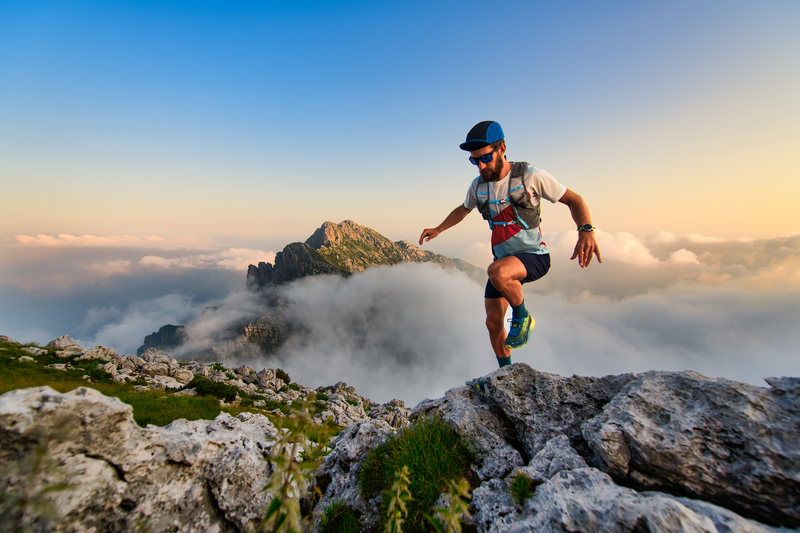
95% of researchers rate our articles as excellent or good
Learn more about the work of our research integrity team to safeguard the quality of each article we publish.
Find out more
ORIGINAL RESEARCH article
Front. Environ. Sci. , 08 June 2022
Sec. Interdisciplinary Climate Studies
Volume 10 - 2022 | https://doi.org/10.3389/fenvs.2022.846243
This article is part of the Research Topic Climate Change, Extreme Weather, and Vector-Borne Diseases: Spatial and Temporal Risks View all 5 articles
Environmentally suitable habitats of Aedes albopictus (Ae. albopictus) in Europe are identified by several modeling studies. However, it is noticeable that even after decades of invasion process in Europe, the vector mosquito has not yet been established in all its environmentally suitable areas. Natural barriers and human-mediated transport play a role, but the potential of wind speed to explain Ae. albopictus’ absences and its inability to establish in its suitable areas are largely unknown. This study therefore evaluates the potential of wind speed as an explanatory parameter of the non-occurrence of Ae. albopictus. We developed a global ecological niche model with relevant environmental parameters including wind speed and projected it to current climatic conditions in Europe. Differences in average wind speed between areas of occurrence and non-occurrence of Ae. albopictus within its modeled suitable areas were tested for significance. A second global ecological niche model was trained with the same species records and environmental parameters, excluding windspeed parameters. Using multiple linear regression analyses and a test of average marginal effect, the effect of increasing wind speed on the average marginal effect of temperature and precipitation on the projected habitat suitability was estimated. We found that climatically suitable and monitored areas where Ae. albopictus is not established (3.12 ms-1 ± 0.04 SD) have significantly higher wind speed than areas where the species is already established (2.54 ms-1 ± 0.04 SD). Among temperature-related bioclimatic variables, the annual mean temperature was the most important variable contributing to the performance of both global models. Wind speed has a negative effect on the predicted habitat suitability of Ae. albopictus and reduces false-positive rates in model predictions. With increasing wind speed, the average marginal effect of annual mean temperatures decreases but that of the annual precipitation increases. Wind speed should be considered in future modeling efforts aimed at limiting the spread and dispersal of Ae. albopictus and in the implementation of surveillance and early warning systems. Local-scale data collected from fieldwork or laboratory experiments will help improve the state of the art on how wind speed influences the distribution, flight, and dispersal activity of the mosquito.
A growing number of modeling studies have identified European regions to be climatically suitable for the establishment of the Asian tiger mosquito (Aedes albopictus, Diptera, Culicidae). Southern and south-eastern Europe show favorable habitat conditions for the mosquito, taking into account climatic factors of temperature and precipitation (Caminade et al., 2012; Fischer et al., 2014; Roiz et al., 2010), as well as human mobility data (Kraemer et al., 2019) or cargo movement data (Thomas et al., 2014). Aedes albopictus (Ae. albopictus) is a competent vector for arboviruses such as dengue, chikungunya, West Nile virus, Zika virus, and filarial infections such as Dirofilaria immitis (Pereira-dos-Santos et al., 2020). The invasive species can rapidly adapt to new environmental conditions (Benedict et al., 2007) and is continually spreading (ISSG, 2020)1 toward central Europe. But even after decades of spreading, Ae. albopictus has not yet been established in all its climatically suitable areas in Europe (Kraemer et al., 2015; European Centre for Disease Prevention and Control (ECDC) and European Food Safety Authority (ESA, 2021b)). Also, recent species distribution model approaches use sets of environmental parameters related to temperature, precipitation, and day length to project the habitat suitability of Ae. albopictus in space and time (Cunze et al., 2016; Thomas et al., 2018; Ibáñez-Justicia et al., 2020). Although there exists a broad range of literature that uses laboratory and field experiments to study the impact of wind on control actions such as spraying or sterile insect techniques at a local scale (Bibbs et al., 2020), the role of wind speed in explaining the non-occurrence of Ae. albopictus within areas predicted as climatically suitable for the mosquito’s establishment remains unclear.
Under climate change, a global increase in annual mean surface wind speed by 2050 is expected (IPCC, 2013). Recent global climate model projections reveal stronger magnitude of wind speed across northern and central Europe and weaker magnitude across southern Europe, owing to polar shifts in jet streams and storms caused by climate change (Eichelberger et al., 2018).
High wind speed reduces the flight activities of mosquitoes, especially in seeking hosts for blood feeding and nectar feeding (Cardé, 2015). The wind speed above which flight is found to be impaired is species-specific and appears to depend on local adaptation. However, species in the Arctic tundra hardly show impaired flight activity at 2.22 ms−1; the flight activity seems to be limited at 3.05 ms−1 and above. Maximum values of 8.05 ms−1 have been reported from subarctic Canada, above which mosquito flight activity declines. Mosquitoes in central Alaska and Wisconsin stopped flying at 2.22 ms−1 (Service, 1980). The wind speed above 0.9 ms−1 has currently been identified as the major deterring factor to the abundance and flight activities of arboviral mosquitoes on urban green roofs in China; elevated buildings acted as a deterrent to mosquito flight due to high wind exposure (Wong and Jim, 2017). Khan et al. (2018) suggested that only wind speeds below 5.14 ms−1 provide favorable climatic conditions for Ochlerotatus caspius and other mosquitoes in Saudi Arabia. In projecting the suitable areas for the establishment of Ae. j. japonicus in Germany, Kerkow et al. (2019) used wind speed data in a fuzzy modeling approach in addition to climatic suitability and landscape data. They found that the mosquito does not occur in regions of Germany characterized by wind speeds higher than 4.7 ms−1. Other studies have found a significant relationship between high wind speed and low arboviral disease incidences, for example, in Australia, the Caribbean, Latin America, and South Asia (Rosa-Freitas et al., 2006; Sedda et al., 2018). Mala and Jat (2019) found out that wind speeds above 1.67 ms−1 reduce dengue fever incidences in New Delhi, India. They estimated that the decrease in average wind speed from 1.81 ms−1 to 0.42 ms−1 over a 2-month period is associated with an expansion of the spatial pattern of dengue fever incidences and the spread of dengue vectors in the area. For urban areas of Malaysia, it was demonstrated that in addition to water volume and distance from habitation, wind velocity was statistically significant toward Aedes dengue vectors (Che Dom et al., 2016). Adult Ae. albopictus captures at a local scale in Medellín, Colombia, were inversely correlated with wind speed and temperature (Camargo et al., 2021).
The wind direction influences the flight range of mosquitoes. The estimated flight range of Ae. albopictus differs a lot between studies, and the results of laboratory-based studies cannot be compared with those of field studies. When some studies (Sallam et al., 2017; Vavassori et al., 2019) have found that it is limited to 250m, others indicate that it may reach up to 1 km or even 8.6 km (Kaufmann et al., 2013; Medeiros et al., 2017). Wind speed and wind direction determine the dispersal range of Ae. albopictus. The long-distance migration of Aedes mosquitoes into new areas is aided by winds blowing in the same direction as mosquito flights (Provost, 1952). However, high wind speed also reduces mosquito host-seeking activity due to its influence on mosquito flight range and patterns (Cardé, 2015). The long-range dispersal and short-range dispersal of mosquitoes and mosquito-borne diseases by wind have been comprehensively reviewed (e.g. Elbers et al., 2015). The northward and eastward components of wind speed at a height above the earth’s surface describe the direction of wind speed and might be relevant parameters in predicting the spread and distribution of Ae. albopictus. The northward component (v-component) of wind speed at 10 m above the earth’s surface captures the horizontal speed of winds moving toward the north, while the eastward component (u-component) captures the movement toward the east. A negative u-component and a negative v-component of wind speed indicate a southward and a westward movement of wind speed, respectively. In South America, high dengue fever incidences are associated with a low northward component of wind and a low magnitude of wind speed (Sedda et al., 2018).
Temperature and precipitation are major predictors of the habitat suitability of Ae. albopictus in Europe. The annual mean temperature and annual precipitation are bioclimatic variables that have been previously identified as major limiting factors to Ae. albopictus distribution and abundance (Fischer et al., 2011, Guzzetta et al., 2016). Several physiological traits of Ae. albopictus such as vector-biting rates, vector population dynamics, and larva and egg development largely depend on atmospheric thermal and moisture conditions (Delatte et al., 2009; Reinhold et al., 2018). Ecological niche models (ENMs) have used bioclimatic variables, which are derived from monthly temperature and rainfall values to generate biologically meaningful variables (Roiz et al., 2010) to model the habitat suitability of Ae. albopictus. Bioclimatic variables are commonly used in ENMs as they enhance or limit species distribution (Fick and Hijmans, 2017). Many recent MaxEnt models of Ae. albopictus have trained their models using a selected set of these parameters (Ibáñez-Justicia et al., 2020). Temperature-related parameters commonly have higher contributions to the predictions of the geographical distribution of Ae. albopictus than moisture-related parameters (Ding et al., 2018). An explanation for this finding is that Ae. albopictus can establish in areas where water is being stored in open containers and small natural or artificial habitats even in the absence of rainfall (Vezzani, 2007). In dry urban areas in Europe, human water storage in containers compensates for the non-availability of natural breeding sites for egg deposition (Medlock et al., 2015), while heavy rainfall can result in a population loss of Ae. albopictus through flushing out eggs, larvae, and pupae (Dieng et al., 2012). Yet, sufficient precipitation is needed in the summer months for the sustenance of water stored in natural aquatic habitats (Cunze et al., 2016). Additional moisture-related parameters taken into consideration in recent modeling studies of Ae. albopictus include absolute humidity, relative humidity, and the enhanced vegetation index (EVI) (Kraemer et al., 2015; Ding et al., 2018). Absolute humidity measures the density of water vapor in the atmosphere, while relative humidity relates this measure to the maximum humidity of the atmosphere. Due to the dependence of relative humidity on temperature and saturation vapor pressure, which leads to strong linear correlations among these parameters, absolute humidity has been proposed as a better proxy of the atmospheric moisture content in global distribution models of Ae. albopictus (Dickens et al., 2018). Absolute humidity is a useful predictor of the suitability of Ae. albopictus in areas of high atmospheric moisture availability such as in coastal areas. The survival and establishment success of Ae. albopictus are highly dependent on sufficient moisture properties of the atmosphere. The enhanced vegetation index (EVI) is a proxy for vegetation canopy greenness and soil surface moisture levels. EVI is a useful predictor of mosquito development sites having sufficient soil moisture levels and vegetation canopy cover that are essential for the survival of the eggs and larvae of Ae. albopictus (Kraemer et al., 2015).
Specific areas showing suitable climatic conditions for the establishment of Ae. albopictus but where no occurrence records of the vector were found, although monitoring is taking place (European Centre for Disease Prevention and Control (ECDC) and European Food Safety Authority (ESA, 2021b)), are referred to as suitable non-occurrence areas of Ae. albopictus. In modeling terminology, these areas are referred to as the false-positive rates of model predictions. In Europe, suitable non-occurrence areas of Ae. albopictus have been identified mainly in Spain and Portugal (Kraemer et al., 2015; European Centre for Disease Prevention and Control (ECDC) and European Food Safety Authority (ESA, 2021a)). They are also observed in other regions of the world, for example, in the western coast of North America, in the northern part of South America, and in parts of Asia and Africa (Fischer et al., 2011; Kraemer et al., 2015). These areas have also been identified as high-risk areas for autochthonous transmission of chikungunya (Tjaden et al., 2021). The introduction of Ae. albopictus into new areas is engendered largely by human-mediated transport and importation of used tires from countries where the species is established (Thomas et al., 2014). Human-mediated transport overcomes major barriers (Lounibos et al., 2003), such as large rivers and mountains (e.g., River Rhine in Germany, River Rhone in France, and the Alps in Italy) that limit the spread and establishment of Ae. albopictus in Europe. However, it remains unclear whether high wind speed plays a role in limiting the establishment of Ae. albopictus in its climatic niche.
This study examines the potential of wind speed in predicting the non-occurrence of Ae. albopictus observed in otherwise climatically suitable areas for its establishment in Europe. It is hypothesized that wind speed is higher in the non-occurrence locations than in the occurrence locations of Ae. albopictus within its projected climatically suitable areas. The study also hypothesized that suitable non-occurrence areas of Ae. albopictus are decreased in a MaxEnt model calibrated with wind speed parameters compared to a calibration without wind speed parameters. Last, it is hypothesized that wind speed limits the contribution of the most important temperature-related and precipitation-related bioclimatic variables to habitat suitability predictions of Ae. albopictus in Europe.
The database of global geo-referenced locations of Ae. albopictus was obtained from the review of the literature (see Supplemental Reference list for a list of selected publications, Supplementary S6). The database consists of about 8,478 documented occurrences of Ae. albopictus over the period 1994–2020. To reduce spatial autocorrelation, the thin function from the spThin R package is used to separate these occurrences by 5 km. Finally, 7,207 occurrence locations remained at the end of the preparation and pre-processing step for modeling.
Bioclimatic variables (n = 19) representing temperature and rainfall values for the period 1970–2000 at a spatial resolution of 2.5 arcmin (Supplementary Table S1) were downloaded as raster layers from the WorldClim database (WorldClim version 2.1, 2020; Fick and Hijmans, 2017). WorldClim is a repository of high-resolution global data sets on historical and future climate conditions. Monthly averaged reanalysis data sets of wind speed and related wind data sets on the northward and eastward components of wind speed at 10-m height above the earth’s surface, as well as dew point temperature at 2 m above the earth’s surface, were downloaded from the ERA-Interim database (Hersbach et al., 2020). ERA5 is a database of global climate and weather reanalysis data sets for the period of 1 January 1979 to August 2019 obtained at a spatial resolution of 0.25°. The mean of the monthly wind data sets was calculated to obtain an annual average of wind speed, the annual average of the northward component of wind speed, and the annual average of the eastward component of wind speed, all at 10-m height over the whole time period. Absolute humidity was calculated from the ERA5 dew point temperature data using the Tetens formula (Tetens, 1930). A global raster layer of the enhanced vegetation index (EVI) was obtained from the LP DAAC database at a spatial resolution of 1 km (Didan, 2015). To ensure uniformity in spatial resolution and accuracy of model predictions, all data sets were rescaled to the 2.5 arcmin resolution of the bioclimatic variables.
An expert knowledge approach—which is based on insights from recent studies—is used to drop a set of bioclimatic variables, which have been identified as not useful for ecological niche models of Ae. albopictus. We removed bioclimatic variables, which are derived from other variables—for example, mean diurnal range, isothermality, and temperature seasonality—which can add potential complexity to the MaxEnt model (Tjaden et al., 2021). Other bioclimatic variables, which refer to the wettest or driest month or quarter, have been identified as having potential limitations for modeling species distribution in Europe because these months can refer either to winter or to summer in Europe (Tjaden et al., 2021). For instance, there are rainy winters and dry summers in southern Europe, while this condition is reversed for other parts of Europe where there are mostly dry winters and wet summers. We drop bioclimatic variables—such as the mean temperature of the wettest quarter, precipitation of the wettest month, and precipitation of the driest quarter. As a result, the following variables remained: annual mean temperature, maximum temperature of the warmest month, minimum temperature of the coldest month, temperature annual range, mean temperature of the warmest quarter, mean temperature of the coldest quarter, annual precipitation, precipitation of the warmest quarter, precipitation of the coldest quarter, wind speed, the enhanced vegetation index (EVI), northward (v-) component of wind speed, eastward (u-) component of wind speed, and absolute humidity (Supplementary Figure S3).
A statistical variable selection approach is used to select a set of relevant environmental parameters. First, the values of each of the raster layers of the environmental variables were extracted at the occurrence locations of Ae. albopictus for statistical analysis. To account for collinearity among the remaining parameters, we applied a Pearson correlation analysis using the stats package in R. The Pearson correlation analysis is a test of linear correlation between each pair of variables, with coefficients ranging from 0 to 1. The closer the linear correlation coefficient to 1, the higher was the correlation. Here, we set a threshold of 0.7 and dropped one of a pair of variables having correlation coefficients greater than this threshold (Dormann et al., 2013). As a result, the following variables were selected: annual mean temperature, maximum temperature of the warmest month, annual precipitation, precipitation of the warmest quarter, precipitation of the coldest quarter, wind speed, EVI, northward (v-) component of wind speed, and eastward (u-) component of wind speed (Supplementary Figure S3).
We developed global ecological niche models for Ae. albopictus using a correlative modeling approach to compare suitable non-occurrence areas of Ae. albopictus in a model calibrated with wind speed parameters compared to a calibration without wind speed parameters. These models are denoted as Model-with-wind speed and Model-without-wind speed, respectively. The maximum entropy (MaxEnt) modeling approach is a widely used approach in ecology, biogeography, and conservation biology (Elith and Leathwick, 2009) to model disease vector distribution or vector-borne disease transmission (Peterson, 2014). MaxEnt models produce a prediction of species distribution, which has maximum entropy and is constrained by environmental conditions (Phillips et al., 2017). In studies estimating the impact of climate change on vector distribution, the most widely used modeling approach is MaxEnt (Tjaden et al., 2018). The MaxEnt is preferred to other machine learning algorithms because of its robustness to incomplete or biased occurrence records and correlated environmental variables. The MaxEnt is a presence-only model that takes as input a list of species presence locations, environmental variables, and a sample of background or pseudo-absence locations, which is usually generated over the entire spatial extent of the environmental variables (Merow et al., 2013). Here, we used a buffer-based approach as recommended by previous studies (VanDerWal et al., 2009); we generated 10,000 background points within a buffer of 1500 km around the global occurrence locations of Ae. albopictus. Varying buffer sizes from 1 to 5000 km were generated around these occurrence locations, and a series of test models were implemented and evaluated using the area under the receiver operating characteristic (AUC) curve of each model. The AUC of each model increases with increasing buffer sizes and stabilizes at 1500 km. Therefore, a buffer radius of 1500 km was determined as appropriate for the generation of background points for modeling (Supplementary Figure S4). Outputs of MaxEnt models are maps of relative “environmental suitability” ranging from 0 to 1, representing the probability of the presence of Ae. albopictus.
Model-with-wind speed is trained with global occurrence records of Ae. albopictus and a selected set of relevant environmental variables including wind speed-related parameters. Model-without-wind speed is trained with the same records and environmental parameters, however excluding wind speed-related parameters. Global habitat suitability maps of Ae. albopictus were generated from both models and projected to current habitat suitability conditions in Europe. The global models were developed using the ENMeval package in R with the latest MaxEnt version (3.4.4). The ENMeval package offers the benefit of choosing an optimal model from a range of models defined with several combinations of parameter settings (Phillips et al., 2017). Several models were created based on two MaxEnt parameter settings, namely, the regularization multiplier and feature class combinations. A total of ten regularization estimates (0.5–5; by 0.5 increments) and five feature class combinations (L, LQ, LQH, LQP, and LQT, where L = linear, Q = quadratic, P = product, H = hinge, and T = threshold) were created. The model combinations were then compared based on the average test omission rate of each of the models. Here, the model with the lowest average test omission rate is selected as an optimal model. The optimal model among models trained with wind speed-related parameters takes the linear, quadratic, and hinge (LQH) feature classes and has a regularization multiplier of 4.5. When the optimal model among models trained without wind speed-related parameters also takes the linear, quadratic, and hinge (LQH) feature classes, it has a regularization multiplier of 1.5. The presence and background records of Ae. albopictus were hierarchically partitioned using the checkerboard2 cross-validation method, which splits the localities into k = 4 spatial groups for model evaluation. Here, each of the model combinations is iteratively run (k times) with k-1 localities used as training samples and the remaining localities used as validation samples. Afterward, model evaluation metrics are averaged over the total number of model iterations (Muscarella et al., 2014). The aim of partitioning the localities is to reduce spatial autocorrelation between training and validation samples, which may lead to the overestimation of model evaluation metrics (Veloz, 2009).
The predictive performance of MaxEnt models is estimated using the partial area under the receiver operating characteristic curve (pAUC), which is a better metric for model evaluation than the traditional area under the receiver operating characteristic curve (AUC) (Peterson et al., 2008). The pAUC is derived from the traditional AUC and focuses only on specific parts of the receiver operating characteristic curve, which are relevant in estimating the discriminatory power of a model. We calculated the pAUC using the function “kuenm_proc” from the “kuenm” package in R (Cobos et al., 2019). After partitioning the occurrence data into 25% testing data and 75% training data, the function generates 1,000 bootstrap simulations of the pAUC, which were then averaged to get a mean value. The standardized pAUC ranges from 0.5 to 1, where 0.5 indicates random model discrimination, and 1 indicates excellent model discrimination (McClish, 1989). The importance of each environmental variable for model predictions was assessed from the permutation importance feature of MaxEnt models.
The habitat suitability maps are classified using three established threshold probability values, namely, the 5-percentile thresholds, the 10-percentile thresholds, and the equal sensitivity–specificity threshold (Tjaden et al., 2021). The 5-percentile or 10-percentile thresholds were obtained by dropping the 5 or 10 per cent of the lowest habitat suitability values (associated with the occurrence locations of Ae. albopictus) and using the minimum of the remaining suitability values as the threshold (Bean et al., 2012). The equal sensitivity–specificity (eqSS) threshold is the threshold at which sensitivity (true-positive rate) and specificity (true-negative rate) predictions are equal in MaxEnt models. All thresholds are derived separately for each of the MaxEnt models and applied individually for the classification of the suitability maps. For easier interpretations of the habitat suitability maps, we assigned the following tags to the classes defined by the thresholds: “very high habitat suitability” (≥eqSS), “high habitat suitability” (≥10 percentile), and “medium habitat suitability” (≥5 percentile).
To compare wind speed between non-occurrence and occurrence areas of Ae. albopictus within its projected climatically suitable areas, a random sample of non-occurrence points was generated within monitored areas (active or passive) by the European Centre for Disease Prevention and Control (ECDC) and European Food Safety Authority (ESA, 2021b), with at least medium habitat suitability. A random sample of non-occurrence of the same number as the occurrence points were generated. A simple raster extraction method was performed to extract wind speed values from the raster layer at the cells in which the occurrence and non-occurrence points fall. A Student two-sample t-test was then performed to test for significant differences in wind speed between the occurrence and non-occurrence areas of Ae. albopictus within its climatic niche in Europe. To get more robust estimates, we generated 1,000 bootstrap simulations of the result from the two-sample t-test and calculated the average of these values.
Changes in environmental variable contribution between Model-with-wind speed and Model-without-wind speed were analyzed. Using a raster-to-value coercion method, we extracted the cell values from the raster objects of the predicted habitat suitability of Ae. albopictus in Europe, wind speed, and the most important temperature-related and precipitation-related bioclimatic variables. A multiple linear regression analysis with interaction terms was then performed to examine the relationship between the predicted habitat suitability of Ae. albopictus (dependent variable) and the most important temperature-related and precipitation-related bioclimatic variables. The interactions between these variables and wind speed were also added as interaction terms to the regression model. Finally, using the margins package in R2, we estimated the average marginal effect of the most important temperature-related and precipitation-related bioclimatic variables, as well as wind speed, on the predicted habitat suitability of Ae. albopictus. The average marginal effect (AME) was estimated to determine the percentage of change in the predicted habitat suitability of Ae. albopictus, given a unit change in temperature, precipitation, and wind speed. We also estimated the impact of increasing values of wind speed (0–8 ms−1; by 0.5 increments) on the AME of temperature and precipitation. This was estimated by taking the sum of the beta coefficients of each parameter and the beta coefficients of the interaction term, multiplied by each increasing value of wind speed, as shown in Eq. 1. We also estimated the standard errors of the obtained values using the msm package in R (Mandel, 2013).
where y = average marginal effect of temperature or precipitation
a = beta coefficient of temperature or precipitation
b = beta coefficient of the interaction term
x = increasing values of wind speed (0 to 8ms−1; by 0.5 increments)
All modelling, geospatial and statistical analyses were performed using the R statistical software version 4.0.2 (R Core Team, 2020, Supplementary S6). All figures and maps were prepared using the ggplot2 package in R (Wickham, 2016) and the QGIS version 3.4.
Wind speeds in areas with established or introduced Ae. albopictus populations (Natural Earth3 2021, continental Europe excluding Russia) are decreasing from north to south (Figure 1, wind speed invasive mosquito surveillance regions; see Supplementary Figure S1). We observed that very low (<1.5 ms−1) to moderate (1.5–4.5 ms−1) wind speed prevails in most areas with 109,000 km2 and 5,000,000 km2, respectively. Areas with very high wind speed (above 4.5 ms−1) only span about 305,000 km2 and are found in Italy (Sassari, Lecce), France (Bouches-du-Rhone), Spain (Cadiz), Portugal (Algarve), the Netherlands (Kop van Nord-Holland), Greece (Attiki, Lakonia), Bulgaria (Burga), and the United Kingdom (East Kent).
FIGURE 1. Wind speed (ms−1) in areas with established or introduced Aedes albopictus populations. Most areas where the mosquito has become established show wind speeds ranging from 1.5 ms−1 to 4.5 ms−1. The wind speed values represent air movement at 10-m height and were acquired from the ERA5 monthly average reanalysis data set for the period of 1999–2018 (Copernicus Climate Change Service, 2021). The establishment status across Europe was adopted from the ECDC/EFSA5 known distribution of the species (March 2021).
Based on the Model-with-wind speed, very high habitat suitability of Ae. albopictus is observed in the southern part of Europe for Italy, western France, Portugal, northern Spain, Greece, and along the coastlines of Croatia, Montenegro, Albania, Macedonia, and Bosnia and Herzegovina and in Slovenia (Figure 2). We observed medium habitat suitability conditions in central Europe for western Germany, Lichtenstein, Austria, Hungary, Switzerland, and Romania. In western Europe, medium habitat suitability is observed in Luxembourg, Belgium, the Netherlands, France, the United Kingdom, and Ireland.
FIGURE 2. Categorized habitat suitability map of Aedes albopictus in Europe under current climatic conditions based on an ecological niche model (Model-with-wind speed) of 7,207 global occurrence localities. Explanatory parameters used for model training include bioclimatic variables (derived from the WorldClim database6, the enhanced vegetation index (derived from the LP DAAC database), and wind speed (derived from the ERA5 database). All databases were accessed on 2 September 2021. The habitat suitability map was classified into binary results, according to three established threshold probability values: the 5-percentile, 10-percentile, and the equal sensitivity and specificity threshold (EQSS).
The false-positive rate of model predictions from Model-with-wind speed and Model-without-wind speed (Supplementary Figure S2a) is comparable with 0.335 and 0.349, respectively. Model performance assessed from the standardized partial AUC (pAUC) estimate of the ENMs showed that the two models had almost similar discriminatory power, with Model-with-wind speed and Model-without-wind speed having mean pAUC values of 0.937 ± 0.002 SD and 0.930 ± 0.002 SD, respectively. Both pAUC values are indicators of excellent model discrimination. This suggested that Model-with-wind speed performed only slightly better than Model-without-wind speed. However, we observed that some suitable non-occurrence areas of Ae. albopictus in Model-without-wind speed are predicted as unsuitable in Model-with-wind speed. These areas are found in Portugal, Spain, France, Hungary, Romania, and Bulgaria.
The most important parameters contributing to the performance of the ENMs are annual mean temperature and annual precipitation. Among temperature-related bioclimatic variables, annual mean temperature had the highest variable importance in terms of the permutation importance criteria in both models (Model-with-wind speed, 27.96%; Model-without-wind speed, 33.19%) (Table 1). Among precipitation-related bioclimatic variables, annual precipitation had the highest variable importance in terms of the permutation importance criteria in both models. (Model-with-wind speed, 17.55%; Model-without-wind speed, 18.87%). The permutation importance of wind speed is 8.23%, which was the highest contribution to model performance among the wind speed-related parameters.
TABLE 1. Permutation importance (%) of temperature- and precipitation-related bioclimatic variables used in the training of MaxEnt models. The annual mean temperature and annual precipitation are the most important temperature-related and precipitation-related bioclimatic variables in both models, followed by the enhanced vegetation index. The most important wind speed-related variable is wind speed. The most important variables in each category are highlighted in bold text.
The most important temperature- and precipitation-related bioclimatic variables contributing to the performance of the ENMs are annual mean temperature and annual precipitation. Our results showed that the AME values of both environmental parameters and wind speed on the habitat suitability predictions of Ae. albopictus are 0.0044, 0.0001, and −0.0316, respectively. This suggests that a unit change in the annual mean temperature and annual precipitation is associated with a 0.44 and 0.01% increase in the predicted habitat suitability of Ae. albopictus. However, a unit change in wind speed is associated with a 3.16% decrease in the predicted habitat suitability of Ae. albopictus. The AME of the annual mean temperature decreases with increasing values of wind speed, but the AME of annual precipitation increases (Figure 3).
FIGURE 3. Effects of increasing wind speed on the average marginal effect of (A) the annual mean temperature and (B) the annual precipitation on the predicted habitat suitability of Aedes albopictus. The average marginal effect of the annual mean temperature decreases with increasing wind speed, and the average marginal effect of the annual precipitation increases.
The difference in wind speed between real occurrence and non-occurrence of Ae. albopictus within areas modeled as suitable (i.e., ≥5-percentile threshold in Model-with-wind speed) for mosquito establishment is significant (p < 0.05). Average wind speed in the suitable non-occurrence localities (3.12 ms-1 ± 0.04 SD) is higher than in occurrence localities (2.54 ms-1 ± 0.04 SD) (Figure 4). It is also observed that maximum values of wind speed are higher in areas where Ae. albopictus is not found (7.01 ms-1) than in the areas where it is found (5.45 ms-1). Wind speed values in the suitable non-occurrence group are highly clustered above the mean wind speed in the suitable occurrence group.
FIGURE 4. Differences in wind speed (ms−1) between occurrence and non-occurrence areas of Aedes albopictus within its climatic niche in Europe based on the 5-percentile thresholded ecological niche model (Model-with-wind-speed, Supplementary Figure S2b). Mean, minimum, and maximum wind speeds in the suitable non-occurrence areas are significantly higher than those in the suitable occurrence areas.
The impact of high wind speed on mosquito distribution, flight range, and disease incidences has occasionally been observed in studies of vector mosquitoes (Kerkow et al., 2019; Mala and Jat, 2019), but it remains unclear whether high wind speed explains the non-occurrence of Ae. albopictus within its climatic niche in Europe. In this study, wind speed at real occurrence localities of Ae. albopictus was compared with wind speed at non-occurrence localities within areas having suitable climatic conditions for the establishment of the vector mosquito. In addition, two global ENMs were developed to predict the current habitat suitability conditions of Ae. albopictus in Europe (Model-with-wind speed and Model-without-wind speed).
Within the modeled suitable area, the mean and maximum values of wind speed are higher in areas where Ae. albopictus is not established than in areas in Europe where it is already established. This result provides initial evidence that wind speed has a negative impact on the ability of Ae. albopictus to establish. The occurrence and abundance of Ae. albopictus were negatively associated with wind speed based on a mixed-effect zero-inflated negative binomial regression of mosquito data comprising 200,000 trap days from 2004 to 2018 in Florida (Yang et al., 2021). Non-occurrence of the species in a climatically suitable area depends on diverse factors. Mosquitoes are actively or passively monitored (European Centre for Disease Prevention and Control (ECDC) and European Food Safety Authority (ESA, 2021b)); otherwise, non-occurrence cannot be understood as a confirmed absence. Increasing the mean wind speed results in fewer submissions when mosquitoes are passively monitored, that is by citizen science projects as high wind speed prevents volunteers from collecting mosquitoes outside (Pernat et al., 2021). Climatically suitable locations need to be accessible for the species, and barriers such as dense vegetation or open water do not hinder the dispersal process (Verdonschot and Besse-Lototskaya, 2013). The accessibility of a climatically suitable area is also dependent on the dispersal and flight capacity of the mosquito and passive transport. Ae. albopictus is known to have a weak dispersal capacity with an average maximum flight distance of 676 m and an average flight distance of 75 m based on mark–recapture experiments (Verdonschot and Besse-Lototskaya, 2013). The likelihood of the introduction of the vector via human-mediated transport of goods is increased in areas with high amounts of freight imports from the mosquito’s endemic countries via harbors and railways (Thomas et al., 2014) in Europe. Bennett et al. (2019) found a high infestation rate in garages trading used tires along the highways, allowing for a rapid dispersal across Panama. Up until now, the mosquito has primarily expanded in association with humans and only to a limited extent on the active dispersal of female mosquitoes (Egizi et al., 2016; Trájer et al., 2017). Wind-assisted long-distance migratory flights as known for Anopheles vectors, with a simulated mean nightly displacement of up to 300 km for 9-h flight duration (Huestis et al., 2019), have not been shown for Ae. albopictus. In this study, only areas that were monitored according to European Centre for Disease Prevention and Control (ECDC) and European Food Safety Authority (ESA, 2021b) were considered in the analysis comparing the wind speed at non-occurrence and real occurrence of the species.
The wind speed data used for this comparison relate to the movement of air at 10-m height whose measurement considers land cover variables such as the local terrain, vegetation cover, and buildings. These landscape features make wind observations vary at small scales. However, the calculation of average wind speed from 1,000 bootstrap samples provides a robust estimate of the differences in wind speed that is not likely to vary if the analysis is repeated or new random points are generated. The set of wind speed-related parameters considered in this study includes wind speed at 10-m height and northward (v-) and eastward (u-) components of wind speed at 10-m height. Wind speed and the eastward (u-) component of wind speed contributed mostly to model performance. The eastward component of wind speed represents wind speed moving from west to east direction. Westerly winds (i.e., winds moving from west to east) are prevalent during the summer months in Europe.
We developed habitat suitability models of Ae. albopictus using recent global occurrence records of the mosquito and a set of relevant environmental parameters selected using expert knowledge and a statistical variable selection approach. In accordance with previous modeling results, we found very high habitat suitability of Ae. albopictus in southern Europe, areas that are also identified as high-risk regions for chikungunya transmission (Tjaden et al., 2021). Our model predictions also depict moderate habitat suitability in western and central Europe in accordance with Caminade et al. (2012) and Cunze et al. (2016). Low habitat suitability predictions are found for the majority of the countries in northern and eastern Europe (Ibáñez-Justicia et al., 2020).
We compared a model trained with wind speed parameters to a model trained without wind speed. The Model-with-wind speed shows a slightly higher model performance, and the average omission rate (false-positive fraction) is slightly lower. Although both models take the same set of feature classes, the Model-with-wind speed has a higher regularization multiplier (RM). This suggests that it is a simpler model as higher RM values impose significant constraints on model complexity. A higher RM value in association with a low average omission rate is also an indicator of a lower probability of overfitting in model predictions (Radosavljevic and Anderson, 2014). Our results indicated that notable suitable non-occurrence areas of Ae. albopictus found in the Model-without-wind speed are absent in the Model-with-windspeed. These areas are found in France, Hungary, Romania, Bulgaria, Spain, and Portugal with moderate to high wind speed and active ECDC surveillance systems.
Variable selection was based on a Pearson correlation analysis to check for collinearity among variables selected by an expert knowledge approach. The result of the Pearson collinearity test reveals that wind speed-related parameters have low correlations with other relevant environmental parameters. Consequently, the wind speed was added to the list of relevant parameters used in training the ENM of Ae. albopictus. Previous studies have dropped wind speed, according to the results of other statistical variable selection approaches. Using a simple correlation analysis and a principal component analysis for variable selection, Medley (2010) dropped wind speed from the list of relevant environmental parameters as it does not have significant correlations with the most important eigenvalues of the PCA. A jackknife test of variable importance also reveals that wind speed parameters achieved less significant training gains to the occurrence of Ae. albopictus in Europe. Kerkow et al. (2019) implemented a climate model for Ae. j. japonicus trained without wind speed; however, wind speed data selected based on expert knowledge were considered in a fuzzy modeling approach.
Temperature-related parameters had higher contributions than precipitation-related parameters, to predictions of the presence of Ae. albopictus in MaxEnt models. Recent species distribution models of Ae. albopictus have found similar results explaining that the survival and seasonal activity of the mosquito are more influenced by thermal environmental conditions than moisture conditions (Fischer et al., 2011; Caminade et al., 2012). An explanation for this finding is that artificial water storage in open containers compensates for the non-availability of natural breeding sites in arid areas or during dry seasons in humid areas (Cunze et al., 2016). However, precipitation is required for the long-term sustenance of small natural habitats and artificial containers that support egg deposition and larval development. Our findings support previous findings (e.g. Ding et al., 2018) that temperature is a better predictor of the presence of Ae. albopictus than precipitation.
Increasing wind speed decreases the AME of temperature on the predicted habitat suitability of Ae. albopictus but increases the AME of precipitation. This finding suggests that with increasing wind speed and constant annual mean temperatures in Europe, the predicted habitat suitability of Ae. albopictus decreases. The AME of temperature is positive; however, it becomes negative as it interacts with increasing wind speed values. Consequently, due to the interaction between these parameters, “suitable” predictions of Ae. albopictus become “unsuitable” predictions. This finding suggests that due to the interaction between temperature and wind speed, false-positive predictions of Ae. albopictus are adequately resolved in a statistical model trained with both parameters. As previously discussed, our results suggested that high wind speed values are found in suitable non-occurrence areas of Ae. albopictus and that these areas are absent in a model trained with wind speed. Therefore, a relevant deduction from these findings is that a model trained with wind speed parameters would better resolve false-positive predictions or suitable non-occurrence areas of Ae. albopictus.
This finding contributes to progress in understanding the interactions between amplifying environmental factors (such as temperature) and delimiting factors (such as wind speed) to predictions of Ae. albopictus distribution. This study focuses on the interactions between parameters in relation to the predicted habitat suitability of Ae. albopictus, while previous studies have mostly compared the individual correlations of wind speed, temperature, and precipitation with the densities of dengue vectors or dengue fever incidences. Depradine and Lovell (2004) found a significant negative correlation between wind speed and the incidence of dengue cases in the Caribbean Island of Barbados but significant positive correlations with temperature and precipitation. Khan et al. (2018) also found a significant negative correlation between wind speed and the abundance of dengue fever mosquitoes Aedes and Anopheles in Saudi Arabia but positive correlations with temperature and precipitation. As an example of studies that examined the densities of dengue vectors, Yin et al. (2019) identified a negative correlation between wind speed and densities of Ae. albopictus vectors in Guangzhou, China, but positive correlations with temperature and humidity.
In addition to the precipitation-related bioclimatic variables considered in this study, other moisture-related parameters that were considered as explanatory parameters of the global distribution of Ae. albopictus include absolute humidity and the enhanced vegetation index (EVI). According to the results of the statistical variable selection using the Pearson correlation analysis, absolute humidity showed high collinearity with other temperature-related bioclimatic variables. This variable was dropped from the list of relevant environmental parameters for modeling, although previous studies (e.g. Ding et al., 2018) have emphasized the usefulness of absolute humidity in global distribution models of Ae. albopictus. The EVI showed a very low correlation with other parameters; therefore, we trained the global models with this parameter. Our results suggested that the EVI had significant contributions in terms of permutation importance to predictions of Ae. albopictus in both models (Kraemer et al., 2015). Therefore, we recommended the use of the EVI in future global or regional models of Ae. albopictus as this variable characterizes the suitability of Ae. albopictus in habitats where evaporation and wind speed are minimal.
The ENM of Ae. albopictuswas trained with only environmental parameters. Land cover and demographic parameters such as accessibility, land use, urbanization, distance to human settlement, and population density, which have been suggested as relevant predictors of the distribution of Ae. albopictus, were not considered. Generally, the importance of these anthropogenic parameters for species distribution is perceived better on local scales than on regional scales (Pearson and Dawson, 2003). Moreover, studies of Ae. albopictus (e.g. Kraemer et al., 2015; Dickens et al., 2018; Ding et al., 2018) that consider these parameters have found higher contributions of climatic or environmental parameters to occurrence predictions of the mosquito.
This study faces the challenge of the non-availability of real absence records of Ae. albopictus in Europe. Therefore, we generated random background points (excluding the observed occurrence points) from areas where the mosquito is actively or passively monitored and regarded these as non-occurrence or absence records of Ae. albopictus. To compare wind speed at the occurrence and non-occurrence localities of Ae. albopictus, sample points were randomly selected. Inconsistent time coverage between the occurrence records of Ae. albopictus, bioclimatic variables, and the other environmental variables is a common problem. The bioclimatic variables cover a historical time period from 1970 to 2000, while the occurrence records and other environmental variables cover the time period from 1994 to 2020. However, since the bioclimatic variables were obtained from the most recent version of the WorldClim (i.e. version 2.1), we assumed that this historical time coverage provides a satisfactory representation of current conditions of global temperature and precipitation (Fick and Hijmans, 2017).
Future efforts may be geared toward obtaining absence records of Ae. albopictus, comparing wind speed in real occurrence with real absences, and applying presence–absence models to test the similar hypothesis. The observed non-occurrence of Ae. albopictus may also be due to the strict monitoring systems and control programs already in place in some European countries. For example, the implementation of a surveillance strategy in the Netherlands has greatly reduced the successful establishment of Ae. albopictus in Dutch tire companies and their surroundings (Ibáñez-Justicia et al., 2020). Hence, there is a need to investigate the role of monitoring systems and control programs in explaining non-occurrence of Ae. albopictus in Europe. A raster layer of meteorological wind direction can be obtained from the northward and eastward components of wind speed4,5,6. With such a raster data set of meteorological wind direction, future studies may investigate more closely the direction of high wind speed found in suitable non-occurrence regions of Ae. albopictus. In future, local agent-based models that aim at projecting the pathways of spreading of the species can include wind direction toward suitable vegetation or land cover to test past spreading tendencies and estimate future areas at risk for establishment.
In summary, our findings indicated that wind speed is a limiting environmental factor to the occurrence or successful establishment of Ae. albopictus in Europe. Our findings are relevant for future efforts to limit the spread and dispersal of Ae. albopictus by considering wind speed when planning the implementation of mosquito surveillance and developing early warning systems for mosquito-borne diseases in Europe.
The datasets generated and analyzed for this study can be obtained by contacting the corresponding author.
ST and EA conceptualized the study, wrote the original draft, and wrote, reviewed, and edited the manuscript. EA performed the data analysis. EA and RS visualized the results. ST and CB supervised the study and acquired the funding. ST was responsible for project management. All authors reviewed and edited the manuscript and have read and agreed to the published version of the manuscript.
This research was funded through the 2018–2019 BiodivERsA joint call for research proposals, under the BiodivERsA3 ERA-Net COFUND program, and with the funding organization DFG, German Research Foundation (BE 2192/18-1). This research was funded by the Deutsche Forschungsgemeinschaft (DFG, German Research Foundation)—491183248.
The authors declare that the research was conducted in the absence of any commercial or financial relationships that could be construed as a potential conflict of interest.
All claims expressed in this article are solely those of the authors and do not necessarily represent those of their affiliated organizations, or those of the publisher, the editors, and the reviewers. Any product that may be evaluated in this article, or claim that may be made by its manufacturer, is not guaranteed or endorsed by the publisher.
Marc Q. Benedict kindly provided the original data set of Aedes albopictus occurrence until 2007. Franziska Niemitz collected further data within her master’s thesis until 2010. Dr. Dominik Fischer updated the data until 2013. Dr. Nils Tjaden quality-checked the data and updated the data until April 2021.
The Supplementary Material for this article can be found online at: https://www.frontiersin.org/articles/10.3389/fenvs.2022.846243/full#supplementary-material
1http://www.iucngisd.org/gisd/.
2https://www.semanticscholar.org/paper/Interpreting-Regression-Results-using-Average-with-Leeper/9615c76bd5d81f7ebbbdac9714619863dc3a2337.
3https://www.naturalearthdata.com/downloads/10m-cultural-vectors/10m-admin-0-countries/.
4http://mst.nerc.ac.uk/wind_vect_convs.html.
5https://www.ecdc.europa.eu/en/publications-data/aedes-albopictus-current-known-distribution-march-2021.
Bean, W. T., Stafford, R., and Brashares, J. S. (2012). The Effects of Small Sample Size and Sample Bias on Threshold Selection and Accuracy Assessment of Species Distribution Models. Ecography 35 (3), 250–258. doi:10.1111/j.1600-0587.2011.06545.x
Benedict, M. Q., Levine, R. S., Hawley, W. A., and Lounibos, L. P. (2007). Spread of the Tiger: Global Risk of Invasion by the MosquitoAedes Albopictus. Vector-Borne Zoonotic Dis. 7 (1), 76–85. doi:10.1089/vbz.2006.0562
Bennett, K. L., Gómez Martínez, C., Almanza, A., Rovira, J. R., McMillan, W. O., Enriquez, V., et al. (2019). High infestation of invasive Aedes mosquitoes in used tires along the local transport network of Panama. Parasit. Vectors 12 (1), 165. doi:10.1002/qj.3803
Bibbs, C. S., Kline, J., Kline, D. L., Estaver, J., Strohschein, R., Allan, S. A., et al. (2020). Olfactometric Comparison of the Volatile Insecticide, Metofluthrin, through Behavioral Responses of Aedes albopictus (Diptera: Culicidae). J. Med. Entomology 57 (1), 17–24. doi:10.1093/jme/tjz160
Camargo, C., Alfonso-Parra, C., Díaz, S., Rincon, D. F., Ramírez-Sánchez, L. F., Agudelo, J., et al. (2021). Spatial and Temporal Population Dynamics of Male and Female Aedes albopictus at a Local Scale in Medellín, Colombia. Parasites Vectors 14 (1), 312. doi:10.1186/s13071-021-04806-2
Caminade, C., Medlock, J. M., Ducheyne, E., McIntyre, K. M., Leach, S., Baylis, M., et al. (2012). Suitability of European Climate for the Asian Tiger Mosquito Aedes albopictus : Recent Trends and Future Scenarios. J. R. Soc. Interface. 9 (75), 2708–2717. doi:10.1098/rsif.2012.0138
Cardé, R. T. (2015). Multi-Cue Integration: How Female Mosquitoes Locate a Human Host. Curr. Biol. 25 (18), R793–R795. doi:10.1016/j.cub.2015.07.057
Che Dom, N., Faiz Madzlan, M., Nadira Yusoff, S. N., Hassan Ahmad, A., Ismail, R., and Nazrina Camalxaman, S. (2016). Profile Distribution of juvenileAedesspecies in an Urban Area of Malaysia. Trans. R. Soc. Trop. Med. Hyg. 110 (4), 237–245. doi:10.1093/trstmh/trw015
Cobos, M. E., Peterson, A. T., Barve, N., and Osorio-Olvera, L. (2019). Kuenm: An R Package for Detailed Development of Ecological Niche Models Using Maxent. PeerJ 7, e6281. doi:10.7717/peerj.6281
Copernicus Climate Change Service (2021). Global bioclimatic indicators from 1979 to 2018 derived from reanalysis. doi:10.24381/CDS.BCE175F0
Cunze, S., Kochmann, J., Koch, L. K., and Klimpel, S. (2016). Aedes albopictus and its Environmental Limits in Europe. PLOS ONE 11 (9), e0162116. doi:10.1371/journal.pone.0162116
Delatte, H., Gimonneau, G., Triboire, A., and Fontenille, D. (2009). Influence of Temperature on Immature Development, Survival, Longevity, Fecundity, and Gonotrophic Cycles of Aedes Albopictus, Vector of Chikungunya and Dengue in the Indian Ocean. J. Med. Entomol. 46 (1), 33–41. doi:10.1603/033.046.0105
Depradine, C., and Lovell, E. (2004). Climatological Variables and the Incidence of Dengue Fever in Barbados. Int. J. Environ. Health Res. 14 (6), 429–441. doi:10.1080/09603120400012868
Dickens, B. L., Sun, H., Jit, M., Cook, A. R., and Carrasco, L. R. (2018). Determining Environmental and Anthropogenic Factors Which Explain the Global Distribution of Aedes aegypti and Ae. albopictus. BMJ Glob. Health 3 (4), e000801. doi:10.1136/bmjgh-2018-000801
Didan, K., K. (2015). MOD13A2 MODIS/Terra Vegetation Indices 16-Day L3 Global 1km SIN Grid V006. [Data set]. NASA EOSDIS Land Processes DAAC. doi:10.5067/MODIS/MOD13A2.006
Dieng, H., Rahman, G. M. S., Abu Hassan, A., Che Salmah, M. R, Satho, T., Miake, T., et al. (2012). The Effects of Simulated Rainfall on Immature Population Dynamics of Aedes albopictus and Female Oviposition. Int. J. Biometeorol. 56 (1), 113–120. doi:10.1007/s00484-011-0402-0
Ding, F., Fu, J., Jiang, D., Hao, M., and Lin, G. (2018). Mapping the Spatial Distribution of Aedes aegypti and Aedes albopictus. Acta Trop. 178, 155–162. doi:10.1016/j.actatropica.2017.11.020
Dormann, C. F., Elith, J., Bacher, S., Buchmann, C., Carl, G., Carré, G., et al. (2013). Collinearity: A Review of Methods to Deal with it and a Simulation Study Evaluating Their Performance. Ecography 36, 27–46. doi:10.1111/j.1600-0587.2012.07348.x
Egizi, A., Kiser, J., Abadam, C., and Fonseca, D. M. (2016). The Hitchhiker's Guide to Becoming Invasive: Exotic Mosquitoes Spread Across a US State by Human Transport not Autonomous Flight. Mol. Ecol. 25 (13), 3033–3047. doi:10.1111/mec.13653
Eichelberger, S., Mccaa, J., Nijssen, B., and Wood, A. (2018). Climate Change Effects on Wind Speed. NAm Wind 7, 68–72.
Elbers, A. R. W., Koenraadt, C., and Meiswinkel, R. (2015). Mosquitoes and Culicoides Biting Midges: Vector Range and the Influence of Climate Change. Rev. Sci. Tech. OIE 34 (1), 123–137. doi:10.20506/rst.34.1.2349
Elith, J., and Leathwick, J. R. (2009). Species Distribution Models: Ecological Explanation and Prediction across Space and Time. Annu. Rev. Ecol. Evol. Syst. 40 (1), 677–697. doi:10.1146/annurev.ecolsys.110308.120159
European Centre for Disease Prevention and Control ECDC and European Food Safety Authority ESA (2021a). Aedes albopictus—Current Known Distribution-March Stockholm. https://www.ecdc.europa.eu/en/publications-data/aedes-albopictus-current-known-distribution-march-2021 (Accessed on November 10, 2021)
European Centre for Disease Prevention and Control ECDC and European Food Safety Authority ESA (2021b). Surveillance for Invasive Mosquitoes—Stockholm. https://ecdc.europa.eu/en/disease-vectors/surveillance-and-disease-data/mosquito-maps (Accessed on April 20, 2021)
Fick, S. E., and Hijmans, R. J. (2017). WorldClim 2: New 1‐km Spatial Resolution Climate Surfaces for Global Land Areas. Int. J. Climatol. 37 (12), 4302–4315. doi:10.1002/joc.5086
Fischer, D., Thomas, S. M., Neteler, M., Tjaden, N. B., and Beierkuhnlein, C. (2014). Climatic Suitability of Aedes albopictus in Europe Referring to Climate Change Projections: Comparison of Mechanistic and Correlative Niche Modelling Approaches. Eurosurveillance 19 (6), 20696. doi:10.2807/1560-7917.ES2014.19.6.20696
Fischer, D., Thomas, S. M., Niemitz, F., Reineking, B., and Beierkuhnlein, C. (2011). Projection of Climatic Suitability for Aedes albopictus Skuse (Culicidae) in Europe under Climate Change Conditions. Glob. Planet. Change 78 (1–2), 54–64. doi:10.1016/j.gloplacha.2011.05.008
Guzzetta, G., Montarsi, F., Baldacchino, F. A., Metz, M., Capelli, G., Rizzoli, A., et al. (2016). Potential Risk of Dengue and Chikungunya Outbreaks in Northern Italy Based on a Population Model of Aedes albopictus (Diptera: Culicidae). PLoS Negl. Trop. Dis. 10 (6), e0004762. doi:10.1371/journal.pntd.0004762
Hersbach, H., Bell, B., Berrisford, P., Hirahara, S., Horányi, A., Muñoz‐Sabater, J., et al. (2020). The ERA5 Global Reanalysis. Q.J.R. Meteorol. Soc. 146 (730), 1999–2049. doi:10.1002/qj.3803
Huestis, D. L., Dao, A., Diallo, M., Sanogo, Z. L., Samake, D., Yaro, A. S., et al. (2019). Windborne Long-Distance Migration of Malaria Mosquitoes in the Sahel. Nature 574 (7778), 404–408. doi:10.1038/s41586-019-1622-4
Ibáñez-Justicia, A., Alcaraz-Hernández, J. D., van Lammeren, R., Koenraadt, C. J. M., Bergsma, A., Delucchi, L., et al. (2020). Habitat Suitability Modelling to Assess the Introductions of Aedes albopictus (Diptera: Culicidae) in the Netherlands. Parasites Vectors 13 (1), 217. doi:10.1186/s13071-020-04077-3
IPCC (2013). The Physical Science Basis. Working Group I Contribution to the Fifth Assessment Report of the IPCC. Cambridge: Cambridge University Press.
ISSG (2020). Global Invasive Species Database. http://www.iucngisd.org/gisd/.
Kaufmann, C., Collins, L., and Brown, M. (2013). Influence of Age and Nutritional Status on Flight Performance of the Asian Tiger Mosquito Aedes albopictus (Diptera: Culicidae). Insects 4 (3), 404–412. doi:10.3390/insects4030404)
Kerkow, A., Wieland, R., Koban, M. B., Hölker, F., Jeschke, J. M., Werner, D., et al. (2019). What Makes the Asian Bush Mosquito Aedes Japonicus Japonicus Feel Comfortable in Germany? A Fuzzy Modelling Approach. Parasites Vectors 12 (1), 106. doi:10.1186/s13071-019-3368-0
Khan, M. A., Elhossary, S., Khan, I. A., Zahrani, M. H. A., Zahrani, F. S. A., and Bashri, F. M. A. (2018). The Impact of Climatic Variables with GIS Application on the Abundance of Medically Important Mosquitoes (Diptera: Culicidae) in Jeddah, Saudi Arabia. Int. J. Mosquito Res. 5 (5), 12–18. http://www.dipterajournal.com/archives/2018/5/5/A/5-3-23.
Kraemer, M. U. G., Reiner, R. C., Brady, O. J., Messina, J. P., Gilbert, M., Pigott, D. M., et al. (2019). Past and Future Spread of the Arbovirus Vectors Aedes aegypti and Aedes albopictus. Nat. Microbiol. 4 (5), 854–863. doi:10.1038/s41564-019-0376-y
Kraemer, M. U., Sinka, M. E., Duda, K. A., Mylne, A. Q., Shearer, F. M., Barker, C. M., et al. (2015). The Global Distribution of the Arbovirus Vectors Aedes aegypti and Ae. albopictus. ELife 4, e08347. doi:10.7554/eLife.08347
Lounibos, L. P., Escher, R. L., and Lourenço-De-Oliveira, R. (2003). Asymmetric Evolution of Photoperiodic Diapause in Temperate and Tropical Invasive Populations of Aedes albopictus (Diptera: Culicidae). Ann. Entomol. Soc. Am. 96 (4), 512–518. doi:10.1603/0013-8746(2003)096[0512:aeopdi]2.0.co;2
Mala, S., and Jat, M. K. (2019). Geographic Information System Based Spatio-Temporal Dengue Fever Cluster Analysis and Mapping. Egypt. J. Remote Sens. Space Sci. 22 (3), 297–304. doi:10.1016/j.ejrs.2019.08.002
Mandel, M. (2013). Simulation-Based Confidence Intervals for Functions with Complicated Derivatives. Am. Statistician 67, 76–81. doi:10.1080/00031305.2013.783880
McClish, D. K. (1989). Analyzing a Portion of the ROC Curve. Med. Decis. Mak. 9, 190–195. doi:10.1177/0272989X8900900307
Medeiros, M. C. I., Boothe, E. C., Roark, E. B., and Hamer, G. L. (2017). Dispersal of Male and Female Culex quinquefasciatus and Aedes albopictus Mosquitoes Using Stable Isotope Enrichment. PLoS Negl. Trop. Dis. 11 (1), e0005347. doi:10.1371/journal.pntd.0005347
Medley, K. A. (2010). Niche Shifts during the Global Invasion of the Asian Tiger Mosquito,Aedes albopictusSkuse (Culicidae), Revealed by Reciprocal Distribution Models. Glob. Ecol. Biogeogr. 19 (1), 122–133. doi:10.1111/j.1466-8238.2009.00497.x
Medlock, J. M., Hansford, K. M., Versteirt, V., Cull, B., Kampen, H., Fontenille, D., et al. (2015). An Entomological Review of Invasive Mosquitoes in Europe. Bull. Entomol. Res. 105 (6), 637–663. doi:10.1017/S0007485315000103
Merow, C., Smith, M. J., and Silander, J. A. (2013). A Practical Guide to MaxEnt for Modeling Species' Distributions: what it Does, and Why Inputs and Settings Matter. Ecography 36 (10), 1058–1069. doi:10.1111/j.1600-0587.2013.07872.x
Muscarella, R., Galante, P. J., Soley-Guardia, M., Boria, R. A., Kass, J. M., Uriarte, M., et al. (2014). ENMeval: An R Package for Conducting Spatially Independent Evaluations and Estimating Optimal Model Complexity forMaxentecological Niche Models. Methods Ecol. Evol. 5 (11), 1198–1205. doi:10.1111/2041-210X.12261
Pearson, R. G., and Dawson, T. P. (2003). Predicting the Impacts of Climate Change on the Distribution of Species: Are Bioclimate Envelope Models Useful? Glob. Ecol. Biogeogr. 12 (5), 361–371. doi:10.1046/j.1466-822x.2003.00042.x
Pereira-dos-Santos, T., Roiz, D., Lourenço-de-Oliveira, R., and Paupy, C. (2020). A Systematic Review: Is Aedes albopictus an Efficient Bridge Vector for Zoonotic Arboviruses? Pathogens 9 (4), 266. doi:10.3390/pathogens9040266
Pernat, N., Kampen, H., Ruland, P., Jeschke, J. M., and Werner, D. (2021). Drivers of Spatio-Temporal Variation in Mosquito Submissions to the Citizen Science Project 'Mückenatlas'. Sci. Rep. 11 (1), 1395. doi:10.1038/s41598-020-80365-3
Peterson, A. T. (2014). Mapping Disease Transmission Risk in Geographic and Ecological Contexts (Baltimore: Johns Hopkins University Press).
Peterson, A. T., Papeş, M., and Soberón, J. (2008). Rethinking Receiver Operating Characteristic Analysis Applications in Ecological Niche Modeling. Ecol. Model. 213 (1), 63–72. doi:10.1016/j.ecolmodel.2007.11.008
Phillips, S. J., Anderson, R. P., Dudík, M., Schapire, R. E., and Blair, M. E. (2017). Opening the Black Box: An Open-Source Release of Maxent. Ecography 40 (7), 887–893. doi:10.1111/ecog.03049
Provost, M. W. (1952). The Dispersal of Aedes Taeniorhynchus. I. Preliminary Studies. Mosq. News 12 (3), 174–190. www.biodiversitylibrary.org/part/128038.
R Core Team (2020). A Language and Environment for Statistical Computing. Vienna, Austria: R Foundation for Statistical Computing. https://www.R-project.org/.
Radosavljevic, A., and Anderson, R. P. (2014). Making Better Maxent Models of Species Distributions: Complexity, Overfitting and Evaluation. J. Biogeogr. 41 (4), 629–643. doi:10.1111/jbi.12227
Reinhold, J., Lazzari, C., and Lahondère, C. (2018). Effects of the Environmental Temperature on Aedes aegypti and Aedes albopictus Mosquitoes: A Review. Insects 9 (4), 158. doi:10.3390/insects9040158
Roiz, D., Rosà, R., Arnoldi, D., and Rizzoli, A. (2010). Effects of Temperature and Rainfall on the Activity and Dynamics of Host-SeekingAedes albopictusFemales in Northern Italy. Vector-Borne Zoonotic Dis. 10 (8), 811–816. doi:10.1089/vbz.2009.0098
Rosa-Freitas, M. G., Schreiber, K. V., Tsouris, P., Weimann, E. T. d. S., and Luitgards-Moura, J. F. (2006). Associations between Dengue and Combinations of Weather Factors in a City in the Brazilian Amazon. Rev. Panam. Salud Publica 20 (4), 256–267. doi:10.1590/s1020-49892006000900006
Sallam, M., Fizer, C., Pilant, A., and Whung, P.-Y. (2017). Systematic Review: Land Cover, Meteorological, and Socioeconomic Determinants of Aedes Mosquito Habitat for Risk Mapping. Ijerph 14 (10), 1230. doi:10.3390/ijerph14101230
Sedda, L., Vilela, A. P. P., Aguiar, E. R. G. R., Gaspar, C. H. P., Gonçalves, A. N. A., Olmo, R. P., et al. (2018). The Spatial and Temporal Scales of Local Dengue Virus Transmission in Natural Settings: A Retrospective Analysis. Parasites Vectors 11 (1), 79. doi:10.1186/s13071-018-2662-6
Service, M. W. (1980). Effects of Wind on the Behaviour and Distribution of Mosquitoes and Blackflies. Int. J. Biometeorol. 24 (4), 347–353. doi:10.1007/BF02250577
Thomas, S., Tjaden, N., Frank, C., Jaeschke, A., Zipfel, L., Wagner-Wiening, C., et al. (2018). Areas with High Hazard Potential for Autochthonous Transmission of Aedes Albopictus-Associated Arboviruses in Germany. Ijerph 15 (6), 1270. doi:10.3390/ijerph15061270
Thomas, S., Tjaden, N., Van den Bos, S., and Beierkuhnlein, C. (2014). Implementing Cargo Movement into Climate Based Risk Assessment of Vector-Borne Diseases. Ijerph 11 (3), 3360–3374. doi:10.3390/ijerph110303360
Tjaden, N. B., Caminade, C., Beierkuhnlein, C., and Thomas, S. M. (2018). Mosquito-borne Diseases: Advances in Modelling Climate-Change Impacts. Trends Parasitol. 34 (3), 227–245. doi:10.1016/j.pt.2017.11.006
Tjaden, N. B., Cheng, Y., Beierkuhnlein, C., and Thomas, S. M. (2021). Chikungunya beyond the Tropics: Where and when Do We Expect Disease Transmission in Europe? Viruses 13 (6), 1024. doi:10.3390/v13061024
Trájer, A., Hammer, T., Kacsala, I., Tánczos, B., Bagi, N., Padisák, J., et al. (2017). Decoupling of Active and Passive Reasons for the Invasion Dynamics of Aedes albopictus Skuse (Diptera: Culicidae): Comparisons of Dispersal History in the Apennine and Florida Peninsulas. J. Vector Ecol.: J. Soc. Vector Ecol. 42 (2), 233–242. doi:10.1111/jvec.12263
VanDerWal, J., Shoo, L. P., Graham, C., and Williams, S. E. (2009). Selecting Pseudo-absence Data for Presence-Only Distribution Modeling: How Far Should You Stray from what You Know? Ecol. Model. 220 (4), 589–594. doi:10.1016/j.ecolmodel.2008.11.010
Vavassori, L., Saddler, A., and Müller, P. (2019). Active Dispersal of Aedes albopictus: A Mark-Release-Recapture Study Using Self-Marking Units. Parasites Vectors 12 (1), 583. doi:10.1186/s13071-019-3837-5
Veloz, S. D. (2009). Spatially Autocorrelated Sampling Falsely Inflates Measures of Accuracy for Presence-Only Niche Models. J. Biogeogr. 36 (12), 2290–2299. doi:10.1111/j.1365-2699.2009.02174.x
Verdonschot, P. F., and Besse-Lototskaya, A. A. (2014). Flight Distance of Mosquitoes (Culicidae): A Metadata Analysis to Support the Management of Barrier Zones Around Rewetted and Newly Constructed Wetlands. Limnologica 45, 69–79. doi:10.1016/J.LIMNO.2013.11.002
Vezzani, D. (2007). Review: Artificial Container-Breeding Mosquitoes and Cemeteries: a Perfect Match. Trop. Med. Int. Health 12 (2), 299–313. doi:10.1111/j.1365-3156.2006.01781.x
Wickham, H. (2016). “Programming with Ggplot2,” in Ggplot2: Elegant Graphics for Data Analysis. Editor H. Wickham (Switzerland: Springer International Publishing), 241–253. doi:10.1007/978-3-319-24277-4_12
Wong, G. K. L., and Jim, C. Y. (2017). Urban-microclimate Effect on Vector Mosquito Abundance of Tropical Green Roofs. Build. Environ. 112, 63–76. doi:10.1016/j.buildenv.2016.11.028
Keywords: Asian tiger mosquito, ecological niche models, chikungunya, dengue, climate change, habitat suitability, wind speed, zoonoses
Citation: Adeleke ED, Shittu RA, Beierkuhnlein C and Thomas SM (2022) High Wind Speed Prevents the Establishment of the Disease Vector Mosquito Aedes albopictus in Its Climatic Niche in Europe. Front. Environ. Sci. 10:846243. doi: 10.3389/fenvs.2022.846243
Received: 30 December 2021; Accepted: 11 April 2022;
Published: 08 June 2022.
Edited by:
Sen Li, Huazhong University of Science and Technology, ChinaReviewed by:
Flávia Virginio, Butantan Institute, BrazilCopyright © 2022 Adeleke, Shittu, Beierkuhnlein and Thomas. This is an open-access article distributed under the terms of the Creative Commons Attribution License (CC BY). The use, distribution or reproduction in other forums is permitted, provided the original author(s) and the copyright owner(s) are credited and that the original publication in this journal is cited, in accordance with accepted academic practice. No use, distribution or reproduction is permitted which does not comply with these terms.
*Correspondence: Stephanie Margarete Thomas, c3RlcGhhbmllLnRob21hc0B1bmktYmF5cmV1dGguZGU=
Disclaimer: All claims expressed in this article are solely those of the authors and do not necessarily represent those of their affiliated organizations, or those of the publisher, the editors and the reviewers. Any product that may be evaluated in this article or claim that may be made by its manufacturer is not guaranteed or endorsed by the publisher.
Research integrity at Frontiers
Learn more about the work of our research integrity team to safeguard the quality of each article we publish.