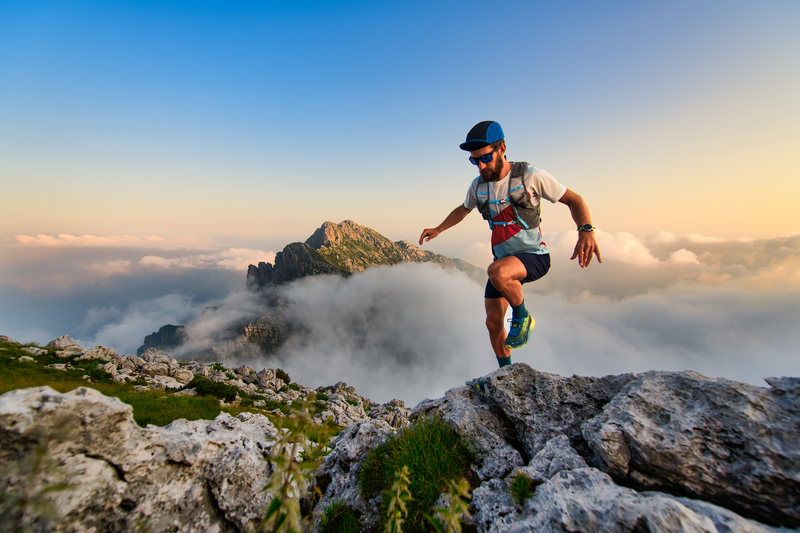
94% of researchers rate our articles as excellent or good
Learn more about the work of our research integrity team to safeguard the quality of each article we publish.
Find out more
ORIGINAL RESEARCH article
Front. Environ. Sci. , 14 April 2022
Sec. Water and Wastewater Management
Volume 10 - 2022 | https://doi.org/10.3389/fenvs.2022.817655
This article is part of the Research Topic Sustainable Sanitation- How Can We Improve Sanitation Systems in the Global South? View all 19 articles
The imbalance of socioeconomic development (SED) in different regions of China has resulted in the variability of rural infrastructure penetration. This study aims to improve the SED of each region in China to increase the penetration rate of rural harmless sanitary toilets (RHST). For this purpose, we used association rule mining to analyze the relationship between SED indicators and the penetration rate of RHST for proposing differentiated improvement strategies. Population urbanization rate, tertiary vs. secondary industry output ratio, nonagricultural output value ratio, nonagricultural employment ratio, per capita gross domestic product, and the proportion of added value of industry in the total added value of commodity were used to measure the SED level of 30 regions in China from 2007 to 2017. Results showed that the SED of each region has been improving, and the proportion of added value of industry in the total added value of commodity accounted for the highest proportion of SED. The penetration rate of RHST in each region increased continuously but with significant variability from 2007 to 2017. The range of six SED indicators corresponding to high and low penetration rates of RHST was determined by association rule mining analysis. On the basis of the degree of RHST penetration rate by region in China in 2017 as a reference, differentiated measures were proposed to improve the penetration of RHST in different regions.
More than half (55%) of the global population lacked access to safely managed sanitation services in 2017 (WHO, 2020b). Developing countries (compared with developed countries) face more serious challenges in achieving the Sustainable Development Goal target of universal sanitation coverage by 2030, which is mainly due to high population growth and low coverage of existing sanitation facilities (United Nations (2017) World Population Prospects, 2017; Trimmer et al., 2020; WHO, 2020b). Moreover, in a recent survey, only 4% of countries were reported to have sufficient financial resources to achieve national sanitation targets (WHO, 2020a). Household sanitation expenditures (compared with government expenditures) account for a higher proportion of all household water, sanitation, and hygiene expenditures (WHO, 2020a). Rural areas in developing countries also face high sanitation failure rates, which is often due to most rural areas not having well-established operation and maintenance systems (Roubík et al., 2020).
Successful sanitation change not only depends on the supply of sanitation infrastructure but also requires changes in the political, economic, social, cultural, and environmental underpinnings of sanitation, as well as systemic behavior change at the individual, household, and societal levels (Gasana et al., 2002; Novotný et al., 2018b; Guo et al., 2021). Rapid socioeconomic development (SED) is a major driving force for the continuous improvement in rural sanitation facilities in China. In 2017, rural residents of 12.519 million (compared with 6.914 million in 2007) new rural households in China had access to sanitary toilets (Ministry of Health of the People’s Republic of China, 2008; China National Health Commission, 2018). However, the imbalance of SED across China’s regions resulted in inconsistent penetration rate of rural harmless sanitary toilets (RHST) (China National Health Commission, 2018; Tian and Wang, 2019; Wei et al., 2020). Therefore, the high-quality SED of each region is the key to achieving rapid penetration rate of RHST in China.
The study of SED has been developed for multiple decades. The per capita income of a country’s inhabitants can be an indicator that reflects SED (Chenery, 1960). According to the classical industrialization theory, the level of SED of a country or region can generally be measured in terms of economic development level, industrial structure, agricultural structure, employment structure, and spatial structure (Chen et al., 2006). According to Engel’s law, the proportion of income spent on food consumption will gradually decrease as a household’s income increases (Kaus, 2013), which means that the more a household will be able to improve household sanitation facilities. With the collection of various statistics becoming more detailed, the total value of the country’s primary, secondary, and tertiary industries is also used to describe the country’s SED (Wolfe, 1955). Chen et al. analyzed the distribution characteristics of China’s regional industrialization process by introducing per capita gross domestic product (GDP), the ratio of the output value of primary, secondary, and tertiary industries, the proportion of added value of industry in the total added value of commodity, the urbanization rate of the population, and the proportion of employment in the primary industry as basic indicators to provide baseline references for the regional economic development (Chen et al., 2006). In this study, we followed Chen’s methodology and collected a multi-indicator of SED to measure the SED levels of 30 regions in China from 2007 to 2017. The indicators include population urbanization rate (as spatial structure), tertiary vs. secondary industry output ratio (as industrial structure), nonagricultural output value ratio (as agricultural structure), nonagricultural employment ratio (as employment structure), per capita GDP (as economic development level), and the proportion of added value of industry in the total added value of commodity (as industrial structure) (Li et al., 2019). In addition, we collected data on the penetration rate of RHST in China from 2007 to 2017.
Many efforts have been made to reveal the relationship between socioeconomic and sanitation facilities (Ghosh and Cairncross, 2013; Novotný et al., 2018a). Researchers have discovered a pattern visible in data at the national level, in which access to sanitation facilities is strongly associated with the socioeconomic status of households (Ghosh and Cairncross, 2013; Chandana and Rao, 2022). Other researchers have also confirmed that taking advantage of economic opportunities, incorporating specialized technology, and follows-up with behavior change could help ensure not only access but also sustainable use, operation, and maintenance of sanitation (Tilley et al., 2014). However, in low- and middle-income countries, precision interventions (e.g., financial subsidies) implemented by governments and nongovernmental organizations in addition to improving regional SED can achieve higher access to sanitation (Deshpande et al., 2020). China is the largest developing country, with a rural population of 714.96 million and 556.68 million in 2007 and 2017, respectively. However, many of these rural people choose to shift from primary to secondary and tertiary industry employment each year to increase their household economic income, which undoubtedly improves household sanitation facilities (Zhang et al., 2020). At the same time, the rural toilet revolution is being implemented in various regions of China to increase rural sanitation penetration rate based on existing SED, which could increase the possibility of achieving some of the Millennium Development Goals and Sustainable Development Goals (Cheng et al., 2018). In this study, we examined the relationship between six selected categories of SED indicators and RHST penetration rates from 2007 to 2017.
We used the frequent pattern growth algorithm of the association rule mining method for data analysis to reveal the association relationships among items in a given dataset (Li et al., 2019; Liu et al., 2020). The association rule mining problem was initially studied to discover regularities in the shopping behavior of supermarket customers, which has created many commercial opportunities for stores (Agrawal et al., 1993). In the past decade, the technique has been widely used in evaluating medical datasets and accident investigation analysis, which is attributed to frequent patterns enabling the prediction of one item based on the emergence of others (Ahmed and Nath, 2021; Çakır et al., 2021). Here, we used association rule mining to investigate which SED indicators correspond to high RHST penetration rate and what other levels are associated with low RHST penetration rate. Moreover, we identified indicators of SED associated with influencing the current RHST penetration rate and summarized differentiated strategies for coordinating SED for regions to further enhance RHST penetration rate.
A total of 30 regions in mainland China were used as the research objects (the data on the penetration rate of RHST in Tibet, Hong Kong, Macao, and Taiwan are lacking; thus, they were excluded in this study). On the basis of the data provided by the China Statistical Yearbook (National Bureau of Statistics of China, 2018), China Health Statistical Yearbook (Ministry of Health of the People’s Republic of China, 2008), and China Health and Family Planning Statistical Yearbook (National Health and Family Planning Commission of the People’s Republic of China, 2013), data on SED from 2007 to 2017 were collected, including population urbanization rate, tertiary vs. secondary industry output ratio, nonagricultural output value ratio (secondary industry output value + tertiary industry output value)/(primary industry output value + secondary industry output value + tertiary industry output value), nonagricultural employment ratio (number of employees in the secondary industry + number of employees in the tertiary industry)/(number of employees in the primary industry + number of employees in the secondary industry + number of employees in the tertiary industry), per capita GDP, the proportion of added value of industry in the total added value of commodity, and RHST penetration rate.
Each SED indicator was divided by a reference value to remove units of measurement to obtain a dimensionless percentage (Supplementary Table S1A). The weights from the hierarchical analysis process of Chen et al. and the weights from the factor analysis of Li et al. were cited (Supplementary Table S2A), and the weights from the hierarchical analysis and the factor analysis were averaged to obtain the final weights for each indicator (Chen et al., 2006; Li et al., 2019). Finally, the indicator values were weighted and summed to obtain a quantitative measure of SED.
We discretized six SED indicators and RHST penetration rate into different categories using k-means clustering for association rule mining (Table 1). We used the frequent pattern growth algorithm for association rule mining (Agarwal et al., 2000; Han et al., 2004). The problem of association rule mining is to find relationships between the items in a database (Assis et al., 2021). Frequent patterns are patterns that repeatedly appear in a dataset. These patterns carry relevant dataset relationships or correlations (Assis et al., 2021).
TABLE 1. Discretization of the socio-economic development (SED) indicators and rural harmless sanitary toilets (RHST) penetration rate for association rule mining.
Let I be the database of frequent item sets, which is obtained through frequent pattern growth algorithm. Transaction set (T) is a collection of each event. An association rule is an expression shaped as X→Y, which indicates that the conditions in X lead to Y. In the study, we use X to represent different SED indicators, and Y to represent the penetration rate of RHST. The support indicates the frequency of the item set in the dataset (Assis et al., 2021). If the “support” of an item set is greater than a given minimum support threshold, then the item set is frequent (Li et al., 2019). The support of X is defined as the probability of event X occurring in dataset I, support (X) = P (X) (Agarwal, 2013). The support of the rule (X→Y) is the number of tuples in X and Y, as described in Eq. 1 (Agarwal, 2013; Heng et al., 2017; Assis et al., 2021).
The “confidence” is a measure of the accuracy of association rules. Confidence of rule X→Y is defined as the conditional probability of the Y event occurring given that the event X has occurred and is expressed by Eq. 2 (Aggarwal et al., 2014; Assis et al., 2021).
Support and confidence constitute the threshold for establishing association rules. However, these measures are still insufficient to filter out worthless association rules (Aggarwal, 2014). Lift can solve this weakness in association rules.
Lift can measure the correlation of association rules, and it is used to evaluate whether the item sets X and Y are independent, positively correlated, or negatively correlated. If the lift is equal to 1, then the item set is independent; if the lift is less than 1, then the item set is negatively correlated; if the lift is greater than 1, then the item set is positively correlated (Heng et al., 2017). The expression of lift is shown in Eq. 3 (Assis et al., 2021).
We set the goal of association rule mining to find the set of all items that have support greater than the minimum support (i.e., 3%) and confidence greater than the minimum confidence (i.e., 20%) and to make comprehensive decisions based on the lift (Li et al., 2019; Shabtay et al., 2021).
In general, the population urbanization rate in China’s regions showed a continuous upward trend from 2007 to 2017, except for Shanghai, which declined after 2014 (Figure 1A). Shanghai, Beijing, Tianjin, Guangdong, Jiangsu, and Zhejiang were ranked in the top six of China’s population urbanization rates, which are all located in the coastal regions of China, except for Beijing, the capital of China. Henan, Xinjiang, Guangxi, Yunnan, Gansu, and Guizhou were the six regions with the lowest population urbanization rates in 2017. Guizhou, Shaanxi, Fujian, Henan, Chongqing, and Jiangsu were the six regions with the fastest population urbanization rates between 2007 and 2017.
FIGURE 1. Trends of different socioeconomic development (SED) indicators in 30 regions of China from 2007 to 2017.
We analyzed the tertiary vs. secondary industry output ratio for each region in China from 2007 to 2017 and found a continuous upward trend overall (Figure 1B). Beijing, Hainan, Shanghai, Heilongjiang, Gansu, and Tianjin ranked among the top six regions in terms of the tertiary vs. secondary industry output ratio in 2017, while Hebei, Henan, Anhui, Jiangxi, Guangxi, and Shaanxi were the six regions with the lowest tertiary vs. secondary industry output ratio in 2017. Beijing’s tertiary vs. secondary industry output ratio exceeded Shaanxi’s by nearly four times in 2017.
The nonagricultural output value ratio of China’s regions generally showed an upward trend from 2007 to 2017 (Figure 1C), except that the ratios in Heilongjiang decreased after 2010. Shanghai, Beijing, Tianjin, Zhejiang, Guangdong, and Shanxi ranked among the top six regions in terms of the nonagricultural output value ratio in 2017. Guangxi, Xinjiang, Yunnan, Guizhou, Heilongjiang, and Hainan were the six regions with the lowest nonagricultural output value ratio in 2017. However, Hunan, Sichuan, Jilin, Guangxi, Anhui, and Hainan were the top six regions with the fastest growth rate of nonagricultural output value ratio from 2007 to 2017.
The nonagricultural employment ratio of all regions in China from 2007 to 2017 showed an overall increasing trend (Figure 1D), except for Shaanxi where the trend fluctuated significantly; it increased first (2007–2011) and then decreased (2011–2012), and it increased again in the end (2012–2017). Shanghai, Beijing, Tianjin, Zhejiang, Jiangsu, and Guangdong ranked among the top six regions in terms of the nonagricultural employment ratio in 2017. Inner Mongolia, Shaanxi, Guangxi, Yunnan, Gansu, and Guizhou were the six regions with the lowest nonagricultural employment ratio in 2017. However, Guizhou was the region with the most rapid growth in the nonagricultural employment ratio from 2007 to 2017.
The per capita GDP of all regions in China also showed an overall upward trend from 2007 to 2017 (Figure 1E), except for Inner Mongolia, which had a downward trend after 2016; Liaoning, which had a downward trend in 2016, showed rebound in 2017. Beijing, Shanghai, Tianjin, Jiangsu, Zhejiang, and Fujian ranked among the top six regions in terms of the per capita GDP, while Shanxi, Guangxi, Heilongjiang, Guizhou, Yunnan, and Gansu were the six regions with the lowest per capita GDP in 2017. The top six regions in terms of per capita GDP nearly exceeded the lowest six regions by nearly one time in 2017.
The pattern of changes in the proportion of added value of industry in the total added value of commodity of each region from 2007 to 2017 was divided into three cases (Figure 1F): 1) The proportion of added value of industry in the total added value of commodity of Hebei, Jilin, Anhui, Fujian, Jiangxi, Henan, Hubei, Hunan, Guangdong, and Sichuan regions maintained a positive growth trend; 2) The proportion of added value of industry in the total added value of commodity of Beijing, Tianjin, Shanghai, Jiangsu, Zhejiang, Shandong, and Guangxi remained relatively constant (with ± 2 gap stabilized in a fixed range); 3) The proportion of added value of industry in the total added value of commodity in Shanxi, Inner Mongolia, Liaoning, Heilongjiang, Hainan, Chongqing, Guizhou, Yunnan, Shaanxi, Gansu, Qinghai, Ningxia, and Xinjiang changed with a negative trend.
The contribution of different indicators to SED is shown in Figure 2. The proportion of added value of industry in the total added value of commodity had the largest contribution, followed by per capita GDP and population urbanization rate. This result corresponds to the researchers’ finding that the country’s industrialization process drove the increase in economic output and contributed to the shift of labor from rural to urban areas (Li et al., 2019). The trends in the overall level of SED of China’s regions from 2007 to 2017 are shown in Figure 3. The SED among China’s regions showed an imbalance. The overall level of SED in the coastal regions of China was generally higher than in the central and western regions.
The penetration rate of RHST in all regions of China showed an increasing trend from 2007 to 2017 (Figure 4), except for Beijing, Tianjin, Hebei, Heilongjiang, Henan, Hubei, Chongqing, Shaanxi, and Xinjiang, which declined after 2016. The top six rural areas in China in terms of the penetration rate of RHST in 2017 included Shanghai, Beijing, Zhejiang, Fujian, Tianjin, and Guangdong, all of which are located in China’s coastal areas, except for Beijing, the capital of China. Gansu, Inner Mongolia, Shaanxi, Jilin, Qinghai, and Heilongjiang were the six regions with the least penetration rate of RHST in China, which are located in North China, Northwest China, and Northeast China. The imbalance in the penetration rate of RHST between regions in China was still significant. Notably, the Chinese government has launched a series of special actions for rural toilet retrofitting since 2018, and it has formulated a number of policies, such as the Three-Year Action Plan for Rural Living Environment Improvement and the Guiding Opinions on Promoting the Special Action of Rural “Toilet Retrofitting,” which offers new opportunities to improve the imbalance in the penetration rate of RHST among China’s regions (Central People’s Government of the People’s Republic of China, 2018; Ministry of Agriculture and Rural Affairs of the People’s Republic of China, 2019). However, each region needs to improve its SED according to the actual situation to improve the penetration rate of RHST and achieve sustainable sanitation.
FIGURE 4. Process of change in the penetration rate of rural harmless sanitary toilets (RHST) in 30 regions of China from 2007 to 2017.
A summary of the association rules between the six selected SED indicators and the penetration rate of RHST is shown in Supplementary Table S3A. Table 2 summarizes the association rules between the six selected SED indicators and the high penetration rate of RHST. Given that the population urbanization rate ranged from 60.30 to 69.85% and from 76.31 to 89.60%, the tertiary vs. secondary industry output ratio ranged between 1.09 and 1.59, the nonagricultural output value ratio ranged from 93.09 to 96.26% and from 97.90 to 99.64%, nonagricultural employment ratio ranged from 68.28 to 82.30% and from 83.20 to 96.91%, per capita GDP ranged between 54,838 and 128,994, and the proportion of added value of industry in the total added value of commodity ranged between 77.21 and 89.58; RHST was of high penetration rate. A set of reasonable ranges of the population urbanization rate, the tertiary vs. secondary industry output ratio, nonagricultural output value ratio, the nonagricultural employment ratio, per capita GDP, and the proportion of added value of industry in the total added value of commodity can be found in Table 2 for high RHST penetration degree.
Table 3 summarizes the association rules between SED indicators and the penetration rate of RHST. We found that a low level of RHST penetration rate was significantly correlated with the population urbanization rate, ranging from 28.24 to 38.16% and from 33.5 to 45.2%. The low penetration rate of RHST with low population urbanization rate may be caused by inadequate rural infrastructure. The low penetration rate of RHST also existed in areas where the tertiary vs. secondary industry output ratio ranged between 0.53 and 0.80%, the nonagricultural output value ratio ranged between 79.35 and 83.71%, between 83.95 and 86.83%, between 86.99 and 89.71%, and between 89.75 and 92.89%, the nonagricultural employment ratio ranged between 25.88 and 47.05%, between 47.36 and 58.05%, and between 58.20 and 68.12%, per capita GDP ranged between 7,878 and 29,963, and the proportion of added value of industry in the total added value of commodity ranged between 47.43 and 65.22% and between 65.78 and 76.97%. Therefore, the association rule analysis showed that the low value of the six selected indicators of SED determined the low level of penetration rate of RHST.
We identified SED indicators related to the penetration rate of RHST by region in China in 2017 according to Supplementary Table S4A, as shown in Figure 5, to propose differentiated development strategies to increase the penetration rate of RHST.
For Beijing, Tianjin, Shanghai, Jiangsu, Zhejiang, Fujian, and Guangdong, the six SED indicator values all corresponded to high RHST penetration rates (R5), and these regions can maintain their current rate of SED to increase per capita GDP. With income increases, local rural residents have more ability to improve household sanitation. Local governments will also have more financial resources for the construction, operation, and maintenance of sanitation facilities.
We found that the SED indicators of Guangxi and Hainan were at the lower rank, except for Hainan where the tertiary vs. secondary industry output ratio was in the T4 range, but they corresponded to a high RHST penetration rate in 2017 (at the R5 rank) given that they belong to the coastal region. Guangxi and Hainan should further increase the proportion of added value of industry in the total added value of commodity, such as investing in high-tech industry technologies, because they are in the lower rank of I2 and I1, respectively. The tertiary industry needs to be optimized to create more jobs and raise the resident’s per capita GDP. Local governments not only need to improve the wastewater treatment facilities in each rural area but also need to form a long-term management and maintenance mechanism due to the abundant water resources, to reduce the pollution of water sources by toilet wastewater.
Among the six socioeconomic indicators studied, the E4, T2, and G2 indicators in Jiangxi, V6, P5, T2, and E4 indicators in Shandong, V6, E4, and P5 indicators in Chongqing, and G2 indicator in Sichuan were associated with the penetration rate of RHST at the R4 level. However, the abovementioned SED indicators associated with the penetration rate of RHST were all at moderate levels in the aforementioned regions, which means that more investments could be made in the tertiary industry and further attract more manufacturers to increase the proportion of added value of industry in the total added value of commodity for enhancing the household wealth of the residents in these regions. The population urbanization rates of Jiangxi and Sichuan were P4 and P3, respectively, which were at middle level. In the process of SED, on one hand, improving household sanitation facilities needs to rely on the efforts of rural residents, and on the other hand, the proportion of financial support from local governments needs to be increased to help more rural residents with financial difficulties, thereby increasing the penetration rate of local RHST. Liu et al. studied the rural toilet retrofitting model in Jiaozhou (located in Shandong Province, China) and found that local rural residents have achieved full access to RHST and have also constructed a complete sustainable sanitation service chain covering collection, transportation, treatments, disposal, and resource recovery of fecal sludge relying on financial support from the provincial and municipal governments (Liu et al., 2019). However, the constructed sustainable sanitation service chain in rural areas requires a large amount of operating capital, part of which needs to be paid by rural residents, such as the cost of manure pumping by septic tankers and the maintenance of sanitation facilities, which is difficult for poor rural households (Roubík et al., 2020). Therefore, preferential policies and funding to help serve families with financial difficulties are also a guarantee of sustainable sanitation (Kaiser, 2015).
Regions in Hebei, Shanxi, Inner Mongolia, Liaoning, Jilin, Heilongjiang, Anhui, Henan, Hunan, Guizhou, Yunnan, Ningxia, Xinjiang, Shaanxi, Gansu, and Qinghai did not have SED indicators associated with high penetration rate of RHST. Therefore, these regions should increase investment in industry to enhance SED. In Guizhou and Yunnan, the tertiary vs. secondary industry ratio (at T3 level) was better than other SED indicators, but the proportion of added value of industry in the total added value of commodity was at I2 level and needs to be further strengthened. Sun et al. also evaluated the SED of Guizhou province finding that regions with proportion of secondary industry have a prosperous economy (Sun et al., 2020). In Heilongjiang and Qinghai, the RHST penetration rate was at R1 level, which was mined by the association analysis to be related to the low nonagricultural employment ratio, per capita GDP, and the proportion of added value of industry in the total added value of commodity. Therefore, the local government should increase investment and attract more domestic and international manufacturers with preferential policies to improve the level of SED indicators. Guo et al. surveyed villages in western China (Gansu and Qinghai) and found that poor sanitation awareness and attitudes impede the progress of the rural toilet revolution (Guo et al., 2021). We hypothesized that sanitation services would be enhanced if the level of SED in the western region also approached that of the coastal region. However, achieving more than 60% RHST penetration rate in a short period based on the current rate of SED alone is difficult given that huge challenges exist in the SED process. Therefore, the national central government should allocate a greater proportion of central financial resources for rural toilet retrofitting in these areas during the rural “toilet revolution” process. Overall, the identification of regional SED indicators related to the penetration rate of RHST can help develop targeted regional SED plans to achieve the penetration of RHST in China.
Variability exists in rural infrastructure investments in different regions of China (Li et al., 2020). Nevertheless, the implementation of the policy successfully promotes SED. However, inadequate financial resources still constrain rapid SED in some rural areas, such as those with low rural infrastructure penetration rates (Zhang et al., 2020). Fortunately, to achieve universal access to sanitation with “safe management services,” which is defined as “access to a sanitation facility that is not shared with other households and where excreta produced can be safely disposed of on-site, or transported and disposed of off-site” (WHO and UNICEF, 2017), China’s central government gives provincial governments the freedom to decide on strategies and methods for rural toilet retrofitting policies to achieve the desired outcomes. Hueso and Bell noted that policies should be people-centered, demand-, incentive-, and practice-oriented, which is ideal for addressing the rural sanitation crisis (Hueso and Bell, 2013). However, the researchers found that availability of policy information, the percentage of subsidies, and the difficulty of obtaining subsidies affected the motivation of rural residents to participate in rural toilet retrofitting, especially poor rural households (Kaiser, 2015; Roubík et al., 2020).
Chinese provinces should clarify short-term development goals and long-term development goals when formulating policies. On the one hand, for economically developed provinces with high penetration of RHST, the supporting facilities to have access to safe transportation, treatment, and disposal of toilet wastes are imperfect. In addition to focusing on SED, the local government needs to formulate more policies to serve the improvement and operation of supporting facilities for toilet waste treatment. On the other hand, for backward economic developing provinces with low penetration of RHST, local governments should focus on the continuity convergence of policies. In a short period of time, the penetration rate of RHST should be improved by adhering to the short-term promotion strategy of “quantity follows quality and progress follows effectiveness.” When the penetration rate of RHST is raised, the policy should focus on the improvement of supporting facilities for toilet waste treatment.
We studied the association pattern between SED indicators and the penetration rate of RHST by regions in China from 2007 to 2017. Overall, the selected six categories of SED indicators of Chinese regions have been increasing in population urbanization rate, tertiary vs. secondary industry output ratio, nonagricultural output value ratio, nonagricultural employment ratio, and per capita GDP. The proportion of added value of industry in the total added value of commodity showed three trend changes, which were 1) a positive trend change, 2) a stable fixed interval with a ±2 ratio difference, and 3) a negative trend change. The proportion of added value of industry in the total added value of commodity accounted for the highest proportion of the level of SED. The penetration rate of RHST continuously increased in all regions of China from 2007 to 2017. The results of the association rule emphasize that reasonable SED indicators are essential for high RHST penetration rate. Differentiated development strategies were proposed to optimize SED indicators in different regions for improving RHST penetration rate.
The original contributions presented in the study are included in the article/Supplementary Material, further inquiries can be directed to the corresponding authors.
YL contributed to the writing of the original draft and visualization of the study. SC contributed to the conceptualization, methodology, writing, reviewing and editing of the manuscript, and supervision of the study. JC contributed to the data curation of the study. MG contributed to the data curation and software of the study. ZL contributed to the methodology, writing, reviewing, and editing of the manuscript. LW, CC, and DB contributed to the methodology of the study. TL contributed to the funding acquisition and supervision of the study. All authors approved the submitted version.
This study was supported by the National Key Research and Development Plan (2018YFC1903206), the Interdisciplinary Research Project for Young Teachers of USTB (Fundamental Research Funds for the Central Universities No. FRF-IDRY-20-012), and the Youth Teacher International Exchange and Growth Program of USTB (QNXM20210029).
The authors declare that the research was conducted in the absence of any commercial or financial relationships that could be construed as a potential conflict of interest.
All claims expressed in this article are solely those of the authors and do not necessarily represent those of their affiliated organizations, or those of the publisher, the editors, and the reviewers. Any product that may be evaluated in this article, or claim that may be made by its manufacturer, is not guaranteed nor endorsed by the publisher.
The authors would like to take this opportunity to express our sincere appreciation for the support of the National Environmental and Energy Science and Technology International Cooperation base.
The Supplementary Material for this article can be found online at: https://www.frontiersin.org/articles/10.3389/fenvs.2022.817655/full#supplementary-material
Agarwal, R. C., Aggarwal, C. C., and Prasad, V. V. V. (2000). “Depth First Generation of Long Patterns,” in Proceedings of the sixth ACM SIGKDD international conference on Knowledge discovery and data mining, Boston, MA, August 2000 (Association for Computing Machinery), 108–118. doi:10.1145/347090.347114
Agarwal, S. (2013). “Data Mining: Data Mining Concepts and Techniques,” in 2013 International Conference on Machine Intelligence and Research Advancement, Katra, December 21–23, 2013, 203–207. doi:10.1109/ICMIRA.2013.45
Aggarwal, C. C. (2014). “An Introduction to Frequent Pattern Mining,” in Frequent Pattern Mining (Cham: Springer), 1–17. doi:10.1007/978-3-319-07821-2_1
Aggarwal, C. C., Bhuiyan, M. A., and Hasan, M. A. (2014). “Frequent Pattern Mining Algorithms: A Survey,” in Frequent Pattern Mining (Cham: Springer), 19–64. doi:10.1007/978-3-319-07821-2_2
Agrawal, R., Imieliński, T., and Swami, A. (1993). “Mining Association Rules between Sets of Items in Large Databases,” in Proceedings of the 1993 ACM SIGMOD international conference on Management of data, Washington, DC, June 1, 1993 (Association for Computing Machinery) 22, 207–216. SIGMOD Rec. doi:10.1145/170036.170072
Ahmed, S. A., and Nath, B. (2021). Identification of Adverse Disease Agents and Risk Analysis Using Frequent Pattern Mining. Inf. Sci. 576, 609–641. doi:10.1016/j.ins.2021.07.061
Assis, B. d. S. d., Ogasawara, E., Barbastefano, R., and Carvalho, D. (2021). Frequent Pattern Mining Augmented by Social Network Parameters for Measuring Graduation and Dropout Time Factors: A Case Study on a Production Engineering Course. Socio-Econ. Plan. Sci., 101200. doi:10.1016/j.seps.2021.101200
Çakır, E., Fışkın, R., and Sevgili, C. (2021). Investigation of Tugboat Accidents Severity: An Application of Association Rule Mining Algorithms. Reliability Eng. Syst. Saf. 209, 107470. doi:10.1016/j.ress.2021.107470
Central People's Government of the People's Republic of China (2018). Three-Year Action Plan for Rural Living Environment Improvement. Available at: http://www.gov.cn/zhengce/2018-02/05/content_5264056.htm (Accessed November 5, 2021).
Chandana, N., and Rao, B. (2022). A Critical Review on Sludge Management from Onsite Sanitation Systems: A Knowledge to Be Revised in the Current Situation. Environ. Res. 203, 111812. doi:10.1016/j.envres.2021.111812
Chen, J., Huang, Q., and Zhong, H. (2006). The Synthetic Evaluation and Analysis on Regional Industrialization. Econ. Res. J. 6, 4–15. [in Chinese, with English summary].
Cheng, S., Li, Z., Uddin, S. M. N., Mang, H.-P., Zhou, X., Zhang, J., et al. (2018). Toilet Revolution in China. J. Environ. Manage. 216, 347–356. doi:10.1016/j.jenvman.2017.09.043
China National Health Commission (2018). China Health Statistics Yearbook. Beijing: China Peking Union Medical University Press, 278. (in Chinese).
Deshpande, A., Miller-Petrie, M. K., Lindstedt, P. A., Baumann, M. M., and Johnson, K. B. (2020). Mapping Geographical Inequalities in Access to Drinking Water and Sanitation Facilities in Low-Income and Middle-Income Countries, 2000-17. Lancet Glob. Health 8, e1162–e1185. doi:10.1016/S2214-109X(20)30278-3
Gasana, J., Morin, J., Ndikuyeze, A., and Kamoso, P. (2002). Impact of Water Supply and Sanitation on Diarrheal Morbidity Among Young Children in the Socioeconomic and Cultural Context of Rwanda (Africa). Environ. Res. 90, 76–88. doi:10.1006/enrs.2002.4394
Ghosh, A., and Cairncross, S. (2013). The Uneven Progress of Sanitation in India. J. Water Sanitation Hyg. Dev. 4, 15–22. doi:10.2166/washdev.2013.185
Guo, S., Zhou, X., Simha, P., Mercado, L. F. P., Lv, Y., and Li, Z. (2021). Poor Awareness and Attitudes to Sanitation Servicing Can Impede China's Rural Toilet Revolution: Evidence from Western China. Sci. Total Environ. 794, 148660. doi:10.1016/j.scitotenv.2021.148660
Han, J., Pei, J., Yin, Y., and Mao, R. (2004). Mining Frequent Patterns without Candidate Generation: A Frequent-Pattern Tree Approach. Data Mining Knowledge Discov. 8, 53–87. doi:10.1023/b:dami.0000005258.31418.83
Heng, J., Wang, J., Xiao, L., and Lu, H. (2017). Research and Application of a Combined Model Based on Frequent Pattern Growth Algorithm and Multi-Objective Optimization for Solar Radiation Forecasting. Appl. Energ. 208, 845–866. doi:10.1016/j.apenergy.2017.09.063
Hueso, A., and Bell, B. (2013). An Untold story of Policy Failure: the Total Sanitation Campaign in India. Water Policy 15, 1001–1017. doi:10.2166/wp.2013.032
Kaus, W. (2013). Beyond Engel's Law - A Cross-Country Analysis. The J. Socio-Economics 47, 118–134. doi:10.1016/j.socec.2013.10.001
Li, T., Li, Y., An, D., Han, Y., Xu, S., Lu, Z., et al. (2019). Mining of the Association Rules between Industrialization Level and Air Quality to Inform High-Quality Development in China. J. Environ. Manage. 246, 564–574. doi:10.1016/j.jenvman.2019.06.022
Li, Y., Cheng, S., Li, Z., Song, H., Guo, M., Li, Z., et al. (2020). Using System Dynamics to Assess the Complexity of Rural Toilet Retrofitting: Case Study in Eastern China. J. Environ. Manage. 111655, 1–10. doi:10.1016/j.jenvman.2020.111655
Liu, J., Shi, D., Li, G., Xie, Y., Li, K., Liu, B., et al. (2020). Data-driven and Association Rule Mining-Based Fault Diagnosis and Action Mechanism Analysis for Building Chillers. Energy and Buildings 216, 109957. doi:10.1016/j.enbuild.2020.109957
Liu, Z., Xu, Y., Liu, B., Liu, Z., and Zang, J. (2019). Study on Jiaozhou Model of Toilet Renovation in Rural China. Beijing: China Society Press. (in Chinese).
Ministry of Agriculture and Rural Affairs of the People's Republic of China (2019). Guiding Opinions on Promoting the Special Action of Rural “Toilet Retrofitting”. Available at: http://www.moa.gov.cn/gk/tzgg_1/tz/201901/t20190108_6166292.htm (Accessed November 5, 2021).
Ministry of Health of the People's Republic of China (2008). China Health Statistics Yearbook. Beijing: Peking Union Medical College Press of China, 251. (in Chinese).
National Bureau of Statistics of China (2018). China Statistical Yearbook. Beijing: China Statistics Press. (in Chinese).
National Health and Family Planning Commission of the People's Republic of China (2013). China Health and Family Planning Statistical Yearbook. Beijing: Peking Union Medical College Press of China. (in Chinese).
Novotný, J., Ficek, F., Hill, J. K. W., and Kumar, A. (2018a). Social Determinants of Environmental Health: A Case of Sanitation in Rural Jharkhand. Sci. Total Environ. 643, 762–774. doi:10.1016/j.scitotenv.2018.06.239
Novotný, J., Humňalová, H., and Kolomazníková, J. (2018b). The Social and Political Construction of Latrines in Rural Ethiopia. J. Rural Stud. 63, 157–167. doi:10.1016/j.jrurstud.2018.08.003
Roubík, H., Mazancova, J., Rydval, J., and Kvasnicka, R. (2020). Uncovering the Dynamic Complexity of the Development of Small–Scale Biogas Technology through Causal Loops. Renew. Energ. 149, 235–243. doi:10.1016/j.renene.2019.12.019
Shabtay, L., Fournier-Viger, P., Yaari, R., and Dattner, I. (2021). A Guided FP-Growth Algorithm for Mining Multitude-Targeted Item-Sets and Class Association Rules in Imbalanced Data. Inf. Sci. 553, 353–375. doi:10.1016/j.ins.2020.10.020
Sun, M., Li, X., Yang, R., Zhang, Y., Zhang, L., Song, Z., et al. (2020). Comprehensive Partitions and Different Strategies Based on Ecological Security and Economic Development in Guizhou Province, China. J. Clean. Prod. 274, 122794. doi:10.1016/j.jclepro.2020.122794
Tian, Y., and Wang, L. (2019). Mutualism of Intra- and Inter-prefecture Level Cities and its Effects on Regional Socio-Economic Development: A Case Study of Hubei Province, Central China. Sust. Cities Soc. 44, 16–26. doi:10.1016/j.scs.2018.09.033
Tilley, E., Strande, L., Lüthi, C., Mosler, H.-J., Udert, K. M., Gebauer, H., et al. (2014). Looking beyond Technology: An Integrated Approach to Water, Sanitation and Hygiene in Low Income Countries. Environ. Sci. Technol. 48, 9965–9970. doi:10.1021/es501645d
Trimmer, J. T., Lohman, H. A. C., Byrne, D. M., Houser, S. A., Jjuuko, F., Katende, D., et al. (2020). Navigating Multidimensional Social-Ecological System Trade-Offs across Sanitation Alternatives in an Urban Informal Settlement. Environ. Sci. Technol. 54, 12641–12653. doi:10.1021/acs.est.0c03296
United Nations (2017). World Population Prospects (2017). The 2017 Revision. New York: United Nations Department of Economic and Social Affairs, Population Division.
Wei, W., Guo, Z., Xie, B., Zhou, J., and Li, C. (2020). Quantitative Simulation of Socio-Economic Effects in mainland China from 1980 to 2015: A Perspective of Environmental Interference. J. Clean. Prod. 253, 119939. doi:10.1016/j.jclepro.2019.119939
WHO (2020a). Hygiene: UN-water GLAAS Findings on National Policies, Plans, Targets and Finance. Geneva: World Health Organization.
WHO (2020b). World Health Statistics 2020: Monitoring Health for the Sdgs, Sustainable Development Goals. Geneva: World Health Organization.
WHO, UNICEF (2017). Progress on Drinking Water, Sanitation and hygiene: 2017 Update and Sustainable Development Goal Baselines. Geneva: World Health Organization (WHO) and the United Nations Children’s Fund UNICEF.
Keywords: socioeconomic development, rural harmless sanitary toilet, association rule, differentiated development, rural toilet retrofitting
Citation: Li Y, Cheng S, Cui J, Gao M, Li Z, Wang L, Chen C, Basandorj D and Li T (2022) Mining of the Association Rules Between Socio-Economic Development Indicators and Rural Harmless Sanitary Toilet Penetration Rate to Inform Sanitation Improvement in China. Front. Environ. Sci. 10:817655. doi: 10.3389/fenvs.2022.817655
Received: 18 November 2021; Accepted: 24 January 2022;
Published: 14 April 2022.
Edited by:
Ahmed El Nemr, National Institute of Oceanography and Fisheries (NIOF), EgyptReviewed by:
Aldona Migała-Warchoł, Rzeszów University of Technology, PolandCopyright © 2022 Li, Cheng, Cui, Gao, Li, Wang, Chen, Basandorj and Li. This is an open-access article distributed under the terms of the Creative Commons Attribution License (CC BY). The use, distribution or reproduction in other forums is permitted, provided the original author(s) and the copyright owner(s) are credited and that the original publication in this journal is cited, in accordance with accepted academic practice. No use, distribution or reproduction is permitted which does not comply with these terms.
*Correspondence: Shikun Cheng, Y2hlbmdzaGlrdW5AdXN0Yi5lZHUuY24=; Tianxin Li, dGlhbnhpbmxpQHVzdGIuZWR1LmNu
Disclaimer: All claims expressed in this article are solely those of the authors and do not necessarily represent those of their affiliated organizations, or those of the publisher, the editors and the reviewers. Any product that may be evaluated in this article or claim that may be made by its manufacturer is not guaranteed or endorsed by the publisher.
Research integrity at Frontiers
Learn more about the work of our research integrity team to safeguard the quality of each article we publish.