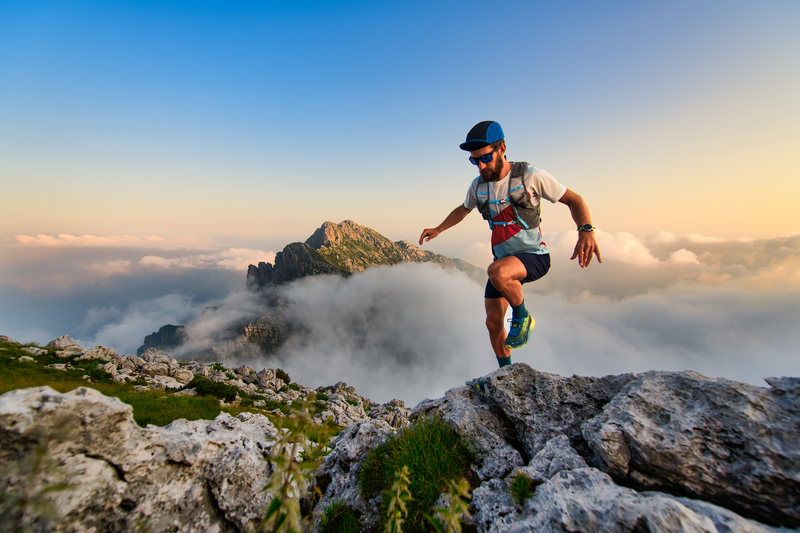
94% of researchers rate our articles as excellent or good
Learn more about the work of our research integrity team to safeguard the quality of each article we publish.
Find out more
ORIGINAL RESEARCH article
Front. Environ. Sci. , 10 March 2022
Sec. Toxicology, Pollution and the Environment
Volume 10 - 2022 | https://doi.org/10.3389/fenvs.2022.840428
This article is part of the Research Topic Antibiotics and Antibiotic Resistance Genes in Waters: Pollution, Risks, and Control View all 11 articles
The emergence of antibiotic resistance genes (ARGs) is a great risk to the ecosystem and human health; however, there are rare systematic studies about the characterization and source identification of ARGs in continental rivers. This study examined the occurrence of bacterial communities and ARGs in the water and soil of the Ili River using bacterial testing and metagenomic sequencing. Total dissolved solids (TDSs) and total bacterial count significantly increased from upstream to downstream sites. Enterococcus showed the highest abundance in Cockdara. Metagenomic sequencing revealed that the bacterial communities of surface water were different from those of nearshore soils. Among the top 10 ARGs, fluoroquinolone and aminoglycoside resistance genes exhibited dominant relative abundance, but only the multidrug resistance gene adeF was common in all water and soil samples. Proteobacteria carried almost 61% of ARGs, suggesting that these could be the main antibiotic-resistant bacteria (ARB) in the Ili River. Proteobacteria and ARB were mainly distributed in Yining city and Cockdara. Furthermore, the distribution pattern among the five sampling sites indicated that human activity and animal husbandry greatly contributed to the ARB and ARG contamination. This study first investigated the occurrence and distribution pattern of ARGs in the Ili River, demonstrating a clear correlation between bacteria and ARGs, and ARGs and mobile genetic elements (MGEs).
Worldwide, deteriorating water quality has become a critical environmental problem, causing a great threat to human health, ecological biodiversity, and even social stability (Landrigan et al., 2020). The river system, a precious fundamental resource for living species, plays critical roles as was for drinking, agricultural irrigation, traffic channel, and recreational activities. However, the river system can be a pool of various pollutants, including physical, chemical, and biological pollutions (Hu et al., 2017). The pollution of surface water is the most severe environmental concern causing diseases (Landrigan et al., 2020). Several studies showed a close link between river water pollution and the increasing incidence of gastrointestinal diseases (Rodriguez-Tapia and Morales-Novelo, 2017), infectious diseases (Johnson et al., 2012), waterborne endemic fluorosis, and arsenic poisoning (Boelee et al., 2019; Karkman et al., 2019).
Environmental microbiota, an important ingredient of the river ecosystem, carries out various essential biogeochemical processes, including nutrient cycling and pollutant degradation (Guan et al., 2018). However, the ever-growing economics and human activities have increased wastewaters discharged to the rivers, increasing community pathogens from hospitals, pharmaceutical industries, and animal husbandry (Molina et al., 2014; Stachler et al., 2017; Jennings et al., 2018). Fecal indicator bacteria, referred to as Escherichia coli and Enterococci, are routinely used to assess bacterial pollution of water systems (Boehm et al., 2018; Aydin et al., 2019). Some polluted waters such as the Haihe River and Taihu Lake contain high density of fecal indicator bacteria (Wang et al., 2017). A study showed the presence of seven potentially pathogenic genera including Acinetobacter, Enterococcus, and Streptococcus in Taihu Lake (Vadde et al., 2019). Bacterial pathogens, with low-dose infection and the possibility of secondary spread, are one of the most serious pollutants.
Antibiotics are widely used in medicine, animal husbandry, and agriculture to treat bacterial infections (Liu et al., 2019). However, the misuse/overuse of antibiotics has induced the emergence of ARGs and ARB in the natural environment, posing potential threats to human health and ecosystems (Stange et al., 2019; Uprety et al., 2020). The transmission and dissemination of ARGs induce ecological toxicity to aquatic ecosystems and severe infection to humans and livestock (Guo et al., 2019). ARGs have been identified as emerging environmental pollutants and one of the most serious threats to human health by the World Health Organization (Zheng et al., 2017; Yang et al., 2018). ARGs have been detected in various environments, such as surface water, sediments, soils, and underground water. Among these, surface water is an important reservoir of ARGs (Zhang et al., 2020). Previous investigations demonstrated that ARG spread is strongly related to human activities, like stock farming, and environmental factors, such as antibiotics, heavy metals, environmental estrogens, and MGEs (Zheng et al., 2017; Stange et al., 2019). Surface water such as the riverine system is more susceptible to human activities and therefore is an ideal setting for the prevalence of ARGs.
The abundance and distribution of ARGs in the global ocean suggest that quinolone, bacitracin, and fosmidomycin ARGs cause great environmental pressure (Cuadrat et al., 2020). In Italy, Sul1, ermB, blatem, tetw, and qnrs resistant E. coli were isolated from the Tiber and Arrone rivers (Pantanella et al., 2020). In China, there have been numerous investigations into ARGs; a study indicated that the most prevalent ARGs in the Haihe River were sulfonamide and tetracycline resistance genes (Dang et al., 2017). In the Bohai Sea, sulfonamide resistance genes (sul1 and sul2) are highly abundant, while other ARGs are present in low amounts. In 2021, 11 types of ARGs including eight tetracycline resistance genes, two sulfonamide resistance genes, and one β-lactam resistance gene were detected in the Yangtze River (Zhang et al., 2021). Likewise, in the Ba River in Xi’an, tetC, blaTEM, ermF, sul1, cmlA, and gyrA were found to be the predominant ARGs. Most published studies are about the ARB and ARG pollution in outflow rivers, while there are rare studies on ARGs in inner rivers.
Inner rivers play important roles in regulating the local climate and biological diversity. Particularly, due to drought and scarce precipitation, the ARB and ARG pollution of inner rivers is more vulnerable to human activities. The Ili River, a typical inner river, originates in the middle of the Tian Shan Mountains, and it is an international river covering about 1,237 km across China and Kazakhstan. This river is a critical source of water for agriculture, husbandry, and industrial production in Xinjiang Province, China. In addition, the Ili River flows through several cities in Xinjiang, thereby receiving significant sewage discharge. So far, there are only few studies about the bacterial and ARG contamination of the Ili River.
The present study selected different sampling points close to the towns with low-population density areas, urban areas with frequent human activities, and grazing areas to investigate the bacterial pollution, and the distribution and abundances of bacterial community and ARGs in the Ili River using metagenomics, bacteriological analysis, and antibiotic susceptibility testing. The objectives of this study were to illustrate the effective factors related to the occurrence and distribution of ARGs and explore the relevance of bacterial community, MGEs, and ARGs.
The detailed sampling sites are shown in Figure 1. According to the climate, ecology, and geographical characteristics of the Ili River, five sampling sites were selected in the mainstream and upstream tributary of the river, namely, upstream tributary Kashi River (BGC), Yining city (YN), Cockdara (KKDL), Huiyuan city (HY), and the National Highway 219 (B219), respectively. At each site, triplicate water samples were collected in sterile 10-L polythene bottles from the selected sampling sites, 100 m upstream and 100 m downstream from the sampling point. At the time of sampling, water temperature (T), pH, and total dissolved solids (TDSs) were measured with a handheld pH meter (pH808, SMART SENSOR, China) and a TDS-measuring instrument (AR8011, SMART SENSOR, China), respectively. Two liters of water from each sample was filtered through a 0.22-µm filter membrane (Millipore, United States), and the membranes containing bacterial filtrates were stored in centrifuge tubes containing phosphate-buffered saline (PBS) at 4°C for the following experiment. In addition to the five water samples, four soil samples, except for the sampling site B219, were collected from the respective riverbank sites.
The total count of culturable and indicator bacteria (total coliforms, E. coli, and Enterococcus) was estimated for each water sample after leaving the samples for more than 30 min. The numbers of total culturable bacteria (CFU/ml) were counted using the 3M Petrifilm Aerobic Count plate (3M, MN, Unites States) (Sinclair et al., 2021). The indicator bacteria (MPN/100 ml), including total coliforms, E. coli, and Enterococcus were enumerated for each sample using the Colilert and Enterolert kits according to the manufacturer’s instructions (IDEXX Laboratories, Westbrook, ME) (Sercu et al., 2011; Ledwaba et al., 2019).
Bacteria in water samples (2 L each) were collected through filtration using a 0.22-µm microporous membrane (Millipore, United States). Then, the trapped bacteria on the microporous membrane surface were resuspended in sterile phosphate-buffered saline (PBS). The bacterial suspension was streaked on the respective selective medium plate to grow Enterococcus (Enterococcus chromogenic medium, Hopebio, Qingdao, China), Staphylococcus aureus (Staphylococcus chromogenic medium, Hopebio, Qingdao, China), Salmonella (Salmonella chromogenic medium, Hopebio, Qingdao, China), and Coliform (E. coli/Coliform Chromogenic Medium, Hopebio, Qingdao, China) at 37°C for 24 h. Typical colonies were picked up according to the color, shape, and properties of the colony as described in the manufacturer’s instructions. Streak plate isolations on the respective selective medium plate were repeated at least three times.
After bacterial isolation, the DNA was extracted from bacterial cell pellets using the TIANamp Bacteria DNA Kit (Tiangen, Beijing, China). DNA samples were sent to the Allwegene Technologies (Beijing, China) for Sanger sequencing. The sequencing results were matched with the NCBI database. The preselected bacterial 16S rRNA sequence and the bacterial sequence were aligned by ClustalW in MEGA 5. After deleting non-aligned bases, the neighbor-joining method was used to construct a phylogenetic tree with 1,000 bootstrap replicates.
After incubation for 24 h, the bacterial suspension was soaked in Mueller–Hinton agar (MH). Then, the antibiotic susceptibility was tested using the disc diffusion method (Kirby–Bauer). The 13 types of antibiotic disc used in this study are listed in Table 2. The antibiotic discs were placed on the plate at a distance of no less than 25 mm, and the results were analyzed after incubation for 18–24 h at 30°C.
The genomic DNA of the soil samples was extracted using the TIANamp Soil DNA Kit (Tiangen Biotech, Beijing, China) following the manufacturer’s instructions. The DNA concentration was measured using the Qubit dsDNA Assay Kit in a Qubit 4.0 Fluorometer (ThermoFisher Scientific, Waltham, MA, United States). To examine the diversity and abundance of bacteria and ARGs, a total amount of 1 μg DNA per sample was used as an input material. Sequencing libraries were generated using the NEBNext® UltraTM DNA Library Prep Kit for Illumina (NEB, United States) following the manufacturer’s recommendations, and index codes were added to attribute the sequences to each sample. The raw data obtained from the Illumina HiSeq sequencing platform was processed using Readfq (V8, https://github.com/cjfields/readfq) to acquire the clean data for subsequent analysis. The specific processing steps were as follows: a) reads containing low-quality bases (default quality threshold value ≤38) above a certain portion (default length of 40 bp) were removed, b) reads with a certain percentage of N bases (default length of 10 bp) were removed, and c) reads sharing an overlap with the adapter above a certain portion (default length of 15 bp) were also removed.
The scaftigs (≥500 bp) assembled from both single and mixed were all predicted the open reading frame (ORF) by MetaGeneMark (V2.10, http://topaz.gatech.edu/GeneMark/) software, and length information was filtered to be shorter than 100 nt from the predicted result with default parameters. For ORF prediction, CD-HIT software (V4.5.8, http://www.bioinformatics.org/cd-hit) was adopted to avoid redundancy and obtain the unique initial gene catalog (the genes here refer to the nucleotide sequences coded by unique and continuous genes), and the parameter options were −c 0.95, −G 0, −aS 0.9, −g 1, and −d 0.
For bacterial community identification, the bacteria, fungi, archaea, and virus sequences were searched against the NR database (version: 2018-01-02, https://www.ncbi.nlm.nih.gov/) of the NCBI using DIAMOND blastp, with a cutoff e-value of 1e−5. In case a sequence was aligned to multiple results, the result with the E-value ≤ the smallest E-value *10 was selected to help the LCA algorithm to ensure the species annotation of sequence. A table containing the number of genes and the abundance information of each sample in the taxonomic hierarchy (kingdom, phylum, class, order, family, genus, and species) was prepared based on the LCA annotation results. The abundance of a species is equal to the sum of the gene abundance annotated for that species; the gene number of a species in a sample equals the number of genes whose abundance is non-zero.
Resistance Gene Identifier (RGI) software was used to align the Unigenes to CARD database (https://card.mcmaster.ca/) with the parameter setting are blastp and E-value ≤ 1e−30. Based on the aligned result, the relative abundance of ARO, the resistance genes’ abundance distribution in each sample, and the species attribution analysis of resistance genes were conducted, and the “relative abundance” (ppm, parts per million) was defined as one hit in one million aligned sequencing genes.
Mobile genetic elements (MGEs) were identified using BLASTP to compare the ARG-carrying genes to A CLAssification of Mobile genetic Elements (ACLAME) amino acid database for plasmids, using an e-value ≤ 10−5 and a cutoff of ≥50% query coverage. The ISfinder database (Siguier, 2006) was used to find insertion sequences (ISs) with an e-value ≤ 10−5 and a cutoff of ≥45% query coverage. In addition, the IntegrALL nucleotide database (Moura et al., 2009) was used to identify integrons, with an e-value ≤ 10−5 and a cutoff of ≥45% query coverage.
The networks were created as follows: a correlation matrix was constructed using ARGs and metagenomic data to explore the potential correlations among ARG subtypes, MGEs, and taxonomy (at the genus level) that occurred in at least 70% of samples, using R (psych package, v3.4.0). A correlation between two nodes was considered statistically significant if r ≥ 0.85 and p ≤ 0.05 and was adjusted using the Benjamini–Hochberg method. The correlation between two nodes was termed the edge file, and a description was added to the node file comprising the phylum taxonomy at the genus level and the ARG type or the ARG subtype. The network analysis was visualized using the interactive platform Gephi (v 0.9.2) (Bastian et al., 2009).
Data were presented as mean ± SD and analyzed using one-way analysis of variance (ANOVA), followed by the Student–Newman–Keuls test (SNK) and Spearman’s correlation analysis using SPSS 22 statistical software. PCA analysis was performed using R (ade4 package, version 2.15.3), showing the different distribution of bacterial community between water and soil samples. Diversity analysis was used to demonstrate the diversities of ARGs and bacterial communities in different sampling sites. Simpson, Shannon, and inverse Simpson indices were calculated using R (vegan package, version 2.15.3). A p value < 0.05 was considered statistically significant.
The physical and biological characteristics of surface water samples are shown in Table 1. The sample pH ranged from 8.1 to 8.3, showing a significant difference among the five sampling sites. TDSs and total bacterial count showed a significant increase from upstream to downstream, that is, the total number of culturable bacteria was the lowest at the sampling site BGC and the highest at B219 (p < 0.05). Concerning the indicator bacteria, the amounts of E. coli showed no significant difference, ranging from 21 to 50 MPN/100 ml (Figure 2). Enterococcus amounts ranged from 18 to 183 MPN/100 ml, and sampling sites BGC and HY had the least amount of Enterococcus, followed by YN, B219, and KKDL. Notably, the KKDL site showed significantly high numbers of Enterococcus compared to the other four sampling sites. After 24-h incubation of the supernatant, Coliform and Enterococcus faecalis were cultured from all five samples, and Staphylococcus aureus was found except for the BGC site (Table 2). These results were proved by 16S rDNA gene identification and phylogenetic analysis (Supplementary Figure S1).
In total, 15 water samples and four soil samples were analyzed. The results of metagenomic sequencing showed a significant difference between the community composition of soil and surface water samples (Figure 4B). Proteobacteria was the most abundant phylum in water samples followed by Bacteroidetes (Figure 3A). In addition to BGC, Cyanobacteria and Planctomycetes were also found in water samples, Proteobacteria showed a higher abundance (75.3%) in BGC samples than others (p < 0.05). Importantly, Acidobacteria, Thaumarchaeota, Gemmatimonadetes, and Chloroflexi were also detected in the soil samples, which showed higher species abundance than water samples (Figure 3A).
FIGURE 3. Relative abundance of bacterial groups of top 10 genera. Phylum level (A); genus level (B). BGC, YN, KKDL, HY, and B219 refer to the relative abundance of bacterial community in water samples, S. BGC, S.YN, S. KKDL, and S.HY refer to the relative abundance of bacterial community in soil samples.
As for the genus level, the bacterial community in the water samples of BGC showed a higher α-diversity than other sites (Table 3). Pseudomonas (most abundant), Hydrogenophaga, Limnobacter, and Flavobacterium were the dominant bacteria in water samples (Figure 3B). This was completely different from the soil samples, which showed a higher abundance of Nocardioides and Sphingomonas. Pseudomonas in BGC and YN water samples showed higher relative abundance than the other sampling sites, while B219 had the highest abundance of Flavobacterium (Supplementary Figure S2). The relative abundance of Streptomyces (almost 5.3%) was higher in BGC samples than in other soil samples. All these indicated the different relative abundance of species at different sampling sites. In addition, the species abundance of water and soil samples varied within the same sampling site. These results agreed with PCA of the water and soil samples (Figure 4A).
As shown in Figure 5A, the composition of ARGs in water samples is more complex than that in soil samples. Also, almost all soil ARGs were present in water samples. Among the top 20 ARGs, 13 belong to antibiotic efflux genes that were extensively present in both water and soil samples. The top 20 ARGs were classified into multidrug resistance, fluoroquinolone, aminoglycoside, and tetracycline resistance genes. The relative abundance of multidrug resistance gene adeF was highest (40–95 ppm) among all target ARGs and distributed in all the water and soil samples. This indicated fluoroquinolones, tetracyclines, or ARB pollution in the whole Ili River.
FIGURE 5. Diversity and relative abundance of ARGs. (A) Diversity analysis of ARGs in all water samples; (B) relative abundance of ARGs of top 20.
Notably, the relative abundance of ARGs at the BGC site was higher than that at the other sampling sites, and the overall trend was BGC > YN > KKDL ≈ HY > B219. Diversity analysis also showed a higher α-diversity of ARGs in BGC samples (Figure 5B). The target ARGs in riverbank were approximately the same (Figure 6). Among the top 10 selected ARGs in water samples, the relative abundance of multidrug ARGs smeF was higher in the YN samples than in the other sampling sites (Supplementary Figure S3), but the other seven ARGs (adeF, ANT2-Ia, APH3-Ib, APH6-Id, qacH, sul1, and tet39) were higher in BGC samples (Supplementary Figure S3). ACC-3 was mainly found in the soil samples of KKDL (35 ppm) while remaining undetected in other soil samples and water samples. Also, the bacteria of BGC sites primarily carried fluoroquinolone, tetracycline, aminoglycoside, and sulfonamide ARGs, while multidrug ARGs TEM-116 was mainly carried by the bacteria of the KKDL site.
FIGURE 6. Distribution of ARGs in every sampling sites. The ARG classes on the left and the sampling locations on the right side are presented as colored bands. Bar lengths on the outer ring represent the percentage of ARGs in each sample. The inner layer numbers indicate the relative abundances of ARG classes from a given sample (left) and the relative abundance of specific ARG classes in samples (right).
Antibiotic susceptibility testing of the total bacteria suggested that YN showed the utmost variety of culturable ARB, while BGC and B219 showed least diversity (Figure 7A). In addition to ampicillin, all the water samples were resistant to vancomycin, which is considered the last line of defense (Figure 7B; Supplementary Tables S1–S4). Enterococcus faecalis, purified from water samples of YN, HY, and B219, was resistant to several antibiotics, suggesting the serious pollution of antibiotic-resistant Enterococcus faecalis. Enterococcus strains were resistant to Streptomycin (Supplementary Table S2). Antibiotic-resistant Staphylococcus aureus was detected only in YN and KKDL samples, while Staphylococcus aureus from HY and B219 was not antibiotic-resistant (Supplementary Table S3). Coliform acquired from the water samples of YN was resistant to multiple antibiotics, especially at downstream sampling sites (Supplementary Table S4). In general, Enterococcus faecalis and E. coli were resistant to a wider variety of antibiotics. The ARB pollution was more serious at YN and KKDL sites, which can be attributed to crowd activities.
FIGURE 7. Numbers of antibiotic-resistant bacteria. (A) The numbers of antibiotic-resistant bacteria in different sampling sites. (B) The numbers of bacteria resistant to different antibiotics.
To investigate the association between ARGs and the bacterial community, a correlation analysis was carried out between the relative abundance of ARGs (based on CARD analysis) and bacteria. The results indicated a significant correlation between ARGs and bacteria in the Ili River. As shown in Supplementary Figure S4, Proteobacteria carried the most multidrug ARGs (61%), and Bacteroidetes mostly carried fluoroquinolone ARGs (such as mfpA and mdtk), macrolide, and glycopeptide ARGs. AxyY and pgpB negatively correlated with Actinobacteria (Figure 8A), indicating their low transfer risk. Also, aminoglycoside APH3-Ib and sulfonamide sul1 positively correlated with many kinds of MGEs (Figure 8B), indicating a high risk of horizontal transfer.
FIGURE 8. Correlation between ARGs and bacteria, and ARGs and MGEs based on network analysis. Co-occurrence patterns between the bacteria and ARG subtypes (A); ARG subtypes and MGEs (B). The red line indicates a positive correlation between two nodes. The blue line represents a negative correlation. The size of each node is proportional to its number of connections.
The Ili River is important for agriculture, husbandry, and industrial activities in Xinjiang Province, China. Drought and scarce precipitation promote the accumulation of ARB and ARGs in the Ili River, causing serious challenge to humans, agriculture, and animal husbandry.
The present study examined the bacterial community and ARG profile in the Ili River. The concentration of fecal indicator bacteria (FIB) is routinely used to report the bacterial pollution in the water environment. This study showed that the total bacteria, E. coli, and Enterococcus were significantly lower in the Ili River than in other rivers with frequent human activities, such as the Haihe and the Songhua rivers (Lee et al., 2013; Ren et al., 2020; Wang et al., 2017). It can be attributed to relatively low population density along the Ili River (Ili Kazakh Autonomous Prefecture 10 people/km2, Haihe River Basin 384 people/km2), which emphasizes that bacterial pollution in rivers is potentially affected by human activities. Similarly, the number of non-redundant genes in the sparsely populated town (BGC) was considerably lower than that in crowded cities (YN and KKDL) and grazing areas (HY and B219) (Supplementary Figure S5). This again suggested that the bacterial pollution in rivers probably originating from human activities or animal husbandry pollution. Also, the higher levels of indicator Enterococcus in KKDL samples (urban areas) indicated that this river environment might be extensively affected by urban sewage discharge (Lutterodt et al., 2012; Lu et al., 2018). In addition, TDSs and total bacterial count were significantly higher at downstream sites, suggesting the poorer water quality downstream than upstream regions.
Analyzing the types of ARGs, fluoroquinolone, aminoglycoside, sulfonamide, and tetracycline resistance genes were the most prevailing types in the Ili River. The antibiotic susceptibility testing also indicated that isolates from the Ili River displayed resistance to fluoroquinolone, aminoglycoside, sulfonamide, chloramphenicol, polypeptide, and tetracycline antibiotics. Previous studies report that tetracycline and sulfonamide resistance genes were prominent in livestock manures (Ji et al., 2012); fluoroquinolone, sulfonamide, and tetracycline are also frequently used in animal husbandry (Zhao et al., 2010), while aminoglycoside, macrolides, and lactams are used in a hospital setting (Jia et al., 2014). Therefore, the ARG pollution in the Ili River might result from animal manures, community sewage, or medical waste. Getahun et al. (Agga et al., 2019) found that the concentration of ARGs remained consistently higher in the feeding area than in the grazing area in the pasture–feedlot-type setting for beef cattle cultivation. Likewise, the free grazing farming in Xinjiang may alter the distribution of ARGs elsewhere. Compared with traditional husbandry (Ji et al., 2012), the ARGs in the Ili River showed significantly lower relative abundance such as for sulfonamide (approximately 10−6 to 10−5 in Ili River, 10−5 to 10−2 in animal husbandry) and tetracycline resistance genes (approximately 10−7 to 10−5 in the Ili River, 10−6 to 10−3 in animal husbandry). Accordingly, the four downstream sites showed no significant difference in the ARGs. Therefore, in contrast to traditional animal husbandry (Ji et al., 2012; Zhu et al., 2013; Agga et al., 2019), we speculate that free grazing did not cause severe pollution of ARGs in the Ili River.
Magnanimous evidence indicates that human activities exacerbate the spread of ARGs (Chen et al., 2019). The present study also suggested the same. This raises the question of whether the presence of an abundant bacterial community would be a potential reservoir for ARGs. This study found that the upstream sampling site BGC had the lowest total bacterial count but the highest abundance of ARGs. The phenomenon can be explained as follows: 1. Proteobacteria showed the highest relative abundance in BGC samples, which carried the most ARGs (61%); therefore, it led to the highest abundance even with a low total bacterial count. 2. Proteobacteria showed a significant relation with adeF, which accounted for almost 15% of the total ARGs, adeF, and thus an antibiotic efflux-related gene might induce other multidrug resistance genes. 3. Much evidence indicates that the spread of ARGs greatly contributes to severe pollution situations (Munro, 2015; Han et al., 2018); apart from adeF, other ARGs such as APH3-Ib, sul1, tet39, APH6-Id, and ANT2-Ia accounted for about 30.5% abundance in BGC samples, which positively correlated with many kinds of MGEs that possibly aggravated the spread of ARGs.
Normally, the abundance of the total ARGs reduces with water flow (Xu et al., 2015; Liu et al., 2019). This study also supported this as the total and relative abundances of seven types of ARGs (adeF, ANT2-Ia, APH3-Ib, APH6-Id, qacH, sul1, and tet39) were significantly higher in BGC samples than in the downstream sites, and the diversity analysis indicated that a higher α-diversity of ARGs was observed in BGC samples than in other sites. The other possible cause might be the inflow of additional tributaries. As the bacteria are critical hosts of ARGs (Zhou et al., 2017), similar composition of the bacterial community in the four downstream sampling sites led to a similar occurrence and distribution of ARGs.
The potential main hosts of ARGs in the Ili River were Proteobacteria, Bacteroidetes, and Actinobacteria, which is consistent with other findings in the Yellow River Delta, Chongqing municipality, and the Karst River (Li et al., 2020; Wang et al., 2020; Xiang et al., 2020); however, the distribution of ARGs was different in different studies. It is reported that human activity promoted the spread of sulfonamide and tetracycline resistance genes in many rivers like the Yangtze River, Liaohe River, and the northern Yellow Sea, while this study found the fluoroquinolone, tetracycline, and aminoglycoside resistance genes as the most abundant ARGs. This could be due to different antibiotic usage in this area. The frequently reported antibiotics in both influents and effluents include sulfonamides and tetracyclines ranged from a few ng/L to 10 μg/L (Qiao et al., 2018), while some studies in Xinjiang showed that quinolone was present in the highest concentration with hundreds to thousands ng/L, followed by sulfonamides and tetracyclines ranging from tens to hundreds ng/L along the sewage treatment process (Liu et al., 2017). Previous studies demonstrated the correlation between ARGs and other pollutants induced by human activity, such as the concentration of antibiotics, heavy metals, sewage, and livestock (Zhou et al., 2017; Zhang et al., 2018; Ma et al., 2019; Ohore et al., 2020). Therefore, lower antibiotic discharge may alleviate the pressure of ARG pollution. The relative abundance of sulfonamide resistance genes ranged from 4.12 × 10−5 to 2.47 × 10−2 in the Ba River, Xi’an (Guan et al., 2018); however, the most abundant ARGs (adeF) in the Ili River ranged from 9.49 × 10−6 to 1.09 × 10−4. These results indicated that the relative abundance of ARGs in the Ili River was much lower than that in outflow rivers due to the low population density and lesser use of antibiotics in this region. Also, the different usage of antibiotics between free grazing and intensive farming areas seems to be a critical factor. Overall, the humanities and environmental factors that caused the different discharge of ARGs must be further explored. The concentration of antibiotics, heavy metals, physicochemical properties of the river water, season, and rainfall should be evaluated to examine the source identification, prevalence, and subduction of the bacterial community and ARGs in the Ili River.
This study showed that TDSs, total bacterial count, E. coli, and Enterococcus were significantly lower in upstream sampling sites than in the downstream, indicating a deteriorating river environment with increased human and animal husbandry activities. However, the relative abundance of ARGs in the Ili River was much lower than that in outflow rivers; free grazing did not cause severe pollution of ARGs in the Ili River. Fluoroquinolone, aminoglycoside, sulfonamide, and tetracycline resistance genes were the most prevailing types in the Ili River, and the ARGs in crowded cities and grazing area showed no significant difference. The present study first investigated the occurrence and distribution pattern of ARGs in the Ili River and demonstrated the potential risk of ARGs, which will provide a profitable reference to the treatment of water environment of Ili River.
The sequencing data have been uploaded to https://www.ebi.ac.uk/ena/browser/view/PRJEB49834? Accession: PRJEB49834.
The datasets presented in this study can be found in online repositories. The names of the repository/repositories and accession number(s) can be found below: https://www.ebi.ac.uk/ena/browser/view/PRJEB49834?
XY and LY completed the experiments and manuscript. YY, HZ, and SW collected all samples. YC, BX, and CL analyzed the data. ZS, JW, and ZQ designed this study.
This research was supported by the National Natural Science Foundation of China (grant no. 42177414), the National Key Research and Development Program of China (grant no. AWS18J004), and the Natural Science Foundation of Tianjin city (grant no. 17JCZDJC39100).
The authors declare that the research was conducted in the absence of any commercial or financial relationships that could be construed as a potential conflict of interest.
All claims expressed in this article are solely those of the authors and do not necessarily represent those of their affiliated organizations, or those of the publisher, the editors, and the reviewers. Any product that may be evaluated in this article, or claim that may be made by its manufacturer, is not guaranteed or endorsed by the publisher.
We appreciate Zhuosong Cao and Chao Gao for their help in the experiments.
The Supplementary Material for this article can be found online at: https://www.frontiersin.org/articles/10.3389/fenvs.2022.840428/full#supplementary-material
Agga, G. E., Cook, K. L., Netthisinghe, A. M. P., Gilfillen, R. A., Woosley, P. B., and Sistani, K. R. (2019). Persistence of Antibiotic Resistance Genes in Beef Cattle Backgrounding Environment over Two Years after Cessation of Operation. PLoS One 14, e0212510. doi:10.1371/journal.pone.0212510
Aydin, S., Aydin, M. E., Ulvi, A., and Kilic, H. (2019). Antibiotics in Hospital Effluents: Occurrence, Contribution to Urban Wastewater, Removal in a Wastewater Treatment Plant, and Environmental Risk Assessment. Environ. Sci. Pollut. Res. 26, 544–558. doi:10.1007/s11356-018-3563-0
Boehm, A. B., Graham, K. E., and Jennings, W. C. (2018). Can We Swim yet? Systematic Review, Meta-Analysis, and Risk Assessment of Aging Sewage in Surface Waters. Environ. Sci. Technol. 52, 9634–9645. doi:10.1021/acs.est.8b01948
Boelee, E., Geerling, G., van der Zaan, B., Blauw, A., and Vethaak, A. D. (2019). Water and Health: From Environmental Pressures to Integrated Responses. Acta Tropica 193, 217–226. doi:10.1016/j.actatropica.2019.03.011
Chen, J., Su, Z., Dai, T., Huang, B., Mu, Q., Zhang, Y., et al. (2019). Occurrence and Distribution of Antibiotic Resistance Genes in the Sediments of the East China Sea Bays. J. Environ. Sci. 81, 156–167. doi:10.1016/j.jes.2019.01.016
Cuadrat, R. R. C., Sorokina, M., Andrade, B. G., Goris, T., and Dávila, A. M. R. (2020). Global Ocean Resistome Revealed: Exploring Antibiotic Resistance Gene Abundance and Distribution in TARA Oceans Samples. Gigascience 9. doi:10.1093/gigascience/giaa046
Dang, B., Mao, D., Xu, Y., and Luo, Y. (2017). Conjugative Multi-Resistant Plasmids in Haihe River and Their Impacts on the Abundance and Spatial Distribution of Antibiotic Resistance Genes. Water Res. 111, 81–91. doi:10.1016/j.watres.2016.12.046
Guan, Y., Jia, J., Wu, L., Xue, X., Zhang, G., and Wang, Z. (2018). Analysis of Bacterial Community Characteristics, Abundance of Antibiotics and Antibiotic Resistance Genes along a Pollution Gradient of Ba River in Xi'an, China. Front. Microbiol. 9, 3191. doi:10.3389/fmicb.2018.03191
Guo, F., Li, B., Yang, Y., Deng, Y., Qiu, J.-W., Li, X., et al. (2016). Impacts of Human Activities on Distribution of Sulfate-Reducing Prokaryotes and Antibiotic Resistance Genes in marine Coastal Sediments of Hong Kong. FEMS Microbiol. Ecol. 92, fiw128. doi:10.1093/femsec/fiw128
Guo, X., Feng, C., Gu, E., Tian, C., and Shen, Z. (2019). Spatial Distribution, Source Apportionment and Risk Assessment of Antibiotics in the Surface Water and Sediments of the Yangtze Estuary. Sci. Total Environ. 671, 548–557. doi:10.1016/j.scitotenv.2019.03.393
Han, J., Pendleton, S. J., Deck, J., Singh, R., Gilbert, J., Johnson, T. J., et al. (2018). Impact of Co-carriage of IncA/C Plasmids with Additional Plasmids on the Transfer of Antimicrobial Resistance in Salmonella enterica Isolates. Int. J. Food Microbiol. 271, 77–84. doi:10.1016/j.ijfoodmicro.2018.01.018
Hu, A., Ju, F., Hou, L., Li, J., Yang, X., Wang, H., et al. (2017). Strong Impact of Anthropogenic Contamination on the Co-occurrence Patterns of a Riverine Microbial Community. Environ. Microbiol. 19, 4993–5009. doi:10.1111/1462-2920.13942
Jang, H. M., Kim, Y. B., Choi, S., Lee, Y., Shin, S. G., Unno, T., et al. (2018). Prevalence of Antibiotic Resistance Genes from Effluent of Coastal Aquaculture, South Korea. Environ. Pollut. 233, 1049–1057. doi:10.1016/j.envpol.2017.10.006
Jennings, W. C., Chern, E. C., O'Donohue, D., Kellogg, M. G., and Boehm, A. B. (2018). Frequent Detection of a Human Fecal Indicator in the Urban Ocean: Environmental Drivers and Covariation with Enterococci. Environ. Sci. Process. Impacts 20, 480–492. doi:10.1039/c7em00594f
Ji, X., Shen, Q., Liu, F., Ma, J., Xu, G., Wang, Y., et al. (2012). Antibiotic Resistance Gene Abundances Associated with Antibiotics and Heavy Metals in Animal Manures and Agricultural Soils Adjacent to Feedlots in Shanghai; China. J. Hazard. Mater. 235-236, 178–185. doi:10.1016/j.jhazmat.2012.07.040
Jia, W., Li, G., and Wang, W. (2014). Prevalence and Antimicrobial Resistance of Enterococcus Species: a Hospital-Based Study in China. Ijerph 11, 3424–3442. doi:10.3390/ijerph110303424
Johnson, P. T. J., Preston, D. L., Hoverman, J. T., Henderson, J. S., Paull, S. H., Richgels, K. L. D., et al. (2012). Species Diversity Reduces Parasite Infection through Cross-Generational Effects on Host Abundance. Ecology 93, 56–64. doi:10.1890/11-0636.1
Karkman, A., Pärnänen, K., and Larsson, D. G. J. (2019). Fecal Pollution Can Explain Antibiotic Resistance Gene Abundances in Anthropogenically Impacted Environments. Nat. Commun. 10, 80. doi:10.1038/s41467-018-07992-3
Landrigan, P. J., Stegeman, J. J., Fleming, L. E., Allemand, D., Anderson, D. M., Backer, L. C., et al. (2020). Human Health and Ocean Pollution. Ann. Glob. Health 86, 151. doi:10.5334/aogh.2831
Ledwaba, S. E., Becker, P., Traore-Hoffman, A., and Potgieter, N. (2019). Bacterial Contamination of Children's Toys in Rural Day Care Centres and Households in South Africa. Ijerph 16, 2900. doi:10.3390/ijerph16162900
Lee, G.-C., Jheong, W.-H., Kim, M.-j., Choi, D. H., and Baik, K.-H. (2013). A 5-year Survey (2007-2011) of Enteric Viruses in Korean Aquatic Environments and the Use of Coliforms as Viral Indicators. Microbiol. Immunol. 57, 46–53. doi:10.1111/j.1348-0421.2012.00515.x
Li, Y., Zeng, C., and Long, M. (2020). Variation of Soil Nutrients and Bacterial Community Diversity of Different Land Utilization Types in Yangtze River Basin, Chongqing Municipality. PeerJ 8, e9386. doi:10.7717/peerj.9386
Liu, J., Lu, J., Tong, Y., and Li, C. (2017). Occurrence and Elimination of Antibiotics in Three Sewage Treatment Plants with Different Treatment Technologies in Urumqi and Shihezi, Xinjiang. Water Sci. Technol. 75, 1474–1484. doi:10.2166/wst.2017.013
Liu, X., Zhang, G., Liu, Y., Lu, S., Qin, P., Guo, X., et al. (2019). Occurrence and Fate of Antibiotics and Antibiotic Resistance Genes in Typical Urban Water of Beijing, China. Environ. Pollut. 246, 163–173. doi:10.1016/j.envpol.2018.12.005
Lu, J., Wu, J., Zhang, C., Zhang, Y., Lin, Y., and Luo, Y. (2018). Occurrence, Distribution, and Ecological-Health Risks of Selected Antibiotics in Coastal Waters along the Coastline of China. Sci. Total Environ. 644, 1469–1476. doi:10.1016/j.scitotenv.2018.07.096
Lutterodt, G., Foppen, J. W. A., and Uhlenbrook, S. (2012). Transport of Escherichia coli Strains Isolated from Natural spring Water. J. Contaminant Hydrol. 140-141, 12–20. doi:10.1016/j.jconhyd.2012.08.011
Ma, X., Guo, N., Ren, S., Wang, S., and Wang, Y. (2019). Response of Antibiotic Resistance to the Co-existence of Chloramphenicol and Copper during Bio-Electrochemical Treatment of Antibiotic-Containing Wastewater. Environ. Int. 126, 127–133. doi:10.1016/j.envint.2019.02.002
Molina, M., Hunter, S., Cyterski, M., Peed, L. A., Kelty, C. A., Sivaganesan, M., et al. (2014). Factors Affecting the Presence of Human-Associated and Fecal Indicator Real-Time Quantitative PCR Genetic Markers in Urban-Impacted Recreational Beaches. Water Res. 64, 196–208. doi:10.1016/j.watres.2014.06.036
Munro, N. (2015). Antimicrobial Resistance. AACN Adv. Crit. Care 26, 225–230. doi:10.4037/nci.0000000000000102
Ohore, O. E., Addo, F. G., Han, N., Li, X., and Zhang, S. (2020). Profiles of ARGs and Their Relationships with Antibiotics, Metals and Environmental Parameters in Vertical Sediment Layers of three lakes in China. J. Environ. Manage. 255, 109583. doi:10.1016/j.jenvman.2019.109583
Pantanella, F., Lekunberri, I., Gagliardi, A., Venuto, G., Sànchez-Melsió, A., Fabiani, M., et al. (2020). Effect of Urban Wastewater Discharge on the Abundance of Antibiotic Resistance Genes and Antibiotic-Resistant Escherichia coli in Two Italian Rivers. Ijerph 17, 6813. doi:10.3390/ijerph17186813
Qiao, M., Ying, G.-G., Singer, A. C., and Zhu, Y.-G. (2018). Review of Antibiotic Resistance in China and its Environment. Environ. Int. 110, 160–172. doi:10.1016/j.envint.2017.10.016
Ren, W., Su, X., Zhang, X., Chen, Y., and Shi, Y. (2020). Influence of Hydraulic Gradient and Temperature on the Migration of E. coli in Saturated Porous media during Bank Filtration: a Case Study at the Second Songhua River, Songyuan, Northeastern China. Environ. Geochem. Health 42, 1977–1990. doi:10.1007/s10653-019-00459-4
Rodríguez-Tapia, L., and Morales-Novelo, J. (2017). Bacterial Pollution in River Waters and Gastrointestinal Diseases. Ijerph 14, 479. doi:10.3390/ijerph14050479
Sercu, B., Van De Werfhorst, L. C., Murray, J. L. S., and Holden, P. A. (2011). Cultivation-independent Analysis of Bacteria in IDEXX Quanti-Tray/2000 Fecal Indicator Assays. Appl. Environ. Microbiol. 77, 627–633. doi:10.1128/AEM.01113-10
Sharma, V. K., Johnson, N., Cizmas, L., McDonald, T. J., and Kim, H. (2016). A Review of the Influence of Treatment Strategies on Antibiotic Resistant Bacteria and Antibiotic Resistance Genes. Chemosphere 150, 702–714. doi:10.1016/j.chemosphere.2015.12.084
Sinclair, R. G., Somsamouth, K., Sahar, D., Englert, R., and Singh, P. (2021). Microbial Contamination in the Communal-Use Lao Tobacco Waterpipe. Int. Health 13, 344–349. doi:10.1093/inthealth/ihaa078
Stachler, E., Kelty, C., Sivaganesan, M., Li, X., Bibby, K., and Shanks, O. C. (2017). Quantitative CrAssphage PCR Assays for Human Fecal Pollution Measurement. Environ. Sci. Technol. 51, 9146–9154. doi:10.1021/acs.est.7b02703
Stange, C., Yin, D., Xu, T., Guo, X., Schäfer, C., and Tiehm, A. (2019). Distribution of Clinically Relevant Antibiotic Resistance Genes in Lake Tai, China. Sci. Total Environ. 655, 337–346. doi:10.1016/j.scitotenv.2018.11.211
Uprety, S., Dangol, B., Nakarmi, P., Dhakal, I., Sherchan, S. P., Shisler, J. L., et al. (2020). Assessment of Microbial Risks by Characterization of Escherichia coli Presence to Analyze the Public Health Risks from Poor Water Quality in Nepal. Int. J. Hyg. Environ. Health 226, 113484. doi:10.1016/j.ijheh.2020.113484
Vadde, K. K., McCarthy, A. J., Rong, R., and Sekar, R. (2019). Quantification of Microbial Source Tracking and Pathogenic Bacterial Markers in Water and Sediments of Tiaoxi River (Taihu Watershed). Front. Microbiol. 10, 699. doi:10.3389/fmicb.2019.00699
Wang, J., Wang, J., Zhang, Z., Li, Z., Zhang, Z., Zhao, D., et al. (2020). Shifts in the Bacterial Population and Ecosystem Functions in Response to Vegetation in the Yellow River Delta Wetlands. mSystems 5. doi:10.1128/mSystems.00412-20
Wang, Y., Chen, Y., Zheng, X., Gui, C., and Wei, Y. (2017). Spatio-temporal Distribution of Fecal Indicators in three rivers of the Haihe River Basin, China. Environ. Sci. Pollut. Res. 24, 9036–9047. doi:10.1007/s11356-015-5907-3
Wang, Z., Du, Y., Yang, C., Liu, X., Zhang, J., Li, E., et al. (2017). Occurrence and Ecological hazard Assessment of Selected Antibiotics in the Surface Waters in and Around Lake Honghu, China. Sci. Total Environ. 609, 1423–1432. doi:10.1016/j.scitotenv.2017.08.009
Wang, Z., Han, M., Li, E., Liu, X., Wei, H., Yang, C., et al. (2020). Distribution of Antibiotic Resistance Genes in an Agriculturally Disturbed lake in China: Their Links with Microbial Communities, Antibiotics, and Water Quality. J. Hazard. Mater. 393, 122426. doi:10.1016/j.jhazmat.2020.122426
Xiang, S., Wang, X., Ma, W., Liu, X., Zhang, B., Huang, F., et al. (2020). Response of Microbial Communities of Karst River Water to Antibiotics and Microbial Source Tracking for Antibiotics. Sci. Total Environ. 706, 135730. doi:10.1016/j.scitotenv.2019.135730
Xu, J., Xu, Y., Wang, H., Guo, C., Qiu, H., He, Y., et al. (2015). Occurrence of Antibiotics and Antibiotic Resistance Genes in a Sewage Treatment Plant and its Effluent-Receiving River. Chemosphere 119, 1379–1385. doi:10.1016/j.chemosphere.2014.02.040
Yang, Y., Song, W., Lin, H., Wang, W., Du, L., and Xing, W. (2018). Antibiotics and Antibiotic Resistance Genes in Global Lakes: A Review and Meta-Analysis. Environ. Int. 116, 60–73. doi:10.1016/j.envint.2018.04.011
Zhang, G., Lu, S., Wang, Y., Liu, X., Liu, Y., Xu, J., et al. (2020). Occurrence of Antibiotics and Antibiotic Resistance Genes and Their Correlations in Lower Yangtze River, China. Environ. Pollut. 257, 113365. doi:10.1016/j.envpol.2019.113365
Zhang, W., Suyamud, B., Lohwacharin, J., and Yang, Y. (2021). Large-scale Pattern of Resistance Genes and Bacterial Community in the Tap Water along the Middle and Low Reaches of the Yangtze River. Ecotoxicology Environ. Saf. 208, 111517. doi:10.1016/j.ecoenv.2020.111517
Zhang, Y., Niu, Z., Zhang, Y., and Zhang, K. (2018). Occurrence of Intracellular and Extracellular Antibiotic Resistance Genes in Coastal Areas of Bohai Bay (China) and the Factors Affecting Them. Environ. Pollut. 236, 126–136. doi:10.1016/j.envpol.2018.01.033
Zhao, L., Dong, Y. H., and Wang, H. (2010). Residues of Veterinary Antibiotics in Manures from Feedlot Livestock in Eight Provinces of China. Sci. Total Environ. 408, 1069–1075. doi:10.1016/j.scitotenv.2009.11.014
Zheng, J., Gao, R., Wei, Y., Chen, T., Fan, J., Zhou, Z., et al. (2017). High-throughput Profiling and Analysis of Antibiotic Resistance Genes in East Tiaoxi River, China. Environ. Pollut. 230, 648–654. doi:10.1016/j.envpol.2017.07.025
Zhou, Z.-C., Zheng, J., Wei, Y.-Y., Chen, T., Dahlgren, R. A., Shang, X., et al. (2017). Antibiotic Resistance Genes in an Urban River as Impacted by Bacterial Community and Physicochemical Parameters. Environ. Sci. Pollut. Res. 24, 23753–23762. doi:10.1007/s11356-017-0032-0
Keywords: bacterial community, antibiotic resistance genes, indicator bacteria, metagenomic analysis, antibiotic-resistant bacteria
Citation: Yang X, Yan L, Yang Y, Zhou H, Cao Y, Wang S, Xue B, Li C, Zhao C, Zhang X, Shen Z, Wang J and Qiu Z (2022) The Occurrence and Distribution Pattern of Antibiotic Resistance Genes and Bacterial Community in the Ili River. Front. Environ. Sci. 10:840428. doi: 10.3389/fenvs.2022.840428
Received: 21 December 2021; Accepted: 21 February 2022;
Published: 10 March 2022.
Edited by:
Marcelo Pedrosa Gomes, Federal University of Paraná, BrazilReviewed by:
Zeyou Chen, Nankai University, ChinaCopyright © 2022 Yang, Yan, Yang, Zhou, Cao, Wang, Xue, Li, Zhao, Zhang, Shen, Wang and Qiu. This is an open-access article distributed under the terms of the Creative Commons Attribution License (CC BY). The use, distribution or reproduction in other forums is permitted, provided the original author(s) and the copyright owner(s) are credited and that the original publication in this journal is cited, in accordance with accepted academic practice. No use, distribution or reproduction is permitted which does not comply with these terms.
*Correspondence: Zhigang Qiu, emhpZ2FuZ3FpdTk5QGdtYWlsLmNvbQ==; Jingfeng Wang, amluZ2Zlbmd3YW5nQGhvdG1haWwuY29t
†These authors have contributed equally to this work
Disclaimer: All claims expressed in this article are solely those of the authors and do not necessarily represent those of their affiliated organizations, or those of the publisher, the editors and the reviewers. Any product that may be evaluated in this article or claim that may be made by its manufacturer is not guaranteed or endorsed by the publisher.
Research integrity at Frontiers
Learn more about the work of our research integrity team to safeguard the quality of each article we publish.