- 1Department of Geography, Landscape Ecology and Geoinformation Science, Kiel University, Kiel, Germany
- 2Department of Geography, Earth Observation and Modelling, Kiel University, Kiel, Germany
Soil compaction results whenever applied soil stress by machinery exceed the soil strength. Both, soil strength and stress, are spatially and temporally highly variable, depending on the weather situation, the current crop type, and the machinery used. Thus, soil compaction risk is very dynamic, changes from day to day and from field to field. The objective of this study was to analyze the spatio-temporal dynamics of soil compaction risk and to identify hot-spot areas of high soil compaction risk at regional scale. Therefore, we selected a study area (∼2,000 km2) with intensive arable farming in Northern Germany, having a high share of cereals, maize and sugar beets. Sentinel-2 images were used to derive the crop types for a 5-years crop rotation (2016–2020). We calculated the soil compaction risk using an updated version of the SaSCiA-model (Spatially explicit Soil Compaction risk Assessment) for each single day of the period, with a spatial resolution of 20 m. The results showed the dynamic changes of soil compaction risk within a year and throughout the entire crop rotation. The relatively dry years 2016 and 2018–2020 reduced the soil compaction risk even at high wheel loads applied to soil during maize and sugar beet harvest. Contrary, high precipitation in 2017 increased the soil compaction risk considerably. Focusing on the complete 5-year period, 2.7% of the cropland area was identified as hot-spots of soil compaction risk, where the highest soil compaction risk class (“extremely high”) occurred every year. Additionally, 39.8% of the cropland was affected by “extremely high” soil compaction at least in one of the 5 years. Although the soil compaction risk analysis does not provide information on the actual extent of the compacted area, the identification of risk areas within a period may contribute to understand the dynamics of soil compaction risk in crop rotation at regional scale and provide advice to mitigate further soil compaction in areas classified as high risk.
Introduction
Soil compaction is one of the main soil degradation processes on agricultural land worldwide (FAO, 2015). This degradation process is expected to continue in the coming centuries due to intensive field traffic activities with heavy machinery (Keller et al., 2017; Keller et al., 2019; Techen et al., 2020). Continued soil compaction, however, contradicts the sustainable development goal 15 (SDG15), which aims to achieve land degradation neutrality by sustainable land use management. Reducing soil compaction is therefore necessary to achieve the SDG 15 and to enable a sustainable soil use.
Soil compaction is defined as an increase of bulk density while pore volume decreases (Horn et al., 1995). Compared to an uncompacted soil, compacted soils have a lower air capacity, reduced water infiltration, lower air permeability, lower biological activity, reduced root and plant growth (e.g., Horn et al., 1995; Weisskopf et al., 2010; Destain et al., 2016; Szatanik-Kloc et al., 2018). Therefore, compacted soils are more susceptible to surface water runoff and soil erosion (Alaoui et al., 2018; Keller et al., 2019). In addition, soil compaction results often in lower yields (Arvidsson and Håkansson, 2014; Daigh et al., 2020). As soil compaction is persistent (Keller et al., 2017; Seehusen et al., 2021), especially in the subsoil, the environmental effects are present in the long term. As exemplarily shown by Graves et al. (2015) for England and Wales, the total economic costs of soil degradation caused by soil compaction can be three times that of soil erosion. Thus, quantifying and localizing already compacted soils is as important as identifying areas where the risk of soil compaction is increased to prevent further soil degradation.
Calculating area percentages is challenging as soil compaction on arable land is a spatio-temporal highly dynamic process (Schjønning et al., 2015b), because crop type, soil, weather and used machinery interact (Kuhwald et al., 2018). Comparing soil strength against soil stress is the most common approach to evaluate whether soil compaction may occur during wheeling (Horn and Fleige, 2003; Horn et al., 2005). Soil strength results from soil texture, carbon content, soil structure and soil moisture (Horn et al., 1995; Horn and Fleige, 2003; Rücknagel et al., 2012; Gut et al., 2015). Soil stress depends on the wheel load, tire inflation pressure, contact area and amount of wheel passes (Horn, 2003; Horn et al., 2003; Arvidsson and Keller, 2007; Botta et al., 2009; Schjønning et al., 2012). Several studies exist that analyzed the various effects of wheeling on stress propagation and distribution inside the soil as on physical soil properties as well (e.g., Gebhardt et al., 2009; Lamandé and Schjønning, 2011; Hartmann et al., 2012; Keller et al., 2012; Berisso et al., 2013; Seehusen et al., 2019).
From field and laboratory experiments, numerous functions were derived to calculate e.g. the contact area, the soil strength at a certain soil moisture state or the stress distribution while wheeling (Keller et al., 2007; Schjønning et al., 2008; Diserens, 2009; Rücknagel et al., 2012). These functions, incorporated into more complex assessment models, allow to estimate soil compaction behavior at different spatial scales. Table 1 lists common spatial approaches and models in soil compaction research.
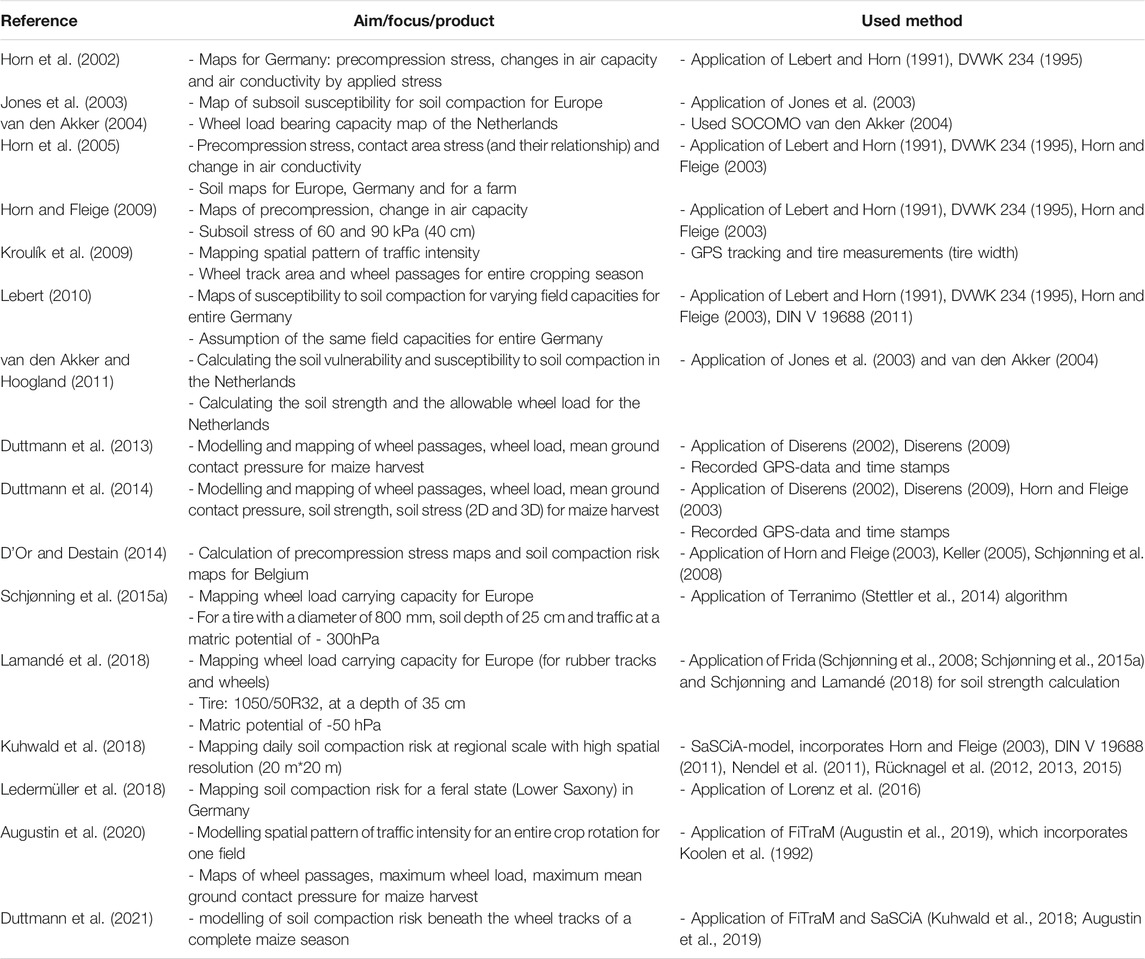
TABLE 1. Common spatial models and spatial approaches in soil compaction research (according to Kuhwald (2019).
The growing availability of high resolute data from global navigation satellite systems (GNSS) received and recorded by modern farm vehicles enables to precisely predict traffic intensity (e.g., Kroulík et al., 2009; Duttmann et al., 2013) and traffic related compaction effects at field scale (e.g., Duttmann et al., 2014; Augustin et al., 2020; Duttmann et al., 2021).
From field to larger scales, the pre-compression (or pre-consolidation stress)-concept (e.g., Lebert and Horn, 1991) has been applied in various studies (e.g., Horn et al., 2002; Horn et al., 2005; Horn and Fleige, 2009; Lebert, 2010; Destain et al., 2016). The results are maps showing the susceptibility to soil compaction (Jones et al., 2003; Lebert, 2010), the soil compaction risk (D'Or and Destain, 2014) or, by using further equations, changes in air conductivity and air capacity (Horn et al., 2002; Horn et al., 2005; Horn and Fleige, 2009). Furthermore, the wheel load carrying capacity can be derived by calculating the soil strength and setting threshold values for soil stress. Examples are given by van den Akker (2004) for the Netherlands and by Schjønning et al. (2015b) and Lamandé et al. (2018) for Europe.
One limitation of all these large scale predictions is the assumption of static conditions, either for the soil or for the used machinery data or for both. Dynamic changes of soil stress and soil strength in space and time were rarely considered.
Newer approaches try to consider the dynamics of soil stress and soil strength in soil compaction risk modelling at regional scale (Kuhwald et al., 2018; Ledermüller et al., 2018). The approach of Ledermüller et al. (2018) uses available soil moisture information at a spatial resolution of 1*1 km2 provided by the German weather service (DWD) and land use data from the Integrated Administration and Control System (IACS) to calculate soil compaction risk according to Lorenz et al. (2016). This method aims at a long-term assessment of topsoil compaction risk, which can support famers in decision making.
Kuhwald et al. (2018) developed a different approach to calculate soil compaction risk, called “SaSCiA” (Spatially explicit Soil Compaction risk Assessment). The raster-based model incorporates spatial information of the soil and current crop types and calculates the daily soil strength depending on actual soil moisture. For the calculation of soil stress, the current crop type, the actual soil moisture, crop-dependent used machinery and field traffic days are considered. The modelling results are daily maps of soil compaction risk at a spatial resolution of 20 m. As shown in a first study by Kuhwald et al. (2018), the SaSCiA-model was successfully used to simulate the daily changes of soil compaction risk, separated by different soil depths (20, 35 and 50 cm), within 1 year for two study areas. Among other aspects, the high variability of soil compaction risk within a year was evident, confirming the significant influence of the actual weather conditions in a respective region. However, Kuhwald et al. (2018) analyzed only 1 year for each study area. Therefore, variations between years for a specific study area could not accounted for.
Thus, the following research questions arise for this study: (i) whether and to what extend does soil compaction risk vary between individual years, and (ii) does a continuous calculation of soil compaction risk over a longer period enable the detection of areas within the region that are more often affected by high soil compaction risk than other areas? To answer both questions, we analyzed the soil compaction risk for a 5-year period for an intensively cropped region using an updated version of the SaSCiA-model. The objectives of this study are (i) to model and analyze the variation of soil compaction risk within the individual years and within the 5-years period (2016–2020), and (ii) to identify areas with recurring patterns of high soil compaction risk within this period. We hypothesize that (i) the variation in soil compaction risk between the single years will be strong depending on actual weather conditions, but that (ii) classes of higher soil compaction risk can be observed for the same areas in each year, which enables to delineate “hot-spot” areas of increased soil compaction risk.
This study focusses on a perennial analysis of soil compaction risk dynamics at regional scale to understand the spatio-temporal characteristics of soil compaction. Furthermore, identifying areas of different soil compaction risk during a crop rotation enables selective and specific intervention through soil management to mitigate potential soil compaction. In this way, this study may support to a more sustainable soil use as envisaged by the SDG 15.
Materials and Methods
Study Area
A 2,000 km2 region in Lower Saxony (Germany) near the city of Hildesheim was selected as study area (Figure 1). Intensive agricultural use characterizes this region. Most important crop types are winter wheat (Triticum aestivum), sugar beets (Beta vulgaris), silage maize (Zea mays) and rapeseed (Brassica napus). The study area is part of the Lower Saxony Loess Hill Country. The geology of the region is complex, resulting in diversely distributed soil parent material. Deeply weathered loess predominates along the hill slopes, while loamy deposits occur in the valleys. Shallow layers of sandy and clayey weathered materials are mostly found at the hilltops. Typical soil types at the hill slopes and valleys are Luvisols (often stagnic) and Cambisols, while Leptosols and Regosols (FAO, 2014) occur at the hilltops. Forests cover the hilly areas and the areas with shallow soil development. The climate is humid with a mean annual precipitation of 649 mm and mean annual temperature of 10.0°C (weather station Liebenburg-Othfresen; DWD, 2021). The weather conditions for the investigated period are exemplarily shown for the weather station “Liebenburg-Othfresen,” which is centrally located in the study area (Figure 2).
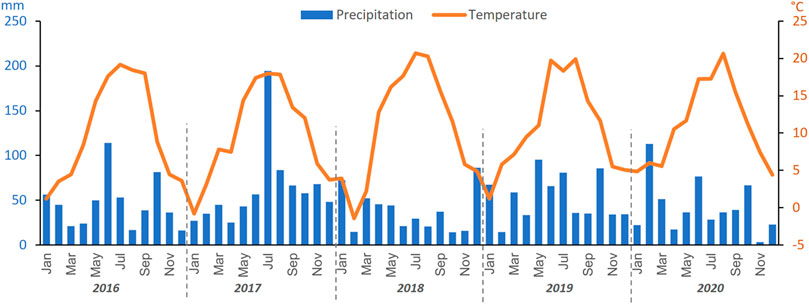
FIGURE 2. Monthly precipitation and air temperature of the weather station Liebenburg-Othfresen, located central in the study area (DWD, 2021).
Modelling, Model Structure and Input Data
The SaSCiA-model (Spatially explicit Soil Compaction risk Assessment) was used for the 5-years analysis. The freely available study by Kuhwald et al. (2018) describes the model and its components in detail. Here, we briefly explain the model and highlight the changes in the model and the used input data.
The SaSCiA-model incorporates crop type data, weather information, soil data, machinery information, the crop model “MONICA” and various pedotransfer functions (e.g., Horn and Fleige, 2003; Nendel et al., 2011; Rücknagel et al., 2012; Rücknagel et al., 2013; Rücknagel et al., 2015). Based on these components, it calculates the daily soil compaction risk for each raster cell within a selected area (Figure 3). In this study, we modelled and analyzed the subsoil compaction risk at a soil depth of 40 cm. Since this depth will not be reached by regular primary tillage and related loosening effects, soil compaction persists (Keller et al., 2017; Keller et al., 2021) and may not recover in short-term.
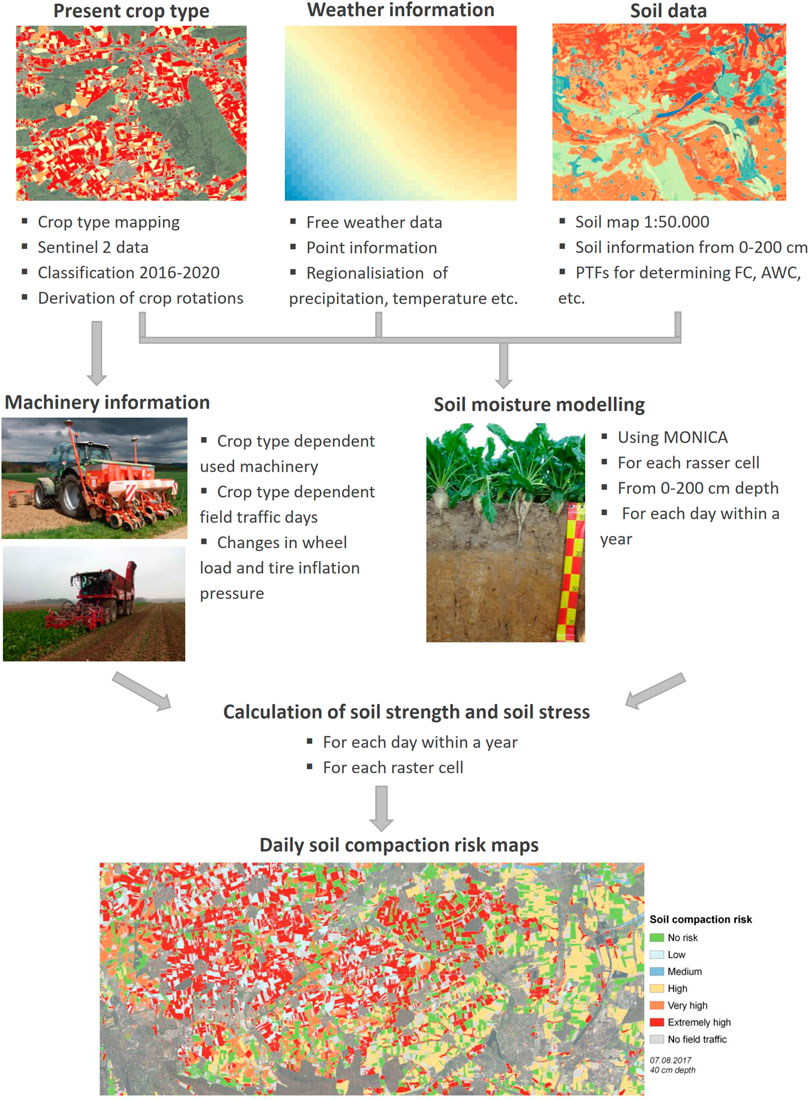
FIGURE 3. Schematic overview of the SaSCiA-model (according to Kuhwald et al., 2018).
Crop Types and Crop Rotations
The crop types and crop rotations for the period (2016–2020) were derived by land use mapping and land use classification. During each summer (July–September) of the investigation period, we mapped crop types in the study area for ground control. Field mapping data encompassed the following target classes: cereals (e.g., winter wheat, rye, barley), maize, winter rapeseed, sugar beets and grassland. Google Earth imagery supported the identiflcation of less changing land cover classes such as water bodies, evergreen/deciduous forest and sealed areas. The mapped data formed the basis for a remote sensing approach to obtain spatially continuous maps of cultivated crop types at 20 m spatial resolution.
To this end, we selected 20 cloud-free or almost cloud-free Sentinel-2 scenes, which were acquired during the vegetation periods 2016–2020 (Supplementary Table S1). Since its launch in 2015, Sentinel-2 data are widely used and established for crop type mapping (e.g., Immitzer et al., 2016). To ensure data comparability, we downloaded atmospherically corrected (Sen2Cor (Müller-Wilm, 2016); Level-2A products from the Copernicus Open Access Hub (https://scihub.copernicus.eu/). Crop types were classified by using a random forest classification algorithm implemented as QGIS plugin (dzetsaka classification; Karasiak, 2016). The random forest algorithm is a supervised ensemble classifier and well known for crop type classification. To learn the spectral signatures of the target classes (training), the classifier requires georeferenced data on known crop types (Belgiu and Drăguţ, 2016). For this purpose, we used the crop types mapped annually in the study area. The Sentinel-2 bands 2-7, 8A, 11 and 12 (20 m spatial resolution) of all selected scenes from a year served as spectral input, whereas we excluded cloudy pixels based on the standard cloud mask of the Level-2A products.
Speckle in the crop type maps was reduced by using a 3 × 3 pixel median filter. Here, speckle means natural classification noise. At field borders, for instance, different land cover are represented within one 20 m × 20 m large pixel (e.g., the crop type, field path and hedgerow or crop type of adjacent field). The result are single, erroneously classified pixels and a grainy appearing land cover map. Filtering is a common post-classification tool to refine and smoothen the map. Furthermore, we applied the IRSeL tool (Rathjens et al., 2014). IRSeL is a post-classification tool for refining remote sensing based land cover maps (e.g., interpolation of no data gaps, reduction of misclassifications between crop types and less dynamic classes; Kandziora et al., 2014; Rathjens et al., 2014). Accuracy assessment followed the suggestions by Foody (2002) using independent field mapping data, which were not used for classifier training (Supplementary Table S2).
Subsequently, the received crop types were used to calculate soil moisture, to select the machinery employed for crop-specific field traffic activities and to define time periods for these activities.
Weather Data
The weather data is used to calculate the soil moisture within the crop model MONICA. Required weather information are temperature (minimum, maximum, average), precipitation, relative humidity, Sun duration and wind speed. In the original SaSCiA-version, only one weather station was used for deriving weather information for an entire region. As weather is highly variable in space, one station may not adequately represent the weather conditions within a region of 2,000 km2. For this reason, we newly incorporated regionalized weather data in the model. In a first step, all available weather stations within the federal state “Lower Saxony” were identified and the available data automatically downloaded using the R-package “papros” (Hamer, 2019). Afterwards the data were processed in R (version 4.1.0; R Core Team, 2020) to generate regionalized weather information for each day for each of the required weather variable. From these grids, the weather input-files for each crop-soil grid cell were automatically generated. As a result, the spatial variation of weather can be considered in the calculation of soil compaction risk.
Soil Data
Soil data was extracted from the digital soil map BK 50 (scale 1:50,000) provided by the federal state agency of Lower Saxony (LBEG, 2020). The BK 50 contains information of e.g., soil type, soil horizons, soil depths, soil texture class, carbon content, gravel content and dry bulk density class, which were used for modelling. Further information about air capacity, field capacity, available field capacity and wilting point were derived according to Wessolek et al. (2009). In total, 1,800 different soil profiles were located within the study area and used for further soil compaction analysis.
Machinery Data
The study area represents a region with highly mechanized agriculture, i.e., nearly all field processes are conducted with heavy machinery. The SaSCiA-model considers the used machinery based on the present crop type. For each crop type, a machinery with fixed wheel load and tire inflation pressure is defined and the periods for potential field traffic (Supplementary Table S3; cf. Kuhwald et al., 2018). For instance, it is assumed that the winter wheat is harvested between the first and 15th of August each year using a 220 kw combine harvester (max wheel load: 8,200 Mg, tire inflation pressure: 2.0 bar). We used the same machinery setups and field traffic days as selected by Kuhwald et al. (2018).
Soil Moisture Modelling
Soil moisture is calculated from the present crop type, weather information and soil data for each day for each grid cell using the MONICA-model (Nendel et al., 2011). Among others, the model provides the soil moisture in 10 cm intervals for the depth between 0 and 200 cm (cf. Kuhwald et al., 2018).
Calculation of Soil Strength, Soil Stress and Soil Compaction Risk
The soil strength is calculated by the static soil properties (e.g., soil texture, gravel content) in combination with the modelled soil moisture by the MONICA-model using the pedotransfer functions of Horn and Fleige (2003), DIN V 19688 (2011), Rücknagel et al. (2012), Rücknagel et al. (2013) and Rücknagel et al. (2015). The result is a dynamic change of soil strength on a daily basis, depending on present crop type, weather situation and soil properties.
The soil strength is compared against the soil stress caused by the used machinery. The latter depends on the present crop type and time of year. The soil stress is calculated by the pedotransfer function from Koolen et al. (1992) and Rücknagel et al. (2015). Input data is the wheel load, the tire inflation pressure and the desired depth of risk analyses.
The comparison of soil strength and soil stress results in the soil compaction index (Rücknagel et al., 2015). The index is divided into five classes ranging from “no risk” (value <0) to “extremely high” (value >0.4) soil compaction risk. Though, the final result is a soil compaction risk class for each grid cell for each day within a year.
Spatio-Temporal Analysis and Hot-Spots Detection
The temporal analysis of soil compaction risk within a year was performed by stringing together the daily results for the investigated period.
For the analyses of recurring patterns, all grid cells of 1 year with the class “extremely high” soil compaction risk were summed up. The results were summed raster for each year showing the areas of the highest soil compaction risk class. By summarizing and counting these five grids, we can identify the hot-spots of extremely high soil compaction risk and areas, that were never affected by such a high soil compaction risk class.
Results
Spatial Characterization of the Study Area
The total size of the study area is 2,000 km2. The cover by arable land had a share of 50.2%, followed by forest (27.2%), grassland (16.0%), sealed area (5.4%) and water bodies (1.2%; Supplementary Table S2).
Organic soils cannot be processed by the SaSCiA-model since the incorporated functions (Horn and Fleige, 2003; Rücknagel et al., 2015) are invalid for soil with organic matter content >30%. Therefore, 1,562 of the originally 1,800 soil profiles could be used for soil compaction risk analysis, representing 116,309 ha under arable use.
At the desired depth of 40 cm, a wide range of soil texture classes occurred (Figure 4). Soil texture classes having high clay (Tt, Tl, Ts) and silt content (Uu, Ut2) were absent, as were those with low silt but high sand content (St2, St3, Ls4, Ts4, Ts3; according to the German classification scheme; Ad-Hoc-AG Boden, 2005).
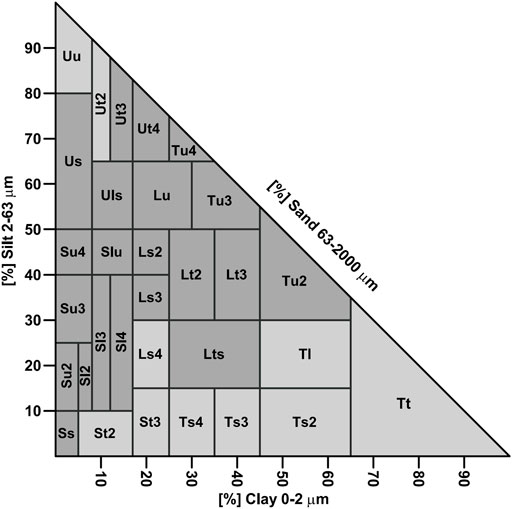
FIGURE 4. Soil texture triangle identifying the soil texture classes within the region (German soil classification; Ad-Hoc-AG Boden, 2005; Moeys, 2018). Dark grey shaded patches are present in the depth of 40 cm in the study area, while light grey patches are not.
Within the 116,309 ha area, cereals share ranged from 54 to 68% and was the predominating crop type in all years, while rapeseed always had the lowest share (4–12%; Table 2).

TABLE 2. Absolute and percentage area of the classified crop types for the single years between 2016 and 2020.
Spatial Distribution of Soil Compaction Risk
Figure 5 exemplarily shows the spatial distribution of soil compaction risk on 07 August 2017 for the depth of 40 cm. On this date, all soil compaction risk classes (“no risk” to “extremely high risk”) occurred in the study area, each with varying percentages of area (Table 3). In total, one quarter of the cropland area was not affected by field traffic, while another quarter revealed no soil compaction risk. The remaining area was distributed among the classes “low” to “extremely high” soil compaction risk, with the “extremely high” class having the highest share at 19.3%.
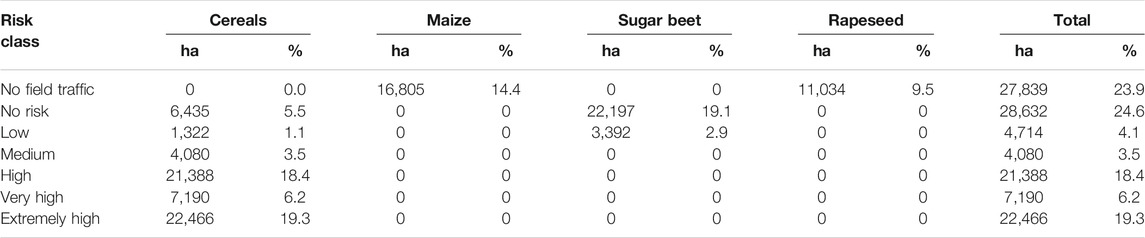
TABLE 3. Absolute and percentage area of soil compaction risk classes for the four crop types on 07 August 2017 at a depth of 40 cm.
No field traffic occurred for maize and rapeseed as no field traffic was assumed for these crop types on this date. The plant height of maize prevents any field traffic without damaging the plants; rapeseed has already been harvested and the sowing of a subsequent crop is too early in the year. For sugar beet, the class “no risk” dominated (19.1%), while 2.9% of the sugar beet area had a “low” soil compaction risk. Thus, the risk classes “medium” to “extremely high” referred to the crop type cereals on this date.
Temporal Variation of Soil Compaction Risk
Summarizing all daily soil compaction risk maps of a year and calculating the area shares of each soil compaction risk class, results in a temporal overview of soil compaction risk (Figures 6A–E). Focusing on all years, there were periods without soil compaction risk, e.g., December and January. Here, no field traffic was assumed, thus no soil compaction could occur. During the rest of the year, the percentage of arable land, which was potentially affected by soil compaction, varies from 10 to 100%. Autumn was the period with most field traffic activities. The risk class “no risk” predominates in all years. The highest risk class “extremely high” mainly occurred in spring. From February to mid of June, all soil compaction risk classes occurred in all years, which was not the case in summer and autumn.
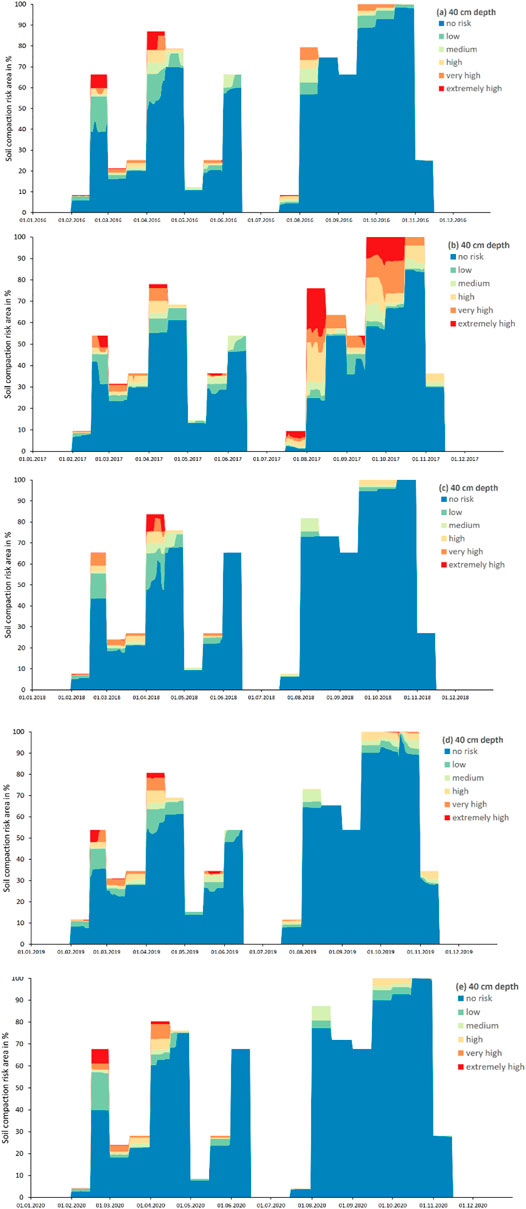
FIGURE 6. Temporal variation of soil compaction risk area share (% of total arable land) at 40 cm depth for (A) 2016, (B) 2017, (C) 2018, (D) 2019 and (E) 2020.
Comparing the years with each other, 2017 showed a distinctly different distribution of soil compaction risk. In particular from mid of July to November, the soil compaction risk was much higher than in all other years. For instance, the classes “high” to “extremely high” accounted for 43.6% of the arable land in mid of August. At the same time the year before and the years after, this percentage ranged between 0.0 and 10.0%; the “extremely high risk” class was absent in August in any other year than 2017.
Hot-Spots of Soil Compaction Risk Within the Region
Focusing on the class “extremely high” soil compaction risk enables the detection of areas that are mostly at risk for soil compaction within the region. In a first step, all daily soil compaction risk maps with the class “extremely high” risk were summarized for each year (Supplementary Figures S1–S5). This yielded in the amount of days, on which a certain area/raster cell falls into the highest compaction risk class within 1 year. In 2016 and 2018–2020, the highest soil compaction risk class is absent in more than 90% of the cropland area. In 2017, however, only 60.3% of the area were not at “extremely high” risk (Table 4). Additionally, the number of days with “extremely high” soil compaction risk is increased in 2017 compared to the four other years, e.g., 21,985 ha with 11–20 days of “extremely high” soil compaction risk Table 4.

TABLE 4. Absolute and percentage area of days with the class “extremely high” soil compaction risk summarized for each year (2016–2020).
In a second step, all soil compaction risk maps of the 5 years (= 1,827 days) with the class “extremely high” were summarized to identify the area of highest soil compaction risk (Figure 7). It showed that 39.8% of the cropland was exposed to “extremely high” soil compaction risk at least once during the entire study period (Table 5). Overall, 2.7% of the area revealed an “extremely high” soil compaction risk in each year. The areas, which were never or only once affected by “extremely high” soil compaction risk, were heterogeneously distributed across the study area. The north-western part of the study area showed a remarkable cluster with increased soil compaction risk.
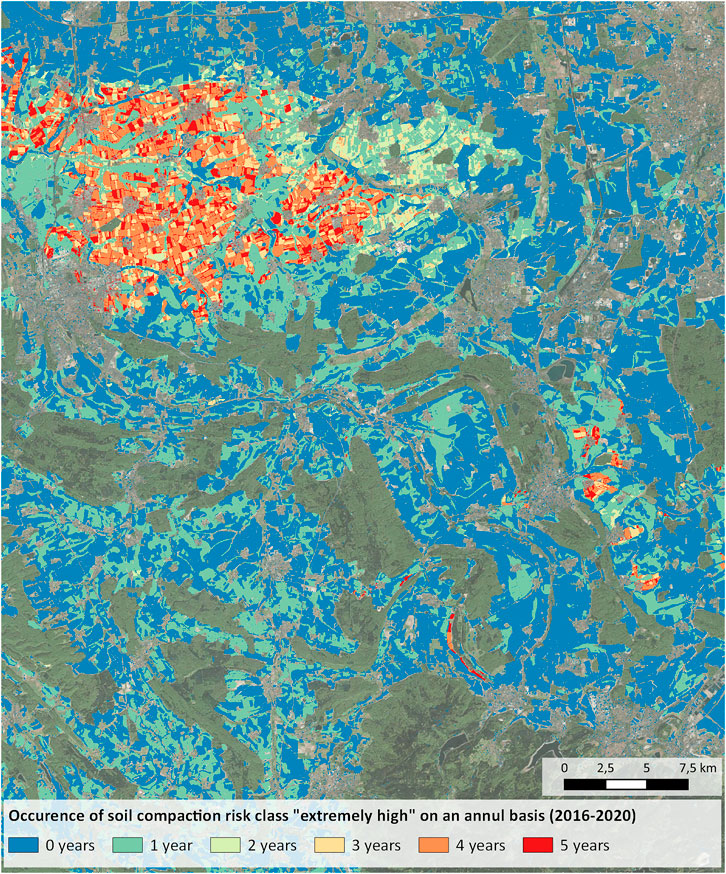
FIGURE 7. Spatial location of hot-spots (class “extremely high” soil compaction risk) of soil compaction risk on an annual basis for the period 2016–2020.

TABLE 5. Absolute and percentage area of hot-spots (containing only the class “extremely high” soil compaction risk) of soil compaction risk, grouped by number of years of their occurrence.
Analyzing the 2.7% area exposed to “extremely high” soil compaction risk in all 5 years in more detail revealed that a high percentage of this area (40.3–86%) corresponds to the crop type cereals (Table 6), followed by maize with a share ranging between 12.0 and 28.7%. An exception was 2017, where cereals (40.3%) and sugar beets (39.1%) reached similar area percentages.

TABLE 6. Absolute and percentage crop type area of the areas with every year soil compaction risk class “extremely high”.
Focusing on the soil characteristics of the 2.7% area showed that the soil texture class “Ut4” (German classification scheme; clay content: 17–25%, silt content: 65–83%) predominated this area with 3,055 ha. Further affected soil texture classes were Sl2, Lt2 and Lu. However, almost all soil texture classes (Ls2, Lt2, Lt3, Lts, Lu, Sl2, Ss, Su3, Su4, Tu2, Tu3, Tu4, Uls, Us, Ut3, Ut4) occurred at the areas exposed to “extremely high” soil compaction risk in at least 1 year.
Discussion
Spatio-Temporal Variation of Soil Compaction Risk
Our study showed the high spatio-temporal variation of soil compaction risk within a region. This applies to the annual changes in the same way as to the changes between the individual years.
These variations result from the interaction of actual soil strength and soil stress, which was calculated from the present crop type, soil data, weather data, machinery characteristics and field traffic days. The combination and interaction of these factors resulted in a heterogenous distribution of soil compaction risk across the region (exemplarily shown in Figure 5). Cereals harvest with wheel loads of 8.2 Mg (Supplementary Table S3) and relatively wet soil conditions in 2017 (discussion below) resulted in an increased share of high” to “extremely high” soil compaction risk at that date. The areas with sugar beets were less affected, as only spraying operation with wheel loads of 1.1/2.4 Mg were assumed, causing only “low” soil compaction risk (Table 3). The selected wheel loads represent typical ones for the study area. However, as wheel loads continuously increase in intensive agriculture (Keller et al., 2019; Kuhwald, 2019), higher wheel loads will frequently occur in the study area as well, resulting in higher soil stress and thus in higher soil compaction risk.
Weather is one highly variable and strongly influencing factor affecting variation of soil compaction risk, which exerts a large influence both within a year and between years. Within a year, spring is the period with the highest soil compaction risk in all years. The reason is a decreased evapotranspiration during winter, while precipitation is at a high level (Figure 2). Both factors increase soil moisture until spring. Generally, an increase in soil moisture leads to a decrease in soil strength (Rücknagel et al., 2012; Gut et al., 2015; Edwards et al., 2016). Thus, the soil strength is decreased to a minimum when field traffic activities occur in spring, usually in March and at the beginning of April. Consequently, it can be assumed that a high share of field traffic activities occurring at this time are associated with an increased soil compaction risk. Many field studies investigated the negative effects of field traffic in spring under wet soil conditions on soil functions (e.g., Schjønning et al., 2016; Pulido-Moncada et al., 2019; Ren et al., 2019).
During summer, evapotranspiration increases. The soils may become drier, which generally results in higher soil strength and lower soil compaction risk in general, as seen for the years 2016 and 2018–2020. In contrast, precipitation was higher in 2017. The mean annual precipitation was 750 mm this year, while it ranged between 454 and 641 mm in the other 4 years (DWD, 2021). Especially in July and August, the precipitation was significantly higher amounting to 278 mm in 2017 compared to 50–117 mm as registered for 2016 and 2018–2020 (Figure 2; Supplementary Table S4). The high amount of precipitation in summer increased the soil moisture even in the subsoil. Thus, the soil strength was reduced and the soil compaction risk noticeably increased in 2017 during cereal harvest. The effects of increased soil moisture were also evident in autumn for 2017, where the soil compaction risk remained at a high level during maize and sugar beet harvest. The harvest of maize and sugar beets are accompanied with high wheel loads, resulting in high soil stress and increased soil compaction risk (e.g., Peth et al., 2006; Barik et al., 2014; Duttmann et al., 2014; Destain et al., 2016; Götze et al., 2016). Thus, an increased susceptibility to soil compaction can generally be expected for maize and sugar beet.
Nevertheless, modelled soil compaction risk was relatively low during autumn in 2016 and 2018–2020. These years were extremely dry (e.g., Zscheischler and Fischer, 2020; Kowalski et al., 2022), especially in the summer months. The dryness increased soil strength and resulted in a reduced soil compaction risk even for the heavy machineries used for maize and sugar beet harvest. Thus, an increased soil compaction risk in autumn can be expected for “normal” years, during which the precipitation is around the long-term average.
Overall, analyses of the spatio-temporal dynamics revealed that 1 year with increased precipitation can considerably affect the soil compaction risk. This is particularly problematic as soil compaction, especially subsoil compaction, can be persistent for many years (Etana et al., 2013; Keller et al., 2017; Keller et al., 2021; Seehusen et al., 2021). Thus, 1 year of increased soil compaction risk accompanied by unsuited field traffic activities can be sufficient to affect soil functions and soil health in the long term.
Hot-Spots of Soil Compaction Risk
The hot-spot analysis revealed an area share of 2.7% that was exposed to the highest soil compaction risk class (“extremely high”) in each year of the 5-years period. From the perspective of sustainable soil use and soil protection, these areas are suggested to be the most endangered ones. In order to prevent harmful soil changes, they have to be managed carefully.
The soil texture class “Ut4” dominated the 2.7% area. Generally, silty clay soils are highly susceptible to soil compaction compared to other soil texture classes (Lorenz et al., 2016). In the study area, 260 soil profiles are characterized by the soil texture class “Ut4” at the investigated depth, representing an area of 39,574 ha. Out of these, only 54 soil profiles were part of the 2.7% hot-spot area. Thus, soil texture of “Ut4” is not necessarily associated with the highest soil compaction risk.
Focusing on the area that is affected at least in 1 year by the highest soil compaction risk class revealed an area share of 39.8%. The majority of these area is affected in 1 year (Table 5). As described above, soil compaction, especially subsoil compaction, is persistent and hardly to recover. Thus, even a one-time increased soil compaction risk can result in soil compaction that persists for many years if field traffic is conducted with machinery unsuitable for the current situation. In practice, this will not be the case for the entire 39.8% area; however, the analysis showed the high area share that is potentially affected.
As described above, the 5-years period was characterized by mainly dry years. The mean annual precipitation between 2016 and 2020 was 582 mm, while it was 680 mm between the years 2005 and 2015 (Supplementary Table S4). Lower precipitation and soil desiccation during the investigated period compared to previous years resulted in a potentially underestimated hot-spot area. Thus, we assume that the area percentage of the highest risk class would have been larger when the weather and soil moisture conditions of a longer period would have been considered. However, it remains to be seen whether drier conditions will be the new “normal” in the study area.
The potential area exposed to high soil compaction risk will further increase when changing the definition of “hot-spot”. The presented hot-spot analysis was conducted for the “extremely high” soil compaction risk class. When considering the two highest classes (“very high” and “extremely high”), the hot-spot area increased considerably (Table 7). The area share affected at least one time every year by “very high” and/or “extremely high” soil compaction risk was at 11.1%. Only 40% of the study area were never affected by “very high” and/or “extremely high” soil compaction risk.

TABLE 7. Absolute and percentage area of hot-spots (containing the classes “very high” and “extremely high” soil compaction risk) of soil compaction risk, grouped by number of years of their occurrence.
The definition of the term “hot-spot” is therefore very important to provide information on the spatial extent of critical soil compaction risk. This also raises the question of how to assess the respective risk classes. The used approach classifies the soil compaction risk in five classes (Rücknagel et al., 2015; Götze et al., 2016). Terranimo, for instance, uses only three classes (Stettler et al., 2014), while Jones et al. (2003) uses four classes. It remains unclear, however, how these classes are to be evaluated. Is a “medium” soil compaction risk tolerable, or will it result in severe soil compaction? Thus, an evaluation of the calculated soil compaction risk is necessary, as exemplarily shown by Götze et al. (2016), in order to derive the critical risk classes.
Advances and Limitations of the Soil Compaction Risk Analysis
Generally, it must be kept in mind that the used SaSCiA-model calculates the risk of soil compaction. Thus, the model does not predict the real occurrence and distribution of soil compaction, but indicates where soil compaction is likely to occur. Furthermore, the SaSCiA-model has some limitations, which are discussed in detail by Kuhwald et al. (2018). An important limitation is that the wheel pass frequency is not considered in the SaSCiA-model, although the amount of wheel passes has an impact on soil compaction (e.g., Botta et al., 2009; Pulido-Moncada et al., 2019). Another limitation is associated with the raster approach, which leads to the fact that the entire raster cell is always affected by field traffic. This results in an overestimation of the risk area, since almost never all parts of a field are wheeled during one field traffic activity (except for sugar beet harvest with a self-propelled harvester; Augustin et al., 2020).
However, two limitations of the original SaSCiA-version were addressed in the present study. The first one was the use of all available weather stations (a total of 55 stations) within the federal state to regionalize the weather information; in the original approach, only one weather station was used (cf. Kuhwald et al., 2018). Thus, spatial variations, e.g., in precipitation, could be accounted for in the 5-years analysis. The second one was the use of a soil map at a scale of 1:50.000 instead of a soil map of 1:200.000 (Kuhwald et al., 2018), which increased the accuracy of the spatial representation of soil characteristics. However, even a soil map of at a scale of 1:50.000 shows a relatively coarse spatial resolution compared to a Sentinel 2-pixel size of 20 m. Therefore, a higher spatial resolution soil map is needed to increase the reliability of the soil compaction risk assessment, but is not yet available for the region.
Independent on model limitations, the model approach and model results may be useful to support sustainable soil use and soil protection.
Conclusion
This study modelled the spatio-temporal soil compaction risk on a daily basis for 5 years for a region. Thus, for the first time, it was feasible to analyze and show how the dynamic characteristic of soil compaction risk vary in space and time over such a long period of time with high spatial (20 m) and temporal (daily) resolution.
One main result of this analysis is that 1 year of increased precipitation can contribute to a tremendous increase in soil compaction risk, which was the case in this study in 2017. Another important finding was that 2.7% of the area exhibited the highest soil compaction risk class (“extremely high”) in each year of the study period. Looking at the areas that were affected by “extremely high” soil compaction risk at least once, this percentage increased to 39.8%.
As discussed above, soil compaction is persistence and many years are required to reach the pre-soil compaction state, if it is achievable at all. Assuming a share of nearly 40% of compacted soil in the study area would therefore have strong environmental effects and will reduce the yield substantially. However, the modelled results show the risk of soil compaction, not the actual state. But by identifying the risk, the extent of possible soil degradation becomes apparent.
Thus, this study may contribute to increased awareness of soil compaction risk dynamics in order to mitigate further soil degradation. In this sense, a next step must be the integration of weather forecast to enable the prediction of soil compaction risk for the following days. This prediction will enable to decide on which day a field might by trafficked by identifying the day with the lowest soil compaction risk.
Data Availability Statement
The data analyzed in this study is subject to the following licenses/restrictions: - The soil map used (BK 50) must be paied for use. - The weather data used is free available (https://opendata.dwd.de/climate_environment/CDC/)—The satellite data used is free available (Sentinel 2; https://scihub.copernicus.eu/). Requests to access these datasets should be directed to https://opendata.dwd.de/climate_environment/CDC/; https://scihub.copernicus.eu/.
Author Contributions
MK designed the study, conducted the modelling, analyzed the data and wrote the majority of the text. KK prepared the satellite data, conducted the crop type classification and contributed to the text. RD contributed in reviewing and finalizing the manuscript.
Funding
The Federal Ministry of Education and Research (BMBF) supported this study within the framework of the BonaRes-initiative (Grant No.: 031B1065C). We acknowledge financial support for publications cost by Land Schleswig-Holstein within the funding programme Open Access Publikationsfonds.
Conflict of Interest
The authors declare that the research was conducted in the absence of any commercial or financial relationships that could be construed as a potential conflict of interest.
Publisher’s Note
All claims expressed in this article are solely those of the authors and do not necessarily represent those of their affiliated organizations, or those of the publisher, the editors, and the reviewers. Any product that may be evaluated in this article, or claim that may be made by its manufacturer, is not guaranteed or endorsed by the publisher.
Acknowledgments
We are grateful to the Rebecca Kessler, Franziska Wagner, Frida Schneeberg and Celina Thomas, who conducted the crop type mapping in the field. We thank the reviewers who improved the manuscript with helpful comments.
Supplementary Material
The Supplementary Material for this article can be found online at: https://www.frontiersin.org/articles/10.3389/fenvs.2022.823030/full#supplementary-material
References
Alaoui, A., Rogger, M., Peth, S., and Blöschl, G. (2018). Does Soil Compaction Increase Floods? A Review. J. Hydrol. 557, 631–642. doi:10.1016/j.jhydrol.2017.12.052
Arvidsson, J., and Håkansson, I. (2014). Response of Different Crops to Soil Compaction-Short-Term Effects in Swedish Field Experiments. Soil Tillage Res. 138, 56–63. doi:10.1016/j.still.2013.12.006
Arvidsson, J., and Keller, T. (2007). Soil Stress as Affected by Wheel Load and Tyre Inflation Pressure. Soil Tillage Res. 96, 284–291. doi:10.1016/j.still.2007.06.012
Augustin, K., Kuhwald, M., Brunotte, J., and Duttmann, R. (2019). FiTraM: A Model for Automated Spatial Analyses of Wheel Load, Soil Stress and Wheel Pass Frequency at Field Scale. Biosyst. Eng. 180, 108–120. doi:10.1016/j.biosystemseng.2019.01.019
Augustin, K., Kuhwald, M., Brunotte, J., and Duttmann, R. (2020). Wheel Load and Wheel Pass Frequency as Indicators for Soil Compaction Risk: A Four-Year Analysis of Traffic Intensity at Field Scale. Geosciences 10, 292. doi:10.3390/geosciences10080292
Barik, K., Aksakal, E. L., Islam, K. R., Sari, S., and Angin, I. (2014). Spatial Variability in Soil Compaction Properties Associated with Field Traffic Operations. CATENA 120, 122–133. doi:10.1016/j.catena.2014.04.013
Belgiu, M., and Drăguţ, L. (2016). Random forest in Remote Sensing: A Review of Applications and Future Directions. ISPRS J. Photogrammetry Remote Sensing 114, 24–31. doi:10.1016/j.isprsjprs.2016.01.011
Berisso, F. E., Schjønning, P., Lamandé, M., Weisskopf, P., Stettler, M., and Keller, T. (2013). Effects of the Stress Field Induced by a Running Tyre on the Soil Pore System. Soil Tillage Res. 131, 36–46. doi:10.1016/j.still.2013.03.005
Boden, Ad-Hoc-A. G. (2005). Bodenkundliche Kartieranleitung. 5. Stuttgart: Aufl.Hannover. Schweizbart.
Botta, G. F., Becerra, A. T., and TournBellora, F. B. (2009). Effect of the Number of Tractor Passes on Soil Rut Depth and Compaction in Two Tillage Regimes. Soil Tillage Res. 103, 381–386. doi:10.1016/j.still.2008.12.002
D'Or, D., and Destain, M.-F. (2014). Toward a Tool Aimed to Quantify Soil Compaction Risks at a Regional Scale: Application to Wallonia (Belgium). Soil Tillage Res. 144, 53–71. doi:10.1016/j.still.2014.06.008
Daigh, A. L. M., DeJong‐Hughes, J., and Acharya, U. (2020). Projections of Yield Losses and Economic Costs Following Deep Wheel‐traffic Compaction during the 2019 Harvest. Agric. Environ. Lett. 5, e20013. doi:10.1002/ael2.20013
Destain, M.-F., Roisin, C., Dalcq, A.-S., and Mercatoris, B. C. N. (2016). Effect of Wheel Traffic on the Physical Properties of a Luvisol. Geoderma 262, 276–284. doi:10.1016/j.geoderma.2015.08.028
DIN V 19688 (2011). Soil Quality - Determination of Compactibilty Risk of mineral Sub-soils Based on the Assessed Preconsolidation Stress.
Diserens, E. (2009). Calculating the Contact Area of Trailer Tyres in the Field. Soil Tillage Res. 103, 302–309. doi:10.1016/j.still.2008.10.020
Diserens, E. (2002). Ermittlung der Reifen-Kontaktfläche im Feld mittels Rechenmodell: Eine wichtige Voraussetzung, um die Bodenbeanspruchung im Ackerbau zu beurteilen. FAT Berichte 1–11.
Duttmann, R., Augustin, K., Brunotte, J., and Kuhwald, M. (2021). Modeling of Field Traffic Intensity and Soil Compaction Risks in Agricultural Landscapes. (accepted).
Duttmann, R., Brunotte, J., and Bach, M. (2013). Spatial Analyses of Field Traffic Intensity and Modeling of Changes in Wheel Load and Ground Contact Pressure in Individual fields during a Silage maize Harvest. Soil Tillage Res. 126, 100–111. doi:10.1016/j.still.2012.09.001
Duttmann, R., Schwanebeck, M., Nolde, M., and Horn, R. (2014). Predicting Soil Compaction Risks Related to Field Traffic during Silage maize Harvest. Soil Sci. Soc. America J. 78, 408–421. doi:10.2136/sssaj2013.05.0198
DVWK 234 (1995). Gefügestabilität Ackerbaulich Genutzter Mineralböden - Teil 1: Mechanische Belastbarkeit.
Edwards, G., White, D. R., Munkholm, L. J., Sørensen, C. G., and Lamandé, M. (2016). Modelling the Readiness of Soil for Different Methods of Tillage. Soil Tillage Res. 155, 339–350. doi:10.1016/j.still.2015.08.013
Etana, A., Larsbo, M., Keller, T., Arvidsson, J., Schjønning, P., Forkman, J., et al. (2013). Persistent Subsoil Compaction and its Effects on Preferential Flow Patterns in a Loamy till Soil. Geoderma 192, 430–436. doi:10.1016/j.geoderma.2012.08.015
FAO (2014). World Reference Base for Soil Resources. International Soil Classification System for Naming Soils and Creating Legends for Soil Maps. Rome: World Soil Resources Report 106.
Foody, G. M. (2002). Status of Land Cover Classification Accuracy Assessment. Remote Sensing Environ. 80, 185–201. doi:10.1016/S0034-4257(01)00295-4
Gebhardt, S., Fleige, H., and Horn, R. (2009). Effect of Compaction on Pore Functions of Soils in a Saalean Moraine Landscape in North Germany. Z. Pflanzenernähr. Bodenk. 172, 688–695. doi:10.1002/jpln.200800073
Götze, P., Rücknagel, J., Jacobs, A., Märländer, B., Koch, H.-J., and Christen, O. (2016). Environmental Impacts of Different Crop Rotations in Terms of Soil Compaction. J. Environ. Manage. 181, 54–63. doi:10.1016/j.jenvman.2016.05.048
Graves, A. R., Morris, J., Deeks, L. K., Rickson, R. J., Kibblewhite, M. G., Harris, J. A., et al. (2015). The Total Costs of Soil Degradation in England and Wales. Ecol. Econ. 119, 399–413. doi:10.1016/j.ecolecon.2015.07.026
Gut, S., Chervet, A., Stettler, M., Weisskopf, P., Sturny, W. G., Lamandé, M., et al. (2015). Seasonal Dynamics in Wheel Load-Carrying Capacity of a Loam Soil in the Swiss Plateau. Soil Use Manage 31, 132–141. doi:10.1111/sum.12148
Hartmann, P., Zink, A., Fleige, H., and Horn, R. (2012). Effect of Compaction, Tillage and Climate Change on Soil Water Balance of Arable Luvisols in Northwest Germany. Soil Tillage Res. 124, 211–218. doi:10.1016/j.still.2012.06.004
Horn, R., Domżżał, H., Słowińska-Jurkiewicz, A., and van Ouwerkerk, C. (1995). Soil Compaction Processes and Their Effects on the Structure of Arable Soils and the Environment. Soil Tillage Res. 35, 23–36. doi:10.1016/0167-1987(95)00479-C
Horn, R., and Fleige, H. (2003). A Method for Assessing the Impact of Load on Mechanical Stability and on Physical Properties of Soils. Soil Tillage Res. 73, 89–99. doi:10.1016/S0167-1987(03)00102-8
Horn, R., Fleige, H., Richter, F.-H., Czyz, E. A., Dexter, A., Diaz-Pereira, E., et al. (2005). SIDASS Project. Soil Tillage Res. 82, 47–56. doi:10.1016/j.still.2005.01.007
Horn, R., and Fleige, H. (2009). Risk Assessment of Subsoil Compaction for Arable Soils in Northwest Germany at Farm Scale. Soil Tillage Res. 102, 201–208. doi:10.1016/j.still.2008.07.015
Horn, R., Simota, C., Fleige, H., Dexter, A., Rajkai, K., and de la Rosa, D. (2002). Prognose der mechanischen Belastbarkeit und der auflastabhängigen Änderung des Lufthaushaltes in Ackerböden anhand von Bodenkarten. Z. für Pflanzenernährung Bodenkunde 165, 235–239. doi:10.1002/1522-2624(200204)165:2<235::aid-jpln235>3.0.co;2-h
Horn, R. (2003). Stress-strain Effects in Structured Unsaturated Soils on Coupled Mechanical and Hydraulic Processes. Geoderma 116, 77–88. doi:10.1016/S0016-7061(03)00095-8
Horn, R., Way, T., and Rostek, J. (2003). Effect of Repeated Tractor wheeling on Stress/strain Properties and Consequences on Physical Properties in Structured Arable Soils. Soil Tillage Res. 73, 101–106. doi:10.1016/S0167-1987(03)00103-X
Immitzer, M., Vuolo, F., and Atzberger, C. (2016). First Experience with Sentinel-2 Data for Crop and Tree Species Classifications in Central Europe. Remote Sensing 8, 166. doi:10.3390/rs8030166
Jones, R. J. A., Spoor, G., and Thomasson, A. J. (2003). Vulnerability of Subsoils in Europe to Compaction: a Preliminary Analysis. Soil Tillage Res. 73, 131–143. doi:10.1016/S0167-1987(03)00106-5
Kandziora, M., Dörnhöfer, K., Oppelt, N., and Müller, F. (2014). Detecting Land Use and Land Cover Changes in Northern German Agricultural Landscapes to Assess Ecosystem Service Dynamics. Landscape Online 1–24. doi:10.3097/lo.201435
Keller, T. (2005). A Model for the Prediction of the Contact Area and the Distribution of Vertical Stress below Agricultural Tyres from Readily Available Tyre Parameters. Biosyst. Eng. 92, 85–96. doi:10.1016/j.biosystemseng.2005.05.012
Keller, T., Arvidsson, J., Schjønning, P., Lamandé, M., Stettler, M., and Weisskopf, P. (2012). In Situ Subsoil Stress-Strain Behavior in Relation to Soil Precompression Stress. Soil Sci. 177, 490–497. doi:10.1097/SS.0b013e318262554e
Keller, T., Colombi, T., Ruiz, S., Manalili, M. P., Rek, J., Stadelmann, V., et al. (2017). Long-Term Soil Structure Observatory for Monitoring Post-Compaction Evolution of Soil Structure. Vadose Zone J. 16. doi:10.2136/vzj2016.11.0118
Keller, T., Colombi, T., Ruiz, S., Schymanski, S. J., Weisskopf, P., Koestel, J., et al. (2021). Soil Structure Recovery Following Compaction: Short‐term Evolution of Soil Physical Properties in a Loamy Soil. Soil Sci. Soc. Am. J. 85, 1002–1020. doi:10.1002/saj2.20240
Keller, T., Défossez, P., Weisskopf, P., Arvidsson, J., and Richard, G. (2007). SoilFlex: A Model for Prediction of Soil Stresses and Soil Compaction Due to Agricultural Field Traffic Including a Synthesis of Analytical Approaches. Soil Tillage Res. 93, 391–411. doi:10.1016/j.still.2006.05.012
Keller, T., Sandin, M., Colombi, T., Horn, R., and Or, D. (2019). Historical Increase in Agricultural Machinery Weights Enhanced Soil Stress Levels and Adversely Affected Soil Functioning. Soil Tillage Res. 194, 104293–104312. doi:10.1016/j.still.2019.104293
Koolen, A. J., Lerink, P., Kurstjens, D. A. G., van den Akker, J. J. H., and Arts, W. B. M. (1992). Prediction of Aspects of Soil-Wheel Systems. Soil Tillage Res. 24, 381–396. doi:10.1016/0167-1987(92)90120-Z
Kowalski, K., Okujeni, A., Brell, M., and Hostert, P. (2022). Quantifying Drought Effects in Central European Grasslands through Regression-Based Unmixing of Intra-annual Sentinel-2 Time Series. Remote Sensing Environ. 268, 112781. doi:10.1016/j.rse.2021.112781
Kroulík, M., Kumhála, F., Hůla, J., and Honzík, I. (2009). The Evaluation of Agricultural Machines Field Trafficking Intensity for Different Soil Tillage Technologies. Soil Tillage Res. 105, 171–175. doi:10.1016/j.still.2009.07.004
Kuhwald, M. (2019). Detection and Modelling of Soil Compaction of Arable Soils: From Field Survey to Regional Risk Assessment, 1–108.
Kuhwald, M., Dörnhöfer, K., Oppelt, N., and Duttmann, R. (2018). Spatially Explicit Soil Compaction Risk Assessment of Arable Soils at Regional Scale: The SaSCiA-Model. Sustainability 10, 1618–1629. doi:10.3390/su10051618
Lamandé, M., Greve, M. H., and Schjønning, P. (2018). Risk Assessment of Soil Compaction in Europe - Rubber Tracks or Wheels on Machinery. CATENA 167, 353–362. doi:10.1016/j.catena.2018.05.015
Lamandé, M., and Schjønning, P. (2011). Transmission of Vertical Stress in a Real Soil Profile. Part I: Site Description, Evaluation of the Söhne Model, and the Effect of Topsoil Tillage. Soil Tillage Res. 114, 57–70. doi:10.1016/j.still.2011.05.004
LBEG (2020). Bodenkarte 1:50.000 (BK 50). Landesamt für Bergbau, Energie und Geologie (LBEG). Hannover, Germany.
Lebert, M. (2010). Entwicklung eines Prüfkonzeptes zur Erfassung der tatsächlichen Verdichtungsgefährdung landwirtschaftlich genutzter Böden. UBA-Texte, 1–96.
Lebert, M., and Horn, R. (1991). A Method to Predict the Mechanical Strength of Agricultural Soils. Soil Tillage Res. 19, 275–286. doi:10.1016/0167-1987(91)90095-F
Ledermüller, S., Lorenz, M., Brunotte, J., and Fröba, N. (2018). A Multi-Data Approach for Spatial Risk Assessment of Topsoil Compaction on Arable Sites. Sustainability 10, 2915–2922. doi:10.3390/su10082915
Lorenz, M., Brunotte, J., Vorderbrügge, T., Brandhuber, R., Koch, H.-J., Senger, M., et al. (2016). Adaptation of Load Input by Agricultural Machines to the Susceptibility of Soil to Compaction - Principles of Soil Conserving Traffic on Arable Land. Appl. Agric. For. Res., 101–144. doi:10.3220/LBF1473334823000
Nendel, C., Berg, M., Kersebaum, K. C., Mirschel, W., Specka, X., Wegehenkel, M., et al. (2011). The MONICA Model: Testing Predictability for Crop Growth, Soil Moisture and Nitrogen Dynamics. Ecol. Model. 222, 1614–1625. doi:10.1016/j.ecolmodel.2011.02.018
Peth, S., Horn, R., Fazekas, O., and Richards, B. G. (2006). Heavy Soil Loading its Consequence for Soil Structure, Strength, Deformation of Arable Soils. Z. Pflanzenernähr. Bodenk. 169, 775–783. doi:10.1002/jpln.200620112
Pulido-Moncada, M., Munkholm, L. J., and Schjønning, P. (2019). Wheel Load, Repeated wheeling, and Traction Effects on Subsoil Compaction in Northern Europe. Soil Tillage Res. 186, 300–309. doi:10.1016/j.still.2018.11.005
R Core Team (2020). R: A Language and Environment for Statistical Computing. Vienna, Austria: R foundation for Statistical Computing.
Rathjens, H., Dörnhöfer, K., and Oppelt, N. (2014). IRSeL-An Approach to Enhance Continuity and Accuracy of Remotely Sensed Land Cover Data. Int. J. Appl. Earth Observation Geoinformation 31, 1–12. doi:10.1016/j.jag.2014.02.010
Ren, L., D’Hose, T., Ruysschaert, G., De Pue, J., Meftah, R., Cnudde, V., et al. (2019). Effects of Soil Wetness and Tyre Pressure on Soil Physical Quality and maize Growth by a Slurry Spreader System. Soil Tillage Res. 195, 104344. doi:10.1016/j.still.2019.104344
Rücknagel, J., Christen, O., Hofmann, B., and Ulrich, S. (2012). A Simple Model to Estimate Change in Precompression Stress as a Function of Water Content on the Basis of Precompression Stress at Field Capacity. Geoderma 178, 1–7. doi:10.1016/j.geoderma.2012.01.035
Rücknagel, J., Götze, P., Hofmann, B., Christen, O., and Marschall, K. (2013). The Influence of Soil Gravel Content on Compaction Behaviour and Pre-compression Stress. Geoderma 209, 226–232. doi:10.1016/j.geoderma.2013.05.030
Rücknagel, J., Hofmann, B., Deumelandt, P., Reinicke, F., Bauhardt, J., Hülsbergen, K.-J., et al. (2015). Indicator Based Assessment of the Soil Compaction Risk at Arable Sites Using the Model REPRO. Ecol. Indicators 52, 341–352. doi:10.1016/j.ecolind.2014.12.022
Schjønning, P., Lamandé, M., Keller, T., Pedersen, J., and Stettler, M. (2012). Rules of Thumb for Minimizing Subsoil Compaction. Soil Use Manag. 28, 378–393. doi:10.1111/j.1475-2743.2012.00411.x
Schjønning, P., and Lamandé, M. (2018). Models for Prediction of Soil Precompression Stress from Readily Available Soil Properties. Geoderma 320, 115–125. doi:10.1016/j.geoderma.2018.01.028
Schjønning, P., Lamandé, M., Munkholm, L. J., Lyngvig, H. S., and Nielsen, J. A. (2016). Soil Precompression Stress, Penetration Resistance and Crop Yields in Relation to Differently-Trafficked, Temperate-Region sandy Loam Soils. Soil Tillage Res. 163, 298–308. doi:10.1016/j.still.2016.07.003
Schjønning, P., Lamandé, M., Tøgersen, F. A., Arvidsson, J., and Keller, T. (2008). Modelling Effects of Tyre Inflation Pressure on the Stress Distribution Near the Soil-Tyre Interface. Biosyst. Eng. 99, 119–133. doi:10.1016/j.biosystemseng.2007.08.005
Schjønning, P., Stettler, M., Keller, T., Lassen, P., and Lamandé, M. (2015a). Predicted Tyre-Soil Interface Area and Vertical Stress Distribution Based on Loading Characteristics. Soil Tillage Res. 152, 52–66. doi:10.1016/j.still.2015.03.002
Schjønning, P., van den Akker, J. J. H., Keller, T., Greve, M. H., Lamandé, M., Simojoki, A., et al. (2015b). “Driver-Pressure-State-Impact-Response (DPSIR) Analysis and Risk Assessment for Soil Compaction-A European Perspective,” in Advances in Agronomy. Editor D. L. Sparks, 183–237. doi:10.1016/bs.agron.2015.06.001
Seehusen, T., Mordhorst, A., Riggert, R., Fleige, H., Horn, R., and Riley, H. (2021). Subsoil Compaction of a clay Soil in South-East Norway and its Amelioration after 5 Years. Int. Agrophys. 35, 145–157. doi:10.31545/intagr/135513
Seehusen, T., Riggert, R., Fleige, H., Horn, R., and Riley, H. (2019). Soil Compaction and Stress Propagation after Different wheeling Intensities on a silt Soil in South-East Norway. Acta Agriculturae Scand. Section B - Soil Plant Sci. 69, 343–355. doi:10.1080/09064710.2019.1576762
Stettler, M., Keller, T., Weisskopf, P., Lamandè, M., Lassen, P., and Schjønning, P. (2014). Terranimo- a Web-Based Tool for Evaluating Soil Compaction. Landtechnik 69 (3), 132–138.
Szatanik-Kloc, A., Horn, R., Lipiec, J., Siczek, A., and Szerement, J. (2018). Soil Compaction-Induced Changes of Physicochemical Properties of Cereal Roots. Soil Tillage Res. 175, 226–233. doi:10.1016/j.still.2017.08.016
Techen, A.-K., Helming, K., Brüggemann, N., Veldkamp, E., Reinhold-Hurek, B., Lorenz, M., et al. (2020). “Soil Research Challenges in Response to Emerging Agricultural Soil Management Practices,” in Advances in Agronomy. Editor D. L. Sparks (Amsterdam: Academic Press), 179–240. doi:10.1016/bs.agron.2020.01.002
van den Akker, J. J. H., and Hoogland, T. (2011). Comparison of Risk Assessment Methods to Determine the Subsoil Compaction Risk of Agricultural Soils in The Netherlands. Soil Tillage Res. 114, 146–154. doi:10.1016/j.still.2011.04.002
van den Akker, J. J. H. (2004). SOCOMO: a Soil Compaction Model to Calculate Soil Stresses and the Subsoil Carrying Capacity. Soil Tillage Res. 79, 113–127. doi:10.1016/j.still.2004.03.021
Weisskopf, P., Reiser, R., Rek, J., and Oberholzer, H.-R. (2010). Effect of Different Compaction Impacts and Varying Subsequent Management Practices on Soil Structure, Air Regime and Microbiological Parameters. Soil Tillage Res. 111, 65–74. doi:10.1016/j.still.2010.08.007
Wessolek, G., Kaupenjohann, M., and Renger, M. (2009). Bodenökologie und Bodengenese.Bodenphysikalische Kennwerte und Berechnungsverfahren für die Praxis
Keywords: soil degradation, sustainable management, modelling, field traffic, SaSCiA-model
Citation: Kuhwald M, Kuhwald K and Duttmann R (2022) Spatio-Temporal High-Resolution Subsoil Compaction Risk Assessment for a 5-Years Crop Rotation at Regional Scale. Front. Environ. Sci. 10:823030. doi: 10.3389/fenvs.2022.823030
Received: 26 November 2021; Accepted: 22 February 2022;
Published: 09 March 2022.
Edited by:
Thomas Keller, Swedish University of Agricultural Sciences, SwedenReviewed by:
Cássio Tormena, Universidade Estadual de Maringá, BrazilMiriam Muñoz-Rojas, University of New South Wales, Australia
Copyright © 2022 Kuhwald, Kuhwald and Duttmann. This is an open-access article distributed under the terms of the Creative Commons Attribution License (CC BY). The use, distribution or reproduction in other forums is permitted, provided the original author(s) and the copyright owner(s) are credited and that the original publication in this journal is cited, in accordance with accepted academic practice. No use, distribution or reproduction is permitted which does not comply with these terms.
*Correspondence: Michael Kuhwald, a3Vod2FsZEBnZW9ncmFwaGllLnVuaS1raWVsLmRl