- 1Post-graduate Program at Federal University of Goiás, Goiânia, Brazil
- 2Brazilian Agricultural Research Corporation (Embrapa Rice and Beans), Santo Antônio de Goiás, GO, Brazil
- 3USDA-ARS-PWA-CPCRC, Pendleton, OR, United States
- 4Brazilian Agricultural Research Corporation (Embrapa Mid-North), Teresina, PI, Brazil
Land degradation and reduction in productivity have resulted in losses of soil organic carbon (SOC) in agricultural areas in Brazil. Our objectives were to 1) evaluate the predictive performance of CQESTR model for a tropical savannah; and 2) examine the effect of integrated management systems, including Integrated Crop-Livestock System (ICLS) scenarios on SOC stocks. Two long-term paddocks, under similar edaphic and climate conditions were used in this study. In Paddock 4 (P4) the rotation was corn (Zea mays L.) and 3.5/4.5 years pasture (Urochloa ruziziensis), while rotations in Paddock 5 (P5) included 2.5 years of soybean (Glycine max L.), dryland rice (Oryza sativa L.), and corn followed by 2.5/3.5 years pasture (U. brizantha). Measured and CQESTR simulated values were significantly (0.0001) correlated (r = 0.94) with a mean square deviation (MSD) of 7.55, indicating that the model captured spatial-temporal dynamics of SOC. Predicted SOC increased by 18.0 and 12.04 Mg ha−1 at the rate of 0.90 and 0.60 Mg ha−1 year−1 under current ICLS management for P4 and P5, respectively, by 2039. ICLS increased soil C sequestration compared to simple grain cropping systems under both NT and CT due to high biomass input into the production system.
Introduction
Land-use changes or agricultural management practices can change soil organic carbon (SOC) stocks. Furthermore, the soil is an important carbon (C) pool and can be a source or sink of atmospheric CO2 depending on the agriculture management adopted (Carvalho et al., 2010). In Brazil, unsustainable soil management has resulted in land degradation, loss of productive capacity of agricultural areas, loss of SOC, resulting in CO2 emissions. It is estimated that 60–70% of Brazilian pastures show signs of degradation (Soares et al., 2020; Lapig, 2021), and up to 80% of the degraded pastures are in Central Brazil (Balbino et al., 2012). In general, pastures are considered degraded when they support very low stocking rates (<0.5 AU), show low plant cover, are invaded by non-palatable plant species, and are often densely populated with termite mounds (Boddey et al., 2004). Cardoso et al. (2016) report that the area of pasture required to produce 1 kg of a carcass (dead weight of animal) on a degraded pasture is approximately 320 m2 but this falls to 71 m2 on managed pastures, like mixed grass/legume.
Integrated Crop-Livestock Systems (ICLS) have emerged as an economically feasible management to restore degraded pastures (Cortner et al., 2019; Reis et al., 2019). Reis et al. (2019) report that the payback period of ICLS is shorter than continuous livestock or continuous cropping, and Cortner et al. (2019) showed that ranchers perceived ICLS as a necessity to maintain their livelihood amidst declining profits. In Brazil, integrated systems or mixed farming systems have received considerable attention from farmers and policy makers. They were part of the Low Carbon Emission Agriculture Plan (ABC Plan, known in Brazil as “Plano ABC”, Brasil, 2012), which is the contribution of the agriculture sector to the National Climate Change Program, also launched as part of Brazil’s Nationally Appropriate Mitigation Actions (NAMAs) in 2009, at the 15th UNFCCC-Conference of the Parties (COP 15). The ABC Plan consisted of six selected agricultural practices, one of which was integrated systems, supported by specific credit lines at low-interest rates for the period 2010–2020 (Mozzer, 2011; Brasil, 2012). Despite difficulties in measuring the effectiveness of positive incentive measures in agriculture such as the ABC Plan (Carauta et al., 2021), following the Paris Agreement during the 21st UNFCCC-Conference of the Parties, Brazil has presented a new edition of the ABC Plan for 2020–2030 (Plano ABC+, Brasil, 2021a), part of which is also included in the country’s Nationally Determined Contributions (NDC, Brasil, 2020; Brasil, 2021b). The new edition of the ABC Plan aims at the additional adoption of 10 million hectares under ICLS and other integrated systems by farmers.
Well-designed ICLS both spatially and temporally allow better regulation of biogeochemical cycles and showing synergies between crop and livestock systems in system-wide evaluations of production and environmental quality (Franzluebbers et al., 2014; Lemaire et al., 2014). Long-term ICLS enables constant and efficient nutrient cycling because the animal, pasture, and crop residues release nutrients at different rates (Assmann et al., 2017). When grasses are intercropped, especially in the form of consortium (e.g., palisade grass planted during the same cycle as a grain crop), there is a higher biomass input and consequently higher nutrient recycling (i.e., N, P, K, and Mg) in the production system (Pariz et al., 2017). Measured nitrous oxide emissions are reduced in both ICLS (Sato et al., 2017) and ICLF (integrated crop-livestock-forestry), the most complex version of integrated systems with tree components (Franzluebbers et al., 2016; Carvalho et al., 2017). Integrated crop-livestock systems are also shown to improve nutrient cycling by re-coupling nitrogen (N) and C cycles (Ryschawy et al., 2017). Sant-Anna et al. (2017) investigated changes in SOC of pastures, crop production systems, and ICLS in the Brazilian Cerrado and found the highest SOC stocks under ICLS; however, not all ICLS increased SOC even under zero tillage. Oliveira et al. (2018) reported that soil N deficiency negatively affected SOC accumulation in ICLF and concluded that N management is a key to increasing SOC accumulation in integrated production systems. Damian et al. (2021) evaluated SOC changes in a poorly managed pasture with more intensively fertilized and diversified pasture systems (FP) and ICLS. They concluded that fertilization every year (FP) and the implementation of a cropping phase alternating with pasture (ICLS) resulted in the highest SOC stocks. When the pasture is recovered by inserting a crop (usually a grain crop), the residual fertilizer after cropping enhances pasture productivity. In this case, the cost of fertilizer application is covered by the income received for the crop (Kluthcouski and Yokoyama, 2003; Macedo, 2009). Meanwhile, perennial grasses such as brachiaria grass (Urochloa spp.) with large above-ground and root biomass contribute to SOC and increase in grain yields more than in a production system specialized for crop production only, particularly in years with poor rainfall distribution (Salton et al., 2014; Bieluczyk et al., 2020). Moraes Sá et al. (2014) reported a close correlation between SOC stocks and grain yield of soybean and corn from a long-term experiment in an Oxisol in southern Brazil under a subtropical humid climate. The added plant residues in ICLS differ in quantity and quality, being more recalcitrant compared with continuous pasture. Therefore, ICLS have been suggested as promising agricultural management contributing to the decarbonization of Brazilian agriculture (Tadini et al., 2021).
Assessing SOC dynamics in agroecosystems is challenging (Tornquist et al., 2009) mainly because of the complex interactions among components such as soil, vegetation, grazing animals, and humans (Godde et al., 2020). These changes often occur gradually and are difficult to detect in the short-term against the larger background (McGill et al., 1986; Ghani et al., 1996; Bolinder et al., 1999). Since the ICLS consists of a mixture of different grain crops often in rotation with several forage species (e.g., grass or legume), with varying grazing intensity and livestock densities, it can be difficult to determine whether the agricultural system is a C source or sink. Detecting small management-induced changes in SOC during short periods of time is difficult because of large differences in spatial and temporal SOC stocks (Kravchenko and Robertson, 2011). Long-term experiments, with historical datasets, have been used to assess impacts of past agricultural management practices on SOC dynamics. Process-based C models are potential research tools to predict the SOC changes; however, they need validation for the edaphoclimatic conditions of each region or country. Additionally, process-based C models are useful tools to analyze soil management options and to compare impacts of different management scenarios on SOC stocks. Thus, these models can be used to supplement field experiments to study SOC dynamics and to estimate the distribution of C in soil (Al-Adamat et al., 2007; Gollany et al., 2012). Furthermore, C models are useful in predicting the effects of potential management changes on the soil C stocks (Gollany et al., 2021). This makes it possible to test different scenarios and seek strategies to mitigate the negative impacts of such changes.
Carbon models have been used extensively for ecosystems and soil types under temperate conditions, while their evaluation under tropical conditions is less common (Kamoni et al., 2007; Tornquist et al., 2009; Damian et al., 2021). In these regions soil organic matter cycling is very different from that observed in temperate regions, because of the predominance of highly weathered acidic soils with low cation exchange capacity, high temperatures and high annual precipitation, where processes, including SOC decomposition rate, can be tenfold faster (Moreira and Siqueira, 2006). Given the importance of Brazil for global agriculture, it is necessary to validate C models and use them to investigate management options to increase SOC stocks in complex and diversified agriculture systems like integrated crop-livestock production.
Because of its relative simplicity, the readily available model inputs, and the possibility to compute SOC in a soil up to 5 layers, the CQESTR model was selected to study how different agricultural management practices affect soil C dynamics under tropical climate over time in two agroecosystems under ICLS. The CQESTR model was used previously to predict soil C dynamics in two tillage systems under tropical soils (Ultisol and Oxisol) in southeastern and northeastern Brazil (Leite et al., 2009); however, never used to predict SOC stocks under ICLS in Brazilian Cerrado. Due to the paucity of measured long-term SOC stocks data for the intensified and diversified pastures in Brazil, we hypothesize that process-based models can be an efficient and cost-effective tool to predict soil C stocks under several management practices and to project SOC stocks change for several ICLS scenarios. Therefore, the objectives of this study were to: 1) validate the CQESTR model for a tropical savanna (Cerrado) and predict the effect of several agricultural management systems and practices, including ICLS and no-tillage (NT); and 2) simulate the effect of conventional tillage and NT production scenarios on SOC dynamics in diversified ICLS.
Materials and Methods
Study Site
The study was conducted at the “Capivara Research Farm” of the National Rice and Bean Research Center of the Brazilian Agricultural Research Corporation (Embrapa Rice and Beans), located in the Cerrado biome, in Santo Antônio de Goiás (16°28″ S, 49°17″ W; elevation 803 m. a.s.l), Goiás State, Brazil. The native vegetation is a semideciduous forest (cerradão) composed of about 30 tree species at a density of 2,800 individual plants ha−1, of which seven (Hirtella glandulosa Spreng; Hirtella gracilipes (Hook.f.) Prance; Protium heptaphyllum (Aubl.) March.; Tapirira guianensis Aubl.; Emmotum nitens (Benth.) Miers.; Copaifera langsdorfi Desf; Pterodon emarginatus Vog.) are the most common (Silveira, 2010). The soil is a Typic Acrustox (clay, kaolinitic, thermic Typic Acrustox) or a Rhodic Ferralsol (WRB/FAO) or (Latossolo Vermelho Acriférrico Típico (Brazilian Soil Classification System)) with an average content of 524 g kg−1 clay and 349 g kg−1 sand. According to Köppen’s classification, the climate is tropical megathermic savanna (Aw), where two well-defined seasons occur, a dry season from May to September and a rainy season, extending from October to April (IBGE, 2002). The average annual rainfall over the last 35 years (1983–2020) was 1,479 mm (Agritempo, 2014) of which about 90% was concentrated between October and April. The mean annual air temperature is 23.7°C.
Crop-Livestock Management
Two areas under ICLS and a nearby area under native vegetation (reference site) were studied. The areas under ICLS are part of a Technological and Research Reference Unit (TRRU) which is part of the ILPF Network Association (Rede ILPF, 2021) that aims to accelerate the widespread adoption of crop-livestock-forestry integration technologies by rural producers as part of an effort aimed at the sustainable intensification of Brazilian agriculture. This TRRU is composed of six areas with sizes ranging from 5.1 to 9.2 ha.
The study area was covered by natural vegetation until 1933 when selective logging began and lasted until 1950 (Figure 1). Deforestation was completed in the 1970s when agriculture started. Common bean, dryland rice and corn were planted as main crops, including the areas currently under ICLS. In 1983 rice cultivation stopped and crop rotations changed to common bean and corn. In 1994, soybean was cultivated for the first time in the area, and 5 years later, soybean entered the rotation. Finally, in 1995, the area was divided into 6 paddocks as ICLS was gradually implemented between 2000–2005. Few variations in the rotation have occurred since 2000. The introduction of the pasture to the system occurred by planting corn in consortium with brachiaria grass. Two of the six long-term paddocks (Figure 2A) were selected for this simulation study, P4 (7.5 ha) and P5 (8.1 ha), because these two paddocks are under a long-term observation study including greenhouse gas (CO2, water vapor and CH4), soil, weather, and radiation sensors; these paddocks also have long-term soil data, including SOC. Initially, corn was the common crop used in the ICLS rotation, alternated with several years of pasture. The crop phase of the rotation became more diversified by introducing soybean, aerobic rice and common bean to the crop phase starting in 2007/2008, first in P5, then in P4 during 2013/2014. From 2014, there is a more pronounced presence of pasture in P5 compared to P4. The current rotation is based on the alternation of crops with a pasture phase (Table 1). The rotation in P4 includes 3 years of summer crops (rainy season) with pasture during the dry season and 2.5 years of pure pasture. In P5 the rotation consists of 3 years of summer crops with pasture in the dry season, and 3.5 years of continuous pasture phase. The whole system is conducted under no-tillage and pasture is always reintroduced by sowing corn in consortium with brachiaria grass. From 2020 on, another option for reintroducing pasture after the crop phase is a dryland rice-brachiaria grass consortium. The grass remaining after corn or rice harvest initiates the pasture phase. In 2020/2021, the summer crop was substituted by pasture in P4 because of the COVID-19 pandemic. Soil preparation until 1995 included tillage with a conventional disc plow (∼10-cm depth) and leveling harrow (first operation at ∼7-cm and a second at ∼3-cm depth). Since then, exclusively direct seeding (no-tillage, NT) was used.
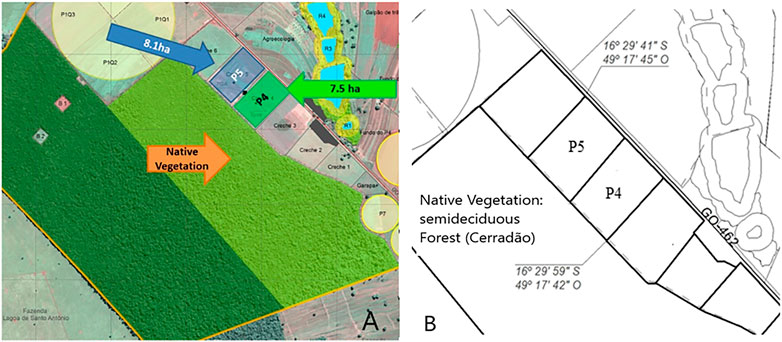
FIGURE 2. Paddock 4 (P4) and Paddock 5 (P5) and native vegetation at Capivara Experimental Farm, Embrapa Rice and Bean in Santo Antônio de Goiás, GO, Brazil.
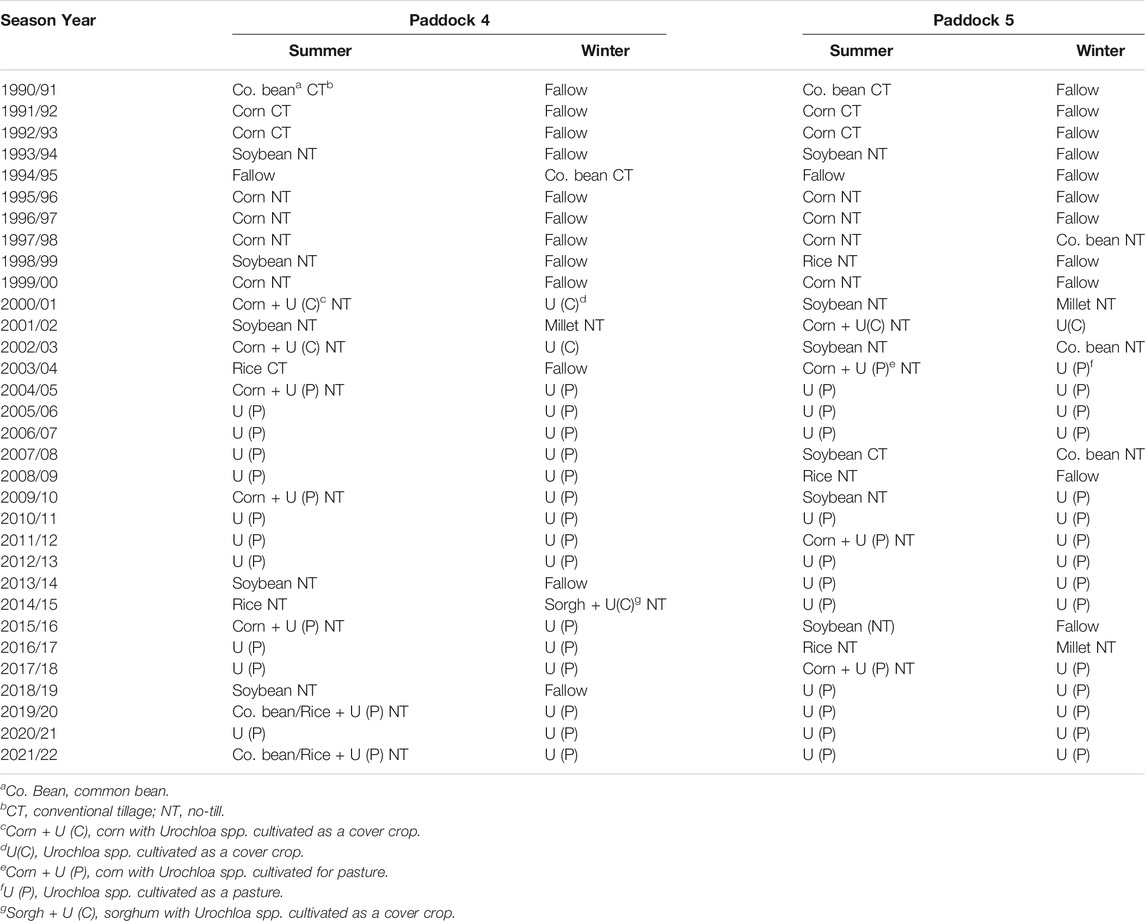
TABLE 1. Cropping sequences and tillage history of the two paddocks (4 and 5) under integrated crop-livestock systems at Santo Antônio de Goiás, Goiás State, Brazil (1990–2022).
Fertilizers were applied according to soil fertility analysis and crop need at seeding or as top-dressing. Nitrogen fertilizer application in P4 varied from 0 to 288 kg ha−1 (averaged 49.6 kg N ha−1): phosphorus 38–116 kg ha−1 (average 73.7 kg P2O5 ha−1); and potassium ranged from 38 to 159 kg ha−1 (average 60.4 kg K2O ha−1); while application rates in P5 varied from 0 to 120 kg ha−1 (average 28.6 kg N ha−1); phosphorous 26–183 kg ha−1 (average 86.2 kg P2O5 ha−1); and potassium 31–129 kg ha−1 (average 65.2 kg K2O ha−1). Fungicides and insecticides were used when needed according to manufacturer’s recommendation and control level. Glyphosate was used to control weeds and brachiaria grass growing to allow seeding in NT.
Soil Sampling and Analysis
In 2010, 2015 and 2020 samples were collected from the paddocks (P4 and P5) to 100 cm depth at 10-cm increments. Four main soil profiles were used in 4 quadrants of each studied site, and the samples were taken to 30-cm depth from the soil profile, and from 4 satellite points at a 5-m distance from each profile. A composite sample for each profile and depth was prepared from these sub-samples. In the other years, 30 individual samples were collected at 10 or 30 cm (0–10 cm in 1999 and 2013; 0–10, 10–30 cm in 2007) in a zigzag pattern. In this study, SOC stocks at 0–10 and 10–30 cm depths were used for each sampling year. In the case of quadrant sampling, each composite sample of 5 sub-samples represented 1.87 ha in P4 and 2.02 ha in P5; however, if considering sub-samples as individuals, the sampling density was 2.66 and 2.47 samples per ha for P4 and P5, respectively. In the case of zigzag sampling, each individual sample represented 0.25 and 0.27 ha, at the sampling density of 4.00 and 3.70 samples per ha, respectively. Machado et al. (2009) studied the spatial variability of SOC for 0–5, 5–10, and 10–20 cm in a 12.5 ha soybean field under NT in a clay (555 g kg−1) Rhodic Ferralsol (Latosssolo Vermelho distroférrico, in the Brazilian Soil Classification System), comparable to our study area. They found that the recommended sampling density was a minimum of 0.64 samples per ha considering sampling depth between 0 and 20-cm depth. Before soil chemical analysis, plant tissues and other non-soil material was removed by hand and sieving. Air-dried soil samples were sieved in a 2 mm sieve. Soil samples were collected for SOC analysis using hand auger. The SOC was determined by the Walkley-Black method (Nelson and Sommers, 1996) without external heating, using sulfuric acid to generate internal heat for the reaction, and a correction factor of 1.3 to calculate SOC. The Walkley-Black method was used because the Dumas method (elemental analysis) was not available before 2010. We opted to use the same method for the sake of comparability of the SOC measurements.
The hydrometer method was used to determine clay, sand, and silt contents, with a standard hydrometer with Bouyoucos scale (Gee et al., 2002). Soil bulk density was determined with the soil core method using Kopecky rings (Grossman et al., 2002). Soil pH was determined in water (Thomas, 1996). Exchangeable calcium, magnesium and extractable potassium were extracted as described by Kuo (1996), then Ca and Mg were determined by atomic absorption spectroscopy and K by flame emission spectrometry (Wright and Stuczynski, 1996). Potential acidity was determined according to Silva (2009). Aluminum was extracted according to Bertsch and Bloom (1996) as modified by Silva (2009). Selected soil properties of the soil surface (0–10 cm depth) are shown in Table 2.

TABLE 2. Mean selected soil chemical properties and soil particle size distributions for the surface 10 cm for two paddocks under integrated crop-livestock systems and an adjacent forest at Santo Antônio de Goiás, Goiás State, Brazil.
The CQESTR Model Data Inputs
Most data inputs required by CQESTR were provided by Embrapa and other required information was obtained from literature. Weather information, such as average daily air temperature and monthly rainfall, was provided by the weather station at Embrapa. Paddock 4 received 9.5 Animal Unit (AU) ha−1 and P5 received 8.8 AU ha−1. The grazing rotations were 10 grazing days and 56 rest days per cycle. The amount of manure each paddock received was estimated at ∼13 and ∼12 Mg ha−1 year−1 for P4 and P5, respectively. Embrapa provided information on above-ground biomass for Urochloa grass production, while below-ground biomass was estimated based on similar grass species root:shoot ratios (Bolinder et al., 2002). These grass species root:shoot estimates were successfully used (r = 0.987) in previous CQESTR simulations of reed canary grass and switchgrass pastures (Dell et al., 2018). For crop yield, we used an extensive dataset from the National Food Supply Company [“Companhia Nacional de Abastecimento”] (Conab, 2021). This information and harvest index were used to calculate the amount of above-ground crop biomass after harvest which was used to develop crop-specific yield files for each vegetation type. Average yields were calculated for different time spans where factors, such as weather and variety improvements, created obvious delineations in the yields for each crop individually. Corn yields of 2,225; 4,481; 4,939 and 7,620 kg ha−1, dryland rice yields of 965 and 2,069 kg ha−1, soybean yields of 1,845 and 2,801 kg ha−1, and common bean yields of 522; 1,718 and 2,738 kg ha−1 were used in these simulations (Conab, 2021). Weeds and volunteer crop growth during fallow periods was estimated at 1,800 kg ha−1 (Pacheco et al., 2011). A 4,266 kg ha−1 residue yield was used for any millet crops; and yields for Urochloa brizantha and U. ruziziensis ranging from 4,850–12,736 and 3,100–15,095 kg ha−1, respectively, were used for the simulation by duration of plant growth ranging from 0.5 to 4 years, based on data provided by Embrapa. A single pass no-till disc planter was used for seeding at 5-cm depth, in all years except summers of 2003 and 2007 for P4 and P5, respectively (Table 1). Prior to seeding of soybean in those years, two passes were made with harrows at 3- and 9-cm depth.
Other information required by CQESTR: below ground biomass, nitrogen content of residue at decomposition initiation, fraction of pre-tillage residue weight remaining on the soil surface after each tillage, were based on literature (Santos et al., 2007; Leite et al., 2009; Pacheco et al., 2011; Mauad et al., 2012). Number and thickness of soil depths, SOC content and soil bulk density of each soil depth were provided by Embrapa (Table 3). The CQESTR input values used for the initial SOC in the 0–10 and 10–30 cm depths were 15 and 25 g kg−1, and corresponding soil bulk densities were 1.34 and 1.35 g cm−3, respectively, which were used for the model spin-up period dating back to native vegetation conversion to agricultural management in the 1970s as a starting point. Concentration of SOC (g C kg−1) was converted to Mg C ha−1 using bulk density measured for each depth (Table 3) to determine soil mass per depth by area.
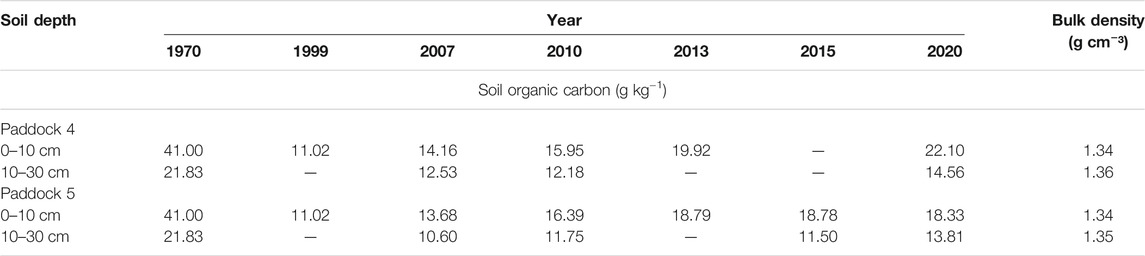
TABLE 3. Soil bulk density and soil organic carbon content of each soil depth at Santo Antônio de Goiás, Goiás State, Brazil.
CQESTR Validation
The exact rotation (as shown in Table 1) was used in the simulations for each of the paddocks. Corn, soybean, and dryland rice were grown in annual rotations from 1990 until 2003 except for an entire fallow crop year in 1994 for P4 and during 1994/1995 for P5. From 2003 to 2039, a 5-years ICLS cycle was used starting with a crop in 2004/2005 for P4 and a 6-years ICLS cycle was used starting with a crop in 2003/2004 for P5, respectively, typified by a first year of a corn intercropped with pasture followed by 3- or 4-years of pasture only. For 3 years following pasture, soybean, dryland rice and common bean were grown in different phases before restarting the cycle. The yield file used for each year during the simulation was crop-specific, as described above, and a soil operation file, created specifically for each management operation, as well as estimated animal manure, were used in the simulations. The SOC dynamics in the 0–10 and 10–30 cm depths were simulated for 50 years (1990–2039), and the simulation results were compared to observed SOC stocks in 1999, 2007, 2010, 2013, 2015 (P5 only) and 2020 for the 0–10 cm depth, and in 2007, 2010, 2015 (P5 only), and 2020 for the 10–30 cm depth.
CQESTR Simulation of Crop Rotation Change Scenarios
At the end of the validation period (1990–2020), SOC was simulated for additional management scenarios to represent the adoption of cropping systems and the transition to ICLS with different crop rotations for nearly two additional decades (2021–2039). Two crop-livestock rotation simulation scenarios were prepared for the two paddocks. Paddock 4 had a 5-years rotation, which included corn as a summer crop followed by 4.5 years pasture. The rotation in P5 was 6 years and included soybean followed by fallow in the first year, dryland rice and fallow in the second year and corn followed by 3.5 years pasture. Actual crop biomass yields estimated pasture residue inputs and animal manure were used in the simulations.
Additionally, four scenarios were prepared including soybean and corn in rotation. In the first scenario, the same crop sequence as P4/P5 was used, but tillage was changed from NT to conventional tillage (CT). The second scenario included soybean as a summer crop followed by corn in a second harvest in the same crop year under no-till (Soy-CS(NT)); in the third scenario, soybean and corn alternated each year as summer crops under no-till (Soy/Corn(NT)) followed by a fallow period; and finally in the fourth scenario, soybean and corn alternated each year as summer crops under conventional tillage (Soy/Corn(CT)). Conventional tillage operations in the simulation consisted of tilling with a conventional disc plow (10-cm depth) and leveling harrow (first operation at 7-cm and a second at 3-cm depth).
CQESTR Model Evaluation
Model performance was evaluated as described by Liang et al. (2009) using regression analysis and mean square deviation (MSD) statistics. The three components of MSD are squared bias (SB), nonunity slope (NU) and lack of correlation (LC), which are entirely independent and related to terms of the linear regression equation (Y = a + bX) and the regression coefficient (r2) (Gauch et al., 2003).
Results
Evaluation of CQESTR Model Performance
The linear fit of simulated vs. observed SOC explained 94% of the variation (Figure 3). Regression analysis of 18 pairs of observed and simulated SOC values for Paddock 4 (P4) and Paddock 5 (P5) was significantly (p < 0.0001) correlated (r = 0.94), with a slope of 0.84 not significantly different from 1.0 for soil sampling depths of 0–10 and 10–30 cm (Figure 3). The total mean square deviation (MSD) was 7.55. It indicates that CQESTR can accurately predict measured SOC stocks at the two soil sampling depths at both ICLS sites without calibration. The MSD was partitioned into its components: lack of correlation (LC), square bias (SB), and non-unity slope (NU), and were 7.55, 0.15, and 0.08 Mg SOC ha−1, respectively. The lack of correlation (LC), the highest contributing component of MSD accounted for 97% of the total MSD, which indicated that prediction errors were associated with data scattering and high standard deviation of observed SOC data. The square bias (SB) accounted for 2%, and non-unity slope accounted for 1% of the total MSD.
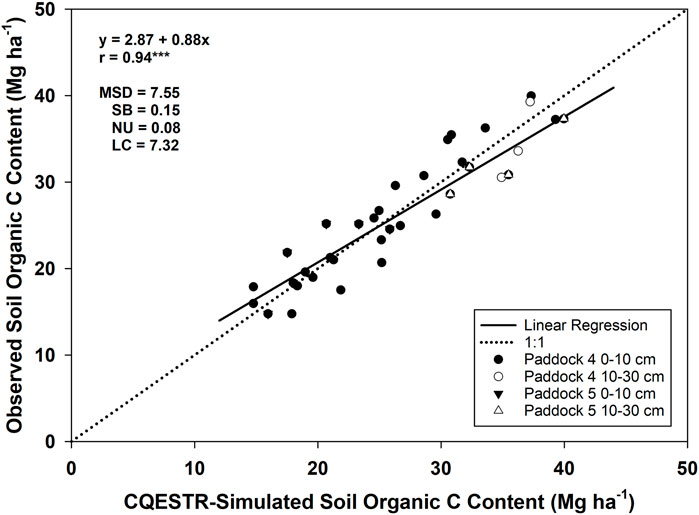
FIGURE 3. Observed and simulated soil organic carbon (SOC) stocks at 0–10 and 10–30 cm depths for Paddock 4 and Paddock 5 in a Typic Acrustox soil under integrated crop-livestock system at Santo Antônio de Goiás, GO, Brazil. Dotted line refers to 1:1 correspondence of measured and simulated values. Observed and simulated data were significantly correlated (p < 0.0001) with a Pearson correlation (r) of 0.94. MSD, mean squared deviation; SB, squared bias; NU, nonunity slope; LC, lack of correlation.
CQESTR Simulated Rotation Scenarios
CQESTR predicted the measured SOC values for P4 at both depths very well (r = 0.94), except underestimating the SOC values by 3.30 Mg SOC ha−1 in the top 10-cm depth in 2020 (Figures 3, 4). CQESTR underestimated the measured SOC values for P5 by 4.14 and 4.33 Mg SOC ha−1 in the top 10-cm depth during 2010 and 2013 sampling periods and overestimated measured SOC values by 4.94 Mg SOC ha−1 in 10–30 cm during the 2015 sampling period. Measured and simulated SOC stocks increased in the topsoil for P4 more with time than for P5 (Figure 3). The measured SOC values for P4 (Figure 4) had a similar pattern to that in P5 (Figure 5), although the measured and simulated SOC stocks increased faster in P4 than in P5.
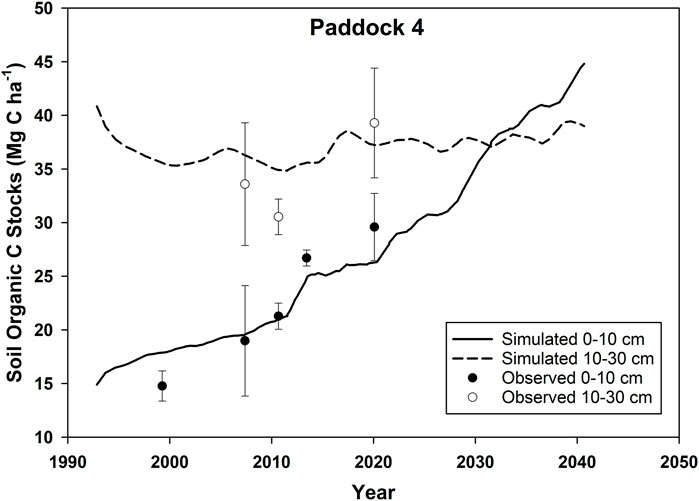
FIGURE 4. Simulated and observed soil organic carbon (SOC) stocks at 0–10 and 10–30 cm depths for Paddock 4 area, in a Typic Acrustox soil under integrated crop-livestock system at Santo Antônio de Goiás, GO, Brazil.
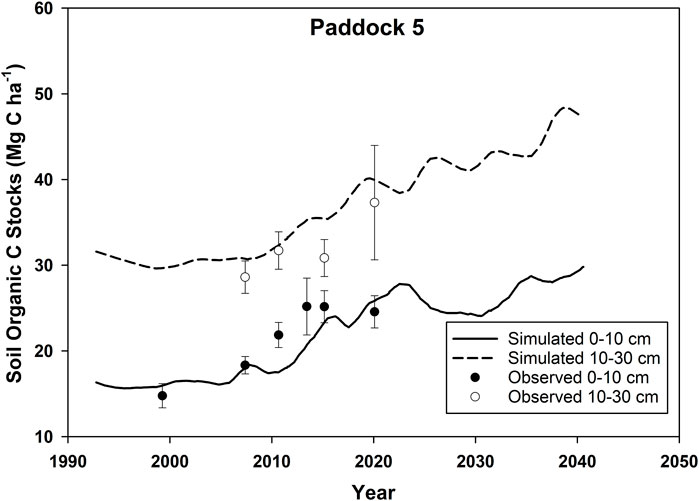
FIGURE 5. Simulated and observed soil organic carbon (SOC) stocks at 0–10 and 10–30 cm depths for Paddock 5 area, in a Typic Acrustox soil under integrated crop-livestock system at Santo Antônio de Goiás, GO, Brazil.
CQESTR Projected Soil Organic Carbon Stock and Rotation Scenarios
A comparison of simulated SOC stocks changes over 40 years for Paddock 4 (P4) and Paddock 5 (P5) indicates more SOC increase in P4 than in P5 (Table 4). CQESTR predicted SOC increase of 18.00 Mg ha−1 at a rate of 0.90 Mg ha−1 year−1 for P4, while the second largest SOC stocks increase of 16.36 Mg ha−1 predicted for P4 in the top 0–30 cm soil depth under CT with the same crop rotation as under NT. This increase exceeds the SOC increase of 12.04 Mg ha−1 at a rate of 0.60 Mg ha−1 year−1 predicted for P5 in the 0–30 cm depth by 2039. CQESTR also predicted a SOC increase of 11.46 Mg ha−1 at a rate of 0.57 Mg ha−1 year−1 for P5 under the CT scenario. An increase of SOC at 2.25 Mg ha−1 in the 0–30 cm soil depth for the Soy-CS(NT) in P5 is about half of 4.25 Mg ha−1 in P4 under the CT management scenario. Predicted SOC increase of 2.42 Mg ha−1 in P4 and 1.89 Mg ha−1 for P5 in the 0–30 cm soil depth are predicted under NT with Soy/Corn(NT), while decreases of 2.78 Mg ha−1 and 3.34 Mg ha−1 in the 0–30 cm soil depth predicted for P4 and P5 under Soy/Corn(CT), respectively.
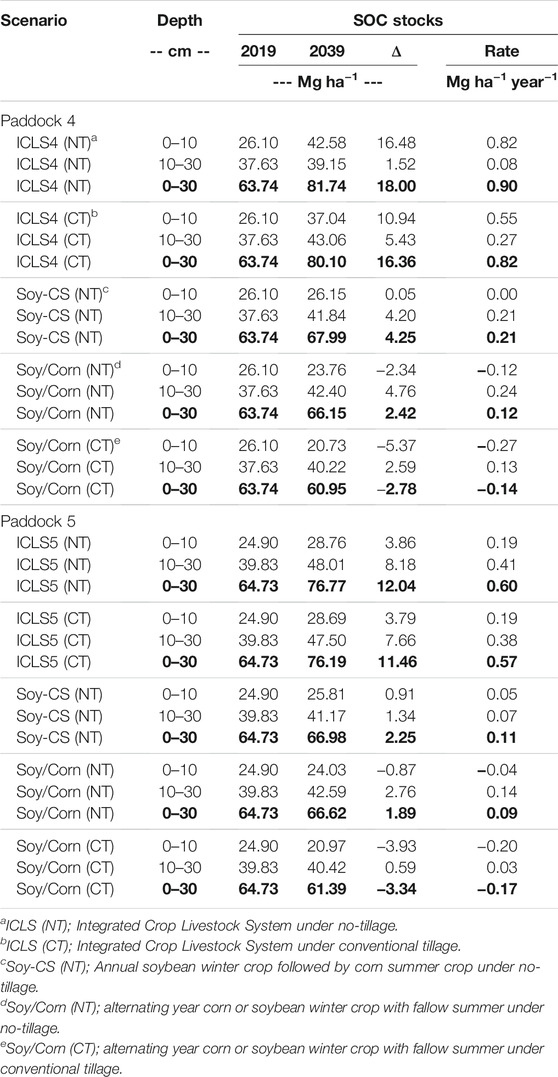
TABLE 4. Simulated soil organic carbon (SOC) stocks and rate of C changes for Paddock 4 and Paddock 5 between 2019–2039 under five management scenarios in a Typic Acrustox soil (Santo Antônio de Goiás, GO, Brazil). Bold values indicate the sum of total SOC content in the top 30-cm soil.
Discussion
CQESTR Model Performance
The regression results illustrate that CQESTR accurately simulates long-term SOC dynamics under ICLS management for the tropical savannah conditions (Figure 3). The linear fit of simulated vs. observed SOC explained 94% of the variation with the MSD of 7.55, indicating that the model captured spatial-temporal dynamics of SOC in the topsoil well despite limited SOC data. Regression analysis for the long-term data from across North America (Liang et al., 2009), however, resulted in a higher r (0.98), feasibly due to a large number of pairs of predicted and measured data analyzed (306) and for the much wider range of SOM examined (7.3–57.9 g kg−1). The relatively small (7.32 Mg ha−1) lack of correlation (LC) between the observed and simulated values indicated that CQESTR prediction errors were mainly associated with data scattering. The factors such as the variability of SOC in the field, especially under paddock conditions, and sensitivity limits of the precision of SOC measurement related to sample collection, processing, and analysis. Leite et al. (2009) reported that square bias (SB) was the highest component of the MSD when simulating SOC under several tillage management systems under tropical conditions. CQESTR simulated SOC dynamics under different management systems in some soils better than others under tropical conditions (Leite et al., 2009).
Measured and simulated SOC stocks in the topsoil increased faster in P4 (Figure 4) than in P5. This increase in SOC stocks is most likely because Urochloa spp. is cultivated in P4 as a cover crop in the rotation for a longer duration than in P5. The measured SOC values in P4 (Figure 4) were similar to that for P5 (Figure 5). The rapid increase of SOC stocks in P4 and P5 is most likely due to the high capacity of these soils to retain SOC because of their high clay content (Table 2) and the presence of iron and aluminum oxides, which results in C stabilization (Leite et al., 2009; Bayer et al., 2011). Also, it could be due to the insufficient removal of plant and dung residues from the samples during soil preparation as evident from the large standard deviation of the samples (Gollany et al., 2013). However, the large standard deviation could also occur because the studies areas were production sites and not small plot experiments with replication.
Higher SOC values in the top 10 cm for P4 in 2010 and 2015 were observed (Figure 3), although during the ICLS, P5 had the more complex crop rotation cycle in that period. Both areas were under similar edaphic conditions; however, P4 had more time in the pasture phase than P5 (Table 1) (4.5 vs. 3.5 years pasture). Another reason could be the soil disturbance at seeding events, even under NT, which occurred more often in P5 at the beginning of the ICLS. Crop intensification started in P4 after 2013/14. In 2019/20, common bean and rice + Urochloa were planted during the summer season, which added more crop residues to P4. The rice + Urochloa consortium is a modification in the rotation of P4 to increase biomass and C input into the system.
Additionally, P4 is smaller (7.5 ha) than P5 (8.1 ha) but has received the same total amount of manure. Therefore, it is expected to reach the pre-agricultural SOC stocks earlier than P5 (Figures 3, 4). Also, the influence of grazing cattle stimulates root growth and exudate production, which can modify the ratio of root and above-ground biomass and the quality of the C added to the soil (Bayer et al., 2011) and consequently influence C stocks and soil organic matter decomposition in the soil profile.
Paddock 5 received more legumes, typically soybean, than P4 and this could be another reason for the difference in SOC stocks of the two paddocks. Soybean with high biological N fixation efficiency is usually cultivated without N fertilizer, using only inoculation with bacteria of the genus Rhizobium spp. Most of this N is exported in grains, and the negative or null net N balance in cropping systems reduces biomass yields and C accumulation (Sisti et al., 2004; Urquiaga et al., 2006; Bayer et al., 2011). These authors recommend the introduction of legumes as green manure in the rotation to promote soil C sequestration. According to Sisti et al. (2004) and Souza et al. (2009), N from legumes that biologically fix N can promote more C accumulation than N from mineral sources. These studies, however, were carried out in long-term experiments of grain crop systems. Our understanding of the C and N cycles in complex systems such as ICLS is still limited (Soussana and Lemaire, 2014).
CQESTR Simulated Scenarios
A comparison among the simulated scenarios by 2039 indicate differences in SOC stocks at the 0–10 cm depth (Table 4). Paddock 4 under NT had the largest SOC stocks increase of 42.58 Mg ha−1 (28%) at a rate of 0.82 Mg ha−1 year−1, exceeding SOC stocks increase of 28.76 Mg ha−1 (19%) at a rate of 0.19 Mg ha−1 year−1 for P5, by 2039. Relative to P4 under NT, 5.55 Mg ha−1 less SOC was predicted at the 0–10 cm soil depth for P4 under CT at an annual biomass input of 24.7 Mg ha−1 year−1 (Table 5), relative to P4 under NT. This is likely because of less soil disturbance and residue decomposition, consequently reduced mineralization rate and less C losses under NT compared to CT. The rate of SOC stocks changes in the 0–30 cm soil depth for ICLS(NT) and ICLS(CT) in P4 were 0.90 and 0.82 Mg ha−1 year−1, respectively (Table 4). This is consistent with other soil tillage studies. No-till promotes slower crop residue turnover and mineralization than CT (Sherrod et al., 2003), and less SOC loss (West and Post, 2002). In P4 under ICLS, especially under NT, most of the simulated SOC increase occurred in the 0–10 cm soil depth, while little changes in SOC were predicted for the 10–30 cm soil depth SOC, leading to more SOC in the top 10 cm than in the underlying 20-cm layer by 2039. The changes in SOC were due to the difference in the SOC accumulation rates of 0.82 vs. 0.08 Mg ha−1 year−1 for the 0–10 cm and 10–30 cm depths, respectively. Whereas in P5, larger SOC increases predicted at 10–30 cm depth in all the simulations. One possible explanation can be the deposition of large amounts of stubble in the ICLS, which under NT stays on the surface and is slowly incorporated into the soil. The stratification of SOC in NT is a widely reported characteristic of this practice (Mrabet, 2002; Sá and Lal, 2009). The proportionally longer participation of the pasture phase in P5 compared to P4 in the 2012–2020 period, that allowed better grass root development, is likely the reason for the higher SOC accumulation rate at the 10-30 cm depth in P5 (0.41 Mg ha−1 year−1), compared to P4 (0.08 Mg ha−1 year−1). The higher SOC accumulation rate in the lower soil layer under CT can be explained by the incorporation of the surface litter into the lower soil layer.
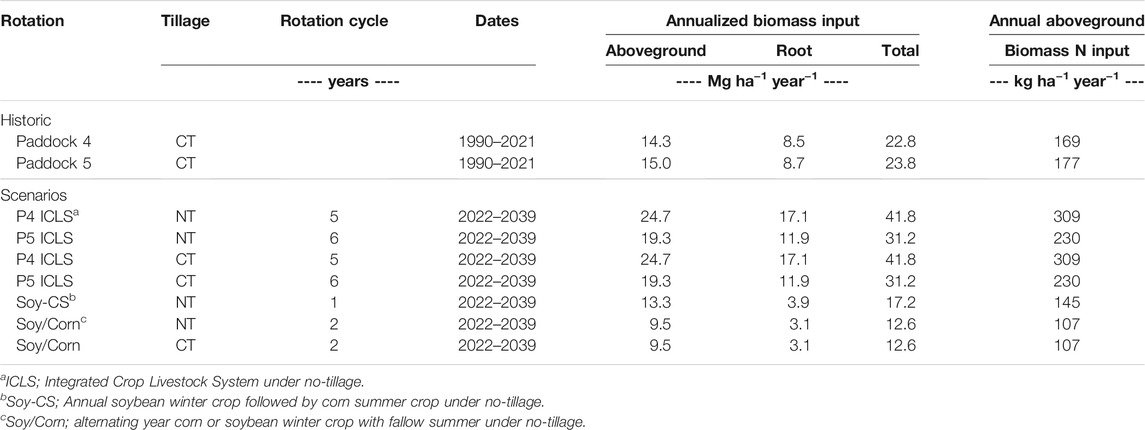
TABLE 5. Annualized biomass inputs for Paddock 4 (P4), Paddock 5 (P5) and future rotation scenarios.
The role of roots in SOC accumulation or decomposition is still not completely understood (Dijkstra et al., 2020). On one hand, rhizodeposition is a great contributor to SOC stabilization, mainly through stimulating microorganisms. Mineral associated organic matter (MAOM) is protected from further microbial decomposition, and according to Cotrufo et al. (2013) MAOM is predominantly formed from microbial products. On the other hand, plant roots are suggested to be responsible for the destabilization of SOC in a process called rhizosphere priming effect, that is, rhizodeposition is used as substrate by a group of microbes, enhancing SOC decomposition (Huo et al., 2017). Nitrogen uptake by plants can increase competition with microbes that can further stimulate SOC decomposition by microorganisms. In fact, the contribution of the root system to SOC is the result of a balance between its SOC stabilizing and reactivating/priming effect.
Organic matter formation and persistence in soils depend on environmental conditions, soil microbiota, the quality of soil minerals, and soil chemical and physical properties, especially soil structure (Hunt et al., 2020). Soil C stabilization and storage can occur by chemical stabilization, biochemical resistance, and physical protection. The adoption of NT increases water retention and lowers soil temperature, which favors microbial activity. Furthermore, fewer soil perturbations, favor build-up of larger soil aggregates (Madari et al., 2005), conferring more physical protection (Barreto et al., 2009).
The amount of crop residue is of key importance in accumulating soil C, particularly in the Cerrado biome where high temperatures and humidity during the rainy season do not favor crop residue maintenance over the soil surface. Soil organic C stocks were predicted to increase only by 2.42 and 1.89 Mg ha−1 at 0–30 cm between 2019 and 2039 for Paddock 4 and 5, respectively, under Soy/Corn(NT), which has alternating years of soybean and corn crops (Table 4). Introducing corn as a second harvest in the same year (Soy-CS (NT)) which resulted in somewhat higher annual biomass input due to corn residues (13.3 Mg ha−1 year−1 vs. 9.5 in Soy-CS and Soy/Corn, respectively), did not increase SOC stocks substantially (4.25 and 2.25 Mg ha−1 in P4 and P5, respectively), especially compared to ICLS (Tables 4 and 5). This indicates that providing N through grain legumes without large increase in crop biomass will not result in substantial soil C accrual. Also, as mentioned before, in the case of grain legumes, like soybean, most of the N is removed with the grain, which results in no positive N balance in the system (Sisti et al., 2004). It is well known that positive N balance in the soil is necessary to achieve C accumulation due to a narrow range (10–14) of soil C:N ratio in most soils.
Therefore, even under NT, low disturbance systems such as Soy/CS(NT) with relatively low residue inputs to the soil are less likely to improve SOC as in ICLS with high residue and manure inputs. Soil organic C stocks loss are predicted for scenarios that have annual alternating corn and soybean crop with fallow under conventional tillage (Soy/Corn(CT)). Decreases in SOC stocks of 2.78 and 3.34 Mg ha−1 at rates of −0.14 and −0.17 Mg ha−1 year−1 in the 0–30 cm soil depth under the Soy/Corn(CT) scenario; respectively, for P4 and P5 are predicted. Stockmann et al. (2013) reported that SOC negatively correlated with tillage. The above discussion shows that single promising conservative management practice adoption will not necessarily result in soil C sequestration; therefore, interactions of all the components need to be considered when managing ICLS (Valkama et al., 2020).
In summary, the CQESTR model predicted an increase in SOC stocks for both the NT and CT scenario under ICLS. Predicted SOC increased by 18.0 (28%) and 12.04 Mg ha−1 (19%) at the rate of 0.90 and 0.60 Mg ha−1 year−1 under current ICLS management for Paddock 4 and Paddock 5, respectively, by 2039. In single crop rotations under NT (i.e., Soy-CS and Soy/Corn) SOC accumulation at 0–30 cm was still predicted (between 6.67 and 2.92%), but in Soy/Corn under NT, at the 0-10 cm depth, SOC loss was predicted. Clearly, SOC accumulation in ICLS was favored by the pasture phase, and by introducing brachiaria grass in the rotation.
Conclusion
The CQESTR model was validated for the edaphoclimatic conditions of the Cerrado biome in Brazil, and successfully predicted the effect of several agricultural management practices in Integrated Crop-Livestock Systems (ICLS). The model captured spatial-temporal dynamics of SOC very well. The CQESTR predicted SOC increases by 18.0 (28%) and 12.04 Mg ha−1 (19%) for Paddock 4 (with the long pasture phase of Urochloa spp.), and Paddock 5 (with the shorter pasture phase of Urochloa spp.), respectively, by 2039. The use of the extended pasture phase without the crop phase was found to be the best management to increase carbon stocks and could assist Brazilian national initiatives aimed at restoring degraded pasture areas (i.e., “ABC Plan”), as well as reducing carbon dioxide emission from the soil.
Because of the limited measured long-term data for the ICLS under tropical climate, process-based models can be a cost-effective tool to predict soil C stocks change under several ICLS scenarios, and to analyze soil management options and compare impacts of each scenario on SOC stocks.
The grass root biomass and root distribution under tropical savanna conditions may diverge widely from the characteristics of temperate grasses. CQESTR’s estimation of SOC stocks could be improved with site-specific below-ground biomass and root distribution data. More long-term studies, SOC data, and root biomass for tropical grasses from diverse tropical biomes are needed to improve SOC stocks prediction. Furthermore, in comparing different management with complex rotations such as ICLS, soil samples must be taken during the same phases of the ICLS rotation. Careful sampling and sample cleaning can reduce plant and animal residue and reduce particulate organic matter or light fraction C incorrectly quantified as part of the stable SOC pool of the sample and improve long-term SOC stocks prediction.
Data Availability Statement
The raw data supporting the conclusion of this article will be made available by the authors, without undue reservation.
Author Contributions
JO: sampling collection and analysis, data collection and curation, investigating, methodology, validation, visualization, formal analysis, writing, review and editing. HG: conceptualization, data curation, CQESTR software, formal analysis, funding, investigating, methodology, project administration, resources, supervision, validation, visualization, formal analysis, writing—original draft, writing—review and editing. RP, data curation, visualization, writing—review and editing. LL, writing—review and editing. BM: data curation, formal analysis, funding, investigating, methodology, project administration, resources, supervision, writing—review and editing. MC: data curation, formal analysis, funding, methodology, project administration, resources, supervision, writing—review and editing. PM: data curation, formal analysis, funding, investigating, methodology, project administration, resources, supervision, writing—review and editing.
Funding
The study was financially supported by Embrapa (02.11.05.001; 01.11.01.002; 20.18.03.043) and CNPq (562601/2010-4). The authors gratefully acknowledge CAPES for scholarship funding number: 14318/13-00 (JMO) and CNPq for PQ2 fellowship (BEM) and the U.S. Department of Agriculture-Agricultural Research Service. This publication is also based upon work supported by the U.S. Department of Agriculture-Agricultural Research Service under the ARS GRACEnet Project. Mention of trade names or commercial products in this publication does not imply recommendation or endorsement by the U.S. Department of Agriculture or Embrapa. USDA and Embrapa are equal opportunity providers and employers.
Conflict of Interest
The authors declare that the research was conducted in the absence of any commercial or financial relationships that could be construed as a potential conflict of interest.
Publisher’s Note
All claims expressed in this article are solely those of the authors and do not necessarily represent those of their affiliated organizations, or those of the publisher, the editors and the reviewers. Any product that may be evaluated in this article, or claim that may be made by its manufacturer, is not guaranteed or endorsed by the publisher.
References
Agritempo, (2014). Sistema de monitoramento agrometeorológico. Embrapa Informática Agropecuária/CEPAGRI-Meteorologia Unicamp. Available at: https://www.agritempo.gov.br/agritempo/ (Accessed Jul 7, 2021).
Al-Adamat, R., Rawajfih, Z., Easter, M., Paustian, K., Coleman, K., Milne, E., et al. (2007). Predicted Soil Organic Carbon Stocks and Changes in jordan between 2000 and 2030 Made Using the Gefsoc Modelling System. Agric. Ecosyst. Environ. 122 (1), 35–45. doi:10.1016/j.agee.2007.01.006
Assmann, J. M., Martins, A. P., Anghinoni, I., de Oliveira Denardin, L. G., de Holanda Nichel, G., de Andrade Costa, S. E. V. G., et al. (2017). Phosphorus and Potassium Cycling in a Long-Term No-Till Integrated Soybean-Beef Cattle Production System under Different Grazing Intensities Insubtropics. Nutr. Cycl. Agroecosyst. 108, 21–33. doi:10.1007/s10705-016-9818-6
Balbino, L. C., Cordeiro, L. A. M., Oliveira, P., Kluthcouski, J., Galerani, P. R., and Vilela, L. (2012). Agricultura sustentável por meio da integração lavoura-pecuária-floresta (ilpf). Inform. Agron. 2 (138), 1–18.
Barreto, R. C., Madari, B. E., Maddock, J. E. L., Machado, P. L. O. A., Torres, E., Franchini, J., et al. (2009). The Impact of Soil Management on Aggregation, Carbon Stabilization and Carbon Loss as CO2 in the Surface Layer of a Rhodic Ferralsol in Southern Brazil. Agric. Ecosyst. Environ. 132 (3-4), 243–251. doi:10.1016/j.agee.2009.04.008
Bayer, C., Amado, T. J. C., Tornquist, C. G., Cerri, C. E. C., Dieckow, J., Zanatta, J. A., et al. (2011). “Estabilização Do carbono no solo e mitigação das emissões de gases de efeito estufa na agricultura conservacionista,” in Tópicos Em Ciência Do Solo. Editors O. Klauberg Filho, A. L. Mafra, L. C. Gatiboni, and M. G. Viçosa (Viçosa, MG: Sociedade Brasileira de Ciência do Solo), 55–117.
Bertsch, P. M., and Bloom, P. R. (1996). “Aluminium,” in Methods of Soil Analysis. Editors D. L. Sparks, A. L. Page, P. A. Helmke, R. H. Loeppert, P. N. Soltanpour, M. A. Tabatabaiet al. (Madison, Wisconsin, USA: Soil Science Society of America and American Society of Agronomy), 517–550.
Bieluczyk, W., Piccolo, M. C., Pereira, M. G., Moraes, M. T., Soltangheisi, A., Bernardi, A. C. C., et al. (2020). Integrated Farming Systems Influence Soil Organic Matter Dynamics in Southeastern Brazil. Geoderma 371(sn), 114368. doi:10.1016/j.geoderma.2020.114368
Boddey, R. M., Macedo, R., Tarré, R. M., Ferreira, E., de Oliveira, O. C., de P. Rezende, C., et al. (2004). Nitrogen Cycling in Brachiaria Pastures: the Key to Understanding the Process of Pasture Decline. Agric. Ecosyst. Environ. 103, 389–403. doi:10.1016/j.agee.2003.12.010
Bolinder, M. A., Angers, D. A., Bélanger, G., Michaud, R., and Laverdière, M. R. (2002). Root Biomass and Shoot to Root Ratios of Perennial Forage Crops in Eastern Canada. Can. J. Plant Sci. 82, 731–737. doi:10.4141/P01-139
Bolinder, M. A., Angers, D. A., Gregorich, E. G., and Carter, M. R. (1999). The Response of Soil Quality Indicators to Conservation Management. Can. J. Soil Sci. 79, 37–45. doi:10.4141/s97-099
Brasil (2021a). Plano Setorial para Adaptação à Mudança do Clima e Baixa Emissão de Carbono na Agropecuária 2020-2030: Plano Operacional/Ministério da Agricultura, Pecuária e Abastecimento. Secretaria de Inovação, Desenvolvimento Rural e Irrigação. Brasília : Mapa/DEPROS. 133p. Available at: https://www.gov.br/agricultura/pt-br/assuntos/sustentabilidade/plano-abc/arquivo-publicacoes-plano-abc/final-isbn-plano-setorial-para-adaptacao-a-mudanca-do-clima-e-baixa-emissao-de-carbono-na-agropecuaria-compactado.pdf (Accessed Jan 13, 2022).
Brasil (2021b). Brazil’s Nationally Determined Contribution (NDC). Annex: Information to Facilitate Clarity, Transparency and Understanding of Brazil’s NDC. Available at: https://www4.unfccc.int/sites/ndcstaging/PublishedDocuments/Brazil%20First/Brazil%20First%20NDC%20(Updated%20submission).pdf (Accessed Jan 13, 2022).
Brasil (2020). Intended Nationally Determined Contribution towards Achieving the Objective of the United Nations Framework Convention on Climate Change. Available at: https://www4.unfccc.int/sites/ndcstaging/PublishedDocuments/Brazil%20First/BRAZIL%20iNDC%20english%20FINAL.pdf (Accessed Jan13, 2022).
Brasil (2012). Plano setorial de mitigação e de adaptação às mudanças climáticas para a consolidação de uma economia de baixa emissão de carbono na agricultura : Plano ABC (Agricultura de Baixa Emissão de Carbono). Ministério da Agricultura, Pecuária e Abastecimento, Ministério do Desenvolvimento Agrário, coordenação da Casa Civil da Presidência da República. Brasília : MAPA/ACS. 173 p. ISBN 978-85-7991-062-0. Available at: https://www.gov.br/agricultura/pt-br/assuntos/sustentabilidade/plano-abc/arquivo-publicacoes-plano-abc/download.pdf (Accessed Jan 13, 2022).
Carauta, M., Troost, C., Guzman-Bustamante, I., Hampf, A., Libera, A., Meurer, K., et al. (2021). Climate-related Land Use Policies in Brazil: How Much Has Been Achieved with Economic Incentives in Agriculture? Land Use Policy 109(sn), 105618. doi:10.1016/j.landusepol.2021.105618
Cardoso, A. S., Berndt, A., Leytem, A., Alves, B. J. R., de Carvalho, I. d. N. O., de Barros Soares, L. H., et al. (2016). Impact of the Intensification of Beef Production in Brazil on Greenhouse Gas Emissions and Land Use. Agric. Syst. 143, 86–96. doi:10.1016/j.agsy.2015.12.007
Carvalho, A. M., de Oliveira, W. R. D., Ramos, M. L. G., Coser, T. R., de Oliveira, A. D., Pulrolnik, K., et al. (2017). Soil N2O Fluxes in Integrated Production Systems, Continuous Pasture and Cerrado. Nutr. Cycl Agroecosyst 108, 69–83. doi:10.1007/s10705-017-9823-4
Carvalho, J. L. N., Raucci, G. S., Cerri, C. E. P., Bernoux, M., Feigl, B. J., Wruck, F. J., et al. (2010). Impact of Pasture, Agriculture and Crop-Livestock Systems on Soil C Stocks in Brazil. Soil Tillage Res. 110 (1), 175–186. doi:10.1016/j.still.2010.07.011
Conab (2021). Série histórica das safras. [Online]. Available: https://www.conab.gov.br/info-agro/safras/serie-historica-das-safras?limitstart=0 (Accessed November 14, 2021).
Cortner, O., Garrett, R. D., Valentim, J. F., Ferreira, J., Niles, M. T., Reis, J., et al. (2019). Perceptions of Integrated Crop-Livestock Systems for Sustainable Intensification in the Brazilian Amazon. Land Use Policy 82, 841–853. doi:10.1016/j.landusepol.2019.01.006
Cotrufo, M. F., Wallenstein, M. D., Boot, C. M., Denef, K., and Paul, E. (2013). The Microbial Efficiency-Matrix Stabilization (MEMS) Framework Integrates Plant Litter Decomposition with Soil Organic Matter Stabilization: Do Labile Plant Inputs Form Stable Soil Organic Matter? Glob. Change Biol. 19, 988–995. doi:10.1111/gcb.12113
Damian, J. M., da Silva Matos, E., e Pedreira, B. C., de Faccio Carvalho, P. C., Premazzi, L. M., Williams, S., et al. (2021). Predicting Soil C Changes after Pasture Intensification and Diversification in Brazil. Catena 202, 105238. doi:10.1016/j.catena.2021.105238
Dell, C. J., Gollany, H. T., Adler, P. R., Skinner, R. H., and Polumsky, R. W. (2018). Implications of Observed and Simulated Soil Carbon Sequestration for Management Options in Corn-Based Rotations. J. Environ. Qual. 47(4), 617–624. doi:10.2134/jeq2017.07.0298
Franzluebbers, A. J., Chappell, J. C., Shi, W., and Cubbage, F. W. (2016). Greenhouse Gas Emissions in an Agroforestry System of the southeastern USA. Nutr. Cycl. Agroecosyst. 108, 85–100. doi:10.1007/s10705-016-9809-7
Franzluebbers, A. J., Sawchik, J., and Taboada, M. A. (2014). Agronomic and Environmental Impacts of Pasture–Crop Rotations in Temperate north and South america. Agric. Ecosyst. Environ. 190 (sn), 18–26. doi:10.1016/j.agee.2013.09.017
Gauch, H. G., Hwang, J. T. G., and Fick, G. W. (2003). Model Evaluation by Comparison of Model‐Based Predictions and Measured Values. Agron.j. 95 (6), 1442–1446. doi:10.2134/agronj2003.1442
Gee, G. W., and Or, D. (2002). “Particle-size Analysis,” in Methods of Soil Analysis. Editors J. H. Dane, G. Clarke-Topp, G. S. Campbell, R. Horton, W. A. Jury, R. N. Donaldet al. (Madison, Wisconsin, USA: Soil Science Society of America), 255–293.
Ghani, A., Sarathchandra, U., Perrott, K. W., Wardle, D. A., Singleton, P., and Dexter, M. (1996). Biological and Biochemical Quality of Pastoral Soils: Spatial and Temporal Variability. ProNZG 58, 211–218. doi:10.33584/jnzg.1996.58.2193
Godde, C. M., De Boer, I. J. M., Ermgassen, E. z., Herrero, M., Van Middelaar, C. E., Muller, A., et al. (2020). Soil Carbon Sequestration in Grazing Systems: Managing Expectations. Climatic Change 161 (sn), 385–391. doi:10.1007/s10584-020-02673-x
Gollany, H. T., Delgrosso, S. J., Dell, C. J., Adler, P. R., and Polumsky, R. W. (2021). Assessing the Effectiveness of Agricultural Conservation Practices in Maintaining Soil Organic Carbon under Contrasting Agroecosystems and a Changing Climate. Soil Sci. Soc. Am. J. 85 (5), 1362–1379. doi:10.1002/saj2.20232
Gollany, H. T., Fortuna, A. M., Samuel, M. K., Young, F. L., Pan, W. L., and Pecharko, M. (2013). Soil Organic Carbon Accretion vs. Sequestration Using Physicochemical Fractionation and CQESTR Simulation. Soil Sci. Soc. America J. 77 (2), 618–629. doi:10.2136/sssaj2012.0303
Grossman, R. B., and Reinsch, T. G. (2002). “Bulk Density and Linear Extensibility,” in Methods of Soil Analysis. Editors J. H. Dana, and G. C. Topp (Madison, Wisconsin, USA: Soil Science Society of America), 201–228.
Hunt, J. R., Celestina, C., and Kirkegaard, J. A. (2020). The Realities of Climate Change, Conservation Agriculture and Soil Carbon Sequestration, Glob. Change Biol. 26(6), 3188–3189. doi:10.1111/gcb.15082
Huo, C., Luo, Y., and Cheng, W. (2017). Rhizosphere Priming Effect: a Meta-Analysis. Soil Biol. Biochem. 111, 78–84. doi:10.1016/J.SOILBIO.2017.04.003
IBGE (2002). Mapa de clima do Brasil. 1:5.000.000. Rio de Janeiro: Ministério do Planejamento, Orçamento e Gestão/Instituto Brasileiro de Geografia e Estatística.
Kamoni, P. T., Gicheru, P. T., Wokabi, S. M., Easter, M., Milne, E., Coleman, K., et al. (2007). Evaluation of Two Soil Carbon Models Using Two Kenyan Long Term Experimental Datasets. Agric. Ecosyst. Environ. 122 (1), 95–104. doi:10.1016/j.agee.2007.01.011
Kluthcouski, J., and Yokoyama, L. P. (2003). “Opções de integração lavoura-pecuária,” in Integração Lavoura-Pecuária. Editors J. Kluthcouski, L. F. Stone, and H. Aidar (Santo Antônio de Goiás: Embrapa Arroz e Feijão), 129–141.
Kravchenko, A. N., and Robertson, G. P. (2011). Whole-profile Soil Carbon Stocks: The Danger of Assuming Too Much from Analyses of Too Little. Soil Sci. Soc. Am. J. 75 (1), 235–240. doi:10.2136/sssaj2010.0076
Kuo, S. (1996). “Phosphorus,” in Methods of Soil Analysis. Editors D. L. Sparks, A. L. Page, P. A. Helmke, R. H. Loeppert, P. N. Soltanpour, M. A. Tabatabaiet al. (Madison, Wisconsin, USA: Soil Science Society of America and American Society of Agronomy), 869–919.
Lapig (2021). Atlas digital das pastagens brasileiras. Digital atlas of Brazilian pasturelands. [Online]. Available: https://pastagem.org/atlas/map (Accessed Nov 11, 2021).
Leite, L. F., Doraiswamy, P. C., Causarano, H. J., Gollany, H. T., Milak, S., and Mendonça, E. S. (2009). Modeling Organic Carbon Dynamics under No-Tillage and Plowed Systems in Tropical Soils of Brazil Using CQESTR. Soil Tillage Res. 102 (1), 118–125. doi:10.1016/j.still.2008.08.003
Lemaire, G., Franzluebbers, A., Carvalho, P. C. d. F., and Dedieu, B. (2014). Integrated Crop-Livestock Systems: Strategies to Achieve Synergy between Agricultural Production and Environmental Quality. Agric. Ecosyst. Environ. 190 (s/n), 4–8. doi:10.1016/j.agee.2013.08.009
Liang, Y., Gollany, H. T., Rickman, R. W., Albrecht, S. L., Follett, R. F., Wilhelm, W. W., et al. (2009). Simulating Soil Organic Matter with CQESTR (V. 2.0): Model Description and Validation against Long-Term Experiments across north america. Ecol. Model. 220 (4), 568–581. doi:10.1016/j.ecolmodel.2008.11.012
Macedo, M. C. M. (2009). Integração lavoura e pecuária: o estado da arte e inovações tecnológicas. R. Bras. Zootec. 38 (Suppl. o especial), 133–146. doi:10.1590/s1516-35982009001300015
Machado, P. L. O. A., Bernardi, A. C. C., Valencia, L. I. O., Meirelles, M. S. P., Silva, C. A., Gimenez, L. M., et al. (2009). Carbon Stocks of a Rhodic Ferralsol under No-Tillage in Southern Brazil: Spatial Variability at a Farm Scale. Soil Res. 47, 253–260. doi:10.1071/SR08140
Madari, B. E., Machado, P. L. O. A., Torres, E., Andrade, A. G., and Valencia, L. I. O. (2005). No Tillage and Rotation Effects on Soil Aggregation and Organic Carbon in a Rhodic Ferralsol from Southern Brazil. Soil Tillage Res. 80 (1-2), 185–200. doi:10.1016/j.still.2004.03.006
Mauad, M., Feltran, J. C., Crusciol, C. A. C., and Alvarez, Â. C. C. (2012). Matéria seca e morfologia radicular de cultivares de arroz de terras altas em função da adubação com zinco. Revista de Ciências Agrárias 35 (1), 251–260. doi:10.19084/rca.16180
McGill, W. B., Cannon, K. R., Robertson, J. A., and Cook, F. D. (1986). Dynamics of Soil Microbial Biomass and Water-Soluble Organic C in Breton L after 50 Years of Cropping to Two Rotations. Can. J. Soil Sci. 66 (1), 1–19. doi:10.4141/cjss86-001
Moraes Sá, J. C., Tivet, F., Lal, R., Briedis, C., Hartman, D. C., dos Santos, J. Z., et al. (2014). Long-term Tillage Systems Impacts on Soil C Dynamics, Soil Resilience and Agronomic Productivity of a Brazilian Oxisol. Soil Tillage Res. 136, 38–50. doi:10.1016/j.still.2013.09.010
Mozzer, G. B. (2011). “Agriculture and Cattle Raising in the Context of a Low Carbon Economy,” in Climate Change in Brazil. Editors R. S. Motta, J. Hargrave, G. Luedemann, and M. B. S. Gutierrez (Brasília: IPEA), 107–122.
Mrabet, R. (2002). Stratification of Soil Aggregation and Organic Matter under Conservation Tillage Systems in Africa. Soil Tillage Res. 66, 119–128. doi:10.1016/S0167-1987(02)00020-X
Nelson, D. W., and Sommers, L. E. (1996). “Total Carbon, Organic Carbon, and Organic Matter,” in Methods of Soil Analysis. Editors D. L. Sparks, A. L. Page, P. A. Helmke, R. H. Loeppert, P. N. Soltanpour, M. A. Tabatabaiet al. (Madison, Wisconsin, USA: Soil Science Society of America and American Society of Agronomy), 961–1010.
Oliveira, J. M., Madari, B. E., Carvalho, M. T. M., Assis, P. C. R., Silveira, A. L. R., Leles Lima, M., et al. (2018). Integrated Farming Systems for Improving Soil Carbon Balance in the Southern Amazon of Brazil. Reg. Environ. Change 18, 105–116. doi:10.1007/s10113-017-1146-0
Pacheco, L. P., Leandro, W. M., Machado, P. L. O. A., Assis, R. L., Cobucci, T., Madari, B. E., et al. (2011). Produção de fitomassa e acúmulo e liberação de nutrientes por plantas de cobertura na safrinha. Pesq. Agropec. Bras. 46 (1), 17–25. doi:10.1590/S0100-204X2011000100003
Pariz, C. M., Costa, C., Crusciol, C. A. C., Meirelles, P. R. L., Castilhos, A. M., Andreotti, M., et al. (2017). Production, Nutrient Cycling and Soil Compaction to Grazing of Grass Companion Cropping with Corn and Soybean. Nutr. Cycl. Agroecosyst. 108, 35–54. doi:10.1007/s10705-016-9821-y
Rede ILPF (2021). Integrated Crop-Livestock Forestry Network [Online]. Available: https://www.redeilpf.org.br/index.php/rede-ilpf/o-que-e-a-rede-ilpf (Accessed November 10, 2021).
Reis, J. C., Kamoi, M. Y. T., Latorraca, D., Chen, R. F. F., Michetti, M., Wruck, F. J., et al. (2019). Assessing the Economic Viability of Integrated Crop−livestock Systems in Mato Grosso, Brazil. Renew. Agric. Food Syst. 35 (6), 631–642. doi:10.1017/s1742170519000280
Ryschawy, J., Martin, G., Moraine, M., Duru, M., and Therond, O. (2017). Designing Crop-Livestock Integration at Different Levels: Toward New Agroecological Models? Nutr. Cycl. Agroecosyst. 108, 5–20. doi:10.1007/s10705-016-9815-9
Sá, J. C. M., and Lal, R. (2009). Stratification Ratio of Soil Organic Matter Pools as an Indicator of Carbon Sequestration in a Tillage Chronosequence on a Brazilian Oxisol. Soil Tillage Res. 103, 46–56. doi:10.1016/j.still.2008.09.003
Salton, J. C., Mercante, F. M., Tomazi, M., Zanatta, J. A., Concenço, G., Silva, W. M., et al. (2014). Integrated Crop-Livestock System in Tropical Brazil: Toward a Sustainable Production System. Agric. Ecosyst. Environ. 190 (s/n), 70–79. doi:10.1016/j.agee.2013.09.023
Sant-Anna, S. A. C., Jantalia, C. P., Sá, J. M., Vilela, L., Marchão, R. L., Marchão, R. L., et al. (2017). Changes in Soil Organic Carbon during 22 Years of Pastures, Cropping or Integrated Crop/livestock Systems in the Brazilian Cerrado. Nutr. Cycl Agroecosyst 108, 101–120. doi:10.1007/s10705-016-9812-z
Santos, R. S. M., Oliveira, I. P., Morais, R. F., Urquiaga, S., Boddey, R. M., and Alves, B. J. R. (2007). Componentes da parte aérea e raízes de pastagens de brachiaria spp. Em diferentes idades após a reforma, como indicadores de produtividade em ambiente de cerrado. Pesquisa Agropecuária Trop. 37 (2), 119–124.
Sato, J. H., Carvalho, A. M., Figueiredo, C. C., Coser, T. R., Sousa, T. R., Vilela, L., et al. (2017). Nitrous Oxide Fluxes in a Brazilian Clayey Oxisol after 24 Years of Integrated Crop-Livestock Management. Nutr. Cycl. Agroecosyst. 108, 55–68. doi:10.1007/s10705-017-9822-5
Sherrod, L. A., Peterson, G. A., Westfall, D. G., and Ahuja, L. R. (2003). Cropping Intensity Enhances Soil Organic Carbon and Nitrogen in a No-Till Agroecosystem. Soil Sci. Soc. Am. J. 67 (5), 1533–1543. doi:10.2136/sssaj2003.1533
Silva, F. C. (2009). Manual de análises químicas de solos, plantas e fertilizantes. Brasília: Embrapa Informação Tecnológica.
Silveira, J. (2010). Estoques e indicadores de carbono em cerradão e cerrado strictu sensu. Mestrado em Engenharia do Meio Ambiente Dissertação. Goiânia: Universidade Federal de Goiás.
Sisti, C. P. J., dos Santos, H. P., Kohhann, R., Alves, B. J. R., Urquiaga, S., and Boddey, R. M. (2004). Change in Carbon and Nitrogen Stocks in Soil under 13 Years of Conventional or Zero Tillage in Southern Brazil. Soil Tillage Res. 76 (1), 39–58. doi:10.1016/j.still.2003.08.007
Soares, M. B., Freddi, O. S., Matos, E. S., Tavanti, R. F. R., Wruck, F. J., Lima, J. P., et al. (2020). Integrated Production Systems: An Alternative to Soil Chemical Quality Restoration in the Cerrado-Amazon Ecotone. CATENA 185, 104279. doi:10.1016/j.catena.2019.104279
Soussana, J.-F., and Lemaire, G. (2014). Coupling Carbon and Nitrogen Cycles for Environmentally Sustainable Intensification of Grasslands and Crop-Livestock Systems. Agric. Ecosyst. Environ. 190 (s/n), 9–17. doi:10.1016/j.agee.2013.10.012
Souza, E. D., Costa, S. E. V. G. A., Anghinoni, I., Carvalho, P. C. F., Andrigueti, M., and Cao, E. (2009). Estoques de carbono orgânico e de nitrogênio no solo em sistema de integração lavoura-pecuária em plantio direto, submetido a intensidades de pastejo. Rev. Bras. Ciênc. Solo 33 (6), 1829–1836. doi:10.1590/s0100-06832009000600031
Stockmann, U., Adams, M. A., Crawford, J. W., Field, D. J., Henakaarchchi, N., Jenkins, M., et al. (2013). The Knowns, Known Unknowns and Unknowns of Sequestration of Soil Organic Carbon. Agric. Ecosyst. Environ. 164, 80–99. doi:10.1016/j.agee.2012.10.001
Tadini, A. M., Xavier, A. A. P., Milori, D. M. B. P., Oliveira, P. P. A., Pezzopane, J. R., Bernardi, A. C. C., et al. (2021). Evaluation of Soil Organic Matter from Integrated Production Systems Using Laser-Induced Fluorescence Spectroscopy. Soil Tillage Res. 211 (sn), 105001. doi:10.1016/j.still.2021.105001
Thomas, G. W. (1996). “Soil pH and Soil Acidity,” in Methods of Soil Analysis. Editors A. L. Page, P. A. Helmke, R. H. Loeppert, P. N. Soltanpour, M. A. Tabatabai, C. T. Johnstonet al. (D.L. Madison, Wisconsin, USA: Soil Science Society of America and American Society of Agronomy), 475–490. Sparks.
Tornquist, C. G., Mielniczuk, J., and Cerri, C. E. P. (2009). Modeling Soil Organic Carbon Dynamics in Oxisols of Ibirubá (Brazil) with the Century Model. Soil Tillage Res. 105 (1), 33–43. doi:10.1016/j.still.2009.05.005
Urquiaga, S., Jantalia, C. P., Resende, A. S., Alves, B. J. R., and Boddey, R. M. (2006). Capítulo 7: Contribuição da fixação biológica de nitrogênio na produtividade dos sistemas agrícolas na américa latina.
Valkama, E., Kunypiyaeva, G., Zhapayev, R., Karabayev, M., Zhusupbekov, E., Perego, A., et al. (2020). Can Conservation Agriculture Increase Soil Carbon Sequestration? A Modelling Approach. Geoderma 369 (sn), 114298. doi:10.1016/j.geoderma.2020.114298
West, T. O., and Post, W. M. (2002). Soil Organic Carbon Sequestration Rates by Tillage and Crop Rotation. Soil Sci. Soc. Am. J. 66 (6), 1930–1946. doi:10.2136/sssaj2002.1930
Wright, R. J., and Stuczynski, T. I. (1996). “Atomic Absorption and Flame Emission Spectrometry,” in Methods of Soil Analysis. Editors D. L. Sparks, A. L. Page, P. A. Helmke, R. H. Loeppert, P. N. Soltanpour, M. A. Tabatabaiet al. (Madison, Wisconsin, USA: Soil Science Society of America and American Society of Agronomy), 65–90.
Keywords: corn, cerrado biome, pasture, soil organic matter, soybean, neotropical savannah, no-tillage, dryland rice
Citation: Oliveira JM, Gollany HT, Polumsky RW, Madari BE, Leite LFC, Machado PLOA and Carvalho MTM (2022) Predicting Soil Organic Carbon Dynamics of Integrated Crop-Livestock System in Brazil Using the CQESTR Model. Front. Environ. Sci. 10:826786. doi: 10.3389/fenvs.2022.826786
Received: 01 December 2021; Accepted: 21 January 2022;
Published: 21 March 2022.
Edited by:
Rosa Francaviglia, Council for Agricultural and Economics Research (CREA), ItalyReviewed by:
Júnior Melo Damian, University of São Paulo, BrazilHaithem Bahri, National Research Institute for Rural Engineering, Water and Forestry (INRGREF), Tunisia
Copyright © 2022 Oliveira, Gollany, Polumsky, Madari, Leite, Machado and Carvalho. This is an open-access article distributed under the terms of the Creative Commons Attribution License (CC BY). The use, distribution or reproduction in other forums is permitted, provided the original author(s) and the copyright owner(s) are credited and that the original publication in this journal is cited, in accordance with accepted academic practice. No use, distribution or reproduction is permitted which does not comply with these terms.
*Correspondence: Hero T. Gollany, aGVyby5nb2xsYW55QHVzZGEuZ292