- Center for Food Safety and Applied Nutrition, Food and Drug Administration, College Park, MD, United States
Leafy greens are responsible for nearly half of the produce-related Shiga toxin-producing Escherichia coli (STEC) outbreaks in the United States and recent investigations have implicated agricultural water as a potential source. Current FDA detection protocols require extensive analysis time. We aimed to use Oxford Nanopore rapid sequencing kits for an in-field determination of agricultural water microbiome and possible detection and characterization of STECs strain(s) in these samples. We tested the performance of the nanopore rapid sequencing kit (RAD004) for fast microbiome determination using the well characterized ZymoBIOMICS mock microbial community and the number of reads for each identified species was present in the expected proportion. Rapid sequencing kit (LRK001 and RAD004) library preparation of DNA extracted from agricultural water resulted in poor nanopore sequencing reactions, with low output (0.3–1.7 M reads), a high proportion of failed reads (50–60%), and highly sheared DNA before and after a magnetic bead clean up. To improve performance, we prepared a DNA library with the ligation kit (LSK109), which includes multiple cleaning steps, reducing inherent inhibitors and producing a better outcome (2.2 M reads, 15% failed reads). No definitive presence of STEC could be confirmed in any of the sites. Approximately 100 reads from each site (0.02% of total reads) were identified as Escherichia coli, but the specific strain or their virulence genes could not be detected. Sites 9, 10, and 12 were found to be positive for STEC presence by microbiological techniques after enrichment. The rapid sequencing kits can be appropriate for genus or species level microbial identification, but we recommend the use of the ligation kit for increased sequencing depth and removal of contaminants in agricultural water. However, we were not able to identify any STEC strains in these nanopore microbiome samples, due to low initial concentrations. The results from this pilot study provide preliminary evidence that MinION sequencing of agricultural water using the ligation kit has the potential to be used for rapid microbiome determination in the field with optimal results for water quality surveillance.
Introduction
Shiga toxin-producing Escherichia coli (STEC) is a foodborne pathogen responsible for approximately 265,000 illnesses per year in the United States (Scallan et al., 2011). STEC infection can cause severe disease, including bloody diarrhea and hemolytic uremic syndrome (HUS) (Tarr et al., 2005; Mellmann et al., 2008; Beutin and Martin, 2012; Gonzalez-Escalona et al., 2019a). STECs are defined by the presence of Shiga toxin genes (stx) and are identified by serotype based on their O and H antigens. While the most common STEC associated with outbreaks and illness is E. coli O157:H7 (Mead et al., 1999; Allos et al., 2004; Scallan et al., 2011), there are over 400 STEC serotypes with varying degrees of pathogenicity, which can be determined in silico by the presence of virulence genes (Gonzalez-Escalona et al., 2019a; National Advisory Committee on Microbiological Criteria for Foods, 2019). Attachment and colonization genes can be found in the locus of enterocyte effacement (LEE), including intimin (eae) and type 3 secretion system (TTSS) effector proteins (esp, esc, tir), Additional non-LEE effectors (nleA, nleB, nleC) and other putative virulence genes (ehxA, etpD, subA, toxB, saa) can also impact virulence (Kaper et al., 2004; Garmendia et al., 2005; Gonzalez-Escalona et al., 2019a; Gonzalez-Escalona and Kase, 2019). It is, therefore, imperative that the serotype and virulence factors are identified to assess potential pathogenicity.
STEC infections have been linked to multiple commodities (e.g., beef, milk, yogurt), including a growing incidence in produce (Olaimat and Holley, 2012; Fischer et al., 2015; Tack et al., 2020), especially leafy greens, with agricultural water implicated as a potential source (Steele and Odumeru, 2004; Uyttendaele et al., 2015; Monaghan and Hutchison, 2012; Oliveira et al., 2012; Allende and Monaghan, 2015; Author Anonymous, 2018; FDA, 2018). Agricultural water can be contaminated by adjacent land use, wild animal activity, or incomplete water sanitization (Uyttendaele et al., 2015). There are currently no approved antimicrobial treatments for agricultural water to prevent against foodborne pathogens; however the FDA has collaborated with the EPA to establish a new protocol for development and registration of treatment of agricultural water (FDA, 2020b). This protocol was developed under the 2020 Leafy Greens STEC Action Plan in which the FDA is focused on improving the safety of leafy greens through a set of guidelines, including extensive meta-analysis of past outbreak data, longitudinal studies, promotion of tech-enabled traceability, monitoring of nearby agricultural land use, compost sampling, and improved whole genome sequencing (WGS) tracing (FDA, 2020a).
WGS has increased the precision and responsiveness of food safety by the ability to produce closed genomes, determining serotype, virulence, antimicrobial resistance, and phylogenetic relationships, particularly during an outbreak (Gonzalez-Escalona et al., 2016; Hoffmann et al., 2016; Gonzalez-Escalona et al., 2019b; Brown et al., 2019; Gonzalez-Escalona and Kase, 2019). While single colony isolation and WGS are the current standard procedure for FDA STEC detection and classification (FDA Bacteriological Analytical Manual Chapter 4A (FDA, 2019), these reporting methods require approximately 2 weeks of analysis time. U.S. Federal regulatory action will continue to require a single isolate, but metagenomic, culture-independent methods are being tested for expedited detection and characterization (Loman et al., 2013; Huang et al., 2017; Brown et al., 2019). Retrospective analysis of clinical fecal samples from E. coli O104:H4 and Salmonella enterica outbreaks have shown promising results in detecting and characterizing the outbreak strain (Loman et al., 2013; Huang et al., 2017) Targeted microbial detection by metagenomic analysis using 16S rRNA profiling or shotgun metagenomic sequencing is increasingly being used (Leonard et al., 2015; Leonard et al., 2016; Kovac et al., 2017; Gigliucci et al., 2018; Lusk Pfefer et al., 2018; Ottesen et al., 2020). Mock microbial communities have been used in long read metagenomic studies and have shown the capability of obtaining closed metagenome assembled genomes (MAGs) for high concentration microbial species (Boykin et al., 2019; Nicholls et al., 2019; Moss et al., 2020). Library preparation with the Oxford Nanopore ligation kit (SQK-LSK109) produces approximately 10–20 Gb of sequencing data. In a study by Nicholls, et al. (2019) sequencing of the ZymoBIOMICS Mock Microbial Community resulted in more than 150X coverage for each of the 8 bacterial species. Long read sequencing is particularly useful in assembling complex, highly repetitive regions that can extend for hundreds of kilobases (Bertrand et al., 2019).
Oxford Nanopore has developed portable rapid sequencing kits that have less stringent storage conditions and require minimal time and equipment (LRK001 and RAD004). Combined with a culture-independent, metagenomics approach, these kits may be useful tools for the characterization of agricultural water microbiome. We aimed to test the efficacy of these rapid kits by using a mock microbial community and design a pilot study for a fast, field-based method for microbial analysis, including the detection and characterization of STECs in agricultural water.
Results
Characterization of a bacterial community standard using the RAD004 rapid sequencing kit
The first step in our investigation for the use of the rapid sequencing kit (RAD004) for fast taxonomic classification of the microbial composition of a sample was to test the performance of our proposed workflow (nanopore sequencing using the RAD004 library preparation followed by WIMP classification tool) with a known microbial standard (ZymoBIOMICS Microbial Community DNA Standard, Zymo Research). The successful characterization of the same microbial standard, using the same instrument, but with a different DNA library preparation, the ligation sequencing kit LSK109, and analysis pipeline has been demonstrated earlier (Nicholls et al., 2019). There are some fundamental differences between these two DNA library preparation methods: 1) DNA gets more fragmented in the rapid kit, 2) there is potential loss of DNA in the ligation kit because of several DNA cleaning steps, and 3) the ligation kit is composed of several more steps than the rapid sequencing kit. All of these could affect the microbial profile of a sample, the speed of the sequencing, and in-field usage of the nanopore sequencing device. As shown in Figure 1 and Table 1, the preparation of the DNA library using the RAD004 kit followed by WIMP analysis resulted in a correct classification of the composition of the mock community across different sequencing intervals (https://epi2me.nanoporetech.com/shared-report-226486?tokenv2=a812d53e-7d47-4294-975c-3550fd037336). This experiment showed that the RAD004 kit could be used as efficiently as the ligation kit (LSK109) for determining the microbial composition of a sample, albeit with lower output.
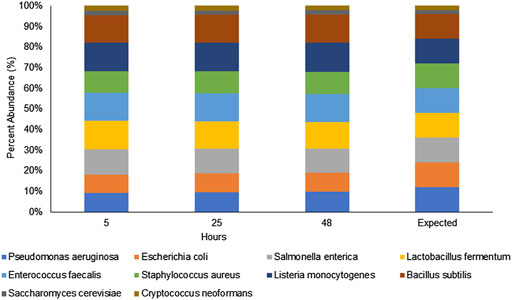
FIGURE 1. Percentage of microbial composition observed by WIMP from nanopore sequencing of the ZymoBIOMICS microbial community DNA standard using the RAD004 kit at different time intervals (5, 24 and 48 h) showing that the composition remained stable across those tested time frames and in a similar composition to the expected proportions.
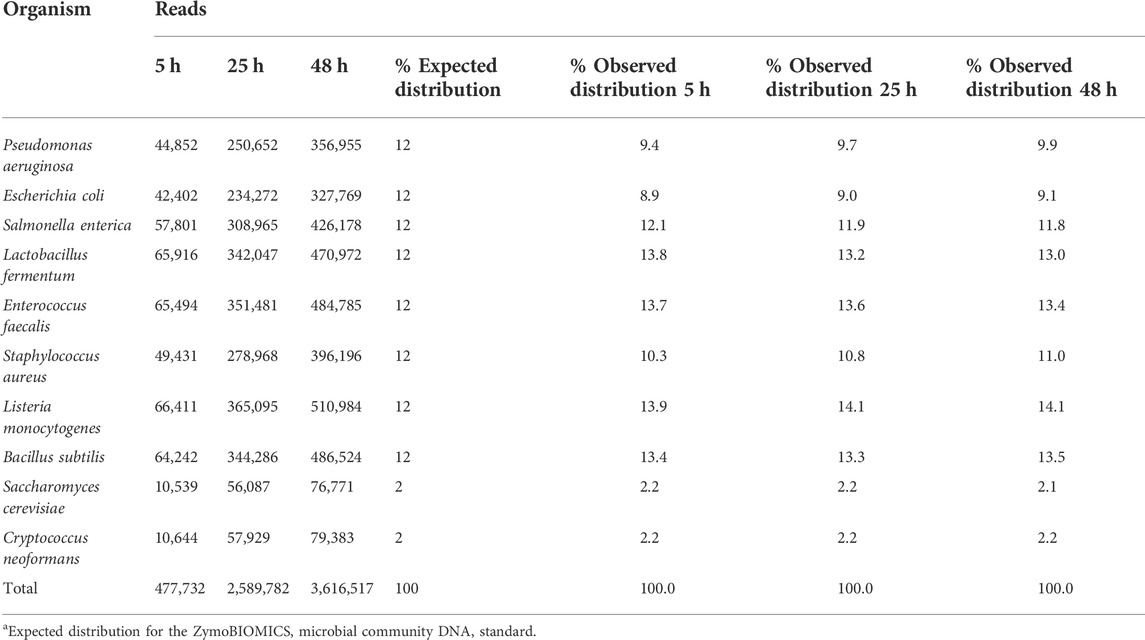
TABLE 1. Summary of the WIMP output for the nanopore sequencing of the ZymoBIOMICS microbial community DNA standard using the RAD004 kit at different time intervals (5, 24 and 48 h). Total number of reads identified for each microorganism and their expected percentage distribution in the sample.
Testing metagenomic characterization of agricultural water using nanopore rapid sequencing kits
Our original goal for this project was to test 1) the on-site, fast characterization of the bacterial composition and 2) detection of STECs in culture-independent, concentrated agricultural water samples (Figure 2) using the Oxford Nanopore hand-held MinION and two versions of DNA library preparation kits (RAD004 rapid sequencing kit and LRK001 field sequencing kit). DNA extraction of each sample (10 ml) resulted in 2.5 ug total DNA per sample. A DNA library for sample 26 was prepared using the RAD004 kit and resulted in a non-productive sequencing reaction with a low output (388,130 reads and 0.4 Gb yield) (Supplementary Additional File S1). Pore availability at the onset was modest (70%) with only ∼40% of pores actively sequencing and steadily declined over the first 24 h. Almost 60% of the total reads did not pass the quality filter (Supplementary Additional File S1). Of the reads that passed the quality filter, a large majority were less than 1,000 bp (103,235 reads) and taxonomy was classified by WIMP for about 45% of them (https://epi2me.nanoporetech.com/shared-report-243787?tokenv2=934deea4-2201-4e0d-bf82-d42c3a03078b). The rapid sequencing kit does not contain a DNA cleaning step and requires the highest DNA quality to maintain optimal performance. The poor performance of sample 26 suggested that the sample contained an inhibitor or other interference with proper sequencing.
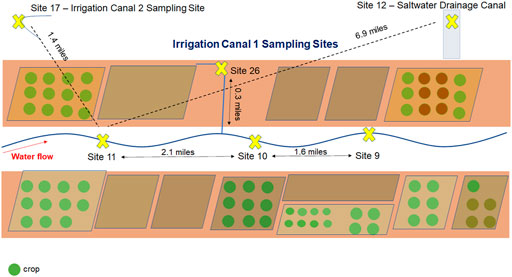
FIGURE 2. Map of sampling sites. Agricultural water samples were collected along irrigation canals and a saltwater drainage canal in the Southwestern United States. The relative (solid) and direct (dotted) distance between sampling sites 9, 10, 11, 12, 17, and 26 are shown.
In order to reduce or eliminate this inhibition, samples 17 and 26 were further cleaned with an Agencourt magnetic bead cleaning step (as described in Methods) and prepared for sequencing using the LRK001 or RAD004 kit. The cleaning resulted in a loss of 40% of the total DNA and the sequencing continued to show inhibition, although less pronounced and with better results (Figure 3). The sequencing run with the LRK001 kit for sample 26 showed a rapid decay of the sequencing pores in less than 24 h, resulted in low read output (322,000 reads and 0.4 Gb yield) (Figure 3A), and only 50% of reads passing the quality filter (Figure 3B). A similar result was obtained with the RAD004 kit for the same sample 26, but with a higher read output (1,700,000 reads and 1.8 Gb yield) (Figure 3C) and a slight increase in the number of reads passing the quality filter (Figure 3D). Nevertheless, the majority of the read sizes were below 1,000 base pairs in length (∼560,000 reads) which resulted in more than 86% of the reads being unclassified (https://epi2me.nanoporetech.com/shared-report-214408?tokenv2=9ed5fce3-da1c-434c-a388-5d98953a7e1c). The DNA was highly sheared due to the Agencourt cleaning and the use of the rapid sequencing kit. Sequencing of sample 17 using the RAD004 kit showed similar results. The total read output was 1,680,000 reads with 67% of reads passing the quality filter and 23% of those reads were classified by WIMP. Additionally, more than 430,000 reads were under 1,000 base pairs in length (https://epi2me.nanoporetech.com/shared-report-214241?tokenv2=e55ce2ae-6d63-4dd4-a1a5-dd3c4f0c567a).
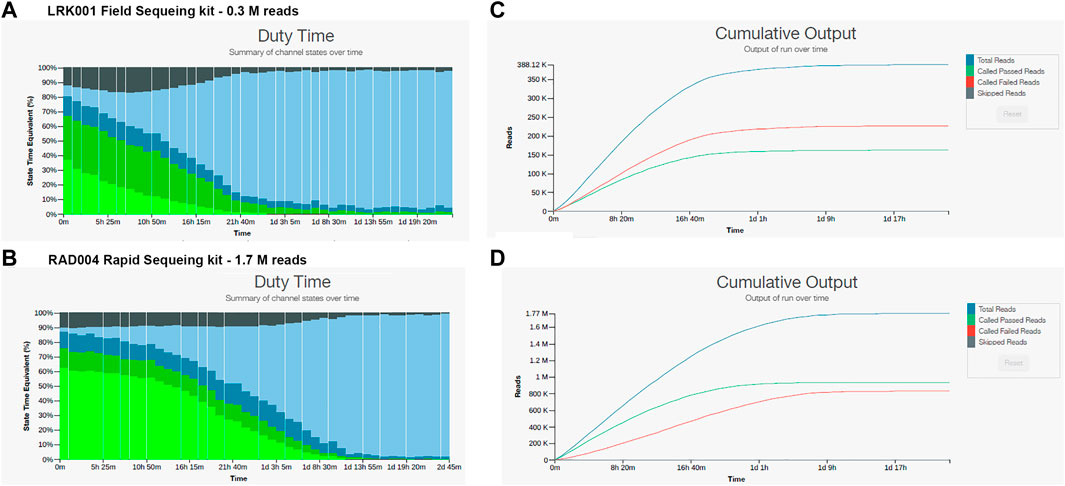
FIGURE 3. Nanopore sequencing outputs for sample 26 using the LKR001 field sequencing kit and the RAD004 rapid sequencing kit. (A) Pore sequencing and availability showing low pore availability and pore death after 16 h. (B) Cumulative output of the LRK001 kit showing that reads passing filter were equal to the reads not passing filter indicative of problems with the sequencing. (C) Pore availability for the RAD004 kit showing that the pores decayed by 20 h of sequencing. (D) Cumulative output for the RAD004 kit, with a similar problem with the read quality with 50% passing filter.
Use of the LSK109 ligation kit for eliminating agricultural water inhibitors
Because we found that the field sequencing kit and the rapid sequencing kit did not produce satisfactory results for our field application, we decided to test the ligation kit (LSK109) on the DNA obtained from the sample from site 9. The ligation kit has several advantages over the rapid sequencing kits such as: higher output, no enzymatic shearing, and with several cleaning steps the inhibitors will diminish to levels that would not interfere with the sequencing reaction. The drawback was that it takes almost 90 min for sample preparation compared to the 15 min required for the rapid sequencing or field sequencing kits and it contains more steps where sample can be lost. Testing produced promising results with 2,210,000 reads and 8.45 Gb yield with more than 60% of pores sequencing over the 24-hour sequencing run. Furthermore, over 85% of the reads passed the quality filter (Figure 4). We decided to process three additional samples (site 10, 11, and 12) with the same ligation kit and compare their taxonomic composition to conduct a baseline metagenomic survey of samples collected across 3.7 contiguous miles in the Southwestern United States (Figure 2).
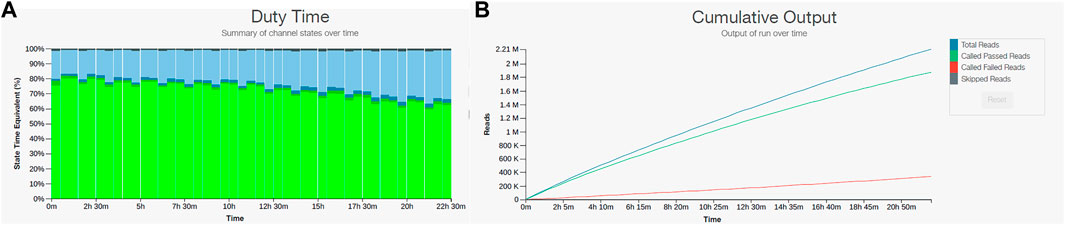
FIGURE 4. Nanopore sequencing output for sample 9 using the LSK109 ligation kit. (A) Pore sequencing and availability remained above 60% for the 24-hour sequencing run. (B) Cumulative output of the LSK109 kit showed more than 85% of total reads passed the quality filter.
Agricultural water metagenomic taxonomic characterization. Each run produced an average of 2,200,000 reads with an average total yield of 8.5 Gb (Supplementary Additional File S2). The base-called reads were passed through a quality filter and reads above 5,000 base pairs in length were analyzed by the EPI2ME WIMP workflow. The agricultural water samples had a diverse composition. The reads were predominately bacterial (89–92%) with the remaining of eukaryotic (6–9%), viral (1–2%), and archaeal (<1%) origin (Supplementary Additional File S3) that could be organized into approximately 50 phyla, 90 classes, and 1,500 genera (Table 2).
Bacterial composition in agricultural water
We identified the bacterial genera with an abundance greater than 1% in at least one sample (Figure 5). The 11 bacterial genera identified include Synechococcus, Cyanobium, Pseudomonas, Streptomyces, Flavobacterium, Candidatus Fonsibacter, Limnohabitans, Hydrogenophaga, Acidovorax, Variovorax, and Rubrivivax. A large portion of reads (∼40%) were classified as various taxa, but the combined genus abundance was less than 1%. Sites 9, 10, and 11 displayed very similar composition with 30–40% Synechococcus, 4% Cyanobium, and 1–2% each Pseudomonas, Streptomyces, Flavobacterium, Candidatus Fonsibacter, and Limnohabitans. Site 12 is located approximately 6.9 miles from site 11 in a saltwater drainage canal and has a similar abundance of Streptomyces (1.3%), and Limnohabitans (1.3%). However, site 12 had almost no Synechococcus (0.3%), Cyanobium (0.1%), and Candidatus Fonsibacter (0.1%), win a hile, Pseudomonas (3.1%), Flavobacterium (5.9%), Hydrogenophaga (3.1%), Acidovorax (1.9%), Variovorax (1.3%), and Rubrivivax (1.2%) were each present in approximately 2–6 times the abundance as sites 9–11.
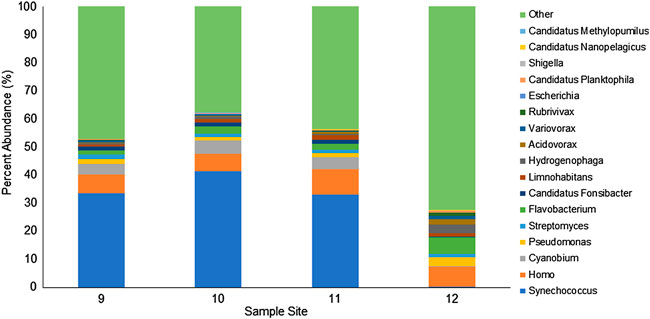
FIGURE 5. Relative abundance of WIMP identified genera for each agricultural water site. Reads were analyzed by the EPI2ME WIMP workflow. Bacterial genera contributing more than 1% of the classified reads are shown and the sum of the remaining genera identified are included as “Other.”
Eukaryotic composition in agricultural water.
Strikingly, approximately 6–9% of the total reads were identified as genus Homo. Eukaryotic DNA was represented by 19,645 to 56,173 reads (Supplementary Additional File S3). Within the eukaryotic reads, approximately 98% of reads were identified as Homo sapiens, with the other 2% being largely fungal in origin. Thus, the fungal composition of the agricultural water was minimal.
Detection of STECs
Each sample site was analyzed for the presence of Shiga toxin-producing E. coli by the FDA BAM Chapter 4A methods. Sites 9, 10, and 12 were confirmed to be STEC positive after enrichment. Contrary to these results, the WIMP analysis of the nanopore sequencing output of the unenriched agricultural water revealed that between 46–152 reads were identified as E. coli. The strain-level identification further classified one read from site 11 and 2 reads from site 12 as the O157 serotype. An NCBI BLAST search of those individual reads revealed that only the read from site 11 matched the O157:H7 genome. Due to the limited coverage, strain level identification could not be obtained. Therefore, the concentration of E. coli in unenriched agricultural water samples was not sufficient for the detection of STECs or E. coli O157:H7 by direct nanopore sequencing.
Discussion
Agricultural water has been implicated in the contamination of produce-related foodborne illness and outbreaks (Steele and Odumeru, 2004; Uyttendaele et al., 2015; Monaghan and Hutchison, 2012; Oliveira et al., 2012; Allende and Monaghan, 2015; Author Anonymous, 2018). Current FDA protocols for the detection and isolation of STECs require multiple rounds of selective plating and WGS of a single isolate. On-site field testing is increasingly becoming a priority to decrease the time to detection of pathogenic microbes and prevention of prospective corrective measures. We have designed a pilot study to test nanopore sequencing methods for the fast determination of concentrated agricultural water microbiome and detection and classification of STECs. We determined that the rapid library preparation kit produced expected results for a mock microbial community but performed poorly within the agricultural water matrix. The DNA library prepared with the ligation kit improved the sequencing output and the bacterial composition, however, we were unable to accurately detect STECs in concentrated agricultural water.
Mock microbial communities are standardized metagenomic samples and are typically used for benchmarking sequencing studies (McIntyre et al., 2017; Bertrand et al., 2019; Nicholls et al., 2019). In a previous work, mock microbial communities sequenced by long-read nanopore technology using the ligation library preparation kit (LSK109) produced sufficient coverage expected to close all the microbial genomes (Nicholls et al., 2019). We have tested the same community as a benchmark for the RAD004 rapid sequencing library preparation kit. The sequencing run produced a total of 4,360,159 reads with an output of 6.5 Gb and with 93,448 reads longer than 5,000 base pairs. The EPI2ME cloud-based service WIMP classifies the reads by taxon and identified each of the expected microbial species. Additionally, we showed that the read abundance, calculated as a percentage of total reads classified, was correlated with the expected microbial proportions.
Oxford Nanopore sequencing has developed rapid and field sequencing DNA library preparation kits (RAD004 and LRK001, respectively) for fast, portable sequencing efforts. These advances have allowed and encouraged researchers to develop in-field testing kits for remote regions to identify the microbial composition in metagenomic samples. Increased access to nanopore technologies can provide rapid information to these remote areas that have previously been subject to outsourcing sequencing, which can take months. Nanopore sequencing has been used for epidemiological surveillance and early detection of Zika (Faria et al., 2016) and Ebola (Quick et al., 2016) viruses. In polar environments the relationship between the changing climate and the microbial community has been of particular interest (Edwards et al., 2017; Johnson et al., 2017; Gowers et al., 2019). Nanopore sequencing has also aided the protection and maintenance of the cassava crop in Africa (Boykin et al., 2019). These successful in-field metagenomic analyses suggest that the technology can be applied to agricultural water for microbiome analysis and possibly foodborne pathogen detection and assembly.
Confident that the rapid kit has the potential to produce a sequencing output appropriate for metagenomic analysis and closing bacterial genomes, we then tested the performance of the rapid sequencing kit on concentrated agricultural water samples. Surprisingly however, nearly half of the output reads failed initial quality control standards for base-calling. The low output despite an additional Agencourt DNA cleanup step, indicated the presence of a carryover inhibitor, such as humic acid which has similar solubility to DNA and is not easily separated (Lakay et al., 2007; Wnuk et al., 2020). The protocol for the LSK109 ligation kit, however, employs additional DNA cleanup steps, which improved the sequencing output and quality of the base-called reads (Figure 4).
While the ligation kit adds time and resources to the rapid and field DNA library preparation kits, we were able to use the total reads sequencing output to identify 11 genera in the microbiome of the agricultural water. The three sites along the canal displayed remarkable similarity and we were able to distinguish these communities from an unrelated, distant site. If the microbiome remains relatively constant over a particular distance, these preliminary results suggest that we may be able to reduce the proximity of the sites and that a distance limit can be established for future baseline survey studies using this type of nanopore metagenomic analysis. Overall, this could aid in reducing the sample number and costs associated with sampling and microbiological and/or metagenomic analysis during longitudinal surveys. While the most abundant species in the microbiome is likely to fluctuate seasonally, they may be an indicator to changing populations and importantly may serve as a means to monitor for deviations in water microbial quality. Interestingly within the eukaryotic reads, most reads were identified as Homo sapiens, suggesting some human contact with the water.
The ability to detect STECs directly from agricultural water could decrease the time to detection by at least 24 h since there is no sample enrichment step. Nanopore sequencing is capable of detecting species present in as few as 50 reads or the equivalent of 4 cells (Nicholls et al., 2019). Therefore, with the high quality and increased output gained from the ligation kit, we expected to be able to accurately detect the presence of E. coli and identify STEC strains. Nanopore sequencing is, however, not typically used as a screening tool due to its high cost compared to other means of detection like qPCR. We have previously established that the limit of complete, fragmented assembly for STECs by nanopore sequencing is 105 CFU/ml (Maguire et al., 2021). This is achievable by sample enrichment where low levels of target are amplified many fold, but herewith we aimed to establish the extent to which virulotyping is possible in agricultural water. We obtained species-level detection of E. coli with less than 150 reads, but we were unable to make accurate strain-level and virulotype identification, which requires a complete genome (Leonard et al., 2016; Gonzalez-Escalona and Kase, 2019; Maguire et al., 2021).
While we applied a high standard to the agricultural water samples, the rapid and field sequencing runs produced data that could identify the microbiome community and better inform water resource managers and others that monitor agricultural water quality with regards to unexpected deviations once a baseline for their particular water source is established (Supplementary Additional File S4). The ligation kit requires additional time and equipment but produces more output (Gb yield) and produced a higher number of quality reads. This amount of data, though, was unable to detect STECs in unenriched agricultural water probably due to low levels present. Depending on the desired outcome, nanopore technology can provide high quality, informative, long reads and provides access to tools that aid in fast comprehensive analysis through the EPI2ME cloud-based service.
Oxford Nanopore’s LRK001 and RAD004 field and rapid sequencing kits can be appropriate for genus or species level identification of microorganisms that are highly abundant. However, the performance of both kits for microbiome characterization from field samples could be affected by the type of sample to be tested, resulting in low number of reads and low sequence quality. On the other hand, the LSK109 ligation kit provided adequate yield with deeper sequencing depth and better pore performance for assessing the metagenomic composition of agricultural water. We were unable to identify the presence of STEC in the sequencing reads which suggests a low E. coli concentration was present. The results from this pilot study provide preliminary evidence that MinION sequencing of agricultural water using the ligation kit has the potential to be used for rapid microbiome determination in the field with optimal results for water quality surveillance.
Materials and methods
Agricultural water collection and concentration
A 100 L of water was collected at each site (Kaper et al., 2004; Garmendia et al., 2005; Monaghan and Hutchison, 2012; Monaghan and Hutchison, 2012; Fischer et al., 2015; Gonzalez-Escalona et al., 2016; Gonzalez-Escalona and Kase, 2019) from irrigation canals in the Southwestern United States (water source is the Colorado River) (Figure 2). Since the canal (and ultimately the Colorado) is traveling for miles, there could be many anthroponotic and zoonotic inputs possible into this water source. Water samples were filtered and concentrated in the field using a Rexeed 25S Ultrafilter (Dial Medical Supply, Chester Springs, PA), with approximately 650 ml recovered upon backflush, according to the FDA BAM Dead-end Ultrafiltration method described in Chapter 19c (FDA, 2020c).
DNA extraction
DNA was extracted directly from a 10 ml aliquot from the 650 ml backflush (concentrated agricultural water). Ten aliquots of 1 ml each were centrifuged at 10,000 × g for 3 min, the supernatant was discarded, and the first pellet was resuspended in 800 μL sterile water and used to combine and resuspend the remaining nine aliquots. DNA was extracted by either the ZymoBIOMICS DNA Miniprep kit (Zymo Research, Irvine, CA) (site 26) according to manufacturer’s instructions or the Maxwell RSC Cultured Cells DNA kit with a Maxwell RSC Instrument (Promega Corporation, Madison, WI) (sites 9, 10, 11, 12, and 17) according to manufacturer’s instructions for Gram-negative bacteria with additional RNase treatment. DNA concentration was determined by Qubit 4 Fluorometer (Invitrogen, Carlsbad, CA) according to manufacturer’s instructions.
Test for accurate metagenomic identification of the ZymoBIOMICS microbial community DNA standard using the Oxford Nanopore rapid sequencing kit RAD004
The ZymoBIOMICS Microbial Community DNA Standard (Zymo Research) is composed of 8 bacteria and 2 yeasts (https://www.zymoresearch.com/collections/zymobiomics-microbial-community-standards/products/zymobiomics-microbial-community-dna-standard). Representative microorganisms contain a wide range of GC content from 15 to 85%, which allows for assessing biases that could arise because of GC content variation. The organisms in this community are distributed equally (12%), except the 2 yeasts (each present at 2%). Four microliters (400 ng) of this ZymoBIOMICS Microbial Community DNA Standard (Zymo Research) was used for preparing the DNA library for sequencing in a MinION device using the rapid sequencing kit RAD004 according to manufacturer’s instructions. The library was run in FLO-MIN106 (R9.4.1) flow cells, according to the manufacturer’s instructions for 48 h (Oxford Nanopore Technologies). The runs were live base called using Guppy v3.2.10 included in the MinKNOW v3.6.5 (v19.12.6) software (Oxford Nanopore Technologies). The run was analyzed by the “What’s in my pot” (WIMP) workflow contained in the EPI2ME cloud service (Oxford Nanopore Technologies) at 5, 25, and 48 h.
Metagenomic sequencing, contig assembly, and annotation
DNA recovered from the agricultural water samples underwent a 0.7X (v/v) Agencourt Bead clean-up (Beckman Coulter, Indianapolis, Indiana). DNA was sequenced using a MinION nanopore sequencer (Oxford Nanopore Technologies, Oxford, United Kingdom). The sequencing libraries were prepared using either the Rapid Sequencing (SQK-RAD004) (sites 26 and 17), the Field Sequencing Kit (SQK-LRK001) (sites 26 and 17), or the Genomic DNA by Ligation kit (SQK-LSK109) (sites 9, 10, 11, and 12) and run in FLO-MIN106 (R9.4.1) flow cells, according to the manufacturer’s instructions for 48 h (Oxford Nanopore Technologies). The runs were live base called using Guppy v3.2.10 included in the MinKNOW v3.6.5 (v19.12.6) software (Oxford Nanopore Technologies). The initial classification of the reads for each run was done using the “What’s in my pot” (WIMP) workflow contained in the EPI2ME cloud service (Oxford Nanopore Technologies). Reads were assessed for quality including a minimum 5,000 bp length filter.
BAM STEC detection
The presence of STEC was determined according to the protocols in Chapter 4A of the FDA Bacterial Analytical Manual (BAM) (https://www.fda.gov/food/laboratory-methods-food/bam-diarrheagenic-escherichia-coli). Briefly, 225 ml of each agricultural water sample was enriched by adding an equal volume of 2X modified Buffered Peptone Water with pyruvate (mBPWp) and incubated at 37°C static for 5 h. Antimicrobial cocktail [Acriflavin-Cefsulodin-Vancomycin (ACV)] was added and incubated at 42°C static overnight (18–24 h). DNA supernatants recovered from boiled samples were analyzed by qPCR detecting stx1, stx2, and wzy.
Metagenomic data accession numbers. The metagenomic sequence data from this study are available in GenBank under BioProject number PRJNA751542.
Data availability statement
The datasets presented in this study can be found in online repositories. The names of the repository/repositories and accession number(s) can be found below: NCBI (accession: PRJNA751542).
Author contributions
EB, MA, SM, and NG-E designed the study. JK provided agricultural water samples and performed BAM analysis. All library preparation and sequencing were performed by NG-E. NG-E and MM analyzed the data and wrote the manuscript. All authors read and approved the final manuscript.
Funding
NG-E research was supported by funding from the MCMi Challenge Grants Program Proposal #2018-646 and the FDA Foods Program Intramural Funds. MM was supported by funding from the MCMi Challenge Grants Program. The study was supported by funding from the MCMi Challenge Grants Program Proposal #2018-646 and the FDA Foods Program Intramural Funds. Maguire acknowledges a Research Fellowship Program (ORISE) for the Center for Food Safety and Applied Nutrition administered by the Oak Ridge Associated Universities through a contract with the U.S. Food and Drug Administration.
Conflict of interest
The authors declare that the research was conducted in the absence of any commercial or financial relationships that could be construed as a potential conflict of interest.
Publisher’s note
All claims expressed in this article are solely those of the authors and do not necessarily represent those of their affiliated organizations, or those of the publisher, the editors and the reviewers. Any product that may be evaluated in this article, or claim that may be made by its manufacturer, is not guaranteed or endorsed by the publisher.
Supplementary material
The Supplementary Material for this article can be found online at: https://www.frontiersin.org/articles/10.3389/fenvs.2022.830300/full#supplementary-material
Abbreviations
eae, intimin gene; HUS, hemolytic uremic syndrome; LEE, locus of enterocyte effacement; MAG(s), metagenome assembled genome(s); STEC, Shiga toxin-producing Escherichia coli; stx, Shiga toxin gene; TTSS, type 3 secretion system; WGS, whole genome sequencing; WIMP, What’s In My Pot taxonomic classification program in Oxford Nanopore’s EPI2ME cloud service.
References
Allende, A., and Monaghan, J. (2015). Irrigation water quality for leafy crops: A perspective of risks and potential solutions. Int. J. Environ. Res. Public Health 12 (7), 7457–7477. doi:10.3390/ijerph120707457
Allos, B. M., Moore, M. R., Griffin, P. M., and Tauxe, R. V. (2004). Surveillance for sporadic foodborne disease in the 21st century: The FoodNet perspective. Clin. Infect. Dis. 38 Suppl 3, S115–S120. doi:10.1086/381577
Author Anonymous, (2018). Environmental assessment of factors potentially contributing to the contamination of romaine lettuce implicated in a multi-state outbreak of E. coli O157:H7. Available from: https://www.fda.gov/food/outbreaks-foodborne-illness/environmental-assessment-factors-potentially-contributing-contamination-romaine-lettuce-implicated.
Bertrand, D., Shaw, J., Kalathiyappan, M., Ng, A. H. Q., Kumar, M. S., Li, C., et al. (2019). Hybrid metagenomic assembly enables high-resolution analysis of resistance determinants and mobile elements in human microbiomes. Nat. Biotechnol. 37 (8), 937–944. doi:10.1038/s41587-019-0191-2
Beutin, L., and Martin, A. (2012). Outbreak of Shiga toxin-producing Escherichia coli (STEC) O104:H4 infection in Germany causes a paradigm shift with regard to human pathogenicity of STEC strains. J. Food Prot. 75 (2), 408–418. doi:10.4315/0362-028x.jfp-11-452
Boykin, L. M., Sseruwagi, P., Alicai, T., Ateka, E., Mohammed, I. U., Stanton, J. L., et al. (2019). Tree Lab: Portable genomics for early detection of plant viruses and pests in sub-saharan Africa. Genes. (Basel) 10 (9), 632. doi:10.3390/genes10090632
Brown, E., Dessai, U., McGarry, S., and Gerner-Smidt, P. (2019). Use of whole-genome sequencing for food safety and public Health in the United States. Foodborne Pathog. Dis. 16 (7), 441–450. doi:10.1089/fpd.2019.2662
Edwards, A., Soares, A., Rassner, S. M. E., Green, P., Félix, J., and Mitchell, A. C. (2017). Deep Sequencing: Intra-terrestrial metagenomics illustrates the potential of off-grid Nanopore DNA sequencing. bioRxiv, 133413.doi:10.1101/133413
Faria, N. R., Sabino, E. C., Nunes, M. R. T., Alcantara, L. C. J., Loman, N. J., and Pybus, O. G. (2016). Mobile real-time surveillance of Zika virus in Brazil. Genome Med. 8 (1), 97. doi:10.1186/s13073-016-0356-2
FDA, (2018). BAM 19c: Dead-end ultrafiltration for the detection of Cyclospora cayetanensis from agricultural water. Available from: https://www.fda.gov/media/140309/download.
FDA, (2020a). BAM chapter 4A: Diarrheagenic Escherichia coli. Available from: https://www.fda.gov/food/laboratory-methods-food/bam-chapter-4a-diarrheagenic-escherichia-coli.
FDA, (2020b). FDA announces new protocol for the development and registration of treatments for preharvest agricultural water. Available from: https://www.fda.gov/news-events/press-announcements/fda-announces-new-protocol-development-and-registration-treatments-preharvest-agricultural-water.
FDA, (2019). Investigation Summary: Factors potentially contributing to the contamination of romaine lettuce implicated in the fall 2018 multi-state outbreak of E. coli O157:H7. Available from: https://www.fda.gov/food/outbreaks-foodborne-illness/investigation-summary-factors-potentially-contributing-contamination-romaine-lettuce-implicated-fall.
FDA, (2020c). Leafy greens STEC action plan. Available from: https://www.fda.gov/food/foodborne-pathogens/leafy-greens-stec-action-plan.
Fischer, N., Bourne, A., and Plunkett, D. (2015). Outbreak alert! 2015: A review of foodborne illness in the US from 2004-2013. Washington, DC: Center for Science in the Public Interest.
Garmendia, J., Frankel, G., and Crepin, V. F. (2005). Enteropathogenic and enterohemorrhagic Escherichia coli infections: Translocation, translocation, translocation. Infect. Immun. 73 (5), 2573–2585. doi:10.1128/iai.73.5.2573-2585.2005
Gigliucci, F., von Meijenfeldt, F. A. B., Knijn, A., Michelacci, V., Scavia, G., Minelli, F., et al. (2018). Metagenomic characterization of the human intestinal microbiota in fecal samples from STEC-infected patients. Front. Cell. Infect. Microbiol. 8, 25. doi:10.3389/fcimb.2018.00025
Gonzalez-Escalona, N., Allard, M. A., Brown, E. W., Sharma, S., and Hoffmann, M. (2019). Nanopore sequencing for fast determination of plasmids, phages, virulence markers, and antimicrobial resistance genes in Shiga toxin-producing Escherichia coli. PLoS One 14 (7), e0220494. doi:10.1371/journal.pone.0220494
Gonzalez-Escalona, N., and Kase, J. A. (2019). Virulence gene profiles and phylogeny of Shiga toxin-positive Escherichia coli strains isolated from FDA regulated foods during 2010-2017. PLoS One 14 (4), e0214620. doi:10.1371/journal.pone.0214620
Gonzalez-Escalona, N., Meng, J., and Doyle, M. P. (2019). “Shiga toxin-producing Escherichia coli,” in Food microbiology: Fundamentals and Frontiers. 5th Edition (Washington, DC: American Society for Microbiology (ASM)).
Gonzalez-Escalona, N., Toro, M., Rump, L. V., Cao, G., Nagaraja, T. G., and Meng, J. (2016). Virulence gene profiles and clonal relationships of Escherichia coli O26:H11 isolates from feedlot cattle as determined by whole-genome sequencing. Appl. Environ. Microbiol. 82 (13), 3900–3912. doi:10.1128/aem.00498-16
Gowers, G. F., Vince, O., Charles, J. H., Klarenberg, I., Ellis, T., and Edwards, A. (2019). Entirely off-grid and solar-powered DNA sequencing of microbial communities during an ice cap traverse expedition. Genes. (Basel). 10 (11), 902. doi:10.3390/genes10110902
Hoffmann, M., Luo, Y., Monday, S. R., Gonzalez-Escalona, N., Ottesen, A. R., Muruvanda, T., et al. (2016). Tracing origins of the Salmonella Bareilly strain causing a food-borne outbreak in the United States. J. Infect. Dis. 213 (4), 502–508. doi:10.1093/infdis/jiv297
Huang, A. D., Luo, C., Pena-Gonzalez, A., Weigand, M. R., Tarr, C. L., and Konstantinidis, K. T. (2017). Metagenomics of two severe foodborne outbreaks provides diagnostic signatures and signs of coinfection not attainable by traditional methods. Appl. Environ. Microbiol. 83 (3), e02577-16. doi:10.1128/aem.02577-16
Johnson, S. S., Zaikova, E., Goerlitz, D. S., Bai, Y., and Tighe, S. W. (2017). Real-time DNA sequencing in the Antarctic dry valleys using the Oxford nanopore sequencer. J. Biomol. Tech. 28 (1), 2–7. doi:10.7171/jbt.17-2801-009
Kaper, J. B., Nataro, J. P., and Mobley, H. L. (2004). Pathogenic Escherichia coli. Nat. Rev. Microbiol. 2 (2), 123–140. doi:10.1038/nrmicro818
Kovac, J., Bakker, H. D., Carroll, L. M., and Wiedmann, M. (2017). Precision food safety: A systems approach to food safety facilitated by genomics tools. TrAC Trends Anal. Chem. 96, 52–61. doi:10.1016/j.trac.2017.06.001
Lakay, F. M., Botha, A., and Prior, B. A. (2007). Comparative analysis of environmental DNA extraction and purification methods from different humic acid-rich soils. J. Appl. Microbiol. 102 (1), 265–273. doi:10.1111/j.1365-2672.2006.03052.x
Leonard, S. R., Mammel, M. K., Lacher, D. W., and Elkins, C. A. (2015). Application of metagenomic sequencing to food safety: Detection of Shiga toxin-producing Escherichia coli on fresh bagged spinach. Appl. Environ. Microbiol. 81 (23), 8183–8191. doi:10.1128/aem.02601-15
Leonard, S. R., Mammel, M. K., Lacher, D. W., and Elkins, C. A. (2016). Strain-level discrimination of Shiga toxin-producing Escherichia coli in spinach using metagenomic sequencing. PLoS One 11 (12), e0167870. doi:10.1371/journal.pone.0167870
Loman, N. J., Constantinidou, C., Christner, M., Rohde, H., Chan, J. Z., Quick, J., et al. (2013). A culture-independent sequence-based metagenomics approach to the investigation of an outbreak of Shiga-toxigenic Escherichia coli O104:H4. JAMA 309 (14), 1502–1510. doi:10.1001/jama.2013.3231
Lusk Pfefer, T., Ramachandran, P., Reed, E., Kase, J. A., and Ottesen, A. (2018). Metagenomic description of preenrichment and postenrichment of recalled Chapati Atta flour using a shotgun sequencing approach. Genome Announc. 6 (21), e00305-18. doi:10.1128/genomea.00305-18
Maguire, M., Kase, J. A., Roberson, D., Muruvanda, T., Brown, E. W., Allard, M., et al. (2021). Precision long-read metagenomics sequencing for food safety by detection and assembly of Shiga toxin-producing Escherichia coli in irrigation water. PLoS One 16 (1), e0245172. doi:10.1371/journal.pone.0245172
McIntyre, A. B. R., Ounit, R., Afshinnekoo, E., Prill, R. J., Hénaff, E., Alexander, N., et al. (2017). Comprehensive benchmarking and ensemble approaches for metagenomic classifiers. Genome Biol. 18 (1), 182. doi:10.1186/s13059-017-1299-7
Mead, P. S., Slutsker, L., Dietz, V., McCaig, L. F., Bresee, J. S., Shapiro, C., et al. (1999). Food-related illness and death in the United States. Emerg. Infect. Dis. 5 (5), 607–625. doi:10.3201/eid0505.990502
Mellmann, A., Bielaszewska, M., Köck, R., Friedrich, A. W., Fruth, A., Middendorf, B., et al. (2008). Analysis of collection of hemolytic uremic syndrome–associated enterohemorrhagic Escherichia coli. Emerg. Infect. Dis. 14 (8), 1287–1290. doi:10.3201/eid1408.071082
Monaghan, J. M., and Hutchison, M. L. (2012). Distribution and decline of human pathogenic bacteria in soil after application in irrigation water and the potential for soil-splash-mediated dispersal onto fresh produce. J. Appl. Microbiol. 112 (5), 1007–1019. doi:10.1111/j.1365-2672.2012.05269.x
Moss, E. L., Maghini, D. G., and Bhatt, A. S. (2020). Complete, closed bacterial genomes from microbiomes using nanopore sequencing. Nat. Biotechnol. 38, 701–707. doi:10.1038/s41587-020-0422-6
National Advisory Committee on Microbiological Criteria for Foods (2019). Response to questions posed by the Food and Drug Administration regarding virulence factors and attributes that define foodborne Shiga toxin-producing Escherichia coli (STEC) as severe human Pathogens. J. Food Prot. 82 (5), 724–767. doi:10.4315/0362-028x.jfp-18-479
Nicholls, S. M., Quick, J. C., Tang, S., and Loman, N. J. (2019). Ultra-deep, long-read nanopore sequencing of mock microbial community standards. Gigascience 8 (5), giz043. doi:10.1093/gigascience/giz043
Olaimat, A. N., and Holley, R. A. (2012). Factors influencing the microbial safety of fresh produce: A review. Food Microbiol. 32 (1), 1–19. doi:10.1016/j.fm.2012.04.016
Oliveira, M., Vinas, I., Usall, J., Anguera, M., and Abadias, M. (2012). Presence and survival of Escherichia coli O157:H7 on lettuce leaves and in soil treated with contaminated compost and irrigation water. Int. J. Food Microbiol. 156 (2), 133–140. doi:10.1016/j.ijfoodmicro.2012.03.014
Ottesen, A., Ramachandran, P., Chen, Y., Brown, E., Reed, E., and Strain, E. (2020). Quasimetagenomic source tracking of Listeria monocytogenes from naturally contaminated ice cream. BMC Infect. Dis. 20 (1), 83. doi:10.1186/s12879-019-4747-z
Quick, J., Loman, N. J., Duraffour, S., Simpson, J. T., Severi, E., Cowley, L., et al. (2016). Real-time, portable genome sequencing for Ebola surveillance. Nature 530 (7589), 228–232. doi:10.1038/nature16996
Scallan, E., Hoekstra, R. M., Angulo, F. J., Tauxe, R. V., Widdowson, M. A., Roy, S. L., et al. (2011). Foodborne illness acquired in the United States--major pathogens. Emerg. Infect. Dis. 17 (1), 7–15. doi:10.3201/eid1701.p11101
Steele, M., and Odumeru, J. (2004). Irrigation water as source of foodborne pathogens on fruit and vegetables. J. Food Prot. 67 (12), 2839–2849. doi:10.4315/0362-028x-67.12.2839
Tack, D. M., Ray, L., Griffin, P. M., Cieslak, P. R., Dunn, J., Rissman, T., et al. (2020). Preliminary incidence and trends of infections with pathogens transmitted commonly through food — foodborne Diseases Active Surveillance Network, 10 U.S. Sites, 2016–2019. MMWR Morb. Mortal. Wkly. Rep. 69, 509–514. doi:10.15585/mmwr.mm6917a1
Tarr, P. I., Gordon, C. A., and Chandler, W. L. (2005). Shiga-toxin-producing Escherichia coli and haemolytic uraemic syndrome. Lancet 365 (9464), 1073–1086. doi:10.1016/s0140-6736(05)71144-2
Uyttendaele, M., Jaykus, L-A., Amoah, P., Chiodini, A., Cunliffe, D., Jacxsens, L., et al. (2015). Microbial hazards in irrigation water: Standards, norms, and testing to manage use of water in fresh produce primary production. Compr. Rev. Food Sci. Food Saf. 14 (4), 336–356. doi:10.1111/1541-4337.12133
Keywords: foodborne pathogens, nanopore sequencing, agricultural water, metagenomics, shiga toxin-producing Escherichia coli, STEC
Citation: Maguire M, Kase JA, Brown EW, Allard MW, Musser SM and González-Escalona N (2022) Metagenomic survey of agricultural water using long read sequencing: Considerations for a successful analysis. Front. Environ. Sci. 10:830300. doi: 10.3389/fenvs.2022.830300
Received: 07 December 2021; Accepted: 20 July 2022;
Published: 10 August 2022.
Edited by:
Maria Ines Zanoli Sato, Companhia Ambiental do Estado de São Paulo (CETESB), BrazilReviewed by:
Séamus Fanning, University College Dublin, IrelandMilena Dropa, Faculty of Public Health, University of São Paulo, Brazil
Copyright © 2022 Maguire, Kase, Brown, Allard, Musser and González-Escalona. This is an open-access article distributed under the terms of the Creative Commons Attribution License (CC BY). The use, distribution or reproduction in other forums is permitted, provided the original author(s) and the copyright owner(s) are credited and that the original publication in this journal is cited, in accordance with accepted academic practice. No use, distribution or reproduction is permitted which does not comply with these terms.
*Correspondence: Narjol González-Escalona, bmFyam9sLmdvbnphbGV6LWVzY2Fsb25hQGZkYS5oaHMuZ292