- 1School of Earth and Environmental Sciences, The University of Queensland, St Lucia, QLD, Australia
- 2The Northwest Institute of Eco-Environment and Resources, Chinese Academy of Sciences, Lanzhou, China
- 3University Library, Southwest University, Chongqing, China
- 4State Key Laboratory of Earth Surface Processes and Resource Ecology, Faculty of Geographical Science, Beijing Normal University, Beijing, China
- 5Global Institute for Water Security, The University of Saskatchewan, Saskatoon, SK, Canada
- 6Department of Civil and Environmental Engineering and Department of Geography and Geographic Information Science, University of Illinois at Urbana-Champaign, Urbana, IL, United States
Understanding the historical evolution of science development for rethinking science in the Anthropocene is crucial for our future survival. This paper analyzed the knowledge development of the top 95 most researched river basins in the Web of Science database in the past 3 decades (1987–2017) using a network metric-based framework, comprising one scalar metric and three structural metrics: equality, efficiency, and resilience. We found that the highly researched river basins accounting about 30% of total publications, including the Yangtze River and the Great Lakes, demonstrated the “ageing” knowledge structures characterized by high inequality, low efficiency, and large redundancy with continuous expansion in scales. Dominations of knowledge interactions among Environmental Sciences, Water Resources, Marine Science and Freshwater Biology contributed to this knowledge structure. Transformations of both the composition and structure of the knowledge system is required to support global river basin management in the Anthropocene.
Introduction
The Anthropocene, in which humans have massively changed various Earth system cycles, has been engined by accumulated science development over generations (Steffen et al., 2011). Understanding the historical evolution of science development for rethinking science in the Anthropocene is crucial for our future survival. River basins are basic components of the Earth system which link major cycles (e.g., water, nutrients, energy, and carbon) of the Earth system as well as the human society. Most of them are degraded and are expected to face further environmental deterioration (Vörösmarty et al., 2010). In the past decades, increasing efforts have been devoted to integrating knowledge from engineering, geomorphology, chemistry, ecology, hydrology, economics, sociology, and law in river basin management (Biggs et al., 2015; Pahl-Wostl, 2015). Yet, the status of global river basins implies a failure of science development.
On one hand, to tackle sustainability problems in the Anthropocene requires scientific knowledge to address the diverse interactions and feedback effects between various human and natural components in the Earth system (Steffen et al., 2015; Liu et al., 2019). On the other hand, science development has been increasingly recognised as a complex and dynamic network within which different disciplines “knit, weave and knot together into an overarching scientific fabric” (Latour, 1987; Shi et al., 2015). Blind spots in the science knowledge network not only occur when there is lack of certain “nodes” (i.e., disciplines), but also when there are missing “links” between different disciplines (Turnbull et al., 2018). Lack of either “nodes” or “links” can result in deficiency of the knowledge system.
Science development is often analysed in two primary ways (Cheng et al., 2020). First, disciplinary experts qualitatively examine state-of-the-art disciplinary advances and provide recommendations for future developments (e.g., (Blöschl et al., 2019; Di Baldassarre et al., 2019). However, this method tracks knowledge development on key research topics, thus has limited capacity in reflecting the structure and development processes of the whole knowledge system (Kallio and Houtbeckers, 2020). Second, science development is structurally analysed using bibliometric methods to reveal interactive links between different research topics (e.g.(Khasseh et al., 2017; Su et al., 2020). This method often focuses on the big data trends and numerical elements of scientific publications such as co-authors, institutions, and literature citations that are hardly recognized by disciplinary scientists. There is no systemic framework that bridges expert knowledge and bibliometric analysis to assess the blind spots in the knowledge system, compromising our capacity in strategic intervention for knowledge development.
This paper aims to develop a network metric-based framework to reveal the endogenous processes of knowledge development for structurally directing future knowledge development. We examine the knowledge development of the top 95 most researched river basins with scientific publications in the Web of Science (WoS) database during 1987–2017. Specifically, we will: 1) describe the temporal and spatial change of the scales and structures of the knowledge systems in these river basins; 2) analyze the relationships among the structural metrics and between the structural metrics and scalar metrics of the knowledge systems in these river basins; 3) identify the deficiencies of these knowledge systems if any and potential strategies for future development. Key findings from this study are expected to cast insights into the evolution of knowledge development in the Earth system.
Methods
Defining the Scale and Structure of a Knowledge System
We proposed an original framework to measure and evaluate the knowledge structures in river basin studies based on the system theory, complex network characteristics (Fortunato et al., 2018) and our previous findings on this field (Wu et al., 2021; Wei and Wu, 2022). In this framework, we considered the river basin knowledge development as a complex system, within which different disciplines interact and feedback on each other in a coevolutionary manner.
As the structure of a complex system may be dependent on its scale (Von Bertalanffy, 1968; Cooper et al., 2020), we firstly defined the scalar metric which is the number of disciplines involved in the knowledge system (Table 1). It indicates how large a system is on a quantitative basis. We then defined three structural metrics of the knowledge system: equality, resilience, and efficiency. They have been widely recognized properties characterizing a complex network system (Walker et al., 2004; Siegenfeld and Bar-Yam, 2020), which are measured with three basic network metrics: degree, betweenness, and closeness, respectively. Rather than measuring the total connections as stated by Newman (2003), we used degree to measure the differences between total connections of one discipline with the average total connections of all disciplines within the network. Higher degree value indicates greater imbalances of disciplinary connections in the knowledge system and therefore higher inequality. Betweenness measures the average times one discipline sits along the shortest distance between all other disciplines in the network. Higher betweenness indicates the more disciplines act as the “medium” for cross-disciplinary knowledge transfer and the more resilient (redundant) the knowledge system is (Freeman, 1977). Closeness measures the inverse of average shortest connections between a discipline and all other disciplines (Latora and Marchiori, 2001). Higher closeness indicates shorter distances among different disciplines, meaning higher efficiency in knowledge propagation and easier to establish collaborations among disciplines.
We further defined the different development stages of a knowledge system based on the system transition theory as outlined in Brugge and Rotmans (2007), which include initialization, take-off, acceleration, and stabilization/decay stages. During the initialization, take-off, and acceleration stages, a knowledge system is considered to be expanding in either scalar, structural, or both metrics. When the scalar metrics of a knowledge system do not change significantly with increasing publications, we consider the knowledge system enters the scalar stabilization stage. Furthermore, the knowledge system is considered to enter the structural stabilization stage when one or two structural metrics degrade with time and the other one or two metrics do not significantly change with scale. A knowledge system is defined to be “ageing” when it enters both the scalar and structural stabilization stages. Thus, this network metric-based framework can be used to identify the evolutionary patterns and the structural deficiencies if any for the river basin knowledge systems. This will enable structural direction of future knowledge development on river basins from a system perspective.
Data Sources and Study Period
We used scientific publications indexed in the Science Citation Index (SCI) of the WoS database as the data source in this study. WoS is a widely used database for analysis of scientific publications, covering approximately 12,000 top-tier international and regional journals in all areas of natural and social sciences and the humanities (Rousseau et al., 2019). It classifies articles according to the ISI Subject Category, which includes 254 disciplinary categories under five major Research Areas: arts and humanities, life sciences and biomedicine, physical sciences, social sciences, and technology https://images.webofknowledge.com/images/help/WOS/hp_subject_category_terms_tasca.html).
Our study period spanned 30 years from 1987 to 2017 to reflect the rapid increase of river basin studies since 1990s (Wu et al., 2021). English research publications in the WoS containing the words river” OR “river basin” OR “watershed” OR “catchment” OR “drainage basin” OR “hydrographic basin” OR “wetland” OR “valley” in the Title, Abstract and Keywords sections were retrieved as the raw dataset (Rebholz-Schuhmann et al., 2012). Each publication was automatically assigned to one or more disciplines based on the disciplinary categories of the journal published. Duplicate publications were removed and publications focusing smaller spatial units (e.g., sub-catchment, wetland or lake) were merged in the river basin which they are affiliated with. We identified the top 100 river basins with the largest number of publications for analysis as they covered major publications on global river basins. After those with ambiguous data (i.e., same river names with different locations) were removed, 95 river basins and a total of 190577 publications covering 216 disciplines were finally used for further analysis.
Data Analysis
Based on the co-occurrence principle (Callon et al., 1983), a disciplinary network was established for each river basin: two disciplines were connected when they were linked to the same publication. It should note that equal weights were assigned to links between disciplines to reduce bias towards highly published disciplines. These disciplinary networks were established annually for each river basin to track the temporal evolution of the knowledge systems. Establishment of the disciplinary networks and calculations of the scalar and structural metrics as outlined in Table 1 for each river basin for each year using the “igraph” package in R (https://igraph.org/). Then the metric values were normalized for comparison between metrics. Finally, both linear and nonlinear least square regression analysis using the “stats” package in R (https://www.rdocumentation.org/packages/stats/versions/3.6.2) were conducted to reveal the temporal relationships with the scalar and structural metrics, the relationships between the scalar and structural metrics, and among the structural metrics.
Results
Evolutions of Publications in the 95 River Basins
The 95 river basins were divided into five groups to reflect the disproportional increase (in logarithmic scale of 10) of the total number of publications among river basins: very highly researched (VH,
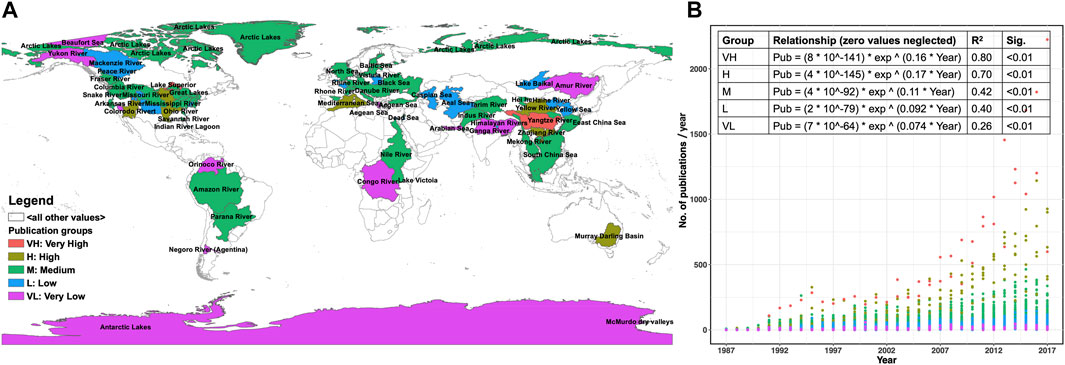
FIGURE 1. (A) Spatial distribution of the five publication groups in the chosen 95 river basins, and (B) temporal evolution of publications in each group.
Spatially, the number of publications were unevenly distributed among the 95 river basins (Figure 1A). Two spatial centers were observed, signified by the two river basins classified into the “VH” group: the Yangtze River (14498 publications in total) and the Great Lakes (11946). The six river basins in the “H” group indicated several hot spots in North America (the Mississippi River, the Colorado River), Asia (the Yellow River; the Pearl River), Europe (the Mediterranean Sea), and Oceania (the Murray-Darling Basin), all of which had publications ranged between 4,000 and 9,000. Moderate research publications were most common in the European rivers, with the “M” group (44 river basins) being comprised mainly by European rivers (43%), followed by 25% in Asia, 23% in North America, and the remaining equally shared between South America and Africa. In the “L” group (30 river basins), 43% were in North America, 33% in Asia, 17% in Europe and only 7% in Africa. The “VL” group (13 river basins) was dominated by Asian rivers (31%), followed by North American rivers (23%), the two Poles and South America (15% respectively), Europe and Africa (7% respectively). The river basins in Arctic and Antarctic tended received the smallest amounts of publications (e.g., the Mcmurdo Dry Valley, the Antarctic Lakes, the Beaufort Sea) with less than 400 publications in total.
The number of publications per year demonstrated exponential growth rates in all five groups of the 95 river basins (Figure 1B). It is shown that the growth rates followed a descending order for river basins moving from the “VH” to the “VL” group. River basins in the “VH” reached as high as over 2,000 publications per year in 2017 and demonstrated the strongest exponential growth. Along with the river basins in the “H” groups, these river basins only comprised of about 8% of the total river basins studied but contributed to 35% of total publications. Although a majority of river basins in the “M” and “L” groups (78%) had average total publications of about 2000 and 700 respectively, these river basins demonstrated similar growth rates to the “VH” and “H” groups but weaker exponential relationships (R2 = 0.42 and 0.40, p < 0.05, respectively). A much weaker exponential relationship was observed with the “VL” group river basins (R2 = 0.26, p < 0.05), with average publications of only 300.
Evolutions of the Scales and Structures of the 95 River Basins’ Knowledge Systems
The scales of the knowledge networks for the 95 river basins linearly increased in time (Figure 2A). The strongest linear relationship was observed for river basins in the “VH” group (R2 = 0.68), which also had the greatest average scale values. The Yangtze River in the “VH” group had the greatest scale metric in 2017 with 88 disciplines in the network and was followed by the Mississippi River (62), the Yellow River 56) and the Pearl River (56), all of which belonged to the “H” group. Although the Great Lakes belonged to the “VH” publication group, it only had 36 disciplines in its knowledge network in 2017. The scalar growth rates reduced in the lower publication groups. The normalized scalar ranges reduced from 0.7 in the “H” group river basins to below 0.6 in the “M” group, 0.3 in the “L” group and 0.2 in the “VL” group. Environmental Sciences remained the most published discipline among the five groups in time. Water Resources and Multidisciplinary Geosciences were in the top three most published disciplines in the “H” group, whereas the “VH” group demonstrated specialized focuses on Marine & Freshwater Biology and Limnology since 1987. Between 1990 and 2000, Environmental Engineering and Civil Engineering emerged as the top five highly published disciplines for the “M” and “L” group river basins. Since 2000, the highly published disciplines remained relatively stable among all five groups. River basins demonstrated similar focuses with Water Resources, Marine & Freshwater Biology, Multidisciplinary Geosciences, and Ecology as the top five most published disciplines that contributed to more than 50% of total publications until 2017 (refer to Supplementary Table S2 for disciplines studied in each publication group).
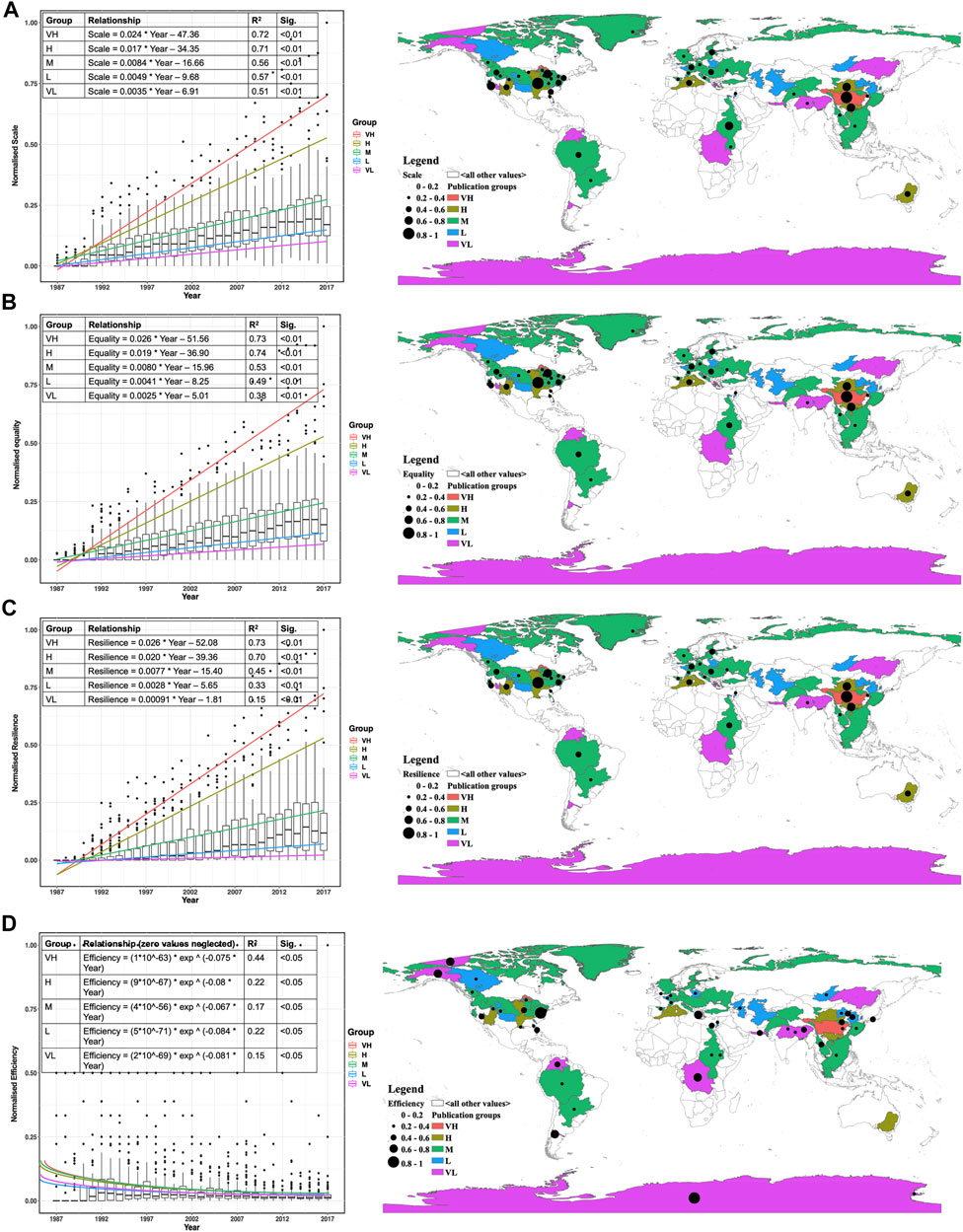
FIGURE 2. Temporal evolutions (left) and spatial distributions (right) of the (A) scale, (B) equality, (C) resilience, and (D) efficiency among the five publication groups during 1987–2017.
Both the equality and resilience metrics for the five groups demonstrated linear relationships in time (Figures 2B,C). As the river basins moved from the highest published group “VH” to the lowest published group “VL”, the growth rates reduced by 10 folds for equality and almost 30 folds for resilience. River basins in the “M”, “L” and “VL” groups were less sensitive to time (R2 < 0.5, p < 0.01), and only received marginal development in their knowledge structures. On the other hand, the efficiency metrics demonstrated more turbulent patterns, decreasing in time in general (Figure 2D). The “VH” group river basins showed the strongest exponential decay in time (R2 = 0.44, p < 0.05), whereas this was less evident in the remaining groups (R2 < 0.3, p < 0.05). Higher efficiency values were more frequent before 2010. The “VL” group river basins had the greatest average efficiency value in time (0.16). Only the Peace River (“L”), the Indian River (“L”) and the Death Valley (“VL”) reached over 0.5 after 2010 and almost 90% of river basins reached their maximum values before 2000. Multidisciplinary Geosciences and Physical Geography were the key disciplines that demonstrated high efficiency to the Death Valley in time, whereas broader range of disciplines with high efficiencies were identified for the Peace River and the Indian River, including Environmental Sciences, Ecology, Soil Sciences, Marine & Freshwater Biology, and Engineering.
The spatial distribution of the average scale, equality, and resilience metrics of the 95 river basins during our study period was consistent to the total number of publications, presenting Asia and North America as the two major spatial centers (normalized metric values > 0.75), and Europe, South America, Oceania, and Africa as the major hot spots (normalized metric values between 0.25 and 0.75). However, the efficiency metrics were consistently low among most of the river basins. The Death Valley in North America had the greatest efficiency, whereas all other river basins had metric values below 0.25.
Relationships Between the Scales and Structures of the 95 River Basins’ Knowledge Systems
The river basins in all groups demonstrated strong power relationships between the number of publications and the scalar metric of their respective knowledge networks (R2 > 0.7, p < 0.01) (Figure 3A). It should note that the “VL” group river basins having the highest coefficient (0.65), implying that although river basins in this group had the lowest publications, their rates of developing new disciplines were fastest. In contrast, although river basins in the “VH” group were more published, their rates in expanding the scales of their knowledge networks were the smallest (growth coefficient = 0.5).
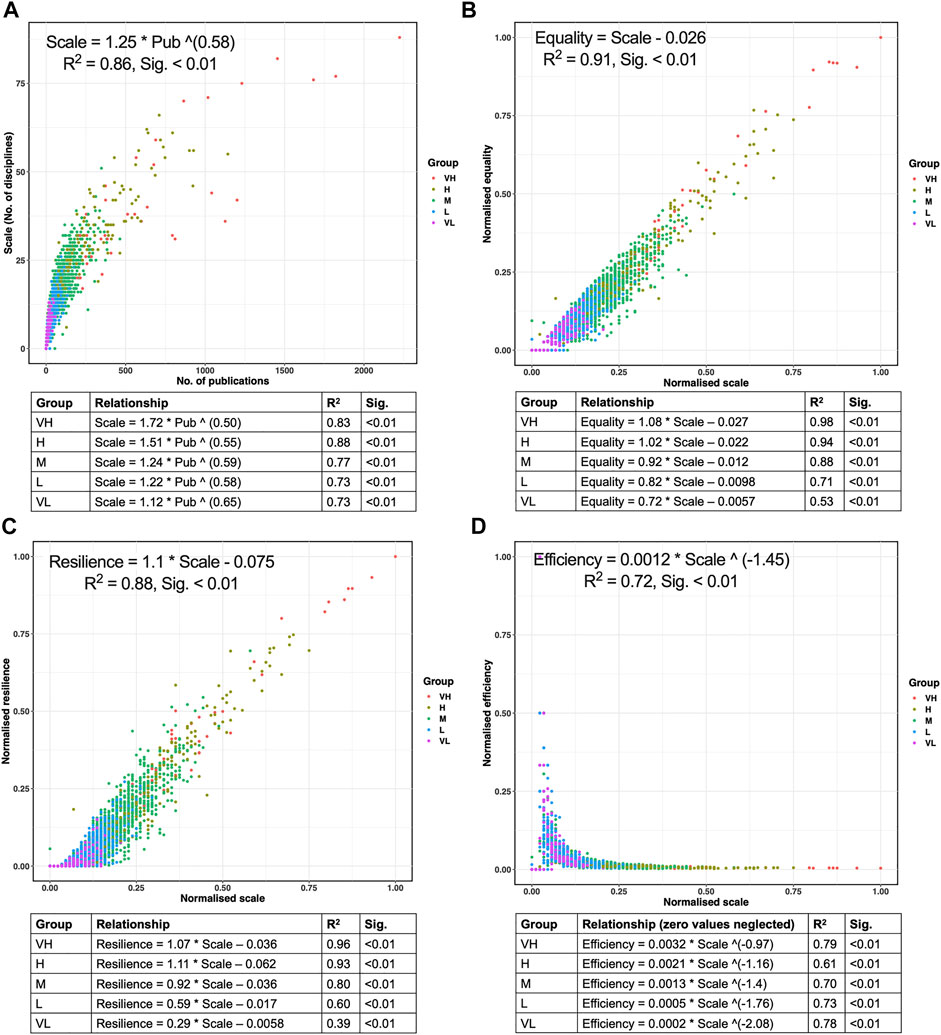
FIGURE 3. (A) Relationships between the publication and scale, and between the scalar and structural metrics of (B) equality and scale, (C) resilience and scale, and (D) efficiency and scale among five publication groups.
The equality and resilience metrics of the 95 river basins demonstrated linear relationships with the scalar metric (Figures 3B,C). These two structural metrics for river basins in the “VH”, “H” and “M” groups (55% of river basins) had growth rates close to 1 with the scalar metrics. This indicates that the inequality and resilience of the knowledge networks proportionally increase with its scale (R2 > 0.8, p < 0.01). For river basins in the “L” and “VL” groups (45% of river basins), the linear relationships were weaker (R2 ranged between 0.53–0.71 for equality and between 0.39–0.60 for resilience). These river basins with smaller scale (normalised scale <0.3) demonstrated more scattered distributions of structural metrics, with slightly lower growth rates with respect to scalar increases.
The efficiency metrics demonstrated an inverse power law relationship with the scalar metric (Figure 3D). This relationship was consistent among the five publication groups (R2 ranged between 0.6 and 0.7, p < 0.01), but the rates of decay increased with reducing publication groups. This mean that although the river basins with low publications (i.e., the “VL” and “L” groups) had smaller scales (<0.3), they demonstrated higher average efficiency values and thus more effective knowledge structures. On the other hand, although the highly published river basins (i.e., the “VH”, “H”, and “M” groups) had greater scales (0.6–1), their knowledge structures were less sensitive to changes in scale.
Relationships Among the Structural Metrics of the 95 River Basins’ Knowledge Systems
It is not surprising that the equality and resilience metrics demonstrated a linear relationship (Figure 4A). This is most evident in the “VH” and “H” group river basins, which indicates proportional development (growth rate close to1) between inequality (increasing degree) and resilience (increasing betweenness) for the knowledge systems in highly published river basins (R2 > 0.8, p < 0.01). It should also note that river basins in the “VH” group covered the broadest equality and resilience value range from 0 to 1, while the “H” group only covered between 0 and 0.8. This relationship also holds for the “M” group, which comprised about 46% of total river basins with increased scattering (R2 = 0.73, p < 0.01). Smaller increase in resilience with increase of inequality was more evident among the lower published river basins in the “L” and “VL” groups (R2 reduced from 0.60 to 0.36 and growth rates reduced from 0.64 to 0.34, p < 0.01).
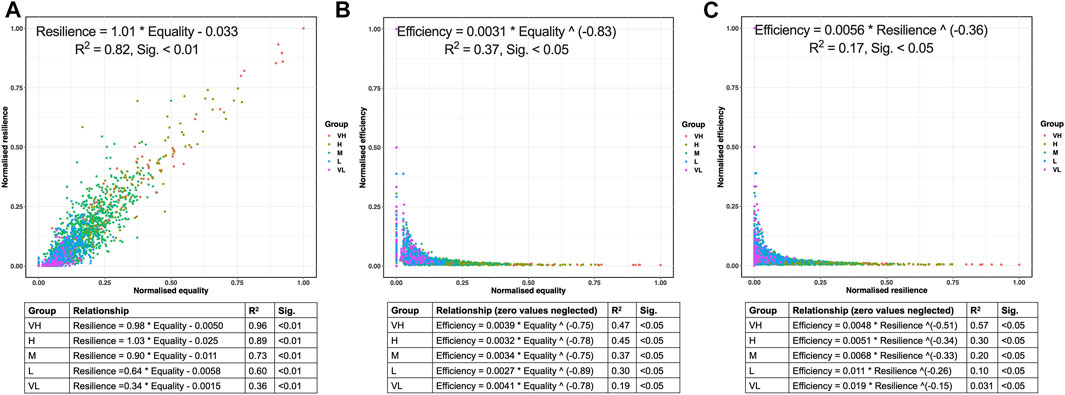
FIGURE 4. Relationships of structural metrics between: (A) resilience and equality, (B) efficiency and equality, and (C) efficiency and resilience among the five publication groups.
Both the equality and resilience metrics demonstrated power decay relationships with the efficiency metric (Figures 4B,C). The rates of decay between efficiency and equality were similar among the five publication groups (ranged between -0.75 and -0.89, p < 0.05). This indicates that the trade-off relationship between efficiency (increasing closeness) and inequality (increasing degree) were insensitive to varying publications among different publication groups. For highly published river basins (i.e., the “VH”, “H” and “M” groups), 90% of the normalized efficiency values remained below 0.1 (i.e., low closeness) with increases in normalized inequality (i.e., high degree). For limited published river basins (i.e., the “VL” and “L” groups), they tended to achieve high efficiency (i.e., high closeness) when the structural inequality was low (i.e., low degree).
On the other hand, the rates between efficiency and resilience reduced with reducing publication groups (reduced from -0.51 to -0.15, p < 0.05). This indicates that the trade-off relationship between efficiency (increasing closeness) and resilience (decreasing betweenness) were more sensitive to varying publications among different publication groups. For highly published river basins (i.e., the “VH”, “H” and “M” groups), there were greater reductions in normalized efficiency (i.e., lower closeness) with increases in normalized resilience (i.e., high betweenness), as 90% of their normalized efficiency values remained below 0.1. For limited published river basins (i.e., the “VL” and “L” groups), the trade-off relationships were even weaker (R2 < 0.1), indicating limited influences of the resilience on efficiency for these river basins.
Discussion and Conclusion
We developed a network metric-based framework comprising scale, equality, efficiency, and resilience to reveal the endogenous processes of knowledge developments. Specifically, we examined the knowledge development in the top 95 most researched global river basins with scientific publications in the WoS database during 1987–2017. Key findings and their implications for future knowledge development on river basins are summarized as follows:
•216 of the total 254 disciplines in the WoS database were involved in river basin studies during 1987–2017. With little exception, Environmental Sciences, Water Resources, Multidisciplinary Geosciences, Ecology, and Marine & Freshwater Biology dominated 50–55% publications. These disciplines had high inequality (degree), high redundancy (betweenness) and low efficiency (closeness) values in the knowledge networks, implying rigid compositions of the knowledge systems in the 95 river basins.
•Spatially, scientific publications in the 95 river basins were diverse with two centers: Asia and North America, comprising a majority of river basins from the “VH” and “H” publication groups. Temporally, river basins in these two groups presented stronger patterns in terms of number of publications, scale, equality, resilience, and efficiency than those in the lowly published “L” and “VL” groups (higher R2).
•The relationships between the number of publications and the scale (number of disciplines) demonstrate that highly published river basins in the “VH” and “H” groups had much slower increase in scale with increases in publications compared to those in the “L” and “VL” groups. This implies that knowledge development in the “VH” and “H” groups river basins entered the stage of scalar stabilization whereas those in the “L” and “VL” groups were still at the stage of initiation or take-off. “M” group river basins were at the stage of transition to stable development.
•The relationships between the structural and scalar metrics differed among different publication groups. When the normalized scale is approximately greater than 0.1–0.15 (i.e., 10–15 disciplines), river basins had linearly increased inequality and resilience with scale, while their efficiency tended to zero. This implies that the knowledge structure in the highly researched, large-scale river basins entered the “ageing” states (high inequality, low efficiency, and high resilience). Unsurprisingly, inequality had positively linear relationships with resilience, and there were trade-offs between efficiency and resilience, and between efficiency and equality.
These findings are of great implications for future knowledge development in these five river basin groups. Specifically, those river basins in the “VH” and “H” groups were with the ageing structures, characterized by decay structure (increasing inequality and resilience and extremely low efficiency), stabilized scales, and rigid composition of disciplines. Substantial transformation of both the composition and structure of the existing knowledge systems are required. This can be done by replacing existing disciplines with and establishing links to a wide spectrum of disciplines in Humanities and Social Sciences, which require substantial strategical intervention and may take a long time. Those river basins in the “M” group were with a developing knowledge structure in either take-off or acceleration stages, progressive actions on changing the scales of their knowledge systems by introducing new disciplines should be considered, which provide leverage to balancing the relationships between the three structural metrics. Those river basins in the “L” and “VL” groups lied in the initialization stage, the planning and design for balanced development of compositions and structures of the knowledge systems should be made as early as possible to avoid falling into the ageing structures in the “VH” and “H” groups.
The finding that the knowledge structures of highly researched river basins (VH and H groups) have entered the ageing stage has also been observed by Blöschl et al. (2019) in hydrology based on a recent survey of over 200 hydrologists, by Ancey (2020) in hydraulics, and by Burt and McDonnell (2015) in hillslope hydrology, all of which express a growing concern in traditional disciplines. In a general sense, this finding follows the paradigm of science development “punctuated growth” or the euphoria-disenchantment cycles (Eldredge and Gould, 1972). In this paradigm, the science development is due to long periods of knowledge accumulation of “normal science”, interrupted by discontinuous transformations generated by new theoretical and empirical approaches that support the transition from an existing scientific paradigm to an emerging one. From the network perspective, the knowlegde structure in “VH” and “H” river basin groups are characterized by the presence of a few nodes (disciplines) with large degrees, small distances between nodes, and resilient to random failures, which were considered as the “small-world” network (Newman, 2003). These knowledge networks are scale-free (most complex at the smallest scales and rapidly drop off as scales increase) (Zhang and Chen, 2021), and the trade-offs between resilience and efficiency (a very resilient system will necessarily have to sacrifice its efficiency largely) (Siegenfeld and Bar-Yam, 2020).
The endogenous structures of knowledge systems in river basins identified in this study are influenced by a multitude of external drivers. Firstly, some scientific disciplines are still organized as a closed and self-regulated knowledge system. They set the research agenda autonomously and are detached from societal needs (Cornell et al., 2013). Secondly, science development is in an era of academic capitalism (Hoffman, 2021). There has been an internal push by some universities and institutes to leverage academic assets toward revenue-driven opportunities. Thirdly, the scientific community is a subsystem of society that is both socially constructed and shaped, thus inevitably reflects the concerns and interests of specific regions, nations and/or human societies (Coccia, 2020). For instance, it is found that some disciplines are consistently developed across countries with different income levels, while others are more or less developed depending on the income levels and industry sectors of the country (Jaffe et al., 2020). For river basin research, studies have demonstrated that the knowledge systems of different river basins developed were different to solve specific local problems (Bouleau, 2014; Su et al., 2020). Transformation of the current river basin knowledge system requires understanding of all these external drivers, yet research on the knowledge development has not been central to scientific work and relies on scientists’ post hoc reflections (Callon, 1994; Kallio and Houtbeckers, 2020). Thus, we argue that science of science development should be as an integral part of scientific research, providing the solid foundation for designing the structures and mechanisms that influences scientific activities (Bacevic, 2020).
We recognize the limitations of our study and a number of interesting avenues that remain unexplored. Firstly, only journal papers on the WoS database were considered and only 95 river basins were studied, which indicated future research with broader study scopes and use of more comprehensive databases. Secondly, the classification system in the WoS database classifies journals, not publications with coarse disciplinary boundaries. Recent research on designing field delineation algorithms using big data have provided potential improvement from this classification system (Lietz, 2020). Thirdly, assigning weights to the links between disciplines based on the number of publications may help more precisely analyse the structural metrics (Wei and Wu, 2022). Finally, further research efforts should be made to answer why metrics demonstrate different statistical behaviors, how and why such differences arise and disappear in different river basins from both endogenous structures investigated in this study and the external drives discussed as above.
The ability of the human society to cope with challenges of the Anthropocene critically depends on the development of its knowledge system. The degradation of global river basins implies the limited capacity in science development and application. This paper has made a solid first step towards the quantification, assessment, and prediction of knowledge development on global river basins by empirically revealing the endogenous structures of science development on river basin. It can assist in avoiding further deterioration of river basins from the perspective of strategical planning and design of knowledge structure.
Data Availability Statement
The datasets presented in this study can be found in online repositories. The names of the repository/repositories and accession number(s) can be found in the article/Supplementary Material.
Author Contributions
YW and SW equally contributed to design of the study, analysed and interpreted the results, drafted and revised the manuscript; ZL and XW collected the data, conducted data analysis, and interpreted the results; XW conducted data analysis and data validation; LX interpreted the results; and MS substantially revised the manuscript for implications and knowledge development. All authors edited and agreed on the manuscript.
Funding
This study is supported by Australian Research Council Special Research Initiative (SR200200186) and the University of Queensland Research Stimulus (UQ RS) Fellowship funding.
Conflict of Interest
The authors declare that the research was conducted in the absence of any commercial or financial relationships that could be construed as a potential conflict of interest.
Publisher’s Note
All claims expressed in this article are solely those of the authors and do not necessarily represent those of their affiliated organizations, or those of the publisher, the editors, and the reviewers. Any product that may be evaluated in this article, or claim that may be made by its manufacturer, is not guaranteed or endorsed by the publisher.
Supplementary Material
The Supplementary Material for this article can be found online at: https://www.frontiersin.org/articles/10.3389/fenvs.2022.821342/full#supplementary-material
References
Ancey, C. (2020). Bedload Transport: a Walk between Randomness and Determinism. Part 2. Challenges and Prospects. J. Hydraulic Res. 58 (1), 18–33. doi:10.1080/00221686.2019.1702595
Bacevic, J. (2020). Unthinking Knowledge Production: from post-Covid to post-carbon Futures. Globalizations 18, 1206–1218. doi:10.1080/14747731.2020.1807855
Biggs, R., Schlüter, M., and Schoon, M. L. (2015). Principles for Building Resilience: Sustaining Ecosystem Services in Social-Ecological Systems. London: Cambridge University Press.
Blöschl, G., Bierkens, M. F. P., Chambel, A., Cudennec, C., Destouni, G., Fiori, A., et al. (2019). Twenty-three Unsolved Problems in Hydrology (UPH) – a Community Perspective. Hydrol. Sci. J. 64 (10), 1141–1158. doi:10.1080/02626667.2019.1620507
Bouleau, G. (2014). The Co-production of Science and Waterscapes: The Case of the Seine and the Rhône Rivers, France. Geoforum 57, 248–257. doi:10.1016/j.geoforum.2013.01.009
Brugge, R. v. d., and Rotmans, J. (2007). Towards Transition Management of European Water Resources. Water Resour. Manage. 21 (1), 249–267. doi:10.1007/s11269-006-9052-0
Burt, T. P., and McDonnell, J. J. (2015). Whither Field Hydrology? The Need for Discovery Science and Outrageous Hydrological Hypotheses. Water Resour. Res. 51 (8), 5919–5928. doi:10.1002/2014wr016839
Callon, M., Courtial, J.-P., Turner, W. A., and Bauin, S. (1983). From Translations to Problematic Networks: An Introduction to Co-word Analysis. Soc. Sci. Inf. 22 (2), 191–235. doi:10.1177/053901883022002003
Callon, M. (1994). Is Science a Public Good? Fifth Mullins Lecture, Virginia Polytechnic Institute, 23 March 1993. Sci. Technol. Hum. Values 19 (4), 395–424. doi:10.1177/016224399401900401
Cheng, Q., Wang, J., Lu, W., Huang, Y., and Bu, Y. (2020). Keyword-citation-keyword Network: a New Perspective of Discipline Knowledge Structure Analysis. Scientometrics 124 (3), 1923–1943. doi:10.1007/s11192-020-03576-5
Coccia, M. (2020). The Evolution of Scientific Disciplines in Applied Sciences: Dynamics and Empirical Properties of Experimental Physics. Scientometrics 124 (1), 451–487. doi:10.1007/s11192-020-03464-y
Cooper, G. S., Willcock, S., and Dearing, J. A. (2020). Regime Shifts Occur Disproportionately Faster in Larger Ecosystems. Nat. Commun. 11 (1), 1175. doi:10.1038/s41467-020-15029-x
Cornell, S., Berkhout, F., Tuinstra, W., Tàbara, J. D., Jäger, J., Chabay, I., et al. (2013). Opening up Knowledge Systems for Better Responses to Global Environmental Change. Environ. Sci. Pol. 28, 60–70. doi:10.1016/j.envsci.2012.11.008
Di Baldassarre, G., Sivapalan, M., Rusca, M., Cudennec, C., Garcia, M., Kreibich, H., et al. (2019). Sociohydrology: Scientific Challenges in Addressing the Sustainable Development Goals. Water Resour. Res. 55 (8), 6327–6355. doi:10.1029/2018wr023901
Eldredge, N., and Gould, S. J. (1972). “Punctuated Equilibria: an Alternative to Phyletic Gradualism,” in Models in Paleobiology. Editor J. M. Thomas (San Francisco: Freeman, Cooper and Company), 82–115.
Fortunato, S., Bergstrom, C. T., Börner, K., Evans, J. A., Helbing, D., Milojević, S., et al. (2018). Science of Science. Science 359 (6379), eaao0185. doi:10.1126/science.aao0185
Freeman, L. C. (1977). A Set of Measures of Centrality Based on Betweenness. Sociometry 40 (1), 35–41. doi:10.2307/3033543
Hoffman, S. G. (2021). A story of Nimble Knowledge Production in an Era of Academic Capitalism. Theor. Soc. 50 (4), 541–575. doi:10.1007/s11186-020-09422-0
Jaffe, K., ter Horst, E., Gunn, L. H., Zambrano, J. D., and Molina, G. (2020). A Network Analysis of Research Productivity by Country, Discipline, and Wealth. PLOS ONE 15 (5), e0232458. doi:10.1371/journal.pone.0232458
Kallio, G., and Houtbeckers, E. (2020). Academic Knowledge Production: Framework of Practical Activity in the Context of Transformative Food Studies. Front. Sustain. Food Syst. 4 (218), 577351. doi:10.3389/fsufs.2020.577351
Khasseh, A. A., Soheili, F., Moghaddam, H. S., and Chelak, A. M. (2017). Intellectual Structure of Knowledge in iMetrics: A Co-word Analysis. Inf. Process. Manage. 53 (3), 705–720. doi:10.1016/j.ipm.2017.02.001
Latora, V., and Marchiori, M. (2001). Efficient Behavior of Small-World Networks. Phys. Rev. Lett. 87 (19), 198701. doi:10.1103/PhysRevLett.87.198701
Latour, B. (1987). Science in Action: How to Follow Scientists and Engineers through Society. London: Harvard University Press.
Lietz, H. (2020). Drawing Impossible Boundaries: Field Delineation of Social Network Science. Scientometrics 125 (3), 2841–2876. doi:10.1007/s11192-020-03527-0
Liu, J., Bawa, K. S., Seager, T. P., Mao, G., Ding, D., Lee, J. S. H., et al. (2019). On Knowledge Generation and Use for Sustainability. Nat. Sustain. 2 (2), 80–82. doi:10.1038/s41893-019-0229-y
Newman, M. E. J. (2003). The Structure and Function of Complex Networks. SIAM Rev. 45 (2), 167–256. doi:10.1137/s003614450342480
Pahl-Wostl, C. (2015). Water Governance in the Face of Global Change: From Understanding to Transformation. Switzerland: Springer International Publishing.
Rebholz-Schuhmann, D., Oellrich, A., and Hoehndorf, R. (2012). Text-mining Solutions for Biomedical Research: Enabling Integrative Biology. Nat. Rev. Genet. 13 (12), 829–839. doi:10.1038/nrg3337
Rousseau, R., Zhang, L., and Hu, X. (2019). “Knowledge Integration: Its Meaning and Measurement,” in Springer Handbook of Science and Technology Indicators. Editors W. Glänzel, H. F. Moed, U. Schmoch, and M. Thelwall (Cham: Springer International Publishing), 69–94. doi:10.1007/978-3-030-02511-3_3
Shi, F., Foster, J. G., and Evans, J. A. (2015). Weaving the Fabric of Science: Dynamic Network Models of Science's Unfolding Structure. Soc. Netw. 43, 73–85. doi:10.1016/j.socnet.2015.02.006
Siegenfeld, A. F., and Bar-Yam, Y. (2020). An Introduction to Complex Systems Science and its Applications. Complexity 2020, 1–16. doi:10.1155/2020/6105872
Steffen, W., Grinevald, J., Crutzen, P., and McNeill, J. (2011). The Anthropocene: Conceptual and Historical Perspectives. Phil. Trans. R. Soc. A. 369 (1938), 842–867. doi:10.1098/rsta.2010.0327
Steffen, W., Richardson, K., Rockström, J., Cornell, S. E., Fetzer, I., Bennett, E. M., et al. (2015). Planetary Boundaries: Guiding Human Development on a Changing Planet. Science 347 (6223), 1259855. doi:10.1126/science.1259855
Su, W., Tao, J., Wang, J., and Ding, C. (2020). Current Research Status of Large River Systems: a Cross-continental Comparison. Environ. Sci. Pollut. Res. 27 (31), 39413–39426. doi:10.1007/s11356-020-09879-7
Turnbull, L., Hütt, M.-T., Ioannides, A. A., Kininmonth, S., Poeppl, R., Tockner, K., et al. (2018). Connectivity and Complex Systems: Learning from a Multi-Disciplinary Perspective. Appl. Netw. Sci. 3 (1), 11. doi:10.1007/s41109-018-0067-2
Vörösmarty, C. J., McIntyre, P. B., Gessner, M. O., Dudgeon, D., Prusevich, A., Green, P., et al. (2010). Global Threats to Human Water Security and River Biodiversity. Nature 467 (7315), 555–561. doi:10.1038/nature09440
Walker, B., Holling, C. S., Carpenter, S. R., and Kinzig, A. (2004). Resilience, Adaptability and Transformability in Social-Ecological Systems. Ecol. Soc. 9 (2), 5. doi:10.5751/es-00650-090205
Wei, Y., and Wu, S. (2022). The Gulf of Cross-Disciplinary Research Collaborations on Global River Basins Is Not Narrowed. Ambio. Accepted. doi:10.1007/s13280-022-01716-0
Wu, S., Wei, Y., and Wang, X. (2021). Global Water Resources Knowledge Gaps. Hydrol. Earth Syst. Sci. 2021, 1–16. doi:10.5194/hess-2021-137
Keywords: complex knowledge system, knowledge structure, knowledge scale, network metric-based framework, river basin management
Citation: Wei Y, Wu S, Lu Z, Wang X, Wu X, Xu L and Sivapalan M (2022) Ageing Knowledge Structure in Global River Basins. Front. Environ. Sci. 10:821342. doi: 10.3389/fenvs.2022.821342
Received: 24 November 2021; Accepted: 21 March 2022;
Published: 06 April 2022.
Edited by:
Chuanbo Guo, Institute of Hydrobiology (CAS), ChinaReviewed by:
Jun Wang, Huazhong Agricultural University, ChinaChuansong Liao, Institute of Hydrobiology (CAS), China
Copyright © 2022 Wei, Wu, Lu, Wang, Wu, Xu and Sivapalan. This is an open-access article distributed under the terms of the Creative Commons Attribution License (CC BY). The use, distribution or reproduction in other forums is permitted, provided the original author(s) and the copyright owner(s) are credited and that the original publication in this journal is cited, in accordance with accepted academic practice. No use, distribution or reproduction is permitted which does not comply with these terms.
*Correspondence: Shuanglei Wu, c2h1YW5nbGVpLnd1QHVxLmVkdS5hdQ==
†These authors have contributed equally to this work