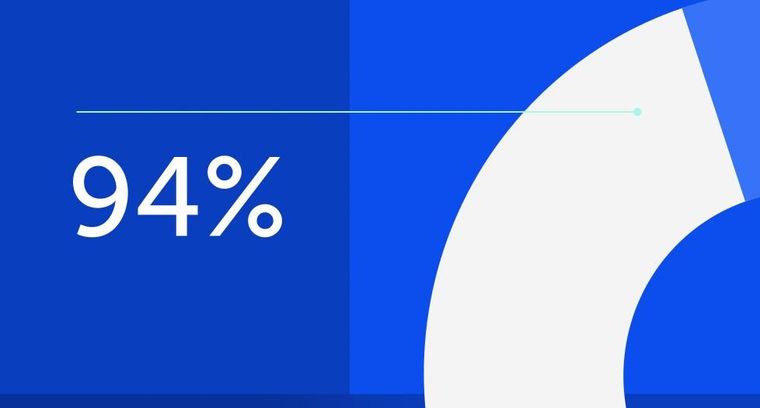
94% of researchers rate our articles as excellent or good
Learn more about the work of our research integrity team to safeguard the quality of each article we publish.
Find out more
ORIGINAL RESEARCH article
Front. Environ. Sci., 16 February 2022
Sec. Freshwater Science
Volume 10 - 2022 | https://doi.org/10.3389/fenvs.2022.840653
This article is part of the Research TopicThe Urban Fluvial and Hydro-Environment SystemView all 21 articles
Among numerous flow resistance formulae for sand-bed channels, this study selected five for evaluation and in order to cover flow conditions in sand-bed river channels as widely as possible, a total of 1,636 sets of field measures were collected from the hydrological stations of two large river systems of China—the Yellow and Yangtze Rivers, in addition to the data compiled by Brownlie. The performance of the selected formulae in yielding the values of Manning resistance coefficient n was evaluated against the total of 6,805 datasets. In many cases, the formula of Ma et al. yielded unreasonable n values of <0, while that of Deng et al. and formulae 1 and 2 of Zhang et al. yielded n values with large errors. The formula of Wu and Wang yielded n values varying within the scope only in the case of n < 0.04. By dividing the absolute relative errors (AREs) from the selected formulae into six groups of 0–0.05, 0.05–1, 0.1–0.2, 0.2–0.5, 0.5–1, and >1, it can be found that, for all five selected formulae, ARE occurred in the group 0.2–0.5 occupied the largest percentage, while in each of the adjacent groups of 0.5–1 and 0.1–0.2 it also occupied a very large percentage. Hence, all five formulae still need to improve their predicting ability.
There are a plethora of flow resistance formulae available for sand-bed river channels, but river scientists and engineers have been facing the very difficult problem of selecting a convincing one in practical problem solving (Zhang et al., 2020). Although the following Manning resistance formula (Manning, 1890; Herschel, 1897) was developed a long time ago to calculate the resistance to flow in fixed-bed open channels, it still is widely applied to determine the flow resistance of sand-bed river channels:
where V is the average velocity of channel flow, R is the hydraulic radius of the channel, J is the energy slope of flow, and n is the Manning roughness coefficient.
Because the roughness of a riverbed can be reflected by the size of the sediments composing the bed, the Manning roughness coefficient n in Eq. 1 has frequently been determined using the following relationship:
where D and A are a representative bed sediment size and a roughness parameter, respectively.
The sediments composing a riverbed, however, are hardly uniform, and there has been no consensus of opinions on what size of the non-uniform sediments can represent the role of D in Eq. 2. While the study by Chang (1939) granted support to the use of D50, nevertheless, Meyer-Peter and Muller (1948) argued that it is more appropriate to use D90 to represent D and consequently found A = 26. However, Maynord (1991) used a large number of flume data collected from various sources and identified that the use of particle size D90 gave slightly better results than the use of D50, with A taking 21.6 and 22.8 respectively for D50 and D90. In the reformulation of the Meyer-Peter and Muller (1948) equation, Huang (2010) also discovered that utilizing D50 or D90 had no effect on the accuracy of flow resistance calculations.
The roughness of a channel can be divided into grain roughness and form roughness for a riverbed with bedforms (Einstein, 1952; Engelund, 1961). While it has been assumed that a representative size of riverbed sediments, such as D90 or D65, may accurately reflect the grain roughness of a flat riverbed (Engelund and Hansen, 1967; van Rijn, 1982; Kamphuis, 1974; Zhang et al.,2012a; Zhang et al., 2012b; Niazkar et al., 2019), form roughness is closely related to the strength of flow acting on a channel bed, usually reflected with the Shields parameter (Yalin, 1963; van Rijn, 1984). Using data from the Compendium of Solids Transport Data compiled by Brownlie (1981), nevertheless, Peterson and Peterson (1988) made a comparison of the bed roughness values between observational data and the results calculated from different flow resistance formulae and commented that the commonly applied formulae with the Shields parameter as the sole factor were insufficient. Importantly, they demonstrated that the roughness of a mobile bed is also a function of the Froude number (Fr) and a dimensionless settling velocity (Zhang et al., 1998). On the basis of both physical reasoning and dimensional analysis, Wu and Wang (1999) proposed a relationship to determine the roughness of a mobile bed by relating the roughness parameter A in Eq. 2 to a non-dimensional shear stress parameter and Fr. Importantly, they tested the relationship with a large number of data observed in experimental flumes and fields collected from different sources and demonstrated that the values of n computed using their relationship yielded results consistent with the measured data at a much higher level than when using the other methods. Table 1 presents the selection of a representative sediment size D and the corresponding value of roughness parameter A by different investigators.
In contrast to the approach of selecting a representative sediment size D in Eq. 2, many studies determined the value of the Manning roughness coefficient n in Eq. 1 directly from a statistical analysis of field observations. Using field observations from the lower Yellow River, Chien and Wan (1983) analyzed the variation of n at various flow and sediment conditions and consequently recognized that n is closely related to both of the relative strength of tractive force and sediment size D65. Zhao and Zhang (1997) presented a detailed physical analysis of the effects of the rough thickness of channel bed on flow velocity, and a relationship expressing n as a complex function of flow depth, sediment size D50, and Fr received a high level of validation with a larger number of field observations obtained from the lower reach of the Yellow River. By reanalyzing the flume experimental data provided by Guy et al. (1966) and field observations at stations located in the middle and lower reaches of the Yellow River, however, Deng et al. (2007) identified that
Using 64 sets of data observed in experimental flumes and more than 1,000 sets of data measured during 1958–1959 at six hydrological stations located in the lower reach of the Yellow River, Ma et al. (2017) evaluated the performance of three roughness formulae proposed respectively by van Rijn (1984), Zhao and Zhang (1997), and Qin et al. (1995). They identified that the formula of Zhao and Zhang fitted best the observations from natural rivers, while the van Rijn formula performed well in laboratory flumes. In addition, Ma et al. (2017) reanalyzed the collected data, and their statistical regression results demonstrated that n showed a very close relationship with Fr. Recently, Zhang et al. (2020) presented a systematic review of the progress on the determination of the roughness of a moving bed channel and highlighted the need to treat Fr as a primary parameter in the establishment of a practically useful flow resistance formula. Consequently, they proposed two formulae to determine n. The first formula is in a relatively simple form considering the effect of Fr only, while the second formula is in a comprehensive form taking into account the effects of Fr, sediment size D50, roughness parameter A, and the von Kármán constant that reflects the effect of sediment concentration on flow energy consumption. While complex in form, the second formula had a much higher level of accuracy. The validation of the two roughness formulae clearly showed that the results generated by both were in a good agreement with a large number of data measured from natural rivers, although deviating slightly from observations in experimental flumes.
It is apparent that most flow resistance formulae were derived with reference to the data observed from experimental flumes and/or from natural rivers collected or conducted by original author(s), supplemented with some data obtained by one or several other researchers. Furthermore, the performance of a formula has often been assessed tentatively based on its agreement with a limited amount of data collected. In addition, there is considerable overlap of the data employed in the development of many formulae, with data incorporated in the subsequent evaluations of their performance. All of these contributed to the proliferation rather than the consolidation of flow resistance formulae for mobile-bed channels (Mark Powell, 2014; Ferro, 2018a; Ferro, 2018b; Di Stefano et al., 2020; Carollo and Ferro, 2021; Nicosia et al., 2021; Yadav et al., 2022). So far, none of the previously developed formulae has been either universally accepted or recognized as being especially appropriate for practical application. Although the performance of many formulae had been evaluated under a relatively wide range of hydraulic conditions, either from laboratory and/or from the field (e.g., Huang et al., 2004; Zhang, 2012a; Zhang, 2012b; Zhang et al., 2020), a collection of more observational datasets has become possible recently, and it is necessary to evaluate which flow resistance formula can give better predictions. For this purpose, this study selected flow resistance formulae for mobile-bed channels that have been most quoted in the literature and collected observational data wide in varying ranges and large in number from various sources. A set of statistical indicators was then used to evaluate the performance of the selected formulae against a large observational dataset collected in this study.
The selection of formulae for evaluation in this study was mainly in terms of popularity and the number and range of data used for developing them. From a detailed literature review of the progress on the development of the formulae, those developed by Wu and Wang (1999), Deng et al. (2007), Ma et al. (2017), and Zhang et al. (2020) satisfied these criteria and so were selected.
The flow resistance formula developed by Wu and Wang (1999) takes a form of:
where g is the acceleration due to gravity, Fr is the Froude number (
To obtain the value of T in Eq. 3, the following relationships need to be applied:
where
where
By reanalyzing the flume experimental data provided by Guy et al. (1966) and field observations at stations located in the middle and lower reaches of the Yellow River, Deng et al. (2007) obtained the following power–function relationship between n and Fr by using a statistical regression method:
Using 64 sets of data observed in experimental flumes and more than 1,000 sets of data measured during 1958–1959 at six hydrological stations located in the lower reach of the Yellow River, based on data from both experimental flumes and field measurements in the Lower Yellow River, Ma et al. (2017) developed the following regression formula:
Zhang et al. (2020) proposed two flow resistance relationships: the simple one, referred to as formula 1 of Zhang et al. in this study, takes the form
When the influences of bed materials and sediment concentration on flow energy expenditure were taken into account, Zhang et al. (2020) presented a more comprehensive formula, referred to as formula 2 of Zhang et al. in this study, taking the form
where
The sources and other details pertaining to the development of the five formulae selected above are summarized in Table 2.
The datasets compiled by Brownlie (1981) contained 7,027 records (5,263 laboratory records and 1,764 field records) in 77 data files. All of the records were collected from various studies conducted during the 20th century and provide a historically complete set of alluvial channel observations. This data collection was inspired by the data compendium of Peterson and Howells (1973), and in comparison with previous data compendiums, this compendium corrected early errors, completed omissions, and added about 2,500 new records consisting of 10 basic hydraulic parameters. If no data were available, it was recorded as −1.
In the drainage basins of the Yellow and Yangtze Rivers, numerous hydrological stations have been set up by the state government of China since the 1950s, and the measured data have been made available in hydrological almanacs, including flow discharge, slope, sediment concentration, average velocity, channel width, flow depth, median size of bed material, and the Manning resistance coefficient (Ma and Huang, 2016; Yellow River hydrological almanac, 2019). This study collected the measured data from the hydrological stations at Huayuankou, Jiahetan, Gaocun, Sunkou, Luokou, Tuchengzi, and Lijin in the Lower Yellow River, at Shizuishan (II), Bayan Gaole, and Toudaoguai in the upper reach of the Yellow River, and at Xianyang (II), Lintong, and Huaxian in the main tributary of the Weihe River. In the Yangtze River basin, the data collected were from the hydrological stations at Yichang, Luoshan, Datong, Jianli, Chenjiawan, and Xinchang in the trunk river and at Buhe, Hanjiang, Xincheng, and Guchen in the main tributaries.
In the following evaluation of the performance of the five selected formulae, datasets that lacked any of D50, gradient, water temperature, and sediment concentration and had a value of <6 for the width/depth ratio were removed from the original data in order to maintain consistency with the studies by van Rijn (1984) and Peterson and Peterson (1988). As a result, 3,327 datasets (3,623 from experimental flumes and 1,546 from the field) compiled by Brownlie (1981) and 958 field datasets from the Yellow and Yangtze Rivers were selected. The sources and varying ranges of the parameters used in this study are provided in Table 3. All datasets are presented in Supplementary Data Sheet S1.
In a comparison of the information presented in Tables 2 and 3, it is clearly seen in Table 2 that the datasets used in this study are larger than those used in the development and validation of each of the five flow resistance formulae selected. In the following analysis, each of the five formulae was examined in light of all datasets collected. Except for minor amendments, which have been discussed in the computational procedure of the Ma et al. (2017) formula, each formula was applied in a precise manner specified by the original author(s).
To evaluate the performance of the five formulae against the large datasets collected in this study, several statistical parameters were adopted in this study. Table 4 shows the expressions of these parameters. RMSE is the root mean square error between the observed data and computed results, CD is the square of Pearson’s correlation coefficient that reflects the proportion of the total variance in the observed data, and NA is the Nash coefficient that evaluates the agreement between the computed and observed values, with NA = 1 indicating perfect agreement between the computed and observed values. Furthermore, ARE (absolute relative error), computed as the ratio of the absolute error between the prediction and observation results to the observed value, was used to depict whether the predicted value was overestimated or underestimated. RE (relative error) was also used, and an MSE = 0 means that the computed value agreed perfectly with the observed value on the whole, while RE > 0 or RE < 0 indicate respectively that an overestimation or an underestimation occurred. Moreover, the percentages of data with relative errors of ≤50% and 25% (P50 and P25, respectively) and the percentages of the data with an overestimated error (POE) were also deployed (Nash and Sutcliffe, 1970; Bjerklie et al., 2005; López et al., 2007).
In terms of the laboratory data compiled by Brownlie (1981), the values of n computed from the five selected formulae against the observed counterparts are presented in Figure 1. It can be seen from Figures 1A–E that the differences between the computed and the observed values of n varied beyond the range of 2:1–1:2 (ratio of the computed to the observed). In many cases, the Ma et al. formula even yielded unreasonable results of <0, as shown in Figure 1C. Relatively speaking, nevertheless, the values of n computed using the formula of Wu and Wang were closer to the observed counterparts, typically when n was <0.04.
FIGURE 1. Computed n values against the observed n valued for the laboratory data of Brownlie. (A) Wu and Wang formula. (B) Deng et al. formula. (C) Ma et al. formula. (D) Formula 1 of Zhang et al. (E) Formula 2 of Zhang et al.
The statistical results on the correlation of the values of n computed from the five formulae against the observed counterparts in terms of the laboratory data of Brownlie are provided in Table 5. The correlation coefficient (CD) of the results computed using the formula of Wu and Wang was 0.351, the highest among the five formulae, while the other four yielded correlation coefficient results of generally <0.3. Among the NA values of the five formulae, only that by Wu and Wang yielded results with NA > 0, manifesting that the results were generally closer to the average level of the observations; that is, the points in Figure 1A are much more evenly distributed on both sides of the 1:1 line. All of these indicated that the formula of Wu and Wang outperformed the other four equations in Brownlie’s laboratory data.
Using the field data compiled by Brownlie (1981), the values of n computed from each formula against the observed n values are presented in Figures 2A–E. It can be noticed from Figure 2 that the differences between the computed and the observed values of n varied beyond the range of 2:1–1:2 (ratio of the computed to the observed). Relatively speaking, nevertheless, the distribution of the n values computed using the Wu and Wang formula against the observed n values was much less scattered than those computed using the other four formulae.
FIGURE 2. Computed n values against the observed n values for the field data of Brownlie. (A) Wu and Wang formula. (B) Deng et al. formula. (C) Ma et al. formula. (D) Formula 1 of Zhang et al. (E) Formula 2 of Zhang et al.
The performance of the five selected formulae in the field data of Brownlie is summarized in Table 6. The CD computed using formula 2 of Zhang et al. was 0.333, which is the highest among the five formulae, while the CD values of the other four formulae were <0.3. Although the AREs of the results computed using formula 2 of Zhang et al. and the formula of Wu and Wang took smaller values of 31.4% and 23.5%, respectively, their P50 values were respectively 92.3% and 81.37%, indicating that the results computed using the Wu and Wang formula and formula 2 of Zhang et al. fitted the observed values at respective degrees of 92.3% and 81.37% within an error of 50%. Hence, the Wu and Wang formula yielded results best fitting the observed values among the five selected formulae.
Using data collected from the hydrological stations in the main tributaries and the trunk reach of the Yellow and Yangtze Rivers, the values of n computed from the five selected formulae against the observed values of n are presented in Figures 3A–E. It can be seen that, except for the results computed using the Wu and Wang formula, the differences between the values of n computed from the other four formulae and the observed n varied within the range of 2:1–1:2 (ratio of the computed to the observed). Relatively speaking, nevertheless, the values of n computed using formula 1 of Zhang et al. were distributed more uniformly on both sides of the 1:1 line and so fitted the observations to the highest level among the five selected formulae.
FIGURE 3. Computed n values against the observed
The statistical results of the performance of the five formulae in the data collected from the drainage basins of the Yellow and Yangtze Rivers are shown in Table 7. The CD values of the results computed using the Deng et al. formula, Ma et al. formula, and formulae 1 and 2 of Zhang et al. were all very high, respectively 0.87, 0.871, 0.88, and 0.896, and the AREs of the results computed using the four formulae were no larger than 22.3%. In addition, the NA and P50 of the results computed using the four formulae were all larger than 0.6% and 95%, respectively. On the contrary, the results computed using the Wu and Wang formula only had values of 0.679 for CD, 51.83% for ARE, 0.173 for NA, and 57.46% for P50. Except for the Wu and Wang formula, hence, the other four selected formulae were all able to yield results best fitting the observed values.
In terms of the laboratory and field data compiled by Brownlie (1981) and the data collected from the hydrological stations in the main tributaries and the trunk reach of the Yellow and Yangtze Rivers in this study, the values of n computed from each selected formula against the observed n values are presented in Figures 4A–E. It can be seen that the formula of Ma et al. yielded an unreasonable result of n < 0 in many cases (Figure 4C). While the formula of Deng et al. and formulae 1 and 2 of Zhang et al. yielded results varying significantly beyond the range of 2:1–1:2 (ratio of the computed to the observed), the formula of Wu and Wang yielded results varying mostly within the scope, typically in cases of n < 0.04.
FIGURE 4. Computed n values against observed n values for all datasets. (A) Wu and Wang formula. (B) Deng et al. formula. (C) Ma et al. formula. (D) Formula 1 of Zhang et al. (E) Formula 2 of Zhang et al.
Statistical results of the performance of the five selected formulae in all datasets used in this study are shown in Table 8. The CD values of the results computed using all five formulae were not very high and varied within the small range of 0.551–0.62. The AREs of the results computed using the five formulae were considerably small and also varied within the small range of 31.46%–37.49%. However, the NA values of the results computed using the Deng et al. formula, the Ma et al. formula, and formula 1 of Zhang et al. were negative or very close to 0, while the NA values of the results computed using the formula of Wu and Wang and formula 2 of Zhang et al. were positive, with the Wu and Wang formula yielding a much larger NA of 0.31. All of these demonstrated that formula 2 of Zhang et al. and the formula of Wu and Wang were the most appropriate in all datasets, despite their low calculation accuracy.
The AREs of n (computed n − Observed n)/Observed n) by all five selected formulae were computed for the three datasets, i.e., the laboratory data and field data compiled by Brownlie (1981) and the data from the drainage basins of the Yellow and Yangtze Rivers collected in this study. The distribution of AREs in the six groups of 0–0.05, 0.05–1, 0.1–0.2, 0.2–0.5, 0.5–1, and >1 is presented in Table 9 and shown in Figure 5. In the laboratory data of Brownlie, the AREs in the group 0.2–0.5 by all five selected formulae occupied around 60%, and there was a very small difference among the percentages in the group for the five formulae. The AREs in the group 0.5–1 occupied around 20%, while the AREs of each of the other groups occupied a very small percentage (Figure 5A).
FIGURE 5. Distribution of the IC50 values by the five selected formulae. (A) Laboratory data of Brownlie. (B) Field data of Brownlie. (C) Data from the Yellow and Yangtze Rivers.
In the field data of Brownlie, however, the AREs in the groups of 0.2–0.5 and 0.5–1 by all five formulae occupied large and nearly equal percentages of around 35%, while those in each of the other groups occupied only a very small percentage (Figure 5B). In the data from the Yellow and Yangtze Rivers, the AREs in the two groups of 0.1–0.2 and 0.2–0.5 by all five formulae occupied large and nearly equal percentages of slightly larger than 30%, while the AREs in the two groups of 0.05–0.1 and <0.05 occupied nearly equal percentages of about 18% (Figure 5C). Overall, it was obvious that the relative errors in the range of 0.2–0.5 accounted for the biggest percentage, with each of the nearby groups of 0.5–1 and 0.1–0.2 accounting for a sizable portion as well. This clearly manifests that the AREs by all five flow resistance formulae occupied a very large percentage almost in the same range of 0.1–1; hence, the five formulae needed to improve their predicting ability. Importantly, it is noticeable from Table 9 and Figure 5 that the AREs by each of the five selected flow resistance formulae were considerably large among the three datasets used.
The formula of Wu and Wang performed better in the laboratory and field data of Brownie, with ARE <30%. In the data from the Yellow and Yangtze Rivers, nevertheless, ARE >50% and the group of ARE >100% still accounted for 14.67% of the total. The formula of Deng et al. considerably yielded large values of 41.3% and 44.6% for ARE in the laboratory data and field data of Brownlie, respectively. In the data from the Yellow and Yangtze Rivers, nevertheless, the formula allowed ARE to take a relatively small value of 22.3%, and the group of ARE <0.5 occupied a very large percentage of 97.37% of the total. The formula of Ma et al. also yielded considerably large values of 44.37% and 33.1% for ARE in the laboratory data and field data of Brownlie, respectively. In the data from the Yellow and Yangtze Rivers, the formula allowed ARE to take a considerably small value of 18.78%, and the group of ARE <0.5 occupied 97.74% of the total. Hence, the formulae of Deng et al. and Ma et al. performed best in the data from the Yellow and Yangtze Rivers among the three datasets used. Formulae 1 and 2 of Zhang et al. yielded relatively small values of <40% for ARE in the laboratory data and field data of Brownlie, respectively. In the data from the Yellow and Yangtze Rivers, meanwhile, the formulae allowed ARE to take considerably small values of 19.32% and 17.96%, and the group of ARE <0.5 occupied 96.39% and 95.54% of the total. Hence, formulae 1 and 2 of Zhang et al. both performed well in the data from the Yellow and Yangtze Rivers among the three datasets used.
Because different formulae performed at considerably different levels in the different datasets used, ARE appears a suitable index to evaluate the performance of the five selected flow resistance formulae in the three datasets. Assuming that the performance of a formula can be ranked to the highest level “I” when 0 < ARE < 20%, to the relatively high level “II” when 20% < ARE < 30%, to the moderate level “III” when 30% < ARE < 40%, to the relatively low level “IV” when 40% < ARE < 50%, and to the lowest level “V” when ARE > 50%, the detailed ranks of the performance of the five selected flow resistance formulae in the three datasets are provided in Table 10.
It can be clearly seen from Table 10 that the formula of Ma et al. and formulae 1 and 2 of Zhang et al. gained the highest rank I in the data from the Yellow and Yangtze Rivers, while they gained the moderate rank III or the relatively low rank IV in the laboratory and field data of Brownlie. In contrast, the formula of Wu and Wang gained the relatively high rank II in the laboratory and field data of Brownlie, yet the lowest rank V in the data from the Yellow and Yangtze Rivers. Within the two relatively extremal cases, the formula of Deng et al. gained a relatively low rank IV in the laboratory and field data of Brownlie and also a relatively high rank II in the data from the Yellow and Yangtze Rivers.
Among the numerous flow resistance formulae for sand-bed channels, this study selected five for evaluation in terms of theoretical advances and the scope and quantity of the data used in the development, including those developed by Wu and Wang (1999), Deng et al. (2007), Ma et al. (2017), and Zhang et al. (2020). In order to cover flow conditions in sand-bed river channels as widely as possible, this study collected 1,636 sets of field measures from the hydrological stations in two large river systems of China (the Yellow and Yangtze Rivers), in addition to the data compiled by Brownlie (1981) that, in complete form, included 3,623 sets of laboratory data and 1,546 sets of field data from different nations and areas. The conclusion of this paper is generally used in alluvial rivers. A detailed statistical analysis of the performance of the five selected flow resistance formulae in yielding the values of the Manning resistance coefficient n using the very large number of datasets, 6,805 sets in total, led to the following important findings:
1) The formula of Wu and Wang yielded n values best fitting the laboratory and field data of Brownie, yet fitting the data from the Yellow and Yangtze Rivers at a relatively low degree. In contrast, the formula of Ma et al. and formulae 1 and 2 of Zhang et al. yielded n values fitting the data from the Yellow and Yangtze Rivers at a very high degree, yet fitting the laboratory and field data of Brownie at a moderate degree. The formula of Deng et al. yielded n values fitting the laboratory and field data of Brownlie and the data from the Yellow and Yangtze Rivers both at a relatively low degree.
2) In all datasets, the Ma et al. formula yielded unreasonable n values of <0 in many cases, while the formula of Deng et al. and formulae 1 and 2 of Zhang et al. yielded n values varying considerably beyond the range of 2:1–1:2. Nevertheless, the Wu and Wang formula yielded n values varying mostly within the scope, typically in cases of n < 0.04.
3) By dividing the AREs from the five selected formulae into six groups of 0–0.05, 0.05–1, 0.1–0.2, 0.2–0.5, 0.5–1, and >1, it can be found that, for all five selected formulae, the AREs in the group 0.2–0.5 occupied the largest percentage, while each of the adjacent groups of 0.5–1 and 0.1–0.2 occupied a very large percentage. This means that all five formulae needed to enhance the accuracy of their predicting capability.
4) The formula of Ma et al. and formulae 1 and 2 of Zhang et al. gained the highest rank for fitting the data from the Yellow and Yangtze Rivers, yet gained a moderate rank or a relatively low rank for the laboratory and field data of Brownlie. In contrast, the Wu and Wang formula gained a relatively high rank for the laboratory and field data of Brownlie, yet gained the lowest rank for the data from the Yellow and Yangtze Rivers. Within the two relatively extremal cases, the formula of Deng et al. gained a relatively low rank for the laboratory and field data of Brownlie, yet gained a relatively high rank for the data from the Yellow and Yangtze Rivers.
The original contributions presented in the study are included in the article/Supplementary Material. Further inquiries can be directed to the corresponding author.
HP, HH, GY, and HZ contributed to the conception and design of the study. HP organized the database, performed the statistical analysis, and wrote the first draft of themanuscript. HH wrote sections of the manuscript. All authors contributed to manuscript revision, read, and approved the submitted version.
This work was supported financially by the National Natural Science Foundation of China (grant nos. 41971010 and 41561144012) and the National Key Research and Development Program of China (grant no. 2016YFC0402502).
The authors declare that the research was conducted in the absence of any commercial or financial relationships that could be construed as a potential conflict of interest.
All claims expressed in this article are solely those of the authors and do not necessarily represent those of their affiliated organizations, or those of the publisher, the editors and the reviewers. Any product that may be evaluated in this article, or claim that may be made by its manufacturer, is not guaranteed or endorsed by the publisher.
The authors would like to thank the Yellow River Water Conservancy Commission and Yangtze River Water Conservancy Commission of China for permission to access the measured hydrological and river channel data.
The Supplementary Material for this article can be found online at: https://www.frontiersin.org/articles/10.3389/fenvs.2022.840653/full#supplementary-material
Bjerklie, D. M., Dingman, S. L., and Bolster, C. H. (2005). Comparison of Constitutive Flow Resistance Equations Based on the Manning and Chezy Equations Applied to Natural Rivers. Water Resour. Res. 41, W11502. doi:10.1029/2004WR003776
Brownlie, W. R. (1981). Compilation of Alluvial Channel Data: Laboratory and Field. Report No. KH-R-43B. Pasadena, California: W. M. Keck Laboratory of Hydraulics and Water Resources, California Institute of Technology.
Carollo, F. G., and Ferro, V. (2021). Experimental Study of Boulder Concentration Effect on Flow Resistance in Gravel Bed Channels. Catena 205, 105458. doi:10.1016/j.catena.2021.105458
Chang, Y. L. (1939). Laboratory Investigation of Flume Traction and Transportation. T. Am. Soc. Civ. Eng. 104, 1246–1284. doi:10.1061/taceat.0005049
Chien, N., and Wan, Z. H. (1983). Mechanics of Sediment Movement. Beijing: Science Publications. (in Chinese).
Deng, A., Guo, Q., and Chen, J. (2007). Study on the Roughness in Sediment-Laden Flows. J. Sediment Res. 2007 (05), 24–29. (in Chinese). doi:10.16239/j.cnki.0468-155x.2007.05.005
Di Stefano, C., Nicosia, A., Palmeri, V., Pampalone, V., and Ferro, V. (2020). Flow Resistance Law under Suspended Sediment Laden Conditions. Flow Meas. Instrumentation 74, 101771. doi:10.1016/j.flowmeasinst.2020.101771
East Parkistan Water and Power Development Authority (1967). Flume Studies of Roughness and Sediment Transport of Movable Bed of Sand. Dahka, Bangladesh: Annu. Rep. of Hydr. Res. Lab.
Einstein, H. A., and Barbarossa, N. L. (1952). River Channel Roughness. T. Am. Soc. Civ. Eng. 117, 1121–1132. doi:10.1061/taceat.0006666
Engelund, F., and Hansen, E. A. (1967). Monograph on Sediment Transport in Alluvial Streams. Copenhagen K: Technical University of Denmark Østervoldgade 10.
Engelund, F. (1961). Hydraulic Resistance of Alluvial Streams. J. Hydraulics Division, ASCE 92 (2), 315–327.
Ferro, V. (2018a). Assessing Flow Resistance in Gravel Bed Channels by Dimensional Analysis and Self-Similarity. Catena 169, 119–127. doi:10.1016/j.catena.2018.05.034
Ferro, V. (2018b). Flow Resistance Law under Equilibrium Bed-Load Transport Conditions. Flow Meas. Instrumentation 64, 1–8. doi:10.1016/j.flowmeasinst.2018.10.008
Gilbert, G. K. (1914). The Transportation of Débris by Running Water. U.S. Geol. Surv. Prof. Pap. 86.
Guy, H. P., Simons, D. B., and Richardson, E. V. (1966). Summary of Alluvial Channel Data from Flume Experiments, 1956-1961. Washington: UNITED STATES GOVERNMENT PRINTING OFFICE.
Huang, H. Q. (2010). Reformulation Of The Bed Load Equation Of Meyer-Peter And Müller in Light of The Linearity Theory For Alluvial Channel Flow[J]. Water Resources Res. 46 (9), 161–170.
Huang, C., Zhao, X., and Gong, M. (2004). Comparisons Of Flow Resistance Equations In Movable Bed. J. Sediment Res. 2004 (5), 1–7. (in Chinese). doi:10.16239/j.cnki.0468-155x.2004.05.001
Kamphuis, J. W. (1974). Determination of Sand Roughness for Fixed Beds. J. Hydraulic Res. 12 (2), 193–203. doi:10.1080/00221687409499737
Kuhnle, R. A. (1993). Fluvial Transport of Sand and Gravel Mixtures with Bimodal Size Distributions. Sediment. Geology. 85, 17–24. doi:10.1016/0037-0738(93)90072-d
Liu, X. L. (1986). Nonuniform Bed Load Transport Rate and Coarsening Stabilization. Master's Thesis. China: Chengdu University of Technology.
López, R., Barragán, J., and Colomer, M. Á. (2007). Flow Resistance Equations without Explicit Estimation of the Resistance Coefficient for Coarse-Grained Rivers. J. Hydraulic 338, 113–121. doi:10.1016/j.jhydrol.2007.02.027
Ma, Y., and Huang, H. Q. (2016). Controls of Channel Morphology and Sediment Concentration on Flow Resistance in a Large Sand-Bed River: A Case Study of the Lower Yellow River. Geomorphology 264, 132–146. doi:10.1016/j.geomorph.2016.03.035
Ma, Y., Xia, J., and Zhang, X. (2017). Verification and Comparison of Flow Resistance Formulae over Movable Beds. Eng. J. Wuhan Univ. 50 (4), 481–486. (in Chinese). doi:10.14188/j.1671-8844.2017-04-001
Manning, R. (1890). On the Flow of Water in Open Channels and Pipes. Proc. Inst. Civil Eng. Ireland 20, 161–206.
Mark Powell, D. (2014). Flow Resistance in Gravel-Bed Rivers: Progress in Research. Earth-Science Rev. 136, 301–338. doi:10.1016/j.earscirev.2014.06.001
Maynord, S. T. (1991). Flow Resistance of Riprap. J. Hydraulic Eng. 117 (6), 687–696. doi:10.1061/(asce)0733-9429(1991)117:6(687)
Meyer-Peter, E., and Müller, R. (1948). “Formulas for Bed Load Transport, Paper Presented at 2nd Meeting,” in Int. Assoc. for Hydroaul. Environ.Eng. and Res. (Madrid).
Nash, J. E., and Sutcliffe, J. V. (1970). River Flow Forecasting through Conceptual Models Part I - A Discussion of Principles. J. Hydrol. 10, 282–290. doi:10.1016/0022-1694(70)90255-6
Niazkar, M., Talebbeydokhti, N., and Afzali, S. H. (2019). Development of a New Flow-Dependent Scheme for Calculating Grain and Form Roughness Coefficients. KSCE J. Civil Eng. 23, 2108–2116. doi:10.1007/s12205-019-0988-z
Nicosia, A., Di Stefano, C., Pampalone, V., Palmeri, V., and Ferro, V. (2021). Assessing an Overland Flow Resistance Approach under Equilibrium Sediment Transport Conditions. CATENA 207, 105578. doi:10.1016/j.catena.2021.105578
Peterson, A. W., and Peterson, A. E. (1988). Mobile Boundary Flow: An Assessment of Velocity and Sediment Discharge Relationships. Can. J. Civ. Eng. 15 (4), 539–546. doi:10.1139/l88-074
Peterson, A. W., and Howells, R. F. (1973). A Compendium of Solids Transport Data for Mobile Boundary Channels. Canada: Department of Civil Engineering, University of Alberta. Report No. HY-1973-ST3.
Qin, R., Liu, S., and Wang, C. (1995). Characteristics Of Channel Resistance And Sediment Transport In The Lower Yellow River. J. Sediment Res. (4), 10–18. (in Chinese).
Samaga, B. R., Raju, K. G. R., and Garde, R. J. (1986). Bed Load Transport of Sediment Mixtures. J. Hydraulic Eng. 112 (11), 1003–1017. doi:10.1061/(asce)0733-9429(1986)112:11(1003)
Taylor, B. D. (1971). Temperature Effects In Alluvial Streams[J]. California Institute of Technology.
van Rijn, L. C. (1982). Equivalent Roughness of Alluvial Bed. J. Hydraulics Division, ASCE 108, HY10. doi:10.1061/jyceaj.0005917
van Rijn, L. C. (1984). Sediment Transport, Part III: Bed Forms and Alluvial Roughness. J. Hydraul. Eng. 110 (12), 1733–1754. doi:10.1061/(asce)0733-9429(1984)110:12(1733)
Wang, S. (1990). Experimental Study on Hydraulic Resistance of Alluvial Streams. J. Hydraulic Eng. 1990 (12), 18–29. (in Chinese).
Wilcock, P. R., and McArdell, B. W. (1993). Surface-based Fractional Transport Rate: Mobilization Thresholds and Partial Transport of a Sand-Gravel Sediment. Water Resour. Res. 29 (24), 1297–1312. doi:10.1029/92wr02748
Wu, W., and Wang, S. S. Y. (1999). Movable Bed Roughness in Alluvial Rivers. J. Hydraulic Eng. 125 (12), 1309–1312. doi:10.1061/(asce)0733-9429(1999)125:12(1309)
Yadav, A., Sen, S., Mao, L., and Schwanghart, W. (2022). Evaluation of Flow Resistance Equations for High Gradient Rivers Using Geometric Standard Deviation of Bed Material. J. Hydrol. 605, 127292. doi:10.1016/j.jhydrol.2021.127292
Yalin, M. S. (1963). An Expression for Bed-Load Transportation[J]. J. Hydraul. Div. Asce 89, 221–250.
Yellow River hydrological almanac (2019). The Yellow River Water Resources Commission. China: Zhengzhou.
Zhang, H., Zhang, L., Peng, H., Cai, R., and Zhang, L. (2020). Research on Cognition and Calculation Method of Alluvial River Roughness. J. Hydraulic Eng. 51 (07), 774–787. (in Chinese). doi:10.13243/j.cnki.slxb.20200130
Zhang, L. (2012a). Analysis of the Present Situation of Open Channels Roughness. J. Hydraulic Eng. 43 (10), 1154–1162. (in Chinese). doi:10.13243/j.cnki.slxb.2012.10.007
Zhang, L. (2012b). Reasons for the Abnormal Channel Roughness of the Yellow River and the Solution to its Problems. J. Hydraulic Eng. 43 (10), 1154–1162. (in Chinese). doi:10.13243/j.cnki.slxb.2012.11.004
Zhang, R., Xie, J., Wang, M., and Huang, J. (1998). Dynamics of River Sedimentation. Beijing: Water Resources and Electric Power Publication. (in Chinese).
Keywords: flow resistance, sand-bed channel, large dataset, Manning roughness coefficient, bedforms, error analysis
Citation: Peng H, Huang HQ, Yu G and Zhang H (2022) Applicability of Flow Resistance Formulae for Sand-Bed Channels: An Assessment Using a Very Large Data Set. Front. Environ. Sci. 10:840653. doi: 10.3389/fenvs.2022.840653
Received: 21 December 2021; Accepted: 06 January 2022;
Published: 16 February 2022.
Edited by:
Jaan H. Pu, University of Bradford, United KingdomReviewed by:
Ming He, Tianjin University, ChinaCopyright © 2022 Peng, Huang, Yu and Zhang. This is an open-access article distributed under the terms of the Creative Commons Attribution License (CC BY). The use, distribution or reproduction in other forums is permitted, provided the original author(s) and the copyright owner(s) are credited and that the original publication in this journal is cited, in accordance with accepted academic practice. No use, distribution or reproduction is permitted which does not comply with these terms.
*Correspondence: He Qing Huang, aHVhbmdocUBpZ3NucnIuYWMuY24=
Disclaimer: All claims expressed in this article are solely those of the authors and do not necessarily represent those of their affiliated organizations, or those of the publisher, the editors and the reviewers. Any product that may be evaluated in this article or claim that may be made by its manufacturer is not guaranteed or endorsed by the publisher.
Research integrity at Frontiers
Learn more about the work of our research integrity team to safeguard the quality of each article we publish.