, this paper introduces the low-carbon city pilot and carbon emission trading pilot into the benchmark model, and sets the multiplier term (Low carbon) between the low-carbon city and the implementation time (Hong et al., 2021), and the multiplier term (Carbon trading) between the carbon emission trading pilot city and the implementation time as the control variables respectively. The benchmark regression results after introducing these dummy variables of regional policies are shown in Table 5. Among them, Model (9) and Model (11) only contain policy variables. Model (10) and Model (12) further introduce control variables. It can be found that after adding other policy variables, the estimation coefficient of the interaction term of the new energy demonstration city is still significantly positive, showing that the conclusion of this paper is still robust after considering the above policy interference.
According to the previous discussion, the policy orientation may have an impact on ECP through technological innovation channels. This paper identifies the impact mechanism of technological innovation based on the mediating effect test model of Eqs 1–3. It then uses Eq. 4 tests to determine whether the technological innovation effect is a full mediating effect. As mentioned above, through the regression estimation and robustness test of the benchmark model in Eq. 1, it is proved that the mediating effect in the first stage is tenable. In order to further test the mediating effect, regression was carried out for Eqs 2,3. Table 6 lists the results of the impact mechanism test. The regression results of Model (13) and Model (14) show that the regression coefficients of the new energy demonstration city policy on urban technological innovation are significantly positive at the confidence level of 1%, indicating that policy orientation effectively promotes urban technological innovation, and hypothesis H2 is confirmed. The possible reasons may include: 1) technological innovation has a sustained driving force for the development of new energy industry and economic growth. 2) local governments have full enthusiasm for technological innovation and increased the guarantee for innovation investment, talent support, and infrastructure construction. 3) the construction of new energy demonstration cities can form an industrial agglomeration and demonstration effect, which plays a key role in promoting technological innovation.
This paper brings the new energy demonstration policy and technological innovation into the model at the same time to further test whether the mediating effect of technological innovation is a full mediating effect, which is regression to Eq. 4. Models (17) and (18) in Table 6 are the regression results after controlling the indirect impact of technological innovation, showing that the impact of policy orientation on urban ECP is still significantly positive. The regression coefficient of policy effect is slightly smaller than that of Model (4) in Table 3, which further proves that technological innovation does exist as a mediating effect. The adjusted mediating effect is 0.0031, accounting for 12.92% of the total effect. Under the premise of controlling the indirect impact of technological innovation, the comprehensive effect of policy orientation on urban ECP of new energy demonstration cities is 0.0209.
In this paper, 182 cities are divided into three regions in the east, middle and west according to their urban locations, and the regression estimation of Eq. 1 is carried out by region to investigate whether there are regional differences in the impact of approved new energy demonstration city construction on ECP. The regression results are shown in Table 7. During the sample period, the construction of new energy demonstration cities had a significant positive impact on the ECP of eastern and western cities, while the driving effect on central cities is not obvious. The central region is relatively rich in coal resources, and industries with high pollution and high energy consumption have long accounted for a large proportion (Cui et al., 2022). In recent years, it has continued to undertake industrial transfers in the eastern region. Limited alternative effect of new energy could not inhibit the continuing increase of energy consumption of industrial enterprises in the production process. Then it would increase traditional fossil energy consumption and carbon dioxide emissions. On the other hand, the construction of demonstration cities depends on the institutional environment and governance capabilities. A relatively poor institutional environment shows that the low efficiency of city’s resource allocation would reduce the enthusiasm of relevant entities to participate, and weaken the policy spillover effect of new energy demonstration cities. The western region has a relatively backward economy. It is relatively easy to carry out popularization projects for a single industrial structure and a low population density. These are conducive to the promotion and implementation of new energy demonstration city construction projects such as photovoltaic power generation, biomass biogas and straw power generation. And it could promote the large-scale development of the new energy industry, resulting in huge returns to scale; On the other hand, western cities are rich in natural resources such as sunlight and the Gobi Desert (land) (Wang and Yi, 2021), which provides safe and reliable green energy for urban economic production and greatly reduces the emission of pollutants such as carbon dioxide, which could significantly improve the ECP. The eastern region has a series of advantages, including more reasonable industrial structure, more diversified urban development, and more efficient government functions (Yang et al., 2022), which can attract high-tech talents, form a high-tech industry cluster and gather sufficient material and intellectual resources for the development of the new energy industry. Therefore, the enhancement of ECP is more obvious. However, the natural resources of the eastern cities are not as rich as those of the western cities, so the promotion effect on ECP is lower than that of the western cities.
Firstly, this paper analyses in theory that the policy orientation of new energy demonstration city construction may either directly affect the city’s ECP or indirectly improve the city’s ECP through technological innovation. By adopting the causal inference method of PSM-DID and the mediating effect analysis method, the direct effect of policy orientation on the improvement of ECP and the mediating effect of technological innovation are studied. The empirical results firstly show that the new energy demonstration city policy can significantly improve the ECP of the approved cities. This conclusion is still valid after passing the placebo test, parallel trend test, counterfactual test, and superposition policy test. Second, the policy orientation of new energy demonstration cities has both direct influence on urban ECP and indirect influence on urban ECP through influencing technological innovation, in which the mediating effect of technological innovation accounts for 12.92% of the total effect, which is a partial mediating effect. Third, the regression results show that external environmental variables also have a certain impact on ECP. Optimising industrial structure, improving economic development level, increasing carbon sink resources, and attracting foreign direct investment have significant impacts on China’s ECP. However, improvement of the urbanisation rate can inhibit the improvement of ECP. Through the heterogeneity analysis of cities in different regions, it is found that the construction of new energy demonstration cities presents the heterogeneity of geographical locations, and the pilot policies are more effective in driving eastern and western cities.
Finally, for improving the China’s ECP and accelerating the realization of the dual-carbon target, the following policy suggestions are put forward based on the research conclusions:
1) Fully promote the positive effect of the new energy demonstration city policy on ECP. In the critical period of the energy system transition from fossil fuels to new energy, the construction of new energy demonstration cities plays a positive role in enhancing the coordinated development of promoting ECP enhancement, new energy development, and urban energy self-sufficiency increasement. We should give full play to the leading role of new energy demonstration cities. The diffusion and trickle-down effect of new energy technologies in the construction of new energy demonstration cities should be utilised. Therefore, we should summarise and promote the advanced experience in the construction of new energy demonstration cities, improve the demonstration city selection system, continue to increase the support for demonstration city construction funds, talents and technologies, orderly expand the pilot scope, and give full play to the demonstration effect of pilot projects. At the same time, it is necessary to strengthen the supervision of the construction quality of the demonstration cities, ensure the construction quality of the demonstration cities, and actively promote the upgrading of urban energy system.
2) Cultivate new economic growth by virtue of the role of technological innovation in the new energy demonstration city policy, and exert the lasting and long-term influence of policy orientation on energy carbon performance by using the means of industrial transition and foreign direct investment. On the one hand, in the process of overall promotion and construction of new energy demonstration cities, local governments should adhere to the guidance of government policies. Technological innovation as the optimal path of economic growth can increase investment in scientific and technological innovation and the training reserve of innovative talents. It also can continuously optimise cooperation mechanism in the field of enterprise production and institution’s research. Scale effects are formed through the collection of innovative resources to further promote the improvement of urban innovation ability. On the other hand, more attention should be paid to the coordination of investment environment, industrial structure and construction of green and low-carbon urbanisation. Governments should build an industrial environment and investment environment conducive to the development and utilisation of urban new energy, and introduce international advanced technology and management experience. It also should give full play to the positive role of industrial structure optimisation. At the same time, when carrying out the construction of forest greening cities, governments should pay attention to the coordinated development of population urbanisation, green and low-carbon energy consumption, and ecological environment construction.
3) Promoting the construction of new energy demonstration cities according to local conditions. In view of the mechanism complexity for the policy orientation of the construction of new energy demonstration cities and the different characteristics of the cities, local governments should seize the location advantage and formulate support policies according to local conditions in the process of promoting new energy demonstration cities. Specifically, cities in the central region should speed up industrial transformation, rely on the construction of new energy demonstration cities to optimize urban resource allocation, increase support for high-tech talents and enterprises, improve government governance capabilities, and provide sufficient material and intellectual resources for the development of new energy industries. Therefor it could enhance the production capacity of renewable energy and optimize the urban energy consumption structure. Cities in the western region should make full use of natural resources and the effect of policy orientation to promote the high-quality development of the new energy industry. At the same time, it is necessary to continuously optimize the industrial structure, enhance regional innovation capabilities, and accelerate the construction of new urbanization. Cities in the eastern region should increase their efforts to attract foreign investment, introduce advanced foreign technology and experience, weaken their dependence on natural resources, increase investment in scientific and technological innovation, and realize the improvement of ECP through technological innovation and structural optimization.
This study has some limitations. Firstly, we included only one undesirable output, CO2 emissions, in our empirical analysis. In this regard, future research should consider a wider range of pollutants to better assess the policy-oriented effects of new energy demonstration city construction. Secondly, there is improvement possibilities in capturing policy spillovers. It should be further explored the causal relationship between geographic location, new energy demonstration city construction and ECP.
The original contributions presented in the study are included in the article/Supplementary Material, further inquiries can be directed to the corresponding author.
XZ: Conceptualization, Methodology, Investigation, Visualization, Writing—original draft. RZ: Resources, Methodology, Supervision, Funding acquisition. MZ: Data curation, Writing—review and editing. YW: Data curation, Software. XC: Writing—review and editing.
This research was funded by the Major Science and Technology Projects in Shanxi Province (No. 20181102017).
The authors declare that the research was conducted in the absence of any commercial or financial relationships that could be construed as a potential conflict of interest.
All claims expressed in this article are solely those of the authors and do not necessarily represent those of their affiliated organizations, or those of the publisher, the editors and the reviewers. Any product that may be evaluated in this article, or claim that may be made by its manufacturer, is not guaranteed or endorsed by the publisher.
The authors are grateful to reviewers and editors for helpful comments and suggestions.
Anser, M. K., Khan, M. A., Nassani, A. A., Aldakhil, A. M., Voo, X. H., and Zaman, K. (2020). Relationship of Environment with Technological Innovation, Carbon Pricing, Renewable Energy, and Global Food Production. Econ. Innovation New Techn. 30 (8), 807–842. doi:10.1080/10438599.2020.1787000
Baron, R. M., and Kenny, D. A. (1986). The Moderator-Mediator Variable Distinction in Social Psychological Research: Conceptual, Strategic, and Statistical Considerations. J. Personal. Soc. Psychol. 51 (6), 1173–1182. doi:10.1037/0022-3514.51.6.1173
BP (2021). BP's Statistical Review of World Energy. Available at: https://www.bp.com/en/global/corporate/energy-economics/statistical-review-of-world energy.html (Accessed November 11, 2021).
Chen, X., and Lin, B. (2020). Energy and CO2 Emission Performance: A Regional Comparison of China's Non-ferrous Metals Industry. J. Clean. Prod. 274, 123168. doi:10.1016/j.jclepro.2020.123168
Chen, X., and Lin, B. (2021). Towards Carbon Neutrality by Implementing Carbon Emissions Trading Scheme: Policy Evaluation in China. Energy Policy 157, 112510. doi:10.1016/j.enpol.2021.112510
Chen, J., Xu, C., Managi, S., and Song, M. (2019). Energy-carbon Performance and its Changing Trend: An Example from China's Construction Industry. Resour. Conserv. Recycl. 145, 379–388. doi:10.1016/j.resconrec.2019.03.014
Chen, J., Gao, M., Cheng, S., Hou, W., Song, M., Liu, X., et al. (2020). County-level CO2 Emissions and Sequestration in China during 1997-2017. Sci. Data 7 (1), 391. doi:10.1038/s41597-020-00736-3
Chen, S., Shi, A., and Wang, X. (2020). Carbon Emission Curbing Effects and Influencing Mechanisms of China's Emission Trading Scheme: The Mediating Roles of Technique Effect, Composition Effect and Allocation Effect. J. Clean. Prod. 264, 121700. doi:10.1016/j.jclepro.2020.121700
Choi, Y., Zhang, N., and Zhou, P. (2012). Efficiency and Abatement Costs of Energy-Related CO2 Emissions in China: A Slacks-Based Efficiency Measure. Appl. Energ. 98, 198–208. doi:10.1016/j.apenergy.2012.03.024
Chung, Y. H., Färe, R., and Grosskopf, S. (1997). Productivity and Undesirable Outputs: A Directional Distance Function Approach. J. Environ. Manage. 51 (3), 229–240. doi:10.1006/jema.1997.0146
Cui, L., Sun, W., and Li, X. (2022). Research on Environmental Effect of New Energy Demonstration City Construction. East China Econ. Manage. 36 (2), 57–65. (in Chinese). doi:10.19629/j.cnki.34-1014/f.210724003
Data Service Network (2021). Available at: http://www.3edata.com/?m=nologin (Accessed November 11, 2021).
Ding, L.-L., Lei, L., Zhao, X., and Calin, A. C. (2020). Modelling Energy and Carbon Emission Performance: A Constrained Performance index Measure. Energy 197, 117274. doi:10.1016/j.energy.2020.117274
Dingbang, C., Cang, C., Qing, C., Lili, S., and Caiyun, C. (2021). Does New Energy Consumption Conducive to Controlling Fossil Energy Consumption and Carbon Emissions?-Evidence from China. Resour. Pol. 74, 102427. doi:10.1016/j.resourpol.2021.102427
Dodd, T., Orlitzky, M., and Nelson, T. (2018). What Stalls a Renewable Energy Industry? Industry Outlook of the Aviation Biofuels Industry in Australia, Germany, and the USA. Energy Policy 123, 92–103. doi:10.1016/j.enpol.2018.08.048
Fei, R., and Lin, B. (2017). The Integrated Efficiency of Inputs-Outputs and Energy - CO2 Emissions Performance of China's Agricultural Sector. Renew. Sustain. Energ. Rev. 75, 668–676. doi:10.1016/j.rser.2016.11.040
Heckman, J. J. (1976). The Common Structure of Statistical Models of Truncation, Sample Selection and Limited Dependent Variables and a Simple Estimator for Such Models. NBER 5 (4), 475–492.
Hong, M., Chen, S., and Zhang, K. (2021). Impact of the "Low-Carbon City Pilot" Policy on Energy Intensity Based on the Empirical Evidence of Chinese Cities. Front. Environ. Sci. 9, 717737. doi:10.3389/fenvs.2021.717737
Iftikhar, Y., He, W., and Wang, Z. (2016). Energy and CO2 Emissions Efficiency of Major Economies: A Non-parametric Analysis. J. Clean. Prod. 139, 779–787. doi:10.1016/j.jclepro.2016.08.072
Iftikhar, Y., Wang, Z., Zhang, B., and Wang, B. (2018). Energy and CO2 Emissions Efficiency of Major Economies: A Network DEA Approach. Energy 147, 197–207. doi:10.1016/j.energy.2018.01.012
Khan, A., Muhammad, F., Chenggang, Y., Hussain, J., Bano, S., and Khan, M. A. (2020). The Impression of Technological Innovations and Natural Resources in Energy-Growth-Environment Nexus: A New Look into BRICS Economies. Sci. Total Environ. 727, 138265. doi:10.1016/j.scitotenv.2020.138265
Kirikkaleli, D., and Adebayo, T. S. (2020). Do renewable Energy Consumption and Financial Development Matter for Environmental Sustainability? New Global Evidence. Sustain. Develop. 29 (4), 583–594. doi:10.1002/sd.2159
Lee, H. (2021). Is Carbon Neutrality Feasible for Korean Manufacturing Firms?: The CO2 Emissions Performance of the Metafrontier Malmquist-Luenberger index. J. Environ. Manage. 297, 113235. doi:10.1016/j.jenvman.2021.113235
Lewis, J. I. (2010). The Evolving Role of Carbon Finance in Promoting Renewable Energy Development in China. Energy Policy 38 (6), 2875–2886. doi:10.1016/j.enpol.2010.01.020
Li, Z., and Yang, S. (2018). Fiscal Decentralization, Government Innovation Preferences and Regional Innovation 646 Efficiency. Manage. World 34, 29–42. (in Chinese). doi:10.19744/j.cnki.11-1235/f.2018.0031
Li, P., Lu, Y., and Wang, J. (2016). Does Flattening Government Improve Economic Performance? Evidence from China. J. Develop. Econ. 123, 18–37. doi:10.1016/j.jdeveco.2016.07.002
Lin, B., and Chen, Y. (2020). Will Land Transport Infrastructure Affect the Energy and Carbon Dioxide Emissions Performance of China's Manufacturing Industry? Appl. Energ. 260, 114266. doi:10.1016/j.apenergy.2019.114266
Lin, B., and Wu, R. (2020). Designing Energy Policy Based on Dynamic Change in Energy and Carbon Dioxide Emission Performance of China's Iron and Steel Industry. J. Clean. Prod. 256, 120412. doi:10.1016/j.jclepro.2020.120412
Lin, B., and Zhou, Y. (2021a). Does the Internet Development Affect Energy and Carbon Emission Performance? Sustain. Prod. Consum. 28, 1–10. doi:10.1016/j.spc.2021.03.016
Lin, B., and Zhou, Y. (2021b). Does Fiscal Decentralization Improve Energy and Environmental Performance? New Perspective on Vertical Fiscal Imbalance. Appl. Energ. 302, 117495. doi:10.1016/j.apenergy.2021.117495
Liu, K., Lu, S., and Zhang, G. (2020). Regional Difference in Global Unified Efficiency of China-Evidence from City-Level Data. Sci. Total Environ. 713, 136355. doi:10.1016/j.scitotenv.2019.136355
Liu, W., Zhan, J., Zhao, F., Wei, X., and Zhang, F. (2021). Exploring the Coupling Relationship between Urbanization and Energy Eco-Efficiency: A Case Study of 281 Prefecture-Level Cities in China. Sustain. Cities Soc. 64, 102563. doi:10.1016/j.scs.2020.102563
Malinauskaite, J., Jouhara, H., Czajczyńska, D., Stanchev, P., Katsou, E., Rostkowski, P., et al. (2017). Municipal Solid Waste Management and Waste-To-Energy in the Context of a Circular Economy and Energy Recycling in Europe. Energy 141, 2013–2044. doi:10.1016/j.energy.2017.11.128
Namahoro, J. P., Wu, Q., Zhou, N., and Xue, S. (2021). Impact of Energy Intensity, Renewable Energy, and Economic Growth on CO2 Emissions: Evidence from Africa across Regions and Income Levels. Renew. Sustain. Energ. Rev. 147, 111233. doi:10.1016/j.rser.2021.111233
Pan, X., Guo, S., Han, C., Wang, M., Song, J., and Liao, X. (2020). Influence of FDI Quality on Energy Efficiency in China Based on Seemingly Unrelated Regression Method. Energy 192, 116463. doi:10.1016/j.energy.2019.116463
Qu, C., Shao, J., and Shi, Z. (2020). Does Financial Agglomeration Promote the Increase of Energy Efficiency in China? Energy Policy 146, 111810. doi:10.1016/j.enpol.2020.111810
Qu, F., Xu, L., and Chen, Y. (2022). Can Market-Based Environmental Regulation Promote Green Technology Innovation? Evidence from China. Front. Environ. Sci. 9, 823536. doi:10.3389/fenvs.2021.823536
Salehi, M., Filimonau, V., Asadzadeh, M., and Ghaderi, E. (2021). Strategies to Improve Energy and Carbon Efficiency of Luxury Hotels in Iran. Sustain. Prod. Consum. 26, 1–15. doi:10.1016/j.spc.2020.09.007
Samant, S., Thakur-Wernz, P., and Hatfield, D. E. (2020). Does the Focus of Renewable Energy Policy Impact the Nature of Innovation? Evidence from Emerging Economies. Energy Policy 137, 111119. doi:10.1016/j.enpol.2019.111119
Shan, S., Ahmad, M., Tan, Z., Adebayo, T. S., Man Li, R. Y., and Kirikkaleli, D. (2021). The Role of Energy Prices and Non-linear Fiscal Decentralization in Limiting Carbon Emissions: Tracking Environmental Sustainability. Energy 234, 121243. doi:10.1016/j.energy.2021.121243
Shan, H. (2008). Reestimating the Capital Stock of China:1952 ∼ 2006. J. Quant. Techn. Econ. 25, 15. (in Chinese). doi:10.1088/0256-307x/25/10/033
Shi, D., and Li, S. (2020). Emissions Trading System and Energy Use Efficiency-Measurements and Empirical Evidence for Cities at and above the Prefecture Level. China Ind. Econ. 09, 5–23. (in Chinese). doi:10.19581/j.cnki.ciejournal.2020.09.001
Song, X., Wang, X., Li, X., Zhang, W., and Scheffran, J. (2021). Policy-oriented versus market-induced: Factors influencing crop diversity across China. Ecol. Econ. 190, 107184. doi:10.1016/j.ecolecon.2021.107184
Sun, X., Zhang, R., Wang, G., Guo, J., and Liu, Z. (2021). Factor decomposition, reduction potential, and rebound effect of energy consumption related PM2.5 in China. J. Clean. Prod. 322, 129088. doi:10.1016/j.jclepro.2021.129088
Szczygielski, K., Grabowski, W., Pamukcu, M. T., and Tandogan, V. S. (2017). Does government support for private innovation matter? Firm-level evidence from two catching-up countries. Res. Pol. 46 (1), 219–237. doi:10.1016/j.respol.2016.10.009
Tan, Z., Koondhar, M. A., Nawaz, K., Malik, M. N., Khan, Z. A., and Koondhar, M. A. (2021). Foreign direct investment, financial development, energy consumption, and air quality: A way for carbon neutrality in China. J. Environ. Manage. 299, 113572. doi:10.1016/j.jenvman.2021.113572
Teng, X., Liu, F.-p., and Chiu, Y.-h. (2021). The change in energy and carbon emissions efficiency after afforestation in China by applying a modified dynamic SBM model. Energy 216, 119301. doi:10.1016/j.energy.2020.119301
Wan, L., Zheng, Q., Wu, J., Wei, Z., and Wang, S. (2022). How does the ecological compensation mechanism adjust the industrial structure? Evidence from China. J. Environ. Manage. 301, 113839. doi:10.1016/j.jenvman.2021.113839
Wang, A., and Lin, B. (2018). Dynamic change in energy and CO2 performance of China's commercial sector: A regional comparative study. Energy Policy 119, 113–122. doi:10.1016/j.enpol.2018.04.036
Wang, Z., and Qiu, S. (2021). Can “energy saving and emission reduction” demonstration city selection actually contribute to pollution abatement in China? Sustain. Prod. Consum. 27, 1882–1902. doi:10.1016/j.spc.2021.04.030
Wang, Q., and Yi, H. (2021). New energy demonstration program and China's urban green economic growth: Do regional characteristics make a difference? Energy Policy 151, 112161. doi:10.1016/j.enpol.2021.112161
Wang, K., Lu, B., and Wei, Y.-M. (2013). China's regional energy and environmental efficiency: A Range-Adjusted Measure based analysis. Appl. Energ. 112, 1403–1415. doi:10.1016/j.apenergy.2013.04.021
Wang, Z., He, W., and Wang, B. (2017). Performance and reduction potential of energy and CO2 emissions among the APEC's members with considering the return to scale. Energy 138, 552–562. doi:10.1016/j.energy.2017.07.059
Wang, R., Wang, Q., and Yao, S. (2021). Evaluation and difference analysis of regional energy efficiency in China under the carbon neutrality targets: Insights from DEA and Theil models. J. Environ. Manage. 293, 112958. doi:10.1016/j.jenvman.2021.112958
Wu, R., and Lin, B. (2022). Environmental regulation and its influence on energy-environmental performance: Evidence on the Porter Hypothesis from China's iron and steel industry. Resour. Conserv. Recycl. 176, 105954. doi:10.1016/j.resconrec.2021.105954
Wu, J., Zuidema, C., and Gugerell, K. (2018). Experimenting with decentralized energy governance in China: The case of New Energy Demonstration City program. J. Clean. Prod. 189, 830–838. doi:10.1016/j.jclepro.2018.04.123
Xiao, Z., Yu, L., Liu, Y., Bu, X., and Yin, Z. (2022). Does Green Credit Policy Move the Industrial Firms Toward a Greener Future? Evidence From a Quasi-Natural Experiment in China. Front. Environ. Sci. 9, 810305. doi:10.3389/fenvs.2021.810305
Yang, D., Zhang, H.-w., Liu, Z.-m., and Zeng, Q. (2021). Do cooperatives participation and technology adoption improve farmers' welfare in China? A joint analysis accounting for selection bias. J. Integr. Agric. 20 (6), 1716–1726. doi:10.1016/S2095-3119(20)63325-1
Yang, J., Xiong, G., and Shi, D. (2022). Innovation and sustainable: Can innovative city improve energy efficiency? Sustain. Cities Soc. 80, 103761. doi:10.1016/j.scs.2022.103761
Yang, X., Zhang, J., Ren, S., and Ran, Q. (2021). Can the new energy demonstration city policy reduce environmental pollution? Evidence from a quasi-natural experiment in China. J. Clean. Prod. 287, 125015. doi:10.1016/j.jclepro.2020.125015
Zhang, N., Zhou, P., and Choi, Y. (2013). Energy efficiency, CO2 emission performance and technology gaps in fossil fuel electricity generation in Korea: A meta-frontier non-radial directional distance functionanalysis. Energy Policy 56, 653–662. doi:10.1016/j.enpol.2013.01.033
Zhang, N., Kong, F., Choi, Y., and Zhou, P. (2014). The effect of size-control policy on unified energy and carbon efficiency for Chinese fossil fuel power plants. Energy Policy 70, 193–200. doi:10.1016/j.enpol.2014.03.031
Zhao, J., Jiang, Q., Dong, X., Dong, K., and Jiang, H. (2022). How does industrial structure adjustment reduce CO2 emissions? Spatial and mediation effects analysis for China. Energ. Econ. 105, 105704. doi:10.1016/j.eneco.2021.105704
Zheng, X., Wu, C., and He, S. (2021). Impacts of China's differential electricity pricing on the productivity of energy-intensive industries. Energ. Econ. 94, 105050. doi:10.1016/j.eneco.2020.105050
Zhou, P., Ang, B. W., and Wang, H. (2012). Energy and CO2 emission performance in electricity generation: A non-radial directional distance function approach. Eur. J. Oper. Res. 221 (3), 625–635. doi:10.1016/j.ejor.2012.04.022
Keywords: new energy demonstration city policy, technological innovation, ECP, PSM-DID, mediating effect model
Citation: Zhang X, Zhang R, Zhao M, Wang Y and Chen X (2022) Policy Orientation, Technological Innovation and Energy-Carbon Performance: An Empirical Study Based on China’s New Energy Demonstration Cities. Front. Environ. Sci. 10:846742. doi: 10.3389/fenvs.2022.846742
Received: 31 December 2021; Accepted: 11 March 2022;
Published: 31 March 2022.
Edited by:
Haikun Wang, Nanjing University, ChinaReviewed by:
Yuanchun Zhou, Nanjing University of Finance and Economics, ChinaCopyright © 2022 Zhang, Zhang, Zhao, Wang and Chen. This is an open-access article distributed under the terms of the Creative Commons Attribution License (CC BY). The use, distribution or reproduction in other forums is permitted, provided the original author(s) and the copyright owner(s) are credited and that the original publication in this journal is cited, in accordance with accepted academic practice. No use, distribution or reproduction is permitted which does not comply with these terms.
*Correspondence: Rui Zhang, enJjdW10YkAxNjMuY29t
Disclaimer: All claims expressed in this article are solely those of the authors and do not necessarily represent those of their affiliated organizations, or those of the publisher, the editors and the reviewers. Any product that may be evaluated in this article or claim that may be made by its manufacturer is not guaranteed or endorsed by the publisher.
Research integrity at Frontiers
Learn more about the work of our research integrity team to safeguard the quality of each article we publish.