- 1Department of Environmental Science, School of Engineering and Sciences, SRM University-AP, Amaravati, Andhra Pradesh, India
- 2Groupe de Recherche Interuniversitaire en Limnologie, Département des Sciences Biologiques, Université du Québec à Montréal, Montréal, QC, Canada
The aerobic oxidation of methane (CH4) by methanotrophic bacteria (MOB) is the major sink of this highly potent greenhouse gas in freshwater environments. Yet, CH4 oxidation is one of the largest uncertain components in predicting the current and future CH4 emissions from these systems. While stable carbon isotopic mass balance is a powerful approach to estimate the extent of CH4 oxidation in situ, its applicability is constrained by the need of a reliable isotopic fractionation factor (αox), which depicts the slower reaction of the heavier stable isotope (13C) during CH4 oxidation. Here we explored the natural variability and the controls of αox across the water column of six temperate lakes using experimental incubation of unamended water samples at different temperatures. We found a large variability of αox (1.004–1.038) with a systematic increase from the surface to the deep layers of lake water columns. Moreover, αox was strongly positively coupled to the abundance of MOB in the γ-proteobacteria class (γ-MOB), which in turn correlated to the concentrations of oxygen and CH4, and to the rates of CH4 oxidation. To enable the applicability in future isotopic mass balance studies, we further developed a general model to predict αox using routinely measured limnological variables. By applying this model to δ13C-CH4 profiles obtained from the study lakes, we show that using a constant αox value in isotopic mass balances can largely misrepresent and undermine patterns of the extent of CH4 oxidation in lakes. Our αox model thus contributes towards more reliable estimations of stable carbon isotope-based quantification of CH4 oxidation and may help to elucidate large scale patterns and drivers of the oxidation-driven mitigation of CH4 emission from lakes.
Introduction
Freshwater aquatic systems are important sources of methane (CH4)—a highly potent greenhouse gas—to the atmosphere (Bastviken et al., 2011; Saunois et al., 2016). However, large uncertainties exist in the estimates of global inland water CH4 emissions, partly due to the lack of a clear understanding on the various processes driving CH4 saturation and emission in aquatic environments. Aerobic methane oxidation (MOX), for example, plays a central role in regulating the global CH4 budget by biologically mitigating CH4 emissions from various natural (e.g., wetlands, rivers, lakes, and ocean) and anthropogenic (e.g., rice paddy fields, landfills) sources (King, 1992; Guérin and Abril, 2007; Reeburgh, 2007; Bastviken et al., 2008; Chanton et al., 2009; Chanton et al., 2011; Serrano-Silva et al., 2014; Cai et al., 2016; Sawakuchi et al., 2016; Li et al., 2022). Despite its pivotal role in controlling CH4 emissions, accurate quantification of MOX is constrained methodologically and often represents one of the largest uncertain components in the budgets of various CH4 sources. While experimental incubations of isolated samples (soil, sediment, or water) and tracking the decrease in CH4 concentration over time is the most often used method, in situ estimations based on the stable isotopic composition of CH4 offers a non-invasive whole-ecosystem technique to estimate the extent of CH4 oxidation (Happell et al., 1994; Tyler et al., 1997; Liptay et al., 1998; Bastviken et al., 2002; Chanton et al., 2008; Chanton et al., 2011; Zhang et al., 2012; Preuss et al., 2013; Sawakuchi et al., 2016; Gebert and Streese-Kleeberg, 2017; Sparrow et al., 2019; Komiya et al., 2020; Bakkaloglu et al., 2021).
The stable carbon isotopic signature of CH4 (δ13C-CH4) is extensively used for isotopic mass balance-based oxidation estimations. This approach relies on the behaviour of the two stable carbon isotopes (12C and 13C) in terms of mass, steric properties, and diffusion rates leading to unequal behaviour in the rates of transport and at the enzyme bonding sites of CH4-oxidizing bacteria (MOB or methanotrophic bacteria). As a result of the slightly faster diffusion and oxidation of 12C, the residual CH4 pool gets enriched in 13C (Barker and Fritz, 1981; Jahnke et al., 1999; Whiticar, 1999; De Visscher et al., 2004; Templeton et al., 2006; Gebert and Streese-Kleeberg, 2017). From the shift in the δ13C signature of CH4 at the point of sampling (δ13C-CH4-sample) relative to the anoxic source (δ13C-CH4-source), the fraction of CH4 oxidized (fox) can be estimated using either open system models at steady state (Happell et al., 1994; Tyler et al., 1997) or non-steady state Rayleigh model for closed systems (Liptay et al., 1998).
Due to the slightly faster uptake of 12C relative to 13C by MOB, the first order rate constant (k) of the oxidation of 12CH4 is greater than that of 13CH4; therefore, the ratio of 12k/13k represents the isotopic fractionation factor (αox). In practice, the αox is determined from the incubation of samples at a desired temperature, with or without CH4 amendments (Chanton and Liptay, 2000; Börjesson et al., 2001; Bastviken et al., 2002; Börjesson et al., 2007; Chanton et al., 2008; Aghdam et al., 2018; Obersky et al., 2018; Sparrow et al., 2019; Fjelsted et al., 2020; but see Gebert and Streese-Kleeberg, 2017). From the concomitant change in the concentration ([CH4]) and stable isotopic signature (δ13C-CH4) of CH4 during the incubation, the fractionation factor αox can be derived from the slope (
While the isotopic method is a simple and powerful tool to estimate CH4 oxidation in situ, an accurate αox value associated with oxidation in the given ecosystem is important in ensuring reliable estimations of the amount of CH4 consumed by methanotrophy. The use of inaccurate αox can induce large differences in the fox estimate (see, Cabral et al., 2010; Capanema and Cabral, 2012; Gebert and Streese-Kleeberg, 2017). It is thus critical to use suitable fractionation factors that are appropriate for each environment or for each place and time within a system.
Even though αox is often used as a constant, experimentally determined stable carbon isotopic fractionation factors for methanotrophic bacterial cultures and various terrestrial and aquatic environments together vary widely (1.003–1.049; Coleman et al., 1981, Bergamaschi et al., 1998, Bastviken et al., 2002, Venkiteswaran and Schiff, 2005, Chanton et al., 2008, Templeton et al., 2006; Gebert and Streese-kleeberg, 2017; Aghdam et al., 2018; Obersky et al., 2018; Sparrow et al., 2019; Fjelsted et al., 2020; Bakkaloglu et al., 2021). This large variability of αox across environments can be attributed to diverse mechanisms; from the supply of CH4 to the oxidizing zone to the rate of enzyme-catalysed oxidation of CH4 by MOB. In an environment with abundant CH4 supply, the irreversible conversion of dissolved CH4 to the cellular carbon pool of MOB controls the isotopic fractionation—a process dependent on the diffusion and dissolution of CH4 in the system, enzyme activity of the MOB, and the rate of CH4 oxidation (Templeton et al., 2006). Since the above factors can be regulated by a variety of environmental conditions such as temperature, production and diffusion of CH4 to oxidation zone, and MOB cell abundance, strong links between these variables and αox are expected. For example, an inverse relationship between temperature and αox is often observed for the forests and landfill soil environments (Tyler et al., 1994; Jahnke et al., 1999; Chanton and Liptay, 2000; Chanton et al., 2008; Nihous et al., 2010; Gebert and Streese-Kleeberg, 2017). The underlying mechanism behind such a pattern is suggested to be the increased oxidation rate at elevated temperature resulting in little fractionation, while low MOX rate is associated with greater discrimination of 13C. However, it should be also noted that an increase of αox with temperature was observed in enriched methanotrophic bacterial cultures (Coleman et al., 1981), which was supported by field estimates in the Alaskan soils (King et al., 1989) and European landfills (Bergamaschi et al., 1998). Furthermore, temperature response of αox is highly inconsistent in aquatic environments; from a complete absence of temperature effect in large lakes and reservoirs (Bastviken et al., 2002; Venkiteswaran and Schiff, 2005) to an inverse relationship between temperature for a small temperate lake (Venkiteswaran and Schiff, 2005). These inconsistent temperature responses in various environments suggest that there may be factors other than MOX rates at play to regulate αox variability. Experimental studies in terrestrial systems and/or methanotrophic bacterial cultures have shown that in addition to MOX rates, αox is dependent on the availability of CH4 and oxygen (O2), and the abundance of MOB (Börjesson et al., 2001; Templeton et al., 2006; Chanton et al., 2008; Gebert and Streese-Kleeberg, 2017).
Despite the long-recognised potential of isotopic mass balance in estimating CH4 oxidation in lakes (Bastviken et al., 2002), this approach is not extensively used in freshwater systems compared to terrestrial environments such as forest soils, landfills, and rice paddy fields. The number of δ13C-CH4 measurements has been increasing recently to derive greater insights on aquatic CH4 dynamics (Schubert et al., 2010; Blees et al., 2014; Rinta et al., 2015; Morana et al., 2015; Cadieux et al., 2016; Begum et al., 2021). Yet, only very few studies have derived the extent of CH4 oxidation based on isotopic data (Kankaala et al., 2007; Itoh et al., 2015; Thottathil et al., 2018; Grasset et al., 2020; Soued and Prairie, 2022) and studies that experimentally determined αox are even lesser (Bastviken et al., 2002; Venkiteswaran and Schiff, 2005; Itoh et al., 2015). Nevertheless, these studies showed variable αox values for lakes and reservoirs; between 1.018 and 1.021 for three Swedish boreal lakes (Bastviken et al., 2002), between 1.019 and 1.021 in a small temperate pond, 1.013–1.014 in temperate reservoirs (Venkiteswaran and Schiff, 2005), and 1.010 in a subtropical reservoir (Itoh et al., 2017). Since the number of αox estimates are limited in lakes, knowledge of the natural variability and environmental control of αox in these ecosystems is critically lacking. Methanotrophy in lakes is controlled by a suite of environmental factors including temperature, pH, total phosphorus (TP), total nitrogen (TN), and dissolved organic carbon (DOC) through regulating MOB population, supply of substrates (CH4 and O2), and by affecting water column light attenuation (Crevecoeur et al., 2019; Thottathil et al., 2019; Guggenheim et al., 2020; Reis et al., 2020; Sawakuchi et al., 2021; Kashi et al., 2022; Nijman et al., 2022). As response to these multiple environmental factors, MOX rates in freshwater lakes vary widely (Bastviken et al., 2002; Bastviken et al., 2008; Thottathil et al., 2019; Denfeld et al., 2016; Sawakuchi et al., 2021; D’Ambrosio and Harrison, 2021). However, whether αox values equally vary along with the MOX rates and/or how multiple environmental factors simultaneously shape the isotopic fractionation during CH4 oxidation in highly dynamic environments such as freshwater lakes remain elusive.
Here, we explicitly examine the variability and controls of stable carbon isotopic fractionation during aerobic CH4 oxidation in temperate lakes. Given the wide variability of MOX rate, we hypothesize that, 1) the inter- and intra-lake variability of αox can be large in the stratified water column of lakes, and 2) the environmental variables relevant in shaping the rate of CH4 oxidation and MOB abundance (e.g., temperature, pH, and concentrations of CH4, O2, nutrients, and DOC), are also relevant in regulating isotopic fractionation factor. To test these hypotheses, we conducted extensive incubation experiments with unamended lake water samples obtained from the stratified water column of six Canadian Shield lakes in southeastern Quebec during the summer stratification period. Using this unique dataset, we show that αox variability in freshwater lakes is large and comparable to the variability observed in terrestrial environments and is modulated by the abundance of a specific MOB group as well as by the rates of CH4 oxidation, which are in turn regulated by a suite of environmental factors. Furthermore, we developed an empirical model using routinely measured limnological variables that can be used to estimate αox in a wide range of freshwater environments. Finally, we show that CH4 oxidation extent in lakes can be significantly misrepresented at both seasonal and spatial scales if the variability of αox is poorly constrained.
Materials and methods
Study area and sampling strategy
We sampled six northern temperate lakes of variable morphometry (surface area: 0.008–0.44 km2; maximum depth: 4.3–20.3 m) during the summer stratification period (June to October) of 2016. Details on the limnological features of study lakes, sampling strategy, and experimental setups were given elsewhere (Thottathil et al., 2019; Reis et al., 2020). In each study lake, a single station was sampled onboard a small aluminium boat anchored at the deepest point located based on the known bathymetry (https://crelaurentides.org/dossiers/eau-lacs/atlasdeslacs). We performed the water column profiling at 1 m interval for temperature, pH, and O2 using a multiparameter probe (Yellow Spring Instruments, YSI; OH, United States). Along with the YSI-profiling, the water samples were collected for dissolved CH4 measurements from discrete depths at 1 m intervals using a continuous flow submersible pump (Proactive Environmental Products, FL, United States) attached to the YSI. At each discrete depth, water was allowed to pump for more than 5 minutes before initiating the sampling for dissolved CH4 using headspace equilibration method and the YSI was monitored continuously to ensure that pump was placed at the desired depth throughout the sampling. For the headspace equilibration, a 60 ml syringe was connected to the pump outlet through a two-way luer-lock valve in such a way that water is directly transferred to the syringe without getting exposed to the air and subsequent degassing. After filling two 60 ml syringes, 30 ml water was removed and the equivalent volume of ultrapure zero air (Praxair Canada Inc., Canada) was transferred to the syringe from a small pressurized zero air tank through a needle (23 G1, Becton–Dickinson) injected through a rubber septum of the zero-air tank outlet. The headspace was equilibrated manually for 2 min by vigorous shaking and the equilibrated headspace gas was then transferred into 12-ml pre-evacuated exetainer vials (Labco Ltd., United Kingdom) using a needle. The water sample for the incubation experiment (∼40 L) was collected in flexible cubitainers (VWR International, Canada) from two to three depths of the oxygenated layer of the water column of each lake using a submersible pump. The pump outlet was placed at the bottom of the cubitainers and filled slowly without bubbling and allowed to overflow for several minutes to replace the entire volume of the cubitainers and closed without trapping air bubbles. Except for the surface samples, the sampling depths for the incubation experiments were chosen differently in each lake due to large difference in total depth and the extent of stratification. Our sampling depths represented the surface mixed layer (surface), metalimnion (intermediate), and the deepest oxic waters in the hypolimnion or above the sediment (deep). This approach allowed us to cover a range of environmental conditions in terms of O2 and CH4 concentrations, as well as in terms of general water column chemistry (pH, TP, TN, and DOC) which are important drivers of the MOB community and CH4 oxidation (Sawakuchi et al., 2016; Crevecoeur et al., 2019; Thottathil et al., 2019; Guggenheim et al., 2020; Reis et al., 2020; Sawakuchi et al., 2021; Kashi et al., 2022; Nijman et al., 2022).
From the depths chosen for incubation experiments, samples for TN and TP were collected in duplicates directly into acid washed (10% Hydrochloric acid) and oven-dried (at 200°C) 50 ml screwcap tubes. For DOC, water samples were first collected into 60 ml plastic syringes directly from the submersible pump outlet and subsequently filtered through a 0.45 μm syringe filters (Sarstedt AG & Co., Germany) into acid-washed 40 ml glass vials. The vials were sealed with plastic caps lined with Teflon and rubber septa. All samples were kept cold in the dark until analyses. Except for L. Triton and L. en Coeur, where the water column was weakly stratified and therefore only two depths (surface and deep) were sampled, in all other lakes three depths (surface, intermediate, and deep) were sampled for the experiment. Thus, a total of 16 samples were collected from six lakes for the experimental incubations.
Experimental setup
In the laboratory, water from the cubitainers were dispersed into thirty 500 ml glass flasks, allowed to overflow and plugged air-tight using silicon stoppers without trapping air bubbles. A set of ten flasks were placed in temperature-controlled incubators set at three different temperatures: surface samples were at 10, 20, and 25°C; intermediate and deep water samples were incubated at 5, 10, and 20°C. All experiments were performed using unamended lake water samples since the addition of CH4 and/or O2 may yield higher than actual fractionation effect (Templeton et al., 2006). The first time point sampling for concentration and the stable carbon isotopic signature of CH4 (δ13C-CH4) was collected after about 3 h of acclimatization period in the incubators and a total of three to five time point samplings were conducted for each set of incubation. In order to examine presence of O2 in the incubation flasks for the aerobic oxidation, the concentration of O2 was monitored in two out of ten incubation flasks using optical O2 sensors spots and a fiber optic meter (PreSens GmbH, Regensburg, Germany). At each time point, two flasks were removed from the incubation system and two 30 ml sub-samples were withdrawn from each flask using 60 ml plastic syringes attached with a long needle to ensure that water was withdrawn from the bottom of the flask to avoid the contact of water with the air. The headspace (30 ml) was created by adding zero air into the syringes from a pressurized tank using a needle attached the luer-lock valve of the syringe and piercing through the rubber septa at the outlet of the regulated pressure valve. The headspace was equilibrated for 2 minutes by vigorous shaking and the equilibrated headspace was transferred into 12 ml pre-evacuated exetainer vials.
Measurement of CH4 concentration, δ13C-CH4 values, methanotrophic bacteria abundance, and environmental variables
The partial pressure and δ13C signature of CH4 in the headspace-equilibrated samples were measured using a Cavity Ring Down Spectrometer (CRDS) equipped with Small Sample Isotopic Module (SSIM, Picarro G2201-i, Picarro Inc., CA, United States). For high concentration samples (i.e., headspace concentration > 200 ppm), CH4 concentration was first measured using gas chromatograph (GC) with a flame ionization detector (GC-2014, Shimadzu, Kyoto, Japan) then the isotopic measurements were performed after dilution using zero air via SSIM. The original pCH4 in the water was calculated using the headspace ratio, incubation temperature, and temperature of the water in the syringe during equilibration using appropriate solubility constants (Yamamoto et al., 1976). The standard curve for δ13C-CH4 signature was prepared using certified isotopic standards obtained from Isometric Instruments, Canada (Liso1: −66.5 ± 0.2‰, Tiso1: −38.3 ± 0.2‰, and Hiso1: −23.9 ± 0.2‰) and values are reported in delta (δ) notation expressed in ‰ relative to the standard Vienna Pee Dee Belemnite.
To estimate the abundance of MOB cells, 40 ml water samples collected at the start of the incubation were fixed with buffered paraformaldehyde and filtered through 0.2 μm polycarbonate filters (Millipore GTTP, 25 mm). The filters were kept frozen until microscopic analysis through Catalysed Reporter Deposition—Fluorescence In Situ Hybridization (CARD-FISH) with probes for aerobic MOB belonging to α- and γ-proteobacteria and epifluorescence microscopy (see Reis et al., 2020 for details). We used extensively applied CARD-FISH probes (Malpha450, Mgamma84, and Mgamma705) to detect MOB cells and the effectiveness of these probes for detecting α-MOB and γ-MOB cells were described previously (see Figure 1; and Supplementary Material in Reis et al., 2022).
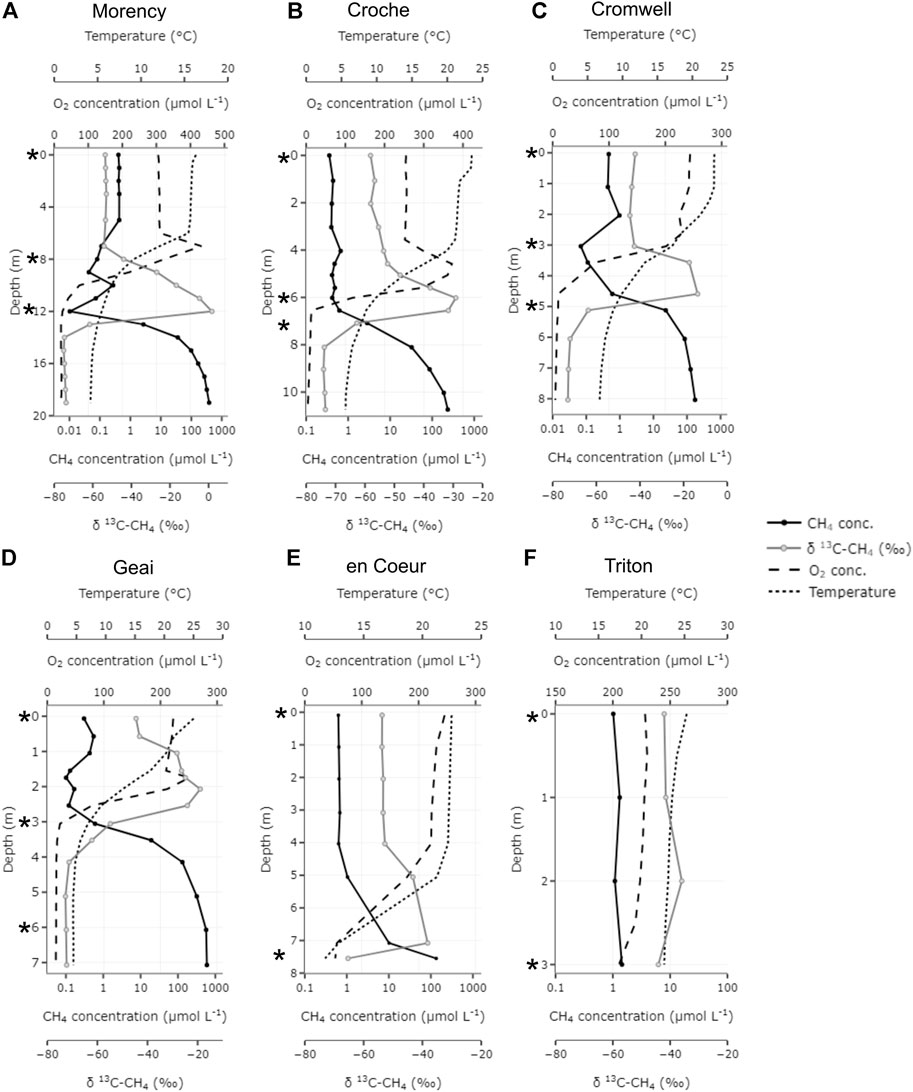
FIGURE 1. Vertical distribution of temperature, dissolved oxygen, methane concentration and stable carbon isotopic signature (13C-CH4) in each study lake (A–F). Symbol (*) in the Y-axis panel indicate the sampling depths for experimental incubations.
The concentration of TP was measured after potassium persulfate digestion of unfiltered samples following ammonium molybdate colorimetric method (Wetzel and Likens, 2000). For the measurement of TN, unfiltered samples were first digested using alkaline persulfate and analysed using a continuous flow analyser (ALPKEM Flow Solution 3100) equipped with a cadmium reactor (Patton and Kryskalla, 2003). The concentration of DOC was measured after sodium persulfate digestion of filtered water samples using a Total Organic Carbon analyzer (OI 1010, OI Analytical, TX, United States).
Calculations and statistical analyses
To estimate the kinetic isotopic fractionation during CH4 oxidation, first we calculated the slope of the regression between ln [CH4] and ln (δ13C-CH4 + 1000) for each incubation and then the fractionation factor (αox) was derived from the slope following Eq. 1 (see Introduction). This estimation is based on the “Simplified Rayleigh approach” recommended for incubation experiments using unamended and unlabelled CH4 (Mahieu et al., 2006). In this approach, the range of CH4 concentration has been shown to have a limited influence on the error of αox. Since our experiments are conducted over a large range of CH4 concentrations, error associated with CH4 concentrations will be minimal with the Simplified Rayleigh approach and therefore can be compared across the experiments. Moreover, this approximation of αox was shown to be superior to the Coleman method (Coleman et al., 1981) since its difference to the original Rayleigh method is relatively smaller (<0.05%) than that of the Coleman method (up to 5%).
Further, we explored the environmental control of αox using multiple regression analysis with Least Square Regression method. We used the incubation temperature, pH, concentrations of DOC, O2, CH4, TN, TP, cell abundance of α-MOB and γ-MOB, and MOX rates as candidate independent variables and only statistically significant variables were selected in the final model. The final environmental model was applied to the data collected in 2014 and 2015 in the same study lakes to explore the implications of our model on the seasonal and vertical variability of αox and the fraction of CH4 oxidized (fox). First, we estimated the seasonality in fox in the surface layers of the study lakes using closed system model (Eq. 2).
where δ13C-CH4sample is the seasonal δ13C-CH4 data obtained from our study lakes during May–November 2015 and δ13C-CH4-source is a fixed value for each lake. For the comparison, we used modelled αox along with a fixed value of 1.020 which is the average αox estimated in the previous studies for the northern lakes (Bastviken et al., 2002; Venkiteswaran and Schiff, 2005). Secondly, we estimated the vertical variability of αox using data obtained from three of our study lakes during the summer 2014.
We used Analysis of Variance (ANOVA) and Tukey’s HSD (Honest Significant Difference) to explore the variations in the water chemistry, CH4 concentrations, stable isotopic signature, and αox across depth zones. All the statistical analyses were performed with JMP® Pro software version 14.0 (SAS Institute, United States) and graphs were plotted using ggplot2 (v3.3.3), cowplot (v1.1.1) and plotly (v4.10.0) packages (Wickham, 2016; Wilke et al., 2019; Sievert, 2020) in R software (v4.0.4) or using Grapher™ (Golden Software LLC, United States).
Results
Environmental conditions, concentration and δ13C signature of CH4, and methanotrophic bacteria abundance
All sampling lakes, but L. Triton, were thermally stratified and the vertical distribution of CH4 concentration and δ13C-CH4 values were mirror images of each other with lowest CH4 concentration and most enriched δ13C-CH4 values coinciding in the metalimnion (Figure 1). Since we sampled the thermally stratified water column for the experiment, various chemical variables (pH, TN, TP, and DOC) at the beginning of the incubations varied largely across layers (Supplementary Figure S1). Similarly, the initial CH4 concentration in the incubations varied widely with significant difference across depth zones; surface and intermediate layers showed a narrower range (0.27–0.59 and 0.06–0.36 μmol L−1, respectively) than the deep layer (0.25–30.02 μmol L−1; Figure 2A). While initial δ13C-CH4 values showed a narrower range in the surface (−55.48‰ to −26.07‰) and deep layer (−55.79‰ to −26.76‰), the intermediate layer exhibited a much larger variability (−49.04‰ to −15.32‰) (Figure 2B). The abundance of α-MOB and γ-MOB at the start of each incubation also varied widely; the abundance of γ-MOB ranged from 1.05 × 102 to 8.11 × 104 cells ml−1 (average: 1.13 × 104 cells ml−1) and α-MOB abundance ranged from 8.27 × 102 to 2.37 × 104 cells ml−1 (average: 6.93 × 103 cells ml−1; Figures 2C,D). While the abundance of α-MOB was unrelated to the concentration of O2 and only slightly related to the CH4 concentrations, the abundance of γ-MOB increased with decreasing O2 concentrations and positively correlated with CH4 concentrations (Supplementary Figure S2). Furthermore, γ-MOB abundance was strongly positively and linearly correlated with TP (R2 = 0.74, p < 0.0001) and TN (R2 = 0.64, p < 0.0001), but unrelated to DOC (Supplementary Figure S3). On the other hand, abundance of α-MOB was not significantly linearly related to the above variables, except a weak positive correlation with TP (R2 = 0.31, p < 0.0001; Supplementary Figure S3).
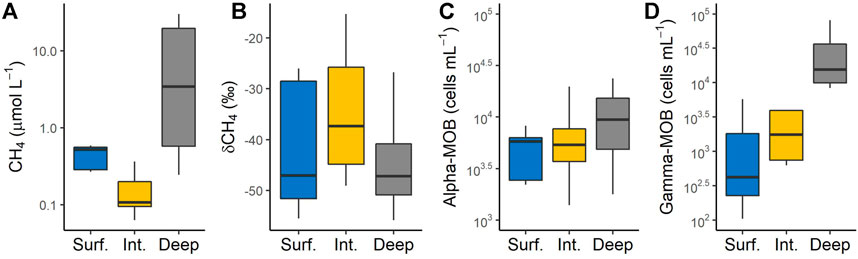
FIGURE 2. Variability of methane (CH4) concentration (A), stable carbon isotopic signature of CH4 (δ13C-CH4) (B), abundance of α (C) and γ (D) groups of methanotrophic bacteria (α-MOB and γ-MOB, respectively) at the start of the incubation experiments across all study lakes. Surf., Surface layer; Inter., Intermediate layer; Deep, Deeper layer. The boxplots represent the median, first and third quartiles (hinges) and the 1.5 × interquartile range (whiskers).
Methane oxidation rate and patterns of isotopic fractionation factor
A total of 48 incubation experiments encompassing samples from six different lakes, two-three depths from each system, and three different incubation temperatures were conducted. The MOX rates across our study lakes, depth layers, and incubation temperature ranged widely from 0.01 to 59.71 μmol L−1 d−1 with consistently high rates in the deep layer (16.27 ± 20.33 μmol L−1 d−1) than in the surface (0.13 ± 0.18 μmol L−1 d−1) and intermediate layer (0.11 ± 0.15 μmol L−1 d−1). The MOX rate was highly positively correlated with the concentrations of TP (R2 = 0.64; p < 0.0001) and TN (R2 = 0.55; p < 0.0001) while unrelated to DOC (Figures 3A–C). Furthermore, MOX rate was strongly positively correlated with γ-MOB abundance (R2 = 0.84; p < 0.0001; Figure 3E), while weakly correlated with the abundance of α-MOB (R2 = 0.33; p < 0.0001; Figure 3D).
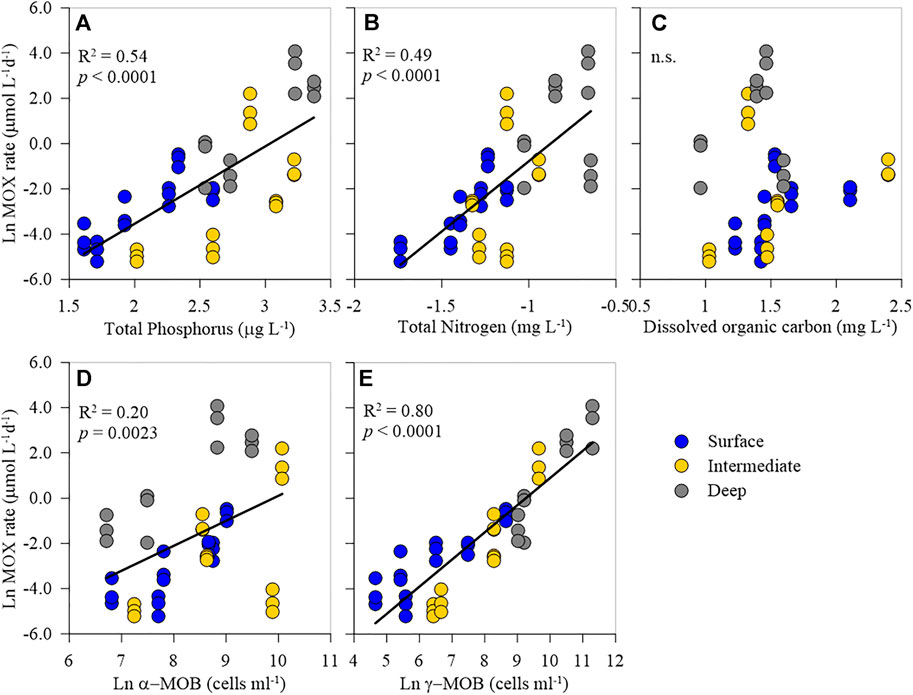
FIGURE 3. Relationships between methane oxidation rate (MOX) and total phosphorus (A), total nitrogen (B), dissolved organic carbon (C), and abundance of α-MOB (D) and γ-MOB (E).
For the water samples from the deeper layers of L. Geai, the initial O2 concentrations were quite low (<26 µmol O2 L−1). Given the measured MOX rate (55.8, 47.7, and 31.3 μmol L−1 d−1 at 20, 10, and 5°C respectively) and assuming a CH4:O2 stoichiometry of 1:1.8 for aerobic CH4 oxidation (Naguib 1976), incubations would have run out of O2 before the second time point sampling within 12 h. Therefore, those results were excluded to limit our analysis to the controls of isotopic fractionation factor during strict aerobic oxidation. Hence, a total of 45 αox estimations representing the water column of six temperate lakes were used for further statistical analysis to understand the controls of αox. During the incubation, declines in CH4 concentration were generally mirrored by the δ13C-CH4 value and the isotopic fractionation factor (αox) was estimated for each depth and incubation temperature (Supplementary Figure S4). Across the water column of the six study lakes, two—three depths, and different incubation temperatures (5–25°C), αox varied from 1.004 to 1.038 (Figure 4; Supplementary Table S1). Notably, this large variability of αox observed in our experiments is not only introduced by the difference in incubation temperature, but the sampling depth also mattered substantially. For instance, αox varied from 1.011 to 1.028 for the surface samples of all lakes incubated at 20°C, while for the intermediate and deep layer samples at the same temperature, αox values varied from 1.020 to 1.031 and 1.030 to 1.032, respectively (Supplementary Table S1). Moreover, combining our estimates at different temperatures by layers showed a remarkably low αox in the surface (1.018 ± 0.007) relative to the intermediate (1.028 ± 0.004) and the deep (1.032 ± 0.004) layers (Figure 4). However, a strong decrease in αox with increasing temperature was generally observed for the intermediate and deep layers (Figure 4). Except for the surface layer of shallow L. Triton, where αox strongly negatively correlated with temperature, a hump-shaped relationship between αox and temperature was observed for the surface of other lakes (Supplementary Figure S5). Together, temperature was negatively correlated with αox (R2 = 0.12; p = 0.0190; Supplementary Figure S5). For a given depth where MOX rate is high and an increase of MOX rate was observed with increasing temperature, a general decline of αox with increasing temperature and therefore MOX rate was evident (Supplementary Figure S6). This pattern suggests that in several cases, particularly in the intermediate and deep layers, MOX rate was strongly negatively correlated to the isotopic fractionation (Supplementary Figure S6).
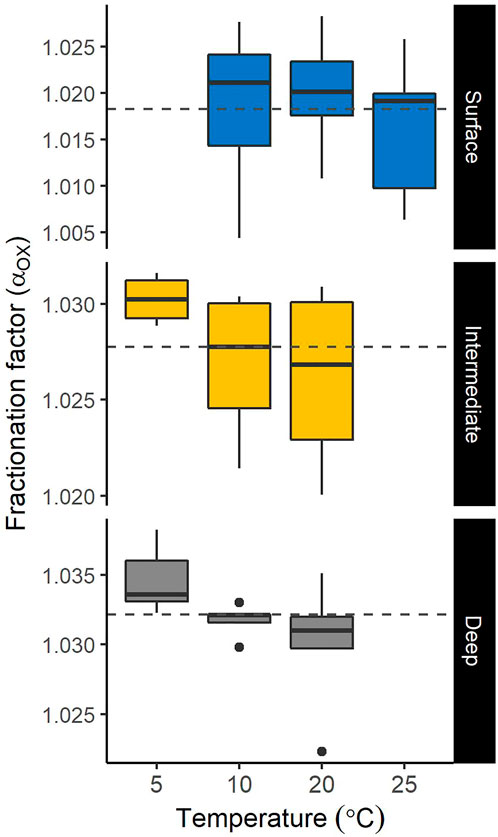
FIGURE 4. Variation of the isotopic fractionation factor (αox) during aerobic CH4 oxidation across different layers and incubation temperatures in the study lakes. Note that αox estimations from all lakes are pooled for a given incubation temperature. Horizontal dashed lines represent the average for each layer across all incubation temperatures. The boxplots represent the median, first and third quartiles (hinges) and the 1.5 × interquartile range (whiskers).
We explored the regulation of αox in the water column of our study lakes; αox was most significantly correlated with γ-MOB abundance (R2 = 0.56, p < 0.0001), while only weakly correlated with α-MOB (R2 = 0.13, p = 0.0139; Figure 5). We developed a simple predictive model for αox using multiple regression. Among all the tested variables, γ-MOB abundance (cells ml−1) and MOX rate (µmol L−1 d−1) emerged as the predictive variables in the multiple regression model (both MOB abundance and MOX rate were highly statistically significant; p < 0.0001) and the resulting model took the form of:
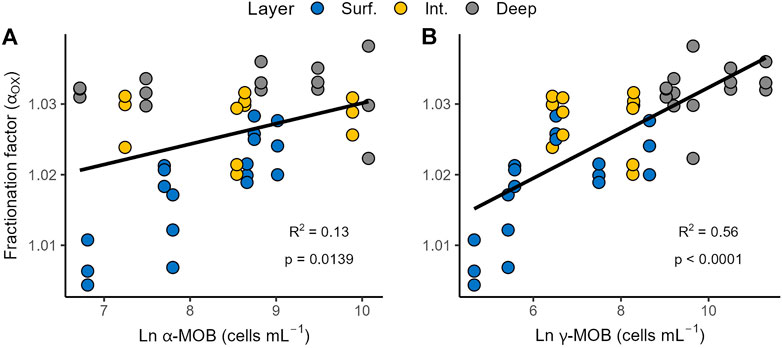
FIGURE 5. Relationships between carbon stable isotopic fractionation factor (αox) and the abundance of α-MOB (A) and γ-MOB (B) in the study lakes.
p < 0.0001, n = 45
While this simple model explains MOB abundance and MOX rates as the key drivers of αox, MOB abundance and MOX rates are not frequently measured variables and therefore may not be suitable as a predictive model for αox to be applied in the isotopic mass balance of other systems. Therefore, we further explored the data to develop a predictive model for αox using routinely measured limnological variables. To do so, we have included all measured variables such as temperature, pH, concentrations of O2, CH4, DOC, TP, TN, and Chlorophyll a in the statistical analysis. However, the final multiple regression model with statistically significant predictive variables took the form of:
R2 = 0.78; p < 0.0001; n = 45, where TP and DOC are in μg L−1 and mg L−1, respectively and temperature is in °C (Supplementary Figure S7). All the parameter estimates of the model were statistically significant; p < 0.0001 for DOC and pH, p = 0.00025 for TP, and p = 0.02045 for temperature.
Variability of modelled αox and implications to fox estimates
Further, we used the temperature, pH, TP, and DOC data of the surface layer of the study lakes in 2015 to predict the fractionation factor by applying the αox model (Eq. 4). We observed a clear seasonality in the estimated αox values with lower values consistently observed during the peak summer stratification period (Figure 6). Across our study lakes, modelled αox varied widely with a larger seasonal variability in L. Morency (1.003–1.025) and L. Croche (1.009–1.025) compared to L. Geai (1.015–1.028) and L. Cromwell (1.016–1.027), while the seasonal αox variability was minimal in L. en Coeur and L. Triton (1.010–1.019 and 1.019–1.029, respectively). Subsequently, the variability of the extent of oxidation (i.e., fox) was different across lakes; much pronounced in some lakes (Morency, Croche, and Geai) while moderate in the others (Figure 6). Further, we compared fox estimates derived from the modelled αox with a fixed value of 1.020 previously used for temperate lakes (Bastviken et al., 2002). Our comparison indicates that using a fixed αox clearly masks seasonality and can substantially misrepresent oxidation estimates at ecosystem scale. Similarly, when we applied the αox model to water column profiles performed in 2014, we observed a large variability of αox with depth (Figure 7), but the extent of the variability was different across lakes. αox ranged from 1.019 to 1.044 in L. Morency, while the variability was only marginal (1.020–1.032) in L. Cromwell. Overall, our estimation showed a large spatial and temporal variability of αox across lakes and therefore shows that inaccurate αox values can cause large errors in the estimation of CH4 oxidation.
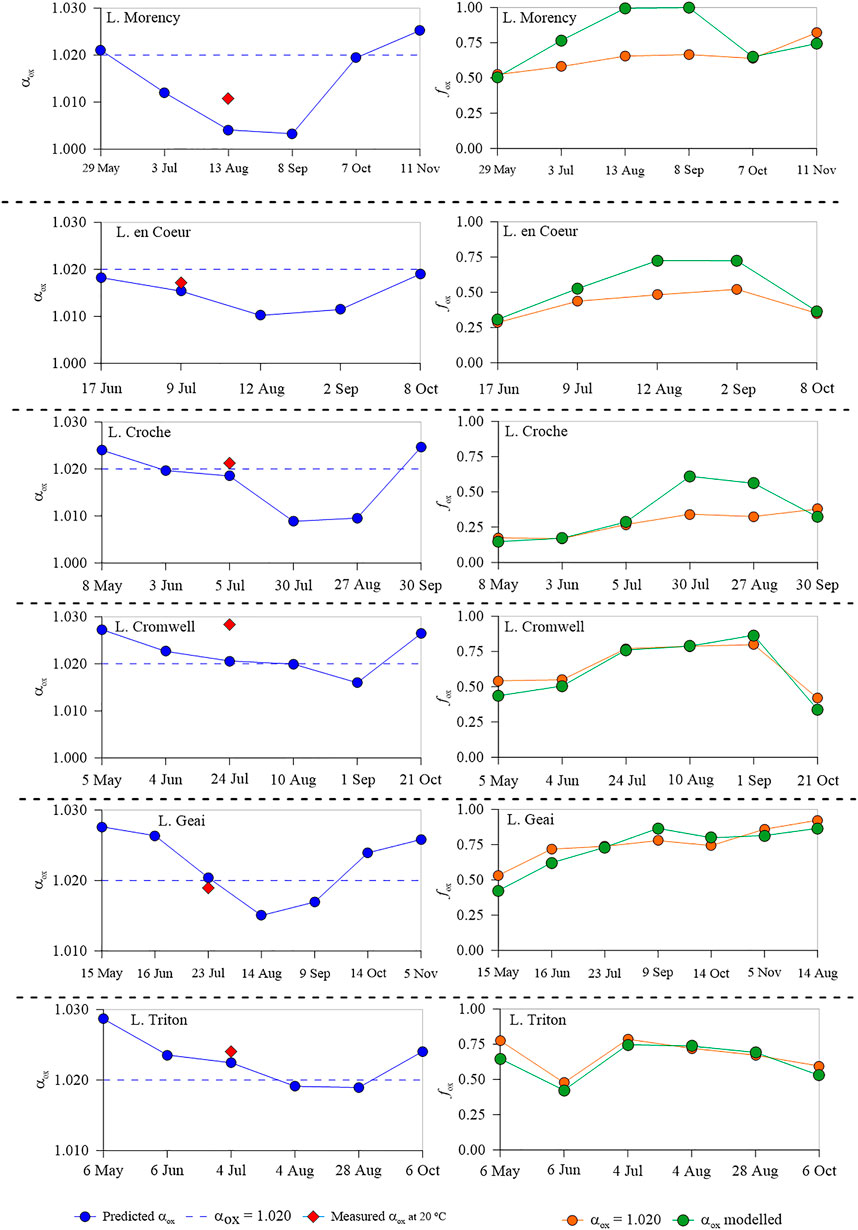
FIGURE 6. Seasonal variation in predicted αox (left panel) and fraction of CH4 oxidised (fox) (right panel) estimated using a fixed αox of 1.020 or the modelled αox in the surface layers of each study lake.
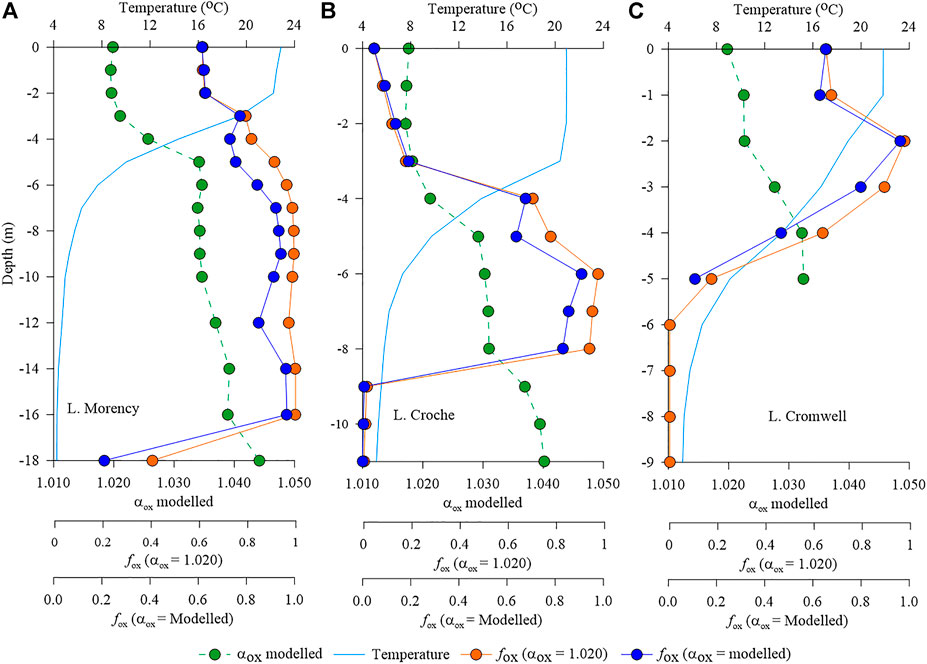
FIGURE 7. Vertical variation in modelled αox, the fraction of CH4 oxidised (fox) estimated using a fixed αox of 1.020, and fox estimated using modelled αox in (A) L. Morency, (B) L. Croche and (C) L. Cromwell.
Discussion
The estimation of CH4 oxidation using stable isotopic approach fundamentally relies on the isotopic fractionation factor. Despite the growing number of stable isotopic measurements of CH4 in inland waters (Bastviken et al., 2002; Kankaala et al., 2007; Schubert et al., 2010; Rinta et al., 2015; Sawakuchi et al., 2016; Thottathil et al., 2018; Grasset et al., 2020; Begum et al., 2021), the use of isotopic mass balance to estimate the extent of CH4 oxidation is restricted to very few studies, and wherever attempted, a single αox was applied across systems (Bastviken et al., 2002; Sawakuchi et al., 2016; Thottathil et al., 2018; Grasset et al., 2020). Our extensive experimental incubations of unamended water samples from six temperate lakes have shown wide variability of αox in natural conditions. Furthermore, we show that αox variability in lake water column is predominantly shaped by the dominant group in the methanotrophic bacterial assemblage (γ-MOB) and MOX rates. However, in a practical point of view, we further developed a model for predicting αox in natural waters using routinely measured limnological variables that are in turn known to regulate γ-MOB assemblage and MOX rates.
Variability and patterns of αox in freshwater lakes
The existing data show a large variability of αox (1.005–1.049) in terrestrial environments (landfill, rice paddy field, and soil) and in various methanotrophic bacterial cultures (Coleman et al., 1981; Bergamaschi, 1997; Templeton et al., 2006; Chanton et al., 2008; Gebert and Streese-kleeberg, 2017; Aghdam et al., 2018; Obersky et al., 2018; Sparrow et al., 2019; Fjelsted et al., 2020; Bakkaloglu et al., 2021). Unlike these systems, only a few experimentally determined αox values exist for aquatic systems: 1.009 in a subtropical reservoir (Itoh et al., 2015), 1.013–1.021 in experimentally flooded boreal reservoir (Venkiteswaran and Schiff, 2005), and 1.018 to 1.021 in three boreal lakes (Bastviken et al., 2002). However, given that MOX rates vary several orders of magnitude across lakes (Bastviken et al., 2002; Thottathil et al., 2019; Denfeld et al., 2016; Sawakuchi et al., 2021; D’Ambrosio and Harrison, 2021) and considering that MOX rate is often coupled with isotopic fractionation (Chanton et al., 2008; Gebert and Streese-Kleeberg, 2017), existing estimates (1.009–1.021) likely underrepresent the extent of αox variability in lakes. Unlike previous studies, we estimated the isotopic fractionation factor across and within several lakes covering large environmental gradients and range in methanotrophic activity. Therefore, our study revealed a large and previously unrealised variability of αox (1.004–1.038; Figure 4) during aerobic CH4 oxidation in aquatic environments.
Our experimentally determined αox values for six temperate lakes encompass nearly the full spectrum of variability shown across methanotrophic bacterial cultures and diverse terrestrial environments (1.003–1.049; Bergamaschi, 1997; Chanton et al., 2008, Templeton et al., 2006; Bakkaloglu et al., 2021). Based on concurrently measured deep-water profiles of CH4 and δ13CH4, αox values were estimated to be 1.008 in the temperate L. Lugano (Blees et al., 2014), 1.037 in the boreal L. Mekkojarvi (Kankaala et al., 2007), and between 1.02 and 1.06 in three Arctic lakes (Cadieux et al., 2016). Unlike these data from different systems, our extensive estimates within the water column of temperate lakes showed a systematic vertical shift in αox with significantly lower values in the surface relative to a few meters below (Figure 4). This systematic variability of αox can be attributed to the observed increase of MOB abundance. While inhibition of CH4 oxidation at high O2 levels combined with low CH4 supply reduce methanotrophic activity in the surface layers, “optimal O2” concentration (∼15 μmol L−1; Thottathil et al., 2019) and high CH4 supply enhance MOB growth, particularly γ-MOB abundance in the deep layer (Zigah et al., 2015; Reis et al., 2020). Together, the vertical distribution of αox in the water column of our study lakes shows greater discrimination of heavier isotopes (13C) at high MOB abundance while relatively less discrimination in the surface layer where MOB abundance is relatively low.
Studies in terrestrial environments shown that for a given site, αox generally decreases with increasing MOX rate which is in turn linked to temporal changes in temperature (Chanton et al., 2008; Gebert and Streese-Kleeberg, 2017). Therefore, the low αox in the surface layer where MOX rate is low and high αox in the deeper layer where MOX rate is high in lakes seems contradictory to the pattern observed in the terrestrial environments. However, at a fixed MOB abundance for a given water layer, temperatures generally enhanced the MOX rates, which resulted in lower αox (Figure 4; Supplementary Figures S5, S6). This is particularly pronounced in the intermediate and deep layers where MOX rate and MOB abundance are consistently high (Supplementary Figure S6). Thus, our data in general is in accordance with the pattern observed in the terrestrial environment where higher MOX rates lead to lower discrimination against heavier isotope and therefore lower values of αox (Chanton et al., 2008; Gebert and Streese-Kleeberg, 2017). However, for a given layer of the stratified water column, αox can be highly sensitive to the factors affecting the temporal changes in CH4 oxidation besides temperature (substrate supply, nutrients, etc.) and these need to be accounted for precise αox estimates. Together, our study clearly shows that using literature-derived αox values, or assuming a single isotopic fractionation factor over space and time within or across systems can lead to miscalculation of oxidation extent in lakes.
The emergence of γ-MOB as a key predictor of αox in the lake water column is an important outcome of this study. Notably, the correlation between γ-MOB and αox was evident within each layer of the water column—even in the surface waters where γ-MOB abundance was generally low (Figure 5). Although previous studies have linked isotopic fractionation to MOB abundance in growth medium with pure and/or mixed methanotrophic cultures (Templeton et al., 2006; Feisthauer et al., 2011), our study establish such connection in natural, unamended samples. It should be noted that the abundance of γ-MOB was higher than the abundance of α-MOB (Figure 2) in our study lakes, particularly in the deeper layer of stratified lakes where multiple environmental factors (low pH, high TP concentration, and strong counter gradients of CH4 and O2; Figure 1; Supplementary Figure S1) support the proliferation of γ-MOB cells (Reis et al., 2020; Nijman et al., 2021). Under these ideal environmental conditions, the greater γ-MOB abundance and higher CH4 oxidation rates result in higher isotopic fractionation. This is further evident from the stronger correlation between γ-MOB abundance and MOX rates than that between α-MOB abundance and MOX rates in our study lakes (Figure 3). However, the stronger relationship between γ-MOB abundance and αox (Figure 5) does not necessarily indicate a greater discrimination against the heavier isotope (13C-CH4) in γ-MOB cells than in α-MOB cells. In systems or environments where α-MOB is dominant over γ-MOB and MOX rate is regulated by α-MOB abundance, changes in α-MOB abundance are expected to drive αox variation. Our estimates suggest that under ideal conditions, enhanced uptake of lighter carbon (12C) can result in αox values as high as 1.038, corroborating previous observation that where there is an ideal condition for methanotrophic growth (i.e., excess CH4 availability and optimal O2 oxygen concentration), αox values should approach its maximum (Templeton et al., 2006). Our data shows that in the stratified lakes, such condition often exists in the deep layers of the water columns resulting in large increase of αox from the surface to deeper layers. Further, multiple regression analysis suggested that γ-MOB abundance and MOX rates essentially control the variability of isotopic fractionation in the water column of our study lakes (Eq. 3), where αox was shown to decrease with increasing oxidation rates while increases with γ-MOB abundance. While the depth-wise variation of αox suggests an increase of isotopic fractionation with MOX rates (Figure 4), the multiple regression analysis shows an inverse relationship between αox and MOX rates consistent with studies from various terrestrial environments (Templeton et al., 2006; Chanton et al., 2008; Gebert and Streese-Kleeberg, 2017). This suggest that for a given γ-MOB cell abundance, an increase in MOX rate induced by increased in temperature and CH4 concentration, and/or “optimal O2” supply would essentially result in less discrimination against heavier isotope.
Modelling αox with routinely measured limnological variables
While the αox model depicts that the abundance of γ-MOB and MOX rates are the most important drivers of isotopic fractionation (Eq. 3), we analysed the data further to develop a more “practical model” that enables the prediction of αox using routinely measured limnological variables (Eq. 4; Supplementary Figure S7). Temperature emerged as a statistically significant parameter (p = 0.0245) in explaining the variability of isotopic fractionation. Systematic decrease of αox with increasing temperature was often observed in terrestrial environments for temperature range of 5–25°C (Tyler et al., 1994; Chanton et al., 2008; Gebert and Streese-Kleeberg, 2017). Despite a comparable temperature range for our incubations, inverse relationship between temperature and αox was weak in our model where all measurements were combined. Yet, for samples where MOX rates were generally high and substantially enhanced by temperature, strong temperature response was observed (Supplementary Figure S6). For instance, small (0.017 km2) and shallow (maximum depth: 4.2 m) unstratified L. Triton where MOX rate was highest among all surface samples, a strikingly strong temperature dependence of αox (Supplementary Figure S5) with a slope (−7.6 × 10–4 and −1.6 × 10−3) comparable with the values reported for soil environments (−4.0 × 10−6 to −1.7 × 10−4; Chanton et al., 2008) was observed. Although we lack estimates from similar systems, a previous study in a similarly sized boreal lake (0.024 km2) in the Experimental Lakes Area, Ontario (Canada) showed a comparable pattern with strong temperature dependence of αox (slope: −2.0 × 10−4; Venkiteswaran and Schiff, 2005). Thus, these data suggest a comparable temperature dependence of αox in small lakes and soil environments, while the pattern is different for the surface layers of larger lakes (Supplementary Figure S5). However, further studies are needed to confirm whether there exists systematic shift in the temperature response of αox in the continuum from soil to small and large lakes. In addition to small L. Triton, for most of the deep layer samples where MOX rates were relatively high, increasing incubation temperature resulted in a general decrease of αox (Figure 4; Supplementary Figure S6). This temperature response of αox that we observed for most our samples is likely linked to the increasing MOX rates with temperature; where a strong positive relationship with temperature and MOX rate was observed, an inverse relationship between temperature and αox was evident (Supplementary Figure S6) indicating that for a given sample, enhanced CH4 oxidation with temperature is associated with less isotopic discrimination. Our result is therefore consistent with the inverse relationship between site specific seasonal temperature changes and αox observed for various terrestrial environments (Tyler et al., 1994; Chanton et al., 2008; Gebert and Streese-Kleeberg, 2017).
Despite the narrow pH range in our data, pH was a highly significant variable (p < 0.0001) in shaping overall variability of isotopic fractionation. Given that in our study lakes αox is shaped by γ-MOB abundance, the emergence of pH in the model is consistent with the effect that pH exerts on bacterial communities in general and on MOB community structure in lakes (Newton et al., 2011; Ren et al., 2015; Crevecoeur et al., 2019; Guggenheim et al., 2020). The pH effect on bacterial abundance can be direct since even small changes in pH can impose strong physiological changes, likely induce changes in competition for resources, and affect the net growth of the population (Madigan et al., 2011; Ren et al., 2015). Furthermore, at the ecosystem-scale, pH also exerts indirect effects on bacterial communities acting as an integrative factor of multiple environmental variables including nutrients and substrate availability (Ren et al., 2015; Crevecoeur et al., 2019; Guggenheim et al., 2020). Therefore, we believe that the effect of pH shown in these studies must be relevant for MOB communities in our study lakes, thereby emerging as a proxy of γ-MOB abundance, which directly controls αox. However, we acknowledge that our understanding on the effect pH on MOB communities and isotopic fractionation is still limited and future studies are warranted to confirm the trend shown here.
The “practical model” for αox showed a highly significant negative relationship between αox and DOC concentrations (p < 0.0001; Supplementary Figure S7). Although this pattern corroborates the role of DOC in shaping MOB community in northern lakes (Samad and Bertilsson, 2017; Crevecoeur et al., 2019), uncoupling of DOC and MOB abundance (Supplementary Figure S3) in our study lakes warrants other explanations for the DOC-αox relationship in the model. It should be noted that DOC concentration was positively correlated with MOX rates in the surface and intermediate layers, while a hump-shaped relationship was found in the deep layer (Supplementary Figure S8). This positive relationship between MOX rate and DOC in the upper layers can be attributed to the strong negative relationship between DOC and O2 concentrations which is in turn induced by higher heterotrophic bacterial respiration of organic carbon with increasing DOC concentration (Supplementary Figure S8). Since high O2 concentration inhibits CH4 oxidation (Rudd et al., 1976; Thottathil et al., 2019), observed decrease of O2 concentration with the increasing concentration of DOC is likely to relive the methanotrophic community from O2 inhibition and thereby enhances the oxidation rate. However, it should be noted that O2 is not selected as a predictive variable in the αox model. Therefore, DOC not only integrates the effect of O2, but also accounts for other potential factors such as the light attenuation-mediated enhancement of MOB activity (Dumestre et al., 1999; Murase and Sugimoto, 2005; Sawakuchi et al., 2016; Thottathil et al., 2018). Together, we suggest that increasing DOC concentration and thereby MOX rates resulted in less discrimination against the heavier isotope as shown in the model (Eq. 3); therefore, in our “practical model” DOC should be interpreted as proxy of CH4 oxidation.
Unlike temperature, pH, and DOC, the model showed a strong positive correlation between TP and αox (Supplementary Figure S7; p = 0.0003). Note that TP was correlated with the abundance of MOB, particularly with γ-MOB (Supplementary Figure S3) suggesting a greater role of P-availability in enhancing the methanotrophic abundance; a pattern previously shown for ice-covered boreal lake water column (Denfeld et al., 2016; Sawakuchi et al., 2021) and subtropical lake sediment (Nijman et al., 2022). Therefore, the positive correlation between TP and αox indicates that TP in our “practical model” is a proxy of the abundance of dominant methanotrophic group (i.e., γ-MOB). The identification of TP as a strong regulator of MOX rates and αox has important implications. For example, a recent study reported strong correlation between the extent of oxidation (i.e., fox) and TP across lake mesocosms (Grasset et al., 2020). Since CH4 oxidation is known to be light inhibited (Dumestre et al., 1999; Murase and Sugimoto, 2005), the authors attributed this pattern to enhanced methanotrophic activity due to greater light attenuation in the P-amended mesocosms where phytoplankton biomass increased substantially (Grasset et al., 2020). However, our data suggests that the role of TP on CH4 oxidation can be rather direct by stimulating CH4 oxidation as shown in recent studies (Sawakuchi et al., 2021; Kashi et al., 2022; Nijman et al., 2022). Hence, our model suggests that overlooking the effect of TP and assuming a single αox for the mass balance calculation across systems with different trophic status could underestimate the fox. It is interesting to note that despite the covariation of TP and TN in our study lakes, TN is not a significant predictive variable in the multiple regression analysis. This is in accordance with the recent study showing that MOB community is P-limited as evident from the increase of P content of the MOB biomass despite the elevated phosphorus and nitrogen levels in the experimental incubations (Nijman et al., 2022). Along with the above study, our data suggests that strong correlation between TP and MOB abundance is not just due to their concomitant increase with depth but caused due to the strong role of P in stimulating MOX rates and increasing MOB abundance in lakes. The γ-MOB abundance is positively correlated with αox in our model (Eq. 3); therefore, the strong correlation between αox and TP in our “practical model” suggests TP as a proxy of γ-MOB abundance and its increase leads to greater isotopic fractionation.
The importance of using appropriate αox values
By applying our environmental model of αox to water column profiles, we found that αox and consequently the seasonal variability of fox was much more pronounced in some lakes (Morency, Croche, and Geai) than in others (Figures 6, 7). Therefore, assuming a single αox value to all lakes would largely misrepresent the oxidation extent and thereby lead to errors in the estimation of ecosystem-scale CH4 oxidation rates. For instance, assuming a constant αox of 1.020 throughout the year in the epilimnion of L. Morency would underestimate the fox by up to 34% during August–September period and underestimate by up to 8% in the spring and autumn period (Figure 6). Similarly, applying an αox of 1.020 throughout the water column overestimates fox by up to 10% in the deeper layers of our study lakes (Figure 7). These potential errors in the fox estimation induced solely by the difference in αox value is comparable with the error estimations reported previously (Cabral et al., 2010; Capanema and Cabral, 2012). Together, our “practical αox model” with routinely measured limnological variables allows more accurate estimation of fox via δ13C-CH4 mass balance and enable to derive large-scale patterns of CH4 oxidation across freshwater aquatic systems without the biases induced by the variability of αox.
Conclusion
In conclusion, we show a large variability in the stable carbon isotopic fractionation during aerobic CH4 oxidation in freshwater lakes with a systematic increase of αox from the surface to deep layers of the water column. Our study showed that the rates of CH4 oxidation and the abundance of γ-MOB explain the variability of αox in temperate lake water columns. However, we suggest that the observed relationship between γ-MOB abundance and αox is due to the dominance of this MOB group in the temperate lake water column and does not necessarily suggest a group specific difference in isotopic fractionation. For a given γ-MOB abundance, αox decreased with increasing MOX rates—a pattern consistent with the data from various terrestrial environments suggesting that with increasing CH4 oxidation rate, there is less discrimination against the heavier stable isotope (13C). For practical purposes, we developed a general model of αox by integrating the effect of various factors on experimentally derived αox values using routinely measured limnological variables. We showed that applying a single αox value in CH4 stable isotopic mass balances can misrepresent its within lake variability, thereby hampering the detection of existing spatial and temporal patterns in the extent of CH4 oxidation across lakes. Our model of αox in conjunction with the growing number of δ13C-CH4 measurements should help to elucidate large scale patterns and drivers of the oxidation-driven mitigation of CH4 emission from freshwater aquatic systems.
Data availability statement
The original contributions presented in the study are included in the article/Supplementary Material, further inquiries can be directed to the corresponding author.
Author contributions
ST and PR developed the research idea and conducted experiments. ST analyzed and plotted data and wrote the first version of the manuscript, and PR analyzed and plotted data, reviewed, and edited the manuscript. YP contributed to analyzing data, reviewed and edited the manuscript, supervised the research, and acquired funding.
Funding
This study was supported by Natural Sciences and Engineering Research Council of Canada Discovery grants to YP and is a contribution to UNESCO Chair in Global Environmental Change. ST and PR were supported by doctoral scholarships from Fonds de recherche Nature et Technologies du Québec, Canada.
Acknowledgments
We thank the reviewers for their helpful comments. We thank Katherine Velghe for the assistance in the laboratory. We also thank the staff at the Station de biologie des Laurentides (Université de Montréal) for assistance.
Conflict of interest
The authors declare that the research was conducted in the absence of any commercial or financial relationships that could be construed as a potential conflict of interest.
Publisher’s note
All claims expressed in this article are solely those of the authors and do not necessarily represent those of their affiliated organizations, or those of the publisher, the editors and the reviewers. Any product that may be evaluated in this article, or claim that may be made by its manufacturer, is not guaranteed or endorsed by the publisher.
Supplementary material
The Supplementary Material for this article can be found online at: https://www.frontiersin.org/articles/10.3389/fenvs.2022.833688/full#supplementary-material
References
Aghdam, E. F., Fredenslund, A. M., Chanton, J., Kjeldsen, P., and Scheutz, C. (2018). Determination of gas recovery efficiency at two Danish landfills by performing downwind methane measurements and stable carbon isotopic analysis. Waste Manag. 73, 220–229. doi:10.1016/j.wasman.2017.11.049
Bakkaloglu, S., Lowry, D., Fisher, R. E., France, J. L., and Nisbet, E. G. (2021). Carbon isotopic characterisation and oxidation of UK landfill methane emissions by atmospheric measurements. Waste Manag. 132, 162–175. doi:10.1016/j.wasman.2021.07.012
Barker, J. F., and Fritz, P. (1981). Carbon isotope fractionation during microbial methane oxidation. Nature 293 (5830), 289–291. doi:10.1038/293289a0
Bastviken, D., Cole, J. J., Pace, M. L., and Van de Bogert, M. C. (2008). Fates of methane from different lake habitats: Connecting whole‐lake budgets and CH4 emissions. J. Geophys. Res. 113 (G2). doi:10.1029/2007jg000608
Bastviken, D., Ejlertsson, J., and Tranvik, L. (2002). Measurement of methane oxidation in lakes: a comparison of methods. Environ. Sci. Technol. 36 (15), 3354–3361. doi:10.1021/es010311p
Bastviken, D., Tranvik, L. J., Downing, J. A., Crill, P. M., and Enrich-Prast, A. (2011). Freshwater methane emissions offset the Continental Carbon Sink. Science 331 (6013), 50. doi:10.1126/science.1196808
Begum, M. S., Bogard, M. J., Butman, D. E., Chea, E., Kumar, S., Lu, X., et al. (2021). Localized pollution impacts on greenhouse gas dynamics in three anthropogenically modified Asian river systems. J. Geophys. Res. Biogeosci. 126, e2020JG006124. doi:10.1029/2020jg006124
Bergamaschi, P., Lubina, C., Königstedt, R., Fischer, H., Veltkamp, A. C., and Zwaagstra, O. (1998). Stable isotopic signatures (δ13C, δD) of methane from European landfill sites. J. Geophys. Res. 103 (D7), 8251–8265. doi:10.1029/98jd00105
Bergamaschi, P. (1997). Seasonal variations of stable hydrogen and carbon isotope ratios in methane from a Chinese rice paddy. J. Geophys. Res. 102 (D21), 25383–25393. doi:10.1029/97jd01664
Blees, J., Niemann, H., Wenk, C. B., Zopfi, J., Schubert, C. J., Kirf, M. K., et al. (2014). Micro‐aerobic bacterial methane oxidation in the chemocline and anoxic water column of deep south‐Alpine Lake Lugano (Switzerland). Limnol. Oceanogr. 59 (2), 311–324. doi:10.4319/lo.2014.59.2.0311
Börjesson, G., Chanton, J., and Svensson, B. H. (2001). Methane oxidation in two Swedish landfill covers measured with carbon‐13 to carbon‐12 isotope ratios. J. Environ. Qual. 30 (2), 369–376. doi:10.2134/jeq2001.302369x
Börjesson, G., Samuelsson, J., and Chanton, J. (2007). Methane oxidation in Swedish landfills quantified with the stable carbon isotope technique in combination with an optical method for emitted methane. Environ. Sci. Technol. 41 (19), 6684–6690. doi:10.1021/es062735v
Cabral, A. R., Capanema, M. A., Gebert, J., Moreira, J. F., and Jugnia, L. B. (2010). Quantifying microbial methane oxidation efficiencies in two experimental landfill biocovers using stable isotopes. Water Air Soil Pollut. 209 (1), 157–172. doi:10.1007/s11270-009-0188-4
Cadieux, S. B., White, J. R., Sauer, P. E., Peng, Y., Goldman, A. E., and Pratt, L. M. (2016). Large fractionations of C and H isotopes related to methane oxidation in Arctic lakes. Geochimica Cosmochimica Acta 187, 141–155. doi:10.1016/j.gca.2016.05.004
Cai, Y., Zheng, Y., Bodelier, P. L., Conrad, R., and Jia, Z. (2016). Conventional methanotrophs are responsible for atmospheric methane oxidation in paddy soils. Nat. Commun. 7 (1), 11728–11810. doi:10.1038/ncomms11728
Capanema, M. A., and Cabral, A. R. (2012). Evaluating methane oxidation efficiencies in experimental landfill biocovers by mass balance and carbon stable isotopes. Water Air Soil Pollut. 223 (9), 5623–5635. doi:10.1007/s11270-012-1302-6
Chanton, J., Abichou, T., Langford, C., Hater, G., Green, R., Goldsmith, D., et al. (2011). Landfill methane oxidation across climate types in the US. Environ. Sci. Technol. 45 (1), 313–319. doi:10.1021/es101915r
Chanton, J., and Liptay, K. (2000). Seasonal variation in methane oxidation in a landfill cover soil as determined by an in situ stable isotope technique. Glob. Biogeochem. Cycles 14 (1), 51–60. doi:10.1029/1999gb900087
Chanton, J. P., Powelson, D. K., Abichou, T., Fields, D., and Green, R. (2008). Effect of temperature and oxidation rate on carbon-isotope fractionation during methane oxidation by landfill cover materials. Environ. Sci. Technol. 42 (21), 7818–7823. doi:10.1021/es801221y
Chanton, J. P., Powelson, D. K., and Green, R. B. (2009). Methane oxidation in landfill cover soils, is a 10% default value reasonable? J. Environ. Qual. 38 (2), 654–663. doi:10.2134/jeq2008.0221
Coleman, D. D., Risatti, J. B., and Schoell, M. (1981). Fractionation of carbon and hydrogen isotopes by methane-oxidizing bacteria. Geochimica Cosmochimica Acta 45 (7), 1033–1037. doi:10.1016/0016-7037(81)90129-0
Crevecoeur, S., Ruiz‐González, C., Prairie, Y. T., and Del Giorgio, P. A. (2019). Large‐scale biogeography and environmental regulation of methanotrophic bacteria across boreal inland waters. Mol. Ecol. 28 (18), 4181–4196. doi:10.1111/mec.15223
D'Ambrosio, S. L., and Harrison, J. A. (2021). Methanogenesis exceeds CH 4 consumption in eutrophic lake sediments. Limnol. Oceanogr. Lett. 6 (4), 173–181. doi:10.1002/lol2.10192
De Visscher, A., De Pourcq, I., and Chanton, J. (2004). Isotope fractionation effects by diffusion and methane oxidation in landfill cover soils. J. Geophys. Res. 109 (D18), D18111. doi:10.1029/2004jd004857
Denfeld, B. A., Ricão Canelhas, M., Weyhenmeyer, G. A., Bertilsson, S., Eiler, A., and Bastviken, D. (2016). Constraints on methane oxidation in ice‐covered boreal lakes. J. Geophys. Res. Biogeosci. 121 (7), 1924–1933. doi:10.1002/2016jg003382
Dumestre, J. F., Guézennec, J., Galy-Lacaux, C., Delmas, R., Richard, S., and Labroue, L. (1999). Influence of light intensity on methanotrophic bacterial activity in Petit Saut Reservoir, French Guiana. Appl. Environ. Microbiol. 65 (2), 534–539. doi:10.1128/aem.65.2.534-539.1999
Feisthauer, S., Vogt, C., Modrzynski, J., Szlenkier, M., Krüger, M., Siegert, M., et al. (2011). Different types of methane monooxygenases produce similar carbon and hydrogen isotope fractionation patterns during methane oxidation. Geochimica Cosmochimica Acta 75 (5), 1173–1184. doi:10.1016/j.gca.2010.12.006
Fjelsted, L., Christensen, A. G., Larsen, J. E., Kjeldsen, P., and Scheutz, C. (2020). Closing the methane mass balance for an old closed Danish landfill. Waste Manag. 102, 179–189. doi:10.1016/j.wasman.2019.10.045
Gebert, J., and Streese-Kleeberg, J. (2017). Coupling stable isotope analysis with gas push‐pull tests to derive in situ values for the fractionation factor αox associated with the microbial oxidation of methane in soils. Soil Sci. Soc. Am. J. 81 (5), 1107–1114. doi:10.2136/sssaj2016.11.0387
Grasset, C., Sobek, S., Scharnweber, K., Moras, S., Villwock, H., Andersson, S., et al. (2020). The CO2‐equivalent balance of freshwater ecosystems is non‐linearly related to productivity. Glob. Chang. Biol. 26 (10), 5705–5715. doi:10.1111/gcb.15284
Guérin, F., and Abril, G. (2007). Significance of pelagic aerobic methane oxidation in the methane and carbon budget of a tropical reservoir. J. Geophys. Res. 112 (G3). doi:10.1029/2006jg000393
Guggenheim, C., Freimann, R., Mayr, M. J., Beck, K., Wehrli, B., and Bürgmann, H. (2020). Environmental and microbial interactions shape methane-oxidizing bacterial communities in a stratified lake. Front. Microbiol. 11, 579427. doi:10.3389/fmicb.2020.579427
Happell, J. D., Chanton, J. P., and Showers, W. S. (1994). The influence of methane oxidation on the stable isotopic composition of methane emitted from Florida swamp forests. Geochimica Cosmochimica Acta 58 (20), 4377–4388. doi:10.1016/0016-7037(94)90341-7
Itoh, M., Kobayashi, Y., Chen, T. Y., Tokida, T., Fukui, M., Kojima, H., et al. (2015). Effect of interannual variation in winter vertical mixing on CH4 dynamics in a subtropical reservoir. J. Geophys. Res. Biogeosci. 120 (7), 1246–1261. doi:10.1002/2015jg002972
Itoh, M., Kojima, H., Ho, P. C., Chang, C. W., Chen, T. Y., Hsiao, S. S. Y., et al. (2017). Integrating isotopic, microbial, and modeling approaches to understand methane dynamics in a frequently disturbed deep reservoir in Taiwan. Ecol. Res. 32, 861–871. doi:10.1007/s11284-017-1502-z
Jahnke, L. L., Summons, R. E., Hope, J. M., and Des Marais, D. J. (1999). Carbon isotopic fractionation in lipids from methanotrophic bacteria II: The effects of physiology and environmental parameters on the biosynthesis and isotopic signatures of biomarkers. Geochimica Cosmochimica Acta 63 (1), 79–93. doi:10.1016/s0016-7037(98)00270-1
Kankaala, P., Taipale, S., Nykänen, H., and Jones, R. I. (2007). Oxidation, efflux, and isotopic fractionation of methane during autumnal turnover in a polyhumic, boreal lake. J. Geophys. Res. 112 (G2), G02033. doi:10.1029/2006jg000336
Kashi, N. N., Hobbie, E. A., Varner, R. K., Wymore, A. S., Ernakovich, J. G., and Giesler, R. (2022). Nutrients alter methane production and oxidation in a thawing permafrost mire. Ecosystems, 1–16. doi:10.1007/s10021-022-00758-5
King, G. M. (1992). “Ecological aspects of methane oxidation, a key determinant of global methane dynamics,” in Advances in microbial ecology. Editor K. C. Marshall (Boston, MA: Springer), 12, 431–468.
King, S. L., Quay, P. D., and Lansdown, J. M. (1989). The 13C/12 C Kinetic isotopic effect for soil oxidation of methane at ambient atmospheric concentrations. J. Geophys. Res. 94 (18), 273277–273318.
Komiya, S., Yazaki, T., Kondo, F., Katano, K., Lavric, J. V., McTaggart, I., et al. (2020). Stable carbon isotope studies of CH4 dynamics via water and plant pathways in a tropical Thai paddy: Insights into diel CH4 transportation. J. Geophys. Res. Biogeosci. 125 (9), e2019JG005112. doi:10.1029/2019jg005112
Li, S., Chen, Y., Yu, F., Zhang, Y., Liu, K., Zhuo, X., et al. (2022). Reducing methane emission by promoting its oxidation in rhizosphere through nitrogen-induced root growth in paddy fields. Plant Soil 474, 541–560. doi:10.1007/s11104-022-05360-1
Liptay, K., Chanton, J., Czepiel, P., and Mosher, B. (1998). Use of stable isotopes to determine methane oxidation in landfill cover soils. J. Geophys. Res. 103 (D7), 8243–8250. doi:10.1029/97jd02630
Madigan, M. T., Martinko, J. M., Stahl, D. A., and Clark, D. P. (2011). Brock biology of microorganisms. 13th ed. San Francisco, CA: Pearson Benjamin Cummings.
Mahieu, K., De Visscher, A., Vanrolleghem, P. A., and Van Cleemput, O. (2006). Carbon and hydrogen isotope fractionation by microbial methane oxidation: Improved determination. Waste Manag. 26 (4), 389–398. doi:10.1016/j.wasman.2005.11.006
Morana, C., Borges, A. V., Roland, F. A. E., Darchambeau, F., Descy, J.-P., and Bouillon, S. (2015). Methanotrophy within the water column of a large meromictic tropical lake (Lake Kivu, East Africa). Biogeosciences 12, 2077–2088. doi:10.5194/bg-12-2077-2015
Murase, J., and Sugimoto, A. (2005). Inhibitory effect of light on methane oxidation in the pelagic water column of a mesotrophic lake (Lake Biwa, Japan). Limnol. Oceanogr. 50 (4), 1339–1343. doi:10.4319/lo.2005.50.4.1339
Naguib, M. (1976). Stoichiometry of methane oxidation in the methane-oxidizing strain M102 under the influence of various CH4/O2 mixtures. Z. Allg. Mikrobiol. 16, 437–444. doi:10.1002/jobm.3630160604
Newton, R. J., Jones, S. E., Eiler, A., McMahon, K. D., and Bertilsson, S. (2011). A guide to the natural history of freshwater lake bacteria. Microbiol. Mol. Biol. Rev. 75 (1), 14–49. doi:10.1128/mmbr.00028-10
Nihous, G. C., Obersky, L., Rafiee, R., Cabral, A. R., Golding, S. D., and Clarke, W. P. (2010). Notes on the temperature dependence of carbon isotope fractionation by aerobic CH4-oxidising bacteriaMethodology to determine the extent of anaerobic digestion, composting and CH4 oxidation in a landfill environment. Isotopes Environ. health studies Waste Manag. 4676 (2), 133364–140373. doi:10.1016/j.wasman.2018.02.029
Nijman, T., Amado, A. M., Bodelier, P. L., and Veraart, A. J. (2022). Relief of phosphate limitation stimulates methane oxidation. Front. Environ. Sci. 10, 202. doi:10.3389/fenvs.2022.804512
Nijman, T. P. A., Davidson, T. A., Weideveld, S. T. J., Audet, J., Esposito, C., Levi, E. E., et al. (2021). Warming and eutrophication interactively drive changes in the methane-oxidizing community of shallow lakes. ISME Commun. 1, 32–36. doi:10.1038/s43705-021-00026-y
Patton, C., and Kryskalla, J. (2003) Methods of analysis by the U.S. Geological Survey National Water Quality Laboratory – evaluation of alakaline persulfate digestion as an alternative to Kjedahl digestion for determmination of total and dissolved nitrogen and phosphorus. Water-Resources Investigations Report 2003-4174
Obersky, L., Rafiee, R., Cabral, A. R., Golding, S. D., and Clarke, W. P. (2018) Methodology to determine the extent of anaerobic digestion, composting and CH4 oxidation in a landfill environment. Waste Manag. 76, 364–373. doi:10.1016/j.wasman.2018.02.029
Preuss, I., Knoblauch, C., Gebert, J., and Pfeiffer, E. M. (2013). Improved quantification of microbial CH4 oxidation efficiency in arctic wetland soils using carbon isotope fractionation. Biogeosciences 10 (4), 2539–2552. doi:10.5194/bg-10-2539-2013
Reeburgh, W. S. (2007). Oceanic methane biogeochemistry. Chem. Rev. 107 (2), 486–513. doi:10.1021/cr050362v
Reis, P. C. J., Thottathil, S. D., and Prairie, Y. T. (2022). The role of methanotrophy in the microbial carbon metabolism of temperate lakes. Nat. Commun. 13 (1), 43–49. doi:10.1038/s41467-021-27718-2
Reis, P. C. J., Thottathil, S. D., Ruiz‐González, C., and Prairie, Y. T. (2020). Niche separation within aerobic methanotrophic bacteria across lakes and its link to methane oxidation rates. Environ. Microbiol. 22 (2), 738–751. doi:10.1111/1462-2920.14877
Ren, L., Jeppesen, E., He, D., Wang, J., Liboriussen, L., Xing, P., et al. (2015). pH influences the importance of niche-related and neutral processes in lacustrine bacterioplankton assembly. Appl. Environ. Microbiol. 81 (9), 3104–3114. doi:10.1128/aem.04042-14
Rinta, P., Bastviken, D., van Hardenbroek, M., Kankaala, P., Leuenberger, M., Schilder, J., et al. (2015). An inter-regional assessment of concentrations and δ 13 C values of methane and dissolved inorganic carbon in small European lakes. Aquat. Sci. 77 (4), 667–680. doi:10.1007/s00027-015-0410-y
Rudd, J. W., Furutani, A., Flett, R. J., and Hamilton, R. D. (1976). Factors controlling methane oxidation in shield lakes: The role of nitrogen fixation and oxygen concentration 1. Limnol. Oceanogr. 21 (3), 357–364. doi:10.4319/lo.1976.21.3.0357
Samad, M. S., and Bertilsson, S. (2017). Seasonal variation in abundance and diversity of bacterial methanotrophs in five temperate lakes. Front. Microbiol. 8, 142. doi:10.3389/fmicb.2017.00142
Saunois, M., Bousquet, P., Poulter, B., Peregon, A., Ciais, P., Canadell, J. G., et al. (2016). The global methane budget 2000-2012. Earth Syst. Sci. Data 8, 697–751. doi:10.5194/essd-8-697-2016
Sawakuchi, H. O., Bastviken, D., Sawakuchi, A. O., Ward, N. D., Borges, C. D., Tsai, S. M., et al. (2016). Oxidative mitigation of aquatic methane emissions in large Amazonian rivers. Glob. Chang. Biol. 22 (3), 1075–1085. doi:10.1111/gcb.13169
Sawakuchi, H. O., Martin, G., Peura, S., Bertilsson, S., Karlsson, J., and Bastviken, D. (2021). Phosphorus regulation of methane oxidation in water from ice‐covered lakes. JGR. Biogeosciences 126 (9), e2020JG006190. doi:10.1029/2020jg006190
Schubert, C. J., Lucas, F. S., Durisch-Kaiser, E., Stierli, R., Diem, T., Scheidegger, O., et al. (2010). Oxidation and emission of methane in a monomictic lake (Rotsee, Switzerland). Aquat. Sci. 72 (4), 455–466. doi:10.1007/s00027-010-0148-5
Serrano-Silva, N., Sarria-Guzmán, Y., Dendooven, L., and Luna-Guido, M. (2014). Methanogenesis and methanotrophy in soil: a review. Pedosphere 24 (3), 291–307. doi:10.1016/s1002-0160(14)60016-3
Sievert, C. (2020). Interactive web-based data visualization with R, plotly, and shiny. Florida: Chapman and Hall/CRC.
Soued, C., and Prairie, Y. T. (2022). Patterns and regulation of hypolimnetic CO2 and CH4 in a tropical reservoir using a process‐based modeling approach. JGR. Biogeosciences 127, e2022JG006897. doi:10.1029/2022jg006897
Sparrow, K. J., Chanton, J. P., Green, R. B., Scheutz, C., Hater, G. R., Wilson, L. C., et al. (2019). Stable isotopic determination of methane oxidation: When smaller scales are better. Waste Manag. 97, 82–87. doi:10.1016/j.wasman.2019.07.032
Templeton, A. S., Chu, K. H., Alvarez-Cohen, L., and Conrad, M. E. (2006). Variable carbon isotope fractionation expressed by aerobic CH 4-oxidizing bacteria. Geochimica Cosmochimica Acta 70 (7), 1739–1752. doi:10.1016/j.gca.2005.12.002
Thottathil, S. D., Reis, P. C., del Giorgio, P. A., and Prairie, Y. T. (2018). The extent and regulation of summer methane oxidation in northern lakes. J. Geophys. Res. Biogeosci. 123 (10), 3216–3230. doi:10.1029/2018jg004464
Thottathil, S. D., Reis, P. C., and Prairie, Y. T. (2019). Methane oxidation kinetics in northern freshwater lakes. Biogeochemistry 143 (1), 105–116. doi:10.1007/s10533-019-00552-x
Tyler, S. C., Bilek, R. S., Sass, R. L., and Fisher, F. M. (1997). Methane oxidation and pathways of production in a Texas paddy field deduced from measurements of flux, δl3C, and δD of CH4. Glob. Biogeochem. Cycles 11 (3), 323–348. doi:10.1029/97gb01624
Tyler, S. C., Crill, P. M., and Brailsford, G. W. (1994). Fractionation of methane during oxidation in a temperate forested soil. Geochimica Cosmochimica Acta 58 (6), 1625–1633. doi:10.1016/0016-7037(94)90564-9
Venkiteswaran, J. J., and Schiff, S. L. (2005). Methane oxidation: isotopic enrichment factors in freshwater boreal reservoirs. Appl. Geochem. 20 (4), 683–690. doi:10.1016/j.apgeochem.2004.11.007
Whiticar, M. J. (1999). Carbon and hydrogen isotope systematics of bacterial formation and oxidation of methane. Chem. Geol. 161 (1), 291–314. doi:10.1016/s0009-2541(99)00092-3
Wilke, C. O., Wickham, H., and Wilke, M. C. O. (2019). Package ‘cowplot’. Streamlined plot theme and plot annotations for ‘ggplot2. R package version 1.0.0. Available at: https://CRAN.R-project.org/package=cowplot.
Yamamoto, S., Alcauskas, J. B., and Crozier, T. E. (1976). Solubility of methane in distilled water and seawater. J. Chem. Eng. Data 21 (1), 78–80. doi:10.1021/je60068a029
Zhang, G., Ji, Y., Ma, J., Xu, H., Cai, Z., and Yagi, K. (2012). Intermittent irrigation changes production, oxidation, and emission of CH4 in paddy fields determined with stable carbon isotope technique. Soil Biol. Biochem. 52, 108–116. doi:10.1016/j.soilbio.2012.04.017
Keywords: methane, aerobic oxidation, isotopic mass balance, fractionation factor, lakes
Citation: Thottathil SD, Reis PCJ and Prairie YT (2022) Variability and controls of stable carbon isotopic fractionation during aerobic methane oxidation in temperate lakes. Front. Environ. Sci. 10:833688. doi: 10.3389/fenvs.2022.833688
Received: 12 December 2021; Accepted: 06 September 2022;
Published: 20 September 2022.
Edited by:
Laodong Guo, University of Wisconsin–Milwaukee, United StatesReviewed by:
Mark Alexander Lever, ETH Zürich, SwitzerlandGuangbin Zhang, Institute of Soil Science (CAS), China
Copyright © 2022 Thottathil, Reis and Prairie. This is an open-access article distributed under the terms of the Creative Commons Attribution License (CC BY). The use, distribution or reproduction in other forums is permitted, provided the original author(s) and the copyright owner(s) are credited and that the original publication in this journal is cited, in accordance with accepted academic practice. No use, distribution or reproduction is permitted which does not comply with these terms.
*Correspondence: Shoji D. Thottathil, c2hvamkudGhvdHRhdGhpbEBnbWFpbC5jb20=
†Present address: Paula C. J. Reis, Institut National de la Recherche Scientifique—Eau Terre Environnement, Québec City, Canada
‡These authors have contributed equally to this work and share first authorship