- 1Department of Biological Sciences, University of Notre Dame, Notre Dame, IN, United States
- 2Department of Ecology and Evolutionary Biology, University of California, Irvine, Irvine, CA, United States
Lake sediment microbial communities mediate carbon diagenesis. However, microbial community composition is variable across lakes, and it is still uncertain how variation in community composition influences sediment responses to environmental change. Sediment methane (CH4) production has been shown to be substantially elevated by increased lake primary productivity and organic matter supply. However, the magnitude of the response of CH4 production varies across lakes, and recent studies suggest a role for the microbial community in mediating this response. Here, we conducted sediment incubation experiments across 22 lakes to determine whether variation in sediment microbial community composition is related to the response of sediment CH4 production to increases in organic matter. We sampled the 22 lakes across a gradient of pH in order to investigate lakes with variable sediment microbial communities. We manipulated the incubations with additions of dried algal biomass and show that variation in the response of CH4 production to changes in organic matter supply is significantly correlated with metrics of sediment microbial community composition. Specifically, the diversity and richness of the non-methanogen community was most predictive of sediment CH4 responses to organic matter additions. Additionally, neither metrics of microbial abundance nor preexisting organic matter availability explained meaningful variation in the response. Thus, our results provide experimental support that differences in sediment microbial communities influences CH4 production responses to changes in organic matter availability.
1 Introduction
Methane (CH4) production in lake sediments fuels lake greenhouse gas emissions and is a contributor to atmospheric CH4 concentrations (Bastviken et al., 2011). Variation in CH4 production across lakes has been shown to be correlated with multiple biotic and abiotic variables, including temperature (Duc et al., 2010; Yvon-Durocher et al., 2014; de Jong et al., 2018), stratification dynamics (Fallon et al., 1980; Ford et al., 2002), organic matter availability and lability (West et al., 2012; West et al., 2015; DelSontro et al., 2016; Grasset et al., 2018), and microbial community composition (Bertolet et al., 2019). Understanding sources of variation in lake CH4 production has improved our ability to predict how lake CH4 production and emission may change under future environmental change scenarios.
Recent work has highlighted the role of autochthonous organic matter (OM) in fueling anaerobic decomposition and CH4 production across lakes (Deemer et al., 2016; West et al., 2016). Thus, the global eutrophication of inland waters is expected to increase lake CH4 emissions (Davidson et al., 2018; DelSontro et al., 2018; Sepulveda-Jauregui et al., 2018; Beaulieu et al., 2019). However, the magnitude of the response of lake CH4 dynamics to increases in autochthonous OM has been shown to differ across lakes at both the laboratory (West et al., 2015) and ecosystem (Bertolet et al., 2020) scales. Determining sources of variation in the relationship between autochthonous OM and lake CH4 production is thus essential for constraining future predictions of lake CH4 dynamics.
Variation in the response of lake CH4 production to autochthonous OM supply may be related to the same factors that influence CH4 production, such as temperature, oxygen dynamics, or historic OM availability. However, during eutrophication, sediment temperature and anoxia are often stabilized due to increased stratification (Foley et al., 2012; Müller et al., 2012), and variation in the CH4 production response to increases in OM remains evident even in laboratory experiments when temperature and anoxia are constant (West et al., 2015). Preexisting OM availability may influence the response if increases in autochthonous OM primes the carbon pool and allows for the use of previously unavailable OM (Guenet et al., 2010; Zhang et al., 2017). However, few studies have tested this in lake sediments.
Cross-lake differences in the sediment microbial community are another potentially important source of variation in sediment responses to incoming autochthonous OM. Advances in our ability to observe and quantify microbial communities have revealed tremendous diversity in sediment microbial community composition both within and across lakes (Steger et al., 2011; Bertolet et al., 2019; Orland et al., 2020; Wang et al., 2020). CH4 is produced via the anaerobic metabolism of methanogenic archaea (hereafter referred to as “methanogens”). However, methanogens can utilize only a limited number of substrates (acetate, CO2/H2, formate, methylated compounds, etc.) and are reliant on other members of the microbial community (hereafter referred to as “non-methanogens”) to breakdown complex autochthonous OM into methanogenesis precursors (Nozhevnikova et al., 2007; Liu and Whitman, 2008). Because of these complex biogeochemical interactions, community composition and abundance have the potential to influence sediment responses to increases in autochthonous OM.
Comparative studies have provided substantial support for the role of microbial community composition in influencing sediment CH4 and CO2 production (Torres et al., 2011; Ji et al., 2016; Bertolet et al., 2019; Xia et al., 2020; Yang et al., 2020). For example, in north temperate lakes, community composition of the non-methanogen community was significantly related to lake CH4 production after accounting for variation in primary productivity across 14 lake sediments (Bertolet et al., 2019). Additionally, Torres et al. (2011) showed that heterotrophic activity was related to microbial biomass in three lake sediments that differed in trophic status. However, few studies have experimentally tested the influence of microbial community composition on sediment function or considered how microbial community composition may interact with changing OM conditions to influence sediment function.
Here, we test the hypothesis that microbial community composition influences the response of lake CH4 production to autochthonous OM. To do so, we designed sediment slurry incubation experiments with variable microbial community composition and OM supply. To capture variation in microbial community composition, we conducted incubations across 22 lake sediments, chosen along a pH gradient (range: 3.9–7.4) at the sediment-water interface, which has been previously shown as an environmental determinant of microbial community composition (Bertolet et al., 2019). We predicted that metrics of both microbial community composition and abundance would be correlated with the magnitude of the response of CH4 production to OM additions. Our experimental design allows for a mechanistic understanding of the role of microbial communities in sediment carbon processing and thus could help to predict future CH4 production using quantitative metrics of sediment microbial communities.
2 Methods and Materials
2.1 Experimental Design
Lakes were located in northern Wisconsin, United States. We sampled 13 lakes between June and August 2019 and an additional 9 lakes between July and August 2020 (Table 1). During each sampling season, the sampled lakes represented the full range of the pH gradient. To construct sediment slurry incubations, we sampled sediment and hypolimnetic water from the deepest point of every lake. Sediment was collected from the top 15 cm of the sediment surface with an Eckman dredge and hypolimnetic water was collected from 0.25 m above the sediment surface with a Van Dorn water sampler. There was some degree of atmospheric oxygen exposure during sampling for both hypolimnion water samples and sediment samples, however we attempted to mitigate the length of exposure. Hypolimnion water samples were sealed immediately after collection the field. Sediment samples were collected in 5-gallon open-air buckets. Upon return to the laboratory, sediment was sampled from the bottom of the bucket for construction of sediment incubations and construction occurred within 5 h of collection. Prior to construction, all samples were stored in the dark at 4°C.
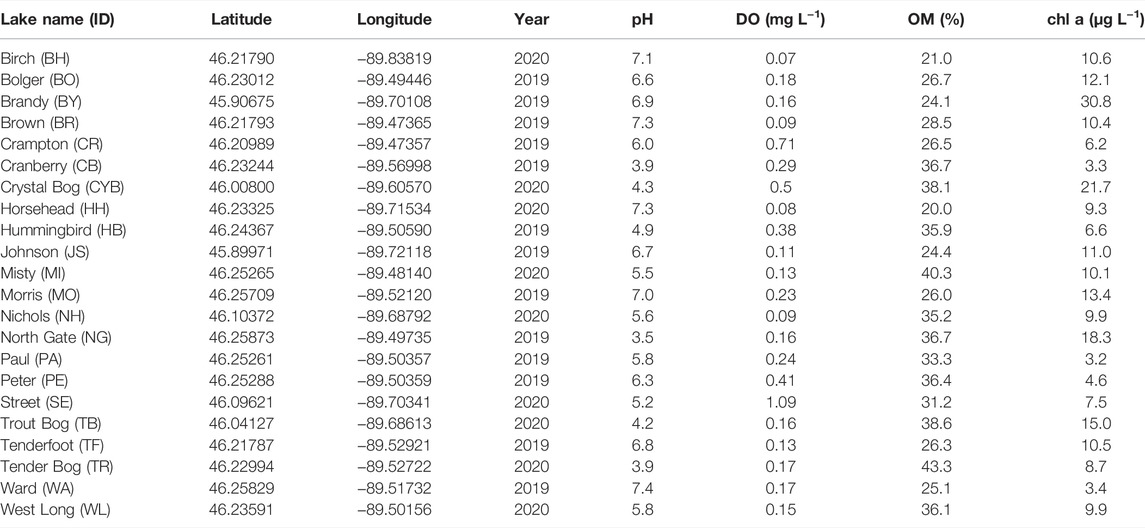
TABLE 1. Summary of environmental variables of sampled lakes, including pH and dissolved oxygen (DO) concentration at the sediment-water interface, sediment organic matter content (OM %), and epilimnion chlorophyll a concentration (chl a).
We constructed experimental sediment incubations in 300 ml glass serum bottles containing 50 ml of sediment and 50 ml of anoxic hypolimnion water. To quantify the difference in CH4 production under increased OM supply, we conducted triplicate incubations with and without additions of OM. For incubations treated with the addition, we added 20 mg of dried algal biomass (Scenedesmus obliquus). S. obliquus was grown in Bold’s Basal Medium under constant aeration with atmospheric carbon dioxide concentrations. To generate a homogeneous dried material for additions, we centrifuged the wet material in 40 ml batches at 4,000 rpm for 10 minutes, extracted the pellet, and dried the pellet for 7 days. All dried material was added to a single vial and homogenized before addition to incubations. After additions, we capped and sealed the serum bottles with rubber septa and aluminum crimp seals and homogenized the sediment slurries by manual shaking for 1 min. We then purged the headspace of each incubation with N2 gas for 5 min to maintain anoxic conditions. We incubated the bottles in the dark at 4°C to simulate conditions at the bottom of a lake and we incubated the bottles for approximately 28 days.
2.2 Net Methane Production
During the length of the incubation period, we estimated net CH4 production rates from each incubation by iteratively sampling from the headspace for CH4 concentration. The first sampling occurred 24 h after purging the headspace and sealing the incubations. We then sampled every 3–5 days, as well as at end of incubation (approximately 28 days), for a minimum of 4 sampling points for each incubation (Supplementary Figure S1). To sample for CH4 concentration, we extracted 10 ml of headspace gas using a 25-gauge needle pierced through the rubber septa. Samples were immediately injected into airtight glass vials for measurement of CH4 concentration on an Agilent 6890 Gas Chromatograph with parameters previously described in West et al. (2016). We then added 10 ml of N2 back to the headspace to maintain pressure. We inferred net rates of CH4 production, after accounting for the headspace dilution, from the slope of a linear regression fit to the time-series (Supplementary Figure S1). For incubations in which CH4 did not accumulate over time (N = 4), we assumed a net CH4 production of zero.
2.3 Microbial Community Composition and Abundance
During the initial collection of lake sediments for construction of incubations, we sampled approximately 1 g of sediment for analysis of microbial community composition and abundance. Sediments were stored in sterile plastic 1.5 ml cryovial tubes at −80°C until DNA extraction. We conducted a single DNA extraction for each lake using 0.25 g of sediment and the DNeasy PowerSoil Pro kit (Qiagen, Hilden, DE) according to manufacturer instructions. The extracted DNA was used as template for 16S rRNA paired-end gene sequencing to determine differences in community composition. Extracted DNA was also used as template in digital droplet polymerase chain reactions (ddPCR) of the 16S rRNA gene and the alpha subunit of methyl coenzyme reductase (mcrA) gene to determine total bacterial + archaeal abundance and methanogen abundance, respectively.
For 16S rRNA paired-end gene sequencing, the V4 region of the 16S rRNA gene was amplified in a 15 μl PCR reaction with dual indexed primers: 515F (5′-GTGCCAGCMGCCGCGGTAA-3′) and 806R (5′-GGACTACHVGGGTWTCTAAT- 3′) (Kozich et al., 2013). Amplified DNA served as template for high-throughput paired-end (2 × 250 bp) sequencing on an Illumina MiSeq v2 Standard flow cell at the Michigan State University Research Technology Support Facility. Sequencing resulted in 1,279,135 high-quality reads across all lake samples. Raw sequence reads are available at the NCBI Sequence Read Archive (Accession # PRJNA813558). To determine differences in microbial community composition, we used the mothur (version 1.44.3) bioinformatics pipeline for merging of paired-end reads, quality filtering, clustering, and taxonomic classification (Kozich et al., 2013). To determine community membership, operational taxonomic units (OTUs) were defined at 97% similarity using the dist.seqs command and a 0.03 cutoff. Representative sequences for each OTU were aligned and classified against the RDP classifier database (version 18) (Wang et al., 2007).
To quantify abundance of the 16S rRNA gene and mcrA gene, we conducted ddPCR using the BioRad QX200 Droplet Digital PCR system. The indicator genes were amplified in separate 20 μl ddPCR reactions using a BioRad C1000 Touch thermocycler with EvaGreen as the reporting dye. For amplification of the 16S rRNA gene, each reaction contained 2 μl of 1/100 diluted sediment DNA template, 1X Q200 ddPCR EvaGreen Supermix, and 0.1 μM of each primer targeting 16S rRNA: 338F (5′-ACTCCTACGGGAGGCAG-3′) and 805R (5′-GACTACCAGGGTATCTAATC- 3′) (Yu et al., 2005). For amplification of the mcrA gene, each reaction contained 1 μL of 1/10 diluted sediment DNA template, 1X Q200 ddPCR EvaGreen Supermix, and 0.25 μM of each primer targeting mcrA: mcrAqF (5′-AYGGTATGGARCAGTACGA- 3′) and mcrAqR (5′-TGVAGRTCGTABCCGWAGAA-3′) (West et al., 2012). Thermocycling conditions for both indicator genes are reported in Supplementary Table S1. Additionally, for both assays, we performed three technical replicates on a subset of the samples to verify precision. Technical replicates indicated high precision of the assays (Supplementary Figure S2), and we normalized copy numbers to copies per gram of sediment to be comparable across samples.
2.4 Lake Environmental Variables
During sampling for construction of incubations, we measured dissolved oxygen concentration and pH at the sediment-water interface using a YSI Professional Plus Multiparameter meter (Yellow Springs Instruments, Yellow Springs, OH, United States). We also collected additional sediment to quantify sediment OM content and epilimnion water for quantification of water column chlorophyll a (chl a) concentrations, as metrics of differences in OM availability and lake trophic status. We quantified percent OM of sediment using loss on ignition measurements of dried sediment samples (Heiri et al., 2001). To quantify water column chl a, we used particles from 450 ml of the epilimnion sample captured on a 0.7 μm glass fiber filter and we analyzed the sample using methanol extraction and fluorometry (Welschmeyer, 1994).
2.5 Statistical Analyses
We conducted all statistical analyses in R (R Core Team, 2018) using the base and vegan (Oksanen et al., 2017) packages. To quantify variation in sediment microbial community composition across the 22 lakes, we first rarified every sample to 39,948 sequence reads to be comparable across sample sequencing depths. We then identified methanogen OTUs by the presence of “Methano” in taxonomic assignments, as a conservative means of identifying methanogens, and manually curated our list of putative methanogen genera for known methanogen taxa. Some methanogenic microbes were likely not identified based on this method (Kharitonov et al., 2021), but our approach did allow for estimation of relative sequence recovery of known methanogenic groups. We then conducted the following analyses for both the methanogen and non-methanogen community.
To quantify variation in microbial community composition across the 22 lakes, we standardized OTU matrices of both communities to reflect relative sequence recovery and generated pairwise Bray-Curtis dissimilarity matrices of each community using the vegdist function. We then generated principal coordinates for each community based on the Bray-Curtis dissimilarity matrices using the cmdscale function. The first principal coordinate axes (PCoA 1) represent 40.6 % and 29.2% of the variation in the methanogen and non-methanogen community, respectively. We also tested for effects of environmental conditions on community composition using permutational multivariate ANOVA (PERMANOVA) tests using the adonis function in R (Anderson, 2001). We evaluated significance of the PERMANOVA statistic using 1000 permutations of the dissimilarity matrices for each community. To quantify differences in microbial community diversity and richness, we also calculated the Shannon Diversity Index and community richness, or the sum of OTUs present in each sample. Thus, we used the Shannon Index, community richness, and the PCoA 1 scores for both communities as metrics of community composition in subsequent analyses.
To quantify the response of sediment CH4 production to the OM additions across the 22 lakes, we calculated the difference in mean net CH4 production between incubations with and without OM additions (∆CH4 production) for every lake. We also quantified the maximum percent utilization of OM additions by calculating the total CH4 produced through the length of the experiment divided by the amount of OM supplied. For the dried autochthonous OM supplied, we assumed a carbon content of 61.5% based on measurements from West et al. (2015). Finally, we determined significant correlations between the log-transformed ∆CH4 production and metrics of microbial community composition and abundance using simple linear regressions. We used the log-transformation to abide by assumptions of normally distributed data. We also determined the correlation between log-transformed ∆CH4 production and metrics of preexisting OM supply (sediment OM %, and water column chl a concentration). Data, metadata, and code for all analyses are available on GitHub at https://github.com/brittnibertolet/CH4response2OM.
3 Results
3.1 Microbial Community Composition and Abundance
Sampling along a gradient of pH resulted in sediment incubations with variable microbial communities. After rarifying, sequencing recovered 367 methanogen OTUs and 26,494 non-methanogen OTUs. Methanogen reads represented 1.5% of the total sequence reads and the relative sequencing reads of methanogens ranged from 0.7 % to 2.8% across all lakes. As hypothesized from previous work (Bertolet et al., 2019), the composition of both the methanogen and non-methanogen community was significantly related to pH and sediment OM (Figure 1). As such, PCoA Axis 1 of both communities was significantly correlated with pH (methanogen: R2 = 0.73, p < 0.001, non-methanogen: R2 = 0.69, p < 0.001) and OM (methanogen: R2 = 0.71, p < 0.001, non-methanogen R2 = 0.76, p < 0.001). Additionally, the relative abundances of different methanogen genera (Supplementary Figure S2) and non-methanogen phyla (Supplementary Figure S3) varied across lakes.
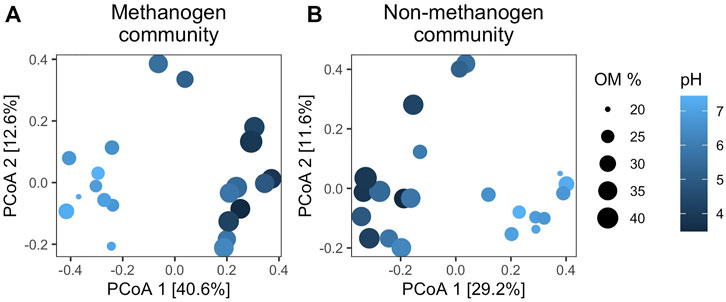
FIGURE 1. First two principal coordinates of the methanogen community composition (A) and the non-methanogen community composition (B). Permutational analysis of variance indicated that methanogen community composition was significantly related to pH (F22,1 = 9.24, p = 0.001) and sediment organic matter content (F22,1 = 8.58, p = 0.001). Similarly, non-methanogen community composition was also significantly related to (F22,1 = 5.77, p = 0.001) and sediment organic matter content (F22,1 = 6.01, p = 0.001).
Microbial richness and diversity varied significantly across the lake samples as well as between the methanogen and non-methanogen communities. Methanogen richness ranged from 13 to 60 OTUs, while non-methanogen richness ranged from 1,404 to 6,059 OTUs. Methanogen Shannon Index ranged from 1.7 to 2.8, while non-methanogen ranged from 4.9 to 7.3. Additionally, neither methanogen richness nor diversity were significantly correlated with environmental variables (pH, sediment OM %, or chl a concentration). In contrast, non-methanogen richness and Shannon Index were both significantly correlated with both pH and sediment OM % (Supplementary Table S2).
Digital droplet PCR of the 16S rRNA and mcrA genes also indicated variation in the total bacterial + archaeal abundance and methanogen abundance across lakes (Supplementary Figure S4). Total bacterial + archaeal abundance ranged from 3.07 × 105 to 1.22 × 108 copies per gram wet sediment. However, total bacterial + archaeal abundance was not related to sediment pH nor sediment OM %. Instead, total bacterial + archaeal abundance was significantly correlated with water column chl a concentration (R2 = 0.24, p < 0.05; Supplementary Figure S5). Similarly, methanogen abundance ranged from 3.31 × 105 to 1.79 × 107 copies per gram wet sediment and was significantly correlated with 16S rRNA copy number (R2 = 0.26, p < 0.05) and water column chl a concentration (R2 = 0.19, p < 0.05; Supplementary Figure S4). However, the relative sequence reads of methanogens, derived from the 16S rRNA Illumina sequencing, was not related to ddPCR estimates of microbial abundance nor chl a concentration. Additionally, neither differences in community composition nor abundance were related to sample year (Supplementary Table S4).
3.2 Methane Production Responses to Organic Matter Additions
Rates of CH4 production were variable across lakes and were significantly influenced by autochthonous OM additions. OM additions increased the net CH4 production rate across all lakes (Kruskal-Wallis test: χ2 = 73.2, p < 0.001; Figure 2). However, the magnitude of the response (∆CH4 production) varied and ranged from 1.1 to 27.3 μmol CH4 L sediment slurry−1 day−1. Additionally, maximum potential utilization of OM ranged from 0.28 % to 7.1% of algal carbon added to a slurry.
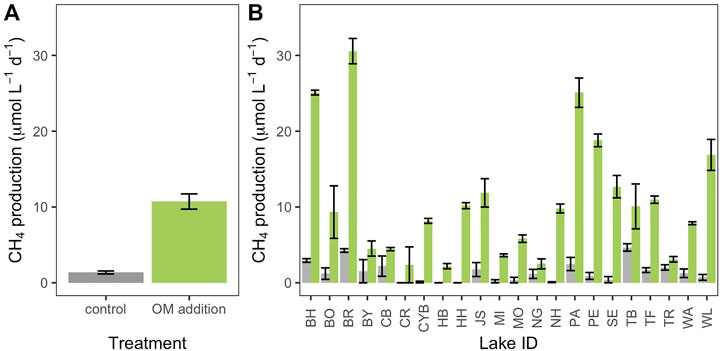
FIGURE 2. Net methane (CH4) production rates of sediment slurry incubations across organic matter (OM) additions (grey: control; green: OM addition). OM additions significantly increased the net CH4 production rate across all lakes ((A); KruskalWallis test: χ2 = 73.2, p < 0.001). However, the magnitude of increase was variable across lakes (B). Error bars indicate one standard error.
Variation in the ∆CH4 production was related to differences in both metrics of sediment microbial community composition and pH across the sampled lake sediments (Figure 3). Specifically, the Shannon Diversity Index and richness of the non-methanogen explained the most amount of variation in ∆CH4 production, and pH and metrics of methanogen community composition were also significantly correlated with the log-transformed ∆CH4 production (Table 2). However, neither abundance metrics nor environmental variables related to OM supply (sediment OM % or water column chl a) explained variation in ∆CH4 production, despite significant influences of sediment OM % on both methanogen and non-methanogen community composition (Figure 1). Additionally, the sample year did not influence patterns ∆CH4 production (Supplementary Table S2).
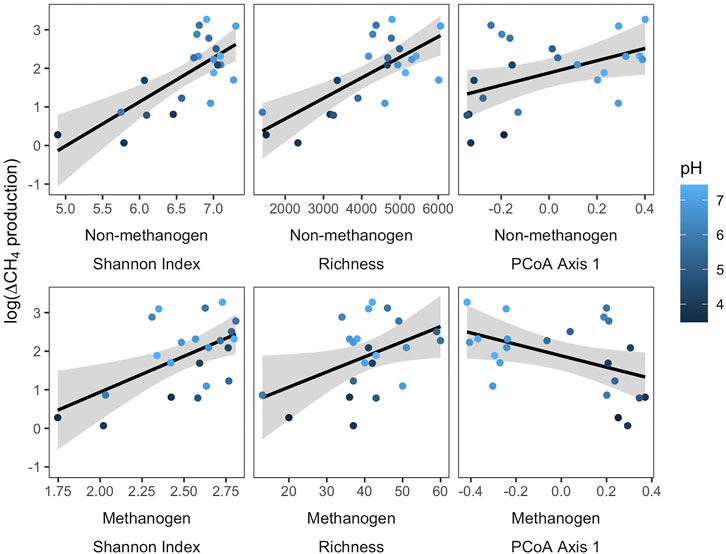
FIGURE 3. Correlations between the log-transformed difference in net methane production amongst organic matter additions (∆CH4 production) and metrics of microbial community composition. Statistics are reported in Table 2.
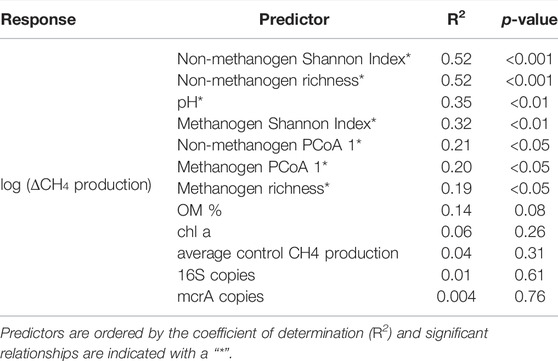
TABLE 2. Summary statistics of linear regressions between the log-transformed difference in net CH4 production between organic matter additions (ΔCH4 production) and environmental and microbial predictors.
4 Discussion
Lake CH4 production and emission are consistently positively related to lake primary productivity and trophic status, but, in both laboratory and field scenarios, there exists large variation in the magnitude of the temporal response of CH4 dynamics to increases in OM (West et al., 2015; Yakimovich et al., 2018; Bertolet et al., 2020; Yang et al., 2020). Understanding sources of this variation is essential for making quantitative predictions under future environmental change scenarios (DelSontro et al., 2018). In the present study, we show that variation in the response of lake CH4 production to increases in OM is correlated with pH-mediated differences in sediment microbial community composition (Figure 3). Specifically, metrics of the non-methanogen community composition were the best predictors of CH4 production responses to increasing OM, especially as compared to the methanogen community or other variables hypothesized to influence CH4 responses to OM, such as preexisting OM availability and metrics of microbial abundance (Table 2). By comparing multiple metrics of community composition, as well as specifically investigating both the methanogen and non-methanogen community, we provide critical evidence of how lake sediment responses to environmental change may be improved by incorporating variation in microbial community composition.
A growing body of literature describes the links between lake sediment microbial community composition and lake pH and OM content (Kotsyurbenko et al., 2004; Bertolet et al., 2019; Orland et al., 2020). We reproduce established patterns in lake sediment microbial community assembly, in which the methanogen and non-methanogen communities are differentiated across pH and sediment OM% (Figure 1). Further, as previous research has established that lakes have variable CH4 production responses to changes in OM (West et al., 2015; Grasset et al., 2018), we used this gradient of pH to test the hypothesis that sediment microbial community composition influences the effect size of the CH4 production and OM relationship.
In our study, there was substantial variation in the CH4 production response to OM additions (Figure 2). The difference in net CH4 production between incubations with and without additions of OM (∆CH4 production) ranged from 1.1 to 27.3 μmol CH4 L sediment slurry−1 day−1. As we hypothesized, variation in ∆CH4 production was significantly correlated with pH-mediated differences in microbial community diversity, richness, and composition (Table 2). Specifically, lakes with microbial communities associated with higher pH environments had greater CH4 production responses to additions in OM (Figure 3). While it is difficult to disentangle the effects of pH and microbial community composition on ∆CH4 production, previous research does suggest that pH structures the differences in microbial community composition across lakes (Xiong et al., 2012; Bertolet et al., 2019; Orland et al., 2020) and that the mechanistic relationship between pH and CH4 production relies on pH effects on competitive interactions between methanogens and other fermenting bacteria (Phelps and Zeikus, 1984; Ye et al., 2012). Additionally, in a replicated common garden experiment with contrasting pH environments, microbial community composition influenced rates of CH4 and CO2 production independent of the pH environment (Bertolet et al., in press).
Our results suggest that relationships between microbial community composition and pH may be used to predict the response of sediment CH4 production to changes in OM. Specifically, information about variation in the non-methanogen community was most predictive of function, and so we suggest that syntrophic interactions, or the supply of substrate by one group to another, between microbial functional groups in lake sediments are likely an important control on rates of CH4 production. Production of methanogenesis precursors has previously been shown to be limiting (Kotsyurbenko et al., 2004) and methanogens are reliant on heterotrophic and acetogenic bacteria for the conversion of complex organic material into substrates that can be utilized (Liu and Whitman, 2008). However, we still lack the ability to identify the functional capacity of various microbial groups, so quantifying these interactions remains difficult.
Further research is needed to identify how variation in microbial community diversity and composition relates to functional diversity within the microbial community, and how interactions between members may lead to differences in emergent function. Currently, few OTUs in our dataset can be functionally identified based on taxonomy or other means. Metagenomic analyses have made significant strides in functionally defining sediment microorganisms (Vavourakis et al., 2018; Rathour et al., 2020), however few studies have measured ecosystem function in conjunction with metagenomic characterization or identified statistical relationships that can be used for prediction. Additionally, measurements of microbial metabolic traits, either in situ or in laboratory, and at both population and community scales, would greatly improve our ability to differentiate important microbial function groups and incorporate their variation across space and time into understanding of ecosystem function.
In the absence of the ability to constrain microbial functional groups, we suggest that aggregated metrics of microbial community composition, such as diversity metrics, richness, and composition scores, may be used to distinguish possible sediment responses to environmental change. Interestingly, diversity and richness metrics explained more variation than composition scores, indicating that evenness of composition is potentially less informative than richness. This approach to establishing links between microbial community diversity and ecosystem function that uses OTU richness and diversity is supported by other studies that suggest that taxonomically resolved information of microbial communities is not predictive of sediment function (Ji et al., 2016; Orland et al., 2020), and which highlight the need to move beyond taxonomy in order to identify metrics of functional groups that can be used in predictive models (Hall et al., 2018). Additionally, we also find that estimates of microbial abundance from ddPCR were not related to differences in ∆CH4 production (Table 2). This is consistent with previous research indicating that changes in sediment CH4 production due to changes in OM supply are likely due to changes in per cell activity and not changes in abundance (West et al., 2012).
It is worth noting that we also tested for effects of preexisting OM conditions on ∆CH4 production, as previous work has hypothesized that temporal response of lake CH4 production to eutrophication may be related to current or historical trophic state (Bertolet et al., 2020; Yang et al., 2020). However, we found that there were no significant correlations between the strength of the response and metrics of preexisting OM availability (sediment OM % and water column chl a; Table 2). Further, we observed no evidence of a priming effect, as the maximum potential utilization of the algal supplied carbon never exceeded even 10%. In temperate lakes, sediment carbon pools are highly recalcitrant and primarily consisting of allochthonous derived material (Sobek et al., 2009), which may decouple the effect of preexisting OM availability from current responses to incoming autochthonous OM.
By conducting experimental manipulations of 22 lake sediments, we provide a direct line of evidence supporting links between lake pH, sediment microbial community composition, lake trophic status, and sediment CH4 production. Specifically, we see that microbial community composition and pH are correlated and positively predict the response of lake CH4 production to increases in OM. Previous research has begun to highlight the importance of incorporating variation in sediment microbial communities into ecosystem conceptual and predictive models (Graham et al., 2016; Hall et al., 2018), and, as the global eutrophication of inland freshwater ecosystems is likely to increase CH4 production (DelSontro et al., 2018; Beaulieu et al., 2019), understanding sources of variation in the magnitude of change is thus critical for predicting future lake carbon cycling. We encourage further research that identifies important microbial functional groups and that utilizes relationships between the environment, microbial community composition, and ecosystem function to understand and predict variation in lake CH4 production and emission.
Data Availability Statement
Data and metadata presented in the study are deposited in the Zenodo repository: https://doi.org/10.5281/zenodo.6368660. Raw sequence reads are available at the NCBI Sequence Read Archive (Accession # PRJNA813558).
Author Contributions
BB and SJ developed research design and hypotheses. BB collected field data and conducted experiments with guidance from SJ. CK and BB conducted molecular analyses. BB conducted statistical analyses with guidance from SJ and CK. BB wrote initial manuscript. All authors contributed to data interpretation, provided written feedback, and approved the final version.
Funding
This research was supported by the National Science Foundation under Grants 1754363, 1754561, 1547866, and 2109350.
Conflict of Interest
The authors declare that the research was conducted in the absence of any commercial or financial relationships that could be construed as a potential conflict of interest.
Publisher’s Note
All claims expressed in this article are solely those of the authors and do not necessarily represent those of their affiliated organizations, or those of the publisher, the editors and the reviewers. Any product that may be evaluated in this article, or claim that may be made by its manufacturer, is not guaranteed or endorsed by the publisher.
Acknowledgments
We thank Sydney Louden, Gabriella Marines-Chio, Carly Olson, Randi Notte, and Ceara Talbot for assisting in field data collection. The University of Notre Dame Environmental Research Center provided logistic support.
Supplementary Material
The Supplementary Material for this article can be found online at: https://www.frontiersin.org/articles/10.3389/fenvs.2022.834829/full#supplementary-material
References
Anderson, M. J. (2001). A New Method for Non-Parametric Multivariate Analysis of Variance. Austral Ecol. 26 (1), 32–46. doi:10.1111/j.1442-9993.2001.01070.pp.x
Bastviken, D., Tranvik, L. J., Downing, J. A., Crill, P. M., and Enrich-Prast, A. (2011). Freshwater Methane Emissions Offset the Continental Carbon Sink. Science 331 (6013), 50. doi:10.1126/science.1196808
Beaulieu, J. J., DelSontro, T., and Downing, J. A. (2019). Eutrophication Will Increase Methane Emissions from Lakes and Impoundments during the 21st century. Nat. Commun. 10, 1375. doi:10.1038/s41467-019-09100-5
Bertolet, B. L., Olson, C. R., Szydlowski, D. K., Solomon, C. T., and Jones, S. E. (2020). Methane and Primary Productivity in Lakes: Divergence of Temporal and Spatial Relationships. J. Geophys. Res. Biogeosci. 125 (9), e2020JG005864. doi:10.1029/2020JG005864
Bertolet, B. L., West, W. E., Armitage, D. W., and Jones, S. E. (2019). Organic Matter Supply and Bacterial Community Composition Predict Methanogenesis Rates in Temperate lake Sediments. Limnol Oceanogr Lett. 4 (5), 164–172. doi:10.1002/lol2.10114
Davidson, T. A., Audet, J., Jeppesen, E., Landkildehus, F., Lauridsen, T. L., Søndergaard, M., et al. (2018). Synergy between Nutrients and Warming Enhances Methane Ebullition from Experimental Lakes. Nat. Clim Change 8, 156–160. doi:10.1038/s41558-017-0063-z
de Jong, A. E. E., in ’t Zandt, M. H., Meisel, O. H., Jetten, M. S. M., Dean, J. F., Rasigraf, O., et al. (2018). Increases in Temperature and Nutrient Availability Positively Affect Methane‐Cycling Microorganisms in Arctic Thermokarst lake Sediments. Environ. Microbiol. 20 (12), 4314–4327. doi:10.1111/1462-2920.14345
Deemer, B. R., Harrison, J. A., Li, S., Beaulieu, J. J., DelSontro, T., Barros, N., et al. (2016). Greenhouse Gas Emissions from Reservoir Water Surfaces: A New Global Synthesis. BioScience 66, 949–964. doi:10.1093/biosci/biw117
DelSontro, T., Beaulieu, J. J., and Downing, J. A. (2018). Greenhouse Gas Emissions from Lakes and Impoundments: Upscaling in the Face of Global Change. Limnol Oceanogr Lett. 3, 64–75. doi:10.1002/lol2.10073
DelSontro, T., Boutet, L., St-Pierre, A., del Giorgio, P. A., and Prairie, Y. T. (2016). Methane Ebullition and Diffusion from Northern Ponds and Lakes Regulated by the Interaction between Temperature and System Productivity. Limnol. Oceanogr. 61, S62–S77. doi:10.1002/lno.10335
Duc, N. T., Crill, P., and Bastviken, D. (2010). Implications of Temperature and Sediment Characteristics on Methane Formation and Oxidation in lake Sediments. Biogeochemistry 100, 185–196. doi:10.1007/s10533-010-9415-8
Fallon, R. D., Harrits, S., Hanson, R. S., and Brock, T. D. (1980). The Role of Methane in Internal Carbon Cycling in Lake Mendota during Summer Stratification. Limnol. Oceanogr. 25 (2), 357–360. doi:10.4319/lo.1980.25.2.0357
Foley, B., Jones, I. D., Maberly, S. C., and Rippey, B. (2012). Long-Term Changes in Oxygen Depletion in a Small Temperate lake: Effects of Climate Change and Eutrophication. Freshw. Biol. 57, 278–289. doi:10.1111/j.1365-2427.2011.02662.x
Ford, P. W., Boon, P. I., and Lee, K. (2002). Methane and Oxygen Dynamics in a Shallow Floodplain Lake: The Significance of Periodic Stratification. Hydrobiologia 485, 97–110. doi:10.1023/a:1021379532665
Graham, E. B., Knelman, J. E., Schindlbacher, A., Siciliano, S., Breulmann, M., Yannarell, A., et al. (2016). Microbes as Engines of Ecosystem Function: When Does Community Structure Enhance Predictions of Ecosystem Processes? Front. Microbiol. 7, 214. doi:10.3389/fmicb.2016.00214
Grasset, C., Mendonça, R., Villamor Saucedo, G., Bastviken, D., Roland, F., and Sobek, S. (2018). Large but Variable Methane Production in Anoxic Freshwater Sediment upon Addition of Allochthonous and Autochthonous Organic Matter. Limnol. Oceanogr. 63, 1488–1501. doi:10.1002/lno.10786
Guenet, B., Neill, C., Bardoux, G., and Abbadie, L. (2010). Is There a Linear Relationship between Priming Effect Intensity and the Amount of Organic Matter Input? Appl. Soil Ecol. 46, 436–442. doi:10.1016/j.apsoil.2010.09.006
Hall, E. K., Bernhardt, E. S., Bier, R. L., Bradford, M. A., Boot, C. M., Cotner, J. B., et al. (2018). Understanding How Microbiomes Influence the Systems They Inhabit. Nat. Microbiol. 3, 977–982. doi:10.1038/s41564-018-0201-z
Heiri, O., Lotter, A. F., and Lemcke, G. (2001). Loss on Ignition as a Method for Estimating Organic and Carbonate Content in Sediments: Reproducibility and Comparability of Results. J. Paleolimnology 25, 101–110. doi:10.1023/a:1008119611481
Ji, Y., Angel, R., Klose, M., Claus, P., Marotta, H., Pinho, L., et al. (2016). Structure and Function of Methanogenic Microbial Communities in Sediments of Amazonian Lakes with Different Water Types. Environ. Microbiol. 18 (12), 5082–5100. doi:10.1111/1462-2920.13491
Kharitonov, S., Semenov, M., Sabrekov, A., Kotsyurbenko, O., Zhelezova, A., and Schegolkova, N. (2021). Microbial Communities in Methane Cycle: Modern Molecular Methods Gain Insights into Their Global Ecology. Environments 8, 16. doi:10.3390/environments8020016
Kotsyurbenko, O. R., Chin, K.-J., Glagolev, M. V., Stubner, S., Simankova, M. V., Nozhevnikova, A. N., et al. (2004). Acetoclastic and Hydrogenotrophic Methane Production and Methanogenic Populations in an Acidic West-Siberian Peat Bog. Environ. Microbiol. 6 (11), 1159–1173. doi:10.1111/j.1462-2920.2004.00634.x
Kozich, J. J., Westcott, S. L., Baxter, N. T., Highlander, S. K., and Schloss, P. D. (2013). Development of a Dual-Index Sequencing Strategy and Curation Pipeline for Analyzing Amplicon Sequence Data on the MiSeq Illumina Sequencing Platform. Appl. Environ. Microbiol. 79, 5112–5120. doi:10.1128/AEM.01043-13
Liu, Y., and Whitman, W. B. (2008). Metabolic, Phylogenetic, and Ecological Diversity of the Methanogenic Archaea. Annu. N.Y Acad. Sci. 1125, 171–189. doi:10.1196/annals.1419.019
Müller, B., Bryant, L. D., Matzinger, A., and Wüest, A. (2012). Hypolimnetic Oxygen Depletion in Eutrophic Lakes. Environ. Sci. Technol. 46, 9964–9971. doi:10.1021/es301422r
Nozhevnikova, A. N., Nekrasova, V., Ammann, A., Zehnder, A. J. B., Wehrli, B., and Holliger, C. (2007). Influence of Temperature and High Acetate Concentrations on Methanogenensis in lake Sediment Slurries. FEMS Microbiol. Ecol. 62, 336–344. doi:10.1111/j.1574-6941.2007.00389.x
Oksanen, J., Guillaume Blanchet, F., Friendly, M., Kindt, R., Legendre, P., McGlinn, D., et al. (2017). Vegan: Community Ecology Package. R package version 2.4-2. Available at: https://CRAN.R-project.org/package=vegan (Accessed November 18, 2021).
Orland, C., Yakimovich, K. M., Mykytczuk, N. C. S., Basiliko, N., and Tanentzap, A. J. (2020). Think Global, Act Local: The Small‐Scale Environment Mainly Influences Microbial Community Development and Function in lake Sediment. Limnol Oceanogr 65, S88–S100. doi:10.1002/lno.11370
Phelps, T. J., and Zeikus, J. G. (1984). Influence of pH on Terminal Carbon Metabolism in Anoxic Sediments from a Mildly Acidic lake. Appl. Environ. Microbiol. 48, 1088–1095. doi:10.1128/aem.48.6.1088-1095.1984
R Core Team (2018). R: A Language and Environment for Statistical Computing. Vienna, Austria: R Foundation for Statistical Computing. URL https://www.R-project.org/ (Accessed November 18, 2021).
Rathour, R., Gupta, J., Mishra, A., Rajeev, A. C., Dupont, C. L., and Thakur, I. S. (2020). A Comparative Metagenomic Study Reveals Microbial Diversity and Their Role in the Biogeochemical Cycling of Pangong lake. Sci. Total Environ. 731, 139074. doi:10.1016/j.scitotenv.2020.139074
Sepulveda-Jauregui, A., Hoyos-Santillan, J., Martinez-Cruz, K., Walter Anthony, K. M., Casper, P., Belmonte-Izquierdo, Y., et al. (2018). Eutrophication Exacerbates the Impact of Climate Warming on lake Methane Emission. Sci. Total Environ. 636, 411–419. doi:10.1016/j.scitotenv.2018.04.283
Sobek, S., Durisch-Kaiser, E., Zurbrügg, R., Wongfun, N., Wessels, M., Pasche, N., et al. (2009). Organic Carbon Burial Efficiency in Lake Sediments Controlled by Oxygen Exposure Time and Sediment Source. Limnol. Oceanogr. 54 (6), 2243–2254. doi:10.4319/lo.2009.54.6.2243
Steger, K., Premke, K., Gudasz, C., Sundh, I., and Tranvik, L. J. (2011). Microbial Biomass and Community Composition in Boreal lake Sediments. Limnol. Oceanogr. 56 (2), 725–733. doi:10.4319/lo.2011.56.2.0725
Torres, I. C., Inglett, K. S., and Reddy, K. R. (2011). Heterotrophic Microbial Activity in Lake Sediments: Effects of Organic Electron Donors. Biogeochemistry 104, 165–181. doi:10.1007/s10533-010-9494-6
Vavourakis, C. D., Andrei, A.-S., Mehrshad, M., Ghai, R., Sorokin, D. Y., and Muyzer, G. (2018). A Metagenomics Roadmap to the Uncultured Genome Diversity in Hypersaline Soda lake Sediments. Microbiome 6, 168. doi:10.1186/s40168-018-0548-7
Wang, Q., Garrity, G. M., Tiedje, J. M., and Cole, J. R. (2007). Naïve Bayesian Classifier for Rapid Assignment of rRNA Sequences into the New Bacterial Taxonomy. Appl. Environ. Microbiol. 73 (16), 5261–5267. doi:10.1128/AEM.00062-07
Wang, W., YujunYi, Y., Yang, Y., Zhou, Y., Zhang, S., Wang, X., et al. (2020). Impact of Anthropogenic Activities on the Sediment Microbial Communities of Baiyangdian Shallow Lake. Int. J. Sediment Res. 35, 180–192. doi:10.1016/j.ijsrc.2019.10.006
Welschmeyer, N. A. (1994). Fluorometric Analysis of Chlorophyll a in the Presence of Chlorophyll B and Pheopigments. Limnol. Oceanogr. 39 (8), 1985–1992. doi:10.4319/lo.1994.39.8.1985
West, W. E., Coloso, J. J., and Jones, S. E. (2012). Effects of Algal and Terrestrial Carbon on Methane Production Rates and Methanogen Community Structure in a Temperate Lake Sediment. Freshw. Biol. 57, 949–955. doi:10.1111/j.1365-2427.2012.02755.x
West, W. E., Creamer, K. P., and Jones, S. E. (2016). Productivity and Depth Regulate Lake Contributions to Atmospheric Methane. Limnol. Oceanogr. 61, S51–S61. doi:10.1002/lno.10247
West, W. E., McCarthy, S. M., and Jones, S. E. (2015). Phytoplankton Lipid Content Influences Freshwater Lake Methanogenesis. Freshw. Biol. 60, 2261–2269. doi:10.1111/fwb.12652
Xia, P., Zhang, J., Liu, J., and Yu, L. (2020). Shifts of Sediment Bacterial Community and Respiration along a Successional Gradient in a Typical Karst Plateau Lake Wetland (China). J. Ocean. Limnol. 39, 880–891. doi:10.1007/s00343-020-0073-y
Xiong, J., Liu, Y., Lin, X., Zhang, H., Zeng, J., Hou, J., et al. (2012). Geographic Distance and pH Drive Bacterial Distribution in Alkaline lake Sediments across Tibetan Plateau. Environ. Microbiol. 14 (9), 2457–2466. doi:10.1111/j.1462-2920.2012.02799.x
Yakimovich, K. M., Emilson, E. J. S., Carson, M. A., Tanentzap, A. J., Basiliko, N., and Mykytczuk, N. C. S. (2018). Plant Litter Type Dictates Microbial Communities Responsible for Greenhouse Gas Production in Amended Lake Sediments. Front. Microbiol. 9, 2662. doi:10.3389/fmicb.2018.02662
Yang, Y., Chen, J., Tong, T., Xie, S., and Liu, Y. (2020). Influences of Eutrophication on Methanogenesis Pathways and Methanogenic Microbial Community Structures in Freshwater Lakes. Environ. Pollut. 260, 114106. doi:10.1016/j.envpol.2020.114106
Ye, R., Jin, Q., Bohannan, B., Keller, J. K., McAllister, S. A., and Bridgham, S. D. (2012). pH Controls over Anaerobic Carbon Mineralization, the Efficiency of Methane Production, and Methanogenic Pathways in Peatlands across an Ombrotrophic-Minerotrophic Gradient. Soil Biol. Biochem. 54, 36–47. doi:10.1016/j.soilbio.2012.05.015
Yu, Y., Lee, C., Kim, J., and Hwang, S. (2005). Group-Specic Primer and Probe Sets to Detect Methanogenic Communities Using Quantitative Real-Time Polymerase Chain Reaction. Biotechnol. Bioeng. 89 (6), 670–679. doi:10.1002/bit.20347
Yvon-Durocher, G., Allen, A. P., Bastviken, D., Conrad, R., Gudasz, C., St-Pierre, A., et al. (2014). Methane Fluxes Show Consistent Temperature Dependence across Microbial to Ecosystem Scales. Nature 507, 488–491. doi:10.1038/nature13164
Keywords: methanogenesis, structure-function links, microbial community structure, eutrophication, sediment carbon cycling
Citation: Bertolet BL, Koepfli C and Jones SE (2022) Lake Sediment Methane Responses to Organic Matter are Related to Microbial Community Composition in Experimental Microcosms. Front. Environ. Sci. 10:834829. doi: 10.3389/fenvs.2022.834829
Received: 13 December 2021; Accepted: 11 March 2022;
Published: 31 March 2022.
Edited by:
Yves T. Prairie, Université du Québec à Montréal, CanadaReviewed by:
Sophie Crevecoeur, Environment and Climate Change, CanadaAnnika Vaksmaa, Royal Netherlands Institute for Sea Research (NIOZ), Netherlands
Copyright © 2022 Bertolet, Koepfli and Jones. This is an open-access article distributed under the terms of the Creative Commons Attribution License (CC BY). The use, distribution or reproduction in other forums is permitted, provided the original author(s) and the copyright owner(s) are credited and that the original publication in this journal is cited, in accordance with accepted academic practice. No use, distribution or reproduction is permitted which does not comply with these terms.
*Correspondence: Brittni L. Bertolet, YnJpdHRuaS5iZXJ0b2xldEB1Y2kuZWR1