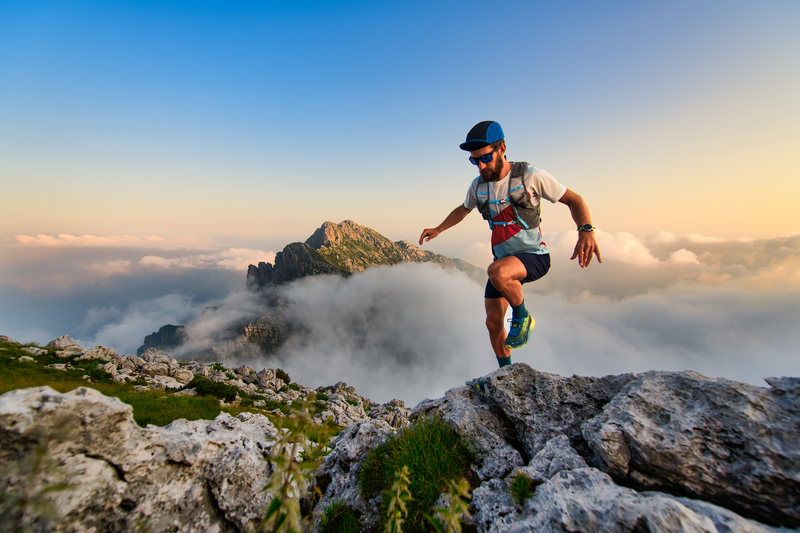
95% of researchers rate our articles as excellent or good
Learn more about the work of our research integrity team to safeguard the quality of each article we publish.
Find out more
ORIGINAL RESEARCH article
Front. Environ. Sci. , 18 February 2022
Sec. Atmosphere and Climate
Volume 10 - 2022 | https://doi.org/10.3389/fenvs.2022.836191
This article is part of the Research Topic Atmospheric Air Pollution and Its Environmental and Health Effects View all 14 articles
Surface ozone (O3) increased by ∼20% in the Yangtze River Delta (YRD) region of China during 2014–2020, but the aggravating trend is highly variable on interannual time and city-level space scales. Here, we employed multiple air quality observations and numerical simulation to describe the increasing but variable trend of O3 and to reveal the main driving factors behind it. In 2014–2017, the governmental air pollution control action plan was mostly against PM2.5 (mainly to control the emissions of SO2, NOx, and primary PM2.5) and effectively reduced the PM2.5 concentration by 18%–45%. However, O3 pollution worsened in the same period with an increasing rate of 4.9 μg m−3 yr−1, especially in the Anhui province, where the growth rate even reached 14.7 μg m−3 yr−1. After 2018, owing to the coordinated prevention and control of both PM2.5 and O3, volatile organic compound (VOC) emissions in the YRD region has also been controlled with a great concern, and the O3 aggravating trend in the same period has been obviously alleviated (1.1 μg m−3 yr−1). We further combined the precursor concentration and the corresponding O3 formation regime to explain the observed trend of O3 in 2014–2020. The leading O3 formation regime in 2014–2017 is diagnosed as VOC-limited (21%) or mix-limited (58%), with the help of a simulated indicator HCHO/NOy. Under such condition, the decreasing NO2 (2.8% yr−1) and increasing VOCs (3.6% yr−1) in 2014–2017 led to a rapid increment of O3. With the continuous reduction in NOx emission and further in ambient NOx/VOCs, the O3 production regime along the Yangtze River has been shifting from VOC-limited to mix-limited, and after 2018, the mix-limited regime has become the dominant O3 formation regime for 55% of the YRD cities. Consequently, the decreases of both NOx (3.3% yr−1) and VOCs (7.7% yr−1) in 2018–2020 obviously slowed down the aggravating trend of O3. Our study argues that with the implementation of coordinated regional reduction of NOx and VOCs, an effective O3 control is emerging in the YRD region.
Tropospheric ozone (O3) is a critical secondary air pollutant featured with strong oxidation (Thompson, 1992), which is produced via complicated photochemical reactions with volatile organic compounds (VOCs) and nitrogen oxides (NOx, x = 1 and 2) acting as its precursors (Seinfeld and Pandis, 2006; Wang et al., 2017). A high concentration of O3 may degrade air quality and pose health risks to humans, such as respiratory illness and premature birth (Barry et al., 2019; Ekland et al., 2021; Guan et al., 2021). Moreover, a severe O3 pollution episode leads to premature mortality as well. A rise of 10 μg m−3 in O3 concentration would increase mortality by 2% (Lei et al., 2019). For the ecosystem, O3 can impair plant production by getting into leaves through stomata (Ainsworth et al., 2012). Agricultural yields are also negatively impacted by O3 with losses ranging from 6% to 15% for wheat products in China (Feng et al., 2015). At the same time, as an oxidant, the elevated O3 also promotes the formation of secondary aerosols (Chen et al., 2020b; Feng et al., 2021). For example, the enhanced O3 and secondary aerosols induced an unexpected haze event in Beijing during the COVID-19 lockdown (Huang et al., 2020; Le et al., 2020).
In 2013, China implemented the Air Pollution Prevention and Control Action Plan (APPCAP), which includes a series of measures for alleviating the current air pollution with particular attention to fine particulate matter (PM2.5). The PM2.5 pollution was, thus, effectively controlled with more than 30% of nationwide PM2.5 reduced by the end of 2018 (Zhai et al., 2019). At the same time, O3 concentration indicated an inverse trend. The average maximum daily average 8-h O3 at the 90th percentile (MDA8-90) in 74 major cities increased by 20% from 2013 to 2017 in China. Major causes for the aggravated O3 are summarized as follows. First, a decrease in NOx and increase in VOC emissions (Zheng et al., 2018) facilitated the nonlinear production of O3 in urban areas that belong to VOC-limited (Wang et al., 2017; Li et al., 2019c; Liu and Wang, 2020b). Second, enhanced radiation intensity and increased HO2 radicals caused by the reduced PM2.5 also posed a nonnegligible influence on O3 pollution (Li et al., 2019a). Besides this, the meteorologically driven variability also contributed to the increase of O3 (Liu and Wang, 2020a; Dang et al., 2021). From 2013 to 2017, humidity and wind speed drove an annual increase of 2.3 ppbv in O3 over the Yangtze River Delta (YRD) region (Li et al., 2019b).
The rapid increase of O3 in China since 2013 has been confirmed by many observational and model studies (Shen et al., 2019; Zhai et al., 2019; Chen et al., 2020c; Shen et al., 2020; Liu et al., 2021; Mousavinezhad et al., 2021). However, the upward trend has significant differences in different regions and different periods. Chen et al. (2021) revealed that annual O3 concentration was in continuous increase across the YRD region during 2014–2019 but rose in volatility in the Pearl River Delta (PRD) region over the same period. In addition, the increment of O3 in the YRD region is more obvious with a rate of 3.2 ppbv yr−1 from 50.7 to 66.3 ppbv, which is approximately four times that in the PRD region.
In this study, we used multiple air quality observations in conjunction with a regional chemical model, weather research and forecasting coupled with chemistry (WRF-Chem) to analyze the O3 pollution in the YRD region from 2014 to 2020 with the aim of exploring the interannual and regional variations of O3 and understanding the chemical principles behind it. Our study differs from previous studies in that 1) we combined surface air quality and meteorology observations, satellite remote sensing observations, numerical model simulation and an indicator-based approach to uncover the increasing but variable pattern of O3 in the YRD region, and 2) we further explored and compared the chemical mechanisms and driving factors in different regions and at different stages.
We adopted air quality monitoring measurements from 2014 to 2020 over the YRD region (Figure 1). Data was derived from the public website of the Ministry of Ecology and Environment (MEE) of China (http://106.37.208.233:20035/). MEE provides hourly in situ measurements of six criteria air pollutants, including PM2.5, PM10, NO2, O3, SO2, and CO. A total of 127 sites from 26 cities in the YRD region were utilized for further analyses. Descriptions for the monitoring sites and cities can be found in Supplementary Table S1; Supplementary Figure S1. It is noted that the number of surface observational sites established by MEE continuously increased since 2013. We selected these sites with complete records during 2014–2020 for analyzing. Meanwhile, for measurements of the gaseous pollutants after September 2018, we converted the mass concentration under reference state into that under standard state in advance (https://www.mee.gov.cn/xxgk2018/xxgk/xxgk01/201808/t20180815_629602.html).
FIGURE 1. Simulation domains of the WRF-Chem model used in this study (left). Right panel illustrates the YRD region as well as the locations of 26 cities in the YRD region. Names of the 26 cities are also labeled with colorful text in this panel.
Moreover, we also obtained the hourly meteorological observation, including surface temperature, relative humidity, and wind speed from the open-source website (https://q-weather.info/) for assessing the meteorological conditions in the YRD region. Four meteorological stations located at the provincial cities of the YRD region were collected, including Shanghai, Nanjing, Hangzhou, and Hefei. Illustration of the meteorological stations used in this study is given in Supplementary Table S2; Supplementary Figure S1.
WRF-Chem (Grell et al., 2005) is a regional air quality model developed by the National Center for Atmospheric Research (NCAR), Pacific Northwest National Laboratory (PNNL), and the National Oceanic and Atmospheric Administration (NOAA) of the United States. We applied WRF-Chem (v3.9.1) to simulate the summertime O3 in July over the YRD region from 2014 to 2020. Each month-long simulation was spinned up for the last 2 days of the previous month. Spatially, two nested domains are configured (Figure 1). The parent domain covers the eastern China (100 × 100 grid cells) with a horizontal resolution of 18 km. The nested domain, whose horizontal resolution is 6 km, mainly includes the YRD region (145 × 166 grid cells). A total of 28 vertical layers extends from the ground to the height of 50 hPa. The bottom seven layers distribute below 1 km. The initial and boundary conditions of meteorological fields are determined by FNL reanalysis data sets by the National Centers for Environmental Prediction (NCEP) of the United States. Physical parameterization for describing subgrid processes, such as radiation, microphysics, and the surface layer are listed in Supplementary Table S3. For chemical species, the initial and boundary conditions are provided via the global chemical transport model MOZART (Emmons et al., 2010) and CAM-Chem (Emmons et al., 2020). Meanwhile, the Statewide Air Pollution Research Center (SAPRC99) mechanism is utilized for the gas phase reaction scheme in our study (Carter, 2000). For aerosols, we apply Model for Simulating Aerosol Interactions and Chemistry (MOSAIC) as the aerosol parameterization (Zaveri et al., 2008).
Multi-resolution Emission Inventory for China (MEIC) developed by Tsinghua University was adopted as the anthropogenic emission (Li et al., 2017). MEIC summarizes the 0.25° × 0.25° gridded emissions of SO2, NOx, CO, CO2, NH3, VOCs, EC, OC, PM2.5, and PM10. All species are categorized into five sectors, i.e., agriculture, industry, power, transportation, and residential activities. Online biogenic emissions were calculated by Model of Emissions of Gases and Aerosols from Nature (MEGAN, v2.0.4), which comprehensively took landcover, meteorology, and atmospheric chemical compositions into consideration (Guenther et al., 2006). Biomass burning emissions were provided by the Fire Inventory from NCAR (FINN). Combined with satellite retrieval constrains, FINN can generate high spatio-temporal resolution products of biomass burning emissions (Wiedinmyer et al., 2011).
The ratio of formaldehyde (HCHO) to NOy was utilized as the indicator (i.e., HCHO/NOy) for diagnosing the formation regime of O3 (Hu, 2021). In this indicator, HCHO can track the contribution of VOCs (Cao et al., 2018), which is a transient oxidation product of many VOCs and is positively correlated with peroxyl radicals. NOy describes the impacts of multiple reactive nitrogenous compounds, including NO, NO2, HONO, HNO3, HO2NO2, N2O5, NO3, peroxy acetyl nitrate (PAN), and other organic nitrates. Reactive nitrogen species play a central role in determining the levels of O3 and hydroxyl radicals in the troposphere. In Supplementary Figure S2, NOy is mainly concentrated in cities along the Yangtze River with 7.2 ppbv on average. Considering that photochemistry is the most active in the presence of abundant radiation, we, thus, investigated the O3 formation regime from 12:00 to 16:00 (local time, UTC + 8). When HCHO/NOy is less than 0.3, the O3 formation regime is considered to be VOC-limited. NOx controls O3 production if the ratio is more than 0.72. Otherwise, it is expected to be under a mixed-limited condition, sensitive to changes both in NOx and VOCs. The threshold values were obtained by counting the distribution of the HCHO/NOy for different O3 formation regimes in the YRD, which allow the HCHO/NOy sensitivity zone to be delineated in a way that is more consistent with the EKMA curve and accurately represents the sensitivity of O3 to the precursors (Hu, 2021; Li, 2021).
Reliable simulation of meteorological parameters and air pollutants could be the crucial premise for analyzing complicated causes of O3 pollution. Comparisons between observation versus simulation are concluded in Table 1. To statistically quantify the model performance, we applied two following statistical metrics, i.e., index of agreement (IOA, varies from 0 to 1) and mean fractional bias (MFB, varies from −2 to 2). IOA and MFB are calculated based on Eqs 1–2, where s, o, and N represent simulation, observation, and the number of samples, respectively.
TABLE 1. Model performance in predicting meteorological parameters and air pollutants over the YRD region during the study period.
First, our model is capable of reproducing meteorological conditions, which is the key to simulate horizontal transportation, chemical transformation, and the removal of air pollutants. As shown in Table 1, the mean observed (simulated) temperature, relative humidity, and wind speed is averaged for 301.3 K (300.2 K), 79.1% (79.5%), and 2.9 m/s (3.1 m/s), respectively, in July from 2014 to 2020. Differences in terms of magnitudes between observation and simulation are quite small. All MFBs are less than 10% as well. IOAs for these meteorological parameters vary from 0.64 to 0.85, highlighting that our model has a good agreement with surface observations. The evaluation indicators of each city for each year can be found in the Supplementary Table S4. The city-level IOAs and MFBs varied in 0.6–0.9 and −0.1–0.3 except for the wind speed in 2016, which again confirmed the reasonable model performance.
Moreover, WRF-Chem reasonably reproduced the concentration levels of photochemical products. The mean observed summer O3 and NO2 concentrations are 51.8 and 12.6 ppbv averaged for 2014–2020. Simulations for these two species posed an overestimation by 28% and 12%, according to the hourly MFB index. Meanwhile, both hourly IOA values exceed 0.60, indicating that our model reasonably captures the temporal variations of O3 and NO2 as well. WRF-Chem also well simulates PM2.5 with a predicted IOA of 0.59 as well as a MFB of –17%.
Supplementary Figures S3, S4 show scatterplots of simulations versus observations in daily MDA8 O3 and NO2 concentrations and their regression parameters in the 26 cities of the YRD region. The simulated O3 demonstrates various model performance in different cities. The daily IOA of O3 ranges from 0.55 (Hefei) to 0.83 (Shanghai) and the corresponding daily MFB poses a range from 13% (Shanghai) to 49% (Wuhu). Meanwhile, most of the fitted slopes of O3 range from 0.9 to 1.3, further confirming that our model is reliable in reproducing concentrations of O3. In addition, we verified the diurnal patterns of O3 in all 26 cities of the YRD region as well. As shown in Supplementary Figure S5, both observation and simulation pose the same diurnal patterns with the peak O3 concentration occurring between 12:00 and 16:00. The correlation coefficients (R) are more than 0.96, implying that diurnal variations of O3 are successfully captured.
We first analyzed the observed spatio-temporal variations of six air pollutants over the YRD region from 2014 to 2020. As illustrated in Supplementary Figure S6, regional mean O3 concentration is 107.1 μg m−3 in 2020, which increased by 18% compared with the concentration level in 2014. In contrast, concentrations of other five air pollutants sharply decreased over the study period. For example, the annual mean SO2 shows a dramatic drop by 16.8 μg m−3 from 2014 to 2020 with a relative reduction of 68%. At the same time, both PM2.5 and PM10 concentrations are also reduced by more than 50%. It is precisely because of this decline that the dominant air pollutant in the YRD region has been changing from PM2.5 to O3. For NO2, the variation trend generally showed a decrease with a rate of 0.8 μg m−3 yr−1 even though an individual slight increase occurred in 2017. The changes of city-level pollutant concentrations are shown in Supplementary Figure S7. In addition, Figure 2; Supplementary Figures S8–S12 show the frequency distribution of O3, NO2, PM2.5, PM10, CO, and SO2 daily concentrations, respectively, which again emphasize that the concentration distribution of O3 is changing from a low to a high value region while other pollutants show an opposite trend.
FIGURE 2. Frequency distribution of the MDA8 O3 concentration in the YRD region during the study period. Red bars represent the values of 2014. Blue bars indicate the values in the other year during 2015–2020. The colored shaded areas show different pollution levels according to the National Ambient Air Quality Standard of China.
Based on the above analysis, we further find that the upward trend of O3 is significantly different at different stages. In general, the increasing trend of O3 concentration during 2014–2017 is more obvious in contrast to the years 2018–2020. Annual mean O3 rapidly increased by 4.9 μg m−3 yr−1 (5.5% yr−1) during the period of 2014–2017, while in 2018–2019, the increasing trend slowed down, with the increase rate dropped to 1.1 μg m−3 yr−1. Even in 2020, O3 in many YRD cities has decreased by 0.1–14.3 μg m−3 compared with 2019, mainly caused by the reduction of human activity and the more precipitation in 2020, especially in mobile sources and industry (Chen H. et al., 2020; Huang et al., 2021).
In addition, the upward trend of O3 is also different in different regions of the YRD. As shown in Figure 3, the growth of O3 in the Anhui Province is faster than that in other YRD regions. Especially during 2014–2017, the O3 concentration in Anhui increased by a rate of 14.7 μg m−3 yr−1—four times that in other provinces (i.e., Shanghai, Jiangsu, and Zhejiang, SJZ). Such trends can also be observed on the city scale (Supplementary Table S5; Supplementary Figure S7). Hefei doubled its O3 concentration between 2014 and 2017 while, in Shanghai, Nanjing, and Hangzhou, the increase was 9.7%–14.3%. To highlight the distinctive variation of O3 in Anhui, we further compared the frequency distributions of O3 in Anhui with that in other regions from 2014 to 2020 (Figure 2). In 2014, the O3 concentration in Anhui was mostly concentrated around 50 μg m−3 while the city-level O3 concentration in SJZ distributed in a range of 14.8–256.7 μg m−3, and the average value was 80% higher than that in Anhui. However, after 2014, the O3 in Anhui prominently increased with the frequency distribution rapidly moved to the high value scope (central at ∼70 μg m−3), and by 2018, it was close to other regions of YRD.
FIGURE 3. Monthly variations of SO2, NO2, PM2.5, CO, O3, and PM10 concentrations over the YRD region in 2014 (filled dots). Also shown are the differences between 2014 and each of 2015–2020 (bars).
We analyze the interannual variation of O3 in the YRD region from 2014 to 2020 through two factors. One is the O3 formation regime, and the other is the precursor (NOx and VOCs) concentrations. The dual role of NOx in O3 generation results in the variation of O3 formation regime. NOx can either promote O3 production through photochemical reactions with atomic oxygen (O), O2, or mitigate O3 formation by removing OH from the oxidation cycle. At the same time, ambient radical budget and weather conditions can also affect the O3 generation regime (Li et al., 2019b; Liu and Wang, 2020a; Lin et al., 2020; Wang et al., 2020).
We employed HCHO/NOy as an indicator to diagnose the formation regime of O3. Figure 4 shows the spatial distributions of the indicator over the YRD region simulated by WRF-Chem. The surrounding areas of the Yangtze River (purple line) are mostly under VOC-limited, mainly due to the abundant NOx emissions from industrial and shipping activities (An et al., 2021). In contrast, the south regions of YRD demonstrate a universal pattern of NOx-limited. This could be attributed to the substantial biogenic VOCs emissions from the forest districts in Zhejiang province (Cao et al., 2021). Other YRD regions are mainly controlled by both NOx and VOCs.
FIGURE 4. Spatial distribution of the indicator (HCHO/NOy) for diagnosing the O3 formation regime over the YRD region in the summertime. (A) indicates 2014–2017, and (B) indicates 2018–2020. Red, green, and blue shaded areas represent VOC-limited, mix-limited, and NOx-limited condition, respectively.
To better understand the fate of O3 formation regime, we primarily explore the emission characteristics of NOx and VOCs during this period. As shown in Figure 5, the NOx emission over the YRD region was continuously reduced by a rate of 3.5% yr−1 from 2014 to 2020, which was largely benefited from the APPCAP (http://www.gov.cn/zwgk/2013-09/12/content_2486773.htm). Unprecedented emission reductions due to COVID-19 in 2020 also enhance this trend (Wang G. et al., 2021; Li et al., 2021b; Hu et al., 2021).
FIGURE 5. The schematic diagram for illustrating changes of precursor emissions and concentrations, O3 formation regime and O3 concentration over the YRD region during the study period.
However, during 2014 and 2017, the VOC emissions in the YRD region were not effectively controlled and even increased by 0.8% yr−1. After 2018, with the implementation of strict VOC control (http://www.gov.cn/zhengce/content/2018-07/03/content_5303158.htm), VOC emissions began to decline, and in 2020, the VOC emissions in the YRD region dropped by 15.4% compared with the highest level in 2017. The decline in aromatics propene/ethene emission from the petrochemical industry as well as gasoline evaporation and vehicular emissions is the main component of the reduction of VOCs emissions in 2020 (Wang M. et al., 2021; Qi et al., 2021). The distribution of emission variation also reveals similar trends with the whole region (Supplementary Figure S13). Emissions of NOx in 2018–2020 decreased by 17.7%–50.8% compared to 2014–2017 in most regions of the YRD. VOCs emissions for 87.8% of YRD areas are reduced in 2018–2020 relative to 2014–2017.
With the changes in NOx and VOCs emissions, the O3 formation regime has also changed in response. In 2014–2017, most cities along the Yangtze River are in VOC-limited areas, and other regions are mainly under mix-limited except for the forested areas in southern Zhejiang. Since 2018, the original VOC-limited region has shifted to mix-limited (36%). It should be noted that there are still 15% areas controlled by VOC-limited over the YRD region.
In addition, the concentrations of NOx and VOCs in the YRD region show a consistent trend as their emissions. As shown in Figure 5, the NO2 concentration clearly demonstrates a continuous decreasing trend over the YRD region during 2014–2020. In term of VOCs, we employed the column density of HCHO via satellite retrievals to represent the interannual variations of VOCs (Li R. et al., 2021). The column density of HCHO shows an inverted “U” shape, which increases from 7.2 × 1015 molec cm2 (2014) to 8.0 × 1015 molec·cm2 (2017) and then drops to 7.4 × 1015 molec cm2 (2020).
As a result of the changes of both O3 formation regime and precursor concentrations, the O3 concentration in the YRD region shows an increasing but variable trend during 2014–2020. Between 2014–2017, the declined NO2 and the elevated VOCs, under the VOC-limited and mix-limited O3 formation regime, led to a rapid increase of O3. However, when it comes to 2018–2020, the VOCs began to slowly decline, and meanwhile, the formation regime shifted to more mix-limited so that the upward trend of O3 has obviously slowed down and even appeared as a downward trend in 2020.
The main reason of the rapid growth of O3 in Anhui, which is different from that in other regions, is the distinctive growth of NOx during 2014–2017. The NO2 in Anhui elevated with a rate of 2 μg m−3 yr−1 while, in other YRD regions, it posed a remarkable decline of 1.1 μg m−3 yr−1. At the same time, the O3 formation regime in Anhui was mostly controlled by NOx-limited (24%) or mix-limited conditions (65%). It is also different from other YRD regions where more than 27% areas were controlled by VOC-limited condition. The ambient non-VOC-limited condition is conducive to generation of O3 when NO2 increases, via enhancing the “NOx cycle” (Wang et al., 2017). In addition, the VOCs concentration in Anhui also increased in 2014–2017, which had not yet received much attention over the early stage. It intensified the O3 production as well. Since 2018, the concentration of O3 in Anhui (130.6 μg m−3) reached the same level as other regions (122.9 μg m−3).
Though the emission control strategy since 2018 is effective for slowing down the worsening O3 pollution over the YRD region, there is still a long way to go to see continuous decline in O3 concentration. Meanwhile, previous studies point out that the O3 formation regime in other Chinese city clusters, such as the north China plain and PRD, are dominated by VOC-limited and changes toward mix-limited (Jiang et al., 2018; Wang et al., 2019). To address this issue, a more efficient synergistic measure should be taken to control both NOx and VOCs (Li et al., 2019c; Huang et al., 2020). O3 concentration can be quickly reduced with a reasonable reduction ratio between these two precursors according to its nonlinear formation regime. In addition, it is also complicated to conduct the reduction of VOCs emissions. A comprehensive source apportionment for detailed VOCs speciation is necessary for planning better control policies (Wu and Xie, 2017).
Meanwhile, the mitigation of O3 that is focused on other seasons except for summertime is also proved to be meaningful. Previous case studies of air quality, such as research during COVID-19 or summit exhibited that O3 could increase resulting from the weakened titration of NOx due to the reduction of its emissions (Le et al., 2020; Li K. et al., 2021; Zhang et al., 2021b). The elevated O3 further facilitated the production of secondary aerosols and even led to haze events (Chang et al., 2020; Zhang et al., 2021a; Wang N. et al., 2021). Thus, controlling O3 concentration during other nonwarm seasons also helps to eliminate the negative environmental impacts by O3 and PM2.5.
In this study, we employed multiple air quality observations and WRF-Chem simulation to describe the increasing but variable trend of O3 concentration in the YRD region from 2014 to 2020, and further revealed the main factors that caused such trends. During the period of 2014–2020, the annual average O3 concentration in the YRD region was 101 μg m−3 with an increase from 93.4 μg m−3 in 2014 to 107 μg m−3 in 2020. The increase rate was 4.9 μg m−3 yr−1 in the first 4 years, which was four times higher than that in 2018–2020. It should be noted that the O3 concentration in Anhui significantly increased by 44.1 μg m−3 during 2014–2017, twofold higher than that in the YRD region (13.0 μg m−3). Since 2018, both Anhui and other regions in the YRD slowed down their increase in O3.
We further analyzed the two main factors driving the interannual variation of O3 in the YRD region, including precursor concentrations and the corresponding O3 formation regime. With the help of the indicator HCHO/NOy simulated by WRF-Chem, we concluded that the O3 production regime along the Yangtze River has shifted from VOC-limited to mix-limited. In 2014–2017, most cities along the Yangtze River were in VOC-limited areas, and other regions were mainly under mix-limited. After 2018, 36% areas that belong to the VOC-limited condition shifted to a mix-limited condition. Therefore, the alleviative NOx and elevated VOCs under a VOC- and mix-limited O3 formation regime generally contributed to the cumulative rise in O3 concentration. However, since 2018, the drop of both precursors under the mix-limited O3 formation regime alleviated the worsening O3 pollution. Our work is of importance to understand the current O3 pollution in a megacity cluster of China and offers an investigative insight for further alleviating O3 pollution in the future.
The original contributions presented in the study are included in the article/Supplementary Material, further inquiries can be directed to the corresponding author.
Data curation, model simulation, visualization, and writing-original draft preparation, KT and HZ; Supervision, funding acquisition, writing-review and editing, NL, HL, and JH; Data curation, WF. All authors have read and agreed to the published version of the manuscript.
This work was supported by the National Key Research and Development Program of China (2019YFA0606804), the National Natural Science Foundation of China (41975171), the Major Research Plan of the National Social Science Foundation (18ZDA052).
The authors declare that the research was conducted in the absence of any commercial or financial relationships that could be construed as a potential conflict of interest.
All claims expressed in this article are solely those of the authors and do not necessarily represent those of their affiliated organizations, or those of the publisher, the editors, and the reviewers. Any product that may be evaluated in this article or claim that may be made by its manufacturer, is not guaranteed or endorsed by the publisher.
The Supplementary Material for this article can be found online at: https://www.frontiersin.org/articles/10.3389/fenvs.2022.836191/full#supplementary-material
Ainsworth, E. A., Yendrek, C. R., Sitch, S., Collins, W. J., and Emberson, L. D. (2012). The Effects of Tropospheric Ozone on Net Primary Productivity and Implications for Climate Change. Annu. Rev. Plant Biol. 63, 637–661. doi:10.1146/annurev-arplant-042110-103829
An, J., Huang, Y., Huang, C., Wang, X., Yan, R., Wang, Q., et al. (2021). Emission Inventory of Air Pollutants and Chemical Speciation for Specific Anthropogenic Sources Based on Local Measurements in the Yangtze River Delta Region, China. Atmos. Chem. Phys. 21 (3), 2003–2025. doi:10.5194/acp-21-2003-2021
Barry, V., Klein, M., Winquist, A., Chang, H. H., Mulholland, J. A., Talbott, E. O., et al. (2019). Characterization of the Concentration-Response Curve for Ambient Ozone and Acute Respiratory Morbidity in 5 US Cities. J. Expo. Sci. Environ. Epidemiol. 29 (2), 267–277. doi:10.1038/s41370-018-0048-7
Cao, H., Fu, T.-M., Zhang, L., Henze, D., Miller, C., Lerot, C., et al. (2018). Adjoint Inversion of Chinese Non-methane Volatile Organic Compound Emissions Using Space-Based Observations of Formaldehyde and Glyoxal. Atmos. Chem. Phys. Discuss. 18, 1–46. doi:10.5194/acp-2017-1136
Cao, J., Situ, S., Hao, Y., Xie, S., and Li, L. (2021). Enhanced Summertime Ozone and SOA from Biogenic Volatile Organic Compound (BVOC) Emissions Due to Vegetation Biomass Variability during 1981–2018 in China. Atmos. Chem. Phys. Discuss. 2021, 1–21. doi:10.5194/acp-2021-675
Carter, W. P. L. (2000). Documentation of the SAPRC-99 Chemical Mechanism for VOC Reactivity Assessment. California: University of California Riverside. Report to the California Air Resources Board, Contract 92-329. 95-308.
Chang, Y., Huang, R. J., Ge, X., Huang, X., Hu, J., Duan, Y., et al. (2020). Puzzling Haze Events in China during the Coronavirus (COVID-19) Shutdown. Geophys. Res. Lett. 47, e2020GL088533. doi:10.1029/2020GL088533
Chen, H., Huo, J., Fu, Q., Duan, Y., Xiao, H., and Chen, J. (2020a). Impact of Quarantine Measures on Chemical Compositions of PM2.5 during the COVID-19 Epidemic in Shanghai, China. Sci. Total Environ. 743, 140758. doi:10.1016/j.scitotenv.2020.140758
Chen, L., Xing, J., Mathur, R., Liu, S., Wang, S., and Hao, J. (2020b). Quantification of the Enhancement of PM2.5 Concentration by the Downward Transport of Ozone from the Stratosphere. Chemosphere 255, 126907. doi:10.1016/j.chemosphere.2020.126907
Chen, L., Zhu, J., Liao, H., Yang, Y., and Yue, X. (2020c). Meteorological Influences on PM2.5 and O3 Trends and Associated Health burden since China's Clean Air Actions. Sci. Total Environ. 744, 140837. doi:10.1016/j.scitotenv.2020.140837
Chen, X., Jiang, Z., Shen, Y., Li, R., Fu, Y., Liu, J., et al. (2021). Chinese Regulations Are Working—Why Is Surface Ozone over Industrialized Areas Still High? Applying Lessons from Northeast US Air Quality Evolution. Geophys. Res. Lett. 48 (14), e2021GL092816. doi:10.1029/2021gl092816
Dang, R., Liao, H., and Fu, Y. (2021). Quantifying the Anthropogenic and Meteorological Influences on Summertime Surface Ozone in China over 2012-2017. Sci. Total Environ. 754, 142394. doi:10.1016/j.scitotenv.2020.142394
Ekland, J., Olsson, D., Forsberg, B., Andersson, C., and Orru, H. (2021). The Effect of Current and Future Maternal Exposure to Near-Surface Ozone on Preterm Birth in 30 European Countries—An EU-wide Health Impact Assessment. Environ. Res. Lett. 16 (5). doi:10.1088/1748-9326/abe6c4
Emmons, L. K., Schwantes, R. H., Orlando, J. J., Tyndall, G., Kinnison, D., Lamarque, J.-F., et al. (2020). The Chemistry Mechanism in the Community Earth System Model Version 2 (CESM2). J. Adv. Model. Earth Syst. 12 (4), e2019MS001882. doi:10.1029/2019MS001882
Emmons, L. K., Walters, S., Hess, P. G., Lamarque, J. F., Pfister, G. G., Fillmore, D., et al. (2010). Description and Evaluation of the Model for Ozone and Related Chemical Tracers, Version 4 (MOZART-4). Geosci. Model. Dev. 3 (1), 43–67. doi:10.5194/gmd-3-43-2010
Feng, T., Zhao, S., Bei, N., Liu, S., and Li, G. (2021). Increasing Atmospheric Oxidizing Capacity Weakens Emission Mitigation Effort in Beijing during Autumn Haze Events. Chemosphere 281, 130855. doi:10.1016/j.chemosphere.2021.130855
Feng, Z., Hu, E., Wang, X., Jiang, L., and Liu, X. (2015). Ground-level O3 Pollution and its Impacts on Food Crops in China: a Review. Environ. Pollut. 199, 42–48. doi:10.1016/j.envpol.2015.01.016
Grell, G. A., Peckham, S. E., Schmitz, R., McKeen, S. A., Frost, G., Skamarock, W. C., et al. (2005). Fully Coupled “Online” Chemistry within the WRF Model. Atmos. Environ. 39 (37), 6957–6975. doi:10.1016/j.atmosenv.2005.04.027
Guan, Y., Xiao, Y., Wang, F., Qiu, X., and Zhang, N. (2021). Health Impacts Attributable to Ambient PM2.5 and Ozone Pollution in Major Chinese Cities at Seasonal-Level. J. Clean. Prod. 311, 127510. doi:10.1016/j.jclepro.2021.127510
Guenther, A., Karl, T., Harley, P., Wiedinmyer, C., Palmer, P. I., and Geron, C. (2006). Estimates of Global Terrestrial Isoprene Emissions Using MEGAN (Model of Emissions of Gases and Aerosols from Nature). Atmos. Chem. Phys. 6 (11), 3181–3210. doi:10.5194/acp-6-3181-2006
Hu, J. (2021). “Exploring the Applicability of Indicators for Determining Ozone Sensitivity (In Chinese),” in The 3rd Workshop on Atmospheric Ozone Pollution Prevention and Control in China (Shanghai, China: Chinese Society For Environmental Sciences).
Hu, X., Liu, Q., Fu, Q., Xu, H., Shen, Y., Liu, D., et al. (2021). A High-Resolution Typical Pollution Source Emission Inventory and Pollution Source Changes during the COVID-19 Lockdown in a Megacity, China. Environ. Sci. Pollut. Res. Int. 28 (33), 45344–45352. doi:10.1007/s11356-020-11858-x
Huang, C., An, J., Wang, H., Liu, Q., Tian, J., Wang, Q., et al. (2021). Highly Resolved Dynamic Emissions of Air Pollutants and Greenhouse Gas CO2 during COVID-19 Pandemic in East China. Environ. Sci. Technology Lett. 8 (10), 853–860. doi:10.1021/acs.estlett.1c00600
Huang, X., Ding, A., Gao, J., Zheng, B., Zhou, D., Qi, X., et al. (2020). Enhanced Secondary Pollution Offset Reduction of Primary Emissions during COVID-19 Lockdown in China. Natl. Sci. Rev. 8 (2), nwaa137. doi:10.1093/nsr/nwaa137
Jiang, M., Lu, K., Su, R., Tan, Z., Wang, H., Li, L., et al. (2018). Ozone Formation and Key VOCs in Typical Chinese City Clusters. Chin. Sci. Bull. 63 (12), 1130–1141. doi:10.1360/n972017-01241
Le, T., Wang, Y., Liu, L., Yang, J., Yung, Y. L., Li, G., et al. (2020). Unexpected Air Pollution with Marked Emission Reductions during the COVID-19 Outbreak in China. Science 369 (6504), 702–706. doi:10.1126/science.abb7431
Lei, R., Zhu, F., Cheng, H., Liu, J., Shen, C., Zhang, C., et al. (2019). Short-term Effect of PM2.5/O3 on Non-accidental and Respiratory Deaths in Highly Polluted Area of China. Atmos. Pollut. Res. 10 (5), 1412–1419. doi:10.1016/j.apr.2019.03.013
Li, K., Jacob, D. J., Liao, H., Qiu, Y., Shen, L., Zhai, S., et al. (2021a). Ozone Pollution in the North China Plain Spreading into the Late-winter Haze Season. Proc. Natl. Acad. Sci. U S A. 118 (10), e2015797118. doi:10.1073/pnas.2015797118
Li, K., Jacob, D. J., Liao, H., Shen, L., Zhang, Q., and Bates, K. H. (2019a). Anthropogenic Drivers of 2013-2017 Trends in Summer Surface Ozone in China. Proc. Natl. Acad. Sci. 116 (2), 422–427. doi:10.1073/pnas.1812168116
Li, K., Jacob, D. J., Liao, H., Shen, L., Zhang, Q., and Bates, K. H. (2019b). Anthropogenic Drivers of 2013-2017 Trends in Summer Surface Ozone in China. Proc. Natl. Acad. Sci. U S A. 116 (2), 422–427. doi:10.1073/pnas.1812168116
Li, K., Jacob, D. J., Liao, H., Zhu, J., Shah, V., Shen, L., et al. (2019c). A Two-Pollutant Strategy for Improving Ozone and Particulate Air Quality in China. Nat. Geosci. 12, 906–910. doi:10.1038/s41561-019-0464-x
Li, M., Wang, T., Xie, M., Li, S., Zhuang, B., Fu, Q., et al. (2021b1994). Drivers for the Poor Air Quality Conditions in North China Plain during the COVID-19 Outbreak. Atmos. Environ. 246, 118103. doi:10.1016/j.atmosenv.2020.118103
Li, M., Zhang, Q., Kurokawa, J. I., Woo, J. H., He, K., Lu, Z., et al. (2017). MIX: a Mosaic Asian Anthropogenic Emission Inventory under the International Collaboration Framework of the MICS-Asia and HTAP. Atmos. Chem. Phys. 17 (2), 935–963. doi:10.5194/acp-17-935-2017
Li, R., Xu, M., Li, M., Chen, Z., Zhao, N., Gao, B., et al. (2021c). Identifying the Spatiotemporal Variations in Ozone Formation Regimes across China from 2005 to 2019 Based on Polynomial Simulation and Causality Analysis. Atmos. Chem. Phys. 21 (20), 15631–15646. doi:10.5194/acp-21-15631-2021
Li, X. (2021). Research On the Applicability of Ozone Sensitivity Determination Indicators in Jiangsu. Master. Nanjing: Nanjing University of Information Science & Technology.
Lin, H., Wang, M., Duan, Y., Fu, Q., Ji, W., Cui, H., et al. (2020). O3 Sensitivity and Contributions of Different NMHC Sources in O3 Formation at Urban and Suburban Sites in Shanghai. Atmosphere 11 (3), 295. doi:10.3390/atmos11030295
Liu, H., Liu, J., Liu, Y., Yi, K., Yang, H., Xiang, S., et al. (2021). Spatiotemporal Variability and Driving Factors of Ground-Level Summertime Ozone Pollution over Eastern China. Atmos. Environ. 265, 118686. doi:10.1016/j.atmosenv.2021.118686
Liu, Y., and Wang, T. (2020a). Worsening Urban Ozone Pollution in China from 2013 to 2017 – Part 1: The Complex and Varying Roles of Meteorology. Atmos. Chem. Phys. 20 (11), 6305–6321. doi:10.5194/acp-20-6305-2020
Liu, Y., and Wang, T. (2020b). Worsening Urban Ozone Pollution in China from 2013 to 2017 – Part 2: The Effects of Emission Changes and Implications for Multi-Pollutant Control. Atmos. Chem. Phys. 20 (11), 6323–6337. doi:10.5194/acp-20-6323-2020
Mousavinezhad, S., Choi, Y., Pouyaei, A., Ghahremanloo, M., and Nelson, D. L. (2021). A Comprehensive Investigation of Surface Ozone Pollution in China, 2015–2019: Separating the Contributions from Meteorology and Precursor Emissions. Atmos. Res. 257, 105599. doi:10.1016/j.atmosres.2021.105599
Qi, J., Mo, Z., Yuan, B., Huang, S., Huangfu, Y., Wang, Z., et al. (2021). An Observation Approach in Evaluation of Ozone Production to Precursor Changes during the COVID-19 Lockdown. Atmos. Environ. 262, 118618. doi:10.1016/j.atmosenv.2021.118618
Seinfeld, J. H., and Pandis, S. N. (2006). Atmospheric Chemistry and Physics: From Air Pollution to Climate Change. Third edition. New York: John Wiley.
Shen, F., Zhang, L., Jiang, L., Tang, M., Gai, X., Chen, M., et al. (2020). Temporal Variations of Six Ambient Criteria Air Pollutants from 2015 to 2018, Their Spatial Distributions, Health Risks and Relationships with Socioeconomic Factors during 2018 in China. Environ. Int. 137, 105556. doi:10.1016/j.envint.2020.105556
Shen, L., Jacob, D. J., Liu, X., Huang, G., Li, K., Liao, H., et al. (2019). An Evaluation of the Ability of the Ozone Monitoring Instrument (OMI) to Observe Boundary Layer Ozone Pollution across China: Application to 2005–2017 Ozone Trends. Atmos. Chem. Phys. 19 (9), 6551–6560. doi:10.5194/acp-19-6551-2019
Thompson, A. M. (1992). The Oxidizing Capacity of the Earth's Atmosphere: Probable Past and Future Changes. Science 256 (5060), 1157–1165. doi:10.1126/science.256.5060.1157
Wang, G., Huang, K., Fu, Q., Chen, J., Huo, J., Zhao, Q., et al. (2021a). Response of PM2.5-bound Elemental Species to Emission Variations and Associated Health Risk Assessment during the COVID-19 Pandemic in a Coastal Megacity. J. Environ. Sci. 122, 115–127. doi:10.1016/j.jes.2021.10.005
Wang, G., Jia, S., Li, R., Ma, S., Chen, X., Wu, Z., et al. (2020). Seasonal Variation Characteristics of Hydroxyl Radical Pollution and its Potential Formation Mechanism during the Daytime in Lanzhou. J. Environ. Sci. 95, 58–64. doi:10.1016/j.jes.2020.03.045
Wang, M., Lu, S., Shao, M., Zeng, L., Zheng, J., Xie, F., et al. (2021b). Impact of COVID-19 Lockdown on Ambient Levels and Sources of Volatile Organic Compounds (VOCs) in Nanjing, China. Sci. Total Environ. 757, 143823. doi:10.1016/j.scitotenv.2020.143823
Wang, N., Lyu, X., Deng, X., Huang, X., Jiang, F., and Ding, A. (2019). Aggravating O3 Pollution Due to NOx Emission Control in Eastern China. Sci. Total Environ. 677, 732–744. doi:10.1016/j.scitotenv.2019.04.388
Wang, N., Xu, J., Pei, C., Tang, R., Zhou, D., Chen, Y., et al. (2021c). Air Quality during COVID-19 Lockdown in the Yangtze River Delta and the Pearl River Delta: Two Different Responsive Mechanisms to Emission Reductions in China. Environ. Sci. Technol. 55 (9), 5721–5730. doi:10.1021/acs.est.0c08383
Wang, T., Xue, L., Brimblecombe, P., Lam, Y. F., Li, L., and Zhang, L. (2017). Ozone Pollution in China: A Review of Concentrations, Meteorological Influences, Chemical Precursors, and Effects. Sci. Total Environ. 575, 1582–1596. doi:10.1016/j.scitotenv.2016.10.081
Wiedinmyer, C., Akagi, S. K., Yokelson, R. J., Emmons, L. K., Al-Saadi, J. A., Orlando, J. J., et al. (2011). The Fire INventory from NCAR (FINN): a High Resolution Global Model to Estimate the Emissions from Open Burning. Geoscientific Model. Development 4 (3), 625–641. doi:10.5194/gmd-4-625-2011
Wu, R., and Xie, S. (2017). Spatial Distribution of Ozone Formation in China Derived from Emissions of Speciated Volatile Organic Compounds. Environ. Sci. Technol. 51 (5), 2574–2583. doi:10.1021/acs.est.6b03634
Zaveri, R. A., Easter, R. C., Fast, J. D., and Peters, L. K. (2008). Model for Simulating Aerosol Interactions and Chemistry (MOSAIC). J. Geophys. Res. 113 (D13). doi:10.1029/2007jd008782
Zhai, S., Jacob, D. J., Wang, X., Shen, L., Li, K., Zhang, Y., et al. (2019). Fine Particulate Matter (PM2.5) Trends in China, 2013–2018: Separating Contributions from Anthropogenic Emissions and Meteorology. Atmos. Chem. Phys. 19 (16), 11031–11041. doi:10.5194/acp-19-11031-2019
Zhang, H., Li, N., Tang, K., Liao, H., Shi, C., Huang, C., et al. (2021a). Estimation of Secondary PM2.5 in China and the United States Using a Multi-Tracer Approach. Atmos. Chem. Phys. Discuss. 2021, 1–36. doi:10.5194/acp-2021-683
Zhang, H., Tang, K., Feng, W., Yan, X., Liao, H., and Li, N. (2021b). Impact of Short-Term Emission Control Measures on Air Quality in Nanjing during the Jiangsu Development Summit. Front. Environ. Sci. 9, 693513. doi:10.3389/fenvs.2021.693513
Keywords: ozone, YRD region, NO2, O3 formation regime, WRF-Chem
Citation: Tang K, Zhang H, Feng W, Liao H, Hu J and Li N (2022) Increasing but Variable Trend of Surface Ozone in the Yangtze River Delta Region of China. Front. Environ. Sci. 10:836191. doi: 10.3389/fenvs.2022.836191
Received: 15 December 2021; Accepted: 10 January 2022;
Published: 18 February 2022.
Edited by:
Hongmei Xu, Xi’an Jiaotong University, ChinaReviewed by:
Chunlei Cheng, Jinan University, ChinaCopyright © 2022 Tang, Zhang, Feng, Liao, Hu and Li. This is an open-access article distributed under the terms of the Creative Commons Attribution License (CC BY). The use, distribution or reproduction in other forums is permitted, provided the original author(s) and the copyright owner(s) are credited and that the original publication in this journal is cited, in accordance with accepted academic practice. No use, distribution or reproduction is permitted which does not comply with these terms.
*Correspondence: Nan Li, bGluYW5AbnVpc3QuZWR1LmNu
Disclaimer: All claims expressed in this article are solely those of the authors and do not necessarily represent those of their affiliated organizations, or those of the publisher, the editors and the reviewers. Any product that may be evaluated in this article or claim that may be made by its manufacturer is not guaranteed or endorsed by the publisher.
Research integrity at Frontiers
Learn more about the work of our research integrity team to safeguard the quality of each article we publish.