- 1Département de Chimie, Université de Sherbrooke, Sherbrooke, QC, Canada
- 2Environmental and Life Sciences Graduate Program, Trent University, Peterborough, ON, Canada
- 3Forensic Science Department, Trent University, Peterborough, ON, Canada
Mercury contamination in aquatic systems poses a serious environmental stress to phototrophic plankton. We used Euglena gracilis to gain an understanding of the physiochemical changes resulting from mercury stress across the transcriptome and metabolome. Using a combination of Fourier Transform Ion Cyclotron Resonance Mass Spectrometry (FT-ICR-MS) and RNA-sequencing, we identified metabolomic and transcriptomic changes both within and outside cellular space after mercury exposure. Metabolic profiles of E. gracilis were less diverse after mercury exposure, highlighting an overall refinement of metabolites produced. Significant fold changes in cysteine, glutathione, and amino acid-based metabolites were significantly higher (p < 0.05) within the mercury exposed cells and in extracellular space than in untreated cultures. Using integrated omics analyses, a significant upregulation of transcripts and metabolites involved in amino acid synthesis, cellular responses to chemical stress, reactive oxygen species detoxification, and electron transport were identified. Together the enrichment of these pathways highlights mechanisms that E. gracilis harness to mitigate oxidative stress at sublethal concentrations of mercury exposure and give rise to new biomarkers of environmental stress in the widely distributed E. gracilis.
Introduction
Since the industrial revolution, anthropogenic activities have contributed to increased mercury (Hg) concentrations in the environment. Despite environmental restrictions on emissions in 1950, legacy anthropogenic derived Hg still cycles around the world (Amos et al., 2013; Lepak et al., 2019). Oxidized Hg (Hg0) enters the atmosphere mainly because of combustion from smelting, manufacturing processes, and infrastructure development (Saiz-Lopez et al., 2018). Hg0 is globally dispersed, travelling in the atmosphere before being reduced to inorganic Hg (Hg (II)) and deposited to terrestrial and aquatic sources. As a result, Hg (II) contamination is a global issue that threatens neurological health in biota across aquatic and terrestrial systems.
The bioavailability and toxicity of dissolved metals is influenced by water chemistry, metal concentration, organism type, and site-specific interactions on biotic surfaces (Le Faucheur et al., 2014; Cheng et al., 2016). In microalgae low parts per million (ppm) concentrations of Hg (II) often results in increased plasma membrane permeability, chlorophyll degradation, reduced cellular growth, the loss of flagella, and the generation of toxic reactive oxygen species (ROS) that eventually results in cellular death (Clemens, 2006; Le Faucheur et al., 2014). While Hg toxicity varies based on algae species, natural concentrations of Hg in freshwater systems usually ranges between 0.5 and 12.7 ng L−1 (Driscoll et al., 2007), well below lethal exposure concentrations for most microalgae (Beauvais-Flück et al., 2017). However, sublethal Hg exposure has been shown to induce both genotypic and phenotypic changes in microalgae (Hughes et al., 2009; Slaveykova et al., 2021). Certain algae have developed mechanisms of metal tolerance allowing them to survive in contaminated systems. For example, the mechanisms involved in metal resistance have been previously explored in microalgae like Chlamydomonas reinhardtii and Chlorella vulgaris in response to cadmium, copper, lead, and recently, Hg exposure (Jamers et al., 2013; Beauvais-Flück et al., 2017; Slaveykova et al., 2021).
Unlike most algae, the flagellate protist Euglena gracilis is capable of meeting energy demands through both autotrophic and heterotrophic mechanisms (Wang et al., 2018). Physically, E. gracilis is distinct with a protein-rich pellicle, and a defining red eyespot that aids in phototaxis (Ebenezer et al., 2019). The large genome of E. gracilis, linked to a multi-endosymbiotic evolutionary history, yields a complex metabolism that has enabled its use in the production of compounds important to the food and pharmaceutical industries (Gissibl et al., 2019). Its high fatty acid and polyunsaturated fatty acid content have enabled its consideration in biodiesel production (Ebenezer et al., 2019; Gissibl et al., 2019; Wang et al., 2018). E. gracilis is also extremely resistant to high metal concentrations of Hg (II) (Devars et al., 1998), Cd2+ (Avilés et al., 2003), and Cu2+ (Winters et al., 2017), and is one of the main photosynthetic species found in industrial effluents (Ebenezer et al., 2019). In other studies that explore metal accumulation in E. gracilis, concentrations up to 1,000 mg L−1 or 5 mmol L−1 of Hg have been used due to the high levels of tolerance (Khatiwada et al., 2020a; Khatiwada et al., 2020b). The metabolic flexibility (Ebenezer et al., 2019; Inwongwan et al., 2019), resistance to acidic conditions (Olaveson and Nalewajko, 2000), and ability to grow in extreme environments (Rodríguez-Zavala et al., 2007) makes E. gracilis an ideal candidate to explore the link between transcriptomic and metabolomic adaptations in response to environmental stress like Hg (II) exposure.
Understanding the transcriptomic and metabolomic responses of euglenoids and other microalgae to Hg (II) exposure is important to further predict how increasingly contaminated environments can affect algal metabolism, Hg (II) distribution, and the total organic matter pool (Chételat and Amyot, 2009). To accomplish this goal, a comprehensive approach identifying the cellular mechanisms responsible for Hg tolerance is required. Combining different untargeted omics-based and transcriptomic methodologies are advantageous since they provide metabolomic and genetic insights into how organisms can respond to environmental stressors (Jamers et al., 2013; Ritter et al., 2014). While it is not possible to directly link the transcriptome to the metabolome, and structural information is not directly inferred without additional analyses, the combination of untargeted metabolomic and transcriptomic methodologies is advantageous in linking phenotypic changes across the overall transcriptome (Cavill et al., 2016). The synthesis of these methodologies when exploring how E. gracilis responds to Hg (II) stress reveals a wealth of unexplored information to the fields of ecotoxicology and bioremediation (Jamers et al., 2013).
In this study, we use transcriptomic and metabolomic analyses to identify cellular responses of E. gracilis after exposure to sublethal Hg (II) concentrations. For the first time, RNA-sequencing, differential gene expression and transcriptomic analyses were combined with an untargeted metabolomic approach using Fourier transform ion cyclotron resonance mass spectrometry (FT-ICR-MS) to identify changes in the metabolome of E. gracilis. Our findings highlight analogous changes across the transcriptome and metabolome related to ROS production, photosynthetic capabilities, and energy production, shedding light on the cellular mechanisms of Hg (II) resistance by E. gracilis.
Materials and Methods
Euglena Gracilis Cultivation, Metabolite Extraction, and Hg Speciation Modelling
E. gracilis (Strain 95) was obtained from the Canadian Phycological Culture Centre (Waterloo, Canada) and grown photoautotrophically at 21°C at a 16:8 light/dark photoperiod at a light intensity of 96 μmol photons m−2 s−1 in a Conviron (CMP5090) environmental chamber (Controlled Environments Ltd., Winnipeg, MB, Canada) in E. gracilis media (EGM) prepared at pH 3.5 (Winters et al., 2017). A total of six subcultures were established including three cultures as control groups and three cultures exposed to 5 μmol L−1 Hg(NO3)2 (ICP-MS Trace grade; SPEX Certiprep) in acid washed glassware for 5 days. The exposed Hg(II) concentration represents the lethal dose to reduce the cell population by 50% (LC50) and mimic a Hg contaminated environment like acid mine tailings (Ali et al., 2000). Cells were harvested after reaching mid exponential growth phase (after 5 days), determined by automatic cell counts (Countess II Fl, ThermoFisher Scientific) (Supplementary Figure S1). Sterile techniques were conducted throughout the cultivation of E. gracilis, but cultures were not necessarily axenic and factors such as algae-bacteria interactions and bacterial activities may have influenced the metabolite pool.
To obtain the extracellular biomolecule fraction, E. gracilis cultures were directly filtered through a 0.7 µm Whatman glass fiber filter (GF/F) and then a 0.45 µm cellulose nitrate filter into 20 ml pre combusted amber vials. Approximately 10 ml of MeOH (HPLC grade, Sigma) was added to the filtrate containing the extracellular biomolecules. The cellular fraction of intracellular biomolecules (combined methanol and water extracts; 50:50 v/v) was obtained after E. gracilis cells were washed three times with deionized water to remove culture medium from cells, lysed using a Retsch MM300 ball mill (Haan, Germany), and centrifuged at 8,000 rpm for approximately 5 min (ThermoScientific Sorvall Legend Micro 17 Centrifuge). The supernatant was collected, filtered through a 0.45 µm cellulose nitrate filter, and 5 ml of MeOH (HPLC grade, Sigma) was added. The intracellular and extracellular samples were separated and stored in a cool dark environment at 4°C for metabolome and transcriptome analyses.
In order to model speciation of Hg (II) in growth media, Visual MINTEQ ver. 3.10 (Gustafsson, 2010) was used. Briefly, the parameters used in the modeling included DOC (Gaussian DOM, 72.3 mg C L−1), 5 μmol L−1 Hg (II), 9.0 × 10–5 mol L−1 Ca2+, and 18 × 10–5 mol L−1 Cl−. The pH and temperature of the media were set to 3.5 and to 25°C, respectively, to represent algal cultivation conditions. After running simulations of Hg (II) exposure cultures, > 99% of Hg was in the form HgCl2 (aq), suggesting that the vast majority of Hg in culture media contributed to the effective exposure concentration of 5 μmol L−1.
Fourier Transform Ion Cyclotron Resonance Mass Spectrometry Analysis
A 7 T Bruker SolariX XR Fourier transform Ion Cyclotron Resonance Mass Spectrometer (FT-ICR-MS; Bruker Daltonics GmbH, Bremen, Germany) equipped with an electrospray ionization (ESI) and housed in the Trent Water Quality Center (Trent University, Ontario, Canada) was used for metabolite characterization. Samples were diluted to 5 mg L−1 carbon using a 50% MeOH: MilliQ mixture and the pH was adjusted to seven using NaOH (Trace Grade) immediately prior injection. A sodium trifluoroacetate (NaTFA) tuning mix was used to calibrate samples using three lock masses externally and internally (248.9603, 656.8848 and 928.8344 m/z). In total, three control samples and 3 Hg (II) treated extracellular and intracellular biomolecule treatments were acquired using negative ESI mode using a direct injection method (Supplementary Figures S3 and S4) (Mangal et al., 2019). Briefly, capillary voltages were 4500 V with a continuous flow rate of 120 μL h−1, and ion accumulation time of 0.45 s. Spectra were collected in absorption mode and 200 scans were coadded. Peak and formula assignment were conducted in Bruker DataAnalysis (v4.4) using the elemental constraints C(1–50), H(1–100), O(1–50), N(0–10), S(0–4), and P(0–3) 22 (Mangal et al., 2019). For all samples, MeOH, MQW, and EG media blanks were all subtracted from generated peak and formula lists of samples. A ±1 ppm mass tolerance and 1% relative intensity cut-off with a S/N ratio >4 was also applied to ensure accurate and significant peaks.34S,13C, and 15N isotopic peaks were also confirmed to ensure accurate formula assignment. After formula assignment, molecules that were confidently assigned were utilized for subsequent metabolite identification and statistical analyses.
Data Analysis and Visualization
On average, 5,203 ± 411 m/z molecules were assigned formulae in released exudates (Supplementary Figure S2) and 7,940 ± 523 m/z molecules were assigned formulae in cellular biomolecules (Supplementary Figure S3). Only common FT-ICR-MS peaks across all replicates in E. gracilis control (n = 3) and Hg (II) treated cultures (n = 3) were used for further analyses using an in-house script (Mangal et al., 2019). In total, 425 common formulae were annotated in cellular biomolecules and 693 common formulae were annotated in released exudates and were utilized for metabolite class and process annotation in Cytoscape. These common m/z peaks were exported to Cytoscape (v3.6.1) equipped with the MetaNetter2 plugin to semi-quantify and visualize 75 different transformations for amino acids, biomolecule classes, and common chemical processes that include enzymatic components, and metabolic process level reactions (Supplementary Figure S4) (Burgess et al., 2017; Mangal et al., 2019). The abundance of each transformation (node) was normalized based on the total number of transformations for a semi-quantifiable measurement of potential molecular transformations in metabolite abundance across samples (Supplementary Figure S3). Although Cytoscape transformations do not structurally identify metabolites, m/z shifts provide compositional insight into the putative metabolites with a high degree of accuracy (Mangal et al., 2020). The relative abundance of each metabolite in cellular biomolecules and released exudates (Supplementary Table S1) were then used in additional statistical analyses conducted in MetaboAnalyst (v 4.0).
Ribonucleic Acid Isolation, cDNA Library Preparation, and Ribonucleic Acid-Sequencing
To isolate E. gracilis RNA, 30 ml of each culture was centrifuged at 12,200 × g for 5 min at 4°C. The pellets were resuspended in 10 ml DEPC-treated water by vortexing and centrifuged at 12,200 × g for 5 min at 4°C to remove remaining EGM. The resulting pellets were immediately resuspended in 1.5 ml TRIzol reagent (Invitrogen) and transferred into 2 ml screw-cap tubes containing Lysing Matrix C (MP Biomedicals). Euglena gracilis cells were disrupted using a FastPrep-24 Instrument (MP Biomedicals, speed setting = 6.5 for 45 s). Tissue homogenization was conducted two times and the samples were cooled on ice for 1 min between cycles. The cell debris were pelleted by centrifugation at 12,200 g for 10 min at 4°C and the supernatant was transferred to 1.5 ml RNase-free microcentrifuge tubes. Total RNA was isolated using TRIzol reagent according to the manufacturer’s protocol. Total RNA was precipitated using RNA precipitation solution (0.8 M disodium citrate/1.2 M NaCl) and isopropanol (Sambrook and Russell, 2001), followed by a wash step using 75% ethanol, and a re-resuspension in nuclease-free water (Ambion). Total RNA was treated with dnase I (RNase-free, New England Biolabs), cleaned up using an RNA precipitation solution, and re-suspended in nuclease-free water.
Total RNA samples were sent to The Centre for Applied Genomics at The Hospital for Sick Children (Toronto, Canada) for quality assessment, cDNA library preparation, and RNA-sequencing. RNA quality was assessed using a Bioanalyzer (Agilent Technologies). Poly(A) mRNA was enriched using oligo dT-beads and cDNA libraries were prepared using the NEBNext Ultra Directional RNA Library Prep Kit for Illumina (New England BioLabs). Barcoded libraries were pooled in equimolar quantities, and the 12 libraries were sequenced on one lane of the HiSeq 2,500 System (Illumina Inc.), yielding 126 bp paired-end reads. Euglena gracilis cultures grown in the absence of Hg were designated as ‘control’ and E. gracilis cultures grown in the presence of Hg were designated as ‘Hg’ RNA-seq libraries.
De Novo Transcriptome Assembly
The sequence quality for the 12 datasets was assessed using FastQC v0.11.7 (Andrews, 2010) and we removed Illumina adapter sequences and low-quality bases from all sequences using Trimmomatic v0.36 (Bolger et al., 2014) with the settings ‘Illuminaclip:TruSeq3-PE-2. fa:2:30:10 sliding window:4:5 leading:5 tailing:5 minlen:25’. The quality trimmed sequences from all 12 libraries were used to generate a single de novo transcript fragment (transfrag) assembly for E. gracilis with Trinity v2.5.0 (Grabherr et al., 2011) with the setting ‘strand-specific mode RF’. We used BUSCO v3.0.2 and the ‘eukaryota_odb9’ dataset to assess the completeness of this de novo transfrag assembly (Waterhouse et al., 2018). The quality trimmed sequences from each library were aligned to the de novo transfrag assembly using Bowtie2 v2.3.3 (Langmead & Salzberg, 2012) with the setting ‘SS_lib_type RF’ and finally the transfrag abundance was estimated for each library using RSEM v.1.3 (Li and Dewey, 2011).
Differential Gene Expression Analysis
DESeq2 v1.16.1 (Love et al., 2014) and edgeR v3.18.1 (Robinson et al., 2010) modules in SARTools v1.5.0 (Varet et al., 2016) were used to assess differential gene expression (DGE) between the E. gracilis cultures grown in the absence of Hg and the E. gracilis cultures grown in the presence of Hg. We used the statistical model ‘∼ group’ for the comparisons and a false discovery rate (FDR) threshold <0.05 (Field et al., 2018). The settings for DESeq2 were: cooksCutoff = TRUE (perform outlier detection), independentFiltering = TRUE, alpha = 0.05 (threshold of statistical significance), pAdjustMethod = BH (Benjamini/Hochberg p-value adjustment method), typeTrans = rlog (transformation for PCA), and locfunc = median (estimate size factors). For edgeR we used the settings: alpha = 0.05, pAdjustMethod = BH, cpmCutoff = 5 (counts-per-million cut-off), normalization. Method = TMM (normalization across samples using the trimmed mean of M-values method). The two DGE analyses use different assumptions and yielded slightly different results; therefore, we focused on the intersect of E. gracilis genes identified by both DESeq2 and edgeR in downstream analyses. These genes were then characterized using NCBI blast 2.7.1 + blastx to search the NCBI non-redundant protein and SWISS-PROT databases (accessed October 2017) with an e-value threshold of 1E-5 to identify proteins with sequence similarity. To characterize genes represented by multiple transfrags, we used the blastx hit with the lowest e-value. To characterize genes for subsequent gene ontology (GO) enrichment analysis, we used blastx to search the Ensembl 90 (Aken et al., 2017) Arabidopsis thaliana, Chlamydomonas reinhardtii, Synechocystis sp., and Homo sapiens protein databases (accessed November 2017) to identify preexisting genes from plant and microalgae databases as well as identifying potentially unexplored pathways using the more comprehensive Homo sapiens database. The resulting gene lists were queried in the PANTHER gene ontology database (http://pantherdb.org/index.jsp) using default settings to test for statistical over-representation of GO-terms (Mi et al., 2019). Notably, blast analyses did not indicate any human sequences among the transcripts used in this analysis, suggesting minimal human contamination (Supplementary Table S2).
Statistical Analyses and Integration Analysis
To conduct multivariable statistical analyses for untargeted metabolomics, MetaboAnalyst (v4.0) was utilized (Chong & Xia, 2018; Chong et al., 2019). The 75 Cytoscape derived abundance of metabolites, metabolite classes and processes molecules were uploaded using the statistical analysis module in an unpaired concentration table. Compiled datasets were normalized by sample-specific normalization by factor of 1.0 prior to subsequent analyses. A partial least square-discriminant analysis (PLS-DA) was conducted that included all 75 different metabolites, classes, and processes to explore relationships between both cellular and exudate metabolites and changes in metabolite abundance with Hg exposure. To ensure the predictability and fitting of the PLS-DA model, or Q2 estimate, a cross validation using five components revealed a Q2 score of 0.7, indicating a significantly predictive model (Szymańska et al., 2012). Variable important in position (VIP) scores were determined as an assessment of variable importance on multivariable analyses. Log fold changes were determined based on Cytoscape molecular abundance (Mangal et al., 2019), for released metabolites and genes and displayed as fold change volcano plots. While all 75 metabolite compounds, classes, and processes were included in the VIP analyses, only the 15 most significantly changed transformations between control and Hg treated cultures were reported. Significant differences for metabolite proportion and gene expression were determined by a one-way analysis of variance (ANOVA) with a Tukey honesty significance differences (HSD) test.
To provide a more detailed comparison between transcriptomic and metabolomic data, an Integrated Molecular Pathway Level Analysis (IMPaLA) was conducted (Kamburov et al., 2011). Enriched transcripts and intracellular metabolites after Hg exposure in E. gracilis cultures were used as inputs for over-representation pathway analyses. Integrated pathways that contained transcripts and metabolites were considered significant when joint p-values were <0.001 (Cavill et al., 2016).
Results
Changes in Metabolite Production With Hg Exposure
Partial least squares-discriminant analyses (PLS-DA) of the relative abundances of metabolites obtained by Cytoscape network analysis was conducted for discriminating metabolite profiles between control and treated cells. An overall refinement in the released metabolite (Figure 1A) and intracellular pools (Figure 1D) after Hg (II) exposure was observed, as depicted by the tighter clustering of metabolites in the Hg treated cultures. In the released metabolites, PLS-DA component 1 explained 42.9% of the total variance and where the positive component 1 was influenced by changes in sulfur containing amino acids like glutathione, cysteine, and methionine along with trisaccharides in the extracellular space (Figure 1B). The distinct shift in the metabolite pool released into the extracellular space after E. gracilis exposure to Hg (II) (Figure 1A) was mainly due to the increased abundance of sulfur containing amino acids and peptides (Figures 1B,C). When examining the top 15 most significantly changed metabolites based on VIP scores, the relative abundance of glutathione and trisaccharides changed by over 3-fold whereas glycine, serine, secondary amines, and cysteine increased 2–2.5-fold after Hg (II) treatment (p < 0.05; Figure 1C). The production of other amino acids like lysine and secondary amines were higher in control cultures, suggesting that Hg (II) exposure may inhibit the production and release of certain amino acids and peptides into extracellular space.
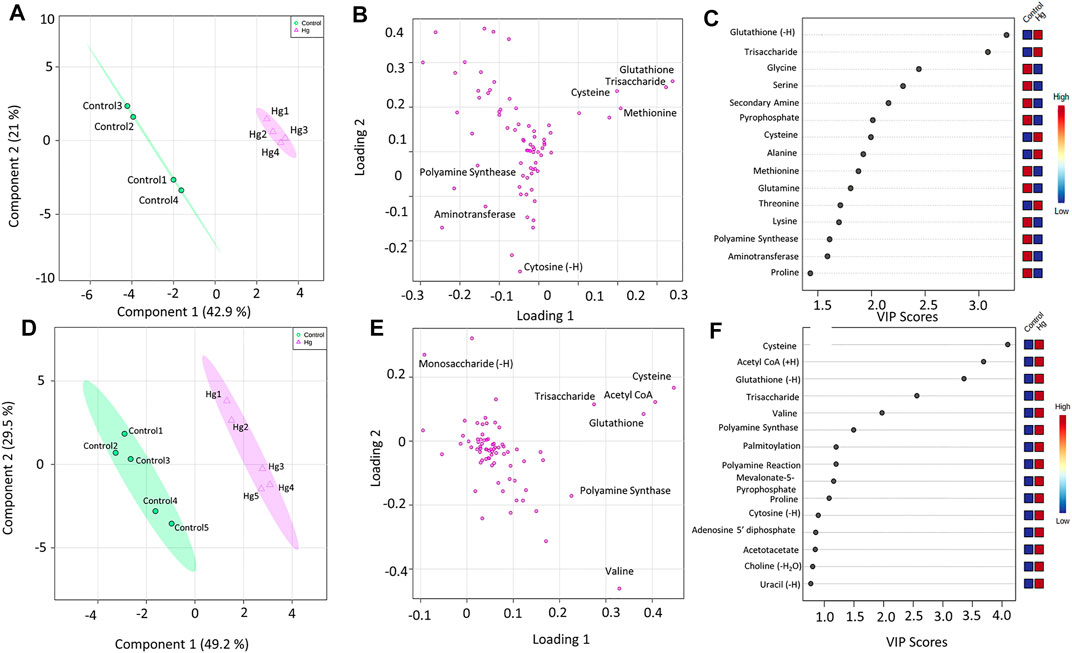
FIGURE 1. The effect of Hg (II) exposure on amino acids, metabolite classes, and metabolic processes E.gracilis in released exudates (A–C) and cellular biomolecules (D–F). A partial least squares discriminant analysis (PLS-DA) (A, D) reveals how an overall refinement of the metabolite pool in control (n=4) cultures change after Hg treatment (n=5) based on the relative abundance of 75 different metabolites (A, D). Individual loading plots (B, E) show key differentiating metabolites like glutathione, cysteine, and trisaccharides driving PLS-DA grouping in control and Hg treated cultures in released exudates (B) and cellular biomolecules (E). Variable importance in projection (VIP) plots (C, F) highlight the top 15 metabolites and metabolic processes with most significant (p < 0.05) fold changes in released exudates (C) and cellular biomolecules (F). Here, blue denotes a significant fold decrease in metabolite abundance while red indicates a significant fold change increase.
Within cells, the metabolite pool shifted along the positive component 1 and explained 49.2% of the total variance, with variance along the vertical component 2 explaining 29.5% of the variation between replicates (Figure 1D). The overall narrowing of the intracellular metabolite pool in Hg (II) treated cultures was much less pronounced than in extracellular fraction, but also shifted along the positive component 1 axis in a manner similar to exudate metabolites (Figures 1A,D). This shift was mainly driven by a 4-fold increase in cysteine followed by significant (p < 0.05) changes in acetyl CoA, glutathione, and trisaccharide transformations ranging from 2.5–3.6-fold changes in Hg (II) treated cultures (Figures 1E,F). Other metabolic enzymes and processes like polyamine synthase and polyamine reactions increased by 1.5-fold, highlighting the overall increase in amino acid and peptide production because of Hg (II) exposure.
Within cells, a wider breadth of metabolites was affected by Hg treatment than in the metabolites released by E. gracilis, but there were key similarities with respect to sulfur containing peptides. The abundance of sulfur containing metabolites significantly increased both within cells and in extracellular space after Hg exposure (Figures 1C,F). Glutathione abundance increased 3-fold in the released biomolecules where cysteine amino acids increased 4-fold within cellular biomolecules, in line with previous studies noting increased sulfur production in microalgae after Hg exposure (Slaveykova et al., 2021). Similarly, the production of trisaccharide like molecules were 3-fold and 2.6-fold higher in Hg treated cultures across the released biomolecule and intracellular space, respectively.
Euglena Gracilis RNA-Sequencing and de Novo Transcriptome Assembly
Illumina RNA-sequencing of control (n = 6) and Hg treated (n = 6) E. gracilis cultures resulted in an average of 24.5 million raw paired-end reads per library (range: 22.3–26.7 million) and an average of 22.7 million trimmed paired-end reads per library (range: 20.5–22.7 million). A combined 272.8 million trimmed paired-end reads were used to create a de novo transfrag assembly representing expressed E. gracilis transcripts and that assembly contained 439,129 genes and 992,204 transfrags (contig N50 = 883). BUSCO analysis showed 99.0% of the eukaryota OrthoDB v9 orthologs were present in our E. gracilis de novo transfrag assembly. An average of 17.6 million paired-end reads per library (range: 15.8–19.1 million) aligned to the de novo transfrag assembly (Supplementary Table S2; Supplementary Figure S4).
Comparative Transcriptome Analysis of Euglena gracilis Grown in the Absence or Presence of Mercury
Clustering analyses showed a clear distinction in the transcriptomes between the control and Hg treatment (Supplementary Figures S5 A,B,D–F). A total of 3,745 differentially expressed genes using DESeq2283 and 21462 up-regulated in the control- and Hg-treatment, respectively) and 1790 differentially expressed genes using edgeR (938 and 852 up-regulated in the control- and Hg-treatment, respectively) were found (Supplementary Figure S5). In total, 1,413 genes were identified by both methods (686 and 727 up-regulated in the control- and Hg-treatment, respectively). Using blastx to characterize the genes identified in both DGE analyses, the translated transfrags for 899/1,413 genes showed sequence similarity to a protein in the NCBI non-redundant or SWISS-PROT protein databases. Differentially expressed genes related to amide biosynthetic pathways, peptide biosynthetic pathways, and photosynthesis light harvesting pathways were found (Supplementary Table S3).
We identified a total of 604 E. gracilis genes, that when translated, had sequence similarity (herein referred to as a ‘hit’) to 490 A. thaliana non-redundant proteins, 623 E. gracilis gene hits to 425 C. reinhardtii non-redundant proteins, 227 E. gracilis gene hits to 132 Synechocystis non-redundant proteins, and 516 E. gracilis gene hits to 132 H. sapiens non-redundant proteins (Supplementary Table S3). In total, 146 enriched GO biologically processes were identified where p < 0.001 using H. sapiens databases that were used in subsequent transcriptomic and integrated analyses. The resulting A. thaliana, C. reinhardtii, Synechocystis, and H. sapiens proteins were used to query the PANTHER database for PANTHER GO-enrichment (Supplementary Table S4). Notably, GO-term enrichment was observed for proteins related to amide and peptide synthesis, photosynthesis, and both purine synthesis and metabolism in at least two databases (Table 1).
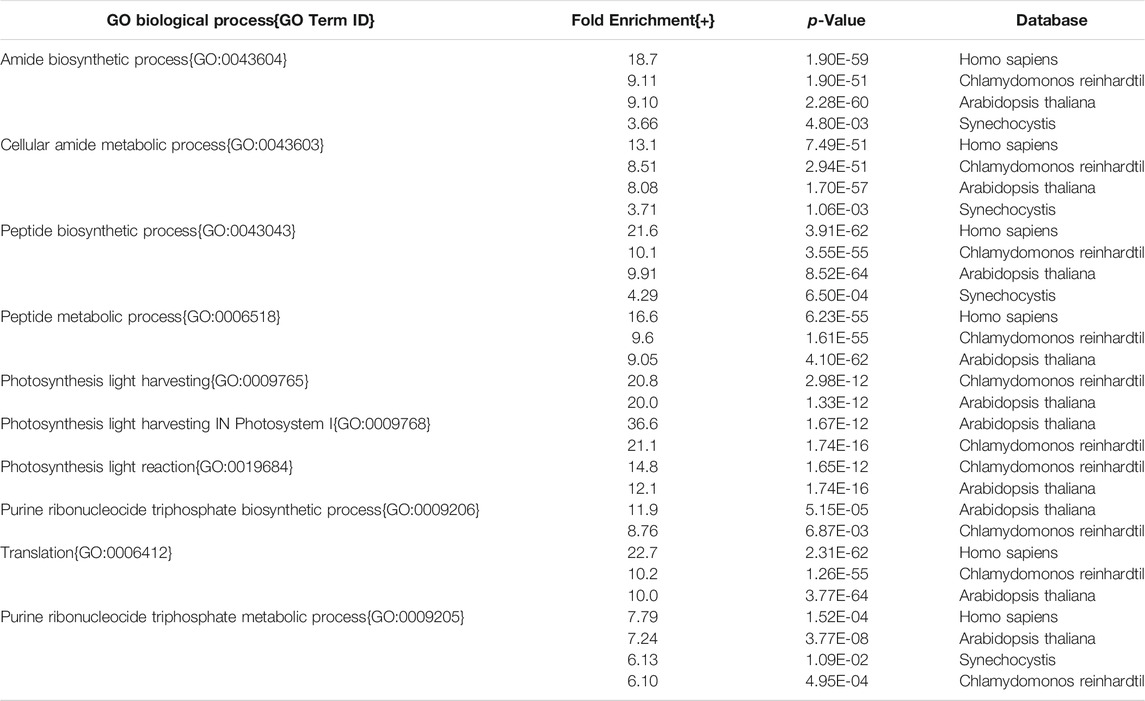
TABLE 1. The top-10 significantly upregulated GO biological processes detected in E. gracilis after Hg exposure. Positive fold enrichments and complementary p-values based on reference databases like Homo Sapies, a green algae Chlamydomonas reinhardtii, a flowering plant Arabidopsis thaliana and freshwater cyanobacteria Synechocystis. In total, 147 processes were identified as being significantly different after Hg (II) treatment.
Integrated Analysis of Transcripts and Metabolites
An integrated analysis of differentially expressed transcripts and enriched metabolites produced by E. gracilis after Hg exposure was conducted to provide a more comprehensive assessment of integrated pathways involved with Hg detoxification. Differentially expressed transcripts representing 516 orthologs to Homo sapiens proteins (identified by blastx) and 27 metabolites enriched by a fold change of 0.5 within E. gracilis cells that were identified by PLS-DA were used in IMPaLA (Figure 2A). Ten pathways were significantly enriched where Pjoint between transcriptomics and metabolomics data were <0.001, including: metabolism of amino acids, cellular responses to chemical stress, detoxification of reactive oxygen species, translation, metabolism, TP53 metabolic gene regulation and DNA repair (Figure 2B). Enriched transcripts that related to specific integration pathways included genes coding for ribosomal subunits (RPL21, RPS10), cytochrome C oxidase subunits (COX5A, COX6B1, CYCS), peroxiredoxin (PRDX2), ATP synthase subunits (ATP5F1A), histone synthesis (H2AC20), and small ubiquitin-related modifiers (SUMO3 (Figure 2B). Enriched metabolites that were involved with pathways included choline, valine, uracil, pyrophosphate, thymine, and adenosine 5-diphosphate. Notably, glutathione was involved in multiple pathways, suggesting an important role in glutathione production and Hg detoxification in E. gracilis. By conducting integration analyses, we gained comprehensive insight into the differentially expressed transcripts and enriched metabolites involved in microbial responses to Hg exposure.
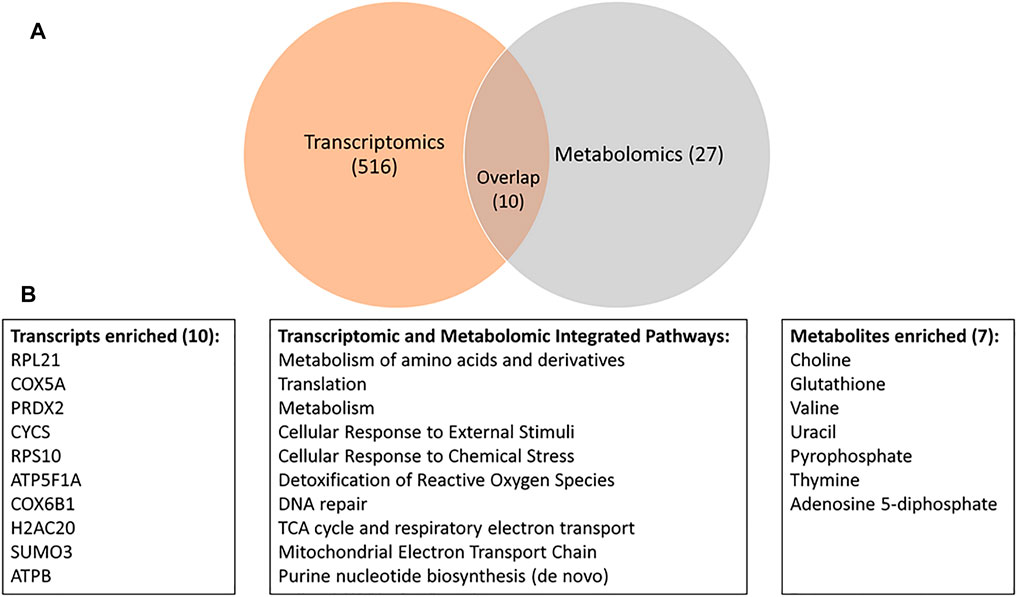
FIGURE 2. (A) Venn diagram highlighting the integration of 517 upregulated transcripts after Hg exposure within cells and 27 metabolites that increased in abundance within cells after Hg exposure. (B) A total of 10 enriched pathways were identified after incorporating both gene and metabolite data where Pjoint<0.001.
Discussion
Enriched Metabolism, Translation and Production of Amino Acids
A significant enrichment of transfrags, metabolites, and pathways corresponding to metabolism and amino acid synthesis was observed within E. gracilis cells after nonlethal exposure to Hg, suggesting that the production of amino acids aids E. gracilis in Hg tolerance. Transcriptomic analyses identified a total of 147 enriched processes (Supplementary Table S4), but only the top enriched GO biological processes with a significant (p < 0.001) across microbe and human databases are reported here. Genes involved in amide biosynthesis and cellular amide metabolism were significantly enriched (Table 1), along with those involved in the production of amino acids (e.g., cysteine, glycine, serine, and valine) both inside and outside cells (Figures 1C,F). The integration of transcriptomic and metabolomics data revealed that the metabolism of amino acids and derivatives, translation, and overall metabolism, were enriched in E. gracilis cells after Hg exposure (Figures 2, 3).
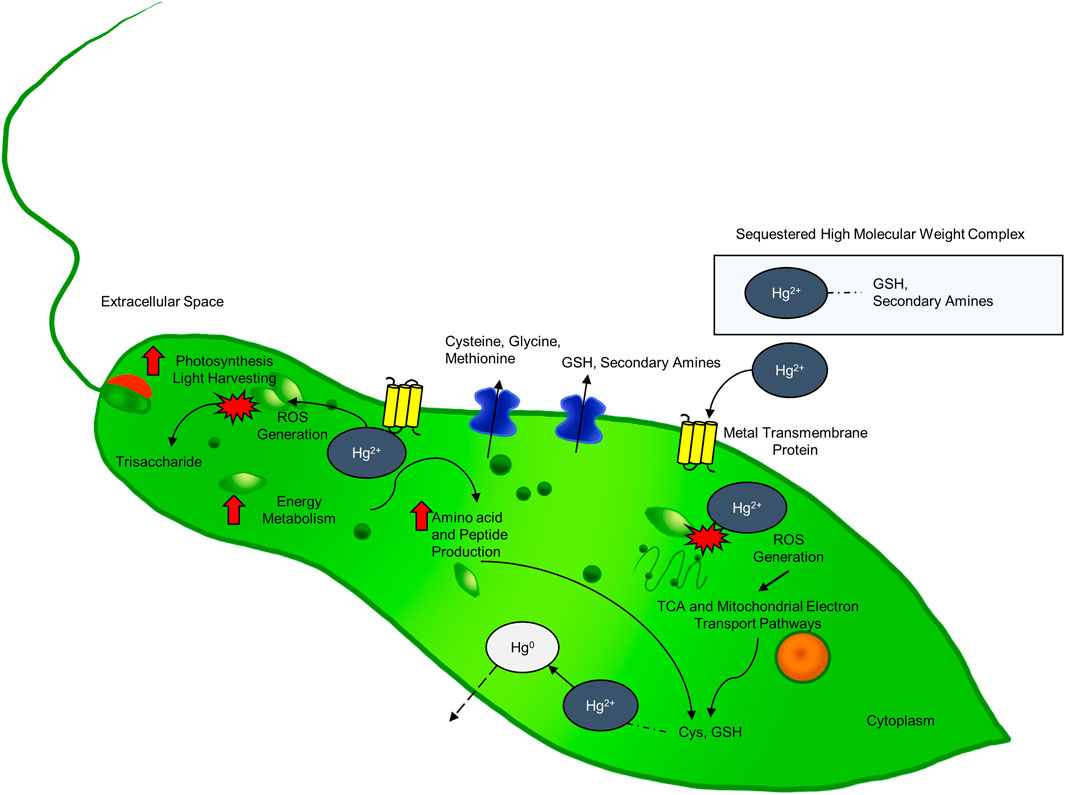
FIGURE 3. Conceptual model of the transcriptomic and metabolomic responses of E. gracilis to Hg (II) stress. After Hg (II) accumulation, ROS generation (red star) in chloroplasts and nucleus (orange circle) may occur as evidence by increased proportions of glutathione (GSH) and cysteine (Cys). Three main transcriptomic processes are significantly affected including 1) amide and peptide biosynthetic processes, 2) photosynthesis light harvesting processes, and 3) increased cellular metabolism and ATP production (red arrows). As a result, ribosomes (green circles) may assemble protein translation to increase intracellular and extracellular Cys and GSH concentrations to reduce Hg (II) to volatile Hg0 in extracellular space or sequester cellular Hg (II). Increased photosynthetic activity can account for the increase in ATP production, trisaccharide production observed to aid E. gracilis with energy for biosynthetic production and larger more condensed molecules for the sequestration of Hg (II).
The increase in amino acid metabolism could be the result of multiple processes, all linked to an increase in energy demand as a product of Hg stress. For example, the increase in amino acids can be the result of limited nitrogen availability during stress, as amino acid and polypeptide synthesis tends to increase to assist with antioxidant defence mechanisms in microorganisms (Sharma & Dietz, 2006), and plants specifically after Hg exposure (Wang et al., 2017). In the case of microalgae like Chlorella vulgaris, total amino acid content like proline, histidine, and glutamine were increased after stressors like cadmium and N-limitation (Chia et al., 2015).
An increase in amino acid synthesis within and outside E. gracilis after Hg exposure can be linked to pathways involved in ribosomal protein synthesis. Significant fold-changes in amino acids like cysteine, glutamine, proline, and serine; all amino acids were enriched after Hg treatment, especially in extracellular fractions (Figures 1C,F). The increase in transcripts corresponding to ribosomal proteins like RPL21 and RPS10 further suggests a shift towards increased protein synthesis, which could assist E. gracilis with a variety of processes like metal detoxification, mitigation of reactive oxygen species, and repairing cellular damage (He et al., 2021). Many of the intermediates in metabolic processes are involved in reactions that generate amino acids and other metabolites that are linked to both detoxification and biosynthesis processes (Ishikawa et al., 2017).
Despite the overall enhancement of metabolism and amino acid synthesis, there remains less diversity in the pool of metabolites produced by E. gracilis after Hg exposure (Figures 1A,D). The tighter clustering of detected metabolites after Hg exposure suggests that the overall diversity of metabolites decreased, but that the production of specific amino acids and peptides increased (Figures 1C,F). Similar patterns have been well documented in algae, where environmental stress factors like salinity can increase the production of nitrogen and carbon, increasing amino acid production and release to extracellular space (Jimenez and Niell, 1991). Photo exposure can also increase the production of essential amino acids like methionine, threonine, and tryptophan in algae (Gouveia, 2014), affecting the metabolites involved in the binding of Hg (II) in extracellular space (Mangal et al., 2019). In other extremophile microalgae, heavy metal stress induced the enrichment of glutamine, asparagine, cystine, and serine metabolites (Puente-Sanchez et al., 2018), largely consistent with enriched metabolites in E. gracilis found in this study (Figures 1C,F), except for asparagine. Overall, the refinement in specialized metabolites after Hg(II) exposure highlights key metabolic perturbations after Hg stress that have subsequent roles specifically pertaining to E. gracilis amino acid metabolism.
Reactive Oxygen Species and Cellular Responses to Chemical Stress
The significant enrichment of glutathione (GSH) both within and outside of cells after Hg(II) treatment (Figures 1C,F) suggests an important involvement of GSH in regulating Hg stress. GSH has an important role in redox cycling within cells, serving as one of the main defences against metal induced oxidative stress (Cobbett and Goldsbrough, 2002; Watanabe and Suzuki, 2002; Pinto et al., 2003; Sharma and Dietz, 2006; Elbaz et al., 2010). Reactive oxygen species (ROS) are generated as by-products of chloroplast and mitochondrial metabolism, but metal stress can overstimulate ROS production that results in significant cellular damage (Elbaz et al., 2010). The generation of free radicals and hydrogen peroxide poses a significant threat to aerobic organisms by oxidizing proteins, lipids, and nucleic acids resulting in mutagenesis (Pinto et al., 2003).
GSH and other sulfur containing metabolites like cysteine and methionine that were enriched in E. gracilis (Figures 1C,F), are important chelators and scavengers for toxic metals like cadmium (Branco et al., 2010), and Hg (Elbaz et al., 2010; Slaveykova et al., 2021). Sulfur containing metabolites, like reduced thiol species, form thermodynamically stable bonds with Hg (II) (Benoit et al., 2001; Mah and Jalilahvand, 2008) and are involved with the sequestration and detoxification of Hg in plants and green algae (D’Alessandro et al., 2013; Khatiwada et al., 2020 a,b). In other microorganisms, the presence of cysteine in exposure media, increased Hg (II) uptake (Le Faucheur et al., 2014). The increased production of both intracellular and extracellular GSH, coupled to enriched cysteine and methionine in the extracellular space, further suggests a scavenging and chelation function of released sulfur bearing metabolites observed after sublethal Hg exposure (Figures 1C,F). This suggests that E. gracilis may release sulfur bearing metabolites into extracellular space to bind to and reduce free Hg (II) species from entering intracellular space thereby minimizing cellular damage of uncomplexed Hg (II).
Transcriptomic and metabolomic integrated pathway analyses also revealed the involvement of GSH and adenosine 5-diphosphate molecules in significantly enriched pathways linked to cellular responses to stress (Figure 2B). For example, pathways including cellular response to chemical stress, detoxification of ROS, cellular response to external stimuli, and DNA repair were significantly enriched pathways that could explain the increased production of glutathione and adenosine 5-diphosphate molecules after Hg exposure. Genes coding for peroxiredoxin (PRDX2) were present in both cellular response to stress and detoxification of ROS pathways. Peroxiredoxin is a thiol-specific peroxidase that reduces peroxides and other by-products generated during Hg(II) induced oxidative stress (Wakao and Niyogi, 2021). The generation of ROS often occurs in electron transport chains present in chloroplasts and mitochondria, and similar peroxiredoxin isoforms have been identified in both chloroplasts and mitochondria of E. gracilis that minimize cellular damage caused by ROS (Ishikawa et al., 2017). The incorporation of metabolites like GSH and adenosine 5-diphosphate coupled to genes coding for peroxiredoxin suggests that oxidative stress induced by Hg(II) exposure to E. gracilis leads to enriched activity of peroxiredoxin related pathways.
Genes coding for cytochrome C oxidase (COX5A, COX6B1) were also present in both cellular responses to stress and detoxification of ROS pathways. Increased production of uracil, thymine, adenosine 5-diphosphate, and pyrophosphate molecules in Hg(II) treated cells can also be linked to their role in DNA repair pathways (Figure 2B). Cytochrome c plays a role in apoptosis, halting the cascading effects of cells affected by ROS damage (Darehshouri et al., 2008). Genes H2AC20 and SUMO3 correspond to histone clusters and small ubiquitin modifiers, respectively, and both are involved in DNA replication and repair. The significant enrichment of transcripts and metabolites from these pathways lends to E. gracilis’ ability to tolerate metal stress via repairing DNA strands and signalling apoptosis in cells that have been subject to damaging agents like ROS.
Cellular Respiration and Electron Transport
A significant increase in GSH, adenosine-5 diphosphate, and acetyl CoA abundance, coupled to significantly enriched COX5A, CYCS, and ATPB transcripts within cells after Hg exposure, suggest the upregulation of integrated pathways like TCA cycle and mitochondrial electron transport chain (Figure 2B). The TCA cycle is responsible for a large portion of energy generation through the oxidation of acetyl CoA from stored metabolites. Upon stress, TCA activity increases resulting in lipid and carbohydrate catabolism to generate NAD(P)H needed to convert oxidized GSH to reduced GSH (Gansemer et al., 2020). TCA cycles and mitochondrial electron transport cycles are intrinsically connected during the generation of ATP and the reduction of GSH. For example, NAD(P)H produced during TCA cycles supply electrons that can be used for reducing oxidized GSH in the mitochondria (Nolfi-Donegan et al., 2020). The production of ATP through mitochondrial electron transport consequentially generates ROS, and both GSH and thioredoxin/peroxiredoxin systems actively regulate peroxide levels that leave the mitochondrion (Nolfi-Donegan et al., 2020; Wakao and Niyogi., 2021). Increased metabolism and ATP generation may be a possible mechanisms for recycling oxidized GSH that has already participated in mitigating ROS stress or generating new reduced GSH to sequester Hg(II). The low abundance of fatty acids in Hg treated cells observed in this study is contradictory to other studies where microalgae were exposed to cadmium (Puente-Sánchez et al., 2018), but consistent with fatty acid degradation in E. gracilis after cadmium treatment (He et al., 2021). Essential amino acids also serve as precursors for the generation of ATP through the TCA cycle (Martínez-Reyes & Chandel, 2020), which offers another possible explanation for increased amide and peptide metabolic and biosynthetic processes observed (Table 1). In the microalgae Chlamydomonas reinhardtii, metabolites involved in amino acid, fatty acid, and TCA cycles, increased after Hg(II) and methylmercury (MeHg) exposure (Slaveykova et al., 2021). These observations are consistent with the highly selected amino acid and peptide biosynthetic processes (Table 1) and integrated transcriptomic-metabolomic metabolism, amino acid generation and translation pathways observed in this study (Figure 2). The increase in energy demand by E. gracilis for amino acid and peptide production for mitigating ROS generation and Hg sequestration may be achieved through catabolism of stored fatty acids through TCA cycles, or the production of amino acids for energy production (Figure 3).
Significantly enriched de novo purine nucleotide biosynthesis pathways were linked to ATPB transcripts and a 1.0–1.5-fold higher abundance of pyrophosphate and adenosine 5-diphosphate in Hg treated E. gracilis cells (Figures 1F, 2B). Purine biosynthesis involves synthesizing energy rich nitrogenous heterocyclic bases found in both DNA and RNA but is also a necessary precursor for the cofactor NAD (Moffat and Ashihara, 2002). The combined upregulation of amino acids and metabolism pathways in Hg treated cells is in line with greater purine production, as amino acids are the precursors to many metabolites and nucleotides (Vallon and Spalding, 2009). Similarly to the TCA cycle, enrichment of de novo nucleotide biosynthesis pathways leading to the production of NAD(P)H can increase the ratio of reduced to oxidized GSH, and in turn, increase GSH abundance for Hg(II) binding and ROS mitigation (Gansemer et al., 2020).
Although no statistical relationship was found integrating metabolites or transcripts for photosynthesis cycles, light harvested photosynthesis processes were significantly upregulated at the transcript level (Table 1), and acetyl CoA abundance was enriched by 3.5-fold within E. gracilis cells after Hg exposure (Figure 1F). This observation may be largely explained by the fact that IMAPaLA pathway integration analysis works primarily with human databases. Nevertheless, upregulation of photosynthesis processes and its tight coupling to electron transport and ATP production, makes light harvesting systems sensitive regions for ROS generation. Specifically, photosystem I is an important site for cyclic electron flow, managing the proton flow for ATP synthesis through the Calvin cycle (Deng et al., 2013; Beauvais-Flück et al., 2017), and has been shown to generate amounts of ROS. In Euglena, a reduction in chlorophyll content, ATP formation, and additional photosynthetic processes have been linked to sub-lethal metal stress (Suresh Kumar et al., 2014). Enhanced photosynthetic activity was observed in Euglena sp., Chlorella sp., and C. reinhardtii when exposed to zinc and Hg (II) (Beauvais-Flück et al., 2017), likely due increased energy demands of oxidative stress-based responses within photosystems (Slaveykova et al., 2021). Despite our lack of identifying integrated metabolomic and transcriptomic pathways, we provide evidence for the transcriptomic enrichment of photosynthetic activity as a possible additional energy resource to synthesize cofactors needed to help mitigate Hg(II) stress.
Conclusion and Future Directions
This study presents the integration of transcriptomics and metabolomics to gain comprehensive information regarding the cellular responses of E. gracilis to sublethal concentrations of Hg(II) exposure. Our results show integrated transcriptomic and metabolomic pathways involving the enrichment of amino acid metabolism and synthesis, cellular responses to external stress, detoxification of reactive oxygen species, and DNA repair, linked to significant production of GSH within and outside cells. We also show significant enrichment of the TCA cycle, mitochondrial electron transport, and purine synthesis supported by the enhancement of adenosine 5-diphosphate and pyrophosphate compounds to meet the energy demands of Hg induced oxidative stress.
It is important to note that the surrounding environment of microbial cultures is highly dynamic even in controlled laboratory settings. Linking metabolomic and transcriptomic data is subject to variation resulting from post translational modification events that were not directly investigated in the presented study. As such, the transcript data presented here reflects a blueprint for insight into potential protein expression, and direct linkage between these omic resources is restricted to genes with limited post translational modifications during expression. Despite the intrinsic variation in metabolite composition and transcriptome comparisons, a high degree of similarity between transcriptomic and metabolomic changes in E. gracilis after Hg (II) exposure was observed and we provide evidence of pathways involved in the Hg(II) resistance by E. gracilis.
While this study provides insight into the different transcriptomic and metabolomic changes in E. gracilis, future studies should consider including a wider range of Hg exposure concentrations and treatments to help assess whether specific pathways are disproportionally affected by environmentally relevant Hg levels. Furthermore, the presented study focused on identifying pathways that contribute to the production of compounds that may bind to Hg and future studies will focus on identifying specific transcripts that could act as bioindicators of Hg contamination in aquatic environments and confirming the changes in their levels using reverse transcription qPCR. While our study focuses on Hg concentrations similar to mine tailings, other recent studies that explore the metabolomics of Hg and methylmercury accumulation in green algae at environmentally relevant concentrations are in line with the higher Hg (II) concentrations used in this study to reflect exposures in more extreme conditions such as mine tailing ponds. Notably both types of studies indicate metabolites involved with nucleotide synthesis, antioxidant, and photorespiration are upregulated in microalgae (Slaveykova et al., 2021). An important caveat to consider is that this study used FT-ICR-MS for the untargeted and putative identification of possible metabolite isomers. While this approach provides compositional level characteristics with high accuracy, more targeted approaches like MS/MS and nuclear magnetic resonance (NMR) in conjunction with other liquid chromatography techniques should be considered in the future to gain more structural information for the metabolites identified in this study. Using an integrated omics-based approach, our results provided novel insights into the systemic responses of E. gracilis to Hg (II). Understanding the adaptive responses of E. gracilis to metals can help refine bioremediation technologies by harnessing biogenic molecules for metal sequestration. Moreover, the combination of metabolomics and transcriptomics can serve as an early warning tool for Hg toxicity before physiological changes occur in larger biota (Slaveykova et al., 2021). As anthropogenic activities continue to increase Hg concentrations in aquatic systems, these findings help identify the specific cellular responses and mechanisms responsible for Hg tolerance that other phototrophic plankton may utilize to cope with environmental stress.
Data Availability Statement
Raw sequence data obtained from high-throughput sequencing are available from the NCBI Sequence Read Archive (https://www.ncbi.nlm.nih.gov/bioproject/656442; accession number PRJNA656442).
Author Contributions
VM, MD, AL, BS, and CG designed research; VM, MD, and AL performed research and equally contributed to data analysis. VM, MD, AL, BS, and CG. CG and BS wrote the paper.
Funding
This work was supported by the Natural Sciences and Engineering Research Council of Canada (VM; CG; BS) and the Canada Foundation for Innovation (CG).
Conflict of Interest
The authors declare that the research was conducted in the absence of any commercial or financial relationships that could be construed as a potential conflict of interest.
Publisher’s Note
All claims expressed in this article are solely those of the authors and do not necessarily represent those of their affiliated organizations, or those of the publisher, the editors and the reviewers. Any product that may be evaluated in this article, or claim that may be made by its manufacturer, is not guaranteed or endorsed by the publisher.
Acknowledgments
We would like to thank Anna Kisiała and Professor RJ Neil Emery for their assistance with cell preparation and NOBLEGEN Inc. for their use of their centrifuges and cell counter. The authors would like to thank the two reviewers for their feedback to improve the quality of this manuscript.
Supplementary Material
The Supplementary Material for this article can be found online at: https://www.frontiersin.org/articles/10.3389/fenvs.2022.836732/full#supplementary-material
References
Aken, B. L., Achuthan, P., Akanni, W., Amode, M. R., Bernsdorff, F., Bhai, J., et al. (2017). Ensembl 2017. Nucleic Acids Res. 45, D635–D642. doi:10.1093/nar/gkw1104
Ali, M. B., Vajpayee, P., Tripathi, R. D., Rai, U. N., Kumar, A., Singh, N., et al. (2000). Mercury Bioaccumulation Induces Oxidative Stress and Toxicity to Submerged Macrophyte Potamogeton crispus L. Bull. Environ. Contam. Toxicol. 65, 573–582.
Amos, H. M., Jacob, D. J., Streets, D. G., and Sunderland, E. M. (2013). Legacy Impacts of All-Time Anthropogenic Emissions on the Global Mercury Cycle. Glob. Biogeochem. Cycles 27, 410–421. doi:10.1002/gbc.20040
Andrews, S. (2010). FastQC: A Quality Control Tool for High Throughput Sequence Data. Available at: http://www.bioinformatics.babraham.ac.uk/projects/fastqc.
Aviles, C. s., Loza-Tavera, H., Terry, N., and Moreno-Senchez, R. (2003). Mercury Pretreatment Selects an Enhanced Cadmium-Accumulating Phenotype in Euglena Gracilis. Arch. Microbiol. 180, 1–10. doi:10.1007/s00203-003-0547-2
Beauvais-Flück, R., Slaveykova, V. I., and Cosio, C. (2017). Cellular Toxicity Pathways of Inorganic and Methyl Mercury in the green Microalga Chlamydomonas Reinhardtii. Sci. Rep. 7, 8034. doi:10.1038/s41598-017-08515-8
Benoit, J. M., Mason, R. P., Gilmour, C. C., and Aiken, G. R. (2001). Constants for Mercury Binding by Dissolved Organic Matter Isolates from the Florida Everglades. Geochimica et Cosmochimica Acta 65, 4445–4451. doi:10.1016/S0016-7037(01)00742-6
Bolger, A. M., Lohse, M., and Usadel, B. (2014). Trimmomatic: a Flexible Trimmer for Illumina Sequence Data. Bioinformatics 30, 2114–2120. doi:10.1093/bioinformatics/btu170
Branco, D., Lima, A., Almeida, S. F. P., and Figueira, E. (2010). Sensitivity of Biochemical Markers to Evaluate Cadmium Stress in the Freshwater Diatom Nitzschia Palea (Kützing) W. Smith. Aquat. Toxicol. 99, 109–117. doi:10.1016/j.aquatox.2010.04.010
Burgess, K. E. V., Borutzki, Y., Rankin, N., Daly, R., and Jourdan, F. (2017). MetaNetter 2: A Cytoscape Plugin for Ab Initio Network Analysis and Metabolite Feature Classification. J. Chromatogr. B 1071, 68–74. doi:10.1016/j.jchromb.2017.08.015
Cavill, R., Jennen, D., Kleinjans, J., and Briedé, J. J. (2016). Transcriptomic and Metabolomic Data Integration. Brief Bioinform 17, 891–901. doi:10.1093/bib/bbv090
Cheng, J., Qiu, H., Chang, Z., Jiang, Z., and Yin, W. (2016). The Effect of Cadmium on the Growth and Antioxidant Response for Freshwater Algae Chlorella Vulgaris. SpringerPlus 5, 1290. doi:10.1186/s40064-016-2963-1
Chételat, J., and Amyot, M. (2009). Elevated Methylmercury in High ArcticDaphniaand the Role of Productivity in Controlling Their Distribution. Glob. Change Biol. 15, 706–718. doi:10.1111/j.1365-2486.2008.01729.x
Chia, M. A., Lombardi, A. T., da Graça Gama Melão, M., and Parrish, C. C. (2015). Combined Nitrogen Limitation and Cadmium Stress Stimulate Total Carbohydrates, Lipids, Protein and Amino Acid Accumulation in Chlorella Vulgaris (Trebouxiophyceae). Aquat. Toxicol. 160, 87–95. doi:10.1016/j.aquatox.2015.01.002
Chong, J., and Xia, J. (2018). MetaboAnalystR: an R Package for Flexible and Reproducible Analysis of Metabolomics Data. Bioinformatics 34, 4313–4314. doi:10.1093/bioinformatics/bty528
Chong, J., Yamamoto, M., and Xia, J. (2019). MetaboAnalystR 2.0: From Raw Spectra to Biological Insights. Metabolites 9, 57. doi:10.3390/metabo9030057
Clemens, S. (2006). Toxic Metal Accumulation, Responses to Exposure and Mechanisms of Tolerance in Plants. Biochimie 88, 1707–1719. doi:10.1016/j.biochi.2006.07.003
Cobbett, C., and Goldsbrough, P. (2002). Phytochelatins and Metallothioneins: Roles in Heavy Metal Detoxification and Homeostasis. Annu. Rev. Plant Biol. 53, 159–182. doi:10.1146/annurev.arplant.53.100301.135154
D’Alessandro, A., Taamalli, M., Gevi, F., Timperio, A. M., Zolla, L., and Ghnaya, T. (2013). Cadmium Stress Responses in Brassica Juncea: Hints from Proteomics and Metabolomics. J. Proteome Res. 12, 4979–4997. doi:10.1021/pr400793e
Darehshouri, A., Affenzeller, M., and Lütz-Meindl, U. (2008). Cell Death upon H2O2induction in the Unicellular green algaMicrasterias. Plant Biol. 10, 732–745. doi:10.1111/j.1438-8677.2008.00078.x
Deng, C., Zhang, D., Pan, X., Chang, F., and Wang, S. (2013). Toxic Effects of Mercury on PSI and PSII Activities, Membrane Potential and Transthylakoid Proton Gradient in Microsorium pteropus. J. Photochem. Photobiol. B: Biol. 127, 1–7. doi:10.1016/j.jphotobiol.2013.07.012
Devars, S., Hernández, R., and Moreno-Sánchez, R. (1998). Enhanced Heavy Metal Tolerance in Two Strains of Photosynthetic Euglena Gracilis by Preexposure to Mercury or Cadmium. Arch. Environ. Contam. Toxicol. 34, 128–135. doi:10.1007/s002449900296
Driscoll, C. T., Han, Y.-J., Chen, C. Y., Evers, D. C., Lambert, K. F., Holsen, T. M., et al. (2007). Mercury Contamination in Forest and Freshwater Ecosystems in the Northeastern United States. BioScience 57, 17–28. doi:10.1641/B570106
Ebenezer, T. E., Zoltner, M., Burrell, A., Nenarokova, A., Novák Vanclová, A. M. G., Prasad, B., et al. (2019). Transcriptome, Proteome and Draft Genome of Euglena Gracilis. BMC Biol. 17, 11. doi:10.1186/s12915-019-0626-8
Elbaz, A., Wei, Y. Y., Meng, Q., Zheng, Q., and Yang, Z. M. (2010). Mercury-induced Oxidative Stress and Impact on Antioxidant Enzymes in Chlamydomonas Reinhardtii. Ecotoxicology 19, 1285–1293. doi:10.1007/s10646-010-0514-z
Field, K. A., Sewall, B. J., Prokkola, J. M., Turner, G. G., Gagnon, M. F., Lilley, T. M., et al. (2018). Effect of Torpor on Host Transcriptomic Responses to a Fungal Pathogen in Hibernating Bats. Mol. Ecol. 27, 3727–3743. doi:10.1111/mec.14827
Gansemer, E. R., McCommis, K. S., Martino, M., King-McAlpin, A. Q., Potthoff, M. J., Finck, B. N., et al. (2020). NADPH and Glutathione Redox Link TCA Cycle Activity to Endoplasmic Reticulum Homeostasis. iScience 23, 101116. doi:10.1016/j.isci.2020.101116
Gissibl, A., Sun, A., Care, A., Nevalainen, H., and Sunna, A. (2019). Bioproducts from Euglena Gracilis: Synthesis and Applications. Front. Bioeng. Biotechnol. 7, 108. doi:10.3389/fbioe.2019.00108
Gouveia, L. (2014). From Tiny Microalgae to Huge Biorefineries. Oceanography 02. doi:10.4172/2332-2632.1000120
Grabherr, M. G., Haas, B. J., Yassour, M., Levin, J. Z., Thompson, D. A., Amit, I., et al. (2011). Full-length Transcriptome Assembly from RNA-Seq Data without a Reference Genome. Nat. Biotechnol. 29, 644–652. doi:10.1038/nbt.1883
Gustafsson, J. P. (2010). Visual MINTEQ Version 3.10.Visual MINTEQ – Visual MINTEQ – a Free Equilibrium Speciation Model. Stockholm, Sweden: kth.se.
He, J., Liu, C., Du, M., Zhou, X., Hu, Z., Lei, A., et al. (2021). Metabolic Responses of a Model Green Microalga Euglena Gracilis to Different Environmental Stresses. Front. Bioeng. Biotechnol. 9, 662655. doi:10.3389/fbioe.2021.662655
Hughes, S. L., Bundy, J. G., Want, E. J., Kille, P., and Stürzenbaum, S. R. (2009). The Metabolomic Responses of Caenorhabditis elegans to Cadmium Are Largely Independent of Metallothionein Status, but Dominated by Changes in Cystathionine and Phytochelatins. J. Proteome Res. 8, 3512–3519. doi:10.1021/pr9001806
Inwongwan, S., Kruger, N. J., Ratcliffe, R. G., and O’Neill, E. C. (2019). Euglena Central Metabolic Pathways and Their Subcellular Locations. Metabolites 9, 115. doi:10.3390/metabo9060115
Ishikawa, T., Tamaki, S., Maruta, T., and Shigeoka, S. (2017). “Biochemistry and Physiology of Reactive Oxygen Species in Euglena,” in Euglena: Biochemistry, Cell and Molecular Biology. Editors S. D. Schwartzbach, and S. Shigeoka (Cham: Springer International Publishing), 47–64. doi:10.1007/978-3-319-54910-1_4
Jamers, A., Blust, R., De Coen, W., Griffin, J. L., and Jones, O. A. H. (2013). An Omics Based Assessment of Cadmium Toxicity in the green Alga Chlamydomonas Reinhardtii. Aquat. Toxicol. 126, 355–364. doi:10.1016/j.aquatox.2012.09.007
Jiménez, C., and Niell, F. X. (1991). Growth ofDunaliella Viridis Teodoresco: Effect of Salinity, Temperature and Nitrogen Concentration. J. Appl. Phycol. 3, 319–327. doi:10.1007/BF02392885
Kamburov, A., Cavill, R., Ebbels, T. M. D., Herwig, R., and Keun, H. C. (2011). Integrated Pathway-Level Analysis of Transcriptomics and Metabolomics Data with IMPaLA. Bioinformatics 27, 2917–2918. doi:10.1093/bioinformatics/btr499
Khatiwada, B., Hasan, M. T., Sun, A., Kamath, K. S., Mirzaei, M., Sunna, A., et al. (2020a). Probing the Role of the Chloroplasts in Heavy Metal Tolerance and Accumulation in Euglena Gracilis. Microorganisms 8, 115. doi:10.3390/microorganisms8010115
Khatiwada, B., Hasan, M. T., Sun, A., Kamath, K. S., Mirzaei, M., Sunna, A., et al. (2020b). Proteomic Response of Euglena Gracilis to Heavy Metal Exposure - Identification of Key Proteins Involved in Heavy Metal Tolerance and Accumulation. Algal Res. 45, 101764. doi:10.1016/j.algal.2019.101764
Langmead, B., and Salzberg, S. L. (2012). Fast Gapped-Read Alignment with Bowtie 2. Nat. Methods 9, 357–359. doi:10.1038/nmeth.1923
Le Faucheur, S., Campbell, P. G. C., Fortin, C., and Slaveykova, V. I. (2014). Interactions between Mercury and Phytoplankton: Speciation, Bioavailability, and Internal Handling. Environ. Toxicol. Chem. 33, 1211–1224. doi:10.1002/etc.2424
Lepak, R. F., Hoffman, J. C., Janssen, S. E., Krabbenhoft, D. P., Ogorek, J. M., DeWild, J. F., et al. (2019). Mercury Source Changes and Food Web Shifts Alter Contamination Signatures of Predatory Fish from Lake Michigan. Proc. Natl. Acad. Sci. USA 116, 23600–23608. doi:10.1073/pnas.1907484116
Li, B., and Dewey, C. N. (2011). RSEM: Accurate Transcript Quantification from RNA-Seq Data with or without a Reference Genome. BMC Bioinformatics 12, 323. doi:10.1186/1471-2105-12-323
Love, M. I., Huber, W., and Anders, S. (2014). Moderated Estimation of Fold Change and Dispersion for RNA-Seq Data with DESeq2. Genome Biol. 15, 550. doi:10.1186/s13059-014-0550-8
Mah, V., and Jalilehvand, F. (2008). Mercury(II) Complex Formation with Glutathione in Alkaline Aqueous Solution. J. Biol. Inorg. Chem. 13, 541–553. doi:10.1007/s00775-008-0342-2
Mangal, V., DeGasparro, S., Beresford, D. V., and Guéguen, C. (2020). Linking Molecular and Optical Properties of Dissolved Organic Matter across a Soil-Water Interface on Akimiski Island (Nunavut, Canada). Sci. Total Environ. 704, 135415. doi:10.1016/j.scitotenv.2019.135415
Mangal, V., Stenzler, B. R., Poulain, A. J., and Guéguen, C. (2019). Aerobic and Anaerobic Bacterial Mercury Uptake Is Driven by Algal Organic Matter Composition and Molecular Weight. Environ. Sci. Technol. 53, 157–165. doi:10.1021/acs.est.8b04909
Martinez-Reyes, I., and Chandel, N. S. (2020). Mitochondrial TCA Cycle Metabolites Control Physiology and Disease. Nature Commun. 11 (102), 1–11.
Mi, H., Muruganujan, A., Ebert, D., Huang, X., and Thomas, P. D. (2019). PANTHER Version 14: More Genomes, a New PANTHER GO-Slim and Improvements in Enrichment Analysis Tools. Nucleic Acids Res. 47, D419–D426. doi:10.1093/nar/gky1038
Moffatt, B. A., and Ashihara, H. (2002). “Pruine and Pyrimidine Nucleotide Synthesis and Metabolism,” in American Society of Plant Biologists, 2–20.
Nolfi-Donegan, D., Braganza, A., and Shiva, S. (2020). Mitochondrial Electron Transport Chain: Oxidative Phosphorylation, Oxidant Production, and Methods of Measurement. Redox Biol. 37, 101674. doi:10.1016/j.redox.2020.101674
Olaveson, M. M., and Nalewajko, C. (2000). Effects of Acidity on the Growth of Two Euglena Species. Hydrobiologia 433, 39–56. doi:10.1023/A:1004006401516
Pinto, E., Sigaud-kutner, T. C. S., Leitão, M. A. S., Okamoto, O. K., Morse, D., and Colepicolo, P. (2003). Heavy Metal-Induced Oxidative Stress in Algae1. J. Phycol. 39, 1008–1018. doi:10.1111/j.0022-3646.2003.02-193.x
Puente-Sánchez, F., Díaz, S., Penacho, V., Aguilera, A., and Olsson, S. (2018). Basis of Genetic Adaptation to Heavy Metal Stress in the Acidophilic green Alga Chlamydomonas Acidophila. Aquat. Toxicol. 200, 62–72. doi:10.1016/j.aquatox.2018.04.020
Ritter, A., Dittami, S. M., Goulitquer, S., Correa, J. A., Boyen, C., Potin, P., et al. (2014). Transcriptomic and Metabolomic Analysis of Copper Stress Acclimation in Ectocarpus Siliculosus Highlights Signaling and Tolerance Mechanisms in Brown Algae. BMC Plant Biol. 14, 116. doi:10.1186/1471-2229-14-116
Robinson, M. D., McCarthy, D. J., and Smyth, G. K. (2010). edgeR: a Bioconductor Package for Differential Expression Analysis of Digital Gene Expression Data. Bioinformatics 26, 139–140. doi:10.1093/bioinformatics/btp616
RodrÍguez-Zavala, J. S., GarcÍa-GarcÍa, J. D., Ortiz-Cruz, M. A., and Moreno-Sánchez, R. (2007). Molecular Mechanisms of Resistance to Heavy Metals in the protistEuglena Gracilis. J. Environ. Sci. Health A 42, 1365–1378. doi:10.1080/10934520701480326
Saiz-Lopez, A., Sitkiewicz, S. P., Roca-Sanjuán, D., Oliva-Enrich, J. M., Dávalos, J. Z., Notario, R., et al. (2018). Photoreduction of Gaseous Oxidized Mercury Changes Global Atmospheric Mercury Speciation, Transport and Deposition. Nat. Commun. 9, 4796. doi:10.1038/s41467-018-07075-3
Sambrook, J., and Russell, D. W. (2001). in Molecular Cloning: A Laboratory Manual. 3rd ed (N.Y: Cold Spring Harbor Laboratory Press).
Sharma, S. S., and Dietz, K.-J. (2006). The Significance of Amino Acids and Amino Acid-Derived Molecules in Plant Responses and Adaptation to Heavy Metal Stress. J. Exp. Bot. 57, 711–726. doi:10.1093/jxb/erj073
Slaveykova, V. I., Majumdar, S., Regier, N., Li, W., and Keller, A. A. (2021). Metabolomic Responses of Green Alga Chlamydomonas Reinhardtii Exposed to Sublethal Concentrations of Inorganic and Methylmercury. Environ. Sci. Technol. 55, 3876–3887. doi:10.1021/acs.est.0c08416
Suresh Kumar, K., Dahms, H.-U., Lee, J.-S., Kim, H. C., Lee, W. C., and Shin, K.-H. (2014). Algal Photosynthetic Responses to Toxic Metals and Herbicides Assessed by Chlorophyll a Fluorescence. Ecotoxicology Environ. Saf. 104, 51–71. doi:10.1016/j.ecoenv.2014.01.042
Szymańska, E., Saccenti, E., Smilde, A. K., and Westerhuis, J. A. (2012). Double-check: Validation of Diagnostic Statistics for PLS-DA Models in Metabolomics Studies. Metabolomics 8, 3–16. doi:10.1007/s11306-011-0330-3
Vallon, O., and Spalding, M. H. (2009). “Amino Acid Metabolism,” in The Chlamydomonas Sourcebook. Editors E. H. Harris, D. B. Stern, and G. B. Witman (London: Academic Press), 115–158. doi:10.1016/b978-0-12-370873-1.00012-5
Varet, H., Brillet-Guéguen, L., Coppée, J.-Y., and Dillies, M.-A. (2016). SARTools: A DESeq2- and EdgeR-Based R Pipeline for Comprehensive Differential Analysis of RNA-Seq Data. PLoS ONE 11, e0157022. doi:10.1371/journal.pone.0157022
Wakao, S., and Niyogi, K. K. (2021). Chlamydomonas as a Model for Reactive Oxygen Species Signaling and Thiol Redox Regulation in the green Lineage. Plant Physiol. 187, 687–698. doi:10.1093/plphys/kiab355
Wang, J., Hou, Q., Li, P., Yang, L., Sun, X., Benedito, V. A., et al. (2017). Diverse Functions of Multidrug and Toxin Extrusion (MATE) Transporters in Citric Acid Efflux and Metal Homeostasis inMedicago Truncatula. Plant J. 90, 79–95. doi:10.1111/tpj.13471
Wang, Y., Seppänen-Laakso, T., Rischer, H., and Wiebe, M. G. (2018). Euglena Gracilis Growth and Cell Composition under Different Temperature, Light and Trophic Conditions. PLoS ONE 13, e0195329. doi:10.1371/journal.pone.0195329
Watanabe, M., and Suzuki, T. (2002). Involvement of Reactive Oxygen Stress in Cadmium-Induced Cellular Damage in Euglena Gracilis. Comp. Biochem. Physiol. C: Toxicol. Pharmacol. 131, 491–500. doi:10.1016/S1532-0456(02)00036-4
Waterhouse, R. M., Seppey, M., Simão, F. A., Manni, M., Ioannidis, P., Klioutchnikov, G., et al. (2018). BUSCO Applications from Quality Assessments to Gene Prediction and Phylogenomics. Mol. Biol. Evol. 35, 543–548. doi:10.1093/molbev/msx319
Keywords: mass spectrometry, mercury, RNA-sequencing, metal stress, algal biomolecules, reactive oxygen species
Citation: Mangal V, Donaldson ME, Lewis A, Saville BJ and Guéguen C (2022) Identifying Euglena Gracilis Metabolic and Transcriptomic Adaptations in Response to Mercury Stress. Front. Environ. Sci. 10:836732. doi: 10.3389/fenvs.2022.836732
Received: 15 December 2021; Accepted: 11 February 2022;
Published: 15 March 2022.
Edited by:
Jian Chen, Florida International University, United StatesReviewed by:
Jiangxin Wang, Shenzhen University, ChinaManoj Kamalanathan, Texas A&M University at Galveston, United States
Copyright © 2022 Mangal, Donaldson, Lewis, Saville and Guéguen. This is an open-access article distributed under the terms of the Creative Commons Attribution License (CC BY). The use, distribution or reproduction in other forums is permitted, provided the original author(s) and the copyright owner(s) are credited and that the original publication in this journal is cited, in accordance with accepted academic practice. No use, distribution or reproduction is permitted which does not comply with these terms.
*Correspondence: Céline Guéguen, Y2VsaW5lLmd1ZWd1ZW5AdXNoZXJicm9va2UuY2E=
†Present address: Vaughn Mangal, Department of Physical and Environmental Sciences, University of Toronto, Toronto, ON, Canada